Thank you for visiting nature.com. You are using a browser version with limited support for CSS. To obtain the best experience, we recommend you use a more up to date browser (or turn off compatibility mode in Internet Explorer). In the meantime, to ensure continued support, we are displaying the site without styles and JavaScript.
- View all journals
- Explore content
- About the journal
- Publish with us
- Sign up for alerts
- Open access
- Published: 26 December 2022

Systematic analysis of healthcare big data analytics for efficient care and disease diagnosing
- Sulaiman Khan 1 ,
- Habib Ullah Khan 1 &
- Shah Nazir 2
Scientific Reports volume 12 , Article number: 22377 ( 2022 ) Cite this article
7608 Accesses
14 Citations
7 Altmetric
Metrics details
- Biotechnology
- Computational biology and bioinformatics
Big data has revolutionized the world by providing tremendous opportunities for a variety of applications. It contains a gigantic amount of data, especially a plethora of data types that has been significantly useful in diverse research domains. In healthcare domain, the researchers use computational devices to extract enriched relevant information from this data and develop smart applications to solve real-life problems in a timely fashion. Electronic health (eHealth) and mobile health (mHealth) facilities alongwith the availability of new computational models have enabled the doctors and researchers to extract relevant information and visualize the healthcare big data in a new spectrum. Digital transformation of healthcare systems by using of information system, medical technology, handheld and smart wearable devices has posed many challenges to researchers and caretakers in the form of storage, minimizing treatment cost, and processing time (to extract enriched information, and minimize error rates to make optimum decisions). In this research work, the existing literature is analysed and assessed, to identify gaps that result in affecting the overall performance of the available healthcare applications. Also, it aims to suggest enhanced solutions to address these gaps. In this comprehensive systematic research work, the existing literature reported during 2011 to 2021, is thoroughly analysed for identifying the efforts made to facilitate the doctors and practitioners for diagnosing diseases using healthcare big data analytics. A set of rresearch questions are formulated to analyse the relevant articles for identifying the key features and optimum management solutions, and laterally use these analyses to achieve effective outcomes. The results of this systematic mapping conclude that despite of hard efforts made in the domains of healthcare big data analytics, the newer hybrid machine learning based systems and cloud computing-based models should be adapted to reduce treatment cost, simulation time and achieve improved quality of care. This systematic mapping will also result in enhancing the capabilities of doctors, practitioners, researchers, and policymakers to use this study as evidence for future research.
Similar content being viewed by others
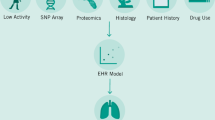
Big data in digital healthcare: lessons learnt and recommendations for general practice
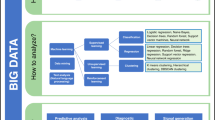
Data harnessing to nurture the human mind for a tailored approach to the child
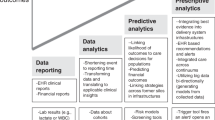
Utilizing big data from electronic health records in pediatric clinical care
Introduction.
Healthcare around the world is under high pressure due to limiting financial resources, over-population, and disease burden. In this modern technological age the healthcare paradigm is shifting from traditional, one-size-fits-all approach to a focus on personalized individual care 1 . Additionally, the healthcare data is varying both in type and amount. The healthcare providers are not only dealing with patient’s historical, physical and namely information, but they also deal with imaging information, labs, and other digital and analogue information consists of ECG, MRI etc. This data is voluminous, varying in type and formats, and of differing structure. These are the capabilities of Big Data to handle not only different types of and forms of data, but can handle 10 V structure including volume, variety, venue, varifocal, varmint, vocabulary, validity, volatility, veracity and velocity. Thus, the doctors facing an increasing burden of rising patient numbers coupled with progressively less time to spend with each patient. In other words, we are facing more patients, more data, and less time.
Big data has significantly attracted the researchers to explore different research fields including healthcare, banking, imaging, smart cities, internet of things (IoT) based smart applications, tracking and transportation system etc. 2 . Software engineers constantly develops new applications for patient’s health and well-being. Both government and non-government organizations develop infrastructure using big data analytics for improved decision making capabilities of both doctors and managers 3 . It was recorded that 80% increase in big data is due to cloud sources, big data analytics, mobile technology and social media technologies 4 . A number of research articles proposed using big data analytics in varying domains especially in healthcare such as Kumar et al. 5 proposed a cognitive technology-based healthcare evaluations system using big data analytics. Chen et al. 6 presented an intelligent healthcare application for brain hemorrhage detection using Big Data analytics and machine learning (ML) techniques. Smart health appointment system is developed by Liang and Zhao using big data analytics is 7 .
Some researchers explored big data analytics in healthcare domain in different ways. They presented survey papers and review papers to understand the meanings of big data analytics in healthcare such as Galetsi and Kasaliasi performed a review of healthcare big data analytics 8 while Lindell defined big data analytics in terms of accounting and business perspectives 9 . Alharthi proposed a review article on healthcare challenges facing in Saudi Arabia by performing analysis of the available literature 10 . Lee et al. 11 presented a survey paper to explore the applications and challenges of healthcare big data analytics. From the literature it is concluded that multiple new applications are developed for big data analysis. Review and survey papers are presented to outline the published literature, but most of these papers are region specific or limited to a few numbers of papers. On the other side systematic review process formulate multiple research question and identifies keywords to explore the available literature from different angles. Systematic analysis of the available literature is presented in many fields like PMIPv6 domain 12 , in smart homes 13 , navigation assistants 14 , and many others, but there is no significant work reported on systematic analysis for healthcare big data domain to find the gaps in the available literature and suggest future research directions.
The inspirational point that led us to pursue this systematic analysis was the pervasive and ubiquitous nature of big data. Efficient management and timely execution are the dire needs of big data, to extract enriched information regarding a certain problem of interest 15 . Many factors involved behind this systematic research work, but the most eminent reasons are:
The exiting research reported on big data does not provide significant information about the key features that should be considered to integrate both structured and unstructured big data in healthcare domain. The pervasiveness of big data features challenging the researchers in pursuing research in this specialized domain. The underlying research on finding the key features will not only help in integrating big data in healthcare domain, but it will also assist in findings new gateways for future research directions.
Digital transformation of healthcare systems after the integration of information system, medical technology and other imaging systems have posed a big barrier for the research community in the form of a vast amount of information to deal with. While the over-population, limited data access, and disease burdens have restricted the doctors and practitioners to check more patients in a limited time. So, finding a suitable model that can efficiently process healthcare big data to extract information for a certain disease symptoms will not only helps the practitioners to suggest accurate medication and check more patients in timely manners, but it will open future research directions for the industrialists and policymakers to develop optimal healthcare big data processing models.
Accurate disease diagnosing by processing of gigantic amount of data, especially a plethora of types of data, within an interested processing domain is a key concern for both researchers and practitioners. Developing an efficient model that can accurately diagnose a certain by classifying images or other historical details of patients will not only helps the doctors to diagnose disease in timely manner and suggest medicine accordingly, but it will encourage the researchers and developers to develop an accurate disease identification model.
The remaining research paper of the paper is organized as follows. Section 2 of the paper outlines the related work reported in the proposed field. Section 3 presents the research framework followed for this systematic research work. Quality assessment is detailed in Sect. 4 . Section 5 outlines the discussion on findings of the proposed systematic research work. Section 6 provides the limitations of this systematic study traced by the conclusion and future work in Sect. 7 of the paper.
Literature review
From the last few decades, we experienced an unprecedented transformation of traditional healthcare systems to digital and portable healthcare applications with the help of information systems, medical technology and other imaging resources 16 . Big data are radically changing the healthcare system by encouraging the healthcare organizations to embrace extraction of relevant information from imaginary data and other clinical records. This information will produce high throughput in terms of accurate disease diagnosing, plummeting treatment cost increase availability. In data visualization context the term ‘big data’, is firstly introduced in 1997 17 , posed an ambitious and exceptional challenge for both policy-makers and doctors with special emphasis on personalized medicine. Nonetheless, data gathering moves faster than both data analysis and data processing, emphasizing the widening gap between the rapid technological progress in data acquisition and the comparatively slow functional characterization of healthcare information. In this regard, the historical information (phonotypical and other genomic information) of an individual patient form electronic health records (EHR) are becoming of critical importance. Figure 1 represents the primary sources of big data.
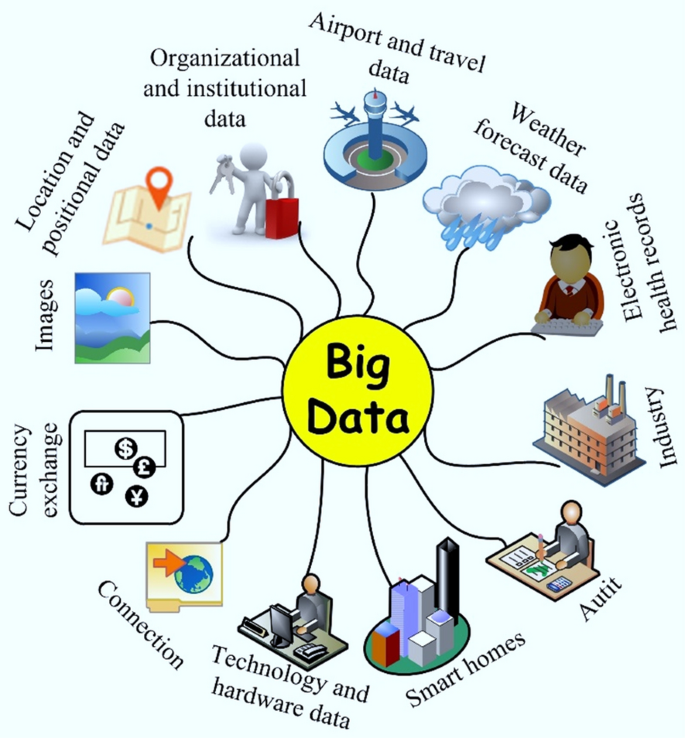
Main steps of the research protocol.
Significant research work has been reported in the domains of healthcare big data analytics. To process this vast amount of information in timely manner and identify someone’s health condition based on his her is more difficult. Researchers proposed numerous applications to address this problem such as; Syed et al. 18 proposed a machine learning-based healthcare system for providing remote healthcare services to both diseased and healthy population using big data analytics and IoT devices. Venkatesh et al. 19 developed heart disease prediction model using big data analytics and Naïve Bayes classification technique. Kaur et al. 20 suggested a machine learning (ML) based healthcare application for disease diagnosing and data privacy restrictions. This model works by considering different aspects like activity monitoring, granular access control and mask encryption. Some researchers presented review and survey papers to outline the recent published work in a specific directions such as Patel and Gandhi reviewed the literature for identifying the machine learning approaches proposed for healthcare big data analytics 21 . Rumbold et al. 22 reviewed the literature for find the research work reported for diabetic diagnosing using big data analytics.
From the above discussions, it is worth mentioning that most of the researchers and industrialists gave significant attention towards the development of new computational models or surveyed the literature in a specific research direction (heart disease detection, diabetes detection, storage and security analysis etc.), but no significant research work is reported to systematically analyze the literature with different perspectives. To address this problem, this research work presents a systematic literature review (SLR) work to analyze the literature reported in healthcare big data analytics domain. This systematic analysis will not only find the gaps in the available literature but it will also suggest new directions of future research to explore.
Research framework
Systematic literature reviews and meta-analysis has gained significant attention and became increasingly important in healthcare domain. Clinicians, developers and researchers follow SLR studies to get updated about new knowledge reported in their fields 23 , 24 , and they are often followed as a starting point for preparing basic records. Granting agencies mostly requires SLR studies to ensure justification of further research 25 , and even some healthcare journals follows this direction 26 . Keeping these SLR applications in mind the proposed systematic analysis is performed following the guidelines presented by Moher et al. 27 (PRISMA) and Kitchenham et al. 28 . This SLR work accumulates the most relevant research work from primary sources. These papers are then evaluated and analyzed to grab the best results for the selected research problem. Figure 2 represents the results after following the PRISMA guidelines. This systematic analysis are performed using the following preliminary steps:
Identification of research questions to systematically analyze the proposed field from different perspectives.
Selection of relevant keywords and queries to download the most relevant research articles.
Selection of peer-reviewed online databases to download relevant research articles published in healthcare big data domain during the period ranging from 2011 – 2021.
Perform inclusion and exclusion process based on title, abstract and the contents presented in the article to remove duplicate records.
Assess the finalized relevant articles for identifying gaps in the available literature and suggest new research directions to explore.
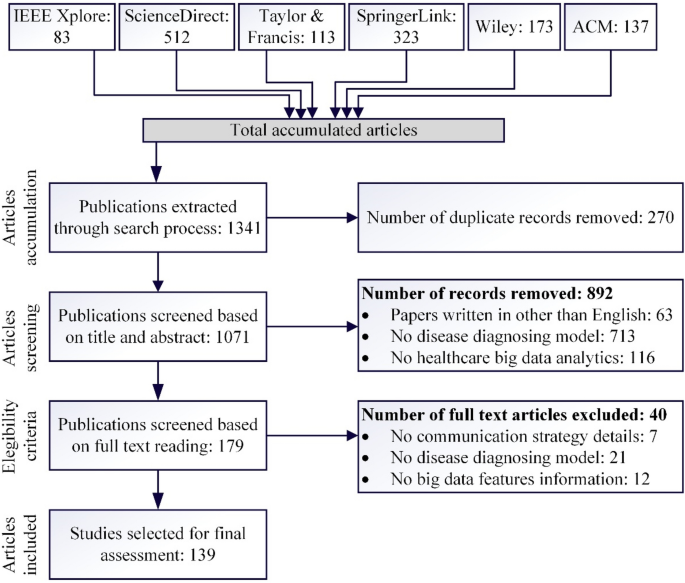
PRISMA process model for articles accumulation, screening, and final selection.
Research questions
Selecting a well-constructed research question(s) is essential for a successful review process. We formulate a set of five research questions based on the Goal Questions Metrics approach proposed by Van Solingen et al. 29 . The formulated research questions are depicted in Table 1 below.
Search strategy
Search strategy is the key step in any systematic research work because this is the step that ensures the most relevant article for the analysis and the assessment process. To define a well-organized search strategy a search string is developed using the formulated relevant keywords. For the accumulation of most relevant articles for a certain research problem, only keywords are not sufficient. These keywords are concatenated in different strings for searching articles in multiple online repositories 30 . Inspired from the SLR work of Achimugu et al. 31 , in software requirement domain, our search strategy consists of four main steps includes identification of keywords relevant to selected research problem, formulation of search string based on the keywords, and selection of online repositories to accumulate relevant articles to the problem selected.
Selection of keywords
List of keywords are defined for each research question to download all relevant articles. Some researchers defined a generic query 32 and starts downloading articles. Although it is simple for the accumulation of articles from online database but mostly it tends to skip some most relevant articles. So, the correct option is to define keywords for each research question. In fact, it is a hectic job, but it ensures the retrieval of each relevant article from online databases regarding a certain research problem.
Formulation of search string
Search strings (queries) are formulated using the keywords identified from the selected research questions. The search string is tested in online databases and was modified according to retrieve each relevant articles from these databases. Inspired from the guidelines proposed by Wohlin 33 , following are the key steps undertaken to develop an optimal search string:
Identification of key terms from the formulated topic and research questions
Selection of alternate words or synonyms for key terms
Use “OR” operator for alternating words or synonyms during query formation
Link all major terms with Boolean “AND” operator to validate every single keyword.
Following all these preliminary steps a generic query/search-string is developed that is depicted in Table 2 . This generic query is further refined for each research question as depicted in Table 3 to retrieve each relevant article.
Selection of online repositories
After identifying keywords and formulating search strings the next step is to download relevant articles specific to the interested research problem. For the accumulation of relevant articles six well-known and peer-reviewed online repositories are selected, as depicted in Table 3 .
Articles accumulation and final database development
For relevant articles accumulation and final database development we followed the guidelines suggested by Kable et al. 34 . After specifying the research questions, identifying keywords, and formulating search queries, and selecting online repositories, the next key step is to develop a relevant articles database for the analysis and assessment purposes that includes three prime steps: (1) identification of inclusion/exclusion criteria for a certain research article(s), and (2) Relevant articles database development. These steps are discussed in detail below.
Inclusion and exclusion criteria
After selecting online database and starts the articles downloading process, the most tedious task that the author (s) facing, is the decision about whether a certain paper should be included in the final database or not? To overcome this problem an inclusion and exclusion criteria is defined for the inclusion of a certain article in the final set of articles. Table 4 represents the inclusion and exclusion criteria followed for this systematic research work.
A manual process is followed by the authors for the inclusion and exclusion of a certain article. These articles are evaluated based on title, abstract and information provided in the overall paper. If more than half authors agree upon the inclusion of a certain article based on these parameters (title, abstract, and contents presented in the article), then that paper was counted in the final database otherwise rejected. A total of 134 relevant primary studies are selected for the final assessment process. To ensure no skip of relevant article snowballing is applied to retrieve each relevant article.
Snowballing To extract each relevant primary article snowballing is applied in the proposed research work 33 . In this systematic analysis both types of snowballing backward and forward snowballing is applied to ensure extraction of each relevant primary article. 145 relevant articles retrieved after applying snowballing process. These articles are then filtered by title and resulted for 53 relevant articles. After further processing by abstract resulted into 19 articles, and at last when filtered by contents presented in the paper resulted into only 5 relevant articles. This overall process is depicted in Fig. 3 . After adding these articles to the accumulated relevant articles, a total of 139 articles added to the final database.
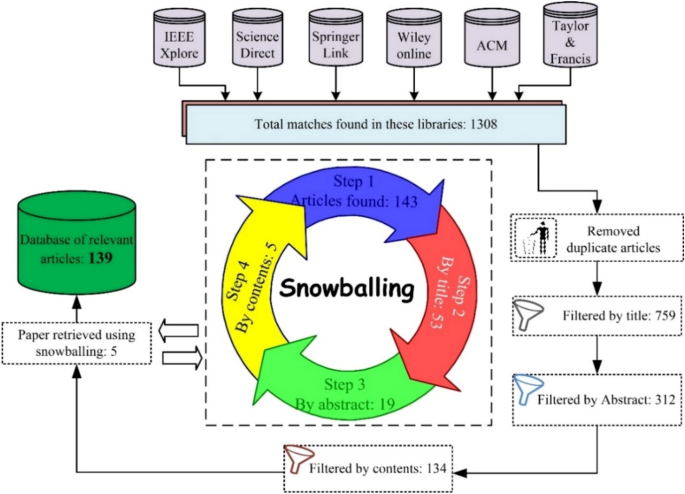
Extraction of each relevant article using snowballing.
Relevant articles database development
After accumulating each primary article reported in the proposed field, a database of relevant articles is developed for the assessment and analysis work, to find the current available trends in healthcare big data analytical domain and investigate the gaps in these research articles to open new gates for future research work. A total of 139 relevant articles are added to the final database. The overall contribution of the selected online repositories in the relevant articles database development is depicted in Fig. 4 .
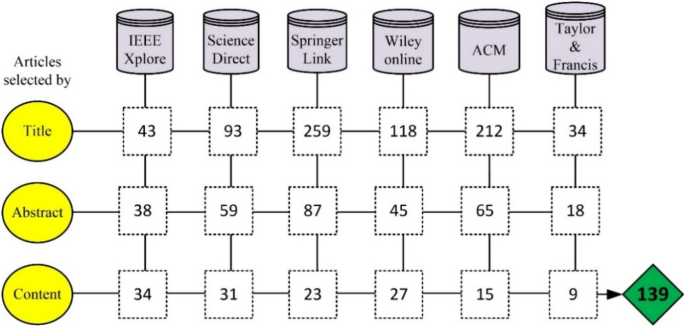
Distribution of primary studies.
From Fig. 4 , it is concluded that IEEE Xplore and Science Direct contributing the more that reflects the interest of research community to present their work with.
After developing a database of relevant articles, it is evaluated using different parameters like type of article (conference proceedings, journal article, book chapter etc.), publication year, and contribution of individual library. Figure 5 represents the information regarding the total contribution of articles by type in the final database.
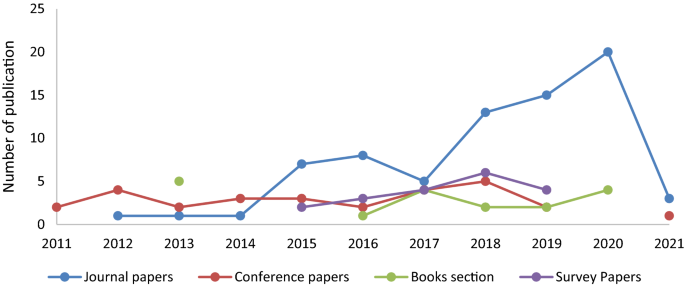
Evolution of final database by type of article and year.
Figure 5 concludes that the researchers paid significant attention towards the development of new healthcare systems instead of finding the gaps in the available systems and develop enhanced solutions accordingly. This enhanced solution can accurately identify and diagnose a certain disease based on patient’s historical medical information. A small amount of work is reported using review articles, survey papers, but no systematic mechanism is followed to analyse the work in specific range of years followed by a set of research questions. The same problem can also be seen from Fig. 6 where highest percentage contribution is shown more comparative to book sections, conference papers etc.
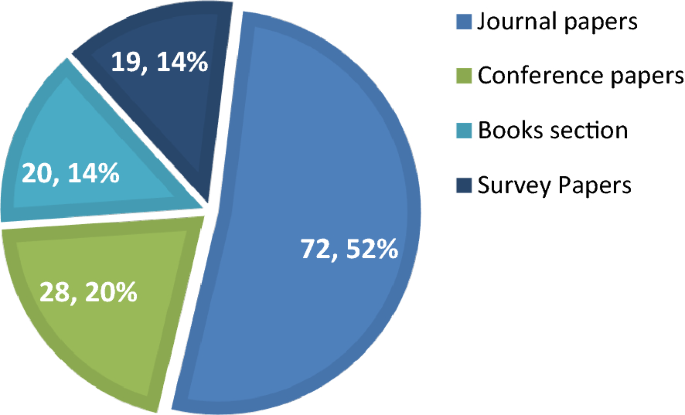
Percentage contribution by type of paper.
Figure 7 depicts the percentage contribution of each library in the proposed assessment work.
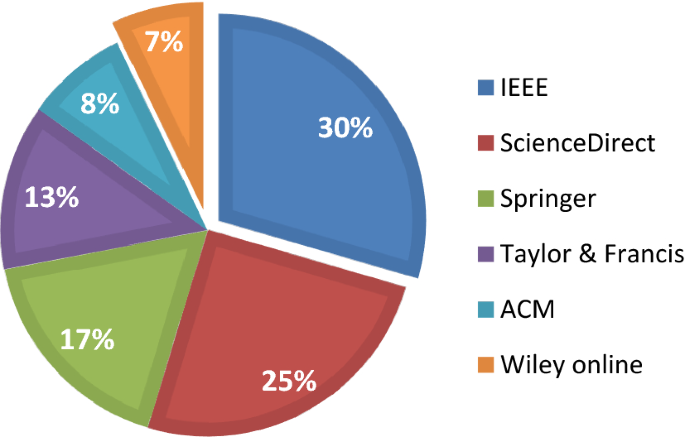
Percentage contribution of each library.
Figure 8 represents the annual distribution of articles selected for the analysis and assessment purposes. Form Fig. 8 it is evident, that with passage of time number of articles increases, and that shows the maturity and interest of the researchers in this specific domain.
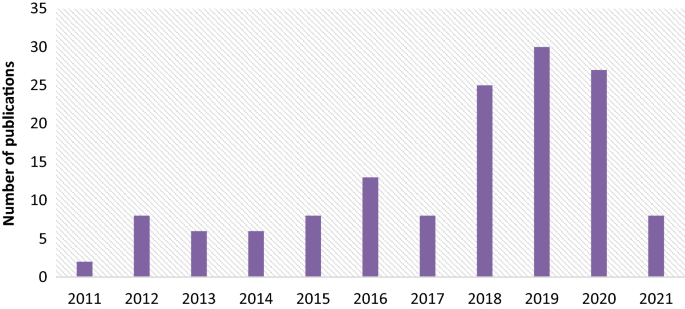
Annual distribution of articles.
From Fig. 8 , it is concluded that IEEE Xplore contributing the more in the final database of relevant articles that shows the trend of researchers to present healthcare relevant works in the IEEE journals. Figure 9 represents the total number of journal articles, survey papers, conference papers, and book sections in the selected relevant articles database.
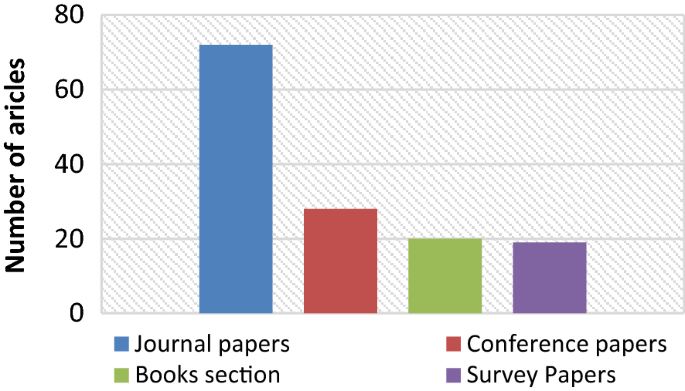
Evolution of database by number of articles by type.
From Fig. 9 it is concluded that significant attention is given towards the development of new healthcare models. This shows the maturity of the proposed field. Dealing with such a mature field and extracting useful information is hectic job for the researchers. A systematic analysis of this research field is needed to provide an overview of the work reported during a specific range of years. This analysis will not only save precious time of the researchers, but it will also open gates for the future research work in this field.
Table 5 represents the annual contribution of studies in the final relevant database.
Overall information regarding type of paper, publication year and number of records is depicted in Fig. 10 below.
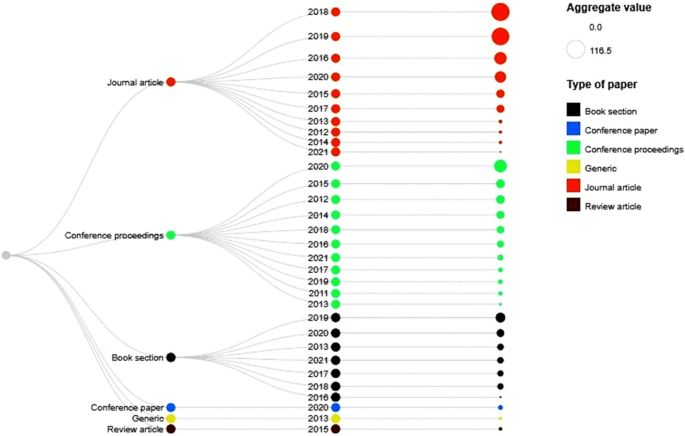
Evolution of final database.
Quality assesment
After executing exclusion and inclusion process, all the relevant articles in the database are manually assessed by authors to check the relevancy of each article with the selected research problem. A quality criterion is defined to check every research article against the formulated research questions. This quality criteria is defined in Table 6 .
Weighted values are assigned against each quality criteria to check the relevancy of an article with a certain research question. These weighted values and description is depicted in Fig. 11 .
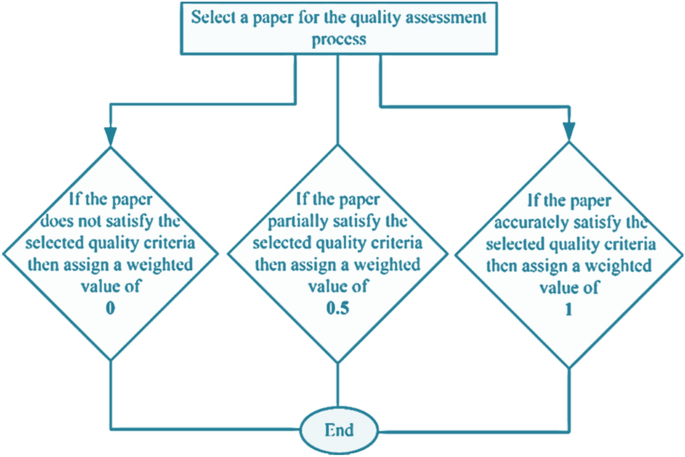
Quality criteria for the proposed SLR work.
After the assessment process, the relevancy of each article is decided based on its aggregated weighting score. If the score is greater than 3 it represents the most relevancy of an article to the selected research topic. Figure 12 represents the aggregate score values of each article based on the defined quality assessment criteria.
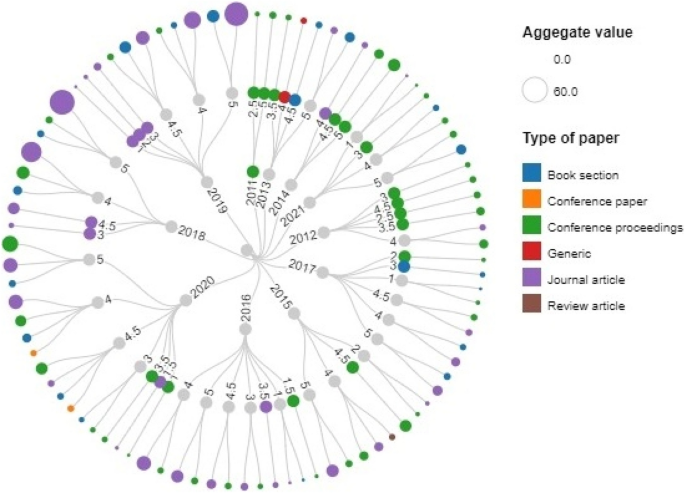
Quality assessment process.
Results and discussion
After executing the quality assessment work, the next key step of an SLR work is, to analyse all the relevant article to identify different techniques proposed for efficient communication between patient and practitioner, accurate feature extraction from healthcare big data and implement it in practical use.
This section of the paper performs a descriptive analysis of each article based on five research questions. In this systematic review process, a total of 139 research articles published during the period ranging from 2011 to 2021.
Healthcare big data
The researcher and data analysts suggested no contextual name for “big data” in healthcare, but for implementation and interpretation purposes they divided it into 5 V architecture. Figure 13 depicts a 5 V architecture of big data.
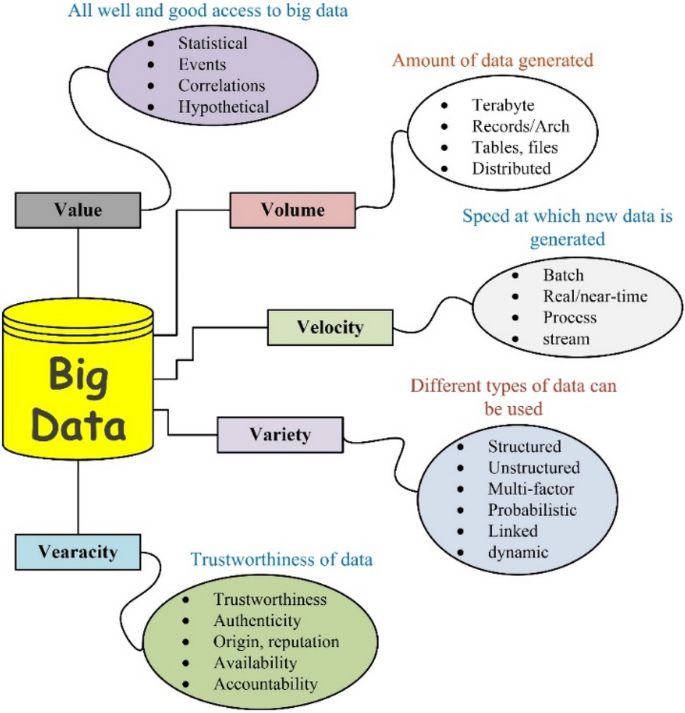
Big Data 5Vs 15 .
The exponential increase in IoT-based smart devices and information systems resulted a plethora of information in healthcare domain. This information increases exponentially on daily basis. These smart IoT based healthcare devices produces a huge of data. An alternated term “Big Data” is selected for this gigantic amount of data. This is the data for which scale, diversity, and complexities require innovative structure, variables, design, and analytics for efficient utilization and management, accurate data extraction and visualization, and to grab hidden stored information regarding a specific problem of interest. Main idea behind the implementation of healthcare big data analytics is to retrieve enriched information from huge amount of data using different machine leering and data mining techniques 191 . These techniques help in improving quality of care, reducing cost of care, and helps the practitioners to suggest medicines based on clinical historical information.
RQ1. What are the key features adapted to integrate the structured and unstructured data in healthcare big data domain?
Big data comprises a huge amount of data to be processed, especially a plethora of types of data to process and extract enriched information regarding a problem of interest. Several features are assessed and analyzed especially in healthcare domain, to integrate both structural and non-structural data. Multiple researchers analyzed semantic based big data features for big data integration purposes while some researchers proposed behavior and structural based features for patient monitoring and activity management purposes 151 , 192 . While some performed real-time analysis using a group of people for data integrating and clustering purposes. Table 7 enlists the research work published for the structural and non-structural data integration purposes.
After analysing the available literature in Table 8 , it was concluded that mostly semantic based, structure-based, and real-time activity-based features are considered for the information extraction and organization purposes. If we consider geometric based feature and adapt clustering mechanism for data organization purposes, then this will not only integrate both structural and non-structural data efficiently, but it will improve the simulation capabilities of different applications.
RQ2. What are different techniques proposed to provide an easy and timely data-access interface for doctors?
Digital transformation of healthcare systems by using of information system, medical technology, handheld and smart wearable devices has posed many challenges for both the researchers and caretakers in the form of storage, dropping the cost of care and processing time (to extract relevant information for refining quality of care and reduce waste and error rates). Prime goal of healthcare big data analytics is, to process this vast amount of data using machine learning and other processing models to extract certain problem relevant information and use it for human well beings 195 . Several supervised and unsupervised classification techniques are followed for the said purposes. ML-based architectures and big data analytical techniques are integrated in healthcare domain for efficient information retrieval and exchange purposes, risk analysis, optimum decision-support system in clinics, and suggesting precise medicines using genomic information 196 . Table 8 represent the literature reported for the providence of an easy and timely data-access interface for the practitioners.
RQ3. What are different ways to improve communication between the doctor and patient?
Healthcare around the world is under high pressure due to limiting financial resources, over-population, and disease burden. In this modern technological age, the healthcare paradigm is shifting from traditional, one-size-fits-all approach to a focus on personalized individual care 1 . Additionally, the healthcare data is varying both in type and amount. The healthcare providers are not only dealing with patient’s historical, physical and namely information, but they also deal with imaging information, labs, and other digital and analogue information consists of ECG, MRI etc. This data is voluminous, varying in type and formats, and of differing structure. These are the capabilities of Big Data to handle not only different types of and forms of data, but can handle 5 V structure including volume, variety, value, veracity, and velocity. Thus, the doctors facing an increasing burden of rising patient numbers coupled with progressively less time to spend with each patient. In other words, we are dealing with more patients, more data, and less time.
Different techniques are proposed in the literature to provide an easy and timely communication interface for both doctors and patients. Table 9 depicts different information exchange tools/techniques reported in the literature.
RQ4. What are different types of classification models proposed for accurate disease diagnosing using patient historical information?
This research question aims to outline different disease diagnosing models proposed in the literature using healthcare big data. Around the world diverse approaches are proposed by researchers for healthcare big data analysis to ensure accurate disease diagnosing capabilities, provide healthcare facilities at doorstep, development of eHealth and mHealth applications, and many others. Multiple statistical and ML-based approaches proposed for accurate diagnosing purposes. Figure 14 represents multiple techniques proposed for automatic disease diagnosing purposes using healthcare big data domain.
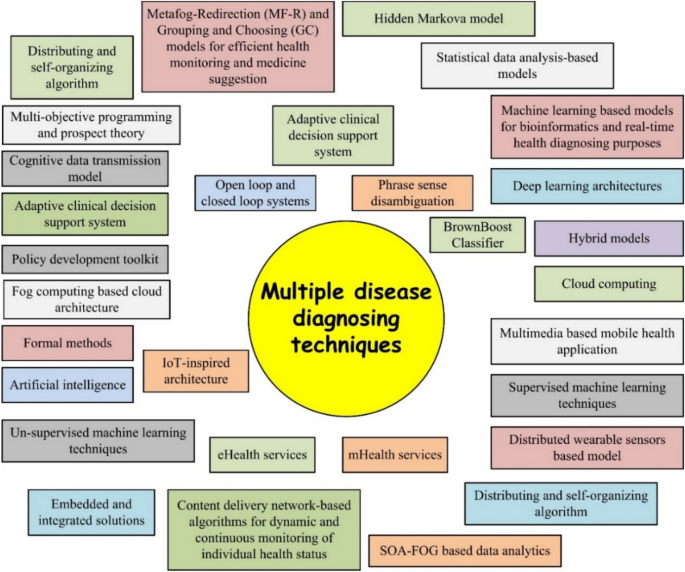
Multiple disease diagnosing techniques proposed in the literature.
All these techniques perform the diagnosing process using semantic-based features or structural based features. But no attention is given towards geometric feature extraction techniques that are prominent in extracting enriched information from data and results in high identification rates. Also, no advanced hybrid neural network and shallow architectures are proposed for the automatic diagnosing purposes. Keeping these gaps in mind, an optimum eHealth application can be developed by applying these hybrid techniques.
RQ5. What are different applications of big data analytics in healthcare domain?
Big data analytics has revolutionized our lives by presenting many state of the art applications in various domains ranging from eHealth to mHealth, weather forecasting to climate changes, traffic management to object detection, and many others. This research question mainly focusing on enlisting different applications of big data analytics in Table 10 .
Limitations
This article has a number of limitations. Some of these limitations are listed below.
For this systematic analysis articles are only accumulated from six different peer-reviewed libraries (ACM, SpringerLink, Taylor & Francis, Science Direct = IEEE Xplore, and Wiley online library), but there exist a number of multi-disciplinary databases for articles accumulation purposes.
This systematic analysis covers a specific range of years (2011 –2021), while a number of articles are reporting on daily basis.
Articles are accumulated from online libraries using search queries, so if a paper has no matching words to the query, then it was skipped during search process.
Google Scholar is skipped during the articles accumulation phase to shorten the searching time. Also, it gives access to both peer-reviewed and non-peer-reviewed journals and we only focused on peer-reviewed journals for the relevant articles.
Being a systematic literature work it can be broadened to grab the knowledge about other varying topics such as healthcare data commercialization, health sociology etc.
Besides these limitations we hope that this systematic research work will be an inspiration for future research in the recommended fields and will open gates for both industrialists and policymakers.
Conclusion and future work
In this research article, the existing research reported during 2011 to 2021 is thoroughly analysed for the efforts made by researchers to help caretakers and clinicians to make authentic decisions in disease diagnosing and suggest medicines accordingly. Based on the research problem and underlying requirements, the researchers proposed several feature extraction, identification, and remote communication frameworks to develop doctor and patient communication in a timely fashion. These real-time or nearer to real-time applications mostly use big data analytics and computational devices. This research work identified several key features and optimum management designs proposed in healthcare big data analytical domain to achieve effective outcomes in disease diagnosing. The results of this systematic work suggests that advanced hybrid machine learning-based models and cloud computing application should be adapted to reduce treatment cost, simulation time, and achieve improved quality of care. The findings of this research work will not only help the policymakers to encourage the researchers and practitioners to develop advanced disease diagnosing models, but it will also assist in presenting an improved quality of treatment mechanism for patients.
Advanced hybrid machine learning architectures for cognitive computing are considered as the future toolbox for the data-driven analysis of healthcare big data. Also, geometric-based features must be considered for feature extraction purposes instead of semantic and structural-based features. These geometric-based feature extraction techniques will not only reduce the simulation time, but it will also improve the identification and disease diagnosing capabilities of smart health devices. Additionally, these features can help in accurate identification of Alzheimer, tumours in PET or MRI images using upgraded machine learning and big data analytics. Cluster-based mechanism should be considered for data organization purposes to improve big data timely-access and easy-management capabilities. Promoting research in these areas will be crucial for future innovation in healthcare domain.
Data availability
The data used and/or analyzed during the current study available from the corresponding author on reasonable request.
Rahman, F. & Slepian, M. J. Application of big-data in healthcare analytics—Prospects and challenges. In 2016 IEEE-EMBS International Conference on Biomedical and Health Informatics (BHI) 13–16 (2016).
Khan, N. et al. Big data: Survey, technologies, opportunities, and challenges. Sci. World J. 2014 , 1–18 (2014).
Google Scholar
Groves, P., Kayyali, B., Knott, D. & Van Kuiken, S. The ‘big data ‘revolution in healthcare. In McKinsey Quarterly (2013).
Andreu-Perez, J., Poon, C. C., Merrifield, R. D., Wong, S. T. & Yang, G.-Z. Big data for health. IEEE J. Biomed. Health Inform. 19 , 1193–1208 (2015).
Article Google Scholar
Kumar, M. A., Vimala, R. & Britto, K. A. A cognitive technology based healthcare monitoring system and medical data transmission. Measurement 146 , 322–332 (2019).
Article ADS Google Scholar
Chen, H., Khan, S., Kou, B., Nazir, S., Liu, W. & Hussain, A. A smart machine learning model for the detection of brain hemorrhage diagnosis based internet of things in smart cities. Complexity 2020 (2020).
Liang, Y. & Zhao, L. Intelligent hospital appointment system based on health data bank. Procedia Comput. Sci. 159 , 1880–1889 (2019).
Galetsi, P. & Katsaliaki, K. A review of the literature on big data analytics in healthcare. J. Oper. Res. Soc. 1–19 (2019).
Lindell, J. What are big data and analytics?. In Analytics and Big Data for Accountants (2018).
Alharthi, H. Healthcare predictive analytics: An overview with a focus on Saudi Arabia. J. Infect. Public Health 11 , 749–756 (2018).
Lee, C. et al. "Big healthcare data analytics: Challenges and applications. In Handbook of Large-Scale Distributed Computing in Smart Healthcare 11–41 (Springer, 2017).
Chapter Google Scholar
Hussain, A., Nazir, S., Khan, S. & Ullah, A. Analysis of PMIPv6 extensions for identifying and assessing the efforts made for solving the issues in the PMIPv6 domain: A systematic review. Comput. Netw. 179 , 107366 (2020).
Khan, H.-U. et al. Systematic analysis of safety and security risks in smart homes. Comput. Mater. Contin. 68 , 1409–1428 (2021).
Khan, S., Nazir, S. & Khan, H.-U. Analysis of navigation assistants for blind and visually impaired people: A systematic review. IEEE Access 9 , 26712–26734 (2021).
Nazir, S. et al. A comprehensive analysis of healthcare big data management, analytics and scientific programming. IEEE Access 8 , 95714–95733 (2020).
Kitchin, R. Big Data, new epistemologies and paradigm shifts. Big Data Soc. 1 , 2053951714528481 (2014).
Cox, M. & Ellsworth, D. Application-controlled demand paging for out-of-core visualization. In Proceedings. Visualization’97 (Cat. No. 97CB36155) 235–244 (1997).
Syed, L., Jabeen, S., Manimala, S. & Elsayed, H. A. Data science algorithms and techniques for smart healthcare using IoT and big data analytics. In Smart Techniques for a Smarter Planet 211–241 (Springer, 2019).
Venkatesh, R., Balasubramanian, C. & Kaliappan, M. Development of big data predictive analytics model for disease prediction using machine learning technique. J. Med. Syst. 43 , 272 (2019).
Article CAS Google Scholar
Kaur, P., Sharma, M. & Mittal, M. Big data and machine learning based secure healthcare framework. Procedia Comput. Sci. 132 , 1049–1059 (2018).
Patel, H. B. & Gandhi, S. A review on big data analytics in healthcare using machine learning approaches. In 2018 2nd International Conference on Trends in Electronics and Informatics (ICOEI) 84–90 (2018).
Rumbold, J. M. M., O’Kane, M., Philip, N. & Pierscionek, B. K. Big Data and diabetes: The applications of Big Data for diabetes care now and in the future. Diabetic Med. (2019).
Oxman, A. D. et al. Users’ guides to the medical literature: VI. How to use an overview. JAMA 272 , 1367–1371 (1994).
Swingler, G. H., Volmink, J. & Ioannidis, J. P. Number of published systematic reviews and global burden of disease: database analysis. BMJ 327 , 1083–1084 (2003).
Research, C. I. O. H. Randomized controlled trials registration/application checklist (12/2006). Available at: http://www.cihr-irsc.gc.ca/e/documents/rct_reg_e.pdf . Accessed 22 June 2009.
Young, C. & Horton, R. Putting clinical trials into context. Lancet 366 , 107–107 (2005).
P. Group, Moher, D., Liberati, A., Tetzlaff, J. & Altman, D. G. Preferred reporting items for systematic reviews and meta-analyses: The PRISMA statement. PLoS Med. 6 , e1000097 (2009).
Kitchenham, B. & Charters, S. Guidelines for performing systematic literature reviews in software engineering (2007).
Van Solingen, R., Basili, V., Caldiera, G. & Rombach, H. D. Goal question metric (gqm) approach. Encycl. Softw. Eng. (2002).
Brereton, P., Kitchenham, B. A., Budgen, D., Turner, M. & Khalil, M. Lessons from applying the systematic literature review process within the software engineering domain. J. Syst. Softw. 80 , 571–583 (2007).
Achimugu, P., Selamat, A., Ibrahim, R. & Mahrin, M. N. R. A systematic literature review of software requirements prioritization research. Inf. Softw. Technol. 56 , 568–585 (2014).
Nazir, S., Ali, Y., Ullah, N. & García-Magariño, I. Internet of things for healthcare using effects of mobile computing: A systematic literature review. Wirel. Commun. Mobile Comput. 109 , 5931315 (2019).
Wohlin, C. Guidelines for snowballing in systematic literature studies and a replication in software engineering. In Proceedings of the 18th International Conference on Evaluation and Assessment in Software Engineering 1–10 (2014).
Kable, A. K., Pich, J. & Maslin-Prothero, S. E. A structured approach to documenting a search strategy for publication: A 12 step guideline for authors. Nurse Educ. Today 32 , 878–886 (2012).
Helmer, A., Kretschmer, F., Müller, F., Eichelberg, M., Deparade, R., Tegtbur, U. et al. Integration of medical models in personal health records using the example of rehabilitation training for cardiopulmonary patients. In 2011 4th International Conference on Biomedical Engineering and Informatics (BMEI) 1887–1892 (2011).
Tian, M. Integrated feature based medical image retrieval. In 2011 International Conference on Control, Automation and Systems Engineering (CASE) 1–3 (2011).
Chaves, R., Ramírez, J., Górriz, J. M., Illán, I. A. & Salas-Gonzalez, D. FDG and PIB biomarker PET analysis for the Alzheimer’s disease detection using Association Rules. In 2012 IEEE Nuclear Science Symposium and Medical Imaging Conference Record (NSS/MIC) 2576–2579 (2012).
Chute, C. G. Obstacles and options for big-data applications in biomedicine: The role of standards and normalizations. In 2012 IEEE International Conference on Bioinformatics and Biomedicine (2012).
Goel, A. & Chandra, N. A prototype model for secure storage of medical images and method for detail analysis of patient records with PACS. In 2012 International Conference on Communication Systems and Network Technologies 167–170 (2012).
Huang, H. & Hsiao, I. Use of anatomical information in a Bayesian reconstruction with an edge-preserving median prior. In 2012 IEEE Nuclear Science Symposium and Medical Imaging Conference Record (NSS/MIC) 3321–3323 (2012).
López, C. M., Welkenhuysen, M., Musa, S., Eberle, W., Bartic, C., Puers, R. et al. Towards a noise prediction model for in vivo neural recording. In 2012 Annual International Conference of the IEEE Engineering in Medicine and Biology Society 759–762 (2012).
Ng, H., Chuang, C. & Hsu, C. Extraction and analysis of structural features of lateral ventricle in brain medical images. In 2012 Sixth International Conference on Genetic and Evolutionary Computing 35–38 (2012).
Patel, A. B., Birla, M. & Nair, U. Addressing big data problem using Hadoop and Map Reduce. In 2012 Nirma University International Conference on Engineering (NUiCONE) 1–5 (2012).
Zheng, G., Yu, L., Feng, Y., Han, Z., Chen, L., Zhang, S. et al. Seizure prediction model based on method of common spatial patterns and support vector machine. In 2012 IEEE International Conference on Information Science and Technology 29–34 (2012).
Li, L., Bagheri, S., Goote, H., Hasan, A. & Hazard, G. Risk adjustment of patient expenditures: A big data analytics approach. In 2013 IEEE International Conference on Big Data 12–14 (2013).
Loshin, D. Chapter 8—Developing big data applications. In Big Data Analytics (ed. Loshin, D.) 73–81 (Morgan Kaufmann, 2013).
Chapter MATH Google Scholar
Loshin, D. Chapter 9—NoSQL data management for big data. In Big Data Analytics (ed. Loshin, D.) 83–90 (Morgan Kaufmann, 2013).
Loshin, D. Chapter 1—Market and business drivers for big data analytics. In Big Data Analytics (ed. Loshin, D.) 1–9 (Morgan Kaufmann, 2013).
MATH Google Scholar
Purkayastha, S. & Braa, J. Big data analytics for developing countries–Using the cloud for operational BI in health. Electron. J. Inf. Syst. Dev. Ctries. 59 , 1–17 (2013).
Lin, C.-H., Huang, L.-C., Chou, S.-C. T., Liu, C.-H., Cheng, H.-F. & Chiang, I. J. Temporal event tracing on big healthcare data analytics. In 2014 IEEE International Congress on Big Data 281–287 (2014)
Martínez, J. G., Ramos-Becerril, F. J., Leija, L., López, F., García, U., Vera, A. et al. Development of an electronic equipment for the pre medical diagnose in the progress of diabetic foot disease. In 2014 International Conference on Control, Decision and Information Technologies (CoDIT) 679–683 (2014).
Mian, M., Teredesai, A., Hazel, D., Pokuri, S. & Uppala, K. Work in progress—In-memory analysis for healthcare big data. In 2014 IEEE International Congress on Big Data 778–779 (2014).
Panahiazar, M., Taslimitehrani, V., Jadhav, A. & Pathak, J. Empowering personalized medicine with big data and semantic web technology: Promises, challenges, and use cases. In 2014 IEEE International Conference on Big Data (Big Data) 790–795 (2014).
Vargheese, R. Dynamic protection for critical health care systems using cisco CWS: Unleashing the power of big data analytics. In 2014 Fifth International Conference on Computing for Geospatial Research and Application 77–81 (2014).
Archenaa, J. & Anita, E. A. M. A survey of big data analytics in healthcare and government. Procedia Comput. Sci. 50 , 408–413 (2015).
Boman, M. & Sanches, P. Sensemaking in intelligent health data analytics. KI Künstliche Intell. 29 , 143–152 (2015).
Chong, D. & Shi, H. Big data analytics: A literature review. J. Manag. Anal. 2 , 175–201 (2015).
Dantanarayana, G., Sahama, T. & Wikramanayake, G. Quality of information for quality of life: Healthcare big data analytics. In 2015 Fifteenth International Conference on Advances in ICT for Emerging Regions (ICTer) 281–281 (2015).
Gomathi, S. & Narayani, V. Implementing big data analytics to predict systemic lupus erythematosus. In 2015 International Conference on Innovations in Information, Embedded and Communication Systems (ICIIECS) 1–5 (2015).
Hussain, S. & Lee, S. Semantic transformation model for clinical documents in big data to support healthcare analytics. In 2015 Tenth International Conference on Digital Information Management (ICDIM) 99–102 (2015).
Kuo, M., Chrimes, D., Moa, B. & Hu, W. Design and construction of a big data analytics framework for health applications. In 2015 IEEE International Conference on Smart City/SocialCom/SustainCom (SmartCity) 631–636 (2015).
Mehmood, R. & Graham, G. Big data logistics: A health-care transport capacity sharing model. Procedia Comput. Sci. 64 , 1107–1114 (2015).
Raj, P., Raman, A., Nagaraj, D. & Duggirala, S. Big data analytics for healthcare. In High-Performance Big-Data Analytics Computer Communications and Networks 1525–1525 (Springer, Cham, 2015).
Viceconti, M., Hunter, P. & Hose, R. Big data, big knowledge: Big data for personalized healthcare. IEEE J. Biomed. Health Inform. 19 , 1209–1215 (2015).
Wang, M. D. Biomedical big data analytics for patient-centric and outcome-driven precision health. In 2015 IEEE 39th Annual Computer Software and Applications Conference 1–2 (2015).
Batarseh, F. A. & Latif, E. A. Assessing the quality of service using big data analytics: With application to healthcare. Big Data Res. 4 , 13–24 (2016).
Buzzi, M. C. et al. Facebook: A new tool for collecting health data?. Multimed. Tools Appl. 76 , 10677–10700 (2016).
Chauhan, R., Jangade, R. & Mudunuru, V. K. A cloud based environment for big data analytics in healthcare. In International Conference on Soft Computing and Pattern Recognition 315–321 (2016).
Stefano, A. D., Corte, A. L., Lió, P. & Scatá, M. Bio-inspired ICT for big data management in healthcare. In Intelligent Agents in Data-intensive Computing 1–26 (Springer, 2016).
Gupta, S. & Tripathi, P. An emerging trend of big data analytics with health insurance in India. In 2016 International Conference on Innovation and Challenges in Cyber Security (ICICCS-INBUSH) 64–69 (2016).
Haas, M. et al. Big data to smart data in Alzheimer’s disease: Real-world examples of advanced modeling and simulation. Alzheimers Dement. 12 , 1022–1030 (2016).
Jiang, P. et al. An intelligent information forwarder for healthcare big data systems with distributed wearable sensors. IEEE Syst. J. 10 , 1147–1159 (2016).
Kankanhalli, A., Hahn, J., Tan, S. & Gao, G. Big data and analytics in healthcare: Introduction to the special section. Inf. Syst. Front. 18 , 233–235 (2016).
Kashyap, H., Ahmed, H. A., Hoque, N., Roy, S. & Bhattacharyya, D. K. Big data analytics in bioinformatics: Architectures, techniques, tools and issues. Netw. Model. Anal. Health Inform. Bioinform. 5 , 28 (2016).
Lv, Z., Chirivella, J. & Gagliardo, P. Bigdata oriented multimedia mobile health applications. J. Med. Syst. 40 , 120 (2016).
Pandey, M. K. & Subbiah, K. A novel storage architecture for facilitating efficient analytics of health informatics big data in cloud. In 2016 IEEE International Conference on Computer and Information Technology (CIT) 578–585 (2016).
Plachkinova, M., Vo, A., Bhaskar, R. & Hilton, B. A conceptual framework for quality healthcare accessibility: A scalable approach for big data technologies. Inf. Syst. Front. 20 , 289–302 (2016).
Rallapalli, S., Gondkar, R. R. & Ketavarapu, U. P. K. Impact of processing and analyzing healthcare big data on cloud computing environment by implementing hadoop cluster. Procedia Comput. Sci. 85 , 16–22 (2016).
Sakr, S. & Elgammal, A. Towards a comprehensive data analytics framework for smart healthcare services. Big Data Res. 4 , 44–58 (2016).
Xu, B. et al. Healthcare data analytics: Using a metadata annotation approach for integrating electronic hospital records. J. Manag. Anal. 3 , 136–151 (2016).
Tresp, V. et al. Going digital: A survey on digitalization and large-scale data analytics in healthcare. Proc. IEEE 104 , 2180–2206 (2016).
Straton, N., Hansen, K., Mukkamala, R. R., Hussain, A., Gronli, T., Langberg, H. et al. Big social data analytics for public health: Facebook engagement and performance. In 2016 IEEE 18th International Conference on e-Health Networking, Applications and Services (Healthcom) 1–6 (2016).
Abouelmehdi, K., Beni-Hssane, A., Khaloufi, H. & Saadi, M. Big data security and privacy in healthcare: A review. Procedia Comput. Sci. 113 , 73–80 (2017).
Alonso, S. G., de la Torre, Diez I., Rodrigues, J. J., Hamrioui, S. & Lopez-Coronado, M. A systematic review of techniques and sources of big data in the healthcare sector. J. Med. Syst. 41 , 183 (2017).
Anjum, A. et al. Big data analytics in healthcare: A cloud-based framework for generating insights. In Cloud Computing 153–170 (Springer, 2017).
Barik, R. K., Dubey, H. & Mankodiya, K. SOA-FOG: Secure service-oriented edge computing architecture for smart health big data analytics. In 2017 IEEE Global Conference on Signal and Information Processing (GlobalSIP) 477–481 (2017).
Cano, I., Tenyi, A., Vela, E., Miralles, F. & Roca, J. Perspectives on big data applications of health information. Curr. Opin. Syst. Biol. 3 , 36–42 (2017).
A. Di Meglio and M. Manca, "From Big Data to Big Insights: The Role of Platforms in Healthcare IT," in New Perspectives in Medical Records, ed: Springer, 2017, pp. 33–47.
Manogaran, G. et al. Big data analytics in healthcare Internet of Things. In Innovative Healthcare Systems for the 21st Century 263–284 (Springer, 2017).
Plageras, A. P., Stergiou, C., Kokkonis, G., Psannis, K. E., Ishibashi, Y., Kim, B. et al. Efficient large-scale medical data (eHealth Big Data) analytics in Internet of Things. In 2017 IEEE 19th Conference on Business Informatics (CBI) 21–27 (2017).
Pramanik, M. I., Lau, R. Y. K., Demirkan, H. & Azad, M. A. K. Smart health: Big data enabled health paradigm within smart cities. Expert Syst. Appl. 87 , 370–383 (2017).
Spanoudakis, G., Katrakazas, P., Koutsouris, D., Kikidis, D., Bibas, A. & Pontopidan, N. H. Public health policy for management of hearing impairments based on big data analytics: EVOTION at genesis. In 2017 IEEE 17th International Conference on Bioinformatics and Bioengineering (BIBE) 525–530 (2017).
Wu, J., Li, H., Liu, L. & Zheng, H. Adoption of big data and analytics in mobile healthcare market: An economic perspective. Electron. Commer. Res. Appl. 22 , 24–41 (2017).
Aceto, G., Persico, V. & Pescape, A. The role of Information and Communication Technologies in healthcare: Taxonomies, perspectives, and challenges. J. Netw. Comput. Appl. 107 , 125–154 (2018).
Antoniou, C., Dimitriou, L. & Pereira, F. Mobility Patterns, Big Data and Transport Analytics: Tools and Applications for Modeling (Elsevier, 2018).
Bates, D. W., Heitmueller, A., Kakad, M. & Saria, S. Why policymakers should care about “big data” in healthcare. Health Policy Technol. 7 , 211–216 (2018).
Choi, T.-M., Wallace, S. W. & Wang, Y. Big data analytics in operations management. Prod. Oper. Manag. 27 , 1868–1883 (2018).
Forestiero, A. & Papuzzo, G. Distributed algorithm for big data analytics in healthcare. In 2018 IEEE/WIC/ACM International Conference on Web Intelligence (WI) 776–779 (2018).
Ganesh, S. & Talukder, A. K. Formal methods, artificial intelligence, big-data analytics, and knowledge engineering in medical care to reduce disease burden and health disparities. In International Conference on Big Data Analytics 307–321 (2018).
Giacalone, M., Cusatelli, C. & Santarcangelo, V. Big data compliance for innovative clinical models. Big Data Res. 12 , 35–40 (2018).
Guha, S. & Kumar, S. Emergence of big data research in operations management, information systems, and healthcare: Past contributions and future roadmap. Prod. Oper. Manag. 27 , 1724–1735 (2018).
Gupta, V., Singh Gill, H., Singh, P. & Kaur, R. An energy efficient fog-cloud based architecture for healthcare. J. Stat. Manag. Syst. 21 , 529–537 (2018).
Hopp, W. J., Li, J. & Wang, G. Big data and the precision medicine revolution. Prod. Oper. Manag. 27 , 1647–1664 (2018).
Huang, H. K. Big data in PACS-based multimedia medical imaging informatics. In PACS Based Multimedia Imaging Informatics (ed Huang, H.) 575–589 (2018).
Istepanian, R. S. H. & Al-Anzi, T. m-Health 2.0: New perspectives on mobile health, machine learning and big data analytics. Methods 151 , 34–40 (2018).
Khaloufi, H., Abouelmehdi, K., Beni-hssane, A. & Saadi, M. Security model for big healthcare data lifecycle. Procedia Comput. Sci. 141 , 294–301 (2018).
Krittanawong, C., Johnson, K. W., Hershman, S. G. & Tang, W. H. W. Big data, artificial intelligence, and cardiovascular precision medicine. Expert Rev. Precis. Med. Drug Dev. 3 , 305–317 (2018).
Ma, X., Wang, Z., Zhou, S., Wen, H. & Zhang, Y. Intelligent healthcare systems assisted by data analytics and mobile computing. In 2018 14th International Wireless Communications & Mobile Computing Conference (IWCMC) 1317–1322 (2018).
Manogaran, G. et al. A new architecture of Internet of Things and big data ecosystem for secured smart healthcare monitoring and alerting system. Future Gener. Comput. Syst. 82 , 375–387 (2018).
Mehta, N. & Pandit, A. Concurrence of big data analytics and healthcare: A systematic review. Int. J. Med. Inform. 114 , 57–65 (2018).
Miller, J. B. Big data and biomedical informatics: Preparing for the modernization of clinical neuropsychology. Clin. Neuropsychol. 33 , 287–304 (2018).
Moutselos, K., Kyriazis, D. & Maglogiannis, I. A web based modular environment for assisting health policy making utilizing big data analytics. In 2018 9th International Conference on Information, Intelligence, Systems and Applications (IISA) 1–5 (2018).
Nair, L. R., Shetty, S. D. & Shetty, S. D. Applying spark based machine learning model on streaming big data for health status prediction. Comput. Electr. Eng. 65 , 393–399 (2018).
Pashazadeh, A. & Navimipour, N. J. Big data handling mechanisms in the healthcare applications: A comprehensive and systematic literature review. J. Biomed. Inform. 82 , 47–62 (2018).
Ravishankar Rao, A., Clarke, D. & Vargas, M. Building an open health data analytics platform: A case study examining relationships and trends in seniority and performance in healthcare providers. J. Healthc. Inform. Res. 2 , 44–70 (2018).
Sahoo, P. K., Mohapatra, S. K. & Wu, S.-L. SLA based healthcare big data analysis and computing in cloud network. J. Parallel Distrib. Comput. 119 , 121–135 (2018).
Sarkar, B. K. & Sana, S. S. A conceptual distributed framework for improved and secured healthcare system. Int. J. Healthc. Manag. 1–13 (2018).
Sebaa, A., Chikh, F., Nouicer, A. & Tari, A. Medical big data warehouse: architecture and system design, a case study: Improving healthcare resources distribution. J. Med. Syst. 42 , 59 (2018).
Shafqat, S., Kishwer, S., Rasool, R. U., Qadir, J., Amjad, T. & Ahmad, H. F. Big data analytics enhanced healthcare systems: A review. J. Supercomput.
Sivaparthipan, C. B., Karthikeyan, N. & Karthik, S. Designing statistical assessment healthcare information system for diabetics analysis using big data. Multimed. Tools Appl.
Tang, V. et al. An adaptive clinical decision support system for serving the elderly with chronic diseases in healthcare industry. Expert. Syst. 36 , e12369 (2018).
Wang, Y., Kung, L. & Byrd, T. A. Big data analytics: Understanding its capabilities and potential benefits for healthcare organizations. Technol. Forecast. Soc. Change 126 , 3–13 (2018).
Agrawal, A. & Choudhary, A. Health services data: Big data analytics for deriving predictive healthcare insights. In Health Services Evaluation 3–18 (2019).
Ahmed, M., Choudhury, S. & Al-Turjman, F. Big data analytics for intelligent internet of things. In Artificial Intelligence in IoT 107–127 (Springer, 2019).
Ahmed, Z. & Liang, B. T. Systematically dealing practical issues associated to healthcare data analytics. In Future of Information and Communication Conference 599–613 (2019).
Bora, D. J. Chapter 3—Big data analytics in healthcare: A critical analysis. In Big Data Analytics for Intelligent Healthcare Management (eds Dey, N. et al. ) 43–57 (Academic Press, 2019).
Chanchaichujit, J., Tan, A., Meng, F. & Eaimkhong, S. Internet of Things (IoT) and big data analytics in healthcare. In Healthcare 4.0 17–36 (Springer, 2019).
Cirillo, D. & Valencia, A. Big data analytics for personalized medicine. Curr. Opin. Biotechnol. 58 , 161–167 (2019).
Dey, N., Das, H., Naik, B. & Behera, H. S. Big Data Analytics for Intelligent Healthcare Management (Academic Press, 2019).
Din, S. & Paul, A. Smart health monitoring and management system: Toward autonomous wearable sensing for Internet of Things using big data analytics. Future Gener. Comput. Syst. 91 , 611–619 (2019).
Galetsi, P., Katsaliaki, K. & Kumar, S. Values, challenges and future directions of big data analytics in healthcare: A systematic review. Soc. Sci. Med. 241 , 112533 (2019).
Guo, C. & Chen, J. Big data analytics in healthcare: data-driven methods for typical treatment pattern mining. J. Syst. Sci. Syst. Eng. 28 , 694–714 (2019).
Hussain, S. et al. Semantic preservation of standardized healthcare documents in big data. Int. J. Med. Inform. 129 , 133–145 (2019).
Mehta, N., Pandit, A. & Shukla, S. Transforming healthcare with big data analytics and artificial intelligence: A systematic mapping study. J. Biomed. Inform. 100 , 103311 (2019).
Muniasamy, A., Tabassam, S., Hussain, M. A., Sultana, H., Muniasamy, V. & Bhatnagar, R. Deep learning for predictive analytics in healthcare. In International Conference on Advanced Machine Learning Technologies and Applications 32–42 (2019).
Palanisamy, V. & Thirunavukarasu, R. Implications of big data analytics in developing healthcare frameworks–A review. J. King Saud Univ. Comput. Inf. Sci. 31 , 415–425 (2019).
Rajabion, L., Shaltooki, A. A., Taghikhah, M., Ghasemi, A. & Badfar, A. Healthcare big data processing mechanisms: The role of cloud computing. Int. J. Inf. Manag. 49 , 271–289 (2019).
Ramasamy, V., Gomathy, B. & Verma, R. K. Smart HIV/AIDS digital system using big data analytics. In Progress in Advanced Computing and Intelligent Engineering 415–421 (Springer, 2019).
Razzak, M. I., Imran, M. & Xu, G. Big data analytics for preventive medicine. Neural Comput. Appl.
Reiz, A. N., de la Hoz, M. A. & García, M. S. Big data analysis and machine learning in intensive care units. Med. Intensiva 43 , 416–426 (2019).
Saheb, T. & Izadi, L. Paradigm of IoT big data analytics in the healthcare industry: A review of scientific literature and mapping of research trends. Telematics Inform. 41 , 70–85 (2019).
Sahoo, A. K. et al. Chapter 9—Intelligence-based health recommendation system using big data analytics. In Big Data Analytics for Intelligent Healthcare Management (eds Dey, N. et al. ) 227–246 (Academic Press, 2019).
Shahbaz, M., Gao, C., Zhai, L., Shahzad, F. & Hu, Y. Investigating the adoption of big data analytics in healthcare: The moderating role of resistance to change. J. Big Data 6 , 6 (2019).
Sivaparthipan, C. B. et al. Innovative and efficient method of robotics for helping the Parkinson’s disease patient using IoT in big data analytics. Trans. Emerg. Telecommun. Technol. 31 , e3838 (2019).
Sousa, M. J., Pesqueira, A. N. M., Lemos, C., Sousa, M. & Rocha, Ãl. Decision-making based on big data analytics for people management in healthcare organizations. J. Med. Syst. 43 , 290 (2019).
Strang, K. D. Problems with research methods in medical device big data analytics. Int. J. Data Sci. Anal.
Thomas, J., Kneale, D., McKenzie, J. E., Brennan, S. E. & Bhaumik, S. Determining the scope of the review and the questions it will address. In Cochrane Handbook for Systematic Reviews of Interventions 13–31 (2019).
Wang, Y., Kung, L., Gupta, S. & Ozdemir, S. Leveraging big data analytics to improve quality of care in healthcare organizations: A configurational perspective. Br. J. Manag. 30 , 362–388 (2019).
Zetino, J. & Mendoza, N. Big data and its utility in social work: Learning from the big data revolution in business and healthcare. Soc. Work Public Health 34 , 409–417 (2019).
Nazir, S., Nawaz, M., Adnan, A., Shahzad, S. & Asadi, S. Big data features, applications, and analytics in cardiology—A systematic literature review. IEEE Access 7 , 143742–143771 (2019).
Shah, G., Shah, A. & Shah, M. Panacea of challenges in real-world application of big data analytics in healthcare sector. J. Data Inf. Manag. 1 , 107–116 (2019).
Galetsi, P., Katsaliaki, K. & Kumar, S. Big data analytics in health sector: Theoretical framework, techniques and prospects. Int. J. Inf. Manag. 50 , 206–216 (2020).
Iyengar, S. P., Acharya, H. & Kadam, M. Big data analytics in healthcare using spreadsheets. In Big Data Analytics in Healthcare 155–187 (Springer, 2002).
Kumar, S. A. & Venkatesulu, M. BrownBoost classifier-based bloom hash data storage for healthcare big data analytics. In Information and Communication Technology for Sustainable Development 53–69 (Springer, 2020).
Kumar, Y., Sood, K., Kaul, S. & Vasuja, R. Big data analytics and its benefits in healthcare. In Big Data Analytics in Healthcare 3–21 (Springer, 2020).
Naqishbandi, T. A. & Ayyanathan, N. Clinical big data predictive analytics transforming healthcare:-An integrated framework for promise towards value based healthcare. In Advances in Decision Sciences 545–561 (Springer, 2020).
Lambay, M. A. & Mohideen, S. P. Big data analytics for healthcare recommendation systems. In 2020 International Conference on System, Computation, Automation and Networking (ICSCAN) 1–6 (2020).
Katarya, R. & Jain, S. Exploration of big data analytics in healthcare analytics. In 2020 4th International Conference on Computer, Communication and Signal Processing (ICCCSP) 1–4 (2020).
Javid, T., Faris, M., Beenish, H. & Fahad, M. Cybersecurity and data privacy in the cloudlet for preliminary healthcare big data analytics. In 2020 International Conference on Computing and Information Technology (ICCIT-1441) 1–4 (2020).
Leung, C. K., Chen, Y., Hoi, C. S. H., Shang, S. & Cuzzocrea, A. Machine learning and OLAP on big COVID-19 data. In 2020 IEEE International Conference on Big Data (Big Data) 5118–5127 (2020).
Akhtar, U., Lee, J. W., Bilal, H. S. M., Ali, T., Khan, W. A. & Lee, S. The impact of big data in healthcare analytics. In 2020 International Conference on Information Networking (ICOIN) 61–63 (2020).
Mung, P. S. & Phyu, S. Effective analytics on healthcare big data using ensemble learning. In 2020 IEEE Conference on Computer Applications (ICCA) 1–4 (2002).
Georgakopoulos, S. V., Gallos, P. & Plagianakos, V. P. Using big data analytics to detect fraud in healthcare provision. In 2020 IEEE 5th Middle East and Africa Conference on Biomedical Engineering (MECBME) 1–3 (2020).
Leung, C. K., Chen, Y., Shang, S. & Deng, D. Big data science on COVID-19 Data. In 2020 IEEE 14th International Conference on Big Data Science and Engineering (BigDataSE) 14–21 (2020).
Juddoo, S. & George, C. A Qualitative assessment of machine learning support for detecting data completeness and accuracy issues to improve data analytics in big data for the healthcare industry. In 2020 3rd International Conference on Emerging Trends in Electrical, Electronic and Communications Engineering (ELECOM) 58–66 (2020).
Chauhan, R. & Yafi, E. Big data analytics for prediction modelling in healthcare databases. In 2021 15th International Conference on Ubiquitous Information Management and Communication (IMCOM) 1–5 (2021).
Islam, M., Karim, R., Khatun, M. A. & Reza, S. A research on big data analytics in healthcare industry. In 2020 International Conference on Information Science and Communications Technologies (ICISCT) 1–5 (2020).
Leung, C. K., Chen, Y., Hoi, C. S. H., Shang, S., Wen, Y. & Cuzzocrea, A. Big data visualization and visual analytics of COVID-19 data. In 2020 24th International Conference Information Visualisation (IV) 415–420 (2020).
Balaji, S. & Prasathkumar, V. Dynamic changes by big data in health care. In 2020 International Conference on Computer Communication and Informatics (ICCCI) 1–4 (2020).
Alahmar, A. & Benlamri, R. Optimizing hospital resources using big data analytics with standardized e-clinical pathways. In 2020 IEEE Intl Conf on Dependable, Autonomic and Secure Computing, Intl Conf on Pervasive Intelligence and Computing, Intl Conf on Cloud and Big Data Computing, Intl Conf on Cyber Science and Technology Congress (DASC/PiCom/CBDCom/CyberSciTech) 650–657 (2020).
Sadineni, P. K. Developing a model to enhance the quality of health informatics using big data. In 2020 Fourth International Conference on I-SMAC (IoT in Social, Mobile, Analytics and Cloud) (I-SMAC) 1267–1272 (2020).
Pramanik, M. I. et al. Healthcare informatics and analytics in big data. Expert Syst. Appl. 152 , 113388 (2020).
Ravikumaran, P., Vimala Devi, K., Kartheeban, K. & Narayanan Prasanth, N. Health data analytics: Framework & review on tool & technology. Mater. Today Proc. (2020).
Ramesh, T. & Santhi, V. Exploring big data analytics in health care. Int. J. Intell. Netw. 1 , 135–140 (2020).
Galetsi, P. & Katsaliaki, K. A review of the literature on big data analytics in healthcare. J. Oper. Res. Soc. 71 , 1511–1529 (2020).
Mehta, N., Pandit, A. & Kulkarni, M. Elements of healthcare big data analytics. In Big Data Analytics in Healthcare 23–43 (Springer, 2020).
Ehwerhemuepha, L. et al. HealtheDataLab–a cloud computing solution for data science and advanced analytics in healthcare with application to predicting multi-center pediatric readmissions. BMC Med. Inform. Decis. Mak. 20 , 1–12 (2020).
Sivasangari, A., Lakshmanan, L., Ajitha, P., Deepa, D. & Jabez, J. Big data analytics for 5G-enabled IoT healthcare. In Blockchain for 5G-Enabled IoT 261.
Ma, S. & Huai, J. Approximate computation for big data analytics. SIGWEB Newsl. (2021).
Uzunbaz, S. & Aref, W. G. Shared execution techniques for business data analytics over big data streams. In Presented at the 32nd International Conference on Scientific and Statistical Database Management, Vienna, Austria (2020).
Chalumporn, G. & Hewett, R. Health data analytics with an opportunistic big data algorithm. In Presented at the Proceedings of the 11th International Conference on Advances in Information Technology, Bangkok, Thailand (2020).
Minami, T. & Ohura, Y. Small data analysis for bigger data analysis. In Presented at the 2021 Workshop on Algorithm and Big Data, Fuzhou, China (2021).
Chakraborty, C. & Rathi, M. Chapter 2—Smart healthcare systems using big data. In Demystifying Big Data, Machine Learning, and Deep Learning for Healthcare Analytics (eds Kautish, P. N. S. & Peng, S.-L.) 17–32 (Academic Press, 2021).
Ilmudeen, A. Chapter 3—Big data-based frameworks for healthcare systems. In Demystifying Big Data, Machine Learning, and Deep Learning for Healthcare Analytics (eds Kautish, P. N. S. & Peng, S.-L.) 33–56 (Academic Press, 2021).
Mendhe, C. H., Henderson, N., Srivastava, G. & Mago, V. A scalable platform to collect, store, visualize, and analyze big data in real time. IEEE Trans. Comput. Soc. Syst. 8 , 260–269 (2021).
Sivabalaselvamani, D., Selvakarthi, D., Yogapriya, J., Thiruvenkatasuresh, M. P., Maruthappa, M. & Chandra, A. S. Artificial Intelligence in data-driven analytics for the personalized healthcare. In 2021 International Conference on Computer Communication and Informatics (ICCCI) 1–5 (2021)
Harb, H., Mansour, A., Nasser, A., Cruz, E. M. & de la Torre Diez, I. A sensor-based data analytics for patient monitoring in connected healthcare applications. IEEE Sens. J. 21 , 974–984 (2021).
Article ADS CAS Google Scholar
Jones, J. & Jones, J. Optimizing healthcare. In 2020 IEEE International Conference on E-health Networking, Application & Services (HEALTHCOM) 1–6 (2021).
Hassan, S., Dhali, M., Zaman, F. & Tanveer, M. Big data and predictive analytics in healthcare in Bangladesh: Regulatory challenges. Heliyon 7 , e07179 (2021).
Khan, S. et al. KNN and ANN-based recognition of handwritten pashto letters using zoning features. Mach. Learn. 9 , 570–577 (2018).
Pant, D., Kumar, V., Kishore, J. & Pal, R. Healthcare data modeling in R. In 2017 1st International Conference on Intelligent Systems and Information Management (ICISIM) 230–233 (2017).
Brennan, P. F. & Bakken, S. Nursing needs big data and big data needs nursing. J. Nurs. Scholarsh. 47 , 477–484 (2015).
Sreedevi, A. G., Nitya Harshitha, T., Sugumaran, V. & Shankar, P. Application of cognitive computing in healthcare, cybersecurity, big data and IoT: A literature review. Inform. Process. Manag. 59 , 102888 (2022).
Sinha, A., Hripcsak, G. & Markatou, M. Large datasets in biomedicine: A discussion of salient analytic issues. J. Am. Med. Inform. Assoc. JAMIA 16 , 759–767 (2009).
Alonso-Betanzos, A. & Bolón-Canedo, V. Big-Data analysis, cluster analysis, and machine-learning approaches (2018).
Dayal, M. & Singh, N. Indian health care analysis using big data programming tool. Procedia Comput. Sci. 89 , 521–527 (2016).
Jayaraman, P. P., Forkan, A. R. M., Morshed, A., Haghighi, P. D. & Kang, Y.-B. Healthcare 4.0: A review of frontiers in digital health. WIREs Data Min. Knowl. Discov. 10 , e1350 (2018).
Gallos, P. et al. CrowdHEALTH: Big data analytics and holistic health records. Stud. Health Technol. Inform. 258 , 255–256 (2019).
Wang, L., Ranjan, R., Kołodziej, J., Zomaya, A. & Alem, L. Software tools and techniques for big data computing in healthcare clouds. Future Gener. Comput. Syst. 43–44 , 38–39 (2015).
Kiourtis, A. et al. An autoscaling platform supporting graph data modelling big data analytics. Stud. Health Technol. Inform. 295 , 376–379 (2022).
Download references
Acknowledgements
This research work is performed by Department of Accounting and Information Systems, Collage of Business and Economics, Qatar University in collaboration with the Department of Computer Science, University of Swabi, Swabi, Pakistan.
Open Access funding provided by the Qatar National Library. This research was funded by Qatar University Internal Grant under Grant No. IRCC-2021–010. The findings achieved herein are solely the responsibility of the authors.
Author information
Authors and affiliations.
Department of Accounting and Information Systems, College of Business and Economics, Qatar University, Doha, Qatar
Sulaiman Khan & Habib Ullah Khan
Department of Computer Science, University of Swabi, Swabi, Pakistan
You can also search for this author in PubMed Google Scholar
Contributions
S.K. wrote the original draft of the paper. He also revised the draft based on the reviewers suggestions. Dr. H.U.K. developed the experimental setup for the proposed systematic research work. Dr. S.N. performed articles accumulation and database development process.
Corresponding author
Correspondence to Habib Ullah Khan .
Ethics declarations
Competing interests.
The authors declare no competing interests.
Additional information
Publisher's note.
Springer Nature remains neutral with regard to jurisdictional claims in published maps and institutional affiliations.
Rights and permissions
Open Access This article is licensed under a Creative Commons Attribution 4.0 International License, which permits use, sharing, adaptation, distribution and reproduction in any medium or format, as long as you give appropriate credit to the original author(s) and the source, provide a link to the Creative Commons licence, and indicate if changes were made. The images or other third party material in this article are included in the article's Creative Commons licence, unless indicated otherwise in a credit line to the material. If material is not included in the article's Creative Commons licence and your intended use is not permitted by statutory regulation or exceeds the permitted use, you will need to obtain permission directly from the copyright holder. To view a copy of this licence, visit http://creativecommons.org/licenses/by/4.0/ .
Reprints and permissions
About this article
Cite this article.
Khan, S., Khan, H.U. & Nazir, S. Systematic analysis of healthcare big data analytics for efficient care and disease diagnosing. Sci Rep 12 , 22377 (2022). https://doi.org/10.1038/s41598-022-26090-5
Download citation
Received : 09 September 2022
Accepted : 09 December 2022
Published : 26 December 2022
DOI : https://doi.org/10.1038/s41598-022-26090-5
Share this article
Anyone you share the following link with will be able to read this content:
Sorry, a shareable link is not currently available for this article.
Provided by the Springer Nature SharedIt content-sharing initiative
This article is cited by
The usage of population and disease registries as pre-screening tools for clinical trials, a systematic review.
- Juliette Foucher
- Louisa Azizi
- Caroline Ingre
Systematic Reviews (2024)
By submitting a comment you agree to abide by our Terms and Community Guidelines . If you find something abusive or that does not comply with our terms or guidelines please flag it as inappropriate.
Quick links
- Explore articles by subject
- Guide to authors
- Editorial policies
Sign up for the Nature Briefing: Translational Research newsletter — top stories in biotechnology, drug discovery and pharma.

- Open access
- Published: 22 June 2022
How can big data analytics be used for healthcare organization management? Literary framework and future research from a systematic review
- Nicola Cozzoli 1 ,
- Fiorella Pia Salvatore ORCID: orcid.org/0000-0001-6294-3360 1 ,
- Nicola Faccilongo 1 &
- Michele Milone 1
BMC Health Services Research volume 22 , Article number: 809 ( 2022 ) Cite this article
20k Accesses
31 Citations
17 Altmetric
Metrics details
Multiple attempts aimed at highlighting the relationship between big data analytics and benefits for healthcare organizations have been raised in the literature. The big data impact on health organization management is still not clear due to the relationship’s multi-disciplinary nature. This study aims to answer three research questions: a) What is the state of art of big data analytics adopted by healthcare organizations? b) What about the benefits for both health managers and healthcare organizations? c) What about future directions on big data analytics research in healthcare?
Through a systematic literature review the impact of big data analytics on healthcare management has been examined. The study aims to map extant literature and present a framework for future scholars to further build on, and executives to be guided by.
The positive relationship between big data analytics and healthcare organization management has emerged. To find out common elements in the studies reviewed, 16 studies have been selected and clustered into 4 research areas: 1) Potentialities of big data analytics. 2) Resource management. 3) Big data analytics and management of health surveillance systems. 4) Big data analytics and technology for healthcare organization.
Conclusions
In conclusion is identified how the big data analytics solutions are considered a milestone for managerial studies applied to healthcare organizations, although scientific research needs to investigate standardization and integration of the devices as well as the protocol in data analysis to improve the performance of the healthcare organization.
Peer Review reports
Big data is transforming and will transform the healthcare organizations in the near future [ 1 , 2 ]. Scientific literature in the managerial context applied to healthcare organizations, consider the Big Data Analytics (BDA) a fundamental tool, so much so that it has attracted the attention of the scientific community and stakeholders [ 3 ]. However, a premise should be made: data by themselves explain little, thus, to be useful in the healthcare organization management, firstly it is necessary to validate their quality, and secondly, find the right correlations. In other words, the data should be processed, analyzed, and interpreted with the appropriate tools [ 4 , 5 ].
Technological applications in healthcare BDA-related are rapidly increasing [ 6 ] and will increasingly characterize managers’ decision-making process. For example, IBM’s Watson project [ 7 ] is a "super-computer" that has scoured through several million scientific articles over the last twenty years and uses artificial intelligence tools (e.g., Machine Learning) to correlate disease symptoms and predict possible diagnostic scenarios. This case helps to understand how and to what extent BDA could really support healthcare managers to improve their decision processes, while increasing the performance of the healthcare organization.
Nowadays, the amount of data is no longer an issue. Internet traffic reports from Cisco and other network operators have estimated the entire digital universe to be 44 zettabytes and 463 exabytes will be the daily information could be generated by 2025. A new era took place in which the processes of production and management of human knowledge will no longer be the exclusive preserve of humans; machines will also play their part as knowledge producers [ 8 ]. From pharmaceutical companies to healthcare organizations, this enormous potential of data products, combined with IoT applications and AI tools [ 9 , 10 , 11 ], will play a significant role in the near future. Today, the medical applications based on IoT allow the monitoring of clinical data through the production of data generated by special devices (e.g., wearable devices) [ 12 ], remotely accessible by a physician rather than by caregivers [ 13 ].
The market size is a useful indicator of how much the healthcare organizations are turning their attention to new management models based on the use of big data. By 2025, the big data market in healthcare will touch $70 billion with a record 568% growth in 10 years. The use of such a tool not only represents a complex challenge [ 14 ], but also opens opportunities for all those involved in the healthcare supply chain who manage decision-making processes. Moreover, if on the one hand this technology will influence the definition of new managerial strategies within healthcare organizations, on the other hand, it will have positive repercussions on the effectiveness and efficiency of healthcare processes [ 15 ]. Indeed, the big data technology is used by healthcare managers to get, for example, information related to the list of doctors and nurses, the list of drugs with their expiration date, etc., in order to have tools for facilitating decision-making processes, improving the quality of services provided, and, at the same time, rationalizing the use of resources, by facilitating the management of the healthcare organization as a whole.
The BDA satisfies multiple needs that, on the one hand, influence the quality of the healthcare organization’s performance and, on the other hand, are useful in directing management strategies to improve the supply of healthcare services. Below there are some strategies, which aim to:
Provide specific services to patients, from diagnostics to preventive medicine passing through therapeutic adherence.
Detect the onset and spread of diseases in advance.
Observe parameters inherent to hospital quality standards, promoting control and prevention actions.
Modify treatment techniques.
Facilitate research and development in pharmacology, reducing the time to market of drugs.
Facilitate research and development of new and specific medical devices.
The main aim of this research is, therefore, to provide both an integrative framework on the state of art, and perspectives on how the BDA can be useful for the management of the healthcare organization. Considering the results, food-for-thought on how this technological and cultural revolution will affect the modus operandi of healthcare organizations will be launched.
Through an overview of recent scientific studies, this research aims to raise awareness among both practitioners and managers about BDA tools applied to healthcare management to address more effectively and efficiently the challenges imposed by an increasing demand for healthcare services.
In this regard, the study provides a systematic literature review (SLR) to explore the effect of BDA on the healthcare management by analyzing articles from the Scopus database during a period of 5 years (2016 – 2021).
Furthermore, the result through a content analysis, aspires to be a privileged starting point to find out potential barriers and opportunities provided by BDA-based management systems for smarter healthcare organization. Specifically, the study answers different research questions (RQs) as different levels of analysis have been performed. By analyzing the relationship between BDA-based management systems and the benefits delivered to the organizations, the research could not be conducted without exploring the state of art of BDA tools deployed in the field of healthcare. Thus, starting from this background the discussion on the future perspectives on BDA development in the healthcare organizations appears as a need.
Theoretical framework
Why use BDA and how to exploit its potential for healthcare organization management? This is the main question asked by managers and decision makers working in the healthcare sector. In recent years there have been multiple attempts in the literature aimed at highlighting the relationship between implementation of BDA and benefits for healthcare organizations, in terms of both resource efficiency and process management.
In 2017, a study by Wang and Hajli [ 16 ] has proposed a model founded on Resource-Based Theory and BDA Capabilities (BDAC) to explain the relationship between BDA, benefits, and value creation for healthcare organizations. As stated by Srinivasan and Swink [ 17 ], BDAC refers to “ organizational facility with tools, techniques, and processes that enable a firm to process, organize, visualize, and analyze data, thereby producing insights that enable data-driven operational planning, decision-making, and execution ”. In the healthcare organization, BDAC represents the ability to collect, store, analyze, and process huge volume variety, and velocity of health data come from various sources to improve data-driven decisions [ 18 , 19 ]. Indeed, the study of Wang and Hajli [ 16 ], validated on an empirical basis by 109 cases of BDA tools implementation in 63 healthcare organizations, has demonstrated how specific "path-to-value" can be identified. By varying degrees of relevance of the identified pathways, it has been shown that alongside the challenges of implementing certain BDA tools, there are corresponding specific benefits for healthcare organizations. Preliminarily, the study has defined the ability to analyze big data through the concept of Information Lifecycle Management (ILM) [ 20 ]. In this perspective, the capabilities of the BDA in healthcare organizations are configured as the abilities to process health data from diverse sources and provide significant information to healthcare managers. Thorough BDA, managers can detect timely indicators and identify business strategies, which allow them to put in place perspective plans, efficient strategies, and programs to increase the performance of organizations.
Researchers have found that BDA capabilities primarily stem from the implementation of various tools and features. Specifically, in order of importance, BDA capabilities are firstly triggered by processing tools (e.g., OLAP, machine learning, NLP), followed by aggregation tools (e.g., data warehouse tools), and, secondly, by data visualization tools and capabilities (e.g., visual dashboards/systems, reporting systems/interfaces).
Among the potentials triggered by the implementation of BDA in the healthcare organization, the analytical one was the main capability, that is the ability to process clinical data characterized by immense volume, variety (from text to graph), and speed (from batch to streaming), using descriptive analysis techniques [ 21 , 22 ]. In this regard, it is important to note that BDA-based management systems are the only ones capable of analyzing semi-structured or unstructured data. This represents a crucial element for revealing correlation patterns that are difficult to determine with traditional management systems [ 23 ]. Furthermore, the launch of these systems in a healthcare organization ensures the ability to effectively manage outputs regarding care process and service in order to constantly improve the performance of the organization. In summary, the characteristics of BDA-based management systems implemented in a healthcare organization, are:
predictive analytics capability, i.e., the ability to explore data and identify useful correlations, patterns and trends, and extrapolate them to predict what is likely to occur in the future [ 24 , 25 ];
interoperability capability, i.e., the ability to integrate data and processes to support management, collaboration, and sharing across different healthcare departments, managers, and facilities [ 26 ], and finally,
traceability capability, i.e., the ability to integrate and track all patient history data from different IT facilities and different healthcare units.
In terms of expected benefits from the BDA implementation, the study of Wang and Hajli [ 16 ] has showed that the most important ones are obtained from improved operational activities, such as improved quality and accuracy of healthcare decisions, rapid processing of issues, and the ability to enable treatments proactively before patients’ conditions worsen. Next, in terms of relevance, they were the benefits related to IT infrastructure, such as standardization and reduced costs for redundant infrastructure and the ability to quickly transfer data between different IT systems. Substantially, they have delivered a useful business model that healthcare managers can draw on to evaluate the specific leverages they need to activate in relation to the implementation of the BDA-based management systems. In addition to highlighting the undoubted benefits, the authors clearly show how specific BDA tools can facilitate the decision-making processes of healthcare managers and make them faster and more effective.
In another study carried out to identify BDA benefits and supports, and to drive organizational strategies, Wang, Kung, and Byrd [ 19 ], through the analysis of 26 case studies related to the BDA applications in the healthcare organization, have identified five "capabilities" of BDA: analytic capability for care patterns, unstructured data analytical capability, decision support, predictive, and traceability capabilities [ 19 ]. The study is remarkably interesting because in addition to mapping precise benefits, it also recommends specific strategies considering the BDA implementation for healthcare organizations. These strategies are useful for achieving effective results by leveraging the potential of BDA.
The first successful strategy is to implement governance based on the use of big data, starting with a definition of objectives, procedures, and key performance indicators (KPIs). Once again, one of the discriminating factors for success in implementing such a strategy remains the integration of information systems and the standardization of data protocols that often come from heterogeneous sources already existing in healthcare organizations. The second strategy is related to developing a culture of data sharing. The third one considers the training of healthcare managers, who cannot ignore knowledge related to BDA, for example on the use of data mining and business intelligence tools. The fourth strategy is related to the storage of big data, often available in heterogeneous formats, and is identified in the transition from the more expensive traditional storage systems (NAS) to more efficient and effective systems such as cloud computing solutions. The last strategic driver involves pathways related to the implementation of predictive BDA models. The mastery of KPIs, interactive visualization and data aggregation tools such as dashboards and reports should be acquired instruments for healthcare managers and in general for healthcare organizations oriented to BDA driven process management strategies.
More recent studies focus attention on the management practices supply chain in healthcare. In the study performed by Yu et al. [ 27 ], the authors, interviewing senior executives in Chinese hospitals, show on both a theoretical and empirical basis, how BDAC positively impacts the three dimensions of hospital supply chain integration (SCI) (inter-functional integration, hospital-patient integration and hospital-supplier integration) and how SCI, in turn, contributes to improve the operational flexibility [ 27 ]. By “operational flexibility” in the healthcare organization, it is meant the ability of a ward to adapt its operating procedures in relation to unforeseen circumstances while meeting the needs of patients [ 28 , 29 ].
The scholars have delivered an important contribution in demonstrating the relationship between BDAC, SCI, and operational flexibility from multiple perspectives, by providing useful management guidance for healthcare executives and managers involved in the supply chain. By analyzing and processing medical and managerial data with advanced analytical techniques, Chinese healthcare organizations were able to facilitate decision-making process with timely and appropriate actions, for example, tracking people's movements during the lockdown caused by the Coronavirus, understanding ongoing health trends, and managing pharmaceutical supplies [ 30 , 31 ].
This theoretical framework provides a key to interpreting the benefits offered by good practices deriving from the use of the BDA in the healthcare organization.
At the same time, the rigorous scientific method allows the validation of empirical experiences in relation to clear theoretical references. In the next paragraph projects that demonstrate what is stated in the literature are shown.
Practical framework
N(ursing) + Care App is an mHealth application that supports the work of frontline health workers (FHW) in developing countries [ 32 ]. The system is designed to collect not only patient data, but also diagnostic images. It is also given the opportunity to add recommended doctors based on the advice of FHWs in case the patient needs to follow a specific hospital visit.
For healthcare managers, predicting the number of emergency department accesses is a critical issue which complicates the optimization of the human resource management. To this end, Intel, and Assistance Publique-Hôpitaux de Paris (AP-HP), the largest hospital university in Europe, leveraging datasets from multiple sources, worked together to build a cloud-based solution to predict the number of patient visits to emergency rooms and hospital admissions. This predictive analytics tool, will enable healthcare managers at AP-HP hospitals to know the number of emergency room visits and hospital admissions at 15 days in order to reduce wait times, optimize human resource (HR) levels based on anticipated needs, accurately plan patient loads, including by pathology, and overall improve the quality and efficiency of services provided by the healthcare organization [ 33 ].
Chronic conditions, if not kept under control through a rigorous program of therapeutic adherence, can become a source of both more serious physical problems for patients and economic burdens for healthcare organizations. Another project that actively introduced BDA tools into healthcare management was carried out by the European Commission to launch production of the drug Enerzair Breezhaler . It was the first drug for the treatment of asthma co-packaged and co-prescribed with the Propeller digital platform. The app sends a reminder to comply with therapeutic adherence and maintains a record of the data, which the patient shares with him or her physician. Studies have demonstrated that the Propeller platform increases the degree of asthma control by up to 63%, therapeutic adherence by up to 58% [ 34 ], and reduces asthma emergency department visits and hospital admissions by up to 57% [ 35 ].
The practical framework described, aided by some empirical experience, only partially reveals the potential offered by BDA. The diffusion of BDA-based management systems in the healthcare organization will trigger a virtuous circle, allowing soon to accumulate increasingly accurate medical data. By exploiting the most advanced AI technologies, BDA will support predictive analysis, allow physicians to make more accurate and faster diagnostic pathways and managers to use results. It will help health practitioners in the decision-making process, optimize the use of resources with a consequent costs reduction and, overall, improve the quality of services provided by healthcare organizations.
The main aim of this study is to update the state of art about the BDA-based management systems adopted in the healthcare organization, underlining management advantages for both the organizations and managers. BDA has the potential to reduce the cost of care, prevent disease outbreaks, and improve the patients’ quality of life. Through its ability to process and cross-reference massive amounts of both management, and clinical information, BDA promises to be an effective support tool for both healthcare managers and patients.
To achieve this aim, a Systematic Literature Review (SLR) was performed. This method identifies, evaluates, and summarizes the updates that raise from the literature about the BDA tools used to improve both the healthcare organizations performance and patients’ quality of life. The method takes inspiration from the protocol used by Khanra S., et al. [ 36 ] which considers inclusion and exclusion criteria.
The present study aims to add a contribute to the literature by addressing three RQs:
What is the state of art of BDA adopted by healthcare organizations?
What about the benefits for both health managers and healthcare organization?
What about future directions on BDA research in healthcare?
To answer the RQs, as widespread electronic database Scopus has been selected. To obtain an international validity of studies, the research only considers papers in English. Utilizing the Boolean operator “AND”, the following keywords have been searched: “big data analytics” AND “healthcare” AND “management”. As inclusion criteria, only papers published from 2016 to 2021 have been considered. As subject areas, “medicine” and “business, management and accounting” have been selected. Instead, as exclusion criteria, article in press and the following documents type: “review”, “book”, “conference review”, “letter” and “note” have not been taken into account. Also, to avoid a dispersal of the study, conference proceedings have been excluded. Following the searching protocol, 34 results have been obtained (Fig. 1 ).
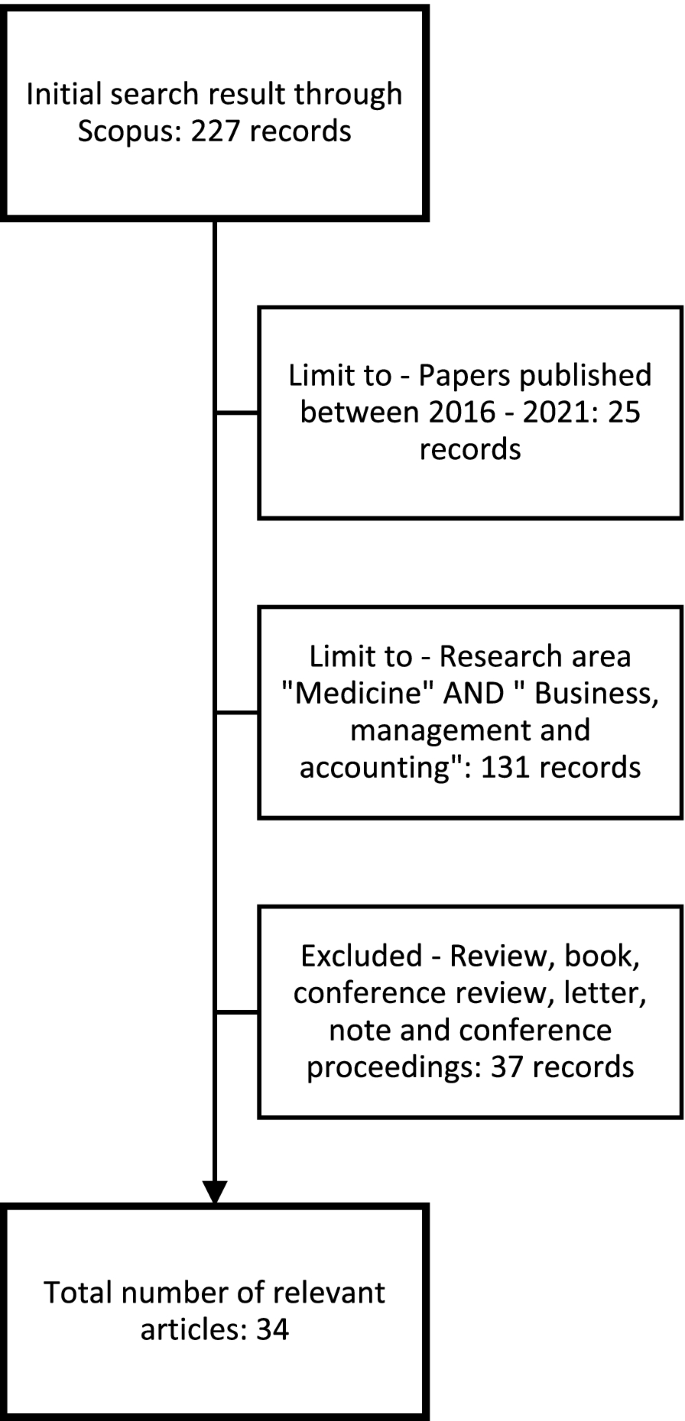
Workflow of articles selection
An excel spreadsheet was used to perform the extraction procedures while the statistical analyses were carried out using the software STATA 16 ©. The list of the extracted papers investigated with the content analysis can be found in the Appendix.
The work proceeds through a descriptive analysis. After that, a content analysis has been performed to identify the most relevant characteristics of the BDA-based management systems, underlining the positive impact for the healthcare organizations, without neglecting to outline the trends for the future scenarios and research directions.
According to the SLR, the iterative process shown in the Fig. 1 , has allowed to delete the duplicates and match the results with the RQs.
As shown in Fig. 1 the initial search on Scopus database has delivered 227 results. By limiting research to papers published between 2016 and 2021, 11% of records have been removed. At the second stage, by selecting the subject areas, the screening has allowed to exclude 131 records; thus, the 57.7% of the results initially selected. The last step of the process has conducted to exclude document types such as Review, Book, Conference Review, Letter, and Note. In other words, 37 records were excluded, representing 16.3% of the sample. At the end of the screening process, 34 articles were selected, representing about 15% of the sample.
In the descriptive analysis the time distribution of the studies from 2016 to 2021 is included. It is important to note the increasing of publication trend from 2017 to 2019. This output confirms a growing interest in the research field of BDA applied to healthcare organizations (Fig. 2 ).
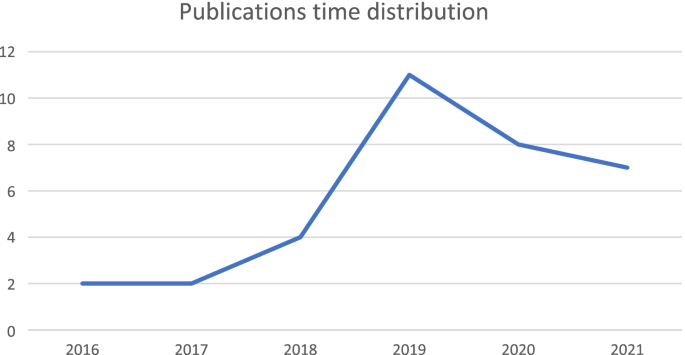
Trend of research steams
The trend of research steams considers a sample of 34 scientific contributions as they come from the screening process above described. Although 6% of the total sample was collected in the years 2016 and 2017, it is only indicative of the growing trend of scientific studies on BDA in healthcare sector. The overall incidence in 2018 was 12% but the turning point was reached in 2019 as 32% of the studies collected in the sample were reached. This outcome could be read considering the Covid-19 pandemic outbreak which has been a representative testing ground for BDA tools by helping managers and decision-makers to plan healthcare managerial strategies.
In this context, the use of the BDA by Chinese healthcare organizations for tracking people's flow during the lockdown, represents an important case study that has registered the peak in the time flow of research. By looking at 2020 and 2021 data, which represent respectively 24% and 21% of the total scientific contributions, the growing trend seems to be confirmed by validating the rising interest in BDA research seen as a planning tool for healthcare processes.
The pie-chart shows the scientific production by country. It is necessary to specify that Scopus database clusters the studies by home country author’s organization, therefore the same study could be referred to more than one country and thus belong to more than one cluster.
The geographical locations of the studies showed in the Fig. 3 outlining India, UK, and USA as more than one third of the total scientific producers. It is well known that IT companies as Google, Apple, Amazon, and Microsoft are investing considerable resources on BDA tools for healthcare. China and India contribute together with 22% of the scientific articles. Big data technology has played a key role in virus tracking during the pandemic crisis. The "Internet Plus Healthcare", a big data center in Zhongwei (China), provides cloud services to both healthcare institutions and IT companies. In Yinchuan (China), an industrial park for big data acts as a catalyst for IT company involved in healthcare sector. India confirms to be one of the heavily adopter countries of artificial intelligence, big data analytics, and IoT technologies. Although India must face the challenge to provide basic healthcare services in a predominantly rural country, start-ups with BDA skills in healthcare are springing up.
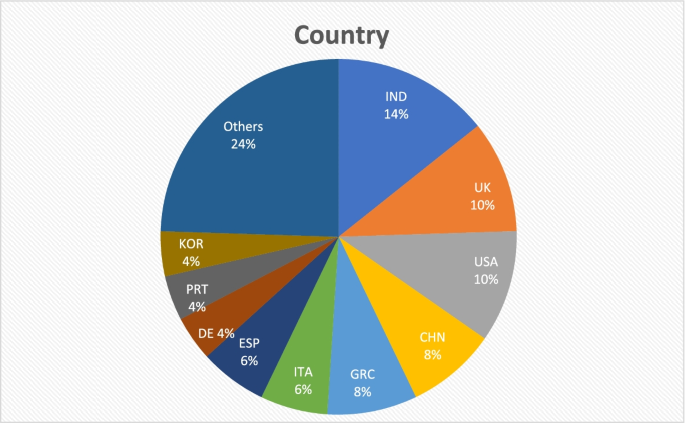
Geographical locations of the studies
It is also important underlining the performance of the European countries. UK, Greece, Italy, Spain, Germany, and Portugal support the research with almost 40% of the studies published, confirming that Europe will be a driving force for the BDA research in the next future. The development of a European Health Data Space (EHDS) is an ambitious project of the European Commission. It will lead member states to share an efficient infrastructure for both exchange and management health data by providing citizens with equal treatment, free access to clinical data, and quality healthcare services.
In the area “Others” all the other countries contributing marginally to research have been included.
The next step of the study is focused on a content analysis to show the experiences of applying BDA in healthcare organizations.
Starting from the 34 articles selected for the descriptive analysis, to identify in detail the core issue of the study, a second screening was performed. 18 articles were excluded because weakly focused on the research objective which concerns specifically how BDA can be used for healthcare organization management. Thus, after an in-depth reading of abstracts and full papers, the scholars have identified 16 papers closer targeted on the mentioned research objective. The 16 studies selected through a content analysis were clustered into 4 research areas (RAs) as showed in the following table (Table 1 ). The clustering procedure identifies 4 relevant topics: Potentialities of BDA (RA1), Resource management (RA2), BDA and management of health surveillance system (RA3), BDA technology for healthcare organization (RA4). The proposed clustering has been though to give an easy-to-go research map and to support the healthcare managers.
RA1: potentialities of BDA
Wang and Hajli [ 16 ] define BDA potentialities in the healthcare context as “ the ability to acquire, store, process and analyze large amounts of health data in various forms, and deliver meaningful information to users, which allows them to discover business values and insights in a timely fashion ”. The relationship between BDA and the benefits for the healthcare organizations it has been well expressed by the theory of the “path to value chain” [ 16 ]. This path represents an important contribution to the exploration of business value, not only for drawing the generic and well-established connection between big data capabilities [ 19 ] and the benefits, but also for empirically showing how capabilities can be developed and what benefits can be achieved in the healthcare organizations. Another study included in this area, explores the key role of BDA capabilities in developing healthcare supply chain integrations and its impact on hospital flexibility [ 27 ]. Specifically, the BDA has a fundamental role in developing healthcare integration supply chain and the operational flexibility. Considering the health and economic crises caused by the Covid-19, this dimension of BDA has been an especially important leverage for managers to improve operational flexibility of the healthcare organizations. The ability to provide predictive models and real-time insights, is a powerful prospective of the BDA for helping healthcare professionals and managers in decision-making process. In this regard, the literature presents several applications of big data in healthcare that support the data collection, management, and integration of data in healthcare organizations [ 37 ]. Moreover, BDA enables the integration of massive datasets, supporting decisions of manager and monitoring the managerial aspects of healthcare organizations. Building a decision-making process based on BDA, firstly means identifying the big data keys that can implement ad-hoc strategies to improve efficiency along the healthcare value chain. To this end, the research carried out by Sousa et al., [ 37 ] underlines the benefits that BDA can give to the decision-making process, through predictive models and real-time analytics, assisting in the collection, management, and integration of data in healthcare organizations.
To date, thanks to an integrated and interconnected ecosystem, is becoming possible to provide personalized healthcare services, collect an enormous quantity of both clinical and biometrics data and, thus, implement BDA instruments. Nevertheless, to take a real advantage from these tools and turn them into useful decision support systems (DSS), is necessary for R&D to be focused on data filtering mechanisms in order to obtain good-quality reliable information [ 38 ]. The healthcare models based on BDA and implementation of new healthcare programs, enable both medical and managerial decision support for the healthcare services provision. New types of interactions with and among users of the healthcare ecosystem will produce in the next future a wide variety of complex data, thus, the main challenges refer to information processing and analytics.
In light of the above, the RA1 includes studies for which the quality of data and the need for high performance filtering mechanisms are becoming keys factor for the success of BDA-based management systems in the healthcare organizations. For example, the study carried out by Maglaveras et al., [ 38 ], included in this area, explores new R&D pathways in biomedical information processing and management, as well as to the design of new intelligent decision support systems.
RA2: resource management
Another important research direction emerged from the literature review, concerns positive impact of the BDA on the resource management. Insufficient policy for managing medical materials waste, energy use and environmental burden, restricts the resources conservation. The BDA is extremely useful in this aspect; it could provide in the next future an important contribution to implement the circular economy processes and to support sustainable development initiatives in the healthcare organizations [ 39 ]. To this end, the study developed by Kazançoğlu et al. [ 39 ], underline the importance of circularity and sustainability concepts to mitigate the sector’s negative impacts on the environment. Furthermore, the study identifies the barriers related to circular economy in the healthcare organization and provides solutions to these barriers by implementing BDA-based management systems. Lastly, the authors, have developed a managerial, policy and theoretical framework to support healthcare managers to launch sustainable initiatives in the context of healthcare organization.
The impact on the performance has been also investigated by studies that have linked benefits of BDA and artificial intelligence with green supply chain integration process [ 40 ]. Digital learning is more becoming a “moderator” of the green supply chain process with a significant positive impact on environmental performance of the healthcare organization. BDA-AI technologies will lead to improvement of the environmental process integration and green supply chain collaboration and, consequently, will support the managers’ decisions involved in the supply processes. This study also provides an important reference framework for logistics/supply chain managers who want to implement BDA-AI technologies for supporting green supply processes and enhancing environmental performance of the healthcare organization [ 40 ].
Nowadays, many scholars are focusing on BDA-driven decision support systems to sustain the healthcare managers [ 41 ]. These types of BDA-based analytical tools will provide a useful quantitative support for managers of healthcare organizations. The authors have reported design and technical details of the system implementations using case studies. They have developed a toolkit which represents a framework reference for resources management, allowing to create strategic models and obtain analytical results for evidence-based decisions and managerial evaluations.
In this RA, two other important topics investigated by BDA are: high quality healthcare service, and healthcare costs. Optimize the supply chain activities is an imperative to keep lower the healthcare costs. The data generated by medical equipment and devices can be successfully used in forecasting, decision-making process, and to make more efficient the healthcare supply chain management [ 42 ]. The study carried out by Alotaibi et al. [ 42 ], thus, presents a review on the use of big data in healthcare organizations underling opportunities and challenges deriving from the application of BDA-based management systems within the organizations.
As already asserted, a good implementation of BDA in the healthcare organization will play a fundamental role in improving the clinical outcomes management, giving helpful insights for decision makers and managers, in order to avoiding diseases, reducing healthcare expenses, and improving the performance of the healthcare organization [ 43 ]. However, to achieve these ambitious outcomes the research will face a crucial challenge: how to rationalize, make easily usable, and at affordable costs, heterogeneous data coming from diverse sources. The research developed by Kundella and Gobinath [ 43 ] represents an important contribute to explore key challenges, techniques, technologies, privacy issues, security algorithms and future directions of the use of BDA in the healthcare organization.
RA3: BDA and management of health surveillance system
The rise of BDA promises to solve many healthcare challenges in the developing countries. The BDA applied to healthcare organization help managers to rationalize the resources, and health system to better delivery treatments to the patients [ 44 ]. In this regard, the government of Zambia is thinking to implement BDA solutions to provide more effective and efficient healthcare services. A well-managed health surveillance system represents an important driver to improve the quality of life and reduce the medical waste, especially in developing countries where the lack of resources is severe and limits economic development. For all these reasons, Europe is investing on BDA initiatives in public health and in the oncology sectors, to generate new knowledge, improve clinical care and make more efficient the management of the public health surveillance system [ 45 ]. The BDA capability for identifying specific population pattern, managing high volume of data and turn it into real (or near real) time insights, contributes to identify it as a powerful tool to support the managers for the decision-making processes. Despite this, implementing a BDA-based management systems within the healthcare organizations requires investment in the human capital, strong collaboration with stakeholders, and data integration with and among the healthcare units. To this end, Gunapal et al., [ 46 ] has highlighted that Singapore has setup a Regional Health System (RHS) database to facilitate BDA for proactive population health management (PHM) and health services research [ 46 ]. The structure of the healthcare database has been built collecting data from four database coming from three RHSs: National Healthcare Group (NHG), Tan Tock Seng Hospital (TTSH), National University Hospital (NUH) and Alexandra Hospital (AH). The result has been a database including information useful for the healthcare managers which incorporates data on patient demographics, chronic disease, and healthcare utilization information. These characteristics facilitate the identification of specific patients’ paths linked by past healthcare utilization and chronic disease information. Converging information into a single database helps to understand the cross-utilization of healthcare services across the three RHSs. A such approach allows to setup the RHSs structure for initiative-taking population health management (PHM) and to improve the performance of healthcare organizations [ 46 ].
RA 4: BDA technology for healthcare organization
The wearable devices and different kind of sensors, able to collect clinical data, in combination with BDA, will constitute the basis of personalized medicine and will be crucial tools to improve the performance of healthcare organizations [ 47 ]. The scientific research has to face the important challenge to adapt data acquisition, storage, transmission and analytics to healthcare demand. Thus, the healthcare data should be categorized, homogenized, and implemented into specific models by adapting machine-learning techniques to the nature of the healthcare organization.
A fruitful field of interest for the application of BDA in healthcare organization is the diagnostic imaging. To take out maximum benefits from it and to be useful for managers of healthcare organizations, it is necessary to implement digital platforms and applications [ 48 ]. Indeed, the simple production of a large amount of data does not automatically translate to an advantage for the healthcare performance. Specific applications are required to favor the correct and advantageous management of diagnostic images [ 48 ]. The link between BDA and IoT technologies, as instrument to incorporate the accessibility, capacity to customize, and practical conveyance of clinical data, emerged as another research direction investigated by the papers included in this RA. These tools allow: (1) the healthcare organizations to decrease expenses; (2) the people to self regulates treatments; (3) practitioners to take as quickly as possible decisions in remote way and keep constant contact with patients [ 49 ].
In light of these results, it is possible to state that IoT, big data, and artificial intelligence as machine-learning algorithms, are three of the most significative innovations in the healthcare organization. These types of organizations are implementing home-centric data collection networks and intelligent BDA systems based on machine learning technologies. For example, a high-level implementation of these systems has been efficiently implemented in Cartagena, Colombia, for hypertensive patients by using an e-Health sensor and Amazon Web Services components [ 50 ]. The authors stress the importance of using the combination of IoT, big data, and artificial intelligence as tools to obtain better health outcomes for the communities and improved performance for healthcare organization. The new generation of machine-learning algorithms can use standardized data sets generated by these sources to improve the effectiveness of public health interventions [ 50 ]. To this end, as pointed out by numerous studies in the field of BDA applied on healthcare organizations, it becomes crucial for the next future research to concentrate R&D efforts towards full standardized dataset protocols.
As highlighted by the results, in Europe, as well as in the rest of the world, a significant trend is emerging among healthcare organizations in adopting BDA-based management systems [ 45 ]. Among the clustering process performed, the common element in the studies reviewed is the positive relationship between BDA tools and achievable benefits for healthcare organizations.
As emerged by the RAs, some studies explore business value for healthcare organizations and the concept of potentialities of BDA (RA1) to explain the evidence of precise path-to-value chains leading to specific benefits [ 16 ]. These perspectives provide useful guidelines for healthcare managers who want to consider implementing BDA tools in their organizations. Some authors in particular focus on the role of BDA capabilities in the development of hospital supply chain integration and operational flexibility, demonstrating a positive relationship between the two dimensions [ 27 ]. During the Covid-19 outbreak, it became clearer how important operational flexibility is to healthcare organizations. The scholars also underline how BDA can impact to the efficiency of the decision-making processes in healthcare organizations, through predictive models and real-time analytics, helping health professionals in the collection, management, and analysis [ 37 ].
In general, BDA-based management systems make personalized care programs possible. However, considering the enormous amount and heterogeneity of information available nowadays, it emerges the necessity to address R&D pathways towards data filtering mechanisms and engineering new intelligent decision support systems within the healthcare organizations [ 38 ].
Circular economy (CE) and sustainability concepts are becoming important key drivers in healthcare organizations to reduce negative impact on the environment (RA2). Some study directions look at BDA as tool to provide solution for barriers related to CE and support sustainable development initiatives in the healthcare organizations [ 39 ]. Empirical studies have demonstrated the benefits of BDA-AI in the supply chain integration process and its impact on environmental performance. By assessing a sample of 168 French hospitals, Benzidia et al. [ 40 ], has observed that the use of BDA-AI technologies has a significant impact on environmental process integration and green supply chain. In particular, this study provides important insights for healthcare managers, who wish to implement BDA-AI technologies for sustaining green supply processes and improving environmental performance [ 40 ]. BDA and web technologies can successfully help managers to redesign healthcare processes making them more effective and efficient. Since healthcare spending is constantly growing in the world’s major regions, there is urgent need to redesign processes optimizing supply chain activities such that high-quality services could be provided at lower costs [ 42 ]. Although BDA-based management systems promise to fulfil this role in the healthcare organization, more in-depth studies are required. Due to heterogeneity of information sources, one of future research direction should deeply investigate the protocol standardization and integration in data analyzing as well as techniques and technologies used, security algorithms of BDA in the healthcare and medical data [ 43 ].
In developing countries, as well as in the rest of the world, the management of health surveillance is a sensitive issue (RA3). Therefore, authors have studied main key factors that hind BDA access in the healthcare organization [ 44 ]. Technology, staff, data management and health policies have been identified as some of decisive variables [ 44 ]. Due to increasing of the ageing population and the related disability, healthcare organizations will face hard challenges soon. To this end, big data can also help healthcare managers to detect patterns and to turn high volumes of data into usable knowledges. In this context investments in technological infrastructures are needed as well as in the human capital [ 45 ]. China is proving, with a large scale of investment, to be a pioneer country in the adoption of BDA-based management systems in the healthcare organization [ 46 ].
The rising of AI, IoT, machine learning [ 49 , 50 , 51 ], and sensors technology, as well as embedded systems able to communicate each other, have boosted the adoption of BDA with valuable benefits for the healthcare organization (RA4). These technologies will play a fundamental role on big data management to improve the performances of the healthcare organizations. Some authors have underlined privacy issues related to healthcare data and the necessity to make sensor data homogeneous and tagged. Furthermore, implementation of clinical records into models and adaptation of machine-learning techniques is required [ 47 ]. Future R&D in this field should be focused on the developing of digital platforms and specific applications based on BDA also for managing diagnostic images [ 48 ].
By exploring the relationship between BDA-based management systems and the benefits delivered to the healthcare organizations, this study replies to 3 RQs: 1) What is the state of art of BDA adopted by healthcare organizations, 2) What are the benefits for both health managers and healthcare organizations and 3) What are the future directions on BDA research in healthcare.
To answer the RQs the SLR has started from an investigation on the recent literature BDA about the BDA in healthcare organizations. Descriptive analysis has been performed on a sample of 34 studies coming from all over the world. The second stage shows a detailed content analysis on 16 studies which better answer to research question about the relationship between benefits for the healthcare organization and BDA solutions.
By analyzing the successful BDA strategies in healthcare context, some authors focus their attention on the BDA potentialities applied in the healthcare organizations [ 16 , 37 ]. Indeed, the research highlights how analytical tools through personal health systems support public health management systems and how BDA suggests new pathways to support healthcare managers in decision-making process.
In the literature, other scholars highlight the positive impact of BDA on resource management. The BDA solutions are analyzed as tools to sustain CE initiatives [ 38 , 39 ] as well as to enable green supply chain process integration and improve hospital performance [ 40 ]. By exploiting KPIs coming from BDA solutions, some researchers present innovative models for planning public health policy [ 41 ]. In this context, the studies consider BDA cloud computing solutions and social media data analytics for supporting the performance of healthcare supply chain management [ 42 , 43 ]. Furthermore, researchers from all around the world are showing particular interest on BDA for health surveillance system management [ 44 , 45 , 46 ].
According to the recent literature, BDA is transforming the healthcare organizations. The SLR has showed how the BDA solutions are now quite considered a milestone for managerial studies applied to healthcare organizations. The Coronavirus pandemic has been a good test run for using BDA to design healthcare policy strategies. Although an extensive literature on BDA to support healthcare management is being produced, the classification into four RAs proposed is an attempt to examine precise key research directions. About that, the limitations of the present research can be detected as the difficulty to review a field of literature constantly evolving. To date, the amount of data is no longer an issue. To be useful in the healthcare context, is necessary to validate their quality and then find the right correlations. In other words, the data should be processed, analyzed, and interpreted correctly. For this reason, emerges the need to address research pathways towards filtering mechanisms, by converting data from big to smart, and engineering new decision support systems within the healthcare organizations [ 38 ].
The content analysis carried out in this research has shown that studies are addressed to find out new models for both predictive and personalized medicine by exploiting BDA technologies [ 47 ]. The researchers underline the added value of using BDA both in the medical diagnostic process [ 48 ] and jointly with IT technologies such as IOT and machine learning [ 49 , 51 ].
Thus, considering the results obtained, it is possible to state that BDA can effectively help healthcare managers to detect common patterns and turn high volumes of data into usable knowledges. Investments on human capital become a priority to exploit the potential of BDA [ 45 ].
To achieve these objectives the future research should provide usable insights and standardized procedures for training healthcare managers and practitioners. AI, machines learning, as well as management strategies, will also play their part as knowledge producers in the healthcare organization. Privacy issues related to healthcare data and also the necessity to make sensor data homogeneous, are becoming crucial research topics to be faced. Finally, due to the heterogeneity of information sources, the future direction of research should investigate the standardization and integration of the protocol in data analysis, as well as the techniques useful for the managerial sector to implement increasingly BDA-based management systems in future healthcare organizations [ 43 ].
Nowadays the challenge for healthcare organizations is the development of useful applications BDA-based. According with the circular economy view, the future research directions should be addressed considering the relationship between digitalization and management resources consumption. The data centralization combined with a BDA approach can effectively support circular economy processes in healthcare supply chain by reducing waste and resource consumptions.
Exploiting the BDA’s capabilities will also be a key factor in forecasting and monitoring outbreaks. Future studies will need to focus on developing more efficient models for sharing data in order to improve the performance of healthcare organizations around the world.
Availability of data and materials
The datasets analyzed during the current study are not publicly available due to data relating to scientific journal names and authors but are available from the corresponding author on reasonable request.
Wang L, Alexander CA. Big data in medical applications and health care. Curr Res Med. 2015;6:1–8.
Article Google Scholar
Aceto G, Persico V, Pescape A. Industry 4.0 and health: internet of things, big data, and cloud computing for healthcare 4.0. J Ind Inf Integr. 2020;18:100129.
Google Scholar
Galetsi P, Katsaliaki K, Kumar S. Values, challenges and future directions of big data analytics in healthcare: A systematic review. Soc Sci Med. 2019;241:112533.
Article CAS PubMed Google Scholar
Obermeyer Z, Emanuel EJ. Predicting the future — big data, machine learning, and clinical medicine. New Engl J Med. 2016;375:1216–9.
Article PubMed Google Scholar
Kumar Y, Sood K, Kaul S, Vasuja R, et al. Big data analytics and its benefits in healthcare. In: Kulkarni J, et al., editors. Big data analytics in healthcare, studies in big data 66. Cham: Springer; 2020. p. 3–21.
Raghupati W, Raghupathi V. Big data analytics in healthcare: promise and potential. Health Inf Sci Syst Vol. 2014;2(1):1–10.
Jain DA, Kumar V, Khanduja D, Sharma K, Bateja R. A detailed study of big data in healthcare: case study of Brenda and IBM Watson. Int J Recent Technol Eng. 2019;7:8–12.
Tremolada, L. (2019), “Quanti dati sono generati in un giorno?” Il Sole24Ore , May 26, 2019, available at: https://www.infodata.ilsole24ore.com/2019/05/14/quanti-dati-sono-generati-in-un-giorno/?refresh_ce=1 (Accessed 17 Feb 2022).
Srivastava P.K., Rakshit P. Cutting edge IoT Technology for Smart Indian Pharma. In: International Conference on Advance Computing and Innovative Technologies in Engineering, (ICACITE) 2021. Greater Noida: Institute of Electrical and Electronics Engineers Inc.; 2021. p. 360–2.
Rayan R.A, Tsagkaris C, Zafar I. IoT for better mobile health applications. In: Kumar P, editor. A fusion of artificial intelligence and internet of things for emerging cyber systemsand internet of things for emerging cyber systems. Cham: Springer; 2022. p. 1–13.
Chung K, Park RC. Chatbot-based healthcare service with a knowledge base for cloud computing. Cluster Comput. 2019;22:1925–37.
Ali F, El-Sappagh S, Islam SMR, Ali A, Attique M, Imran M, Kwak KS. An intelligent healthcare monitoring framework using wearable sensors and social networking data. Fut Generation Comput Syst. 2021;114:23–43.
Yousefi S, Derakhshan F, Karimipour H. Applications of big data analytics and machine learning in the internet of things. In: Choo KK, Dehghantanha A, editors. Handbook of big data privacy. Cham: Springer; 2020. p. 77–108.
Chapter Google Scholar
Mehta N, Pandit A, Kulkarni M. Elements of healthcare big data analytics. In: Big data analytics in healthcare, studies in big data 66. Cham: Springer; 2018.
Han Y, Lie RK, Guo R. The internet hospital as a telehealth model in China: systematic search and content analysis. J Med Int Res. 2020;22:e17995.
Wang Y Hajli, N.,. Exploring the path to big data analytics success in healthcare. J Bus Res. 2017;70:287–99.
Srinivasan R, Swink M. An investigation of visibility and flexibility as complements to supply chain analytics: an organizational information processing theory perspective. Prod Oper Manage. 2018;27:1849–67.
Wang Y, Byrd TA. Business analytics-enabled decision-making effectiveness through knowledge absorptive capacity in health care. J Knowl Manage. 2017;21:517–39.
Wang Y, Kung LA, Byrd TA. Big data analytics: Understanding its capabilities and potential benefits for healthcare organizations. Technol Forecast Soc Change. 2018;126:3–13.
Jagadish HV, Gehrke J, Labrinidis A, Papakonstantinou Y, Patel JM, Ramakrishnan R, Shahabi C. Big data and its technical challenges. Commun ACM. 2014;57:86–94.
Seddon PB, Constantinidis D, Dod H. How does business analytics contribute to business value? In: Information Systems Journal, Proceeding of Thirty Third International Conference on Information Systems. Orlando: Wiley Publishing Ltd; 2012. p. 237–69.
Cao G, Duan Y, Li G. Linking business analytics to decision making effectiveness: a path model analysis. IEEE Trans Eng Manage. 2015;62:384–95.
Watson HJ. Tutorial: big data analytics: concepts, technologies, and applications. Commun Assoc Inf Syst. 2014;34:1247–68.
Negash S. Business intelligence. Commun Assoc Inf Syst. 2004;13:177–95.
Hurwitz J, Nugent A, Hapler F, Kaufman M. Big data for dummies. Hoboken: Wiley; 2013.
Sadeghi P, Benyoucef M, Kuziemsky CE. A mashup-based framework for multimulti-level healthcare interoperability. Inf Syst Front. 2012;14:57–72.
Yu W, Zhao G, Liu Q, Song Y. Role of big data analytics capability in developing integrated hospital supply chains and operational flexibility: An organizational information processing theory perspective. Technol Forecast Soc Change. 2021;163:120417.
Butler TW, Leong GK, Everett LN. The operations management role in hospital strategic planning. J Oper Manag. 1996;14:137–56.
Slack N, Brandon-Jones A, Johnston R. Operations management. 8th ed. Harlow: Pearson; 2016.
Liu, J., (2020), “Deployment of health IT in China’s fight against the COVID-19 pandemic”, available at: https://www.itnonline.com/article/deployment-health-it-china%E2%80%99s-fight-against-covid-19-pandemic (Accessed 20 Dec 2021).
Ting DS, Wei LC, Dzau V, Wong TY. Digital technology and COVID-19. Nat Med. 2020;26:459–61.
Article CAS PubMed PubMed Central Google Scholar
Rajasekera J, Mishal A.V., Mori Y, et al. Innovative mHealth solution for reliable patient data empowering rural healthcare in developing countries. In: Kulkarni A, et al., editors. Big data analytics in healthcare. Studies in big data, vol 66,. Cham: Springer; 2020. p. 83–103.
Ambert, K., Beaune, S., Chaibi, A., Briard, L., Bhattacharjee, A., Bharadwaj, V., Sumanth, K., Crowe, K. (2016), “French Hospital Uses Trusted Analytics Platform to Predict Emergency Department Visits and Hospital Admissions”, available at: https://www.intel.com/content/dam/www/public/us/en/documents/white-papers/french-hospital-analytics-predict-admissions-paper.pdf , (Accessed 13 Mar 2022).
Van Sickle D, Barrett M, Humblet O, Henderson K, Hogg C. Randomized, controlled study of the impact of a mobile health tool on asthma SABA use, control and adherence. Eur Respir J . 2016;48(Suppl. 60):1018.
Merchant R, Szefler SJ, Bender BG, Tuffli M, Barrett MA, Gondalia R, Kaye L, Van Sickle D, Stempel DA. Impact of a digital health intervention on asthma resource utilization. World Allergy Org J. 2018;411:28.
Khanra S, Dhir A, Islam N, Mäntymäki M. Big data analytics in healthcare: a systematic literature review. Enterprise Inf Syst. 2020;14:878–912.
Sousa MJ, Pesqueira AM, Lemos C, Sousa M, Rocha Á. Decision-making based on big data analytics for people management in healthcare organizations. J Med Syst. 2019;43:290.
Maglaveras N, Kilintzis V, Koutkias V, Chouvarda I. Integrated care and connected health approaches leveraging personalised health through big data analytics. Stud Health Technol Inf. 2016;224:117–22.
Kazançoğlu Y, Sağnak M, Lafcı Ç, Luthra S, Kumar A, Taçoğlu C. Big Data-enabled solutions framework to overcoming the barriers to circular economy initiatives in healthcare sector. Int J Environ Res Public Health. 2021;18:7513.
Article PubMed PubMed Central Google Scholar
Benzidia S, Makaoui N, Bentahar O. The impact of big data analytics and artificial intelligence on green supply chain process integration and hospital environmental performance. Technol Forecast Soc Change. 2021;165:120557.
Moutselos K, Maglogiannis I. Evidence-based public health policy models development and evaluation using big data analytics and web technologies. Med Arch (Sarajevo, Bosnia and Herzegovina). 2020;74:47–53.
Alotaibi S, Mehmood R, Katib I, Chlamtac I. The role of big data and twitter data analytics in healthcare supply chain management. In: Mehmood R, See S, Katib I, editors. Smart infrastructure and applications. Cham: EAI/Springer Innovations in Communication and Computing, Springer; 2020. p. 267–79.
Kundella S, Gobinath R. A survey on big data analytics in medical and healthcare using cloud computing. Int J Sci Technol Res. 2019;8:1061–5.
Chellah RC, Kunda D. An assessment of factors that affect the implementation of big data analytics in the Zambian health sector for strategic planning and predictive analysis: a case of Copperbelt province. Int J Electron Healthc. 2020;11:101–22.
Pastorino R, De Vito C, Migliara G, Glocker K, Binenbaum I, Ricciardi W, Boccia S. Benefits and challenges of big data in healthcare: an overview of the European initiatives. Eur J Public Health. 2019;29:23–7.
Gunapal PPG, Kannapiran P, Teow KL, Zhu Z, You AX, Saxena N, Singh V, Tham L, Choo PWJ, Chong P-N, Sim JHJ, Wong JEL. Setting up a regional health system database for seamless population health management in Singapore. Proc Singapore Healthc. 2016;25:27–34.
Clim A, Zota RD, Tinica G. Big data in home healthcare: A new frontier in personalized medicine. Medical emergency services and prediction of hypertension risks. Int J Healthc Manage. 2019;12:241–9.
Aiello M, Cavaliere C, D’Albore A, Salvatore M. The challenges of diagnostic imaging in the era of big data. J Clin Med. 2019;8:316.
Article PubMed Central Google Scholar
Bharathi MJ, Rajavarman VN. A survey on big data management in health care using IOT. Int J Recent Technol Eng. 2019;7:196–8.
Lai A, Rossignoli F, Stacchezzini R. How integrated reporting meets the investors and other stakeholders’information needs . (In Vrontis D., Weber Y., Tsoukatos E.) Global and national business theories and practice: bridging the past with the future. Cyprus: EuroMed Press; 2017.
Martinez F.E.L, Núñez-Valdez E.R, et al. Big data and machine learning: a way to improve outcomes in population health management. In: González García C, et al., editors. Protocols and applications for the industrial internet of things. Hershey: IGI Global; 2018. p. 225–39.
Download references
Acknowledgements
Not applicable.
The research was carried out without funding.
Author information
Authors and affiliations.
Department of Economics, University of Foggia, Via Caggese n.1, Foggia, Italy
Nicola Cozzoli, Fiorella Pia Salvatore, Nicola Faccilongo & Michele Milone
You can also search for this author in PubMed Google Scholar
Contributions
NC and FPS designed and conducted the empirical study, wrote and revised the manuscript. NC and FPS carried out the analysis and wrote the results, discussion and conclusions. NC, FPS, NF, and MM revised the manuscript. All authors read the manuscript and approved the final version.
Corresponding author
Correspondence to Fiorella Pia Salvatore .
Ethics declarations
Ethics approval and consent to participate, consent for publication, competing interests.
The authors declare that they have no competing interests.
Additional information
Publisher's note.
Springer Nature remains neutral with regard to jurisdictional claims in published maps and institutional affiliations.
Supplementary Information
Additional file 1..
List of articles.
Rights and permissions
Open Access This article is licensed under a Creative Commons Attribution 4.0 International License, which permits use, sharing, adaptation, distribution and reproduction in any medium or format, as long as you give appropriate credit to the original author(s) and the source, provide a link to the Creative Commons licence, and indicate if changes were made. The images or other third party material in this article are included in the article's Creative Commons licence, unless indicated otherwise in a credit line to the material. If material is not included in the article's Creative Commons licence and your intended use is not permitted by statutory regulation or exceeds the permitted use, you will need to obtain permission directly from the copyright holder. To view a copy of this licence, visit http://creativecommons.org/licenses/by/4.0/ . The Creative Commons Public Domain Dedication waiver ( http://creativecommons.org/publicdomain/zero/1.0/ ) applies to the data made available in this article, unless otherwise stated in a credit line to the data.
Reprints and permissions
About this article
Cite this article.
Cozzoli, N., Salvatore, F.P., Faccilongo, N. et al. How can big data analytics be used for healthcare organization management? Literary framework and future research from a systematic review. BMC Health Serv Res 22 , 809 (2022). https://doi.org/10.1186/s12913-022-08167-z
Download citation
Received : 02 March 2022
Accepted : 06 June 2022
Published : 22 June 2022
DOI : https://doi.org/10.1186/s12913-022-08167-z
Share this article
Anyone you share the following link with will be able to read this content:
Sorry, a shareable link is not currently available for this article.
Provided by the Springer Nature SharedIt content-sharing initiative
- Healthcare management
- Healthcare organization
- Healthcare governance
- Big data analytics
BMC Health Services Research
ISSN: 1472-6963
- General enquiries: [email protected]
Advertisement
Data Analytics in Healthcare: A Tertiary Study
- Review Article
- Open access
- Published: 09 December 2022
- Volume 4 , article number 87 , ( 2023 )
Cite this article
You have full access to this open access article
- Toni Taipalus ORCID: orcid.org/0000-0003-4060-3431 1 ,
- Ville Isomöttönen ORCID: orcid.org/0000-0002-5274-236X 1 ,
- Hanna Erkkilä 1 &
- Sami Äyrämö ORCID: orcid.org/0000-0002-7532-2771 1
5141 Accesses
5 Citations
Explore all metrics
The field of healthcare has seen a rapid increase in the applications of data analytics during the last decades. By utilizing different data analytic solutions, healthcare areas such as medical image analysis, disease recognition, outbreak monitoring, and clinical decision support have been automated to various degrees. Consequently, the intersection of healthcare and data analytics has received scientific attention to the point of numerous secondary studies. We analyze studies on healthcare data analytics, and provide a wide overview of the subject. This is a tertiary study, i.e., a systematic review of systematic reviews. We identified 45 systematic secondary studies on data analytics applications in different healthcare sectors, including diagnosis and disease profiling, diabetes, Alzheimer’s disease, and sepsis. Machine learning and data mining were the most widely used data analytics techniques in healthcare applications, with a rising trend in popularity. Healthcare data analytics studies often utilize four popular databases in their primary study search, typically select 25–100 primary studies, and the use of research guidelines such as PRISMA is growing. The results may help both data analytics and healthcare researchers towards relevant and timely literature reviews and systematic mappings, and consequently, towards respective empirical studies. In addition, the meta-analysis presents a high-level perspective on prominent data analytics applications in healthcare, indicating the most popular topics in the intersection of data analytics and healthcare, and provides a big picture on a topic that has seen dozens of secondary studies in the last 2 decades.
Similar content being viewed by others
A Systematic Review on Application of Data Mining Techniques in Healthcare Analytics and Data-Driven Decisions
Big Data Analytics in Healthcare: A Review of Opportunities and Challenges
Health Data Analytics: Current Perspectives, Challenges, and Future Directions
Explore related subjects.
- Artificial Intelligence
Avoid common mistakes on your manuscript.
Introduction
The purpose of data analytics in healthcare is to find new insights in data, at least partially automate tasks such as diagnosing, and to facilitate clinical decision-making [ 1 , 2 ]. Higher hardware cost-efficiency and the popularization and advancement of data analysis techniques have led to data analytics gaining increasing scholarly and practical footing in the healthcare sector in recent decades [ 3 ]. Some data analytics solutions have also been demonstrated to surpass human effort [ 4 ]. As healthcare data is often characterized as diverse and plentiful, especially big data analysis techniques, prospects, and challenges have been discussed in scientific literature [ 5 ]. Other related concepts such as data mining, machine learning, and artificial intelligence have also been used either as buzzwords to promote data analytics applications or as genuine novel innovations or combinations of previously tested solutions.
The terms big data , big data analytics , and data analytics are often used interchangeably, which makes the search for related scientific works difficult. Especially, big data is often used as a synonym for analytics [ 6 ], a view contested in multiple sources [ 7 , 8 , 9 ]. In addition, the term data analytics is wide and usually at least partly subsumes concepts such as statistical analyses, machine learning, data mining, and artificial intelligence, many of which overlap with each other as well in terms of, e.g., using similar algorithms for different purposes. Finally, it is not uncommon that scientific works that are not focused on technical details discuss concepts such as machine learning at different levels of specificity. For example, some studies consider merely high-level paradigms such as supervised on unsupervised learning, while some discuss different tasks such as classification or clustering, and others focus on specific modeling techniques such as decision trees, kernel methods, or different types of artificial neural networks. These concerns of nomenclature and terminology apply to healthcare as well, and we adapt the broad view of both healthcare and data analytics in this study. In other words, with data analytics we refer to general data analytics encompassing terms such as data mining, machine learning, and big data analytics, and with healthcare we refer to different fields of medicine such as oncology and cardiology, some closely related concepts such as diagnosis and disease profiling, and diseases in the broad sense of the word, including but not limited to symptoms, injuries, and infections.
Naturally, because of growing interest in the intersection of data analytics and healthcare, the scientific field has seen numerous secondary studies on the applications of different data analysis techniques to different healthcare subfields such as disease profiling, epidemiology, oncology, and mental health. As the purpose of systematic reviews and mapping studies is to summarize and synthesize literature for easier conceptualization and a higher level view [ 10 , 11 ], when the number of secondary studies renders the subjective point of understanding a phenomenon on a high level arduous, a tertiary study is arguably warranted. In fact, we deemed the number of secondary studies high enough to conduct a tertiary study. In this study, we review systematic secondary studies on healthcare data analytics during 2000–2021, with the research goals to map publication fora, publication years, numbers of primary studies utilized, scientific databases utilized, healthcare subfields, data analytics subfields, and the intersection of healthcare and data analytics. The results indicate that the number of secondary studies is rising steadily, that data analytics is widely applied in a myriad of healthcare subfields, and that machine learning techniques are the most widely utilized data analytics subfield in healthcare. The relatively high number of secondary studies appears to be the consequence of over 6800 primary studies utilized by the secondary studies included in our review. Our results present a high-level overview of healthcare data analytics: specific and general data analytics and healthcare subfields and the intersection thereof, publication trends, as well as synthesis on the challenges and opportunities of healthcare data analytics presented by the secondary studies.
The rest of the study is structured as follows. In the next section, we describe the systematic method behind secondary study search and selection. In Section “ Results ” we present the results of this tertiary study, and in Section “ Discussion ” discuss the practical implications of the results as well as threats to validity. Section “ Conclusion ” concludes the study.
Search Strategy
We searched for eligible secondary studies using five databases: ACM Digital Library (ACM DL), IEEExplore, ScienceDirect, Scopus, and PubMed. In addition, we utilized Google Scholar, but the search returned too many results to be considered in a feasible timeframe. The search strings and publications returned from the respective databases are detailed in Table 1 . Because the relevant terms healthcare , big data and data analytics have been used in an ambiguous manner in the literature, we performed two rounds of backward snowballing, i.e., followed the reference lists of included articles to capture works not found by the database searches. The search and selection processes are detailed in Fig. 1 .
Study Selection
After the secondary studies were searched for closer eligibility inspection, the first author applied the exclusion criteria listed in Table 2 . In case the first author was unsure about a study, the second author was consulted. In case a consensus was not reached, the third author was consulted with the final decision on whether to include or exclude the study. Regarding exclusion criterion E5, we only considered secondary studies, i.e., mapping studies and different types of literature reviews. Furthermore, due to different levels of systematic approaches, we deemed a study systematic if (i) the utilized databases were explicitly stated (i.e., stated with more detail than “we used databases such as...” or “we mainly used Scopus”), (ii) search terms were explicitly stated, and (iii) inclusion or exclusion criteria or both were explicitly stated. Regarding exclusion criteria E6, E7 and E8, several studies considered healthcare in related fields such as healthcare from administrative perspectives [ 12 ], healthcare data privacy [ 13 , 14 ], data quality [ 15 ], and comparing human performance with data analytics solutions [ 4 ]. Such studies were excluded. Similarly, studies returned by the database searches on data analytics related fields such as big data and its challenges [ 16 ], Internet-of-Things [ 17 ], and studies with a focus on software or hardware architectures behind analytics platforms [ 18 , 19 ] rather than on the process of analysis were also excluded.
It is worth noting that we followed the respective secondary study authors’ classification of techniques, e.g., whether a technique is considered machine learning or deep learning. In the case a study considered more than one data analytics or healthcare subfield, we categorized the study according to what was to our understanding the primary focus. This is the reason we have refrained from defining terms such as deep learning in this study—the definitions are numerous and by defining the terms, we might give the reader the impression that we have judged whether a secondary study is concerned with, e.g., machine learning or deep learning.
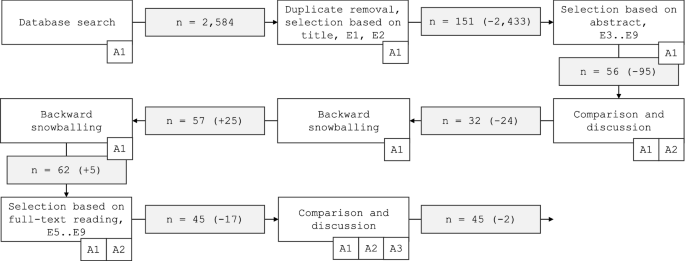
Study selection process showing the process step by step as well as the numbers of secondary studies in each step—A1, A2 and A3 refer to the authors responsible for each step, E refers to an exclusion criterion described in Table 2 , and n indicates the number of included papers after a step was completed
Publication Fora and Years
We included 45 secondary studies (abbreviated SE in the figures, cf. 7 for full bibliographic details). A total of 34 (76%) of the selected secondary studies were published in academic journals, nine (20%) in conference proceedings, and two (4%) were book chapters. Most of the studies were published in distinct fora (cf. Table 3 ), and fora with more than one selected secondary study consisted of Journal of Medical Systems , International Journal of Medical Informatics , Journal of Biomedical Informatics , and IEEE Access . As expected, the publication fora were aimed at either computer science, healthcare, or both. Finally, as can be observed in Fig. 2 , the trend of systematic secondary studies in the intersection of data analytics and healthcare is growing.
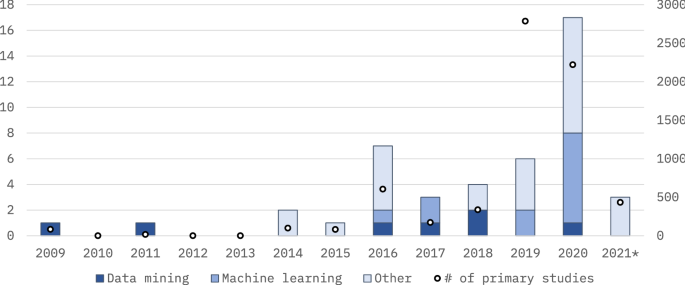
Number of included secondary studies by publication year (bars, left y-axis), and the number of included primary studies by publication year (dots, right y-axis)—the year 2021 was only considered from January to April; the figure shows that the number of secondary studies is rising
Secondary Study Qualities
The selected secondary studies utilized a total of 37 different databases. The most frequently used databases were PubMed, Scopus, IEEExplore, and Web of Science, respectively. Other relatively frequently used databases were ACM Digital Library, Google Scholar, and Springer Link. Most of the secondary studies (33, or 73%) utilized four or fewer databases ( M = 3.6, Mdn = 3). However, many bibliographic databases subsume others, and the number of utilized databases should not be taken as a metric for a systematic review quality. For example, a PubMed search implicitly searches MEDLINE records, and Google Scholar indexes works from most other scientific databases. The extended coverage of a wider range of academic works naturally results in numerous studies to further inspect, posing a challenge in the amount of work required. The most popular databases used in the secondary studies are visualized in Fig. 3 .
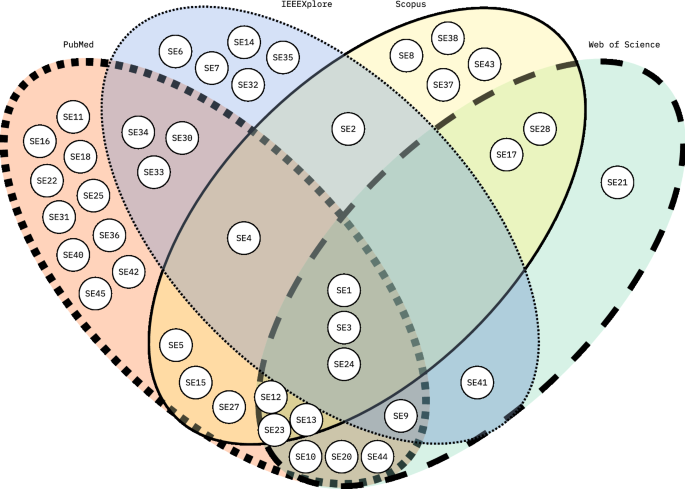
Four most popular databases used by the secondary studies were PubMed, IEEEXplore, Scopus and Web of Science—4 studies did not use any of these four databases, and other databases are not considered, e.g., the secondary study SE14, in addition to IEEExplore, might have also utilized other databases not visualized here
The secondary studies reported an average of 155 selected primary studies ( Mdn = 63, SD = 379.2), with a minimum of 6 (SE44) and a maximum of 2,421 primary studies (SE31). Five secondary studies selected more than 200 primary studies (cf. Fig 5 ). In total, the secondary studies utilized 6,838 primary studies. The number of secondary and primary studies categorized by the data analytics approach is summarized in Fig. 4 .
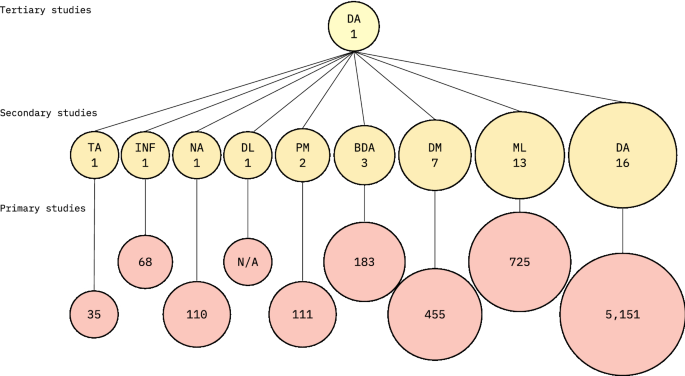
Number of secondary studies included in this tertiary study, and the number of primary studies utilized by the secondary studies, categorized by data analytics approach; DA general data analytics, TA text analytics, INF informatics, NA network analytics, DL deep learning, PM process mining, BDA big data analytics, DM data mining, ML machine learning; the figure shows that the general term data analytics was the most popular in the secondary studies
Some secondary studies reported similar details on their respective primary studies, such as visualizations of publication years (22 studies), research approach summaries such as the number of qualitative and quantitative studies (8 studies), research field summaries (4 studies), and details on the geographic distribution of the primary study authors (5 studies). The use of PRISMA (preferred reporting items for systematic reviews and meta-analyses) [ 41 ] guidelines was reported in 15 studies.
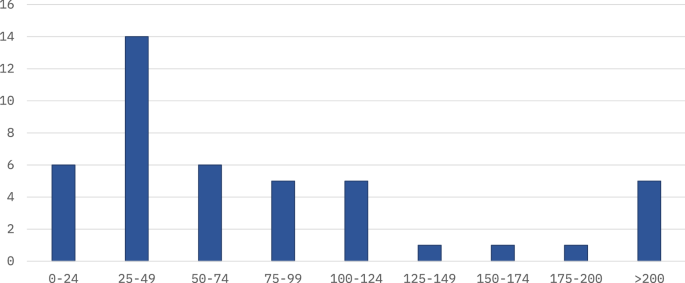
Number of primary studies (x-axis) selected for final inclusion in the secondary studies (y-axis), e.g., the chart shows that six secondary studies included 0–24 primary studies—one study (SE6) did not disclose the number of primary studies, and one study (SE15) reported two numbers: 24 primary studies for a quantitative analysis, and 28 primary studies for a qualitative analysis, and we reported that study using the latter number
Subject Areas Identified
Some selected studies considered the relationship between healthcare in general and a specific data analysis technique, while other studies considered the relationship between data analytics in general and a specific healthcare subfield. Most of the studies, however, considered the relationship between a specific data analysis technique and a specific healthcare subfield. These considerations are summarized in Fig. 6 . Readers interested mainly in general healthcare in the context of a specific analysis topic should refer to the secondary studies on the left-hand side, readers interested in general data analytics in the context of a specific healthcare topic should refer to secondary studies on the right-hand side, readers interested in a specific analysis topic applied to a specific healthcare topic should consider the studies in the middle, and readers interested in the applicability of analytics techniques in general to healthcare in general should consider the studies in the top row. Additional information on the secondary studies is presented in 6 .
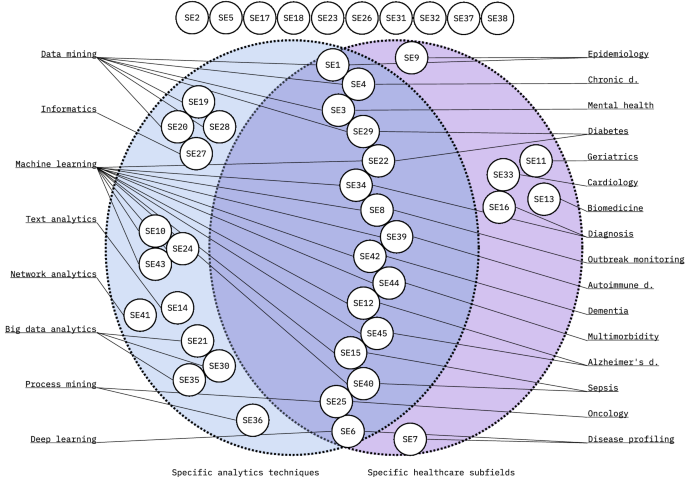
Selected secondary studies and whether they consider only specific data analytics techniques (left side), only specific healthcare subfields (right side), both (center), or neither (top); the figure may be utilized in finding relevant secondary studies on desired subfields
Implications
Considering the number of primary studies utilized, only 12 studies (27%) used more than a hundred primary studies. Figure 5 seems to indicate that the threshold for conducting a literature review or a mapping study in healthcare data analytics is typically between 25 and 100 studies. Furthermore, and on the basis of the evidence currently available, it seems reasonable to argue that at least 25 primary studies (84% of the secondary studies) warrant a systematic review, and the results of systematic reviews can be seen as valuable synthesizing contributions to the field. This observation arguably also supports the relevance of this study, although this study covers a relatively large intersection of the two research areas.
The earliest included secondary study was published in 2009, which might be explained by the relative novelty of data analysis in healthcare, at least with computerized automation rather than merely applying statistical analyses. In addition, although systematic reviews are relatively common in medicine, they have only recently gained popularity and visibility in information technology [ 10 ]. As may be observed in Fig. 2 , the trend of secondary studies is growing, which consequently indicates that the number of primary studies in the intersection of data analytics and healthcare is gaining research interest. The rising popularity of machine learning algorithms may be explained by the rising popularity of unstructured data, the growing utilization of graphics processing units, and the development of different machine learning tools and software libraries. Indeed, many of the techniques behind modern machine learning implementations have been around since the 1980s, but only the combination of large amounts of data, and developments in methods and computer hardware in recent years have made such implementations more cost-effective. The development of trends illustrated in, e.g., Fig. 2 propounds the view that machine learning algorithms will gain more and more practical applications in healthcare and related fields, such as molecular biology [ 42 ]. Finally, some studies have argued [ 43 ] as well as demonstrated [ 44 , 45 ] that the evolution of machine learning is changing the way research hypotheses are formulated. Instead of theory-driven hypothesis formulation, machine learning can be used to facilitate the formulation of data-driven hypotheses, also in the field of medicine.
Secondary study publication fora were numerous and focused either on information technology, healthcare, or both, without obvious anomalies. The secondary studies utilized dozens of different databases in their primary study searches. It seems that the coverage of these databases is not always understood, or it is disregarded, regardless that utilizing non-overlapping databases results in less work in duplicate publication removal. For example, Scopus indexes some of ACM DL, some of Web of Science, and all of IEEExplore, effectively rendering IEEExplore search redundant if Scopus is utilized—a fact we as well understood only after conducting our searches. In addition, Google Scholar appears to index the bibliographic details of effectively all published research, yet the number of search results returned may be overwhelming for a systematic review. In practice, the selection of databases is balanced by the amount of work needed to examine the results on one end of the scales, and coverage on the other. Backward or forward snowballing may be utilized to limit the amount of work and to extend coverage.
Secondary study topics summarized in Fig. 6 give some implications for subject areas of healthcare data analytics that are mature enough to warrant a secondary study. As the figure shows, these areas are aplenty, and the most frequent data analytics techniques applied seem to be machine learning (13 secondary studies) and data mining (7 secondary studies). It is worth noting that the nomenclature we applied in this study reflects that of the secondary study authors. As explained earlier in this study, attempts at defining, e.g., machine learning and data mining in this study would inevitably contradict the definitions given in some of the included secondary studies. For further reading, Cabatuan and Maguerra [ 46 ] provide a high-level overview of machine learning and deep learning, and Shukla, Patel and Sen [ 47 ] on data mining. For more technical approaches, both Ahmad, Qamar and Rizvi [ 30 ] and Harper [ 48 ] review data mining techniques and algorithms in healthcare.
Opportunities and Challenges in Healthcare Data Analytics
Many of the selected secondary studies provided syntheses on the current challenges and opportunities in healthcare data analytics. As the secondary studies inspected over 6800 studies of healthcare data analytics, we have summarized recurring insights here.
It was a generally accepted view in the secondary studies that healthcare data analytics is an opportunity that has already been partly realized, yet needs to be more studied and applied in more diverse contexts and in-depth scenarios [ 49 , 50 , 51 ]. For example, it has been noted that while big data applications are relatively mature in bio-informatics, this is not necessarily the case in other biomedical fields [ 52 ]. In general, healthcare data analytics is rather uniformly perceived as an opportunity for more cost-efficient healthcare [ 52 , 53 ] through many applications such as automating a specialist’s routine tasks so that they may focus on tasks more crucial in a patient’s treatment. The cost-efficiency is likely to be more concretized by novel deep learning techniques such as large language models [ 54 ], which are also offered through implementations that perform tasks faster while consuming less resources [ 55 ]. In addition to faster diagnoses, data analytics solutions may also offer more objective diagnoses in, e.g., pathology, if the models are trained with data from multiple pathologists.
Challenges regarding healthcare data analytics are more diverse. Perhaps the most discussed challenge was the nature of the data and how it can be treated. Many secondary studies highlighted problems with missing data [ 56 , 57 ], low-quality data [ 54 ], and datasets stored in various formats which are not interoperable with each other [ 52 , 55 , 56 ]. Furthermore, some studies raised the concern of missing techniques to visualize the outputs given by different data analyses [ 56 , 58 ]. Rather intuitively, many new implementations and the increases in the amount of data require new computational infrastructure for feasible use [ 54 , 58 , 59 , 60 ]. Some studies raised ethical concerns regarding data collection, merging, and sharing, as data privacy is a multifaceted concept [ 52 , 54 , 58 , 59 ], especially when the datasets cover multiple countries with different legislations. Many studies also called for multidisciplinary collaboration between medical and computing experts, stating that it is crucial that the analytics implementations are based on the same vocabulary and rules as medical experts use [ 49 , 57 , 61 , 62 , 63 , 64 ], and that the technical experts understand, e.g., how feasible it is to collect training data for a model to find patterns in medical images. Closely related, many of the more complex analytics solutions operate on a black box principle, meaning that it is not obvious how the implementation reaches the conclusion it reaches [ 56 , 59 , 65 , 66 , 67 ]. Open solutions, on the other hand, are typically understandable only for technical experts and may be outperformed by the more complex black box solutions. Finally, it has been observed that the already existing analytics solutions implemented in different environments, e.g., different hospitals [ 56 , 59 , 64 ], are not portable into other environments. In addition, it may be that the existing solutions are not fully integrated into actual day-to-day work [ 57 ]. Fleuren et al. [ 68 ] summarize the issue aptly, urging “ to bridge the gap between bytes and bedside. ”
Threats to Validity
As is typical for studies involving human judgment, it is possible for another group of researchers to select at least a slightly different group of studies. Furthermore, the categorization of studies into specific healthcare and data analytics topics is a likely candidate for the subject of change. We tried to mitigate the effect of human judgment by following the systematic mapping study guidelines, such as utilizing and reporting explicit exclusion criteria and search terms [ 11 ], following the PRISMA flow of information guidelines [ 41 ], and discussing discrepancies and disagreements among the authors until consensus was reached. Regarding the challenges related to the wide and rather ambiguous subject areas of data analytics and healthcare, we utilized two rounds of backward snowballing to mitigate the threat of missing relevant studies.
In this study, we systematically mapped systematic secondary studies on healthcare data analytics. The results implicate that the number of secondary—and naturally primary—studies are rising, and the scientific publication fora around the topics are numerous. We also discovered that the number of primary studies included in the secondary studies varies greatly, as do the scientific databases used in primary study search. The results also show that while machine learning and data mining seem to be the most popular data analytics subfields in healthcare, specific healthcare topics are more diverse. This meta-analysis provides researchers with a high-level overview of the intersection of data analytics and healthcare, and an accessible starting point towards specific studies. What was not considered in this study is whether or not and how much the selected secondary studies overlap in their primary study selection, which could indicate the level of either deliberate or unaware overlap of similar work.
Data availability
The datasets generated during and/or analysed during the current study are available from the corresponding author on reasonable request.
Mikalef P, Boura M, Lekakos G, Krogstie J. Big data analytics and firm performance: findings from a mixed-method approach. J Bus Res. 2019;98:261–76. https://doi.org/10.1016/j.jbusres.2019.01.044 .
Article Google Scholar
Yang H, Kundakcioglu OE, Zeng D. Healthcare data analytics. Inf Syst e-Bus Manag. 2015;13(4):595–7. https://doi.org/10.1007/s10257-015-0297-0 .
Wang Y, Hajli N. Exploring the path to big data analytics success in healthcare. J Bus Res. 2017;70:287–99. https://doi.org/10.1016/j.jbusres.2016.08.002 .
Liu X, Faes L, Kale AU, Wagner SK, Fu DJ, Bruynseels A, et al. A comparison of deep learning performance against health-care professionals in detecting diseases from medical imaging: a systematic review and meta-analysis. Lancet Digit Health 2019;1(6):e271–e297. https://www.sciencedirect.com/science/article/pii/S2589750019301232 . https://doi.org/10.1016/S2589-7500(19)30123-2 .
Abidi SSR, Abidi SR. Intelligent health data analytics: a convergence of artificial intelligence and big data. Healthc Manag Forum. 2019;32(4):178–82. https://doi.org/10.1177/0840470419846134 .
Article MATH Google Scholar
Akoka J, Comyn-Wattiau I, Laoufi N. Research on big data—a systematic mapping study. Comput Stand Interfaces. 2017;54:105–15. https://doi.org/10.1016/j.csi.2017.01.004 .
Daniel BK. Big data and data science: a critical review of issues for educational research. Br J Educ Technol. 2017;50(1):101–13. https://doi.org/10.1111/bjet.12595 .
Khan N, Alsaqer M, Shah H, Badsha G, Abbasi AA, Salehian S. The 10 Vs, issues and challenges of big data. In: Proceedings of the 2018 International Conference on big data and education. ACM; 2018, https://doi.org/10.1145/3206157.3206166 .
Opresnik D, Taisch M. The value of big data in servitization. Int J Prod Econ. 2015;165:174–84. https://doi.org/10.1016/j.ijpe.2014.12.036 .
Petersen K, Feldt R, Mujtaba S, Mattsson M. Systematic mapping studies in software engineering. BCS Learn Dev. 2008. https://doi.org/10.14236/ewic/ease2008.8 .
Petersen K, Vakkalanka S, Kuzniarz L. Guidelines for conducting systematic mapping studies in software engineering: an update. Inf Softw Technol. 2015;64:1–18. https://doi.org/10.1016/j.infsof.2015.03.007 .
Sharma A, Mansotra V. Emerging applications of data mining for healthcare management—a critical review. In: 2014 International Conference on computing for sustainable global development (INDIACom). IEEE; 2014, https://doi.org/10.1109/indiacom.2014.6828163 .
Rahim FA, Ismail Z, Samy GN. Information privacy concerns in electronic healthcare records: a systematic literature review. In: 2013 International Conference on Research and innovation in information systems (ICRIIS). IEEE; 2013, https://doi.org/10.1109/icriis.2013.6716760 .
Sajedi H. Applications of data hiding techniques in medical and healthcare systems: a survey. Netw Model Anal Health Inf Bioinform. 2018. https://doi.org/10.1007/s13721-018-0169-x .
Biancone PP, Secinaro S, Brescia V, Calandra D. Data quality methods and applications in health care system: a systematic literature review. Int J Bus Manag. 2019;14(4):35. https://doi.org/10.5539/ijbm.v14n4p35 .
Strang KD, Sun Z. Hidden big data analytics issues in the healthcare industry. Health Inf J. 2019;26(2):981–98. https://doi.org/10.1177/1460458219854603 .
Saheb T, Izadi L. Paradigm of IoT big data analytics in the healthcare industry: A review of scientific literature and mapping of research trends. Telematics Inf. 2019;41:70–85. https://doi.org/10.1016/j.tele.2019.03.005 .
Imran S, Mahmood T, Morshed A, Sellis T. Big data analytics in healthcare a systematic literature review and roadmap for practical implementation. IEEE/CAA J Autom Sin. 2021;8(1):1–22. https://doi.org/10.1109/jas.2020.1003384 .
Senthilkumar S. Big data in healthcare management: a review of literature. Am J Theoret Appl Bus. 2018;4(2):57. https://doi.org/10.11648/j.ajtab.20180402.14 .
Lim TC. Review of data mining methodologies for healthcare applications. J Med Imaging Health Inf. 2013;3(2):288–93. https://doi.org/10.1166/jmihi.2013.1164 .
Gupta S, Goel L, Agarwal AK. Technologies in health care domain: a systematic review. Int J e-Collab (IJeC). 2020;16(1):33–44.
Google Scholar
Hiller JS. Healthy predictions? questions for data analytics in health care. Am Bus Law J. 2016;53(2):251–314. https://doi.org/10.1111/ablj.12078 .
Article MathSciNet Google Scholar
Sterling M, Situated big data and big data analytics for healthcare. In,. IEEE Global Humanitarian Technology Conference (GHTC). IEEE. 2017;2017. https://doi.org/10.1109/ghtc.2017.8239322 .
Wang L, Alexander CA. Big data analytics in medical engineering and healthcare: methods, advances and challenges. J Med Eng Technol. 2020;44(6):267–83. https://doi.org/10.1080/03091902.2020.1769758 .
Nagaraj K, Sharvani G, Sridhar A. Emerging trend of big data analytics in bioinformatics: a literature review. Int J Bioinform Res Appl. 2018;14(1/2):144. https://doi.org/10.1504/ijbra.2018.10009206 .
Kaur PC. A study on role of machine learning in detect in heart disease. In: 2020 Fourth International Conference on computing methodologies and communication (ICCMC). IEEE; 2020, https://doi.org/10.1109/iccmc48092.2020.iccmc-00037 .
Nagavci D, Hamiti M, Selimi B. Review of prediction of disease trends using big data analytics. Int J Adv Comput Sci Appl. 2018. https://doi.org/10.14569/ijacsa.2018.090807 .
Pandit A, Garg A. Artificial neural networks in healthcare: A systematic review. In: 2021 11th International Conference on cloud computing, data science & engineering (Confluence). IEEE; 2021, https://doi.org/10.1109/confluence51648.2021.9377086 .
Schinkel M, Paranjape K, Panday RN, Skyttberg N, Nanayakkara P. Clinical applications of artificial intelligence in sepsis: a narrative review. Comput Biol Med. 2019;115: 103488. https://doi.org/10.1016/j.compbiomed.2019.103488 .
Ahmad P, Qamar S, Rizvi SQA. Techniques of data mining in healthcare: a review. Int J Comput Appl. 2015;120(15).
Cichosz SL, Johansen MD, Hejlesen O. Toward big data analytics: review of predictive models in management of diabetes and its complications. J Diabetes Sci Technol. 2016;10(1):27–34.
Zainab K, Dhanda N. Big data and predictive analytics in various sectors. In: 2018 International Conference on system modeling & advancement in research trends (SMART). IEEE; 2018, https://doi.org/10.1109/sysmart.2018.8746929 .
Thakur S, Ramzan M. A systematic review on cardiovascular diseases using big-data by hadoop. In: 2016 6th International Conference—cloud system and big data engineering (Confluence). IEEE. 2016;2016. https://doi.org/10.1109/confluence.2016.7508142 .
Yeng PK, Nweke LO, Woldaregay AZ, Yang B, Snekkenes EA. Data-driven and artificial intelligence (AI) approach for modelling and analyzing healthcare security practice: a systematic review. In: Advances in intelligent systems and computing. Springer International Publishing; 2020, p. 1–18. https://doi.org/10.1007/978-3-030-55180-3_1 .
Kruse CS, Goswamy R, Raval Y, Marawi S. Challenges and opportunities of big data in health care: A systematic review. JMIR Med Inf. 2016;4(4): e38. https://doi.org/10.2196/medinform.5359 .
Swenson ER, Bastian ND, Nembhard HB. Healthcare market segmentation and data mining: a systematic review. Health Mark Q. 2018;35(3):186–208. https://doi.org/10.1080/07359683.2018.1514734 .
Sousa MJ, Pesqueira AM, Lemos C, Sousa M, Rocha A. Decision-making based on big data analytics for people management in healthcare organizations. J Med Syst. 2019. https://doi.org/10.1007/s10916-019-1419-x .
Galetsi P, Katsaliaki K, Kumar S. Values, challenges and future directions of big data analytics in healthcare: a systematic review. Soc Sci Med. 2019;241: 112533. https://doi.org/10.1016/j.socscimed.2019.112533 .
Chung Y, Bagheri N, Salinas-Perez JA, Smurthwaite K, Walsh E, Furst M, et al. Role of visual analytics in supporting mental healthcare systems research and policy: a systematic scoping review. Int J Inf Manag. 2020;50:17–27. https://doi.org/10.1016/j.ijinfomgt.2019.04.012 .
Niaksu O, Skinulyte J, Duhaze HG. A systematic literature review of data mining applications in healthcare. In: Web Information Systems Engineering WISE 2013 Workshops. Springer Berlin Heidelberg; 2014, p. 313–324. https://doi.org/10.1007/978-3-642-54370-8_27 .
Moher D. Preferred reporting items for systematic reviews and meta-analyses: the PRISMA statement. Ann Intern Med. 2009;151(4):264. https://doi.org/10.7326/0003-4819-151-4-200908180-00135 .
Jumper J, Evans R, Pritzel A, et al. Highly accurate protein structure prediction with AlphaFold. Nature. 2021;596:583–9. https://doi.org/10.1038/s41586-021-03819-2 .
Oquendo MA, Baca-García E, Artés-Rodríguez A, Perez-Cruz F, Galfalvy HC, Blasco-Fontecilla H, et al. Machine learning and data mining: strategies for hypothesis generation. Mol Psychiatry 2012;17(10):956–959. http://www.ncbi.nlm.nih.gov/pubmed/22230882 .
Jauhiainen S, Kauppi JP, Leppänen M, Pasanen K, Parkkari J, Vasankari T, et al. New machine learning approach for detection of injury risk factors in young team sport athletes. Int J Sports Med. 2020;42(02):175–82. https://doi.org/10.1055/a-1231-5304 .
Joensuu L, Rautiainen I, Äyrämö S, Syväoja HJ, Kauppi JP, Kujala UM, et al. Precision exercise medicine: predicting unfavourable status and development in the 20-m shuttle run test performance in adolescence with machine learning. BMJ Open Sport Exerc Med. 2021;7(2): e001053. https://doi.org/10.1136/bmjsem-2021-001053 .
Cabatuan M, Manguerra M. Machine learning for disease surveillance or outbreak monitoring: a review. In: 2020 IEEE 12th International Conference on humanoid, nanotechnology, information technology, communication and control, environment, and management (HNICEM). IEEE; 2020, https://doi.org/10.1109/hnicem51456.2020.9400088 .
Shukla D, Patel SB, Sen AK. A literature review in health informatics using data mining techniques. Int J Softw Hardw Res Eng. 2014;2(2):123–9.
Harper PR. A review and comparison of classification algorithms for medical decision making. Health Policy. 2005;71(3):315–31. https://doi.org/10.1016/j.healthpol.2004.05.002 .
de la Torre Díez I, Cosgaya HM, Garcia-Zapirain B, López-Coronado M. Big data in health: a literature review from the year 2005. J Med Syst. 2016. https://doi.org/10.1007/s10916-016-0565-7 .
Malik MM, Abdallah S, Ala’raj M. Data mining and predictive analytics applications for the delivery of healthcare services: a systematic literature review. Ann Oper Res. 2016;270(1–2):287–312. https://doi.org/10.1007/s10479-016-2393-z .
Article MathSciNet MATH Google Scholar
Khanra S, Dhir A, Islam AKMN, Mäntymäki M. Big data analytics in healthcare: a systematic literature review. Enterp Inf Syst. 2020;14(7):878–912. https://doi.org/10.1080/17517575.2020.1812005 .
Luo J, Wu M, Gopukumar D, Zhao Y. Big data application in biomedical research and health care: a literature review. Biomed Inf Insights. 2016;8:BII.S31559. https://doi.org/10.4137/bii.s31559 .
Kamble SS, Gunasekaran A, Goswami M, Manda J. A systematic perspective on the applications of big data analytics in healthcare management. Int J Healthc Manag. 2018;12(3):226–40.
Elbattah M, Arnaud E, Gignon M, Dequen G. The role of text analytics in healthcare: a review of recent developments and applications. In: Proceedings of the 14th International Joint Conference on biomedical engineering systems and technologies. SCITEPRESS—Science and Technology Publications; 2021, https://doi.org/10.5220/0010414508250832 .
Alonso SG, de la Torre Diez I, Rodrigues JJ, Hamrioui S, Lopez-Coronado M. A systematic review of techniques and sources of big data in the healthcare sector. J Med Syst. 2017;41(11):1–9.
Carroll LN, Au AP, Detwiler LT, Chieh FuT, Painter IS, Abernethy NF. Visualization and analytics tools for infectious disease epidemiology: A systematic review. J Biomed Inf. 2014;51:287–98. https://doi.org/10.1016/j.jbi.2014.04.006 .
Islam M, Hasan M, Wang X, Germack H, Noor-E-Alam M. A systematic review on healthcare analytics: application and theoretical perspective of data mining. Healthcare. 2018;6(2):54. https://doi.org/10.3390/healthcare6020054 .
Iavindrasana J, Cohen G, Depeursinge A, Müller H, Meyer R, Geissbuhler A. Clinical data mining: a review. Yearb Med Inf. 2009;18(01):121–33.
Peiffer-Smadja N, Rawson T, Ahmad R, Buchard A, Georgiou P, Lescure FX, et al. Corrigendum to ‘machine learning for clinical decision support in infectious diseases: a narrative review of current applications’ clinical microbiology and infection (2020) 584–595. Clin Microbiol Infect. 2020;26(8):1118. https://doi.org/10.1016/j.cmi.2020.05.020 .
Toor R, Chana I. Network analysis as a computational technique and its benefaction for predictive analysis of healthcare data: a systematic review. Arch Comput Methods Eng. 2020;28(3):1689–711. https://doi.org/10.1007/s11831-020-09435-z .
Behera RK, Bala PK, Dhir A. The emerging role of cognitive computing in healthcare: a systematic literature review. Int J Med Inf. 2019;129:154–66. https://doi.org/10.1016/j.ijmedinf.2019.04.024 .
Kurniati AP, Johnson O, Hogg D, Hall G. Process mining in oncology: a literature review. In: 2016 6th International Conference on information communication and management (ICICM). IEEE; 2016, https://doi.org/10.1109/infocoman.2016.7784260 .
Waschkau A, Wilfling D, Steinhäuser J. Are big data analytics helpful in caring for multimorbid patients in general practice?–a scoping review. BMC Family Pract. 2019. https://doi.org/10.1186/s12875-019-0928-5 .
Rojas E, Munoz-Gama J, Sepúlveda M, Capurro D. Process mining in healthcare: a literature review. J Biomed Inf. 2016;61:224–36. https://doi.org/10.1016/j.jbi.2016.04.007 .
Kumar ES, Bindu CS. Medical image analysis using deep learning: a systematic literature review. In: International Conference on emerging technologies in computer engineering. Springer; 2019, p. 81–97.
Dallora AL, Eivazzadeh S, Mendes E, Berglund J, Anderberg P. Prognosis of dementia employing machine learning and microsimulation techniques: a systematic literature review. Proc Comput Sci. 2016;100:480–8. https://doi.org/10.1016/j.procs.2016.09.185 .
Buettner R, Klenk F, Ebert M, A systematic literature review of machine learning-based disease profiling and personalized treatment. In,. IEEE 44th Annual Computers, Software, and Applications Conference (COMPSAC). IEEE. 2020;2020. https://doi.org/10.1109/compsac48688.2020.00-15 .
Fleuren LM, Klausch TL, Zwager CL, Schoonmade LJ, Guo T, Roggeveen LF, et al. Machine learning for the prediction of sepsis: a systematic review and meta-analysis of diagnostic test accuracy. Intensive Care Med. 2020;46(3):383–400.
Download references
Open Access funding provided by University of Jyväskylä (JYU). This research did not receive any specific grant from funding agencies in the public, commercial, or not-for-profit sectors.
Author information
Authors and affiliations.
Faculty of Information Technology, University of Jyväskylä, P.O. Box 35, FI-40014, Jyvaskyla, Finland
Toni Taipalus, Ville Isomöttönen, Hanna Erkkilä & Sami Äyrämö
You can also search for this author in PubMed Google Scholar
Corresponding author
Correspondence to Toni Taipalus .
Ethics declarations
Conflicts of interest.
On behalf of all authors, the corresponding author states that there is no conflict of interest.
Additional information
Publisher's note.
Springer Nature remains neutral with regard to jurisdictional claims in published maps and institutional affiliations.

Appendix A. Secondary Study Qualities
See Table 4 .
Appendix B. Secondary Studies
] Albahri AS, Hamid RA, Alwan Jk, Al-qays ZT, Zaidan AA, Zaidan BB, Albahri AOS, AlAmoodi AH, Khlaf JM, Almahdi EM, Thabet E, Hadi SM, Mohammed KI, Alsalem MA, Al-Obaidi JR, Madhloom HT. Role of biological data mining and machine learning techniques in detecting and diagnosing the novel coronavirus (COVID-19): a systematic review. J Med Syst. 2020;44(7).
] Alkhatib M, Talaei-Khoei A, Ghapanchi A. Analysis of research in healthcare data analytics. In: Australasian Conference on Information Systems, 2016.
] Alonso SG, de la Torre-Díez I, Hamrioui S, López-Coronado M, Barreno DC, Nozaleda LM, Franco M. Data mining algorithms and techniques in mental health: a systematic review. J Med Syst. 2018;42(9):1–15.
] Alonso SG, de la Torre Diez I, Rodrigues JJPC, Hamrioui S, Lopez-Coronado M. A systematic review of techniques and sources of big data in the healthcare sector. J Med Syst. 2017;41(11):1–9.
] Behera RK, Bala PK, Dhir A. The emerging role of cognitive computing in healthcare: a systematic literature review. Int J Med Inf. 2019;129:154–166.
] Buettner R, Bilo M, Bay N, Zubac T. A systematic literature review of medical image analysis using deep learning. In: 2020 IEEE Symposium on Industrial Electronics & Applications (ISIEA). IEEE, 2020.
] Buettner R, Klenk F, Ebert M. A systematic literature review of machine learning-based disease profiling and personalized treatment. In: 2020 IEEE 44th Annual Computers, Software, and Applications Conference (COMPSAC). IEEE, 2020.
] Cabatuan M, Manguerra M. Machine learning for disease surveillance or outbreak monitoring: a review. In: 2020 IEEE 12th International Conference on Humanoid, Nanotechnology, Information Technology, Communication and Control, Environment, and Management (HNICEM). IEEE, 2020.
] Carroll LN, Au AP, Detwiler LT, Fu Tc, Painter IS, Abernethy NF. Visualization and analytics tools for infectious disease epidemiology: a systematic review. J Biomed Inf. 2014;51:287–298.
] Choudhury A, Asan O. Role of artificial intelligence in patient safety outcomes: systematic literature review. JMIR Med Inf. 2020;8(7):e18599.
] Choudhury A, Renjilian E, Asan O. Use of machine learning in geriatric clinical care for chronic diseases: a systematic literature review. JAMIA Open. 2020;3(3):459–471.
] Dallora AL, Eivazzadeh S, Mendes E, Berglund J, Anderberg P. Prognosis of dementia employing machine learning and microsimulation techniques: a systematic literature review. Proc Comput Sci. 2016;100:480–8.
] de la Torre Díez I, Cosgaya HM, Garcia-Zapirain B, López-Coronado M. Big data in health: a literature review from the year 2005. J Med Syst. 2016;40(9).
] Elbattah M, Arnaud E, Gignon M, Dequen G. The role of text analytics in healthcare: a review of recent developments and applications. In: Proceedings of the 14th International Joint Conference on Biomedical Engineering Systems and Technologies. SCITEPRESS—Science and Technology Publications, 2021.
] Fleuren LM, Klausch TLT, Zwager CL, Schoonmade LJ, Guo T, Roggeveen LF, Swart EL, Girbes ARJ, Thoral P, Ercole A, et al. Machine learning for the prediction of sepsis: a systematic review and meta-analysis of diagnostic test accuracy. Intensive Care Med. 2020;46(3):383–400.
] Gaitanou P, Garoufallou E, Balatsoukas P. The effectiveness of big data in health care: A systematic review. In: Communications in Computer and Information Science, pp. 141–153. Springer International Publishing; 2014.
] Galetsi P, Katsaliaki K. A review of the literature on big data analytics in healthcare. J Oper Res Soc. 2020;71(10):1511–1529.
] Gesicho MB, Babic A. Analysis of usage of indicators by leveraging health data warehouses: A literature review. In: Studies in Health Technology and Informatics, pages 184–187. IOS Press; 2019.
] Iavindrasana J, Cohen G, Depeursinge A, Müller H, Meyer R, Geissbuhler A. Clinical data mining: a review. Yearb Med Inf. 2009;18(01):121–133.
] Islam Md, Hasan Md, Wang X, Germack H, Noor-E-Alam Md. A systematic review on healthcare analytics: Application and theoretical perspective of data mining. Healthcare. 2018;6(2):54.
] Kamble SS, Gunasekaran A, Goswami M, Manda J. A systematic perspective on the applications of big data analytics in healthcare management. Int J Healthc Manag. 2018;12(3):226–240.
] Kavakiotis I, Tsave O, Salifoglou A, Maglaveras N, Vlahavas I, Chouvarda I. Machine learning and data mining methods in diabetes research. Comput Struct Biotechnol J. 2017;15:104–116.
] Khanra S, Dhir A, Najmul Islam AKM, Mäntymäki M. Big data analytics in healthcare: a systematic literature review. Enterp Inf Syst. 2020;14(7):878–912.
] Sudheer Kumar E, Shoba Bindu C. Medical image analysis using deep learning: a systematic literature review. In: International Conference on Emerging Technologies in Computer Engineering, pages 81–97. Springer; 2019.
] Kurniati AP, Johnson O, Hogg D, Hall G. Process mining in oncology: a literature review. In: 2016 6th International Conference on Information Communication and Management (ICICM). IEEE, 2016.
] Li J, Ding W, Cheng H, Chen P, Di D, Huang W. A comprehensive literature review on big data in healthcare. In: Twenty-second Americas Conference on Information Systems (AMCIS), 2016.
] Luo J, Wu M, Gopukumar D, Zhao Y. Big data application in biomedical research and health care: A literature review. Biomed Inf Insights. 2016;8:BII.S31559.
] Malik MM, Abdallah S, Ala’raj M. Data mining and predictive analytics applications for the delivery of healthcare services: a systematic literature review. Ann Oper Res. 2016;270(1-2):287–312.
] Marinov M, Mohammad Mosa AS, Yoo I, Boren SA. Data-mining technologies for diabetes: A systematic review. J Diabetes Sci Technol. 2011;5(6):1549–1556.
] Mehta N, Pandit A. Concurrence of big data analytics and healthcare: a systematic review. Int J Med Inf. 2018;114:57–65.
] Mehta N, Pandit A, Shukla S. Transforming healthcare with big data analytics and artificial intelligence: a systematic mapping study. J Biomed Inf. 2019;100:103311.
] Nazir S, Khan S, Khan HU, Ali S, Garcia-Magarino I, Atan RB, Nawaz M. A comprehensive analysis of healthcare big data management, analytics and scientific programming. IEEE Access. 2020;8:95714–95733.
] Nazir S, Nawaz M, Adnan A, Shahzad S, Asadi S. Big data features, applications, and analytics in cardiology—a systematic literature review. IEEE Access. 2019;7:143742–143771.
] Peiffer-Smadja N, Rawson TM, Ahmad R, Buchard A, Georgiou P, Lescure F-X, Birgand G, Holmes AH. Machine learning for clinical decision support in infectious diseases: a narrative review of current applications. Clin Microbiol Infect. 2020;26(5):584–595.
] Raja R, Mukherjee I, Sarkar BK. A systematic review of healthcare big data. Sci Programm. 2020;2020.
] Rojas E, Munoz-Gama J, Sepúlveda M, Capurro D. Process mining in healthcare: a literature review. J Biomed Inform. 2016;61:224–236.
] Salazar-Reyna R, Gonzalez-Aleu F, Granda-Gutierrez EMA, Diaz-Ramirez J, Garza-Reyes JA, Kumar A. A systematic literature review of data science, data analytics and machine learning applied to healthcare engineering systems. Manag Decis. 2020.
] Secinaro S, Calandra D, Secinaro A, Muthurangu V, Biancone P. The role of artificial intelligence in healthcare: a structured literature review. BMC Med Inf Decis Making. 2021;21(1).
] Stafford IS, Kellermann M, Mossotto E, Beattie RM, MacArthur BD, Ennis S. A systematic review of the applications of artificial intelligence and machine learning in autoimmune diseases. NPJ Digit Med. 2020;3(1):1–11.
] Teng AK, Wilcox AB. A review of predictive analytics solutions for sepsis patients. Appl Clin Inf. 2020;11(03):387–398.
] Toor R, Chana I. Network analysis as a computational technique and its benefaction for predictive analysis of healthcare data: a systematic review. Arch Comput Methods Eng. 2020;28(3):1689–1711.
] Tsang G, Xie X, Zhou S-M. Harnessing the power of machine learning in dementia informatics research: Issues, opportunities, and challenges. Rev Biomed Eng. 2020;13:113–129.
] Waring J, Lindvall C, Umeton R. Automated machine learning: review of the state-of-the-art and opportunities for healthcare. Artif Intell Med. 2020;104:101822.
] Waschkau A, Wilfling D, Steinhäuser J. Are big data analytics helpful in caring for multimorbid patients in general practice?—a scoping review. Family Pract. 2016;20(1).
] Zhang R, Simon G, Yu F. Advancing Alzheimer’s research: a review of big data promises. Int J Med Inf. 2017;106:48–56.
Rights and permissions
Open Access This article is licensed under a Creative Commons Attribution 4.0 International License, which permits use, sharing, adaptation, distribution and reproduction in any medium or format, as long as you give appropriate credit to the original author(s) and the source, provide a link to the Creative Commons licence, and indicate if changes were made. The images or other third party material in this article are included in the article's Creative Commons licence, unless indicated otherwise in a credit line to the material. If material is not included in the article's Creative Commons licence and your intended use is not permitted by statutory regulation or exceeds the permitted use, you will need to obtain permission directly from the copyright holder. To view a copy of this licence, visit http://creativecommons.org/licenses/by/4.0/ .
Reprints and permissions
About this article
Taipalus, T., Isomöttönen, V., Erkkilä, H. et al. Data Analytics in Healthcare: A Tertiary Study. SN COMPUT. SCI. 4 , 87 (2023). https://doi.org/10.1007/s42979-022-01507-0
Download citation
Received : 07 December 2021
Accepted : 14 November 2022
Published : 09 December 2022
DOI : https://doi.org/10.1007/s42979-022-01507-0
Share this article
Anyone you share the following link with will be able to read this content:
Sorry, a shareable link is not currently available for this article.
Provided by the Springer Nature SharedIt content-sharing initiative
- Data analytics
- Machine learning
- Data mining
- Artificial intelligence
- Find a journal
- Publish with us
- Track your research
- Open access
- Published: 06 January 2022
The use of Big Data Analytics in healthcare
- Kornelia Batko ORCID: orcid.org/0000-0001-6561-3826 1 &
- Andrzej Ślęzak 2
Journal of Big Data volume 9 , Article number: 3 ( 2022 ) Cite this article
82k Accesses
148 Citations
28 Altmetric
Metrics details
The introduction of Big Data Analytics (BDA) in healthcare will allow to use new technologies both in treatment of patients and health management. The paper aims at analyzing the possibilities of using Big Data Analytics in healthcare. The research is based on a critical analysis of the literature, as well as the presentation of selected results of direct research on the use of Big Data Analytics in medical facilities. The direct research was carried out based on research questionnaire and conducted on a sample of 217 medical facilities in Poland. Literature studies have shown that the use of Big Data Analytics can bring many benefits to medical facilities, while direct research has shown that medical facilities in Poland are moving towards data-based healthcare because they use structured and unstructured data, reach for analytics in the administrative, business and clinical area. The research positively confirmed that medical facilities are working on both structural data and unstructured data. The following kinds and sources of data can be distinguished: from databases, transaction data, unstructured content of emails and documents, data from devices and sensors. However, the use of data from social media is lower as in their activity they reach for analytics, not only in the administrative and business but also in the clinical area. It clearly shows that the decisions made in medical facilities are highly data-driven. The results of the study confirm what has been analyzed in the literature that medical facilities are moving towards data-based healthcare, together with its benefits.
Introduction
The main contribution of this paper is to present an analytical overview of using structured and unstructured data (Big Data) analytics in medical facilities in Poland. Medical facilities use both structured and unstructured data in their practice. Structured data has a predetermined schema, it is extensive, freeform, and comes in variety of forms [ 27 ]. In contrast, unstructured data, referred to as Big Data (BD), does not fit into the typical data processing format. Big Data is a massive amount of data sets that cannot be stored, processed, or analyzed using traditional tools. It remains stored but not analyzed. Due to the lack of a well-defined schema, it is difficult to search and analyze such data and, therefore, it requires a specific technology and method to transform it into value [ 20 , 68 ]. Integrating data stored in both structured and unstructured formats can add significant value to an organization [ 27 ]. Organizations must approach unstructured data in a different way. Therefore, the potential is seen in Big Data Analytics (BDA). Big Data Analytics are techniques and tools used to analyze and extract information from Big Data. The results of Big Data analysis can be used to predict the future. They also help in creating trends about the past. When it comes to healthcare, it allows to analyze large datasets from thousands of patients, identifying clusters and correlation between datasets, as well as developing predictive models using data mining techniques [ 60 ].
This paper is the first study to consolidate and characterize the use of Big Data from different perspectives. The first part consists of a brief literature review of studies on Big Data (BD) and Big Data Analytics (BDA), while the second part presents results of direct research aimed at diagnosing the use of big data analyses in medical facilities in Poland.
Healthcare is a complex system with varied stakeholders: patients, doctors, hospitals, pharmaceutical companies and healthcare decision-makers. This sector is also limited by strict rules and regulations. However, worldwide one may observe a departure from the traditional doctor-patient approach. The doctor becomes a partner and the patient is involved in the therapeutic process [ 14 ]. Healthcare is no longer focused solely on the treatment of patients. The priority for decision-makers should be to promote proper health attitudes and prevent diseases that can be avoided [ 81 ]. This became visible and important especially during the Covid-19 pandemic [ 44 ].
The next challenges that healthcare will have to face is the growing number of elderly people and a decline in fertility. Fertility rates in the country are found below the reproductive minimum necessary to keep the population stable [ 10 ]. The reflection of both effects, namely the increase in age and lower fertility rates, are demographic load indicators, which is constantly growing. Forecasts show that providing healthcare in the form it is provided today will become impossible in the next 20 years [ 70 ]. It is especially visible now during the Covid-19 pandemic when healthcare faced quite a challenge related to the analysis of huge data amounts and the need to identify trends and predict the spread of the coronavirus. The pandemic showed it even more that patients should have access to information about their health condition, the possibility of digital analysis of this data and access to reliable medical support online. Health monitoring and cooperation with doctors in order to prevent diseases can actually revolutionize the healthcare system. One of the most important aspects of the change necessary in healthcare is putting the patient in the center of the system.
Technology is not enough to achieve these goals. Therefore, changes should be made not only at the technological level but also in the management and design of complete healthcare processes and what is more, they should affect the business models of service providers. The use of Big Data Analytics is becoming more and more common in enterprises [ 17 , 54 ]. However, medical enterprises still cannot keep up with the information needs of patients, clinicians, administrators and the creator’s policy. The adoption of a Big Data approach would allow the implementation of personalized and precise medicine based on personalized information, delivered in real time and tailored to individual patients.
To achieve this goal, it is necessary to implement systems that will be able to learn quickly about the data generated by people within clinical care and everyday life. This will enable data-driven decision making, receiving better personalized predictions about prognosis and responses to treatments; a deeper understanding of the complex factors and their interactions that influence health at the patient level, the health system and society, enhanced approaches to detecting safety problems with drugs and devices, as well as more effective methods of comparing prevention, diagnostic, and treatment options [ 40 ].
In the literature, there is a lot of research showing what opportunities can be offered to companies by big data analysis and what data can be analyzed. However, there are few studies showing how data analysis in the area of healthcare is performed, what data is used by medical facilities and what analyses and in which areas they carry out. This paper aims to fill this gap by presenting the results of research carried out in medical facilities in Poland. The goal is to analyze the possibilities of using Big Data Analytics in healthcare, especially in Polish conditions. In particular, the paper is aimed at determining what data is processed by medical facilities in Poland, what analyses they perform and in what areas, and how they assess their analytical maturity. In order to achieve this goal, a critical analysis of the literature was performed, and the direct research was based on a research questionnaire conducted on a sample of 217 medical facilities in Poland. It was hypothesized that medical facilities in Poland are working on both structured and unstructured data and moving towards data-based healthcare and its benefits. Examining the maturity of healthcare facilities in the use of Big Data and Big Data Analytics is crucial in determining the potential future benefits that the healthcare sector can gain from Big Data Analytics. There is also a pressing need to predicate whether, in the coming years, healthcare will be able to cope with the threats and challenges it faces.
This paper is divided into eight parts. The first is the introduction which provides background and the general problem statement of this research. In the second part, this paper discusses considerations on use of Big Data and Big Data Analytics in Healthcare, and then, in the third part, it moves on to challenges and potential benefits of using Big Data Analytics in healthcare. The next part involves the explanation of the proposed method. The result of direct research and discussion are presented in the fifth part, while the following part of the paper is the conclusion. The seventh part of the paper presents practical implications. The final section of the paper provides limitations and directions for future research.
Considerations on use Big Data and Big Data Analytics in the healthcare
In recent years one can observe a constantly increasing demand for solutions offering effective analytical tools. This trend is also noticeable in the analysis of large volumes of data (Big Data, BD). Organizations are looking for ways to use the power of Big Data to improve their decision making, competitive advantage or business performance [ 7 , 54 ]. Big Data is considered to offer potential solutions to public and private organizations, however, still not much is known about the outcome of the practical use of Big Data in different types of organizations [ 24 ].
As already mentioned, in recent years, healthcare management worldwide has been changed from a disease-centered model to a patient-centered model, even in value-based healthcare delivery model [ 68 ]. In order to meet the requirements of this model and provide effective patient-centered care, it is necessary to manage and analyze healthcare Big Data.
The issue often raised when it comes to the use of data in healthcare is the appropriate use of Big Data. Healthcare has always generated huge amounts of data and nowadays, the introduction of electronic medical records, as well as the huge amount of data sent by various types of sensors or generated by patients in social media causes data streams to constantly grow. Also, the medical industry generates significant amounts of data, including clinical records, medical images, genomic data and health behaviors. Proper use of the data will allow healthcare organizations to support clinical decision-making, disease surveillance, and public health management. The challenge posed by clinical data processing involves not only the quantity of data but also the difficulty in processing it.
In the literature one can find many different definitions of Big Data. This concept has evolved in recent years, however, it is still not clearly understood. Nevertheless, despite the range and differences in definitions, Big Data can be treated as a: large amount of digital data, large data sets, tool, technology or phenomenon (cultural or technological.
Big Data can be considered as massive and continually generated digital datasets that are produced via interactions with online technologies [ 53 ]. Big Data can be defined as datasets that are of such large sizes that they pose challenges in traditional storage and analysis techniques [ 28 ]. A similar opinion about Big Data was presented by Ohlhorst who sees Big Data as extremely large data sets, possible neither to manage nor to analyze with traditional data processing tools [ 57 ]. In his opinion, the bigger the data set, the more difficult it is to gain any value from it.
In turn, Knapp perceived Big Data as tools, processes and procedures that allow an organization to create, manipulate and manage very large data sets and storage facilities [ 38 ]. From this point of view, Big Data is identified as a tool to gather information from different databases and processes, allowing users to manage large amounts of data.
Similar perception of the term ‘Big Data’ is shown by Carter. According to him, Big Data technologies refer to a new generation of technologies and architectures, designed to economically extract value from very large volumes of a wide variety of data by enabling high velocity capture, discovery and/or analysis [ 13 ].
Jordan combines these two approaches by identifying Big Data as a complex system, as it needs data bases for data to be stored in, programs and tools to be managed, as well as expertise and personnel able to retrieve useful information and visualization to be understood [ 37 ].
Following the definition of Laney for Big Data, it can be state that: it is large amount of data generated in very fast motion and it contains a lot of content [ 43 ]. Such data comes from unstructured sources, such as stream of clicks on the web, social networks (Twitter, blogs, Facebook), video recordings from the shops, recording of calls in a call center, real time information from various kinds of sensors, RFID, GPS devices, mobile phones and other devices that identify and monitor something [ 8 ]. Big Data is a powerful digital data silo, raw, collected with all sorts of sources, unstructured and difficult, or even impossible, to analyze using conventional techniques used so far to relational databases.
While describing Big Data, it cannot be overlooked that the term refers more to a phenomenon than to specific technology. Therefore, instead of defining this phenomenon, trying to describe them, more authors are describing Big Data by giving them characteristics included a collection of V’s related to its nature [ 2 , 3 , 23 , 25 , 58 ]:
Volume (refers to the amount of data and is one of the biggest challenges in Big Data Analytics),
Velocity (speed with which new data is generated, the challenge is to be able to manage data effectively and in real time),
Variety (heterogeneity of data, many different types of healthcare data, the challenge is to derive insights by looking at all available heterogenous data in a holistic manner),
Variability (inconsistency of data, the challenge is to correct the interpretation of data that can vary significantly depending on the context),
Veracity (how trustworthy the data is, quality of the data),
Visualization (ability to interpret data and resulting insights, challenging for Big Data due to its other features as described above).
Value (the goal of Big Data Analytics is to discover the hidden knowledge from huge amounts of data).
Big Data is defined as an information asset with high volume, velocity, and variety, which requires specific technology and method for its transformation into value [ 21 , 77 ]. Big Data is also a collection of information about high-volume, high volatility or high diversity, requiring new forms of processing in order to support decision-making, discovering new phenomena and process optimization [ 5 , 7 ]. Big Data is too large for traditional data-processing systems and software tools to capture, store, manage and analyze, therefore it requires new technologies [ 28 , 50 , 61 ] to manage (capture, aggregate, process) its volume, velocity and variety [ 9 ].
Undoubtedly, Big Data differs from the data sources used so far by organizations. Therefore, organizations must approach this type of unstructured data in a different way. First of all, organizations must start to see data as flows and not stocks—this entails the need to implement the so-called streaming analytics [ 48 ]. The mentioned features make it necessary to use new IT tools that allow the fullest use of new data [ 58 ]. The Big Data idea, inseparable from the huge increase in data available to various organizations or individuals, creates opportunities for access to valuable analyses, conclusions and enables making more accurate decisions [ 6 , 11 , 59 ].
The Big Data concept is constantly evolving and currently it does not focus on huge amounts of data, but rather on the process of creating value from this data [ 52 ]. Big Data is collected from various sources that have different data properties and are processed by different organizational units, resulting in creation of a Big Data chain [ 36 ]. The aim of the organizations is to manage, process and analyze Big Data. In the healthcare sector, Big Data streams consist of various types of data, namely [ 8 , 51 ]:
clinical data, i.e. data obtained from electronic medical records, data from hospital information systems, image centers, laboratories, pharmacies and other organizations providing health services, patient generated health data, physician’s free-text notes, genomic data, physiological monitoring data [ 4 ],
biometric data provided from various types of devices that monitor weight, pressure, glucose level, etc.,
financial data, constituting a full record of economic operations reflecting the conducted activity,
data from scientific research activities, i.e. results of research, including drug research, design of medical devices and new methods of treatment,
data provided by patients, including description of preferences, level of satisfaction, information from systems for self-monitoring of their activity: exercises, sleep, meals consumed, etc.
data from social media.
These data are provided not only by patients but also by organizations and institutions, as well as by various types of monitoring devices, sensors or instruments [ 16 ]. Data that has been generated so far in the healthcare sector is stored in both paper and digital form. Thus, the essence and the specificity of the process of Big Data analyses means that organizations need to face new technological and organizational challenges [ 67 ]. The healthcare sector has always generated huge amounts of data and this is connected, among others, with the need to store medical records of patients. However, the problem with Big Data in healthcare is not limited to an overwhelming volume but also an unprecedented diversity in terms of types, data formats and speed with which it should be analyzed in order to provide the necessary information on an ongoing basis [ 3 ]. It is also difficult to apply traditional tools and methods for management of unstructured data [ 67 ]. Due to the diversity and quantity of data sources that are growing all the time, advanced analytical tools and technologies, as well as Big Data analysis methods which can meet and exceed the possibilities of managing healthcare data, are needed [ 3 , 68 ].
Therefore, the potential is seen in Big Data analyses, especially in the aspect of improving the quality of medical care, saving lives or reducing costs [ 30 ]. Extracting from this tangle of given association rules, patterns and trends will allow health service providers and other stakeholders in the healthcare sector to offer more accurate and more insightful diagnoses of patients, personalized treatment, monitoring of the patients, preventive medicine, support of medical research and health population, as well as better quality of medical services and patient care while, at the same time, the ability to reduce costs (Fig. 1 ).
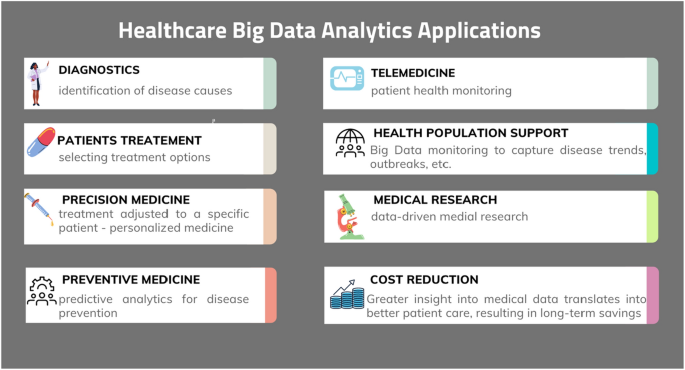
(Source: Own elaboration)
Healthcare Big Data Analytics applications
The main challenge with Big Data is how to handle such a large amount of information and use it to make data-driven decisions in plenty of areas [ 64 ]. In the context of healthcare data, another major challenge is to adjust big data storage, analysis, presentation of analysis results and inference basing on them in a clinical setting. Data analytics systems implemented in healthcare are designed to describe, integrate and present complex data in an appropriate way so that it can be understood better (Fig. 2 ). This would improve the efficiency of acquiring, storing, analyzing and visualizing big data from healthcare [ 71 ].
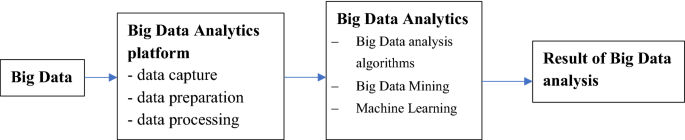
Process of Big Data Analytics
The result of data processing with the use of Big Data Analytics is appropriate data storytelling which may contribute to making decisions with both lower risk and data support. This, in turn, can benefit healthcare stakeholders. To take advantage of the potential massive amounts of data in healthcare and to ensure that the right intervention to the right patient is properly timed, personalized, and potentially beneficial to all components of the healthcare system such as the payer, patient, and management, analytics of large datasets must connect communities involved in data analytics and healthcare informatics [ 49 ]. Big Data Analytics can provide insight into clinical data and thus facilitate informed decision-making about the diagnosis and treatment of patients, prevention of diseases or others. Big Data Analytics can also improve the efficiency of healthcare organizations by realizing the data potential [ 3 , 62 ].
Big Data Analytics in medicine and healthcare refers to the integration and analysis of a large amount of complex heterogeneous data, such as various omics (genomics, epigenomics, transcriptomics, proteomics, metabolomics, interactomics, pharmacogenetics, deasomics), biomedical data, talemedicine data (sensors, medical equipment data) and electronic health records data [ 46 , 65 ].
When analyzing the phenomenon of Big Data in the healthcare sector, it should be noted that it can be considered from the point of view of three areas: epidemiological, clinical and business.
From a clinical point of view, the Big Data analysis aims to improve the health and condition of patients, enable long-term predictions about their health status and implementation of appropriate therapeutic procedures. Ultimately, the use of data analysis in medicine is to allow the adaptation of therapy to a specific patient, that is personalized medicine (precision, personalized medicine).
From an epidemiological point of view, it is desirable to obtain an accurate prognosis of morbidity in order to implement preventive programs in advance.
In the business context, Big Data analysis may enable offering personalized packages of commercial services or determining the probability of individual disease and infection occurrence. It is worth noting that Big Data means not only the collection and processing of data but, most of all, the inference and visualization of data necessary to obtain specific business benefits.
In order to introduce new management methods and new solutions in terms of effectiveness and transparency, it becomes necessary to make data more accessible, digital, searchable, as well as analyzed and visualized.
Erickson and Rothberg state that the information and data do not reveal their full value until insights are drawn from them. Data becomes useful when it enhances decision making and decision making is enhanced only when analytical techniques are used and an element of human interaction is applied [ 22 ].
Thus, healthcare has experienced much progress in usage and analysis of data. A large-scale digitalization and transparency in this sector is a key statement of almost all countries governments policies. For centuries, the treatment of patients was based on the judgment of doctors who made treatment decisions. In recent years, however, Evidence-Based Medicine has become more and more important as a result of it being related to the systematic analysis of clinical data and decision-making treatment based on the best available information [ 42 ]. In the healthcare sector, Big Data Analytics is expected to improve the quality of life and reduce operational costs [ 72 , 82 ]. Big Data Analytics enables organizations to improve and increase their understanding of the information contained in data. It also helps identify data that provides insightful insights for current as well as future decisions [ 28 ].
Big Data Analytics refers to technologies that are grounded mostly in data mining: text mining, web mining, process mining, audio and video analytics, statistical analysis, network analytics, social media analytics and web analytics [ 16 , 25 , 31 ]. Different data mining techniques can be applied on heterogeneous healthcare data sets, such as: anomaly detection, clustering, classification, association rules as well as summarization and visualization of those Big Data sets [ 65 ]. Modern data analytics techniques explore and leverage unique data characteristics even from high-speed data streams and sensor data [ 15 , 16 , 31 , 55 ]. Big Data can be used, for example, for better diagnosis in the context of comprehensive patient data, disease prevention and telemedicine (in particular when using real-time alerts for immediate care), monitoring patients at home, preventing unnecessary hospital visits, integrating medical imaging for a wider diagnosis, creating predictive analytics, reducing fraud and improving data security, better strategic planning and increasing patients’ involvement in their own health.
Big Data Analytics in healthcare can be divided into [ 33 , 73 , 74 ]:
descriptive analytics in healthcare is used to understand past and current healthcare decisions, converting data into useful information for understanding and analyzing healthcare decisions, outcomes and quality, as well as making informed decisions [ 33 ]. It can be used to create reports (i.e. about patients’ hospitalizations, physicians’ performance, utilization management), visualization, customized reports, drill down tables, or running queries on the basis of historical data.
predictive analytics operates on past performance in an effort to predict the future by examining historical or summarized health data, detecting patterns of relationships in these data, and then extrapolating these relationships to forecast. It can be used to i.e. predict the response of different patient groups to different drugs (dosages) or reactions (clinical trials), anticipate risk and find relationships in health data and detect hidden patterns [ 62 ]. In this way, it is possible to predict the epidemic spread, anticipate service contracts and plan healthcare resources. Predictive analytics is used in proper diagnosis and for appropriate treatments to be given to patients suffering from certain diseases [ 39 ].
prescriptive analytics—occurs when health problems involve too many choices or alternatives. It uses health and medical knowledge in addition to data or information. Prescriptive analytics is used in many areas of healthcare, including drug prescriptions and treatment alternatives. Personalized medicine and evidence-based medicine are both supported by prescriptive analytics.
discovery analytics—utilizes knowledge about knowledge to discover new “inventions” like drugs (drug discovery), previously unknown diseases and medical conditions, alternative treatments, etc.
Although the models and tools used in descriptive, predictive, prescriptive, and discovery analytics are different, many applications involve all four of them [ 62 ]. Big Data Analytics in healthcare can help enable personalized medicine by identifying optimal patient-specific treatments. This can influence the improvement of life standards, reduce waste of healthcare resources and save costs of healthcare [ 56 , 63 , 71 ]. The introduction of large data analysis gives new analytical possibilities in terms of scope, flexibility and visualization. Techniques such as data mining (computational pattern discovery process in large data sets) facilitate inductive reasoning and analysis of exploratory data, enabling scientists to identify data patterns that are independent of specific hypotheses. As a result, predictive analysis and real-time analysis becomes possible, making it easier for medical staff to start early treatments and reduce potential morbidity and mortality. In addition, document analysis, statistical modeling, discovering patterns and topics in document collections and data in the EHR, as well as an inductive approach can help identify and discover relationships between health phenomena.
Advanced analytical techniques can be used for a large amount of existing (but not yet analytical) data on patient health and related medical data to achieve a better understanding of the information and results obtained, as well as to design optimal clinical pathways [ 62 ]. Big Data Analytics in healthcare integrates analysis of several scientific areas such as bioinformatics, medical imaging, sensor informatics, medical informatics and health informatics [ 65 ]. Big Data Analytics in healthcare allows to analyze large datasets from thousands of patients, identifying clusters and correlation between datasets, as well as developing predictive models using data mining techniques [ 65 ]. Discussing all the techniques used for Big Data Analytics goes beyond the scope of a single article [ 25 ].
The success of Big Data analysis and its accuracy depend heavily on the tools and techniques used to analyze the ability to provide reliable, up-to-date and meaningful information to various stakeholders [ 12 ]. It is believed that the implementation of big data analytics by healthcare organizations could bring many benefits in the upcoming years, including lowering health care costs, better diagnosis and prediction of diseases and their spread, improving patient care and developing protocols to prevent re-hospitalization, optimizing staff, optimizing equipment, forecasting the need for hospital beds, operating rooms, treatments, and improving the drug supply chain [ 71 ].
Challenges and potential benefits of using Big Data Analytics in healthcare
Modern analytics gives possibilities not only to have insight in historical data, but also to have information necessary to generate insight into what may happen in the future. Even when it comes to prediction of evidence-based actions. The emphasis on reform has prompted payers and suppliers to pursue data analysis to reduce risk, detect fraud, improve efficiency and save lives. Everyone—payers, providers, even patients—are focusing on doing more with fewer resources. Thus, some areas in which enhanced data and analytics can yield the greatest results include various healthcare stakeholders (Table 1 ).
Healthcare organizations see the opportunity to grow through investments in Big Data Analytics. In recent years, by collecting medical data of patients, converting them into Big Data and applying appropriate algorithms, reliable information has been generated that helps patients, physicians and stakeholders in the health sector to identify values and opportunities [ 31 ]. It is worth noting that there are many changes and challenges in the structure of the healthcare sector. Digitization and effective use of Big Data in healthcare can bring benefits to every stakeholder in this sector. A single doctor would benefit the same as the entire healthcare system. Potential opportunities to achieve benefits and effects from Big Data in healthcare can be divided into four groups [ 8 ]:
Improving the quality of healthcare services:
assessment of diagnoses made by doctors and the manner of treatment of diseases indicated by them based on the decision support system working on Big Data collections,
detection of more effective, from a medical point of view, and more cost-effective ways to diagnose and treat patients,
analysis of large volumes of data to reach practical information useful for identifying needs, introducing new health services, preventing and overcoming crises,
prediction of the incidence of diseases,
detecting trends that lead to an improvement in health and lifestyle of the society,
analysis of the human genome for the introduction of personalized treatment.
Supporting the work of medical personnel
doctors’ comparison of current medical cases to cases from the past for better diagnosis and treatment adjustment,
detection of diseases at earlier stages when they can be more easily and quickly cured,
detecting epidemiological risks and improving control of pathogenic spots and reaction rates,
identification of patients who are predicted to have the highest risk of specific, life-threatening diseases by collating data on the history of the most common diseases, in healing people with reports entering insurance companies,
health management of each patient individually (personalized medicine) and health management of the whole society,
capturing and analyzing large amounts of data from hospitals and homes in real time, life monitoring devices to monitor safety and predict adverse events,
analysis of patient profiles to identify people for whom prevention should be applied, lifestyle change or preventive care approach,
the ability to predict the occurrence of specific diseases or worsening of patients’ results,
predicting disease progression and its determinants, estimating the risk of complications,
detecting drug interactions and their side effects.
Supporting scientific and research activity
supporting work on new drugs and clinical trials thanks to the possibility of analyzing “all data” instead of selecting a test sample,
the ability to identify patients with specific, biological features that will take part in specialized clinical trials,
selecting a group of patients for which the tested drug is likely to have the desired effect and no side effects,
using modeling and predictive analysis to design better drugs and devices.
Business and management
reduction of costs and counteracting abuse and counseling practices,
faster and more effective identification of incorrect or unauthorized financial operations in order to prevent abuse and eliminate errors,
increase in profitability by detecting patients generating high costs or identifying doctors whose work, procedures and treatment methods cost the most and offering them solutions that reduce the amount of money spent,
identification of unnecessary medical activities and procedures, e.g. duplicate tests.
According to research conducted by Wang, Kung and Byrd, Big Data Analytics benefits can be classified into five categories: IT infrastructure benefits (reducing system redundancy, avoiding unnecessary IT costs, transferring data quickly among healthcare IT systems, better use of healthcare systems, processing standardization among various healthcare IT systems, reducing IT maintenance costs regarding data storage), operational benefits (improving the quality and accuracy of clinical decisions, processing a large number of health records in seconds, reducing the time of patient travel, immediate access to clinical data to analyze, shortening the time of diagnostic test, reductions in surgery-related hospitalizations, exploring inconceivable new research avenues), organizational benefits (detecting interoperability problems much more quickly than traditional manual methods, improving cross-functional communication and collaboration among administrative staffs, researchers, clinicians and IT staffs, enabling data sharing with other institutions and adding new services, content sources and research partners), managerial benefits (gaining quick insights about changing healthcare trends in the market, providing members of the board and heads of department with sound decision-support information on the daily clinical setting, optimizing business growth-related decisions) and strategic benefits (providing a big picture view of treatment delivery for meeting future need, creating high competitive healthcare services) [ 73 ].
The above specification does not constitute a full list of potential areas of use of Big Data Analysis in healthcare because the possibilities of using analysis are practically unlimited. In addition, advanced analytical tools allow to analyze data from all possible sources and conduct cross-analyses to provide better data insights [ 26 ]. For example, a cross-analysis can refer to a combination of patient characteristics, as well as costs and care results that can help identify the best, in medical terms, and the most cost-effective treatment or treatments and this may allow a better adjustment of the service provider’s offer [ 62 ].
In turn, the analysis of patient profiles (e.g. segmentation and predictive modeling) allows identification of people who should be subject to prophylaxis, prevention or should change their lifestyle [ 8 ]. Shortened list of benefits for Big Data Analytics in healthcare is presented in paper [ 3 ] and consists of: better performance, day-to-day guides, detection of diseases in early stages, making predictive analytics, cost effectiveness, Evidence Based Medicine and effectiveness in patient treatment.
Summarizing, healthcare big data represents a huge potential for the transformation of healthcare: improvement of patients’ results, prediction of outbreaks of epidemics, valuable insights, avoidance of preventable diseases, reduction of the cost of healthcare delivery and improvement of the quality of life in general [ 1 ]. Big Data also generates many challenges such as difficulties in data capture, data storage, data analysis and data visualization [ 15 ]. The main challenges are connected with the issues of: data structure (Big Data should be user-friendly, transparent, and menu-driven but it is fragmented, dispersed, rarely standardized and difficult to aggregate and analyze), security (data security, privacy and sensitivity of healthcare data, there are significant concerns related to confidentiality), data standardization (data is stored in formats that are not compatible with all applications and technologies), storage and transfers (especially costs associated with securing, storing, and transferring unstructured data), managerial skills, such as data governance, lack of appropriate analytical skills and problems with Real-Time Analytics (health care is to be able to utilize Big Data in real time) [ 4 , 34 , 41 ].
The research is based on a critical analysis of the literature, as well as the presentation of selected results of direct research on the use of Big Data Analytics in medical facilities in Poland.
Presented research results are part of a larger questionnaire form on Big Data Analytics. The direct research was based on an interview questionnaire which contained 100 questions with 5-point Likert scale (1—strongly disagree, 2—I rather disagree, 3—I do not agree, nor disagree, 4—I rather agree, 5—I definitely agree) and 4 metrics questions. The study was conducted in December 2018 on a sample of 217 medical facilities (110 private, 107 public). The research was conducted by a specialized market research agency: Center for Research and Expertise of the University of Economics in Katowice.
When it comes to direct research, the selected entities included entities financed from public sources—the National Health Fund (23.5%), and entities operating commercially (11.5%). In the surveyed group of entities, more than a half (64.9%) are hybrid financed, both from public and commercial sources. The diversity of the research sample also applies to the size of the entities, defined by the number of employees. Taking into account proportions of the surveyed entities, it should be noted that in the sector structure, medium-sized (10–50 employees—34% of the sample) and large (51–250 employees—27%) entities dominate. The research was of all-Poland nature, and the entities included in the research sample come from all of the voivodships. The largest group were entities from Łódzkie (32%), Śląskie (18%) and Mazowieckie (18%) voivodships, as these voivodships have the largest number of medical institutions. Other regions of the country were represented by single units. The selection of the research sample was random—layered. As part of medical facilities database, groups of private and public medical facilities have been identified and the ones to which the questionnaire was targeted were drawn from each of these groups. The analyses were performed using the GNU PSPP 0.10.2 software.
The aim of the study was to determine whether medical facilities in Poland use Big Data Analytics and if so, in which areas. Characteristics of the research sample is presented in Table 2 .
The research is non-exhaustive due to the incomplete and uneven regional distribution of the samples, overrepresented in three voivodeships (Łódzkie, Mazowieckie and Śląskie). The size of the research sample (217 entities) allows the authors of the paper to formulate specific conclusions on the use of Big Data in the process of its management.
For the purpose of this paper, the following research hypotheses were formulated: (1) medical facilities in Poland are working on both structured and unstructured data (2) medical facilities in Poland are moving towards data-based healthcare and its benefits.
The paper poses the following research questions and statements that coincide with the selected questions from the research questionnaire:
From what sources do medical facilities obtain data? What types of data are used by the particular organization, whether structured or unstructured, and to what extent?
From what sources do medical facilities obtain data?
In which area organizations are using data and analytical systems (clinical or business)?
Is data analytics performed based on historical data or are predictive analyses also performed?
Determining whether administrative and medical staff receive complete, accurate and reliable data in a timely manner?
Determining whether real-time analyses are performed to support the particular organization’s activities.
Results and discussion
On the basis of the literature analysis and research study, a set of questions and statements related to the researched area was formulated. The results from the surveys show that medical facilities use a variety of data sources in their operations. These sources are both structured and unstructured data (Table 3 ).
According to the data provided by the respondents, considering the first statement made in the questionnaire, almost half of the medical institutions (47.58%) agreed that they rather collect and use structured data (e.g. databases and data warehouses, reports to external entities) and 10.57% entirely agree with this statement. As much as 23.35% of representatives of medical institutions stated “I agree or disagree”. Other medical facilities do not collect and use structured data (7.93%) and 6.17% strongly disagree with the first statement. Also, the median calculated based on the obtained results (median: 4), proves that medical facilities in Poland collect and use structured data (Table 4 ).
In turn, 28.19% of the medical institutions agreed that they rather collect and use unstructured data and as much as 9.25% entirely agree with this statement. The number of representatives of medical institutions that stated “I agree or disagree” was 27.31%. Other medical facilities do not collect and use structured data (17.18%) and 13.66% strongly disagree with the first statement. In the case of unstructured data the median is 3, which means that the collection and use of this type of data by medical facilities in Poland is lower.
In the further part of the analysis, it was checked whether the size of the medical facility and form of ownership have an impact on whether it analyzes unstructured data (Tables 4 and 5 ). In order to find this out, correlation coefficients were calculated.
Based on the calculations, it can be concluded that there is a small statistically monotonic correlation between the size of the medical facility and its collection and use of structured data (p < 0.001; τ = 0.16). This means that the use of structured data is slightly increasing in larger medical facilities. The size of the medical facility is more important according to use of unstructured data (p < 0.001; τ = 0.23) (Table 4 .).
To determine whether the form of medical facility ownership affects data collection, the Mann–Whitney U test was used. The calculations show that the form of ownership does not affect what data the organization collects and uses (Table 5 ).
Detailed information on the sources of from which medical facilities collect and use data is presented in the Table 6 .
The questionnaire results show that medical facilities are especially using information published in databases, reports to external units and transaction data, but they also use unstructured data from e-mails, medical devices, sensors, phone calls, audio and video data (Table 6 ). Data from social media, RFID and geolocation data are used to a small extent. Similar findings are concluded in the literature studies.
From the analysis of the answers given by the respondents, more than half of the medical facilities have integrated hospital system (HIS) implemented. As much as 43.61% use integrated hospital system and 16.30% use it extensively (Table 7 ). 19.38% of exanimated medical facilities do not use it at all. Moreover, most of the examined medical facilities (34.80% use it, 32.16% use extensively) conduct medical documentation in an electronic form, which gives an opportunity to use data analytics. Only 4.85% of medical facilities don’t use it at all.
Other problems that needed to be investigated were: whether medical facilities in Poland use data analytics? If so, in what form and in what areas? (Table 8 ). The analysis of answers given by the respondents about the potential of data analytics in medical facilities shows that a similar number of medical facilities use data analytics in administration and business (31.72% agreed with the statement no. 5 and 12.33% strongly agreed) as in the clinical area (33.04% agreed with the statement no. 6 and 12.33% strongly agreed). When considering decision-making issues, 35.24% agree with the statement "the organization uses data and analytical systems to support business decisions” and 8.37% of respondents strongly agree. Almost 40.09% agree with the statement that “the organization uses data and analytical systems to support clinical decisions (in the field of diagnostics and therapy)” and 15.42% of respondents strongly agree. Exanimated medical facilities use in their activity analytics based both on historical data (33.48% agree with statement 7 and 12.78% strongly agree) and predictive analytics (33.04% agrees with the statement number 8 and 15.86% strongly agree). Detailed results are presented in Table 8 .
Medical facilities focus on development in the field of data processing, as they confirm that they conduct analytical planning processes systematically and analyze new opportunities for strategic use of analytics in business and clinical activities (38.33% rather agree and 10.57% strongly agree with this statement). The situation is different with real-time data analysis, here, the situation is not so optimistic. Only 28.19% rather agree and 14.10% strongly agree with the statement that real-time analyses are performed to support an organization’s activities.
When considering whether a facility’s performance in the clinical area depends on the form of ownership, it can be concluded that taking the average and the Mann–Whitney U test depends. A higher degree of use of analyses in the clinical area can be observed in public institutions.
Whether a medical facility performs a descriptive or predictive analysis do not depend on the form of ownership (p > 0.05). It can be concluded that when analyzing the mean and median, they are higher in public facilities, than in private ones. What is more, the Mann–Whitney U test shows that these variables are dependent from each other (p < 0.05) (Table 9 ).
When considering whether a facility’s performance in the clinical area depends on its size, it can be concluded that taking the Kendall’s Tau (τ) it depends (p < 0.001; τ = 0.22), and the correlation is weak but statistically important. This means that the use of data and analytical systems to support clinical decisions (in the field of diagnostics and therapy) increases with the increase of size of the medical facility. A similar relationship, but even less powerful, can be found in the use of descriptive and predictive analyses (Table 10 ).
Considering the results of research in the area of analytical maturity of medical facilities, 8.81% of medical facilities stated that they are at the first level of maturity, i.e. an organization has developed analytical skills and does not perform analyses. As much as 13.66% of medical facilities confirmed that they have poor analytical skills, while 38.33% of the medical facility has located itself at level 3, meaning that “there is a lot to do in analytics”. On the other hand, 28.19% believe that analytical capabilities are well developed and 6.61% stated that analytics are at the highest level and the analytical capabilities are very well developed. Detailed data is presented in Table 11 . Average amounts to 3.11 and Median to 3.
The results of the research have enabled the formulation of following conclusions. Medical facilities in Poland are working on both structured and unstructured data. This data comes from databases, transactions, unstructured content of emails and documents, devices and sensors. However, the use of data from social media is smaller. In their activity, they reach for analytics in the administrative and business, as well as in the clinical area. Also, the decisions made are largely data-driven.
In summary, analysis of the literature that the benefits that medical facilities can get using Big Data Analytics in their activities relate primarily to patients, physicians and medical facilities. It can be confirmed that: patients will be better informed, will receive treatments that will work for them, will have prescribed medications that work for them and not be given unnecessary medications [ 78 ]. Physician roles will likely change to more of a consultant than decision maker. They will advise, warn, and help individual patients and have more time to form positive and lasting relationships with their patients in order to help people. Medical facilities will see changes as well, for example in fewer unnecessary hospitalizations, resulting initially in less revenue, but after the market adjusts, also the accomplishment [ 78 ]. The use of Big Data Analytics can literally revolutionize the way healthcare is practiced for better health and disease reduction.
The analysis of the latest data reveals that data analytics increase the accuracy of diagnoses. Physicians can use predictive algorithms to help them make more accurate diagnoses [ 45 ]. Moreover, it could be helpful in preventive medicine and public health because with early intervention, many diseases can be prevented or ameliorated [ 29 ]. Predictive analytics also allows to identify risk factors for a given patient, and with this knowledge patients will be able to change their lives what, in turn, may contribute to the fact that population disease patterns may dramatically change, resulting in savings in medical costs. Moreover, personalized medicine is the best solution for an individual patient seeking treatment. It can help doctors decide the exact treatments for those individuals. Better diagnoses and more targeted treatments will naturally lead to increases in good outcomes and fewer resources used, including doctors’ time.
The quantitative analysis of the research carried out and presented in this article made it possible to determine whether medical facilities in Poland use Big Data Analytics and if so, in which areas. Thanks to the results obtained it was possible to formulate the following conclusions. Medical facilities are working on both structured and unstructured data, which comes from databases, transactions, unstructured content of emails and documents, devices and sensors. According to analytics, they reach for analytics in the administrative and business, as well as in the clinical area. It clearly showed that the decisions made are largely data-driven. The results of the study confirm what has been analyzed in the literature. Medical facilities are moving towards data-based healthcare and its benefits.
In conclusion, Big Data Analytics has the potential for positive impact and global implications in healthcare. Future research on the use of Big Data in medical facilities will concern the definition of strategies adopted by medical facilities to promote and implement such solutions, as well as the benefits they gain from the use of Big Data analysis and how the perspectives in this area are seen.
Practical implications
This work sought to narrow the gap that exists in analyzing the possibility of using Big Data Analytics in healthcare. Showing how medical facilities in Poland are doing in this respect is an element that is part of global research carried out in this area, including [ 29 , 32 , 60 ].
Limitations and future directions
The research described in this article does not fully exhaust the questions related to the use of Big Data Analytics in Polish healthcare facilities. Only some of the dimensions characterizing the use of data by medical facilities in Poland have been examined. In order to get the full picture, it would be necessary to examine the results of using structured and unstructured data analytics in healthcare. Future research may examine the benefits that medical institutions achieve as a result of the analysis of structured and unstructured data in the clinical and management areas and what limitations they encounter in these areas. For this purpose, it is planned to conduct in-depth interviews with chosen medical facilities in Poland. These facilities could give additional data for empirical analyses based more on their suggestions. Further research should also include medical institutions from beyond the borders of Poland, enabling international comparative analyses.
Future research in the healthcare field has virtually endless possibilities. These regard the use of Big Data Analytics to diagnose specific conditions [ 47 , 66 , 69 , 76 ], propose an approach that can be used in other healthcare applications and create mechanisms to identify “patients like me” [ 75 , 80 ]. Big Data Analytics could also be used for studies related to the spread of pandemics, the efficacy of covid treatment [ 18 , 79 ], or psychology and psychiatry studies, e.g. emotion recognition [ 35 ].
Availability of data and materials
The datasets for this study are available on request to the corresponding author.
Abouelmehdi K, Beni-Hessane A, Khaloufi H. Big healthcare data: preserving security and privacy. J Big Data. 2018. https://doi.org/10.1186/s40537-017-0110-7 .
Article Google Scholar
Agrawal A, Choudhary A. Health services data: big data analytics for deriving predictive healthcare insights. Health Serv Eval. 2019. https://doi.org/10.1007/978-1-4899-7673-4_2-1 .
Al Mayahi S, Al-Badi A, Tarhini A. Exploring the potential benefits of big data analytics in providing smart healthcare. In: Miraz MH, Excell P, Ware A, Ali M, Soomro S, editors. Emerging technologies in computing—first international conference, iCETiC 2018, proceedings (Lecture Notes of the Institute for Computer Sciences, Social-Informatics and Telecommunications Engineering, LNICST). Cham: Springer; 2018. p. 247–58. https://doi.org/10.1007/978-3-319-95450-9_21 .
Bainbridge M. Big data challenges for clinical and precision medicine. In: Househ M, Kushniruk A, Borycki E, editors. Big data, big challenges: a healthcare perspective: background, issues, solutions and research directions. Cham: Springer; 2019. p. 17–31.
Google Scholar
Bartuś K, Batko K, Lorek P. Business intelligence systems: barriers during implementation. In: Jabłoński M, editor. Strategic performance management new concept and contemporary trends. New York: Nova Science Publishers; 2017. p. 299–327. ISBN: 978-1-53612-681-5.
Bartuś K, Batko K, Lorek P. Diagnoza wykorzystania big data w organizacjach-wybrane wyniki badań. Informatyka Ekonomiczna. 2017;3(45):9–20.
Bartuś K, Batko K, Lorek P. Wykorzystanie rozwiązań business intelligence, competitive intelligence i big data w przedsiębiorstwach województwa śląskiego. Przegląd Organizacji. 2018;2:33–9.
Batko K. Możliwości wykorzystania Big Data w ochronie zdrowia. Roczniki Kolegium Analiz Ekonomicznych. 2016;42:267–82.
Bi Z, Cochran D. Big data analytics with applications. J Manag Anal. 2014;1(4):249–65. https://doi.org/10.1080/23270012.2014.992985 .
Boerma T, Requejo J, Victora CG, Amouzou A, Asha G, Agyepong I, Borghi J. Countdown to 2030: tracking progress towards universal coverage for reproductive, maternal, newborn, and child health. Lancet. 2018;391(10129):1538–48.
Bollier D, Firestone CM. The promise and peril of big data. Washington, D.C: Aspen Institute, Communications and Society Program; 2010. p. 1–66.
Bose R. Competitive intelligence process and tools for intelligence analysis. Ind Manag Data Syst. 2008;108(4):510–28.
Carter P. Big data analytics: future architectures, skills and roadmaps for the CIO: in white paper, IDC sponsored by SAS. 2011. p. 1–16.
Castro EM, Van Regenmortel T, Vanhaecht K, Sermeus W, Van Hecke A. Patient empowerment, patient participation and patient-centeredness in hospital care: a concept analysis based on a literature review. Patient Educ Couns. 2016;99(12):1923–39.
Chen H, Chiang RH, Storey VC. Business intelligence and analytics: from big data to big impact. MIS Q. 2012;36(4):1165–88.
Chen CP, Zhang CY. Data-intensive applications, challenges, techniques and technologies: a survey on big data. Inf Sci. 2014;275:314–47.
Chomiak-Orsa I, Mrozek B. Główne perspektywy wykorzystania big data w mediach społecznościowych. Informatyka Ekonomiczna. 2017;3(45):44–54.
Corsi A, de Souza FF, Pagani RN, et al. Big data analytics as a tool for fighting pandemics: a systematic review of literature. J Ambient Intell Hum Comput. 2021;12:9163–80. https://doi.org/10.1007/s12652-020-02617-4 .
Davenport TH, Harris JG. Competing on analytics, the new science of winning. Boston: Harvard Business School Publishing Corporation; 2007.
Davenport TH. Big data at work: dispelling the myths, uncovering the opportunities. Boston: Harvard Business School Publishing; 2014.
De Cnudde S, Martens D. Loyal to your city? A data mining analysis of a public service loyalty program. Decis Support Syst. 2015;73:74–84.
Erickson S, Rothberg H. Data, information, and intelligence. In: Rodriguez E, editor. The analytics process. Boca Raton: Auerbach Publications; 2017. p. 111–26.
Fang H, Zhang Z, Wang CJ, Daneshmand M, Wang C, Wang H. A survey of big data research. IEEE Netw. 2015;29(5):6–9.
Fredriksson C. Organizational knowledge creation with big data. A case study of the concept and practical use of big data in a local government context. 2016. https://www.abo.fi/fakultet/media/22103/fredriksson.pdf .
Gandomi A, Haider M. Beyond the hype: big data concepts, methods, and analytics. Int J Inf Manag. 2015;35(2):137–44.
Groves P, Kayyali B, Knott D, Van Kuiken S. The ‘big data’ revolution in healthcare. Accelerating value and innovation. 2015. http://www.pharmatalents.es/assets/files/Big_Data_Revolution.pdf (Reading: 10.04.2019).
Gupta V, Rathmore N. Deriving business intelligence from unstructured data. Int J Inf Comput Technol. 2013;3(9):971–6.
Gupta V, Singh VK, Ghose U, Mukhija P. A quantitative and text-based characterization of big data research. J Intell Fuzzy Syst. 2019;36:4659–75.
Hampel HOBS, O’Bryant SE, Castrillo JI, Ritchie C, Rojkova K, Broich K, Escott-Price V. PRECISION MEDICINE-the golden gate for detection, treatment and prevention of Alzheimer’s disease. J Prev Alzheimer’s Dis. 2016;3(4):243.
Harerimana GB, Jang J, Kim W, Park HK. Health big data analytics: a technology survey. IEEE Access. 2018;6:65661–78. https://doi.org/10.1109/ACCESS.2018.2878254 .
Hu H, Wen Y, Chua TS, Li X. Toward scalable systems for big data analytics: a technology tutorial. IEEE Access. 2014;2:652–87.
Hussain S, Hussain M, Afzal M, Hussain J, Bang J, Seung H, Lee S. Semantic preservation of standardized healthcare documents in big data. Int J Med Inform. 2019;129:133–45. https://doi.org/10.1016/j.ijmedinf.2019.05.024 .
Islam MS, Hasan MM, Wang X, Germack H. A systematic review on healthcare analytics: application and theoretical perspective of data mining. In: Healthcare. Basel: Multidisciplinary Digital Publishing Institute; 2018. p. 54.
Ismail A, Shehab A, El-Henawy IM. Healthcare analysis in smart big data analytics: reviews, challenges and recommendations. In: Security in smart cities: models, applications, and challenges. Cham: Springer; 2019. p. 27–45.
Jain N, Gupta V, Shubham S, et al. Understanding cartoon emotion using integrated deep neural network on large dataset. Neural Comput Appl. 2021. https://doi.org/10.1007/s00521-021-06003-9 .
Janssen M, van der Voort H, Wahyudi A. Factors influencing big data decision-making quality. J Bus Res. 2017;70:338–45.
Jordan SR. Beneficence and the expert bureaucracy. Public Integr. 2014;16(4):375–94. https://doi.org/10.2753/PIN1099-9922160404 .
Knapp MM. Big data. J Electron Resourc Med Libr. 2013;10(4):215–22.
Koti MS, Alamma BH. Predictive analytics techniques using big data for healthcare databases. In: Smart intelligent computing and applications. New York: Springer; 2019. p. 679–86.
Krumholz HM. Big data and new knowledge in medicine: the thinking, training, and tools needed for a learning health system. Health Aff. 2014;33(7):1163–70.
Kruse CS, Goswamy R, Raval YJ, Marawi S. Challenges and opportunities of big data in healthcare: a systematic review. JMIR Med Inform. 2016;4(4):e38.
Kyoungyoung J, Gang HK. Potentiality of big data in the medical sector: focus on how to reshape the healthcare system. Healthc Inform Res. 2013;19(2):79–85.
Laney D. Application delivery strategies 2011. http://blogs.gartner.com/doug-laney/files/2012/01/ad949-3D-Data-Management-Controlling-Data-Volume-Velocity-and-Variety.pdf .
Lee IK, Wang CC, Lin MC, Kung CT, Lan KC, Lee CT. Effective strategies to prevent coronavirus disease-2019 (COVID-19) outbreak in hospital. J Hosp Infect. 2020;105(1):102.
Lerner I, Veil R, Nguyen DP, Luu VP, Jantzen R. Revolution in health care: how will data science impact doctor-patient relationships? Front Public Health. 2018;6:99.
Lytras MD, Papadopoulou P, editors. Applying big data analytics in bioinformatics and medicine. IGI Global: Hershey; 2017.
Ma K, et al. Big data in multiple sclerosis: development of a web-based longitudinal study viewer in an imaging informatics-based eFolder system for complex data analysis and management. In: Proceedings volume 9418, medical imaging 2015: PACS and imaging informatics: next generation and innovations. 2015. p. 941809. https://doi.org/10.1117/12.2082650 .
Mach-Król M. Analiza i strategia big data w organizacjach. In: Studia i Materiały Polskiego Stowarzyszenia Zarządzania Wiedzą. 2015;74:43–55.
Madsen LB. Data-driven healthcare: how analytics and BI are transforming the industry. Hoboken: Wiley; 2014.
Manyika J, Chui M, Brown B, Bughin J, Dobbs R, Roxburgh C, Hung BA. Big data: the next frontier for innovation, competition, and productivity. Washington: McKinsey Global Institute; 2011.
Marconi K, Dobra M, Thompson C. The use of big data in healthcare. In: Liebowitz J, editor. Big data and business analytics. Boca Raton: CRC Press; 2012. p. 229–48.
Mehta N, Pandit A. Concurrence of big data analytics and healthcare: a systematic review. Int J Med Inform. 2018;114:57–65.
Michel M, Lupton D. Toward a manifesto for the ‘public understanding of big data.’ Public Underst Sci. 2016;25(1):104–16. https://doi.org/10.1177/0963662515609005 .
Mikalef P, Krogstie J. Big data analytics as an enabler of process innovation capabilities: a configurational approach. In: International conference on business process management. Cham: Springer; 2018. p. 426–41.
Mohammadi M, Al-Fuqaha A, Sorour S, Guizani M. Deep learning for IoT big data and streaming analytics: a survey. IEEE Commun Surv Tutor. 2018;20(4):2923–60.
Nambiar R, Bhardwaj R, Sethi A, Vargheese R. A look at challenges and opportunities of big data analytics in healthcare. In: 2013 IEEE international conference on big data; 2013. p. 17–22.
Ohlhorst F. Big data analytics: turning big data into big money, vol. 65. Hoboken: Wiley; 2012.
Olszak C, Mach-Król M. A conceptual framework for assessing an organization’s readiness to adopt big data. Sustainability. 2018;10(10):3734.
Olszak CM. Toward better understanding and use of business intelligence in organizations. Inf Syst Manag. 2016;33(2):105–23.
Palanisamy V, Thirunavukarasu R. Implications of big data analytics in developing healthcare frameworks—a review. J King Saud Univ Comput Inf Sci. 2017;31(4):415–25.
Provost F, Fawcett T. Data science and its relationship to big data and data-driven decisionmaking. Big Data. 2013;1(1):51–9.
Raghupathi W, Raghupathi V. An overview of health analytics. J Health Med Inform. 2013;4:132. https://doi.org/10.4172/2157-7420.1000132 .
Raghupathi W, Raghupathi V. Big data analytics in healthcare: promise and potential. Health Inf Sci Syst. 2014;2(1):3.
Ratia M, Myllärniemi J. Beyond IC 4.0: the future potential of BI-tool utilization in the private healthcare, conference: proceedings IFKAD, 2018 at: Delft, The Netherlands.
Ristevski B, Chen M. Big data analytics in medicine and healthcare. J Integr Bioinform. 2018. https://doi.org/10.1515/jib-2017-0030 .
Rumsfeld JS, Joynt KE, Maddox TM. Big data analytics to improve cardiovascular care: promise and challenges. Nat Rev Cardiol. 2016;13(6):350–9. https://doi.org/10.1038/nrcardio.2016.42 .
Schmarzo B. Big data: understanding how data powers big business. Indianapolis: Wiley; 2013.
Senthilkumar SA, Rai BK, Meshram AA, Gunasekaran A, Chandrakumarmangalam S. Big data in healthcare management: a review of literature. Am J Theor Appl Bus. 2018;4:57–69.
Shubham S, Jain N, Gupta V, et al. Identify glomeruli in human kidney tissue images using a deep learning approach. Soft Comput. 2021. https://doi.org/10.1007/s00500-021-06143-z .
Thuemmler C. The case for health 4.0. In: Thuemmler C, Bai C, editors. Health 4.0: how virtualization and big data are revolutionizing healthcare. New York: Springer; 2017.
Tsai CW, Lai CF, Chao HC, et al. Big data analytics: a survey. J Big Data. 2015;2:21. https://doi.org/10.1186/s40537-015-0030-3 .
Wamba SF, Gunasekaran A, Akter S, Ji-fan RS, Dubey R, Childe SJ. Big data analytics and firm performance: effects of dynamic capabilities. J Bus Res. 2017;70:356–65.
Wang Y, Byrd TA. Business analytics-enabled decision-making effectiveness through knowledge absorptive capacity in health care. J Knowl Manag. 2017;21(3):517–39.
Wang Y, Kung L, Wang W, Yu C, Cegielski CG. An integrated big data analytics-enabled transformation model: application to healthcare. Inf Manag. 2018;55(1):64–79.
Wicks P, et al. Scaling PatientsLikeMe via a “generalized platform” for members with chronic illness: web-based survey study of benefits arising. J Med Internet Res. 2018;20(5):e175.
Willems SM, et al. The potential use of big data in oncology. Oral Oncol. 2019;98:8–12. https://doi.org/10.1016/j.oraloncology.2019.09.003 .
Williams N, Ferdinand NP, Croft R. Project management maturity in the age of big data. Int J Manag Proj Bus. 2014;7(2):311–7.
Winters-Miner LA. Seven ways predictive analytics can improve healthcare. Medical predictive analytics have the potential to revolutionize healthcare around the world. 2014. https://www.elsevier.com/connect/seven-ways-predictive-analytics-can-improve-healthcare (Reading: 15.04.2019).
Wu J, et al. Application of big data technology for COVID-19 prevention and control in China: lessons and recommendations. J Med Internet Res. 2020;22(10): e21980.
Yan L, Peng J, Tan Y. Network dynamics: how can we find patients like us? Inf Syst Res. 2015;26(3):496–512.
Yang JJ, Li J, Mulder J, Wang Y, Chen S, Wu H, Pan H. Emerging information technologies for enhanced healthcare. Comput Ind. 2015;69:3–11.
Zhang Q, Yang LT, Chen Z, Li P. A survey on deep learning for big data. Inf Fusion. 2018;42:146–57.
Download references
Acknowledgements
We would like to thank those who have touched our science paths.
This research was fully funded as statutory activity—subsidy of Ministry of Science and Higher Education granted for Technical University of Czestochowa on maintaining research potential in 2018. Research Number: BS/PB–622/3020/2014/P. Publication fee for the paper was financed by the University of Economics in Katowice.
Author information
Authors and affiliations.
Department of Business Informatics, University of Economics in Katowice, Katowice, Poland
Kornelia Batko
Department of Biomedical Processes and Systems, Institute of Health and Nutrition Sciences, Częstochowa University of Technology, Częstochowa, Poland
Andrzej Ślęzak
You can also search for this author in PubMed Google Scholar
Contributions
KB proposed the concept of research and its design. The manuscript was prepared by KB with the consultation of AŚ. AŚ reviewed the manuscript for getting its fine shape. KB prepared the manuscript in the contexts such as definition of intellectual content, literature search, data acquisition, data analysis, and so on. AŚ obtained research funding. Both authors read and approved the final manuscript.
Corresponding author
Correspondence to Kornelia Batko .
Ethics declarations
Ethics approval and consent to participate.
Not applicable.
Consent for publication
Competing interests.
The author declares no conflict of interest.
Additional information
Publisher's note.
Springer Nature remains neutral with regard to jurisdictional claims in published maps and institutional affiliations.
Rights and permissions
Open Access This article is licensed under a Creative Commons Attribution 4.0 International License, which permits use, sharing, adaptation, distribution and reproduction in any medium or format, as long as you give appropriate credit to the original author(s) and the source, provide a link to the Creative Commons licence, and indicate if changes were made. The images or other third party material in this article are included in the article's Creative Commons licence, unless indicated otherwise in a credit line to the material. If material is not included in the article's Creative Commons licence and your intended use is not permitted by statutory regulation or exceeds the permitted use, you will need to obtain permission directly from the copyright holder. To view a copy of this licence, visit http://creativecommons.org/licenses/by/4.0/ .
Reprints and permissions
About this article
Cite this article.
Batko, K., Ślęzak, A. The use of Big Data Analytics in healthcare. J Big Data 9 , 3 (2022). https://doi.org/10.1186/s40537-021-00553-4
Download citation
Received : 28 August 2021
Accepted : 19 December 2021
Published : 06 January 2022
DOI : https://doi.org/10.1186/s40537-021-00553-4
Share this article
Anyone you share the following link with will be able to read this content:
Sorry, a shareable link is not currently available for this article.
Provided by the Springer Nature SharedIt content-sharing initiative
- Big Data Analytics
- Data-driven healthcare

An official website of the United States government
The .gov means it’s official. Federal government websites often end in .gov or .mil. Before sharing sensitive information, make sure you’re on a federal government site.
The site is secure. The https:// ensures that you are connecting to the official website and that any information you provide is encrypted and transmitted securely.
- Publications
- Account settings
Preview improvements coming to the PMC website in October 2024. Learn More or Try it out now .
- Advanced Search
- Journal List
- Healthcare (Basel)

A Systematic Review on Healthcare Analytics: Application and Theoretical Perspective of Data Mining
Md saiful islam.
1 Mechanical and Industrial Engineering, Northeastern University, Boston, MA 02115, USA; [email protected] (M.S.I.); [email protected] (M.M.H.); [email protected] (X.W.); [email protected] (H.D.G.)
Md Mahmudul Hasan
Xiaoyi wang, hayley d. germack.
2 National Clinician Scholars Program, Yale University School of Medicine, New Haven, CT 06511, USA
3 Bouvé College of Health Sciences, Northeastern University, Boston, MA 02115, USA
Md Noor-E-Alam
Associated data.
The growing healthcare industry is generating a large volume of useful data on patient demographics, treatment plans, payment, and insurance coverage—attracting the attention of clinicians and scientists alike. In recent years, a number of peer-reviewed articles have addressed different dimensions of data mining application in healthcare. However, the lack of a comprehensive and systematic narrative motivated us to construct a literature review on this topic. In this paper, we present a review of the literature on healthcare analytics using data mining and big data. Following Preferred Reporting Items for Systematic Reviews and Meta-Analyses (PRISMA) guidelines, we conducted a database search between 2005 and 2016. Critical elements of the selected studies—healthcare sub-areas, data mining techniques, types of analytics, data, and data sources—were extracted to provide a systematic view of development in this field and possible future directions. We found that the existing literature mostly examines analytics in clinical and administrative decision-making. Use of human-generated data is predominant considering the wide adoption of Electronic Medical Record in clinical care. However, analytics based on website and social media data has been increasing in recent years. Lack of prescriptive analytics in practice and integration of domain expert knowledge in the decision-making process emphasizes the necessity of future research.
1. Introduction
Healthcare is a booming sector of the economy in many countries [ 1 ]. With its growth, come challenges including rising costs, inefficiencies, poor quality, and increasing complexity [ 2 ]. U.S. healthcare expenditures increased by 123% between 2010 and 2015—from $2.6 trillion to $3.2 trillion [ 3 ]. Inefficient—non-value added tasks (e.g., readmissions, inappropriate use of antibiotics, and fraud)—constitutes 21–47% of this enormous expenditure [ 4 ]. Some of these costs were associated with low quality care—researchers found that approximately 251,454 patients in the U.S. die each year due to medical errors [ 5 ]. Better decision-making based on available information could mitigate these challenges and facilitate the transition to a value-based healthcare industry [ 4 ]. Healthcare institutions are adopting information technology in their management system [ 6 ]. A large volume of data is collected through this system on a regular basis. Analytics provides tools and techniques to extract information from this complex and voluminous data [ 2 ] and translate it into information to assist decision-making in healthcare.
Analytics is the way of developing insights through the efficient use of data and application of quantitative and qualitative analysis [ 7 ]. It can generate fact-based decisions for “planning, management, measurement, and learning” purposes [ 2 ]. For instance, the Centers for Medicare and Medicaid Services (CMS) used analytics to reduce hospital readmission rates and avert $115 million in fraudulent payment [ 8 ]. Use of analytics—including data mining, text mining, and big data analytics—is assisting healthcare professionals in disease prediction, diagnosis, and treatment, resulting in an improvement in service quality and reduction in cost [ 9 ]. According to some estimates, application of data mining can save $450 billion each year from the U.S. healthcare system [ 10 ]. In the past ten years, researchers have studied data mining and big data analytics from both applied (e.g., applied to pharmacovigilance or mental health) and theoretical (e.g., reflecting on the methodological or philosophical challenges of data mining) perspectives.
In this review, we systematically organize and summarize the published peer-reviewed literature related to the applied and theoretical perspectives of data mining. We classify the literature by types of analytics (e.g., descriptive, predictive, prescriptive), healthcare application areas (i.e., clinical decision support, mental health), and data mining techniques (i.e., classification, sequential pattern mining); and we report the data source used in each review paper which, to our best knowledge, has never done before.
Motivation and Scope
There is a large body of recently published review/conceptual studies on healthcare and data mining. We outline the characteristics of these studies—e.g., scope/healthcare sub-area, timeframe, and number of papers reviewed—in Table 1 . For example, one study reviewed awareness effect in type 2 diabetes published between 2001 and 2005, identifying 18 papers [ 11 ]. This current review literature is limited—most of the papers listed in Table 1 did not report the timeframe and/or number of papers reviewed (expressed as N/A).
Characteristics of existing review/conceptual studies on the related topics.
Paper | Scope | Timeframe Considered | Number of Papers Reviewed |
---|---|---|---|
[ ] | Awareness effect in type 2 diabetes | 2001–2005 | 18 |
[ ] | Fraud detection | N/A | N/A |
[ ] | Data mining techniques and guidelines for clinical medicine | N/A | N/A |
[ ] | Text mining, Ontologies | N/A | N/A |
[ ] | Challenges and future direction | N/A | N/A |
[ ] | Data mining algorithm, their performance in clinical medicine | 1998–2008 | 84 |
[ ] | Clinical medicine | N/A | N/A |
[ ] | Skin diseases | N/A | N/A |
[ ] | Clinical medicine | N/A | 84 |
[ ] | Algorithms, and guideline | N/A | N/A |
[ ] | Data mining process and algorithms | N/A | N/A |
[ ] | Algorithms for locally frequent disease in healthcare administration, clinical care and research, and training | N/A | N/A |
[ ] | Electronic Medical Record (EMR) and Visual analytics | N/A | N/A |
[ ] | Big data, Level of data usage | N/A | N/A |
[ ] | MapReduce architectural framework based big data analytics | 2007–2014 | 32 |
[ ] | Big data analytics and its opportunities | N/A | N/A |
[ ] | Big data analytics in image processing, signal processing, and genomics | N/A | N/A |
[ ] | Social media data mining to detect Adverse Drug Reaction, Natural language processing techniques (NLP) | 2004–2014 | 39 |
[ ] | Text mining, Adverse Drug Reaction detection | N/A | N/A |
[ ] | Big data analytics in critical care | N/A | N/A |
[ ] | Methodology of big data analytics in healthcare | N/A | N/A |
N/A represents Not Reported.
There is no comprehensive review available which presents the complete picture of data mining application in the healthcare industry. The existing reviews (16 out of 21) are either focused on a specific area of healthcare, such as clinical medicine (three reviews) [ 16 , 17 , 19 ], adverse drug reaction signal detection (two reviews) [ 25 , 26 ], big data analytics (four reviews) [ 8 , 10 , 22 , 24 ], or the application and performance of data mining algorithms (five reviews) [ 9 , 13 , 14 , 20 , 21 ]. Two studies focused on specific diseases (diabetes [ 11 ], skin diseases [ 18 ]). To the best of our knowledge, none of these studies present the universe of research that has been done in this field. These studies are also limited in the rigor of their methodology except for four articles [ 11 , 16 , 22 , 25 ], which provide key insights including the timeframe covered in the study, database search, and literature inclusion or exclusion criteria, but they are limited in their scope of topics covered (see Table 1 ).
Beyond condensing the applied literature, our review also adds to the body of theoretical reviews in the analytics literature. Current theoretical reviews are limited to methodological challenges and techniques to overcome those challenges [ 15 , 16 , 27 ] and application and impact of big data analytics in healthcare [ 23 ]. In summary, the current reviews listed in Table 1 lacks in (1) width of coverage in terms of application areas, (2) breadth of data mining techniques, (3) assessment of literature quality, and (4) systematic selection and analysis of papers. In this review, we aim to fill the above-mentioned gaps. We add to this literature by covering the applied and theoretical perspective of data mining and big data analytics in healthcare with a more comprehensive and systematic approach.
2. Methodology
The methodology of our review followed the checklist proposed by the Preferred Reporting Items for Systematic Reviews and Meta-Analyses (PRISMA) [ 28 ]. We assessed the quality of the selected articles using JBI Critical Appraisal Checklist for analytical cross sectional studies [ 29 ] and Critical Appraisal Skills Programme (CASP) qualitative research checklist [ 30 ].
2.1. Input Literature
Selected literature and their selection process for the review are described in this section. Initially a two phase advance keyword search was conducted on the database Web of Science and one phase (Phase 2) search in PubMed and Google Scholar with time filter 1 January 2005 to 31 December 2016 in “All Fields”. Journal articles written in English was added as additional filters. Keywords listed in Table 2 were used in different phases. The complete search procedure was conducted using the following procedure:

Preferred Reporting Items for Systematic Reviews and Meta-Analyses (PRISMA) flow chart [ 28 ] illustrating the literature search process.
- Exclusion criteria: This included articles reporting on results of: qualitative study, survey, focus group study, feasibility study, monitoring device, team relationship measurement, job satisfaction, work environment, “what-if” analysis, data collection technique, editorials or short report, merely mention data mining, and articles not published in international journals . Duplicates were removed (33 articles). Finally, 117 articles were retained for the review. Figure 1 provides a PRISMA [ 28 ] flow diagram of the review process and Supplementary Information File S1 (Table S1) provides the PRISMA checklist.
Keywords for database search.
) | ) | ||
1 | Healthcare, Health care | Data analysis | |
2 | Healthcare, Health care, Cancer , Disease, Genomics | Data mining, Big data |
1 A logical operator used between the keywords during database search. 2 Cancer was listed independently because other dominant associations have the word “disease” associated with them (i.e., heart disease, skin disease, mental disease etc.).
2.2. Quality Assessment and Processing Steps
The full text of each of the 117 articles was reviewed separately by two researchers to eliminate bias [ 28 ]. To assess the quality of the cross sectional studies, we applied the JBI Critical Appraisal Checklist for Analytical Cross Sectional Studies [ 29 ]. For theoretical papers, we applied the Critical Appraisal Skills Programme (CASP) qualitative research checklist [ 30 ]. We modified the checklist items, as not all items specified in the JBI or CASP checklists were applicable to studies on healthcare analytics ( Supplementary Materials Table S2 ). We evaluated each article’s quality based on inclusion of: (1) clear objective and inclusion criteria; (2) detailed description of sample population and variables; (3) data source (e.g., hospital, database, survey) and format (e.g., structured Electronic Medical Record (EMR), International Classification of Diseases code, unstructured text, survey response); (4) valid and reliable data collection; (5) consideration of ethical issues; (6) detailed discussion of findings and implications; (7) valid and reliable measurement of outcomes; and (8) use of an appropriate data mining tool for cross-sectional studies and (1) clear statement of aims; (2) appropriateness of qualitative methodology; (3) appropriateness of research design; (4) clearly stated findings; and (5) value of research for the theoretical papers. Summary characteristics from any study fulfilling these criteria were included in the final data aggregation ( Supplementary Materials Table S3 ).
To summarize the body of knowledge, we adopted the three-step processing methodology outlined by Levy and Ellis [ 31 ] and Webster and Watson [ 32 ] ( Figure 2 ). During the review process, information was extracted by identifying and defining the problem, understanding the solution process and listing the important findings (“Know the literature”). We summarized and compared each article with the articles associated with the similar problems (“Comprehend the literature”). This simultaneously ensured that any irrelevant information was not considered for the analysis. The summarized information was stored in a spreadsheet in the form of a concept matrix as described by Webster and Watson [ 32 ]. We updated the concept matrix periodically, after completing every 20% of the articles which is approximately 23 articles, to include new findings (“Apply”). Based on the concept matrix, we developed a classification scheme (see Figure 3 ) for further comparison and contrast. We established an operational definition (see Table 3 ) for each class and same class articles were separated from the pool (“Analyze and Synthesis”). We compared classifications between researchers and we resolved disagreements (on six articles) by discussion. The final classification provided distinguished groups of articles with summary, facts, and remarks made by the reviewers (“Evaluate”).

Three stages of effective literature review process, adapted from Levy and Ellis [ 31 ].

Classification scheme of the literature.
Operational definition of the classes.
Class | Operational Definition * |
---|---|
Analytics | Knowledge discovery by analyzing, interpreting, and communicating data |
3A. Types of Analytics | Data Interpretation and Communication method |
Exploration and discovery of information in the dataset [ ] | |
Prediction of upcoming events based on historical data [ ] | |
Utilization of scenarios to provide decision support [ ] | |
3B. Types of Data | Type or nature of data used in the study |
Data extracted from websites, blogs, social media like Facebook, Twitter, LinkedIn [ ] | |
Readings from medical devices and sensors [ ] | |
“Finger prints, genetics, handwriting, retinal scans, X-ray and other medical images, blood pressure, pulse and pulse-oximetry readings, and other similar types of data” [ ] | |
Healthcare bill, insurance claims and transections [ ] | |
Semi-structured and unstructured documents like prescription, Electronic Medical Record (EMR), notes and emails [ ] | |
3C. Data mining techniques | Techniques applied to extract and communicate information from the dataset |
Relationship estimation between variables | |
Finding relation between variables | |
Mapping to predefined class based on shared characteristics | |
Identification of groups and categories in data | |
Detection of out-of-pattern events or incidents | |
A large storage of data to facilitate decision-making | |
Identification of statistically significant patterns in a sequence of data | |
3D. Application Area | Different areas in healthcare where data mining is applied for knowledge discovery and/or decision support |
Analytics applied to analyze, extract and communicate information about diseases, risk for clinical use | |
Application of analytics to improve quality of care, reduce the cost of care and to improve overall system dynamics | |
Privacy: Protection of patient identity in the dataset; Fraud detection: Deceptive and unauthorized activity detection | |
Analytical decision support for psychiatric patients or patient with mental disorder | |
Analysis of problems which affect a mass population, a region, or a country | |
Post market monitoring of Adverse Drug Reaction (ADR) | |
3E. Theoretical study | Discusses impact, challenges, and future of data mining and big data analytics in healthcare |
* Most of the definitions listed in this table are well established in literature and well know. Therefore, we did not use any specific reference. However, for some classes, specifically for types of analytics and data, varying definitions are available in the literature. We cited the sources of those definitions.
2.3. Results
The network diagram of selected articles and the keywords listed by authors in Figure 4 represents the outcome of the methodological review process. We elaborate on the resulting output in the subsequent sections using the structure of the developed classification scheme ( Figure 3 ). We also report the potential future research areas.

Visualization of high-frequency keywords of the reviewed papers. The white circles symbolize the articles and the blue circles represent keywords. The keywords that occurred only once are eliminated as well as the corresponding articles. The size of the blue circles and the texts represent how often that keyword is found. The size of the white circles is proportional to the number of keywords used in that article. The links represents the connections between the keywords and the articles. For example, if a blue circle has three links (e.g., Decision-Making) that means that keyword was used in three articles. The diagram is created with the open source software Gephi [ 34 ].
2.3.1. Methodological Quality of the Studies
Out of 117 papers included in this review, 92 applied analytics and 25 were qualitative/conceptual. The methodological quality of the analytical studies (92 out of 117) were evaluated by a modified version of 8 yes/no questions suggested in JBI Critical Appraisal Checklist for Analytical Cross Sectional Studies [ 29 ]. Each question contains 1 point (1 if the answer is Yes or 0 for No). The score achieved by each paper is provided in the final column of Supplementary Materials Table S3 . On average, each paper applying analytics scored 7.6 out of 8, with a range of 6–8 points. Major drawbacks were the absence of data source and performance measure of data mining algorithms. Out of 92 papers, 23 did not evaluate or mention the performance of the applied algorithms and eight did not mention the source of the data. However, all the papers in healthcare analytics had a clear objective and a detailed discussion of sample population and variables. Data used in each paper was either de-identified/anonymized or approved by institute’s ethical committee to ensure patient confidentiality.
We applied the Critical Appraisal Skills Programme (CASP) qualitative research checklist [ 30 ] to evaluate the quality of the 25 theoretical papers. Five questions (out of ten) in that checklist were not applicable to the theoretical studies. Therefore, we evaluated the papers in this section in a five-point scale (1 if the answer is Yes or 0 for No). Papers included in this review showed high methodological quality as 21 papers (out of 25) scored 5. The last column in the Supplementary Materials Table S3 provides the score achieved by individual papers.
2.3.2. Distribution by Publication Year
The distribution of articles published related to data mining and big data analytics in healthcare across the timeline of the study (2005–2016) is presented in Figure 5 . The distribution shows an upward trend with at least two articles in each year and more than ten articles in the last four years. Additionally, this trend represents the growing interest of government agencies, healthcare practitioners, and academicians in this interdisciplinary field of research. We anticipate that the use of analytics will continue in the coming years to address rising healthcare costs and need of improved quality of care.

Distribution of publication by year (117 articles).
2.3.3. Distribution by Journal
Articles published in 74 different journals were included in this study. Table 4 lists the top ten journals in terms of number of papers published. Expert System with Application was the dominant source of literature on data mining application in healthcare with 7 of the 117 articles. Journals were interdisciplinary in nature and spanned computational journals like IEEE Transection on Information Technology in Biomedicine to policy focused journal like Health Affairs . Articles published in Expert System with Application, Journal of Medical Systems, Journal of the American Medical Informatics Association, Healthcare Informatics Research were mostly related to analytics applied in clinical decision-making and healthcare administration. On the other hand, articles published in Health Affairs were predominantly conceptual in nature addressing policy issues, challenges, and potential of this field.
Top 10 journals on application of data mining in healthcare.
Journal | Number of Articles | |
---|---|---|
Expert Systems with Applications | 7 | |
IEEE Transection on Information Technology in Biomedicine | 6 | |
Journal of Medical Internet Research | 5 | |
Journal of Medical Systems | 4 | |
Journal of the American Medical Informatics Association | 4 | |
Health Affairs | 4 | |
Journal of Biomedical Informatics | 4 | |
Healthcare Informatics Research | 3 | |
Journal of Digital Imaging | 3 | |
PLoS ONE | 3 |
3. Healthcare Analytics
Out of 117 articles, 92 applied analytics for decision-making in healthcare. We discuss the types of analytics, the application area, the data, and the data mining techniques used in these articles and summarize them in Supplementary Materials Table S4 .
3.1. Types of Analytics
We identified three types of analytics in the literature: descriptive (i.e., exploration and discovery of information in the dataset), predictive (i.e., prediction of upcoming events based on historical data) and prescriptive (i.e., utilization of scenarios to provide decision support). Five of the 92 studies employed both descriptive and predictive analytics. In Figure 6 , which displays the percentage of healthcare articles using each analytics type, we show that descriptive analytics is the most commonly used in healthcare (48%). Descriptive analytics was dominant in all the application areas except in clinical decision support. Among the application areas, pharmacovigilance studies only used descriptive analytics as this application area is focused on identifying an association between adverse drug effects with medication. Predictive analytics was used in 43% articles. Among application areas, clinical decision support had the highest application of predictive analytics as many studies in this area are involved in risk and morbidity prediction of chest pain, heart attack, and other diseases. In contrast, use of prescriptive analytics was very uncommon (only 9%) as most of these studies were focused on either a specific population base or a specific disease scenario. However, some evidence of prescriptive analytics was found in public healthcare, administration, and mental health (see Supplementary Materials Table S4 ). These studies create a data repository and/or analytical platform to facilitate decision-making for different scenarios.

Types of analytics used in literature. ( a ) Percentage of analytics type; ( b ) Analytics type by application area.
3.2. Types of Data
To identify types of data, we adopted the classification scheme identified by Raghupathi and Raghupathi [ 23 ] which takes into account the nature (i.e., text, image, number, electronic signal), source, and collection method of data together. Table 3 provides the operational definitions of taxonomy adopted in this paper. Figure 7 a presents the percentage of data type used and Figure 7 b, the number of usage by application area. As expected, human generated (HG) data, including EMR, Electronic Health Record (HER), and Electronic Patient Record (EPR), is the most commonly (77%) used form. Web or Social media (WS) data is the second dominant (11%) type of data, as increasingly more people are using social media now and ongoing digital revolution in the healthcare sector [ 35 ]. In addition, recent development in Natural Language Processing (NLP) techniques is making the use of WS data easier than before [ 36 ]. The other three types of data (SD, BT, and BM) consist of only about 12% of total data usage, but popularity and market growth of wearable personal health tracking devices [ 37 ] may increase the use of SD and BM data.

Percentage of data type used ( a ) and type of data used by application area ( b ).
3.3. Data Mining Techniques
Data mining techniques used in the articles reviewed include classification, clustering, association, anomaly detection, sequential pattern mining, regression, and data warehousing. While elaborate description of each technique and available algorithms is out of scope of this review, we report the frequency of each technique and its sector wise distribution in Figure 8 a,b, respectively. Among the articles included in the review, 57 used classification techniques to analyze data. Association and clustering were used in 21 and 18 articles, respectively. Use of other techniques was less frequent.

Utilization of data mining techniques, ( a ) by percentage and ( b ) by application area.
A high proportion (8 out of 9) of pharmacovigilance papers used association. Use of classification was dominant in every sector except pharmacovigilance ( Figure 8 b). Data warehousing was mostly used in healthcare administration ( Figure 8 b).
We delved deeper into classification as it was utilized in the majority (57 out of 92) of the papers. There are a number of algorithms used for classification, which we present in a word cloud in Figure 9 . Support Vector Machine (SVM), Artificial Neural Network (ANN), Logistic Regression (LR), Decision Tree (DT), and DT based algorithms were the most commonly used. Random Forest (RF), Bayesian Network and Fuzzy-based algorithms were also often used. Some papers (three papers) introduced novel algorithms for specific applications. For example, Yeh et al. [ 38 ] developed discrete particle swarm optimization based classification algorithm to classify breast cancer patients from a pool of general population. Self-organizing maps and K-means were the most commonly used clustering algorithm in healthcare. Performance (e.g., accuracy, sensitivity, specificity, area under the ROC curve, positive predictive value, negative predictive value etc.) of each of these algorithms varied by application and data type. We recommend applying multiple algorithms and choosing the one which achieves the best accuracy.

Word cloud [ 39 ] with classification algorithms.
4. Application of Analytics in Healthcare
Table 3 provides the operational definitions of the six application areas (i.e., clinical decision support, healthcare administration, privacy and fraud detection, mental health, public health, and pharmacovigilance) identified in this review. Figure 10 shows the percentage of articles in each area. Among different classes in healthcare analytics, data mining application is mostly applied in clinical decision support (42%) and administrative purposes (32%). This section discusses the application of data mining in these areas and identifies the main aims of these studies, performance gaps, and key features.

Percentage of papers utilized healthcare analytics by application area (92 articles out of 117).
4.1. Clinical Decision Support
Clinical decision support consists of descriptive and/or predictive analysis mostly related to cardiovascular disease (CVD), cancer, diabetes, and emergency/critical care unit patients. Some studies developed novel data mining algorithms which we review. Table 5 describes the topics investigated and data sources used by papers using clinical decision-making, organized by major diseases category.
Topics and data sources of papers using clinical decision-making, organized by major disease category.
Reference | Major Disease | Topic Investigated | Data Source |
---|---|---|---|
[ ] | Cardiovascular disease (CVD) | Risk factors associated with Coronary heart disease (CHD) | Department of Cardiology, at the Paphos General Hospital in Cyprus |
[ ] | Diagnosis of CHD | Invasive Cardiology Department, University Hospital of Ioannina, Greece | |
[ ] | Classification of uncertain and high dimensional heart disease data | UCI machine learning laboratory repository | |
[ ] | Risk prediction of Cardiovascular adverse event | U.S. Midwestern healthcare system | |
[ ] | Cardiovascular event risk prediction | HMO Research Network Virtual Data Warehouse | |
[ ] | Mobile based cardiovascular abnormality detection | MIT BIH ECG database | |
[ ] | Management of infants with hypoplastic left heart syndrome | The University of Iowa Hospital and Clinics | |
[ ] | Diabetes | Identification of pattern in temporal data of diabetic patients | Synthetic and real world data (not specified) |
[ ] | Exploring the examination history of Diabetic patients | National Health Center of Asti Providence, Italy | |
[ ] | Important factors to identify type 2 diabetes control | The Ulster Hospital, UK | |
[ ] | Comparison of classification accuracy of algorithms for diabetes | Iranian national non-communicable diseases risk factors surveillance | |
[ ] | Type 2 diabetes risk prediction | Independence Blue Cross Insurance Company | |
[ ] | Evaluation of HTCP algorithm in classifying type 2 diabetes patients from non-diabetic patient | Olmsted Medical Center and Mayo Clinic in Rochester, Minnesota, USA | |
[ ] | Predicting and risk diagnosis of patients for being affected with diabetes. | 1991 National Survey of Diabetes data | |
[ ] | Cancer | Survival prediction of prostate cancer patients | The Surveillance, Epidemiology, and End Results (SEER) Program of the National Cancer Institute, USA |
[ ] | Classification of breast cancer patients with novel algorithm | Wisconsin Breast cancer data set, UCI machine learning laboratory repository | |
[ ] | Classification of uncertain and high dimensional breast cancer data | UCI machine learning laboratory repository | |
[ ] | Visualization tool for cancer | Taiwan National Health Insurance Database | |
[ ] | Lung cancer survival prediction with the help of a predictive outcome calculator | SEER Program of the National Cancer Institute, USA | |
[ ] | Emergency Care | Classification of chest pain in emergency department | Hospital (unspecified) emergency department EMR |
[ ] | Grouping of emergency patients based on treatment pattern | Melbourne’s teaching metropolitan hospital | |
[ ] | Intensive care | Mortality rate of ICU patients | University of Kentucky Hospital |
[ ] | Prediction of 30 day mortality of ICU patients | MIMIC-II database | |
[ ] | Other applications | Treatment plan in respiratory infection disease | Various health center throughout Malaysia |
[ ] | Pressure ulcer prediction | Cathy General Hospital (06–07), Taiwan | |
[ ] | Pressure ulcer risk prediction | Military Nursing Outcomes Database (MilNOD), US | |
[ ] | Association of medication, laboratory and problem | Brigham and Women’s Hospital, US | |
[ ] | Chronic disease (asthma) attack prediction | Blue Angel 24 h Monitoring System, Tainan; Environmental Protection Administration Executive, Yuan; Central Weather Bureau Tainan, Taiwan | |
[ ] | Personalized care, predicting future disease | No specified | |
[ ] | Correlation between disease | Sct. Hans Hospital | |
[ ] | Glaucoma prediction using Fundus image | Kasturba Medical college, Manipal, India | |
[ ] | Reducing follow-up delay from image analysis | Department of Veterans Affairs health-care facilities | |
[ ] | Disease risk prediction in imbalanced data | National Inpatient Sample (NIS) data, available at by Healthcare Cost and Utilization Project (HCUP) | |
[ ] | Survivalist prediction of kidney disease patients | University of Iowa Hospital and Clinics | |
[ ] | Comparison surveillance techniques for health care associated infection | University of Alabama at Birmingham Hospital | |
[ ] | Parkinson disease prediction based on big data analytics | Big data archive by Parkinson’s Progression Markers Initiative (PPMI) | |
[ ] | Hospitalization prediction of Hemodialysis patients | Hemodialysis center in Taiwan | |
[ ] | 5 year Morbidity prediction | Northwestern Medical Faculty Foundation (NMFF) | |
[ ] | Algorithm development for real-time disease diagnosis and prognosis | Not specified |
4.1.1. Cardiovascular Disease (CVD)
CVD is one of the most common causes of death globally [ 45 , 77 ]. Its public health relevance is reflected in the literature—it was addressed by seven articles (18% of articles in clinical decision support).
Risk factors related to Coronary Heart Disease (CHD) were distilled into a decision tree based classification system by researchers [ 40 ]. The authors investigated three events: Coronary Artery Bypass Graft Surgery (CABG), Percutaneous Coronary Intervention (PCI), and Myocardial Infarction (MI). They developed three models: CABG vs. non-CABG, PCI vs. non-PCI, and MI VS non-MI. The risk factors for each event were divided into four groups in two stages. The risk factors were separated into before and after the event at the 1st stage and modifiable (e.g., smoking habit or blood pressure) and non-modifiable (e.g., age or sex) at the 2nd stage for each group. After classification, the most important risk factors were identified by extracting the classification rules. The Framingham equation [ 78 ]—which is widely used to calculate global risk for CHD was used to calculate the risk for each event. The most important risk factors identified were age, smoking habit, history of hypertension, family history, and history of diabetes. Other studies on CHD show similar results [ 79 , 80 , 81 ]. This study had implications for healthcare providers and patients by identifying risk factors to specifically target, identify and in the case of modifiable factors, reduce CHD risk [ 40 ].
Data mining has also been applied to diagnose Coronary Artery Disease (CAD) [ 41 ]. Researchers showed that in lieu of existing diagnostic methods (i.e., Coronary Angiography (CA))—which are costly and require high technical skill—data mining using existing data like demographics, medical history, simple physical examination, blood tests, and noninvasive simple investigations (e.g., heart rate, glucose level, body mass index, creatinine level, cholesterol level, arterial stiffness) is simple, less costly, and can be used to achieve a similar level of accuracy. Researchers used a four-step classification process: (1) Decision tree was used to classify the data; (2) Crisp classification rules were generated; (3) A fuzzy model was created by fuzzifying the crisp classifier rules; and (4) Fuzzy model parameters were optimized and the final classification was made. The proposed optimized fuzzy model achieved 73% of prediction accuracy and improved upon an existing Artificial Neural Network (ANN) by providing better interpretability.
Traditional data mining and machine learning algorithms (e.g., probabilistic neural networks and SVM) may not be advanced enough to handle the data used for CVD diagnosis, which is often uncertain and highly dimensional in nature. To tackle this issue, researchers [ 42 ] proposed a Fuzzy standard additive model (SAM) for classification. They used adaptive vector quantization clustering to generate unsupervised fuzzy rules which were later optimized (minimized the number of rules) by Genetic Algorithm (GA). They then used the incremental form of a supervised technique, Gradient Descent, to fine tune the rules. Considering the highly time consuming process of the fuzzy system given large number of features in the data, the number of features was reduced with wavelet transformation. The proposed algorithm achieved better accuracy (78.78%) than the probabilistic neural network (73.80%), SVM (74.27%), fuzzy ARTMAP (63.46%), and adaptive neuro-fuzzy inference system (74.90%). Another common issue in cardiovascular event risk prediction is the censorship of data (i.e., the patient’s condition is not followed up after they leave hospital and until a new event occurs; the available data becomes right-censored). Elimination and exclusion of the censored data create bias in prediction results. To address the censorship of the data in their study on CVD event risk prediction after time, two studies [ 43 , 44 ] used Inverse Probability Censoring Weighting (IPCW). IPCW is a pre-processing step used to calculate the weights on data which are later classified using Bayesian Network. One of these studies [ 43 ] provided an IPCW based system which is compatible with any machine learning algorithm.
Electrocardiography (ECG)—non-invasive measurement of the electrical activity of the heartbeat—is the most commonly used medical studies in the assessment of CVD. Machine learning offers potential optimization of traditional ECG assessment which requires decompressing before making any diagnosis. This process takes time and large space in computers. In one study, researchers [ 45 ] developed a framework for real-time diagnosis of cardiovascular abnormalities based on compressed ECG. To reduce diagnosis time—which is critical for clinical decision-making regarding appropriate and timely treatment—they proposed and tested a mobile based framework and applied it to wireless monitoring of the patient. The ECG was sent to the hospital server where the ECG signals were divided into normal and abnormal clusters. The system detected cardiac abnormality with 97% accuracy. The cluster information was sent to patient’s mobile phone; and if any life-threatening abnormality was detected, the mobile phone alerted the hospital or the emergency personnel.
Data analytics have also been applied to more rare CVDs. One study [ 46 ] developed an intervention prediction model for Hypoplastic Left Heart Syndrome (HLHS). HLHS is a rare form of fatal heart disease in infants, which requires surgery. Post-surgical evaluation is critical as patient condition can shift very quickly. Indicators of wellness of the patients are not easily or directly measurable, but inferences can be made based on measurable physiological parameters including pulse, heart rhythm, systemic blood pressure, common atrial filling pressure, urine output, physical exam, and systemic and mixed venous oxygen saturations. A subtle physiological shift can cause death if not noticed and intervened upon. To help healthcare providers in decision-making, the researchers developed a prediction model by identifying the correlation between physiological parameters and interventions. They collected 19,134 records of 17 patients in Pediatric Intensive Care Units (PICU). Each record contained different physiological parameters measured by devices and noted by nurses. For each record, a wellness score was calculated by the domain experts. After classifying the data using a rough set algorithm, decision rules were extracted for each wellness score to aid in making intervention plans. A new measure for feature selection—Combined Classification Quality (CCQ)—was developed by considering the effect of variations in a feature values and distinct outcome each feature value leads to. Authors showed that higher value of CCQ leads to higher classification accuracy which is not always true for commonly used measure classification quality (CQ). For example, two features with CQ value of 1 leads to very different classification accuracy—35.5% and 75%. Same two features had CCQ value 0.25 and 0.40, features with 0.40 CCQ produced 75% classification accuracy. By using CCQ instead of CQ, researchers can avoid such inconsistency.
4.1.2. Diabetes
The disease burden related to diabetes is high and rising in every country. According to the World Health Organization’s (WHO) prediction, it will become the seventh leading cause of death by 2030 [ 82 ]. Data mining has been applied to identify rare forms of diabetes, identify the important factors to control diabetes, and explore patient history to extract knowledge. We reviewed 7 studies that applied healthcare analytics to diabetes.
Researchers extracted knowledge about diabetes treatment pathways and identified rare forms and complications of diabetes using a three level clustering framework from examination history of diabetic patients [ 48 ]. In this three-level clustering framework, the first level clustered patients who went through regular tests for monitoring purposes (e.g., checkup visit, glucose level, urine test) or to diagnose diabetes-related complications (e.g., eye tests for diabetic retinopathy). The second level explored patients who went through diagnosis for specific or different diabetic complications only (e.g., cardiovascular, eye, liver, and kidney related complications). These two level produced 2939 outliers out of 6380 patients. At the third level, authors clustered these outlier patients to gain insight about rare form of diabetes or rare complications. A density based clustering algorithm, DBSCAN, was used for clustering as it doesn’t require to specify the number of clusters apriori and is less sensitive to noise and outliers. This framework for grouping patients by treatment pathway can be utilized to evaluate treatment plans and costs. Another group of researchers [ 49 ] investigated the important factors related to type 2 diabetes control. They used feature selection via supervised model construction (FSSMC) to select the important factors with rank/order. They applied naïve bayes, IB1 and C4.5 algorithm with FSSMC technique to classify patients having poor or good diabetes control and evaluate the classification efficiency for different subsets of features. Experiments performed with physiological and laboratory information collected from 3857 patients showed that the classifier algorithms performed best (1–3% increase in accuracy) with the features selected by FSSMC. Age, diagnosis duration, and Insulin treatment were the top three important factors.
Data analytics have also been applied to identify patients with type 2 diabetes. In one study [ 52 ], using fragmented data from two different healthcare centers, researchers evaluated the effect of data fragmentation on a high throughput clinical phenotyping (HTCP) algorithm to identify patients at risk of developing type 2 diabetes. When a patient visits multiple healthcare centers during a study period, his/her data is stored in different EMRs and is called fragmented. In such cases, using HTPC algorithm can lead to improper classification. An experiment performed in a rural setting showed that using data from two healthcare centers instead of one decreased the false negative rate from 32.9% to 0%. In another study, researchers [ 51 ] utilized sparse logistic regression to predict type 2 diabetes risk from insurance claims data. They developed a model that outperformed the traditional risk prediction methods for large data sets and data sets with missing value cases by increasing the AUC value from 0.75 to 0.80. The dataset contained more than 500 features including demography, specific medical conditions, and comorbidity. And in another study, researchers [ 53 ] developed prediction and risk diagnosis model using a hybrid system with SVM. Using features like blood pressure, fasting blood sugar, two-hour post-glucose tolerance, cholesterol level along with other demographic and anthropometric features, the SVM algorithm was able to predict diabetes risk with 97% accuracy. One reason for achieving high accuracy compared to the study using insurance claims data [ 51 ] is the structured nature of the data which came from a cross-sectional survey on diabetes.
Different statistical and machine learning algorithms are available for classification purposes. Researchers [ 50 ] compared the performance of two statistical method (LR and Fisher linear discriminant analysis) and four machine learning algorithms (SVM (using radial basis function kernel), ANN, Random Forest, and Fuzzy C-mean) for predicting diabetes diagnosis. Ten features (age, gender, BMI, waist circumference, smoking, job, hypertension, residential region (rural/urban), physical activity, and family history of diabetes) were used to test the classification performance (diabetes or no diabetes). Parameters for ANN and SVM were optimized through Greedy search. SVM showed best performance in all performance measures. SVM was at least 5% more accurate than other classification techniques. Statistical methods performed similar to the other machine learning algorithms. This study was limited by a low prevalence of diabetes in the dataset, however, which can cause poor classification performance. Researchers [ 47 ] also proposed a novel pattern recognition algorithm by using convolutional nonnegative matrix factorization. They considered a patient as an entity and each of patients’ visit to the doctor, prescriptions, test result, and diagnosis are considered as an event over time. Finding such patterns can be helpful to group similar patients, identify their treatment pathway as well as patient management. Though they did not compare the pattern recognition accuracy with existing methods like single value decomposition (SVD), the matrix-like representation makes it intuitive.
4.1.3. Cancer
Cancer is another major threat to public health [ 83 ]. Machine learning has been applied to cancer patients to predict survival, and diagnosis. We reviewed five studies that applied healthcare analytics to cancer.
Despite many advances in treatment, accurate prediction of survival in patients with cancer remains challenging considering the heterogeneity of cancer complexity, treatment options, and patient population. Survival of prostate cancer patients has been predicted using a classification model [ 54 ]. The model used a public database-SEER (Surveillance, Epidemiology, and End Result) and applied a stratified ten-fold sampling approach. Survival prediction among prostate cancer patients was made using DT, ANN and SVM algorithm. SVM outperformed other algorithms with 92.85% classification accuracy wherein DT and ANN achieved 90% and 91.07% accuracy respectively. This same database has been used to predict survival of lung cancer patients [ 56 ]. After preprocessing the 11 features available in the data set, authors identified two features (1. removed and examined regional lymph node count and 2. malignant/in-situ tumor count) which had the strongest predictive power. They used several supervised classification methods on the preprocessed data; ensemble voting of five decision tree based classifiers and meta-classifiers (J48 DT, RF, LogitBoost, Random Subspace, and Alternating DT) provided the best performance—74% for 6 months, 75% for 9 months, 77% for 1 year, 86% for 2 years, and 92% for 5 years survival. Using this technique, they developed an online lung cancer outcome calculator to estimate the risk of mortality after 6 months, 9 months, 1 year, 2 years and 5 years of diagnosis.
In addition to predicting survival, machine learning techniques have also been used to identify patients with cancer. Among patients with breast cancer, researchers [ 38 ] have proposed a new hybrid algorithm to classify breast cancer patient from patients who do not have breast cancer. They used correlation and regression to select the significant features at the first stage. Then, at the second stage, they used discrete Particle Swarm Optimization (PSO) to classify the data. This hybrid algorithm was applied to Wisconsin Breast Cancer Data set available at UCI machine learning repository. It achieved better accuracy (98.71%) compared to a genetic algorithm (GA) (96.14%) [ 84 ] and another PSO-based algorithm (93.4%) [ 85 ].
Machine learning has also been used to identify the nature of cancer (benign or malignant) and to understand demographics related to cancer. Among patients with breast cancer, researchers [ 42 ] applied the Fuzzy standard additive model (SAM) with GA (discussed earlier in relation to CVD)-predicting the nature of breast cancer (benign or malignant). They used a UCI machine learning repository which was capable of classifying uncertain and high dimensional data with greater accuracy (by 1–2%). Researchers have also used big data [ 55 ] to create a visualization tool to provide a dynamic view of cancer statistics (e.g., trend, association with other diseases), and how they are associated with different demographic variables (e.g., age, sex) and other diseases (e.g., diabetes, kidney infection). Use of data mining provided a better understanding of cancer patients both at demographic and outcome level which in terms provides an opportunity of early identification and intervention.
4.1.4. Emergency Care
The Emergency department (ED) is the primary route to hospital admission [ 58 ]. In 2011, 20% of US population had at least one or more visits to the ED [ 86 ]. EDs are experiencing significant financial pressure to increase efficiency and throughput of patients. Discrete event simulation (i.e., modeling system operations with sequence of isolated events) is a useful tool to understand and improve ED operations by simulating the behavior and performance of EDs. Certain features of the ED (e.g., different types of patients, treatments, urgency, and uncertainty) can complicate simulation. One way to handle the complexity is to group the patients according to required treatment. Previously, the “casemix” principle, which was developed by expert clinicians to groups of similar patients in case-specific settings (e.g., telemetry or nephrology units), was used, but it has limitations in the ED setting [ 58 ]. Researchers applied [ 58 ] data mining (clustering) to the ED setting to group the patients based on treatment pattern (e.g., full ward test, head injury observation, ECG, blood glucose, CT scan, X-ray). The clustering model was verified and validated by ED clinicians. These grouping data were then used in discrete event simulation to understand and improve ED operations (mainly length of stay) and process flows for each group.
Chest pain admissions to the ED have also been examined using decision-making framework. Researchers [ 57 ] proposed a three stage decision-making framework for classifying severity of chest pain as: AMI, angina pectoris, or other. At the first stage, lab tests and diagnoses were collected and the association between them were extracted. In the second stage, experts developed association rules between lab tests diagnosis to help physicians make quick diagnostic decisions based diagnostic tests and avoid further unnecessary lab tests. In the third stage, authors developed a classification tree to classify the chest pain diagnosis based on selected lab test, diagnosis and medical record. This hybrid model was applied to the emergency department at one hospital. They developed the classification system using 327 association rules to selected lab tests using C5.0, Neural Network (NN) and SVM. C5.0 algorithm achieved 94.18% accuracy whereas NN and SVM achieved 88.89% and 85.19% accuracy respectively.
4.1.5. Intensive Care
Intensive care units cater to patients with severe and life-threatening illness and injury which require constant, close monitoring and support to ensure normal bodily function. Death is a much more common event in an ICU compared to a general medical unit—one study showed that 22.4% of total death in hospitals occurred in the ICU [ 87 ]. Survival predictions and identification of important factors related to mortality can help healthcare providers plan care. We identified two papers [ 59 , 60 ] that developed prediction models for ICU mortality rate prediction. Using a large amount of ICU patient data (specifically from the first 24 h of the stay) collected from University of Kentucky Hospital from 1998 to 2007 (38,474 admissions), one group of researchers identified 15 out of 40 significant features using Pearson’s Chi-square test (for categorical variables) and Student-t test (for continuous variable) [ 59 ]. The mortality rate was predicted by DT, ANN, SVM and APACHE III, a logistic regression based approach. Compared to the other methods applied, DT’s AUC value was higher by 0.02. The study was limited, however, by only considering the first 24 h of admission to the ICU, which may not be enough to make prediction on mortality rate. Another team of researchers [ 60 ] applied a similarity metric to predict 30-day mortality prediction in 17,152 ICU admissions data extracted from MIMIC-II database [ 88 ]. Their analysis concluded that a large group of similar patient data (e.g., vital sign, laboratory test result) instead of all patient data would lead to slightly better prediction accuracy. The logistic regression model for mortality prediction achieved 0.83 AUC value when 5000 similar patients were used for training but, its performance declined to 0.81 AUC when all the available patient data were used.
4.1.6. Other Applications
In addition to CVD, diabetes, cancer, emergency care, and ICU care, data mining has been applied to various clinical decision-making problems like pressure ulcer risk prediction, general problem lists, and personalized medical care. To predict pressure ulcer formation (localized skin and tissue damage because of shear, friction, pressure or any combination of these factors), researchers [ 62 ] developed two classification-based predictive models. One included all 14 features (including age, sex, course, Anesthesia, body position during operation, and skin status) and another, reduced model, including significant features only (5 in DT model, 7 in SVM, LR and Mahalanobis Taguchi System model). Mahalanobis Taguchi System (MTS), SVM, DT, and LR were used for both classification and feature selection (in the second model only) purposes. LR and SVM performed slightly better when all the features were included, but MTS achieved better sensitivity and specificity in the reduced model (+10% to +15%). These machine learning techniques can provide better assistance in pressure ulcer risk prediction than the traditional Norton and Braden medical scale [ 62 ]. Though the study provides the advantages of using data mining algorithms, the data set used here was imbalanced as it only had 8 cases of pressure ulcer in 168 patients. Also among patients with pressure ulcers, another team of researchers [ 63 ] recommended a data mining based alternative to the Braden scale for prediction. They applied data mining algorithms to four years of longitudinal patient data to identify the most important factors related to pressure ulcer prediction (i.e., days of stay in the hospital, serum albumin, and age). In terms of C-statistics, RF (0.83) provided highest predictive accuracy over DT (0.63), LR (0.82), and multivariate adaptive regression splines (0.78).
For data mining algorithms, which often show poor performance with imbalanced (i.e., low occurrence of one class compared to other classes) data, researchers [ 70 ] developed a sub-sampling technique. They designed two experiments, one considered sub-sampling technique and another one did not. For a highly imbalanced data set, Random Forest (RF), SVM, and Bagging and Boosting achieved better classification accuracy with this sub-sampling technique in classifying eight diseases (male genital disease, testis cancer, encephalitis, aneurysm, breast cancer, peripheral atherosclerosis, and diabetes mellitus) that had less than 5% occurrences in the National Inpatient Sample (NIS) data of Healthcare Cost and Utilization Project (HCUP). Surprisingly, possibly due to balancing the dataset through sub-sampling, RF slightly outperformed (+0.01 AUC) the other two methods.
The patient problem list is a vital component of clinical medicine. It enables decision support and quality measurement. But, it is often incomplete. Researchers have [ 64 ] suggested that a complete list of problems leads to better quality treatment in terms of final outcome [ 64 ]. Complete problem lists enable clinicians to get a better understanding of the issue and influence diagnostic reasoning. One group of researchers proposed a data mining model to find an association between patient problems and prescribed medications and laboratory tests which can act as a support to clinical decision-making [ 64 ]. Currently, domain experts spend a large amount of time for this purpose but, association rule mining can save both time and other resources. Additionally, consideration of unstructured data like doctor’s and/or nurse’s written comments and notes can provide additional information. These association rules can aid clinicians in preventing errors in diagnosis and reduce treatment complexity. For example, a set of problems and medications can co-occur frequently. If a clinician has knowledge about this relation, he/she can prescribe similar medications when faced with a similar set of problems. One group of researchers [ 61 ] developed an approach which achieved 90% accuracy in finding association between medications and problems, and 55% accuracy between laboratory tests and problems. Among outpatients diagnosed with respiratory infection, 92.79% were treated with drugs. Physicians could choose any of the 100,013 drugs available in the inventory. Moreover, in an attempt to examine the treatment plan patterns, they identified the 78 most commonly used drugs which could be prescribed, regardless of patient’s complaints and demography. The classification model used to identify the most common drugs achieved 74.73% accuracy and most importantly found variables like age, race, gender, and complaints of patients were insignificant.
Personalized medicine—tailored treatment based on a patient’s predicted response or risk of disease—is another venue for data mining algorithms. One group of researchers [ 66 ] used a big data framework to create personalized care system. One patient’s medical history is compared with other available patient data. Based on that comparison, possibility of a disease of an individual was calculated. All the possible diseases were ranked from high risk to low risk diseases. This approach is very similar to how online giants Netflix and Amazon suggest movies and books to the customer [ 66 ]. Another group of researchers [ 67 ] used the Electronic Patient Records (EPR), which contains structured data (e.g., disease code) and unstructured data (e.g., notes and comments made by doctors and nurses at different stages of treatment) to develop personalized care. From the unstructured text data, the researchers extracted clinical terms and mapped them to an ontology. Using this mapped codes and existing structured data (disease code), they created a phenotypic profile for each patient. The patients were divided into different clusters (with 87.78% precision) based on the similarity of their phenotypic profile. Correlation of diseases were captured by counting the occurrences of two or more diseases in patient phenotype. Then, the protein/gene structure associated with the diseases was identified and a protein network was created. From the sharing of specific protein structure by the diseases, correlation was identified.
Among patients with asthma, researchers [ 65 ] used environmental and patient physiological data to develop a prediction model for asthma attack to give doctors and patients a chance for prevention. They used data from a home-care institute where patients input their physical condition online; and environmental data (air pollutant and weather data). Their data mining model involved feature selection through sequential pattern mining and risk prediction using DT and association rule mining. This model can make asthma attack risk prediction with 86.89% accuracy. Real implementation showed that patients found risk prediction helpful to avoid severe asthma attacks.
Among patients with Parkinson’s disease, researchers [ 73 ] introduced a comprehensive end-to-end protocol for complex and heterogeneous data characterization, manipulation, processing, cleaning, analysis and validation. Specifically, the researchers used a Synthetic Minority Over-sampling Technique (SMOTE) to rebalance the data set. Rebalancing the dataset using SMOTE improved SVM’s classification accuracy from 76% to 96% and AdaBoost’s classification accuracy from 96% to 99%. Moreover, the study found that traditional statistical classification approaches (e.g., generalized linear model) failed to generate reliable predictions but machine learning-based classification methods performed very well in terms of predictive precision and reliability.
Among patients with kidney disease, researchers [ 71 ] developed a prediction model to forecast survival. Data collected from four facilities of University of Iowa Hospital and Clinics contains 188 patients with over 707 visits and features like blood pressure measures, demographic variables, and dialysis solution contents. Data was transformed using functional relation (i.e., the similarity between two or more features when two features have same values for a set of patients, they are combined to form a single feature) between the features. The data set was randomly divided into eight sub-sets. Sixteen classification rules were generated for the eight sub-sets using two classification algorithms—Rough Set (RS) and DT. Classes represented survival beyond three years, less than three years and undetermined. To make predictions, each classification rule (out of 16) had one vote and the majority vote decided the final predictive class. Transformed data increased predictive accuracy by 11% than raw data and DT (67% accuracy) performed better than RS (56% accuracy). The researchers suggested that this type of predictive analysis can be helpful in personalized treatment selection, resource allocation for patients, and designing clinical study. Among patients on kidney dialysis, another group of researchers [ 74 ] applied temporal pattern mining to predict hospitalization using biochemical data. Their result showed that amount of albumin—a type of protein float in blood—is the most important predictor of hospitalization due to kidney disease.
Among patients over 50 years of age, researchers [ 75 ] developed a data mining model to predict five years mortality using the EHR of 7463 patients. They used Ensemble Rotating Forest algorithm with alternating decision tree to classify the patients into two classes of life expectancy: (1) less than five years and (2) equal or greater than five years. Age, comorbidity count, previous record of hospitalization record, and blood urea nitrogen were a few of the significant features selected by correlation feature selection along with greedy stepwise search method. Accuracy achieved by this approach (AUC 0.86) was greater than the standard modified Charlson Index (AUC 0.81) and modified Walter Index (AUC 0.78). Their study showed that age, hospitalization prior the visit, and highest blood urea nitrogen were the most important factors for predicting five years morbidity. This five-year morbidity prediction model can be very helpful to optimally use resources like cancer screening for those patients who are more likely to be benefit from the resources.
Another group of researchers [ 76 ] addressed the limitations of existing software technology for disease diagnosis and prognosis, such as inability to handle data stream (DT), impractical for complex and large systems (Bayesian Network), exhaustive training process (NN). To overcome these restriction, authors proposed a decision tree based algorithm called “Very Fast Decision Tree (VFDT)”. Comparison with a similar system developed by IBM showed that VFDT utilizes lesser amount of system resources and it can perform real time classification.
Researchers have also used data mining to optimize the glaucoma diagnosis process [ 68 ]. Traditional approaches including Optical Coherence Tomography, Scanning Laser Polarimetry (SLP), and Heidelberg Retina Tomography (HRT) scanning methods are costly. This group used Fundus image data which is less costly and classified patient as either normal or glaucoma patient using SVM classifier. Before classification, authors selected significant features by using Higher Order Spectra (HOS) and Discrete Wavelet Transform (DWT) method combined and separately. Several kernel functions for SVM—all delivering similar levels of accuracy—were applied. Their approach produced 95% accuracy in glaucoma prediction. For diagnostic evaluation of chest imaging for suspicion for malignancy, researchers [ 69 ] designed trigger criteria to identify potential follow-up delays. The developed trigger predicted the patients who didn’t require follow-up evaluation. The analysis of the experiment result indicated that the algorithm to identify patients’ delays in follow-up of abnormal imaging is effective with 99% sensitivity and 38% specificity.
Data mining has also been applied to [ 72 ] compare three metrics to identify health care associated infections—Catheter Associated Bloodstream Infections, Catheter Associated Urinary Tract Infections and Ventilator Associated Pneumonia. Researchers compared traditional surveillance using National Healthcare Safety Network methodology to data mining using MedMined Data Mining Surveillance (CareFusion Corporation, San Diego, CA, USA), and administrative coding using ICD-9-CM. Traditional surveillance proved to be superior than data mining in terms of sensitivity, positive predictive value and rate estimation.
Data mining has been used in 38 studies of clinical decision-making CVD (7 articles), diabetes (seven articles), cancer (five articles), emergency care (two articles), intensive care (two articles), and other applications (16 articles). Most of the studies developed predictive models to facilitate decision-making and some developed decision support system or tools. Authors often tested their models with multiple algorithms; SVM was at the top of that list and often outperformed other algorithms. However, 15 [ 38 , 40 , 42 , 45 , 47 , 51 , 54 , 56 , 58 , 60 , 61 , 66 , 73 , 74 , 76 ] of the studies did not incorporate expert opinion from doctors, clinician, or appropriate healthcare personals in building models and interpreting results (see the study characteristics in Supplementary Materials Table S3 ). We also noted that there is an absence of follow-up studies on the predictive models, and specifically, how the models performed in dynamic decision-making situations, if doctors and healthcare professionals comfortable in using these predictive models, and what are the challenges in implementing the models if any exist? Existing literature does not focus on these salient issues.
4.2. Healthcare Administration
Data mining was applied to administrative purposes in healthcare in 32% (29 articles) of the articles reviewed. Researchers have applied data mining to: data warehousing and cloud computing; quality improvement; cost reduction; resource utilization; patient management; and other areas. Table 6 provides a list of these articles with major focus areas, problems analyzed and the data source.
Problem analyzed and data sources in healthcare administration.
Reference | Focusing Area | Problem Analyzed | Data Source |
---|---|---|---|
[ ] | Data warehousing and cloud computing | Developing a platform to analyze the causes of readmission | Emory Hospital, US |
[ ] | Development of a clinical data warehouse and analytical tools for traditional Chinese medicine | Traditional Chinese Medicine hospitals/wards | |
[ ] | Cloud and big data analytics based cyber-physical system for patient-centric healthcare applications and services | Not specified | |
[ ] | Repository of radiology reports | Not specified | |
[ ] | Creation of large data repository and knowledge discovery with unsupervised learning | University of Virginia University Health System | |
[ ] | Development of a mobile application to gather, store and provide data for rural healthcare | Not specified | |
[ ] | Healthcare cost, quality and resource utilization | Treatment error prevention to improve quality and reduce cost | National Taiwan University Hospital |
[ ] | Healthcare cost prediction | US health insurance company | |
[ ] | Healthcare resource utilization by lung cancer patients | Medicare beneficiaries for 1999, US | |
[ ] | Length of stay prediction of Coronary Artery Disease (CAD) | Rajaei Cardiovascular Medical and Research Center, Tehran, Iran | |
[ ] | Methodology for structured development of monitoring systems and a primary HC network resource allocation monitoring model | National Institute of Public Health; Health Care Institute, Celje; Slovenian Social Security Database, and Slovenian Medical Chamber | |
[ ] | Assess the ability of regression tree boosting to risk-adjust health care cost predictions | Thomson Medstat’s Commercial Claims and Encounters database. | |
[ ] | Evidence based recommendation in prescribing drugs | Dalhousie University Medical Faculty | |
[ ] | Efficient pathology ordering system | Pathology company in Australia | |
[ ] | Identifying people with or without insurance based on demographic and socio-economic factors | Behavioral Risk Factor Surveillance System 2004 Survey Data | |
[ ] | Predicting care quality from patient experience | English National Health Service website | |
[ ] | Patient management | Scheduling of patients | A south-east rural U.S. clinic |
[ ] | Care plan recommendation system | A community hospital in the Mid-West U.S. | |
[ ] | Examination of risk factors to predict persistent healthcare frequent attendance | Tampere Health Centre, Finland | |
[ ] | Forecasting number of patient visit for administrative task | Health care center in Jaen, Spain | |
[ ] | Critical factors related to fall | 1000 bed hospital in Taiwan | |
[ ] | Verification of structured data, and codes in EMR of fall related injuries from unstructured data | Veterans Health Administration database, US | |
[ ] | Other applications | Relation between medical school training and practice | Center for Medicare and Medicaid Service (CMS) |
[ ] | Analysis of physician reviews from online platform | Good Doctor Online health community | |
[ ] | Evaluation of Key Performance Indicator (KPIs) of hospital | Greek National Health Systems for the year of 2013 | |
[ ] | Post market performance evaluation of medical devices | HCUPNet data (2002–2011) | |
[ ] | Feasibility of measuring drug safety alert response from HC professional’s information seeking behavior | UpToDate, an online medical resource | |
[ ] | Influencing factors of home healthcare service outcome | U.S. home and hospice care survey (2000) | |
[ ] | Compilation of various data types for tracing, and analyzing temporal events and facilitating the use of NoSQL and cloud computing techniques | Taiwan’s National Health Insurance Research Database (NHIRD) |
4.2.1. Data Warehousing and Cloud Computing
Data warehousing [ 90 ] and cloud computing are used to securely and cost-effectively store the growing volume of electronic patient data [ 1 ] and to improve hospital outcomes including readmissions. To identify cause of readmission, researchers [ 89 ] developed an open source software—Analytic Information Warehouse (AIW). Users can design a virtual data model (VDM) using this software. Required data to test the model can be extracted in terms of a temporal ontology from the data warehouse and analysis can be performed using any standard analyzing tool. Another group of researchers took a similar approach to develop a Clinical Data Warehouse (CDW) for traditional Chinese medicine (TCM). The warehouse contains clinical information (e.g., symptoms, disease, and treatment) for 20,000 inpatients and 20,000 outpatients. Data was collected in a structured way using pre-specified ontology in electronic form. CDW provides an interface for online data mining, online analytical processing (OLAP) and network analysis to discover knowledge and provide clinical decision support. Using these tools, classification, association and network analysis between symptoms, diseases and medications (i.e., herbs) can be performed.
Apart from clinical purposes, data warehouses can be used for research, training, education, and quality control purposes. Such a data repository was created using the basic idea of Google search engine [ 92 ]. Users can pull the radiology report files by searching keywords like a simple google search following the predefined patient privacy protocol. Another data repository was created as a part of collaborative study between IBM and University of Virginia and its partner, Virginia Commonwealth University Health System was created [ 93 ]. The repository contains 667,000 patient record with 208 attributes. HealthMiner—a data mining package for healthcare created by IBM—was used to perform unsupervised analysis like finding associations, pattern and knowledge discovery. This study also showed the research benefits of this type of large data repository. Researchers [ 91 ] proposed a framework based on cloud computing and big data to unify data collected from different sources like public databases and personal health devices. The architecture was divided into 3 layers. The first layer unified heterogeneous data from different sources, the second layer provided storage support and facilitated data processing and analytics access, and the third layer provided result of analysis and platform for professionals to develop analytical tools. Some researchers [ 94 ] used mobile devices to collect personal health data. Users took part in a survey on their mobile devices and got a diagnosis report based on their health parameters input in the survey. Each survey data were saved in a cloud-based interface for effective storage and management. From user input stored in cloud, interactive geo-spatial maps were developed to provide effective data visualization facility.
4.2.2. Healthcare Cost, Quality and Resource Utilization
Ten articles applied data mining to cost reduction, quality improvement and resource utilization issues. One group of researchers predicted healthcare costs using an algorithmic approach [ 96 ]. They used medical claim data of 800,000 people collected by an insurance company over the period of 2004–2007. The data included diagnoses, procedures, and drugs. They used classification and clustering algorithms and found that these data mining algorithms improve the absolute prediction error more than 16%. Two prediction models were developed, one using both cost and medical information and the other used only cost information. Both models had similar accuracy on predicting healthcare costs but performed better than traditional regression methods. The study also showed that including medical information does not improve cost prediction accuracy. Risk-adjusted health care cost predictions, with diagnostic groups and demographic variables as inputs, have also been assessed using regression tree boosting [ 100 ]. Boosted regression tree and main effects linear models were used and fitted to predict current (2001) and prospective (2002) total health care costs per patient. The authors concluded that the combination of regression tree boosting and a diagnostic grouping scheme are a competitive alternative to commonly used risk-adjustment systems.
A sizable amount ($37.6 billion) of healthcare costs is attributable to medical errors, 45% of which stems from preventable errors [ 95 ]. To aid in physician decision-making and reduce medical errors, researchers [ 95 ] proposed a data mining-based framework-Sequential Clustering Algorithm. They identified patterns of treatment plans, tests, medication types and dosages prescribed for specific diseases, and other services provided to treat a patient throughout his/her stay in the hospital. The proposed framework was based on cloud computing so that the knowledge extracted from the data could be shared among hospitals without sharing the actual record. They proposed to share models using Virtual Machine (VM) images to facilitate collaboration among international institutions and prevent the threat of data leakage. This model was implemented in two hospitals, one in Taiwan and another in Mongolia. To identify best practices for specific diseases and prevent medical errors, another group of researchers [ 101 ] proposed a decision support system using information extraction from online documents through text and data mining. They focused on evidence based management, quality control, and best practice recommendations for medical prescriptions.
Length of Stay (LOS) is another important indicator of cost and quality of care. Accurate prediction of LOS can lead to efficient management of hospital beds and resources. To predict LOS for CAD patients, researchers [ 98 ] compared multiple models—SVM, ANN, DT and an ensemble algorithm, combing SVM, C5.0, and ANN. Ensemble algorithm and SVM produced highest accuracy, 95.9% and 96.4% respectively. In contrast, ANN was least accurate with 53.9% accuracy wherein DT achieved 83.5% accuracy. Anticoagulant drugs, nitrate drugs, and diagnosis were the top three predictors along with diastolic blood pressure, marital status, sex, presence of comorbidity, and insurance status.
To predict healthcare quality, researchers [ 104 ] used sentiment analysis (computationally categorizing opinions into categories like positive, negative and neutral) on patients’ online comments about their experience. They found above 80% agreement between sentiment analysis from online forums and traditional paper based surveys on quality prediction (e.g., cleanliness, good behavior, recommendation). Proposed approach can be an inexpensive alternative to traditional surveys and reports to measure healthcare quality.
Identification of influential factors in insurance coverage using data mining can aid insurance providers and regulators to design targeted service, additional service or proper allocation of resources to increase coverage rates. To develop a classification model to identify health insurance coverage, researchers [ 103 ] used data mining techniques. Based on 23 socio-economic, lifestyle and demographic factors, they developed a classification model with two classes, Insured and uninsured. The model was solved by ANN and DT. ANN provided 4% more accuracy than DT in predicting health insurance coverage. Among the factors, income, employment status, education, and marital status were the most important predictive factors of insurance coverage.
Among patients with lung cancer, researchers [ 97 ] investigated healthcare resource utilization (i.e., the number of visits to the medical oncologists) characteristics. They used DT, ANN and LR separately and an ensemble algorithm combining DT and ANN which resulted in the greatest accuracy (60% predictive accuracy). DT was employed to identify the important predictive features (among demographics, diagnosis, and other medical information) and ANN for classification. Data mining revealed that the utilization of healthcare resources by lung cancer patients is “supply-sensitive and patient sensitive” where supply represents availability of resources in certain region and patient represents patient preference and comorbidity. A resource allocation monitoring model for better management of primary healthcare network has also been developed [ 99 ]. Researchers considered the primary-care network as a collection of hierarchically connected modules given that patients could visit multiple physicians and physicians could have multiple care location, which is an indication of imbalanced resource distribution (e.g., number of physicians, care locations). The first level of the hierarchy consisted of three modules: health activities, population, and health resources. The second level monitored the healthcare provider availability and dispersion. The third level considered the actual visits, physicians and their availability, accessibility, and unlisted (i.e., without any assigned physician) patients. The top level of this network conducted an overall assessment of the network and made allocation accordingly. This hierarchical model was developed for a specific region in Slovenia, however, it could be easily adapted for any other region.
Overuse of screening and tests by physicians also contributes to inefficiencies and excess costs [ 102 ]. Current practice in pathology diagnosis is limited by disease focus. As an alternative to disease based system, researchers [ 102 ] used data mining in cooperation with case-based reasoning to develop an evidence based decision support system to decrease the use of unnecessary tests and reduce costs.
4.2.3. Patient Management
Patient management involves activities related to efficient scheduling and providing care to patients during their stay in a healthcare institute. Researchers [ 105 ] developed an efficient scheduling system for a rural free clinic in the United States. They proposed a hybrid system where data mining was used to classify the patients and association rule mining was used to assign a “no-show” probability. Results obtained from data mining were used to simulate and evaluate different scheduling techniques. On the other hand, these schedules could be divided into visits with administrative purposes and medical purposes. Researchers [ 108 ] suggested that patients who visit the health center for administrative purposes take less time than the patients with medical reasons. They proposed a predictive model to forecast the number of visits for administrative purposes. Their model improved the scheduling system with time saving of 21.73% (660,538 min). In contrast to administrative information/task seeking patients, some patients come for medical care very frequently and consume a large percentage of clinical workload [ 107 ]. Identifying the risk factors for frequent visit to health centers can help in reducing cost and resource utilization. A study among 85 working age “frequent attenders” identified the primary risk factors using Bayesian classification technique. The risk factors are, “high body mass index, alcohol abstinence, irritable bowel syndrome, low patient satisfaction, and fear of death” [ 107 ].
Improving publicly reported patient safety outcomes is also critical to healthcare institutions. Falls are one such outcome and are the most common and costly source of injury during hospitalization [ 110 ]. Researchers [ 109 ] analyzed the important factors related to patient falls during hospitalization. First, the authors selected significant features by Chi-square test (10 features out of 72 fall related variables were selected) and then applied ANN to develop a predictive model which achieves 0.77 AUC value. Stepwise logistic regression achieved 0.42 AUC value with 3 important variables. Both models showed that the fall assessment by nurses and use of anti-psychotic medication are associated with a lower risk of falls, and the use of diuretics is associated with an increased risk of falls. Another group of researchers [ 110 ] used fall related injury data to validate the structured information in EMR from clinical notes with the help of text mining. A group of nurses manually reviewed the electronic records to separate the correct documents from the erroneous ones which was considered as the basis of comparison. Authors employed both supervised (using a portion of manually labeled files as training set) and unsupervised technique (without considering the file labels) to classify and cluster the records. The unsupervised technique failed to separate the fare documents from the erroneous ones, wherein supervised technique performed better with 86% of fare documents in one cluster. This method can be applicable to semi-automate the EMR entry system.
4.2.4. Other Applications
Data mining has beed applied [ 111 ] to investigate the relationship between physician’s training at specific schools, procedures performed, and costs of the procedure. Researchers explored this relationship at three level: (1) they explored the distribution of procedures performed; (2) the relationship between procedures performed by physician and their alma mater—the institute that a doctor attended or got his/her degree from; and (3) geographic distribution of amount billed and payment received. This study suggested that medical school training does relate to practice in terms of procedures performed and bill charged. Patients can also provide useful information about physicians and their performance. Another group of researchers [ 112 ] used topic modeling algorithm—Latent Dirichlet Allocation (LDA)—to understand patients’ review of physicians and their concerns.
Data mining has also been applied [ 115 ] to analyze the information seeking behavior of health care professionals, and to assess the feasibility of measuring drug safety alert response from the usage logs of online medical information resources. Researchers analyzed two years of user log-in data in UpToDate website to measure the volume of searches associated with medical conditions and the seasonal distribution of those searches. In addition, they used a large collection of online media articles and web log posts as they characterized food and drug alert through the changes in UpToDate search activity compared to the general media activity. Some researchers [ 113 ] examined changes of key performance indicators (KPIs) and clinical workload indicators in Greek National Health System (NHS) hospitals with the help of data mining. They found significant changes in KPIs when necessary adjustments (e.g., workload) were made according to the diagnostic related group. The results remained for general hospitals like cancer hospitals, cardiac surgery as well as small health centers and regional hospitals. Their findings suggested that the assessment methodology of Greek NHS hospitals should be re-evaluated in order to identify the weaknesses in the system, and improve overall performance. And in home healthcare, another group of researchers [ 116 ] reviewed why traditional statistical analysis fails to evaluate the performance of home healthcare agencies. The authors proposed to use data mining to identify the drivers of home healthcare service among patients with heart failure, hip replacement, and chronic obstructive pulmonary disease using length of stay and discharge destination.
The relationship between epidemiological and genetic evidence and post market medical device performance has been evaluated using HCUPNet data [ 114 ]. This feasibility study explored the potential of using publicly accessible data for identifying genetic evidence (e.g., comorbidity of genetic factors like race, sex, body structure, and pneumothorax or fibrosis) related to devices. It focused on the ventilation-associated iatrogenic pneumothorax outcome in discharge of mechanical ventilation and continuous positive airway pressure (CPAP). The results demonstrated that genetic evidence-based epidemiologic analysis could lead to both cost and time efficient identification of predictive features. The literature of data mining applications in healthcare administration encompasses efficient patient management, healthcare cost reduction, quality of care, and data warehousing to facilitate analytics. We identified four studies that used cloud-based computing and analytical platforms. Most of the research proposed promising ideas, however, they do not provide the results and/or challenges during and after implementation. An ideal example of implementation could be the study of efficient appointment scheduling of patients [ 108 ].
4.3. Healthcare Privacy and Fraud Detection
Health data privacy and medical fraud are issues of prominent importance [ 118 ]. We reviewed four articles—displayed and described in Table 7 —that discussed healthcare privacy and fraud detection.
List of papers in healthcare privacy and fraud detection.
Reference | Problem Analyzed | Data Source |
---|---|---|
[ ] | Cloud based big data framework to ensure data security | Not specified |
[ ] | Weakness in de-identification or anonymization of health data | MedHelp and Mp and Th1 (Medicare social networking sites) |
[ ] | Automatic and systematic detection of fraud and abuse | Bureau of National Health Insurance (BNHI) in Taiwan. |
[ ] | Novel algorithm to protect data privacy | Hong Kong Red Cross Blood Transfusion Service (BTS) |
The challenges of privacy protection have been addressed by a group of researchers [ 122 ] who proposed a new anonymization algorithm for both distributed and centralized anonymization. Their proposed model performed better than K-anonymization model in terms of retaining data utility without losing much data privacy (for K = 20, the discernibility ratio—a normalized measure of data quality—of the proposed approach and traditional K-anonymization method were 0.1 and 0.4 respectively). Moreover, their proposed algorithm could handle large scale, high dimensional datasets. To address the limitations of today’s healthcare information systems—EHR data systems limited by lack of inter-operability, data size, and security—a mobile cloud computing-based big data framework has been proposed [ 119 ]. This novel cloud-based framework proposed storing EHR data from different healthcare providers in an Internet provider’s facility, offering providers and patients different levels of access and authority. Security would be ensured by using encryption algorithms, one-time passwords, or 2-factor authentication. Big data analytics would be handled using Google big query or MapReduce software. This framework could reduce cost, increase efficiency, and ensure security compared to the traditional technique which uses de-identification or anonymization technique. This traditional technique leaves healthcare data vulnerable to re-identification. In a case study, researchers demonstrated that hackers can make association between small pieces of information and can identify patients [ 120 ]. The case study made use of personal information provided in two Medicare social networking sites, MedHelp and Mp and Th1 to identify an individual.
Detection of fraud and abuse (i.e., suspicious care activity, intentional misrepresentation of information, and unnecessary repetitive visits) uses big data analytics. Using gynecological hospital data, researchers [ 121 ] developed a framework from two domain experts manually identifying features of fraudulent cases from a data pool of treatment plans doctors frequently follow. They applied this framework to Bureau of National Health Insurance (BNHI) data from Taiwan; their proposed framework detected 69% of the fraudulent cases, which improved the existing model that detected 63% of the fraudulent cases.
In summary, patient data privacy and fraud detection are of major concern given increasing use of social media and people’s tendency to put personal information on social media. Existing data anonymization or de-identification techniques can become less effective if they are not designed considering the fact that a large portion of our personal information is now available on social media.
4.4. Mental Health
Mental illness is a global and national concern [ 123 ]. According to the National Survey on Drug Use and Health (NSDUH) data from 2010 to 2012, 52.2% of U.S. population had either mental illness, or substance abuse/dependence [ 124 ]. Additionally, nearly 30 million people in the U.S. suffer from anxiety disorders [ 125 ]. Table 8 summarizes the four articles we reviewed that apply data mining in analyzing, diagnosing, and treating mental health issues.
List of data mining application in mental health with data sources.
Reference | Problem Analyzed | Data Source |
---|---|---|
[ ] | Identification and intervention of developmental delay of children | Yunlin Developmental Delay Assessment Center |
[ ] | Personalized treatment for anxiety disorder | Volunteer participants |
[ ] | Abnormal behavior detection | Through experiment with human subject |
[ ] | Mental health diagnosis and exploration of psychiatrist’s everyday practice | Queensland Schizophrenia Research center |
To classify developmental delays of children based on illness, researchers [ 126 ] examined the association between illness diagnosis and delays by building a decision tree and finding association between cognitive, language, motor, and social emotional developmental delays. This study has implications for healthcare professionals to identify and intervene on delays at an early stage. To assist physicians in monitoring anxiety disorder, another group of researchers [ 125 ] developed a data mining based personalized treatment. The researchers used Context Awareness Information including static (personal information like, age, sex, family status etc.) and dynamic (stress, environmental, and symptoms context) information to build static and dynamic user models. The static model contained personal information and the dynamic model contained four treatment-supportive services (i.e., lifestyle and habits pattern detection service, context and stress level pattern detection service, symptoms and stress level pattern detection service, and stress level prediction service). Relations between different dynamic parameters were identified in first three services and the last service was used for stress level prediction under different scenarios. The model was validated using data from 27 volunteers who were selected by anxiety measuring test.
To predict early diagnosis for mental disorders (e.g., insomnia, dementia), researchers developed a model detecting abnormal physical activity recorded by a wearable device [ 127 ]. They performed two experiments to compare the development of a reference model using historical user physical movement data. In the first experiment, users wore the watch for one day and based on that day, a reference behavior model was developed. After 22 days, the same user used it again for a day and abnormality was detected if the user’s activities were significantly different from the reference model. In the second experiment, users used the watch regularly for one month. Abnormality was detected with a fuzzy valuation function and validated with user’s reported activity level. In both experiments, users manually reported their activity level, which was used as a validating point, only two out of 26 abnormal events were undetected. Through these two experiments, the researchers claimed that their model could be useful for both online and offline abnormal behavior detection as the model was able to detect 92% of the unusual events.
To classify schizophrenia, another study [ 128 ] used free speech (transcribed text) written or verbalized by psychiatric patients. In a pool of patients with schizophrenia and control subjects, using supervised algorithms (SVM and DT), they discriminated between patients with schizophrenia and normal control patients. SVM achieved 77% classification accuracy whereas DT achieved 78% accuracy. When they added patients with mania to the pool, they were unable to differentiate patients with schizophrenia.
Use of data analytics in diagnosing, analyzing, or treating mental health patients is quite different than applying analytics to predict cancer or diabetes. Context of data (static, dynamic, or unobservable environment) seemed more important than volume in this case [ 125 ], however, this is not always adopted in literature. A model without situational awareness (a context independent model) may lose predictive accuracy due to the confounding effect of surrounding environment [ 129 ].
4.5. Public Health
Seven articles addressed issues that were not limited to any specific disease or a demographic group, which we classified as public health problems. Table 9 contains the list of papers considering public health problems with data sources.
List of data mining application in public health with data sources.
Reference | Problem Analyzed | Data Source |
---|---|---|
[ ] | Designing preventive healthcare programs | World Health Organization (WHO) |
[ ] | Predicting the peak of health center visit due to influenza | Military Influenza case data provided by US Armed Forces Health Surveillance Center and Environmental data from US National Climate Data Center |
[ ] | Contrast patient and customer loyalty, estimating Customer lifetime value, and identifying the targeted customer | Iranian Public Hospital data extracted from Hospital information system |
[ ] | Understanding the information seeking behavior of public and professionals on infectious disease | National electronic Library of Infection and National Resource of Infection Control, Google Trends, and relevant media coverage (LexisNexis). |
[ ] | Knowledge extraction for non-expert user through automation of data mining process | Brazilian health ministry |
[ ] | Innovative use of data mining and visualization techniques for decision-making | Slovenian national Institute of Public Health |
[ ] | Real-time emergency response method using big data and Internet of Things | UCI machine learning repository |
To make data mining accessible to non-expert users, specifically public health decision makers who manage public cancer treatment programs in Brazil, researchers [ 134 ] developed a framework for an automated data mining system. This system performed a descriptive analysis (i.e., identifying relationships between demography, expenditure, and tumor or cancer type) for public decision makers with little or no technical knowledge. The automation process was done by creating pre-processed database, ontology, analytical platform and user interface.
Analysis of disease outbreaks has also applied data analytics. [ 131 , 133 ] Influenza, a highly contagious disease, is associated with seasonal outbreaks. The ability to predict peak outbreaks in advance would allow for anticipatory public health planning and interventions to lessen the effect of the outbreaks. To predict peak influenza visits to U.S. military health centers, researchers [ 131 ] developed a method to create models using environmental and epidemiological data. They compared six classification algorithms—One-Classifier 1, One-Classifier 2 [ 137 ], a fusion of the One-Classifiers, DT, RF, and SVM. Among them, One-Classifier 1 was the most efficient with F-score 0.672 and SVM was second best with F-score 0.652. To examine the factors that drive public and professional search patterns for infectious disease outbreaks another group of researchers [ 133 ] used online behavior records and media coverage. They identified distinct factors that drive professional and layperson search patterns with implications for tailored messaging during outbreaks and emergencies for public health agencies.
To store and integrate multidimensional and heterogeneous data (e.g., diabetes, food, nutrients) applied to diabetes management, but generalizable to other diseases researchers [ 130 ] proposed an intelligent information management framework. Their proposed methodology is a robust back-end application for web-based patient-doctor consultation and e-Health care management systems with implications for cost savings.
A real-time medical emergency response system using the Internet of Things (networking of devices to facilitate data flow) based body area networks (BANs)—a wireless network of wearable computing devices was proposed by researchers [ 136 ]. The system consists of “Intelligent Building”—a data analysis model which processes the data collected from the sensors for analysis and decision. Though the author claims that the proposed system had the capability of efficiently processing wireless BAN data from millions of users to provide real-time response for emergencies, they did not provide any comparison with the state-of-the-art methods.
Decision support tools for regional health institutes in Slovenia [ 135 ] have been developed using descriptive data mining methods and visualization techniques. These visualization methods could analyze resource availability, utilization and aid to assist in future planning of public health service.
To build better customer relations management at an Iranian hospital, researchers [ 132 ] applied data mining techniques on demographic and transactions information. The authors extended the traditional Recency, Frequency, and Monetary (RFM) model by adapting a new parameter “Length” to estimate the customer life time value (CLV) of each patient. Patients were separated into classes according to estimated CLV with a combination of clustering and classification algorithms. Both DT and ANN performed similarly in classification with approximately 90% accuracy. This type of stratification of patient groups with CLV values would help hospitals to introduce new marketing strategies to attract new customers and retain existing ones.
The application of data mining to public health decision-making has become increasingly common. Researchers utilized data mining to design healthcare programs and emergency response, to identify resource utilization, patient satisfaction as well as to develop automated analytics tool for non-expert users. Continuation of this effort could lead to a patient-centered, robust healthcare system.
4.6. Pharmacovigilance
Pharmacovigilance involves post-marketing monitoring and detection of adverse drug reactions (ADRs) to ensure patient safety [ 138 ]. The estimated annual social cost of ADR events exceeds one billion dollars, making it an important part of healthcare system [ 139 ]. Characteristics of the nine papers addressing pharmacovigilance are displayed in Table 10 .
List of data mining application in pharmacovigilance with data sources.
Reference | Problem Analyzed | Data Source |
---|---|---|
[ ] | Sentiment and network analysis based on social media data to find ADR signal | Cancer discussion forum websites |
[ ] | ADR signal detection from multiple data sources | Food and Drug Administration (FDA) database and publicly available electronic health record (HER) in US |
[ ] | ADR detection from EPR through temporal data analysis | Danish psychiatric hospital |
[ ] | ADR (hypersensitivity) signal detection of six anticancer agents | FDA released AERS reports (2004–2009), US |
[ ] | ADR caused by multiple drugs | FDA released AERS reports, US |
[ ] | ADR due to Statins used in Cardiovascular disease (CVD) and muscular and renal failure treatment | FDA released AERS reports, US |
[ ] | Creating a ranked list of Adverse Events (AEs) | EHR form European Union |
[ ] | Detecting ADR signals of Rosuvastatins compared to other statins users | Health Insurance Review and Assessment Service claims database (Seoul, Korea) |
[ ] | Unexpected and rare ADR detection technique | Medicare Benefits Scheme (MBS) and Queensland Linked Data Set (QLDS) |
Researchers considered muscular and renal AEs caused by pravastatin, simvastatin, atorvastatin, and rosuvastatin by applying data mining techniques to the FDA’s Adverse Event Reporting System (FAERS) database reports from 2004 to 2009 [ 143 ]. They found that all statins except simvastatin were associated with muscular AE; rosuvastatin had the strongest association. All statins, besides atorvastatin, were associated with acute renal failure. The criteria used to identify significant association were: proportional reporting ratio (PRR), reporting odds ratio (ROR), information component (IC), and empirical Bayes geometric mean (EBGM). In another study of AEs related to statin family, researchers used a Korean claims database [ 145 ] and showed that a relative risk-based data-mining approach successfully detected signals for rosuvastatin.
Three more studies used the FDA’s AERS report database. In an examination of ADR “hypersensitivity” to six anticancer agents [ 142 ] data mining results showed that Paclitaxel is associated with mild to lethal reaction wherein Docetaxel is associated to lethal reaction, and the other four drugs were not associated to hypersensitivity [ 142 ]. Another researcher [ 139 ] argued that AEs can be caused not only by a single drug, but also by a combination of drugs [ 140 ]. They showed that that 84% of the AERs reports contain an association between at least one drug and two AEs or two drugs and one AE. Another group [ 138 ] increased precision in detecting ADRs by considering multiple data sources together. They achieved 31% (on average) improvement in identification by using publicly available EHRs in combination with the FDA’s AERS reports.
Furthermore, dose-dependent ADRs have been identified by researchers using models developed from structured and unstructured EHR data [ 141 ]. Among the top five drugs associated with ADRs, four were found to be related to dose [ 141 ]. Pharmacovigilance activity has also been prioritized using unstructured text data in EHRs [ 144 ]. In traditional pharmacovigilance, ADRs are unknown. While looking for association between a drug and any possible ADR, it is possible to get false signals. Such false signals can be avoided if a list of possible ADRs is already known. Researchers [ 144 ] developed an ordered list of 23 ADRs which can be very helpful for future pharmacovigilance activities. To detect unexpected and rare ADRs in real-world healthcare administrative databases, another group of researchers [ 146 ] designed an algorithm—Unexpected Temporal Association Rules (UTARs)—that performs more effectively than existing techniques.
We identified one study that used data outside of adverse event reports or HER data. For early detection of ADR, one group of researchers used online forums [ 140 ]. They identified the side effect of a specific drug called “Erlotinib” used for lung cancer. Sentiment analysis—a technique of categorizing opinions—on data collected from different cancer discussion forums showed that 70% of users had a positive experience after using this drug. Users most frequently reported were acne and rash. Apart from pharmacovigilance, this type of analysis can be very helpful for the pharmaceutical companies to analyze customer feedback. Researchers can take advantage of the popularity of social media and online forums for identifying adverse events. These sources can provide signals of AEs quicker than FDA database as it takes time to update the database. By the time AE reports are available in the FDA database, there could already be significant damage to patient and society. Moreover, it can help to avoid the limitations of FDA AERS database like biased reporting and underreporting [ 141 ].
5. Theoretical Study
Twenty-five of the articles we reviewed focus on the theoretical aspects of the application of data mining in healthcare including designing the database framework, data collection, and management to algorithmic development. These intellectual contributions extend beyond the analytical perspective of data—descriptive, predictive or prescriptive analytics—to the sectors and problems highlighted in Table 11 .
Problem analyzed in theoretical studies.
Sector Highlight | Reference | Problem Analyzed |
---|---|---|
Disease Control, Current situation of different diseases (infection, epidemic, cancer, mental health) | [ ] | Proposed an idea for dynamic clinical decision support |
[ ] | Described current situation of infection control and predicted future challenges in this sector | |
[ ] | Described activities taken by national organization to control disease and provide better health care | |
[ ] | Reviewed efficient collection and aggregation of big data and proposed an intelligence based learning framework to help prevent cancer | |
Data quality, database framework and uncertainty quantification | [ ] | Considered the management of uncertainty originating from data mining. |
[ ] | Contemplated the quality of the data when collected from multimodal sources | |
[ ] | Provided the structure of the database of CancerLinQ that comprised of 4 key steps | |
[ ] | Described five major problems that need to be tackled in order to have an effective integration of big data analytics and VPH modeling in healthcare | |
[ ] | Discuss the issues of data quality in the context of big data health care analytics | |
[ ] | Discussed the necessity of proper management and confidentiality of healthcare data along with the benefit of big data analytics | |
Healthcare policy making | [ , , ] | Addressed the challenges faced in implementing health care policies and considered the ethical and legal issues of performing predictive analysis on health care big data |
[ ] | Focused on the US federal regulatory pathway by which CancerLinQ will have legislative authority to use the patients’ records and the approach of ASCO toward the organizing and supervising the information | |
Patient Privacy | [ ] | Focused on ensuring patient privacy while collecting data, storing them and using them for analysis aimed to eliminate discrimination in the health care provided to patients. |
[ ] | Spotted light on ensuring Privacy and security while collecting Personal Health care Information (PHI) | |
[ ] | Highlighted those strategies appropriate for data mining from physicians’ prescriptions while maintaining the patient’s privacy | |
Personalized health care | [ ] | Transforming big data into computational models to provide personalized health care |
[ ] | Development of informed decision-making frameworks for person centered health care | |
[ ] | Looked into the availability of big data and the role of biomedical informatics on the personalized medicine. Also, emphasized on the ethical concerns related to personalized medicines | |
Others | [ ] | Finding the aspects of big data that are most relevant to Health care |
[ ] | Selecting dynamic simulation modeling approach based on the availability and type of big data | |
[ ] | Quantifying performance in the delivery of medical services | |
[ ] | Identifying high risk patients to ensure better care, and explored the analytics procedure, algorithms and challenges to implement analytics | |
[ ] | Addressed barriers for the exploitation of health data in Europe | |
[ ] | Analyzed the opportunity and obstacles in applying predictive analytics based on big data in case of evaluating emergency care | |
[ ] | Provided an overview of the uses of the Person-Event Data Environment to perform command surveillance and policy analysis for Army leadership | |
[ ] | Development of big data analytics in healthcare and future challenges |
The existing theoretical literature on disease control highlighted the current state of epidemics, cancer and mental health. To help physicians make real-time decisions about patient care, one group of researchers [ 147 ] proposed a real-time EMR data mining based clinical decision support system. They emphasized the need to have an anonymized EMR database which can be explored by using a search engine similar to web search engine. In addition, they focused on designing a framework for next generation EMR-based database that can facilitate the clinical decision-making process, and is also capable of updating a central population database once patients’ recent (new) clinical records are available. Another researcher [ 148 ] forecasted future challenges in infection control that entails the importance of having timely surveillance system and prevention programs in place. To that end, they necessitate the formation, control and utilization of fully computerized patient record and data-mining-derived epidemiology. Finally, they recommended performance feedback to caregivers, wide accessibility of infection prevention tools, and access to documents like lessons learned and evidence-based best practices to strengthen the infection control, surveillance, and prevention scheme. Authors in [ 150 ] addressed the activities executed by national Institute of Mental Health (NIMH) in collaboration with other state organizations (e.g., Substance Abuse and Mental Health Service Administration (SAMSHSA), Center for Mental Health Service (CMHS) to promote optimal collection, pooling/aggregation, and use of big data to support ongoing and future researches of mental health practices. The outcome summary showcased that effective pooling/aggregation of state-level data from different sources can be used as a dashboard to set priorities to improve service qualities, measure system performance and to gain specific context-based insights that are generalizable and scalable across other systems, leading to a successful learning-based mental health care system. Another group of researchers [ 150 ] outlined the barriers and potential benefits of using big data from CancerLinQ (a quality and measurement reporting system as an initiative of the American Society of Clinical Oncology (ASCO) that collects information from EHRs of cancer patients for oncologists to improve the outcome and quality of care they provide to their patients). However, the authors also mentioned that these benefits are contingent upon the confidence of the patients, encouraging them to share their data out of the belief that their health records would be used appropriately as a knowledge base to improve the quality of the health care of others, as it is for themselves. This motivated ASCO to ensure that proper policies and procedures are in place to deal with the data quality, data security and data access, and adopt a comprehensive regulatory framework to ensure patients’ data privacy and security.
Another group of researchers [ 151 ] data quality and database management to quantify, and consequentially understand the inherent uncertainty originating from radiology reporting system. They discussed the necessity of having a structured reporting system and emphasized the use of standardize language, leading to Natural Language Processing (NLP). Furthermore, they also indicated the need for creating a Knowledge Discovery Database (KDD) which will be consistent to facilitate the data-driven and automated decision support technologies to help improving the care provided to patients based on enhanced diagnosis quality and clinical outcome. A group of authors in [ 152 ] pointed that the success derived from the current trend of big-data analytics largely depends on how better the quality of the data collected from variety of sources are ensured. Their findings imply that the data quality should be assessed across the entire lifecycle of health data by considering the errors and inaccuracies stemmed from multiple of sources, and should also quantify the impact that data collection purpose on the knowledge and insights derived from the big data analytics. For that to ensure, they recommend that enterprises who deal with healthcare big data should develop a systematic framework including custom software or data quality rule engines, leading to an effective management of specific data-quality related problems. Researchers in [ 155 ] uncovered the lack of connection between phenomenological and mechanistic models in computational biomedicines. They emphasized the importance of big data which, when successfully extracted and analyzed, followed by the combination with Virtual Physiological Human (VPH)—an initiative to encourage personalized healthcare—can afford with effective and robust medicine solutions. In order for that to happen, they mentioned some challenges (e.g., confidentiality, volume and complexity of big data; integration of bioinformatics, systems biology and phenomics data; efficient storage of partial or complete data within organization to maximize the performance of overall predictive analytics) and concluded that these need to be addressed for successful development of big data technologies in computational medicines, enabling their adoption in clinical settings. Even though big data can generate significant value in modern healthcare system, researchers in [ 154 ] stated that without a set of proper IT infrastructures, analytical and visualization tools, and interactive interfaces to represent the work flows, the insights generated from big data will not be able to reach its full potential. To overcome this, they recommended that health care organizations engaging in data sharing devise new policies to protect patients’ data against potential data breaches.
Three papers [ 155 , 156 , 157 ] considered health care policies and ethical and legal issues. One [ 155 ] outlined a national action plan to incorporate sharable and comparable nursing data beyond documentation of care into quality reporting and translational research. The plan advocates for standardized nursing terminologies, common data models, and information structures within EHRs. Another paper [ 157 ] analyzed the major policy, ethical, and legal challenges of performing predictive analytics on health care big data. Their proposed recommendations for overcoming challenges raised in the four-phase life cycle of a predictive analytics model (i.e., data acquisition, model formulation and validation, testing in real-world setting and implementation and use in broader scale) included developing a governance structure at the earliest phase of model development to guide patients and participating stakeholders across the process (from data acquisition to model implementation). They also recommended that model developers strictly comply with the federal laws and regulations in concert with human subject research and patients information privacy when using patients’ data. And another paper [ 156 ] explored four central questions regarding: (i) aspects of big-data most relevant to health care, (ii) policy implications, (iii) potential obstacles in achieving policy objectives, and (iv) availability of policy levers, particularly for policy makers to consider when developing public policy for using big data in healthcare. They discussed barriers (including ensuring transparency among patients and health care providers during data collection) to achieve policy objectives based on a recent UK policy experiment, and argued for providing real-life examples of ways in which data sharing can improve healthcare.
Three papers [ 158 , 159 , 160 ] offered examples of realistic ways such as establishing policy leadership and risk management framework combining commercial and health care entities to recognize existing privacy related problem and devise pragmatic and actionable strategies of maintaining patient privacy in big data analytics. One paper [ 158 ] provided a policy overview of health care and data analytics, outlined the utility of health care data from a policy perspective, reviewed a variety of methods for data collection from public and private sources, mobile devices and social media, examined laws and regulations that protect data and patients’ privacy, and discussed a dynamic interplay among three aspects of today’s big data driven personal health care—policy goals to tackle both cost, population health problem and eliminate disparity in patient care while maintaining their privacy. Another study [ 159 ] proposed a Secure and Privacy Preserving Opportunistic Computing (SPOC) framework to be used in healthcare emergencies focused on collecting intensive personal health information (through mobile devices like smart phone or wireless sensors) with minimal privacy disclosure. The premise of this framework is that when a user of this system (called medical user) faces any emergency, other users in the vicinity with similar disease or symptom (if available) can come to help that user before professional help arrives. It is assumed that two persons with similar disease are skilled enough to help each other and the threshold of similarity is controlled by the user. And in physician prescribing—another paper [ 160 ] identified strategies for data mining from physicians’ prescriptions while maintaining patient privacy.
Theoretical research on personalized-health care services—treatment plans designed for someone based on the susceptibility of his/her genomic structure to a disease—also emerged from the literature review. One study [ 161 ] highlighted the potential of powerful analytical tools to open an avenue for predictive, preventive, participatory, and personalized (P4) medicine. They suggested a more nuanced understanding of the human systems to design an accurate computational model for P4 medicine. Reviewing the research paradgims of current person-centered approaches and traditions, another study [ 162 ] advocated a transdisciplinary and complex systems approach to improve the field. They synthesized the emerging aproaches and methodologies and highlighted the gaps between academic research and accessibility of evaluation, informatics, and big data from health information systems. Another paper [ 163 ] reviewed the availability of big data and the role of biomedical informatics in personalized medicine, emphasizing the ethical concerns related to personalized medicines and health equity. Personalized medicine has a potential to reduce healthcare cost, however, the researchers think it can create race, income, and educational disparity. Certain socioeconomic and demographic groups currently have less or no access to healthcare and data driven personalized medicine will exclude those groups, increasing disparities. They also highlighted the impact of EHRs and CDWs on the field of personalized medicine through acclerated research and decreased the delivery time of new technologies.
A myriad of extant theoretical points has also been identified in the literature. These topics range from exploiting big data to: study the paradigm shift in healthcare policy and management from prioritizing volume to value [ 164 , 167 ]; aid medical device consumers in their decision-making [ 166 ]; improve emergency departments [ 169 ]; perform command surveillance and policy analysis for Army leadership [ 170 ]; to comparing different simulation methods (i.e., systems dynamics, discrete event simulation and agent based modeling) for specific health care system problems like resource allocation, length of stay [ 165 ]; to the ethical challenges of security, management, and ownership [ 170 ]. Another researcher outlined the challenges the E.U. is facing in data mining given numerous historical, technical, legal, and political barriers [ 168 ].
6. Future Research and Challenges
Data mining has been applied in many fields including finance, marketing, and manufacturing [ 172 ]. Its application in healthcare is becoming increasingly popular [ 173 ]. A growing literature addresses the challenges of data mining including noisy data, heterogeneity, high dimensionality, dynamic nature, computational time. In this section, we focus on future research applications including personalized care, information loss in preprocessing, collecting healthcare data for research purposes, automation for non-experts, interdisciplinarity of study and domain expert knowledge, integration into the healthcare system, and prediction-specific to data mining application and integration in healthcare.
- Personalized care
The EMR is increasingly used to document demographic and clinician patient information [ 1 ]. EMR data can be utilized to develop personalized care plans, enhancing patient experience [ 162 ] and improving care quality.
- Loss of information in pre-processing
Pre-processing of data, including handling missing data, is the most time-consuming and costly part of data mining. The most common method used in the papers reviewed was deletion or elimination of missing data. In one study, approximately 46.5% of the data and 363 of 410 features were eliminated due to missing values [ 49 ]. In another, researchers [ 98 ] were only able to use 2064 of 4948 observations (42%) [ 98 ]. By eliminating missing value cases and outliers, we are losing a significant amount of information. Future research should focus on finding a better method of missing value estimation than elimination. Moreover, data collection techniques should be developed or modified to avoid this issue.
Similar to missing data, deletion or elimination is a common way to handle outliers [ 174 ]. However, as illustrated in one of the studies we reviewed [ 48 ], outliers can be used to gain information about rare forms of diseases. Instead of neglecting the outliers, future research should analyze them to gain insight.
- Collecting healthcare data for research purpose
Traditionally, the primary objective of data collection in healthcare is documentation of patient condition and care planning [ 109 ]. Including research objectives in the data collection process through structured fields could yield more structured data with fewer cases of error and missing values [ 64 ]. A successful example of data collection for research purpose is the Study of Health in Pomerania (SHIP) [ 175 ]. The objective of SHIP was to identify common diseases, population level risk factors, and overall health of people living in the north-east region of Germany. This study only suffered from one “mistake” for every 1000 data entries [ 175 ] which ensures a structured form of data with high reliability, less noise and fewer missing values. We can take advantage of current documentation processes (EMR or EHR) by modifying them to collect more reliable and structured data. Long-term vision and planning is required to introduce research purpose in healthcare data collection.
- Automation of data mining process for non-expert users
The end users of data mining in healthcare are doctors, nurses, and healthcare professionals with limited training in analytics. One solution for this problem is to develop an automated (i.e., without human supervision) system for the end users [ 134 ]. A cloud-based automated structure to prevent medical errors could also be developed [ 95 ]; but the task would be challenging as it involves different application areas and one algorithm will not have similar accuracy for all applications [ 134 ].
- Interdisciplinary nature of study and domain expert knowledge
Healthcare analytics is an interdisciplinary research field [ 134 ]. As a form of analytics, data mining should be used in combination with expert opinion from specific domains—healthcare and problem specific (i.e., oncologist for cancer study, cardiologist for CVD) [ 106 ]. Approximately 32% of the articles in analytics did not utilize expert opinion in any form. Future research should include members from different disciplines including healthcare.
- Integration in healthcare system
Very few articles reviewed made an effort to integrate the data mining process into the actual decision-making framework. The impact of knowledge discovery through data mining on healthcare professional’s workload and time is unclear. Future studies should consider the integration of the developed system and explore the effect on work environments.
- Prediction error and “The Black Swan” effect
In healthcare, it is better not to predict than making an erroneous prediction [ 46 ]. A little under half of the literature we identified in analytics is dedicated to prediction but, none of the articles discussed the consequence of a prediction error. High prediction accuracy for cancer or any other disease does not ensure an accurate application to decision-making.
Moreover, prediction models may be better at predicting commonplace events than rare ones [ 176 ]. Researchers should develop more sophisticated models to address the unpredictable, “The Black Swan” [ 176 ]. One study [ 101 ] addressed a similar issue in evidence based recommendations for medical prescriptions. Their concern was, how much evidence should be sufficient to make a recommendation. Many of the studies in this review do not address these salient issues. Future research should address the implementation challenges of predictive models, especially how the decision-making process should adapt in case of errors and unpredictable incidents.
7. Conclusions
The development of an informed decision-making framework stems from the growing concern of ensuring a high value and patient-focused health care system. Concurrently, the availability of big data has created a promising research avenue for academicians and practitioners. As highlighted in our review, the increased number of publications in recent years corroborates the importance of health care analytics to build improved health care systems world-wide. The ultimate goal is to facilitate coordinated and well-informed health care systems capable of ensuring maximum patient satisfaction.
This paper adds to the literature on healthcare and data mining ( Table 1 ) as it is the first, to our knowledge, to take a comprehensive review approach and offer a holistic picture of health care analytics and data mining. The comprehensive and methodologically rigorous approach we took covers the application and theoretical perspective of analytics and data mining in healthcare. Our systematic approach starting with the review process and categorizing the output as analytics or theoretical provides readers with a more widespread review with reference to specific fields.
We also shed light on some promising recommendations for future areas of research including integration of domain-expert knowledge, approaches to decrease prediction error, and integration of predictive models in actual work environments. Future research should recommend ways so that the analytic decision can effectively adapt with the predictive model subject to errors and unpredictable incidents. Regardless of these insightful outcomes, we are not constrained to mention some limitations of our proposed review approach. The sole consideration of academic journals and exclusion of conference papers, which may have some good coverage in this sector is the prime limitation of this review. In addition to this, the search span was narrowed to three databases for 12 years which may have ignored some prior works in this area, albeit the increasing trend since 2005 and less number of publications before 2008 can minimize this limitation. The omission of articles published in languages other than English can also restrict the scope of this review as related papers written in other languages might be evident in the literature. Moreover, we did not conduct forward (reviewing the papers which cited the selected paper) and backward (reviewing the references in the selected paper and authors’ prior works) search as suggested by Levy and Ellis [ 31 ].
Despite these limitations, the systematic methodology followed in this review can be used in the universe of healthcare areas.
Supplementary Materials
The following are available online at http://www.mdpi.com/2227-9032/6/2/54/s1 , Table S1: PRISMA checklist, Table S2: Modified checklists and comparison, Table S3: Study characteristics, Table S4: Classification of reviewed papers by analytics type, application area, data type, and data mining techniques.
Author Contributions
Contribution of the authors can be summarized in following manner. Conceptualization: M.S.I., M.N.-E.-A.; Formal analysis: M.S.I., M.M.H., X.W.; Investigation: M.S.I., M.M.H., X.W.; Methodology: M.S.I.; Project administration: M.S.I., M.N.-E.-A.; Supervision: M.N.-E.-A.; Visualization: M.S.I., X.W.; Writing—draft: M.S.I., M.M.H., H.D.G.; Writing—review and editing: M.S.I., M.M.H., H.D.G., M.N.-E.-A.
Germack is supported by CTSA Grant Number TL1 TR001864 from the National Center for Advancing Translational Science (NCATS), a component of the National Institutes of Health (NIH). The content is solely the responsibility of the authors and does not necessarily represent the official views of this organization.
Conflicts of Interest
The authors declare no conflict of interest.
IEEE Account
- Change Username/Password
- Update Address
Purchase Details
- Payment Options
- Order History
- View Purchased Documents
Profile Information
- Communications Preferences
- Profession and Education
- Technical Interests
- US & Canada: +1 800 678 4333
- Worldwide: +1 732 981 0060
- Contact & Support
- About IEEE Xplore
- Accessibility
- Terms of Use
- Nondiscrimination Policy
- Privacy & Opting Out of Cookies
A not-for-profit organization, IEEE is the world's largest technical professional organization dedicated to advancing technology for the benefit of humanity. © Copyright 2024 IEEE - All rights reserved. Use of this web site signifies your agreement to the terms and conditions.
Log in using your username and password
- Search More Search for this keyword Advanced search
- Latest content
- Topic collections
- BMJ Journals
You are here
- Volume 27, Issue 1
- Target mechanisms of mindfulness-based programmes and practices: a scoping review
- Article Text
- Article info
- Citation Tools
- Rapid Responses
- Article metrics

- http://orcid.org/0000-0002-6939-2298 Shannon Maloney 1 ,
- Merle Kock 2 ,
- Yasmijn Slaghekke 1 ,
- Lucy Radley 3 ,
- Alba Lopez-Montoyo 4 ,
- Jesus Montero-Marin 1 , 5 , 6 ,
- http://orcid.org/0000-0002-8596-5252 Willem Kuyken 1
- 1 Department of Psychiatry , University of Oxford , Oxford , Oxfordshire , UK
- 2 Centre for the Psychology of Learning and Experimental Psychopathology , KU Leuven , Leuven , Flanders , Belgium
- 3 Department of Experimental Psychology , University of Oxford , Oxford , UK
- 4 Universitat Jaume I , Castello de la Plana , Comunitat Valenciana , Spain
- 5 Teaching, Research & Innovation Unit , Parc Sanitari Sant Joan de Déu , Sant Boi de Llobregat , Spain
- 6 Consortium for Biomedical Research in Epidemiology & Public Health (CIBER Epidemiology and Public Health - CIBERESP) , Madrid , Spain
- Correspondence to Dr Shannon Maloney, Psychiatry, University of Oxford, Oxford OX1 2JD, UK; shannon.maloney{at}psych.ox.ac.uk
Question Mindfulness-based programmes (MBPs) and practices have demonstrated effects in mental health and well-being, yet questions regarding the target mechanisms that drive change across the population remain unresolved.
Study selection and analysis Five databases were searched for randomised controlled trials that evaluate the indirect effects (IEs) of an MBP or mindfulness practice in relation to mental health and well-being outcomes through psychological mechanisms.
Findings 27 eligible studies were identified, with only four exploring mechanisms in the context of specific mindfulness practices. Significant IEs were reported for mindfulness skills, decentering and attitudes of mindfulness (eg, self-compassion) across different outcomes, population samples, mental health strategies and active comparators. Evidence gap maps and requirements for testing and reporting IEs are provided to help guide future work.
Conclusions Mindfulness skills, decentering and attitudes of mindfulness may be key intervention targets for addressing the mental health of whole populations. However, future work needs to address significant knowledge gaps regarding the evidence for alternative mechanisms (eg, attention and awareness) in relation to unique outcomes (eg, well-being), mental health strategies (ie, promotion) and active comparators. High-quality trials, with powered multivariate mediation analyses that meet key requirements, will be needed to advance this area of work.
Trial registration number 10.17605/OSF.IO/NY2AH.
- Adult psychiatry
- Depression & mood disorders
Data availability statement
All data relevant to the study are included in the article or uploaded as online supplemental information. Not applicable.
This is an open access article distributed in accordance with the Creative Commons Attribution 4.0 Unported (CC BY 4.0) license, which permits others to copy, redistribute, remix, transform and build upon this work for any purpose, provided the original work is properly cited, a link to the licence is given, and indication of whether changes were made. See: https://creativecommons.org/licenses/by/4.0/ .
https://doi.org/10.1136/bmjment-2023-300955
Statistics from Altmetric.com
Request permissions.
If you wish to reuse any or all of this article please use the link below which will take you to the Copyright Clearance Center’s RightsLink service. You will be able to get a quick price and instant permission to reuse the content in many different ways.
WHAT IS ALREADY KNOWN ON THIS TOPIC
Mindfulness-based programmes (MBPs) and practices have demonstrated effects in mental health and well-being, yet questions regarding the target mechanisms that drive change across the population remain unresolved.
WHAT THIS STUDY ADDS
This review aims to build on past reviews by (1) summarising the evidence for potential mechanisms underlying mindfulness practices as one key subcomponent of MBPs; (2) prioritising high quality and established formal analytical methods of mediation; and (3) examining the evidence for mechanisms in relation to outcomes that map across the population.
HOW THIS STUDY MIGHT AFFECT RESEARCH, PRACTICE OR POLICY
The hope is that the findings of this review will help inform future work and provide novel insight into the broader application of MBPs and the potential mechanisms responsible for shifting more of the population towards the ‘highs’ and away from the ‘lows’.
It has been estimated that around one in every eight individuals have a mental health condition and its attribution to total disease burden continues to rise on a global scale. 1 Mental health conditions concern a broad range of disorders, psychosocial disabilities and mental states of distress. Mental health, on the other hand, refers to the capacity of thought, emotion and behaviour that allows an individual to realise their own potential and positively contribute to their community. It exists on a continuum and, therefore, concerns the entire population. 1 Mental health approaches, which solely address those with mental health conditions (ie, high-risk strategies , eg, treatment), leave a larger percentage of the population susceptible to entering ill health without intervention. To address this concern, global mental health agendas have recognised the importance of population-based strategies , which aim to address the entire population distribution from the ‘lows’ (eg, poor mental health, low well-being) to the ‘highs’ (eg, good mental health, high well-being) and ultimately shift this distribution in a more positive direction.
Mindfulness-based programmes (MBPs) may serve as one potential pathway to help improve mental health and well-being across a wider distribution of the population. MBPs align with broader global mental health objectives by prioritising prevention to reduce the development of mental health conditions, building resilience in individuals and communities and recognising the importance of addressing mental health on a continuum (eg, ‘the lows’ to ‘the highs’). 2 MBPs were introduced in mainstream settings when mindfulness-based stress reduction (MBSR) was developed to help people with physical health conditions manage symptoms, such as chronic pain. 3 Mindfulness-based cognitive therapy (MBCT), an adaptation which includes psychoeducation elements from cognitive–behavioural therapy (CBT), was developed to help prevent depressive relapse. 4 MBSR/MBCT are traditionally formatted as 8-week programmes with weekly group-based sessions, led by a trained mindfulness instructor, and include daily self-led home-based mindfulness practice. These programmes have demonstrated effectiveness in those with recurrent depression (MBCT) and chronic pain (MBSR). 5 6 There is also a growing body of evidence that has demonstrated that mindfulness interventions can help a wider distribution of the population experience improvements in mental well-being. 7 However, more work is needed to further understand effectiveness, and the mechanisms of action of MBPs within this broader global mental health approach.
Theoretical frameworks 8 suggest that the model of change for MBPs is driven by the cultivation of mindfulness skills. Mindfulness, as a multidimensional construct, involves paying attention to the present moment experience with attitudes such as self-compassion, curiosity, kindness and care. It enables people to take a wider perspective (sometimes called decentering or meta-awareness) and see both internal and external stimuli as temporary events. The theory of MBCT and MBSR postulates that through an increase in mindfulness skills individuals with recurrent depression (MBCT) and chronic pain (MBSR), respectively, can attend to their internal experiences (eg, thoughts, feelings, bodily sensations) without judgement—allowing them to see these experiences more objectively (ie, decentering or meta-awareness) and as temporary which can help reduce maladaptive strategies (eg, rumination, reactivity) which exacerbate symptoms. Past reviews have examined mechanisms of change underlying MBPs in the context of more clinical or at-risk populations (eg, individuals with recurrent depression or psychological and physical conditions) and found consistent evidence for mindfulness skills in relation to clinical outcomes. 9 10 Additional reviews 11 12 have investigated mechanisms of MBPs across a wider distribution of the population (eg, clinical and non-clinical samples) and found consistent evidence for mindfulness skills and reactivity (eg, emotional, cognitive) in relation to mental health outcomes (eg, anxiety, depression, psychological distress, stress, negative affectivity). However, these reviews do not evaluate the quality of mediation testing and reporting in alignment with emerging mediation frameworks. 13 In light of the growing evidence base for MBPs and their efficacy across a wider distribution of the population and in relation to outcomes pertaining to the positive valence system (eg, mental well-being), 7 14–18 an updated review that comprehensively maps out the evidence for potential mechanisms that may be shared across and unique to different population samples and outcomes is needed. There is also an argument for investigating mechanisms in the context of independent components of MBPs (ie, individual mindfulness practices) to further understand active ingredients. Past reviews have demonstrated that stand-alone mindfulness practices produce change in outcomes relating to symptoms of depression, anxiety and stress with small-to-moderate effects. 19 20 Moreover, there is a growing body of evidence that has examined potential mechanisms of individual practices and practice modules. 21 22 However, this area of research is underdeveloped and warrants further investigation.
The primary aim of this review is to summarise the evidence for target mechanisms, underlying MBPs and mindfulness practices, which may be shared across or unique to different population samples and outcomes. This review aims to build on past reviews by (1) summarising the evidence for potential mechanisms underlying mindfulness practices as one key subcomponent of MBPs, (2) prioritising high quality and established formal analytical methods of mediation and (3) examining the evidence for mechanisms in relation to outcomes that map across the population. The hope is that the findings of this review will help inform future work and provide novel insight into the broader application of MBPs and the potential mechanisms responsible for shifting more of the population towards the ‘highs’ and away from the ‘lows’.
Study selection and analysis
A scoping review approach was implemented to allow for an iterative and exploratory focus to assess the broad research question, to map the available evidence for mediation in relation to emerging frameworks for testing and reporting the indirect effect (IE) and to map key conceptual terms for mechanisms and outcomes. Several frameworks 23 24 were used as guidance and the reporting follows the checklist for Preferred Reporting Items for Systematic reviews and Meta-Analyses (PRISMA) extension for Scoping Reviews. 25 This review was preregistered with Open Science Framework (OSF) on 16 July 2020 (DOI: 10.17605/OSF.IO/XJDSU ). A pre-print protocol was made available on 14 September 2020, outlining the research questions, methods and data synthesis strategy. The initial search was run on 13 December 2020, with additional searches run on 25 July 2022, 20 December 2022, 3 July 2023 and 7 March 2024. An addendum to the preregistration form was submitted to OSF on 3 November 2021 (DOI: 10.17605/OSF.IO/NY2AH ) and an updated protocol was uploaded to OSF on 1 April 2023 (see online supplemental 1 ). The timeline for these updates is summarised in online supplemental 2 .
Supplemental material
Five electronic databases (PubMed, PsycINFO, Embase, Scopus and Cochrane Central) were searched for relevant records up until 7 March 2024. The search string was developed by first breaking down the research question (‘Through which mediators and mechanisms do MBPs and mindfulness practices produce change in the adult population’) by population (ie, relevant characteristics of participant sample; eg, entire adult population distribution), context (ie, the setting or discipline; eg, mindfulness) and concept terms (ie, research designs, frameworks, theories; eg, mediation). 26 Synonyms and related terms were then generated. The search string was refined by running a mock search in PubMed and consulting experts in the field, such as mindfulness teachers and a research librarian. Key terms in the search string included ‘ mechanism* ’, ‘ mediat* ’, ‘ mindfulness ’ and ‘ mindfulness-based ’. Specific practice terms included: ‘ body scan ’, ‘ mindful movement ’ and ‘ yoga ’. The search string used for each database is provided in online supplemental 3 . Other sources (eg, Web of Science conference proceedings, PsycEXTRA and connectedpapers.com ) were searched for grey literature up until 7 March 2024. The snowballing method was also implemented to identify additional records from the reference lists of the included papers ( online supplemental 4 ) and key systematic reviews. 9–12
The inclusion and exclusion criteria reflect the population, context and concept. 26 In terms of the population, we included any study concerning an adult sample (aged 18 and above) to reflect the entire adult population distribution (from mental health conditions to well-being). For the context, eligible studies evaluated an MBP defined by Crane et al 27 or a formal mindfulness practice that, in the context of an MBP, is home-based, scheduled with audio guidance and practised at least three times per week. This criterion was developed from a content analysis of four key MBP curricula ( online supplemental 5 ). For the concept, we included studies that evaluated the IEs of an MBP or formal mindfulness practice (independent variable) on mental health and well-being outcomes (dependent variable) through psychological mediators (mechanism) (see online supplemental 6 for a visual depiction of the IE). The evaluation of the IE followed recommendations outlined by Zhao et al , 13 which establish five categories of mediation (complementary, competitive, indirect-only, direct-only effect or no effect). For more information regarding these criteria, see online supplemental 7 . Only studies that implemented a randomised controlled trial (RCT) design with a control group that had active ingredients were included to capture the highest-quality evidence. The comparator was loosely defined as including ‘active’ ingredients if it included more than the mere passage of time (eg, wait list control group). For more details on the inclusion and exclusion criteria, see online supplemental 8 .
Each record was screened by at least two independent authors for titles and abstracts (SM, MK, YS, JM-M and LR) and full texts (SM, MK, YS and AL-M). Following Siddaway et al , data extraction was piloted and completed by one author (SM), with 15% of the output randomly cross-checked by a second author (YS, MK and JM-M). 28 Any disagreements were resolved by a third author. For records that did not have the full text, corresponding authors were contacted. The following information was piloted and then extracted for charting: (1) author(s) and year of publication, (2) sample characteristics, (3) type of mental health strategy (ie, treatment, prevention or promotion), (4) type of MBP or formal mindfulness practice, (5) dosage, (6) delivery mode (eg, online or face to face), (7) type of active comparator(s), (8) reported mechanisms, (9) reported outcomes, (10) data analytic approach for testing mediation and (11) key findings. A narrative synthesis was conducted whereby evidence gap maps help elucidate how and why MBPs and practices may work in relation to different outcomes, mental health strategies (eg, population samples) and active comparators. Further details can be found in online supplemental materials on the evidence gap map methodology ( online supplemental 9 ) and conceptual mapping of key terms (ie, outcomes, mechanisms, mental health strategies) ( online supplemental 10 and 11 ). Quality assessments for testing and reporting IEs are provided in online supplemental 12 .
The PRISMA flow chart shows 43 392 records identified across the 5 electronic databases. Before the records were screened for eligibility, 25 394 records were removed as duplicates. Six additional records were identified from either snowballing included papers or past reviews, searching grey literature sources or corresponding authors sharing additional papers. For title and abstract screening, 18 004 records were reviewed by two independent referees, with 16 715 excluded, leaving 1289 records for full-text screening. A total of 30 records were included in the review with 3 records sharing the same sample with another record, leaving 27 eligible studies ( figure 1 ).
- Download figure
- Open in new tab
- Download powerpoint
PRISMA flow chart. The PRISMA flow diagram of the records initially identified from the database search before and after deduplication is illustrated. The chart also outlines the records screened at the title and abstract stage as well as the full-text stage, including the number of records removed after full-text screening with the reasons specified. The additional records found outside of the search (n=6) were identified from either forward or backward citation searching of the included records and relevant reviews, from grey literature sources or from corresponding authors who shared additional records. Out of the 30 records included, 3 records shared the same sample with another record and therefore there were 27 studies included in total. Note that both English and Spanish publications were considered, given the primary languages of the research team. However, all included studies were published in English and, therefore, no Spanish publications met the inclusion criteria. RCT, randomised controlled trial.
General findings, regarding the scope of the evidence, indicate four eligible studies that evaluated a formal mindfulness practice (1 body scan, 1 mindfulness of emotions, 1 breath and 1 body scan and breath) and 23 studies that evaluated an MBP. In terms of outcomes, 11 studies examined outcomes related to mental health conditions, 16 studies examined outcomes related to mental languishing and 9 studies examined outcomes related to well-being. 11 studies examined an MBP or practice as a treatment strategy (10=MBP, 1=practice), 16 as a prevention strategy (14=MBP, 2=practice) and 9 as a promotion strategy only (8=MBP, 1=practice). For the 16 studies that implemented a preventive strategy, 4 examined universal prevention, none examined indicated prevention only, 6 explored selective prevention only and 6 studies examined elements of both indicated and selective prevention. The majority of the included studies compared an MBP to treatment as usual (TAU) (n=13). Five studies compared an MBP or practice to stress management or relaxation (SM/R), three studies compared an MBP to CBT or cognitive interventions (CBT/CI), four studies compared an MBP or practice to attentional control (AC) and three studies compared an MBP to self-help mindfulness (SH-M).
Evidence gap maps were generated to summarise the number of significant, non-significant and opposite direction IEs by outcome (mental health conditions, mental languishing, well-being), type of mental health strategy (treatment, prevention, promotion) and type of active comparator (TAU, SM/R, CBT/CI, AC and SH-M). The largest proportion of significant IEs to non-significant or opposite direction IEs was demonstrated for mindfulness skills, decentering and attitudes of mindfulness in relation to mental languishing outcomes; with mixed findings in the context of outcomes pertaining to mental health conditions; and preliminary evidence in the context of well-being ( figure 2 ). With the evidence by mental health strategy, the largest proportion of significant IEs was found for mindfulness skills, decentering, and attitudes of mindfulness in the context of prevention; with mixed evidence in the context of treatment; and preliminary evidence in the context of promotion ( figure 3 ). The most commonly used active comparator was TAU, whereby there were significant IEs for mindfulness skills, decentering and attitudes of mindfulness in the context of the mindfulness condition but not TAU ( figure 4 ). Online supplemental 13 provides additional information regarding key findings.
Following the Zhao et al ’s 13 framework, all included studies (n=27) reported the significance of the IE. However, out of these studies, only 13 studies fully reported the significance of the direct effect (DE), after controlling for the IE, and reported the sign of the IE and DE to accurately classify the type of mediation. 13 Out of these 13 studies, 5 reported complementary mediation, none reported competitive mediation, 10 reported indirect-only mediation, 3 reported direct-only effects and 1 reported no effects (non-mediation) ( figure 5 ). In terms of overall quality assessment for mediation, following the Zhao et al ’s 13 and Kazdin’s 29 (2007) frameworks, the overall rating (score range 0–30) was high for reporting the IE (score of 22), high for plausibility or coherence (score of 25.5), intermediate (score of 12.5) for timeline and low (score of 6) for gradient ( online supplemental 12 ).
Flow chart of included studies by mediation type and reporting requirements. The flow chart of the included studies is illustrated based on meeting key steps for reporting and categorising mediation: (1) the significance of path a×b, (2) the significance of path c and (3) the sign of paths a×b and c. All included studies reported the significance of the a×b path as this was a key inclusion criteria. From there, the number of included studies (n) that reported the significance of path c was recorded, followed by the number of included studies that reported the sign of the a×b and c paths. At the bottom of the flow chart, we have the total number of studies for each category of mediation. Note that for studies that partially demonstrated the significance of path c and partially demonstrated the signs of a×b and c, this meant that these papers met the criteria for some findings but not for all. Only 13 studies fully met these criteria, and therefore mediation categories were determined. This figure reflects key findings in online supplemental 13 and was adapted from Zhao et al . 13 .
Conclusions and clinical implications
The aim of this scoping review was to summarise the literature on potential target mechanisms underlying MBPs and formal mindfulness practices that may drive change in mental health and well-being across the population. The results of this review demonstrated limited high-quality evidence in the context of individual formal mindfulness practices, as one key component of MBPs. The current review identified additional knowledge gaps in terms of the evidence for potential mechanisms by outcome ( figure 2 ), mental health strategy ( figure 3 ) and active comparator ( figure 4 ).
Looking at the evidence by outcomes, the results provided preliminary support for mindfulness skills, decentering, and attitudes of mindfulness as potential target mechanisms ( figure 2 ). The largest number of significant IEs was found in the context of mental languishing outcomes (ie, mental health symptoms), which builds on the results of past reviews. 9–12 In the context of outcomes relating to mental health conditions, the evidence was mixed. Significant IEs for mindfulness skills, decentering and attitudes of mindfulness were also reported in relation to well-being outcomes; however, future research will need to replicate these findings. The evidence by mental health strategy demonstrated consistent evidence for mindfulness skills, decentering and attitudes of mindfulness in the context of prevention, mixed evidence in the context of treatment and preliminary evidence in the context of promotion ( figure 3 ). In terms of the evidence by active comparator ( figure 4 ), preliminary evidence for changes in mindfulness skills, decentering and attitudes of mindfulness was found when the mindfulness condition was compared with TAU. However, TAU ranged across studies (eg, continuation of medication and/or psychotherapy and/or regular doctor visits) with differing levels of adherence. For many studies, TAU was also included within the mindfulness condition, which made it difficult to understand mindfulness-specific effects. Overall, very few studies replicated findings whereby the same putative mechanism was tested in the context of one active comparator category. The largest number of opposite direction IEs were found in the context of comparing an MBP to cognitive–behavioural therapy or cognitive interventions (CBT/CI), which may suggest that CBT/CI may demonstrate superiority in terms of activating certain putative mechanisms (eg, empathy, reappraisal self-efficacy, safety behaviours) in relation to specific outcomes (eg, social anxiety). This mirrors what past reviews have found in terms of outcomes, with limited evidence for MBP superiority when compared with current gold-standard treatments (eg, CBT). 5 7 Across all evidence gap maps ( figures 2–4 ), one key hypothesis generated was that changes in mindfulness skills, decentering and attitudes of mindfulness may be responsible for shifting the entire population more towards improved outcome ( figure 6 ). However, this hypothesis will need to be tested in future work across a wider range of population samples, outcomes and active comparators.
Conceptual framework of target mechanisms. A conceptual framework of target mechanisms that may be responsible for shifting change across the entire population distribution (eg, from mental health conditions to mental languishing to well-being) is illustrated. In this figure, the ‘red’ region on the left-hand side represents outcomes pertaining to mental health conditions (eg, anxious, depressive and adjustment disorders; psychosomatic disorders; behavioural disorders, etc). The ‘orange-to-yellow’ region represents outcomes pertaining to mental languishing (eg, mental health symptoms, stress, burnout, etc). The ‘blue’ region represents outcomes pertaining to well-being (eg, mental well-being, quality of life, positive affect, positive states of mind, etc). The grey arrow represents the proposed mechanisms of change (ie, mindfulness skills, decentering and attitudes of mindfulness). This conceptual framework was informed by the results of the scoping review and summarises key hypotheses regarding the ‘how’ and ‘why’ mindfulness-based programmes and practices work across the whole population distribution. However, future research will need to further interrogate this conceptual framework in different contexts (eg, mental health strategies, ie, promotion), outcomes (eg, well-being) and active comparators (eg, self-help mindfulness, attentional control). Illustration by Delphine Perrot.
In addition to mapping out the evidence for mechanisms underlying MBPs and mindfulness practices, another aim of this review was to interrogate the quality of the evidence with regard to meeting key testing and reporting requirements for mediation. All eligible studies tested the IE and implemented an RCT design with a comparator that had some active components (ie, more than the mere passage of time) as this was a key inclusion criteria. Some excluded studies explored the IE but only within the mindfulness condition 30–32 and others compared the MBP or mindfulness practice to a passive control group (ie, wait list). 14 33–36 Additional studies were excluded on the basis of using alternative analytic methods (eg, simple correlations) that could not effectively calculate the IEs or its significance and, in consequence, determine the type of mediation. 37–40 Out of the included studies, 13 fully met all reporting requirements ( figure 5 ). Out of these thirteen studies, the majority found indirect-only mediation and demonstrated consistent support for mindfulness skills, decentering and attitudes of mindfulness (ie, self-compassion) as putative mechanisms across a range of outcomes (eg, stress, depression, anxiety, burnout, well-being) and samples (eg, secondary school teachers, partial remitters for MDD) ( online supplemental 13 ).
Additional criteria for establishing and reporting a mechanism of change were also evaluated. 29 The included records scored a high quality score for the reporting of the IE. However, the assumption is that this quality score would be much lower if we looked across all mechanism studies conducted in the mindfulness field, considering the inclusion and exclusion criteria of this review. For the other criteria explored ( online supplemental 12 ), the overall quality score was high for the ‘plausibility and coherence’ criterion, intermediate for the ‘timeline’ criterion and low for the ‘gradient’ criterion. For the timeline criterion, only 12 studies met this criterion which suggests that future work should incorporate research designs or analytic strategies that minimise measurement overlap for proposed mediators and outcomes. This is an area of research that is advancing and there are statistical analyses that can help disentangle this relationship (eg, cross-lagged panel models 41 ) even when there is overlap with measurement timepoints. Overall, this criterion helps with increasing confidence in establishing temporality, which indicates that change in the proposed mediator occurs before change in outcome. For the gradient criterion, only five studies met this criterion which indicates that more work is required to establish that different doses (eg, longer home-based practices or number of sessions) are related to changes in the proposed mediator and outcome to help increase confidence that changes in the mediator are a result of mindfulness-specific components. The ‘high’ score for plausibility and coherence suggests that the selected studies were mostly driven by a proposed theoretical framework, which is one strength of the included studies (n=21) which met this criterion ( online supplemental 12 ).
One strength of this scoping review includes the breadth of the topic and search strategy to provide a comprehensive summary of putative psychological mechanisms and to identify knowledge gaps. Another strength of the review is the adherence to emerging mediation frameworks, 13 29 which meant that the highest-quality evidence for mediation was identified. In terms of limitations, the evidence gap maps captured multiple findings from the same study and many studies presented findings across multiple categories (eg, mechanisms, outcomes, mental health strategy) which could inflate the evidence; however, the number of studies per category are specified to help manage this and the hope is that this presentation will help clearly map out what has and has not been explored. Although many researchers were involved in the conceptual mapping of measurements onto categories, future work is needed to reach a consensus on how mindfulness and overlapping processes (eg, attention and awareness, attitudes of mindfulness, and decentering) are operationally defined. Additionally, the inclusion of RCTs with ‘active comparators’ may have skewed the results to more clinical samples and ‘high-risk’ approaches (eg, treatment and indicated prevention) whereby active comparators are more established compared with alternative strategies (eg, universal prevention, promotion) which address a wider distribution of the population (eg, general population samples). Moreover, the majority of studies included TAU control groups which can be flawed with issues such as resentful demoralisation whereby participants in the TAU arm, aware of the treatment arm, may feel disheartened and report less desirable outcomes. To address these limitations, future work will need to explore active comparators in a range of contexts (eg, mental health strategies, outcomes, population samples) and consider how to reduce potential bias. Qualitative studies and alternative research designs (eg, quasi-experimental) were not included in the current review to isolate the highest quality evidence for mediation; however, these methods may be useful in identifying additional mechanisms of change. Therefore, qualitative methods and alternative designs may serve as starting points and future work can then test hypothesised mechanisms with an RCT design and by following key testing and reporting requirements (see online supplemental 14 ).
Focusing solely on those with mental health conditions or who are languishing leaves the majority of the population susceptible to entering ill health without any intervention. 42 Therefore, there is a global health argument for going beyond treatment and looking at mental health across a wider distribution of the population. Adaptations of MBCT/MBSR for the general population have been developed, and there is preliminary evidence that supports their efficacy. 14 16 43 However, more work is required to extrapolate the putative mechanisms that may be shared across or unique to these adaptations and their parent programmes to help optimise their effectiveness and increase scalability. In this review, the results provided preliminary support for mindfulness skills, decentering and attitudes of mindfulness as key intervention targets for addressing mental health of whole populations ( figure 6 ). However, the field moving forward requires more RCTs, with active comparators that include more than the mere passage of time and TAU, to further understand the unique benefits of MBPs and their potential utility of applying them more broadly. In parallel, proper testing and reporting of mediation 13 29 44 is needed to help refine the proposed theoretical framework to then help support thoughtful adaptations of MBPs.
Ethics statements
Patient consent for publication.
Not applicable.
Ethics approval
Acknowledgments.
The authors greatly appreciate all the time and effort Mrs Karine Barker took to help test the sensitivity and feasibility of the search strategy. She is a research librarian at the Radcliffe Science Library, Bodleian Libraries, Oxford, UK. A special thanks to Ruth Baer and Andreas Voldstad for their feedback in the final stages.
- Lund C , et al
- Kabat-Zinn J
- Williams M ,
- Creswell JD
- Warren FC ,
- Taylor RS , et al
- Galante J ,
- Friedrich C ,
- Dawson AF , et al
- Feldman C ,
- van der Velden AM ,
- Wattar U , et al
- Alsubaie M ,
- Dunn B , et al
- Maddock A ,
- Strauss C ,
- Bond R , et al
- Montero-Marin J , et al
- van Agteren J ,
- Iasiello M ,
- Lo L , et al
- Montero-Marin J ,
- Crane C , et al
- Maloney S ,
- Perleth S ,
- Heidenreich T , et al
- Schumer MC ,
- Lindsay EK ,
- Britton WB ,
- Loucks EB , et al
- Sauer-Zavala SE ,
- Eisenlohr-Moul TA , et al
- Joanna Briggs Institute
- Tricco AC ,
- Zarin W , et al
- Peters MDJ ,
- Godfrey CM ,
- Khalil H , et al
- Feldman C , et al
- Siddaway AP ,
- Garcia-Toro M ,
- Aguilar-Latorre A ,
- Garcia A , et al
- Goldstein E ,
- Topitzes J ,
- Brown RL , et al
- Liu X , et al
- Aizik-Reebs A ,
- Yuval K , et al
- Szepsenwol O , et al
- Nyklíček I ,
- Dijksman SC ,
- Lenders PJ , et al
- Sbarra DA , et al
- Bieling PJ ,
- Hawley LL ,
- Bloch RT , et al
- Bonura KB ,
- Tenenbaum G
- Colgan DD ,
- Christopher M ,
- Michael P , et al
- Hamaker EL ,
- Kuiper RM ,
- Grasman RPPP
- Loucks EB ,
- Gutman R , et al
- Cashin AG ,
- Lamb SE , et al
JM-M and WK are joint senior authors.
X @shann_maloney
Contributors SM, JM-M and WK conceived the work, its methodology and interpretation. SM, JM-M, YS, MK, LR and AL-M all contributed to the initial search and with refining the scope of the review. SM wrote the first draft of this manuscript, and all authors read and approved the final version. SM was responsible for the overall content as the guarantor.
Funding This research was funded by the Wellcome Trust (WT104908/Z/14/Z and WT107496/Z/15/Z) and by the National Institute for Health and Care Research (NIHR) Oxford Health Biomedical Research Centre (NIHR203316). JM-M has a ‘Miguel Servet’ research contract from the ISCIII (CP21/00080) and is grateful to the CIBER of Epidemiology and Public Health (CIBERESP CB22/02/00052; ISCIII) for its support. MK was supported by the Research Foundation—Flanders (FWO-Vlaanderen) under a PhD fellowship (11I1622N).
Disclaimer The views expressed are those of the author(s) and not necessarily those of the Wellcome Trust, NIHR or the Department of Health and Social Care. For the purpose of open access, the author has applied a CC BY public copyright licence to any author-accepted manuscript version arising from this submission.
Competing interests None declared.
Provenance and peer review Not commissioned; externally peer reviewed.
Supplemental material This content has been supplied by the author(s). It has not been vetted by BMJ Publishing Group Limited (BMJ) and may not have been peer-reviewed. Any opinions or recommendations discussed are solely those of the author(s) and are not endorsed by BMJ. BMJ disclaims all liability and responsibility arising from any reliance placed on the content. Where the content includes any translated material, BMJ does not warrant the accuracy and reliability of the translations (including but not limited to local regulations, clinical guidelines, terminology, drug names and drug dosages), and is not responsible for any error and/or omissions arising from translation and adaptation or otherwise.
Read the full text or download the PDF:

IMAGES
COMMENTS
The first is the introduction which provides background and the general problem statement of this research. In the second part, this paper discusses considerations on use of Big Data and Big Data Analytics in Healthcare, and then, in the third part, it moves on to challenges and potential benefits of using Big Data Analytics in healthcare.
Introduction. The purpose of data analytics in healthcare is to find new insights in data, at least partially automate tasks such as diagnosing, and to facilitate clinical decision-making [1, 2].Higher hardware cost-efficiency and the popularization and advancement of data analysis techniques have led to data analytics gaining increasing scholarly and practical footing in the healthcare sector ...
The process of data cleansing, data mining, data preparation, and data analysis used in healthcare applications is reviewed and discussed in the article. The article provides an insight into the status and prospects of big data analytics in healthcare, highlights the advantages, describes the frameworks and techniques used, briefs about the ...
Malik, Abdallah, and Ala'raj ( 2018) reviewed the use of BDA in supply chain management in healthcare. Saheb and Izadi ( 2019) reviewed the use of big data sourced from Internet-of-Things devices in the healthcare industry. Such review studies are not designed to provide a comprehensive review of the literature on BDA in healthcare.
The remaining research paper of the paper is organized as follows. ... Ramesh, T. & Santhi, V. Exploring big data analytics in health care. Int. J. Intell. Netw. 1, 135-140 (2020).
In this paper, we aim to provide a comprehensive literature review on the application of big data analytics in healthcare, focusing on its ecosystem, applications, and data sources. ... Furthermore, this study identifies and discusses key open research challenges in the field of big data analytics in healthcare, aiming to push the boundaries ...
In their review paper on data mining of big data in health analytics, Herland et al. ... Several research avenues for health data analytics are, therefore, defined in this section in an attempt to provide researchers and practitioners with future directions in this domain. ... N. Kulennavar, A survey on big data analytics in health care. Int. J ...
Big data is transforming and will transform the healthcare organizations in the near future [1, 2].Scientific literature in the managerial context applied to healthcare organizations, consider the Big Data Analytics (BDA) a fundamental tool, so much so that it has attracted the attention of the scientific community and stakeholders [].However, a premise should be made: data by themselves ...
In the era of big data, standard analysis tools may be inadequate for making inference and there is a growing need for more efficient and innovative ways to collect, process, analyze and interpret the massive and complex data. We provide an overview of challenges in big data problems and describe how innovative analytical methods, machine learning tools and metaheuristics can tackle general ...
4 ML and Analytics in Healthcare Systems. structu re alignments, signal dete ction algorithms, and lung texture classi cation [5]. The architect ural fr amework of big data in healthcare is ...
Data science is an interdisciplinary field that extracts knowledge and insights from many structural and unstructured data, using scientific methods, data mining techniques, machine-learning algorithms, and big data. The healthcare industry generates large datasets of useful information on patient demography, treatment plans, results of medical examinations, insurance, etc. The data collected ...
The field of healthcare has seen a rapid increase in the applications of data analytics during the last decades. By utilizing different data analytic solutions, healthcare areas such as medical image analysis, disease recognition, outbreak monitoring, and clinical decision support have been automated to various degrees. Consequently, the intersection of healthcare and data analytics has ...
Management and analysis of big data. Big data is the huge amounts of a variety of data generated at a rapid rate. The data gathered from various sources is mostly required for optimizing consumer services rather than consumer consumption. This is also true for big data from the biomedical research and healthcare.
The application of an artificial intelligence system to medical research has the potential to move toward highly advanced e-Health. This analysis aims to explore the main areas of application of big data in healthcare, as well as the restructuring of the technological infrastructure and the integration of traditional data analytical tools and ...
Big Data analytics can improve patient outcomes, advance and personalize care, improve provider relation ships with. patients, and reduce medical sp ending. This paper introduces he althcare data ...
Malik, Abdallah, and Ala'raj (2018) reviewed the use of BDA in supply chain management in healthcare. Saheb and Izadi (2019) reviewed the use of big data sourced from Internet-of-Things devices in the healthcare industry. Such review studies are not designed to provide a comprehensive review of the literature on BDA in healthcare.
This paper is organized as follows. In Section 2, complete literature review about healthcare various issues have been addressed using data mining techniques as well as big data analytics. In Section 3 which talks about data mining in health care and in Section 4, modelling of big data in health care have been addressed. 2. Literature review
The healthcare industry is undergoing a major transformation driven by the emergence of big data and advanced analytics. This paper examines how data analytics is revolutionizing healthcare by ...
The introduction of Big Data Analytics (BDA) in healthcare will allow to use new technologies both in treatment of patients and health management. The paper aims at analyzing the possibilities of using Big Data Analytics in healthcare. The research is based on a critical analysis of the literature, as well as the presentation of selected results of direct research on the use of Big Data ...
The pace of both digital innovation and technology disruption is refining the healthcare industry at an exponential rate. The large volume of healthcare data continues to mount every second, making it harder and very difficult to find any form of useful information. Recently, big data is shifting the traditional way of data delivery into valuable insights using big data analytics method. Big ...
Motivation and Scope. There is a large body of recently published review/conceptual studies on healthcare and data mining. We outline the characteristics of these studies—e.g., scope/healthcare sub-area, timeframe, and number of papers reviewed—in Table 1.For example, one study reviewed awareness effect in type 2 diabetes published between 2001 and 2005, identifying 18 papers [].
The introduction of Big Data Analytics (BDA) in healthcare will allow to use new technologies both in treatment of patients and health management. The paper aims at analyzing the possibilities of ...
Health regulations emphasize regular screenings for individuals with diabetes risk factors, 9 highlighting the importance of timely identification and intervention. Preventive measures are crucial alongside diabetes care. 10 Early diagnosis and lifestyle modifications, such as healthy eating and exercise, can reduce the progression from impaired glucose tolerance to prediabetes. 11 Technology ...
This research paper focuses on the revolutionary effect of instant in-person health analytics by IoT -connected wearable medical devices on health results. By the exploration of currently available literature, technical systems and showing case studies, we provided strong evidence that real time monitoring improved patient outcome and made healthcare delivery better. The research unveils the ...
Question Mindfulness-based programmes (MBPs) and practices have demonstrated effects in mental health and well-being, yet questions regarding the target mechanisms that drive change across the population remain unresolved. Study selection and analysis Five databases were searched for randomised controlled trials that evaluate the indirect effects (IEs) of an MBP or mindfulness practice in ...
The main aim of this paper is to provide a deep analysis on the research field of healthcare data analytics., as well as highlighting some of guidelines and gaps in previous studies. This study ...