Advanced Modern Control System Theory and Design by Stanley M. Shinners
Get full access to Advanced Modern Control System Theory and Design and 60K+ other titles, with a free 10-day trial of O'Reilly.
There are also live events, courses curated by job role, and more.

CONTROL-SYSTEM DESIGN EXAMPLES: COMPLETE CASE STUDIES
7.1. introduction.
In the preceding chapters of this book, we have analyzed and designed control systems from specific viewpoints. For example, the Nyquist and Bode diagrams and the root-locus method were applied to linear control systems in Chapter 1 , and Chapters 2 and 3 , and extended to digital control systems in Chapter 4 . The describing function, phase-plane, circle criterion, Liapunov's and Popov's methods were applied to the analysis and design of nonlinear control systems in Chapter 5 . How do we take a global viewpoint of a control-system design problem and look at it from both linear and nonlinear viewpoints? We must also consider reliability, cost size, weight, and power consumption. We must design a working control system that meets all the specifications, that can be sold at a profit, that can be built on schedule, and that satisfies the customer's requirements.
In this chapter on complete case studies, we will employ the methods of the preceding chapters to design the following:
- Design for the positioning system of a tracking radar which illustrates both linear and nonlinear design considerations jointly.
- Design of the angular control system for a robot's joint.
- State-variable design for the controller and full-order estimator for a space satellite.
- Digital control system design for a microcomputer-controlled temperature control system.
- Robust control system design for controlling the flaps of a hydrofoil.
Get Advanced Modern Control System Theory and Design now with the O’Reilly learning platform.
O’Reilly members experience books, live events, courses curated by job role, and more from O’Reilly and nearly 200 top publishers.
Don’t leave empty-handed
Get Mark Richards’s Software Architecture Patterns ebook to better understand how to design components—and how they should interact.
It’s yours, free.
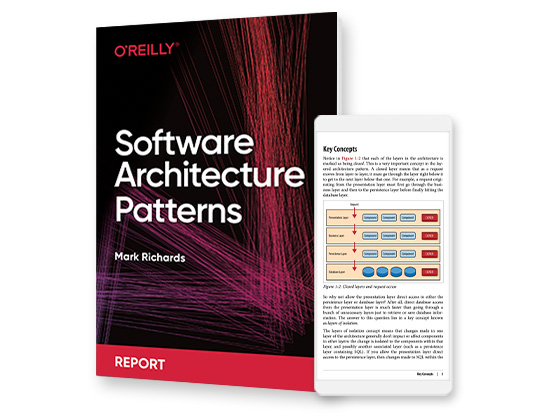
Check it out now on O’Reilly
Dive in for free with a 10-day trial of the O’Reilly learning platform—then explore all the other resources our members count on to build skills and solve problems every day.
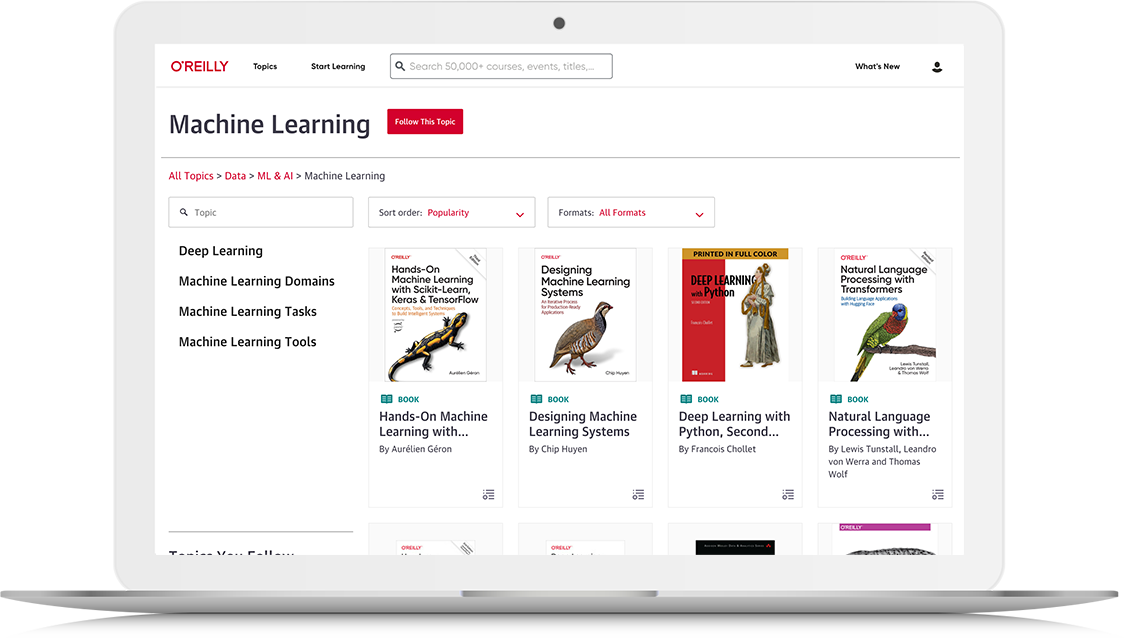
IEEE Account
- Change Username/Password
- Update Address
Purchase Details
- Payment Options
- Order History
- View Purchased Documents
Profile Information
- Communications Preferences
- Profession and Education
- Technical Interests
- US & Canada: +1 800 678 4333
- Worldwide: +1 732 981 0060
- Contact & Support
- About IEEE Xplore
- Accessibility
- Terms of Use
- Nondiscrimination Policy
- Privacy & Opting Out of Cookies
A not-for-profit organization, IEEE is the world's largest technical professional organization dedicated to advancing technology for the benefit of humanity. © Copyright 2024 IEEE - All rights reserved. Use of this web site signifies your agreement to the terms and conditions.
- Awards/Honors
- Download Media Kit
- Tuck MBA Program
- Executive Education
- Course Materials
- Course Syllabus
- Photo Gallery
- Video Gallery
Tel: 603-289-0007 [email protected]
VG personally handles all inquiries. The best way to reach him is his email address. Only as a backup, use VG’s cell phone: 603-289-0007.
Management Control Systems: Mini Cases
For his course on Implementing Strategy: Management Control Systems, Vijay Govindarajan uses mini-cases to illustrate how the design and implementation of ongoing management systems are used to plan and control a company's performance.
3M Corporation
Vijay Govindarajan; Julie Lang Length: 4 pages Publication date: 2002 Case No. 2-0002
3M's strategy was rooted in innovation. 3M's 30 Percent Rule, where 30 percent of revenues must come from products introduced in the last four years, clarifies and drives its innovation mentality. Selected policies and philosophies helped to institutionalize a corporate culture of entrepreneurship and innovation.
Dell Computer Corporation
Vijay Govindarajan; Julie Lang Length: 4 pages Publication date: 2002 Case No. 2-0014
The world's largest direct-selling computer company grew from its philosophy that customers know what they want and Dell can deliver it through custom assembly of outsourced components. Through a combination of financial and non-financial measures, Dell turned itself from a product business into a service industry.
Southwest Airlines
Vijay Govindarajan; Julie Lang Length: 4 pages Publication date: 2002 Case No. 2-0012
Southwest used its short-haul and point-to-point strategy to achieve the lowest operating cost structure in the domestic airline industry. Flexible contracts and a rigorous peer recruiting process aligned its 35,000 employees with this strategy.
Wal-Mart Stores, Inc.
Vijay Govindarajan; Julie Lang Length: 4 pages Publication date: 2002 Case No. 2-0013
Sam Walton, founder of Wal-Mart in 1962, had the vision for his store to sell low cost, branded products. By setting up its own distribution system and truck fleet, and evaluating retail stores as separate investment centers, Wal-Mart's control systems helped to build and entrench its competitive advantage.
Management Control Systems: Teaching Cases
In his course on Implementing Strategy: Management Control Systems, Vijay Govindarajan uses his textbook entitled Management Control Systems, (with Robert N. Anthony), Eleventh edition, 2003, in addition to the following cases.
Analog Devices (A)
The first Analog Devices case can be found in VG's book, Management Control Systems
Analog Devices, a leading semiconductor manufacturer designed performance measurement systems that provided far more than just a financial view. As their system evolved, it incorporated more measures designed to reflect growth, rather than just operational efficiency. A similar approach later was popularized as the "Balanced Scorecard."
Analog Devices (B)
Chris Trimble; Vijay Govindarajan; Jesse Johnson Length: 9 pages Publication date: 2001 Case No. 2-0003
In 2000, ADI posted an unprecedented 78 percent growth rate. Their participation was growing in new consumer markets (electronics and communications) with shorter life cycles and high volatility. ADI had to rethink their scorecard for more dynamic environments.
Crown Point Cabinetry
David VanderSchee; Vijay Govindarajan; Julie Lang Length: 8 pages Publication date: 2001 Case No. 2-0010
In 1993, Brian Stowell, CEO of a family-owned cabinet manufacturing business, created a vision for his 85 employees that focused on high quality products with less rework and wasted material. Eliminating production line managers and adopting a team-based management approach was a risky proposition, but one that paid off in increased sales and margins.
Nucor Corporation (A)
Vijay Govindarajan Length: 15 pages Publication date: 1998 Case No. 2-0015
Under the leadership of CEO Ken Iverson, Nucor thrived. Nucor's structure was decentralized, with only four management layers. Only 22 employees worked at the corporate headquarters; plants were located in rural areas across the US and the general manager of each plant was granted considerable autonomy and encouraged to take reasonable risks. Employee relations stressed pay for productivity and took an egalitarian approach toward employee benefits. Under Iverson's leadership, Nucor pioneered the mini-mill concept, built new plants from scratch, promoted from within, and remained a domestic company.
Nucor Corporation (B)
Vijay Govindarajan Length: 3 pages Publication date: 1999 Case No. 2-0016
In January, 1999, Ken Iverson, the thirty-year leader of Nucor Corporation, was forced into retirement. Five months later, his successor, John Correnti, was asked to leave. The board of directors wanted fundamental shifts in Nucor's strategy and organization that Iverson and Correnti resisted. The board's considerations included pursuing acquisitions, expanding into global markets and adding new organizational layers.
The Trustees of Dartmouth College hold the copyright to all the cases listed above. Please download a single copy for evaluation. For permission to reproduce multiple copies; i.e. for classroom use, please contact Annette Lepine n by e-mail at [email protected] . Ms. Lepine can also provide teaching notes for many of these cases, including an instructor's guide for teaching with the book Ten Rules for Strategic Innovators-from Idea to Execution (2005, Harvard Business School Press).
© The Trustees of Dartmouth College. All rights reserved.
Case Studies

Helping paper facility start production
A system integrator helped a Texas-based facility that developed paper bowls and drink carriers for the fast-food restaurant industry to launch their facility after the COVID-19 pandemic forced changes.
Connected distribution center takes control of intralogistics challenges
Cover Story: A connected distribution center boosts device connectivity and networking capabilities with PC control and EtherCAT while cutting controller hardware costs by 50%.
Circuit breaker promotes automotive-plant energy efficiency
Communications and power metering capabilities built in
PC-based control boosts sterile sampling bag production
Labplas redesigns custom production machines with EtherCAT, robotics and AI for quality control, ensuring ROI of less than one year on all upgrades, for 15 to 35% machine productivity increases.
Case study: Commissioning a solar power plant
Commissioning an integrated security system for solar power plant in Saudi Arabia was successful despite the challenges posed by the COVID-19 pandemic.
How robots package eggs
Robot palletizer safely packs fresh eggs, increases throughput and reduces costs
Automation helps packaging verification
Machine vision and code readers verify packing label quality. As with many machine vision applications proper set-up and fixturing are needed in this packaging automation system integration project.
Case study: Water filtration plant automation
The team had a large-scale automation project goal of integrating 121 water filtration plants
Privacy Overview

Talk to an expert today: 01384 484805
- About Banelec
- Schneider Systems Integrator
- Company Video
- Certificates & Downloads
- Enclosure Options
- Industry 4.0
- Complete Solutions
- Electrical Controls
- Conveyor Controls
- Electrical Control Systems Norway
- In House Services
- Install Relocate & Integrate
- PLC & Robotics Engineers
- Gigafactories
- Case Studies
- Testimonials
Control System Case Studies
From automotive and food processing to oil rigs and cranes, we’ve installed best-in-class electrical control systems into a wide range of industry sectors. Check out our case studies to find out more about some of the recent projects we’ve worked on.
J Barnsley Cranes
Over the years we have worked very closely with J Barnsley Cranes, designing and manufacturing many hazardous area systems for cranes across the globe. With them we have had the opportunity to expand our expertise in the field of hazardous area crane controls and develop revolutionary solutions which we implement frequently into our designs.
Aibel – Innovative double redundancy fail-safe system for oil rig
Tasked with designing a revolutionary control system for a crane on board the Johan Sverdrup platform being built by Aibel, Norway, Banelec’s vast experience in hazardous area systems was called to the test.
Eilbeck Cranes – Fast turnaround on size restricted systems
Banelec have provided solutions for multiple projects for Eilbeck Cranes, this example being the most prominent for showcasing what we are capable of.
GKN – Increased productivity in automotive facility
With more and more demand within the automotive industry – GKN – a key supplier to Jaguar Land Rover, have now expanded their production facility to keep up with demand.
Old Pulteney Distillery/UEA
Banelec have provided solutions for multiple projects for Eilbeck Cranes, this example being the most prominent for showcasing what we are capable of. Banelec has been involved in several major projects over the years to help our clients make the most of their investment in biomass fuel and heating systems. Two of the most notable were on the site of the historic Pulteney Distillery, in Scotland, and at the University of East Anglia (UEA).
SAESL –Improving safety and streamlining operations at aerospace centre of excellence
We provided complete control and safety aspects for SAESL’s on-site jet engine core maintenance in Singapore. Our brief was to create a system that allows aero-engine technicians to ergonomically access any part of a civil aircraft jet engine core without stretching or crouching in enclosed, awkward or potentially hazardous positions.
Contact the team at Banelec
Our team is happy to answer your questions or any quote enquiries. Please fill out the form and we’ll be in touch as soon as possible.
01384 484805

We’re a registered Schneider Electric Systems Integrator .
Banelec Ltd 3 Old Bush Street Brierley Hill West Midlands DY5 1UB
© Copyright 2024
Thank you for visiting nature.com. You are using a browser version with limited support for CSS. To obtain the best experience, we recommend you use a more up to date browser (or turn off compatibility mode in Internet Explorer). In the meantime, to ensure continued support, we are displaying the site without styles and JavaScript.
- View all journals
- Explore content
- About the journal
- Publish with us
- Sign up for alerts
- Open access
- Published: 12 September 2024
An open-source framework for end-to-end analysis of electronic health record data
- Lukas Heumos 1 , 2 , 3 ,
- Philipp Ehmele 1 ,
- Tim Treis 1 , 3 ,
- Julius Upmeier zu Belzen ORCID: orcid.org/0000-0002-0966-4458 4 ,
- Eljas Roellin 1 , 5 ,
- Lilly May 1 , 5 ,
- Altana Namsaraeva 1 , 6 ,
- Nastassya Horlava 1 , 3 ,
- Vladimir A. Shitov ORCID: orcid.org/0000-0002-1960-8812 1 , 3 ,
- Xinyue Zhang ORCID: orcid.org/0000-0003-4806-4049 1 ,
- Luke Zappia ORCID: orcid.org/0000-0001-7744-8565 1 , 5 ,
- Rainer Knoll 7 ,
- Niklas J. Lang 2 ,
- Leon Hetzel 1 , 5 ,
- Isaac Virshup 1 ,
- Lisa Sikkema ORCID: orcid.org/0000-0001-9686-6295 1 , 3 ,
- Fabiola Curion 1 , 5 ,
- Roland Eils 4 , 8 ,
- Herbert B. Schiller 2 , 9 ,
- Anne Hilgendorff 2 , 10 &
- Fabian J. Theis ORCID: orcid.org/0000-0002-2419-1943 1 , 3 , 5
Nature Medicine ( 2024 ) Cite this article
72 Altmetric
Metrics details
- Epidemiology
- Translational research
With progressive digitalization of healthcare systems worldwide, large-scale collection of electronic health records (EHRs) has become commonplace. However, an extensible framework for comprehensive exploratory analysis that accounts for data heterogeneity is missing. Here we introduce ehrapy, a modular open-source Python framework designed for exploratory analysis of heterogeneous epidemiology and EHR data. ehrapy incorporates a series of analytical steps, from data extraction and quality control to the generation of low-dimensional representations. Complemented by rich statistical modules, ehrapy facilitates associating patients with disease states, differential comparison between patient clusters, survival analysis, trajectory inference, causal inference and more. Leveraging ontologies, ehrapy further enables data sharing and training EHR deep learning models, paving the way for foundational models in biomedical research. We demonstrate ehrapy’s features in six distinct examples. We applied ehrapy to stratify patients affected by unspecified pneumonia into finer-grained phenotypes. Furthermore, we reveal biomarkers for significant differences in survival among these groups. Additionally, we quantify medication-class effects of pneumonia medications on length of stay. We further leveraged ehrapy to analyze cardiovascular risks across different data modalities. We reconstructed disease state trajectories in patients with severe acute respiratory syndrome coronavirus 2 (SARS-CoV-2) based on imaging data. Finally, we conducted a case study to demonstrate how ehrapy can detect and mitigate biases in EHR data. ehrapy, thus, provides a framework that we envision will standardize analysis pipelines on EHR data and serve as a cornerstone for the community.
Similar content being viewed by others
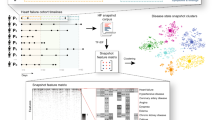
Data-driven identification of heart failure disease states and progression pathways using electronic health records
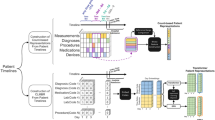
EHR foundation models improve robustness in the presence of temporal distribution shift
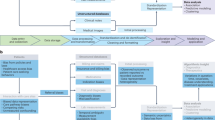
Harnessing EHR data for health research
Electronic health records (EHRs) are becoming increasingly common due to standardized data collection 1 and digitalization in healthcare institutions. EHRs collected at medical care sites serve as efficient storage and sharing units of health information 2 , enabling the informed treatment of individuals using the patient’s complete history 3 . Routinely collected EHR data are approaching genomic-scale size and complexity 4 , posing challenges in extracting information without quantitative analysis methods. The application of such approaches to EHR databases 1 , 5 , 6 , 7 , 8 , 9 has enabled the prediction and classification of diseases 10 , 11 , study of population health 12 , determination of optimal treatment policies 13 , 14 , simulation of clinical trials 15 and stratification of patients 16 .
However, current EHR datasets suffer from serious limitations, such as data collection issues, inconsistencies and lack of data diversity. EHR data collection and sharing problems often arise due to non-standardized formats, with disparate systems using exchange protocols, such as Health Level Seven International (HL7) and Fast Healthcare Interoperability Resources (FHIR) 17 . In addition, EHR data are stored in various on-disk formats, including, but not limited to, relational databases and CSV, XML and JSON formats. These variations pose challenges with respect to data retrieval, scalability, interoperability and data sharing.
Beyond format variability, inherent biases of the collected data can compromise the validity of findings. Selection bias stemming from non-representative sample composition can lead to skewed inferences about disease prevalence or treatment efficacy 18 , 19 . Filtering bias arises through inconsistent criteria for data inclusion, obscuring true variable relationships 20 . Surveillance bias exaggerates associations between exposure and outcomes due to differential monitoring frequencies 21 . EHR data are further prone to missing data 22 , 23 , which can be broadly classified into three categories: missing completely at random (MCAR), where missingness is unrelated to the data; missing at random (MAR), where missingness depends on observed data; and missing not at random (MNAR), where missingness depends on unobserved data 22 , 23 . Information and coding biases, related to inaccuracies in data recording or coding inconsistencies, respectively, can lead to misclassification and unreliable research conclusions 24 , 25 . Data may even contradict itself, such as when measurements were reported for deceased patients 26 , 27 . Technical variation and differing data collection standards lead to distribution differences and inconsistencies in representation and semantics across EHR datasets 28 , 29 . Attrition and confounding biases, resulting from differential patient dropout rates or unaccounted external variable effects, can significantly skew study outcomes 30 , 31 , 32 . The diversity of EHR data that comprise demographics, laboratory results, vital signs, diagnoses, medications, x-rays, written notes and even omics measurements amplifies all the aforementioned issues.
Addressing these challenges requires rigorous study design, careful data pre-processing and continuous bias evaluation through exploratory data analysis. Several EHR data pre-processing and analysis workflows were previously developed 4 , 33 , 34 , 35 , 36 , 37 , but none of them enables the analysis of heterogeneous data, provides in-depth documentation, is available as a software package or allows for exploratory visual analysis. Current EHR analysis pipelines, therefore, differ considerably in their approaches and are often commercial, vendor-specific solutions 38 . This is in contrast to strategies using community standards for the analysis of omics data, such as Bioconductor 39 or scverse 40 . As a result, EHR data frequently remain underexplored and are commonly investigated only for a particular research question 41 . Even in such cases, EHR data are then frequently input into machine learning models with serious data quality issues that greatly impact prediction performance and generalizability 42 .
To address this lack of analysis tooling, we developed the EHR Analysis in Python framework, ehrapy, which enables exploratory analysis of diverse EHR datasets. The ehrapy package is purpose-built to organize, analyze, visualize and statistically compare complex EHR data. ehrapy can be applied to datasets of different data types, sizes, diseases and origins. To demonstrate this versatility, we applied ehrapy to datasets obtained from EHR and population-based studies. Using the Pediatric Intensive Care (PIC) EHR database 43 , we stratified patients diagnosed with ‘unspecified pneumonia’ into distinct clinically relevant groups, extracted clinical indicators of pneumonia through statistical analysis and quantified medication-class effects on length of stay (LOS) with causal inference. Using the UK Biobank 44 (UKB), a population-scale cohort comprising over 500,000 participants from the United Kingdom, we employed ehrapy to explore cardiovascular risk factors using clinical predictors, metabolomics, genomics and retinal imaging-derived features. Additionally, we performed image analysis to project disease progression through fate mapping in patients affected by coronavirus disease 2019 (COVID-19) using chest x-rays. Finally, we demonstrate how exploratory analysis with ehrapy unveils and mitigates biases in over 100,000 visits by patients with diabetes across 130 US hospitals. We provide online links to additional use cases that demonstrate ehrapy’s usage with further datasets, including MIMIC-II (ref. 45 ), and for various medical conditions, such as patients subject to indwelling arterial catheter usage. ehrapy is compatible with any EHR dataset that can be transformed into vectors and is accessible as a user-friendly open-source software package hosted at https://github.com/theislab/ehrapy and installable from PyPI. It comes with comprehensive documentation, tutorials and further examples, all available at https://ehrapy.readthedocs.io .
ehrapy: a framework for exploratory EHR data analysis
The foundation of ehrapy is a robust and scalable data storage backend that is combined with a series of pre-processing and analysis modules. In ehrapy, EHR data are organized as a data matrix where observations are individual patient visits (or patients, in the absence of follow-up visits), and variables represent all measured quantities ( Methods ). These data matrices are stored together with metadata of observations and variables. By leveraging the AnnData (annotated data) data structure that implements this design, ehrapy builds upon established standards and is compatible with analysis and visualization functions provided by the omics scverse 40 ecosystem. Readers are also available in R, Julia and Javascript 46 . We additionally provide a dataset module with more than 20 public loadable EHR datasets in AnnData format to kickstart analysis and development with ehrapy.
For standardized analysis of EHR data, it is crucial that these data are encoded and stored in consistent, reusable formats. Thus, ehrapy requires that input data are organized in structured vectors. Readers for common formats, such as CSV, OMOP 47 or SQL databases, are available in ehrapy. Data loaded into AnnData objects can be mapped against several hierarchical ontologies 48 , 49 , 50 , 51 ( Methods ). Clinical keywords of free text notes can be automatically extracted ( Methods ).
Powered by scanpy, which scales to millions of observations 52 ( Methods and Supplementary Table 1 ) and the machine learning library scikit-learn 53 , ehrapy provides more than 100 composable analysis functions organized in modules from which custom analysis pipelines can be built. Each function directly interacts with the AnnData object and adds all intermediate results for simple access and reuse of information to it. To facilitate setting up these pipelines, ehrapy guides analysts through a general analysis pipeline (Fig. 1 ). At any step of an analysis pipeline, community software packages can be integrated without any vendor lock-in. Because ehrapy is built on open standards, it can be purposefully extended to solve new challenges, such as the development of foundational models ( Methods ).
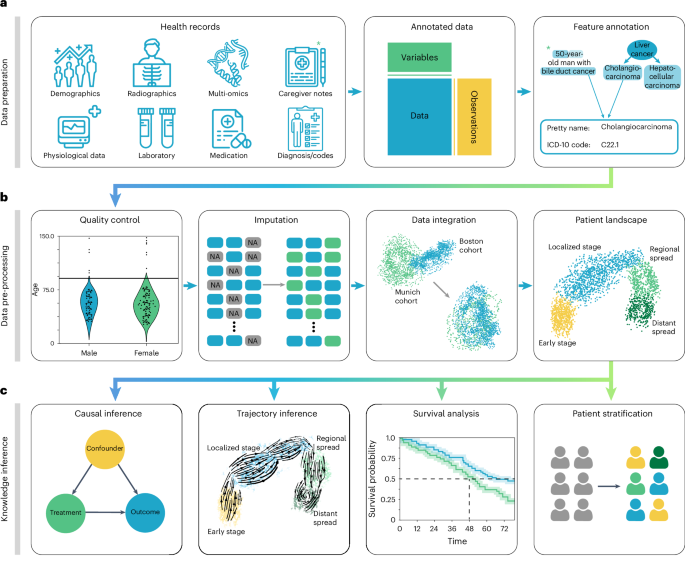
a , Heterogeneous health data are first loaded into memory as an AnnData object with patient visits as observational rows and variables as columns. Next, the data can be mapped against ontologies, and key terms are extracted from free text notes. b , The EHR data are subject to quality control where low-quality or spurious measurements are removed or imputed. Subsequently, numerical data are normalized, and categorical data are encoded. Data from different sources with data distribution shifts are integrated, embedded, clustered and annotated in a patient landscape. c , Further downstream analyses depend on the question of interest and can include the inference of causal effects and trajectories, survival analysis or patient stratification.
In the ehrapy analysis pipeline, EHR data are initially inspected for quality issues by analyzing feature distributions that may skew results and by detecting visits and features with high missing rates that ehrapy can then impute ( Methods ). ehrapy tracks all filtering steps while keeping track of population dynamics to highlight potential selection and filtering biases ( Methods ). Subsequently, ehrapy’s normalization and encoding functions ( Methods ) are applied to achieve a uniform numerical representation that facilitates data integration and corrects for dataset shift effects ( Methods ). Calculated lower-dimensional representations can subsequently be visualized, clustered and annotated to obtain a patient landscape ( Methods ). Such annotated groups of patients can be used for statistical comparisons to find differences in features among them to ultimately learn markers of patient states.
As analysis goals can differ between users and datasets, the ehrapy analysis pipeline is customizable during the final knowledge inference step. ehrapy provides statistical methods for group comparison and extensive support for survival analysis ( Methods ), enabling the discovery of biomarkers. Furthermore, ehrapy offers functions for causal inference to go from statistically determined associations to causal relations ( Methods ). Moreover, patient visits in aggregated EHR data can be regarded as snapshots where individual measurements taken at specific timepoints might not adequately reflect the underlying progression of disease and result from unrelated variation due to, for example, day-to-day differences 54 , 55 , 56 . Therefore, disease progression models should rely on analysis of the underlying clinical data, as disease progression in an individual patient may not be monotonous in time. ehrapy allows for the use of advanced trajectory inference methods to overcome sparse measurements 57 , 58 , 59 . We show that this approach can order snapshots to calculate a pseudotime that can adequately reflect the progression of the underlying clinical process. Given a sufficient number of snapshots, ehrapy increases the potential to understand disease progression, which is likely not robustly captured within a single EHR but, rather, across several.
ehrapy enables patient stratification in pneumonia cases
To demonstrate ehrapy’s capability to analyze heterogeneous datasets from a broad patient set across multiple care units, we applied our exploratory strategy to the PIC 43 database. The PIC database is a single-center database hosting information on children admitted to critical care units at the Children’s Hospital of Zhejiang University School of Medicine in China. It contains 13,499 distinct hospital admissions of 12,881 individual pediatric patients admitted between 2010 and 2018 for whom demographics, diagnoses, doctors’ notes, vital signs, laboratory and microbiology tests, medications, fluid balances and more were collected (Extended Data Figs. 1 and 2a and Methods ). After missing data imputation and subsequent pre-processing (Extended Data Figs. 2b,c and 3 and Methods ), we generated a uniform manifold approximation and projection (UMAP) embedding to visualize variation across all patients using ehrapy (Fig. 2a ). This visualization of the low-dimensional patient manifold shows the heterogeneity of the collected data in the PIC database, with malformations, perinatal and respiratory being the most abundant International Classification of Diseases (ICD) chapters (Fig. 2b ). The most common respiratory disease categories (Fig. 2c ) were labeled pneumonia and influenza ( n = 984). We focused on pneumonia to apply ehrapy to a challenging, broad-spectrum disease that affects all age groups. Pneumonia is a prevalent respiratory infection that poses a substantial burden on public health 60 and is characterized by inflammation of the alveoli and distal airways 60 . Individuals with pre-existing chronic conditions are particularly vulnerable, as are children under the age of 5 (ref. 61 ). Pneumonia can be caused by a range of microorganisms, encompassing bacteria, respiratory viruses and fungi.
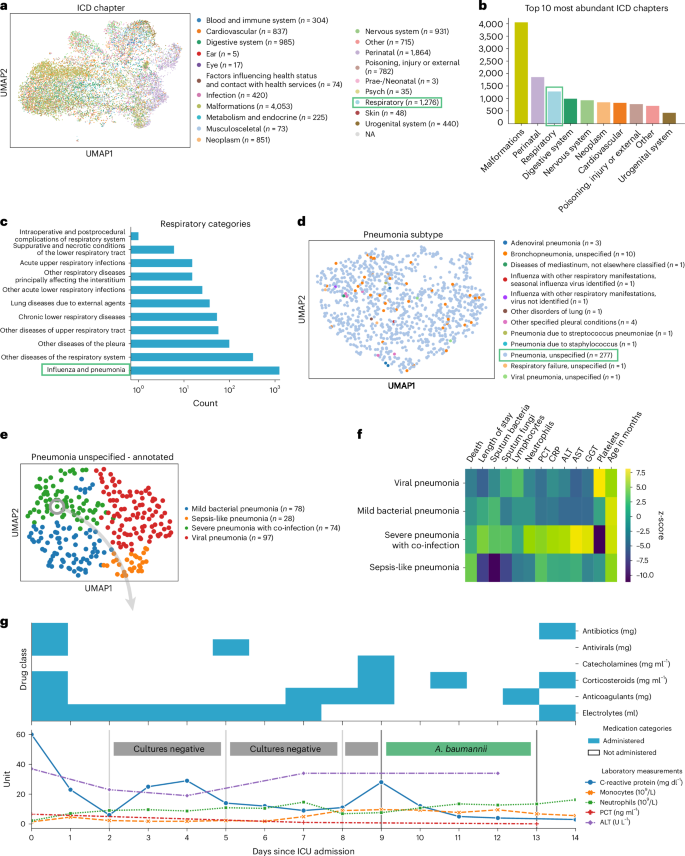
a , UMAP of all patient visits in the ICU with primary discharge diagnosis grouped by ICD chapter. b , The prevalence of respiratory diseases prompted us to investigate them further. c , Respiratory categories show the abundance of influenza and pneumonia diagnoses that we investigated more closely. d , We observed the ‘unspecified pneumonia’ subgroup, which led us to investigate and annotate it in more detail. e , The previously ‘unspecified pneumonia’-labeled patients were annotated using several clinical features (Extended Data Fig. 5 ), of which the most important ones are shown in the heatmap ( f ). g , Example disease progression of an individual child with pneumonia illustrating pharmacotherapy over time until positive A. baumannii swab.
We selected the age group ‘youths’ (13 months to 18 years of age) for further analysis, addressing a total of 265 patients who dominated the pneumonia cases and were diagnosed with ‘unspecified pneumonia’ (Fig. 2d and Extended Data Fig. 4 ). Neonates (0–28 d old) and infants (29 d to 12 months old) were excluded from the analysis as the disease context is significantly different in these age groups due to distinct anatomical and physical conditions. Patients were 61% male, had a total of 277 admissions, had a mean age at admission of 54 months (median, 38 months) and had an average LOS of 15 d (median, 7 d). Of these, 152 patients were admitted to the pediatric intensive care unit (PICU), 118 to the general ICU (GICU), four to the surgical ICU (SICU) and three to the cardiac ICU (CICU). Laboratory measurements typically had 12–14% missing data, except for serum procalcitonin (PCT), a marker for bacterial infections, with 24.5% missing, and C-reactive protein (CRP), a marker of inflammation, with 16.8% missing. Measurements assigned as ‘vital signs’ contained between 44% and 54% missing values. Stratifying patients with unspecified pneumonia further enables a more nuanced understanding of the disease, potentially facilitating tailored approaches to treatment.
To deepen clinical phenotyping for the disease group ‘unspecified pneumonia’, we calculated a k -nearest neighbor graph to cluster patients into groups and visualize these in UMAP space ( Methods ). Leiden clustering 62 identified four patient groupings with distinct clinical features that we annotated (Fig. 2e ). To identify the laboratory values, medications and pathogens that were most characteristic for these four groups (Fig. 2f ), we applied t -tests for numerical data and g -tests for categorical data between the identified groups using ehrapy (Extended Data Fig. 5 and Methods ). Based on this analysis, we identified patient groups with ‘sepsis-like, ‘severe pneumonia with co-infection’, ‘viral pneumonia’ and ‘mild pneumonia’ phenotypes. The ‘sepsis-like’ group of patients ( n = 28) was characterized by rapid disease progression as exemplified by an increased number of deaths (adjusted P ≤ 5.04 × 10 −3 , 43% ( n = 28), 95% confidence interval (CI): 23%, 62%); indication of multiple organ failure, such as elevated creatinine (adjusted P ≤ 0.01, 52.74 ± 23.71 μmol L −1 ) or reduced albumin levels (adjusted P ≤ 2.89 × 10 −4 , 33.40 ± 6.78 g L −1 ); and increased expression levels and peaks of inflammation markers, including PCT (adjusted P ≤ 3.01 × 10 −2 , 1.42 ± 2.03 ng ml −1 ), whole blood cell count, neutrophils, lymphocytes, monocytes and lower platelet counts (adjusted P ≤ 6.3 × 10 −2 , 159.30 ± 142.00 × 10 9 per liter) and changes in electrolyte levels—that is, lower potassium levels (adjusted P ≤ 0.09 × 10 −2 , 3.14 ± 0.54 mmol L −1 ). Patients whom we associated with the term ‘severe pneumonia with co-infection’ ( n = 74) were characterized by prolonged ICU stays (adjusted P ≤ 3.59 × 10 −4 , 15.01 ± 29.24 d); organ affection, such as higher levels of creatinine (adjusted P ≤ 1.10 × 10 −4 , 52.74 ± 23.71 μmol L −1 ) and lower platelet count (adjusted P ≤ 5.40 × 10 −23 , 159.30 ± 142.00 × 10 9 per liter); increased inflammation markers, such as peaks of PCT (adjusted P ≤ 5.06 × 10 −5 , 1.42 ± 2.03 ng ml −1 ), CRP (adjusted P ≤ 1.40 × 10 −6 , 50.60 ± 37.58 mg L −1 ) and neutrophils (adjusted P ≤ 8.51 × 10 −6 , 13.01 ± 6.98 × 10 9 per liter); detection of bacteria in combination with additional pathogen fungals in sputum samples (adjusted P ≤ 1.67 × 10 −2 , 26% ( n = 74), 95% CI: 16%, 36%); and increased application of medication, including antifungals (adjusted P ≤ 1.30 × 10 −4 , 15% ( n = 74), 95% CI: 7%, 23%) and catecholamines (adjusted P ≤ 2.0 × 10 −2 , 45% ( n = 74), 95% CI: 33%, 56%). Patients in the ‘mild pneumonia’ group were characterized by positive sputum cultures in the presence of relatively lower inflammation markers, such as PCT (adjusted P ≤ 1.63 × 10 −3 , 1.42 ± 2.03 ng ml −1 ) and CRP (adjusted P ≤ 0.03 × 10 −1 , 50.60 ± 37.58 mg L −1 ), while receiving antibiotics more frequently (adjusted P ≤ 1.00 × 10 −5 , 80% ( n = 78), 95% CI: 70%, 89%) and additional medications (electrolytes, blood thinners and circulation-supporting medications) (adjusted P ≤ 1.00 × 10 −5 , 82% ( n = 78), 95% CI: 73%, 91%). Finally, patients in the ‘viral pneumonia’ group were characterized by shorter LOSs (adjusted P ≤ 8.00 × 10 −6 , 15.01 ± 29.24 d), a lack of non-viral pathogen detection in combination with higher lymphocyte counts (adjusted P ≤ 0.01, 4.11 ± 2.49 × 10 9 per liter), lower levels of PCT (adjusted P ≤ 0.03 × 10 −2 , 1.42 ± 2.03 ng ml −1 ) and reduced application of catecholamines (adjusted P ≤ 5.96 × 10 −7 , 15% (n = 97), 95% CI: 8%, 23%), antibiotics (adjusted P ≤ 8.53 × 10 −6 , 41% ( n = 97), 95% CI: 31%, 51%) and antifungals (adjusted P ≤ 5.96 × 10 −7 , 0% ( n = 97), 95% CI: 0%, 0%).
To demonstrate the ability of ehrapy to examine EHR data from different levels of resolution, we additionally reconstructed a case from the ‘severe pneumonia with co-infection’ group (Fig. 2g ). In this case, the analysis revealed that CRP levels remained elevated despite broad-spectrum antibiotic treatment until a positive Acinetobacter baumannii result led to a change in medication and a subsequent decrease in CRP and monocyte levels.
ehrapy facilitates extraction of pneumonia indicators
ehrapy’s survival analysis module allowed us to identify clinical indicators of disease stages that could be used as biomarkers through Kaplan–Meier analysis. We found strong variance in overall aspartate aminotransferase (AST), alanine aminotransferase (ALT), gamma-glutamyl transferase (GGT) and bilirubin levels (Fig. 3a ), including changes over time (Extended Data Fig. 6a,b ), in all four ‘unspecified pneumonia’ groups. Routinely used to assess liver function, studies provide evidence that AST, ALT and GGT levels are elevated during respiratory infections 63 , including severe pneumonia 64 , and can guide diagnosis and management of pneumonia in children 63 . We confirmed reduced survival in more severely affected children (‘sepsis-like pneumonia’ and ‘severe pneumonia with co-infection’) using Kaplan–Meier curves and a multivariate log-rank test (Fig. 3b ; P ≤ 1.09 × 10 −18 ) through ehrapy. To verify the association of this trajectory with altered AST, ALT and GGT expression levels, we further grouped all patients based on liver enzyme reference ranges ( Methods and Supplementary Table 2 ). By Kaplan–Meier survival analysis, cases with peaks of GGT ( P ≤ 1.4 × 10 −2 , 58.01 ± 2.03 U L −1 ), ALT ( P ≤ 2.9 × 10 −2 , 43.59 ± 38.02 U L −1 ) and AST ( P ≤ 4.8 × 10 −4 , 78.69 ± 60.03 U L −1 ) in ‘outside the norm’ were found to correlate with lower survival in all groups (Fig. 3c and Extended Data Fig. 6 ), in line with previous studies 63 , 65 . Bilirubin was not found to significantly affect survival ( P ≤ 2.1 × 10 −1 , 12.57 ± 21.22 mg dl −1 ).
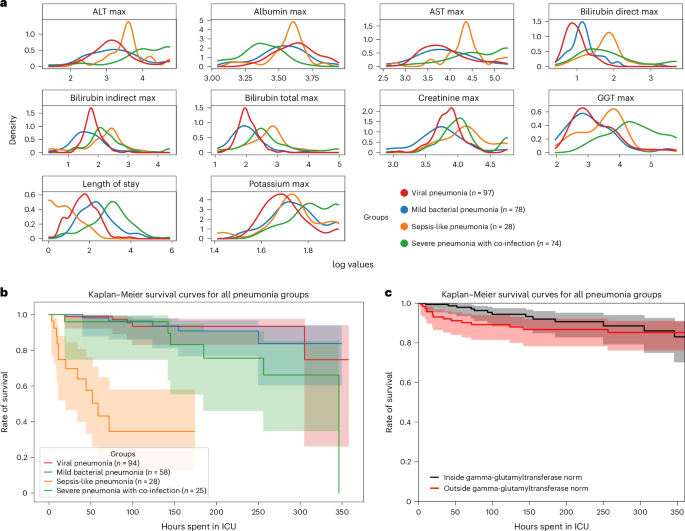
a , Line plots of major hepatic system laboratory measurements per group show variance in the measurements per pneumonia group. b , Kaplan–Meier survival curves demonstrate lower survival for ‘sepsis-like’ and ‘severe pneumonia with co-infection’ groups. c , Kaplan–Meier survival curves for children with GGT measurements outside the norm range display lower survival.
ehrapy quantifies medication class effect on LOS
Pneumonia requires case-specific medications due to its diverse causes. To demonstrate the potential of ehrapy’s causal inference module, we quantified the effect of medication on ICU LOS to evaluate case-specific administration of medication. In contrast to causal discovery that attempts to find a causal graph reflecting the causal relationships, causal inference is a statistical process used to investigate possible effects when altering a provided system, as represented by a causal graph and observational data (Fig. 4a ) 66 . This approach allows identifying and quantifying the impact of specific interventions or treatments on outcome measures, thereby providing insight for evidence-based decision-making in healthcare. Causal inference relies on datasets incorporating interventions to accurately quantify effects.
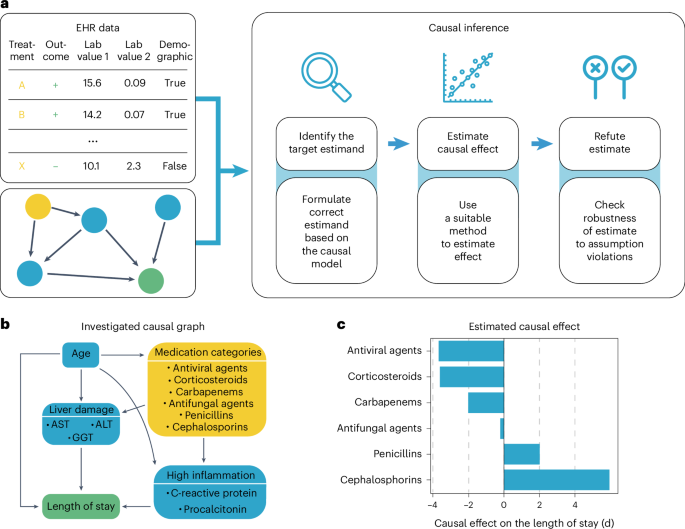
a , ehrapy’s causal module is based on the strategy of the tool ‘dowhy’. Here, EHR data containing treatment, outcome and measurements and a causal graph serve as input for causal effect quantification. The process includes the identification of the target estimand based on the causal graph, the estimation of causal effects using various models and, finally, refutation where sensitivity analyses and refutation tests are performed to assess the robustness of the results and assumptions. b , Curated causal graph using age, liver damage and inflammation markers as disease progression proxies together with medications as interventions to assess the causal effect on length of ICU stay. c , Determined causal effect strength on LOS in days of administered medication categories.
We manually constructed a minimal causal graph with ehrapy (Fig. 4b ) on records of treatment with corticosteroids, carbapenems, penicillins, cephalosporins and antifungal and antiviral medications as interventions (Extended Data Fig. 7 and Methods ). We assumed that the medications affect disease progression proxies, such as inflammation markers and markers of organ function. The selection of ‘interventions’ is consistent with current treatment standards for bacterial pneumonia and respiratory distress 67 , 68 . Based on the approach of the tool ‘dowhy’ 69 (Fig. 4a ), ehrapy’s causal module identified the application of corticosteroids, antivirals and carbapenems to be associated with shorter LOSs, in line with current evidence 61 , 70 , 71 , 72 . In contrast, penicillins and cephalosporins were associated with longer LOSs, whereas antifungal medication did not strongly influence LOS (Fig. 4c ).
ehrapy enables deriving population-scale risk factors
To illustrate the advantages of using a unified data management and quality control framework, such as ehrapy, we modeled myocardial infarction risk using Cox proportional hazards models on UKB 44 data. Large population cohort studies, such as the UKB, enable the investigation of common diseases across a wide range of modalities, including genomics, metabolomics, proteomics, imaging data and common clinical variables (Fig. 5a,b ). From these, we used a publicly available polygenic risk score for coronary heart disease 73 comprising 6.6 million variants, 80 nuclear magnetic resonance (NMR) spectroscopy-based metabolomics 74 features, 81 features derived from retinal optical coherence tomography 75 , 76 and the Framingham Risk Score 77 feature set, which includes known clinical predictors, such as age, sex, body mass index, blood pressure, smoking behavior and cholesterol levels. We excluded features with more than 10% missingness and imputed the remaining missing values ( Methods ). Furthermore, individuals with events up to 1 year after the sampling time were excluded from the analyses, ultimately selecting 29,216 individuals for whom all mentioned data types were available (Extended Data Figs. 8 and 9 and Methods ). Myocardial infarction, as defined by our mapping to the phecode nomenclature 51 , was defined as the endpoint (Fig. 5c ). We modeled the risk for myocardial infarction 1 year after either the metabolomic sample was obtained or imaging was performed.
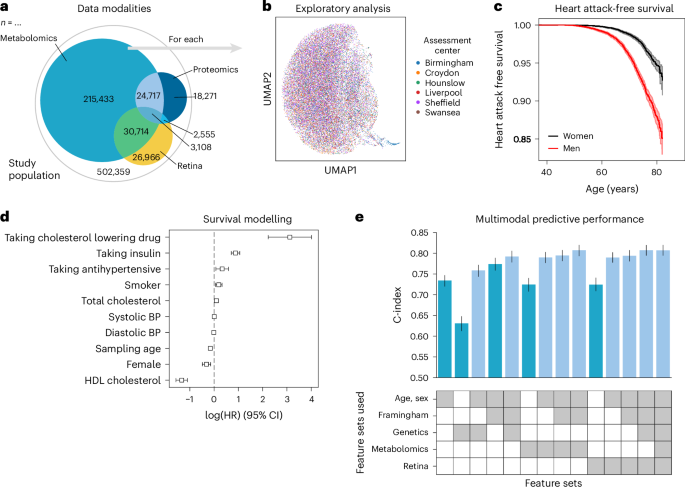
a , The UKB includes 502,359 participants from 22 assessment centers. Most participants have genetic data (97%) and physical measurement data (93%), but fewer have data for complex measures, such as metabolomics, retinal imaging or proteomics. b , We found a distinct cluster of individuals (bottom right) from the Birmingham assessment center in the retinal imaging data, which is an artifact of the image acquisition process and was, thus, excluded. c , Myocardial infarctions are recorded for 15% of the male and 7% of the female study population. Kaplan–Meier estimators with 95% CIs are shown. d , For every modality combination, a linear Cox proportional hazards model was fit to determine the prognostic potential of these for myocardial infarction. Cardiovascular risk factors show expected positive log hazard ratios (log (HRs)) for increased blood pressure or total cholesterol and negative ones for sampling age and systolic blood pressure (BP). log (HRs) with 95% CIs are shown. e , Combining all features yields a C-index of 0.81. c – e , Error bars indicate 95% CIs ( n = 29,216).
Predictive performance for each modality was assessed by fitting Cox proportional hazards (Fig. 5c ) models on each of the feature sets using ehrapy (Fig. 5d ). The age of the first occurrence served as the time to event; alternatively, date of death or date of the last record in the EHR served as censoring times. Models were evaluated using the concordance index (C-index) ( Methods ). The combination of multiple modalities successfully improved the predictive performance for coronary heart disease by increasing the C-index from 0.63 (genetic) to 0.76 (genetics, age and sex) and to 0.77 (clinical predictors) with 0.81 (imaging and clinical predictors) for combinations of feature sets (Fig. 5e ). Our finding is in line with previous observations of complementary effects between different modalities, where a broader ‘major adverse cardiac event’ phenotype was modeled in the UKB achieving a C-index of 0.72 (ref. 78 ). Adding genetic data improves predictive potential, as it is independent of sampling age and has limited prediction of other modalities 79 . The addition of metabolomic data did not improve predictive power (Fig. 5e ).
Imaging-based disease severity projection via fate mapping
To demonstrate ehrapy’s ability to handle diverse image data and recover disease stages, we embedded pulmonary imaging data obtained from patients with COVID-19 into a lower-dimensional space and computationally inferred disease progression trajectories using pseudotemporal ordering. This describes a continuous trajectory or ordering of individual points based on feature similarity 80 . Continuous trajectories enable mapping the fate of new patients onto precise states to potentially predict their future condition.
In COVID-19, a highly contagious respiratory illness caused by severe acute respiratory syndrome coronavirus 2 (SARS-CoV-2), symptoms range from mild flu-like symptoms to severe respiratory distress. Chest x-rays typically show opacities (bilateral patchy, ground glass) associated with disease severity 81 .
We used COVID-19 chest x-ray images from the BrixIA 82 dataset consisting of 192 images (Fig. 6a ) with expert annotations of disease severity. We used the BrixIA database scores, which are based on six regions annotated by radiologists, to classify disease severity ( Methods ). We embedded raw image features using a pre-trained DenseNet model ( Methods ) and further processed this embedding into a nearest-neighbors-based UMAP space using ehrapy (Fig. 6b and Methods ). Fate mapping based on imaging information ( Methods ) determined a severity ordering from mild to critical cases (Fig. 6b–d ). Images labeled as ‘normal’ are projected to stay within the healthy group, illustrating the robustness of our approach. Images of diseased patients were ordered by disease severity, highlighting clear trajectories from ‘normal’ to ‘critical’ states despite the heterogeneity of the x-ray images stemming from, for example, different zoom levels (Fig. 6a ).
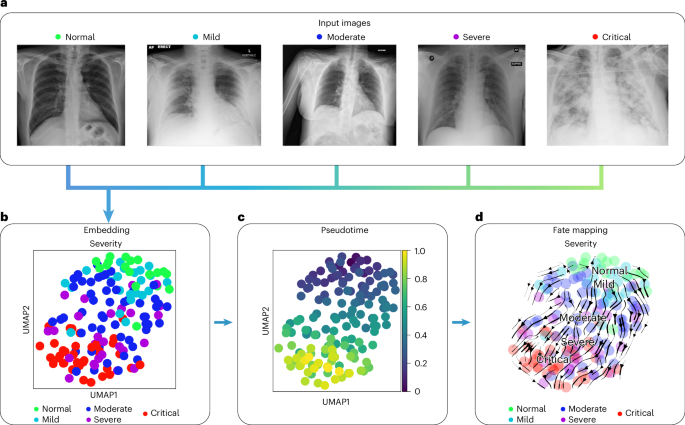
a , Randomly selected chest x-ray images from the BrixIA dataset demonstrate its variance. b , UMAP visualization of the BrixIA dataset embedding shows a separation of disease severity classes. c , Calculated pseudotime for all images increases with distance to the ‘normal’ images. d , Stream projection of fate mapping in UMAP space showcases disease severity trajectory of the COVID-19 chest x-ray images.
Detecting and mitigating biases in EHR data with ehrapy
To showcase how exploratory analysis using ehrapy can reveal and mitigate biases, we analyzed the Fairlearn 83 version of the Diabetes 130-US Hospitals 84 dataset. The dataset covers 10 years (1999–2008) of clinical records from 130 US hospitals, detailing 47 features of diabetes diagnoses, laboratory tests, medications and additional data from up to 14 d of inpatient care of 101,766 diagnosed patient visits ( Methods ). It was originally collected to explore the link between the measurement of hemoglobin A1c (HbA1c) and early readmission.
The cohort primarily consists of White and African American individuals, with only a minority of cases from Asian or Hispanic backgrounds (Extended Data Fig. 10a ). ehrapy’s cohort tracker unveiled selection and surveillance biases when filtering for Medicare recipients for further analysis, resulting in a shift of age distribution toward an age of over 60 years in addition to an increasing ratio of White participants. Using ehrapy’s visualization modules, our analysis showed that HbA1c was measured in only 18.4% of inpatients, with a higher frequency in emergency admissions compared to referral cases (Extended Data Fig. 10b ). Normalization biases can skew data relationships when standardization techniques ignore subgroup variability or assume incorrect distributions. The choice of normalization strategy must be carefully considered to avoid obscuring important factors. When normalizing the number of applied medications individually, differences in distributions between age groups remained. However, when normalizing both distributions jointly with age group as an additional group variable, differences between age groups were masked (Extended Data Fig. 10c ). To investigate missing data and imputation biases, we introduced missingness for the number of applied medications according to an MCAR mechanism, which we verified using ehrapy’s Little’s test ( P ≤ 0.01 × 10 −2 ), and an MAR mechanism ( Methods ). Whereas imputing the mean in the MCAR case did not affect the overall location of the distribution, it led to an underestimation of the variance, with the standard deviation dropping from 8.1 in the original data to 6.8 in the imputed data (Extended Data Fig. 10d ). Mean imputation in the MAR case skewed both location and variance of the mean from 16.02 to 14.66, with a standard deviation of only 5.72 (Extended Data Fig. 10d ). Using ehrapy’s multiple imputation based MissForest 85 imputation on the MAR data resulted in a mean of 16.04 and a standard deviation of 6.45. To predict patient readmission in fewer than 30 d, we merged the three smallest race groups, ‘Asian’, ‘Hispanic’ and ‘Other’. Furthermore, we dropped the gender group ‘Unknown/Invalid’ owing to the small sample size making meaningful assessment impossible, and we performed balanced random undersampling, resulting in 5,677 cases from each condition. We observed an overall balanced accuracy of 0.59 using a logistic regression model. However, the false-negative rate was highest for the races ‘Other’ and ‘Unknown’, whereas their selection rate was lowest, and this model was, therefore, biased (Extended Data Fig. 10e ). Using ehrapy’s compatibility with existing machine learning packages, we used Fairlearn’s ThresholdOptimizer ( Methods ), which improved the selection rates for ‘Other’ from 0.32 to 0.38 and for ‘Unknown’ from 0.23 to 0.42 and the false-negative rates for ‘Other’ from 0.48 to 0.42 and for ‘Unknown’ from 0.61 to 0.45 (Extended Data Fig. 10e ).
Clustering offers a hypothesis-free alternative to supervised classification when clear hypotheses or labels are missing. It has enabled the identification of heart failure subtypes 86 and progression pathways 87 and COVID-19 severity states 88 . This concept, which is central to ehrapy, further allowed us to identify fine-grained groups of ‘unspecified pneumonia’ cases in the PIC dataset while discovering biomarkers and quantifying effects of medications on LOS. Such retroactive characterization showcases ehrapy’s ability to put complex evidence into context. This approach supports feedback loops to improve diagnostic and therapeutic strategies, leading to more efficiently allocated resources in healthcare.
ehrapy’s flexible data structures enabled us to integrate the heterogeneous UKB data for predictive performance in myocardial infarction. The different data types and distributions posed a challenge for predictive models that were overcome with ehrapy’s pre-processing modules. Our analysis underscores the potential of combining phenotypic and health data at population scale through ehrapy to enhance risk prediction.
By adapting pseudotime approaches that are commonly used in other omics domains, we successfully recovered disease trajectories from raw imaging data with ehrapy. The determined pseudotime, however, only orders data but does not necessarily provide a future projection per patient. Understanding the driver features for fate mapping in image-based datasets is challenging. The incorporation of image segmentation approaches could mitigate this issue and provide a deeper insight into the spatial and temporal dynamics of disease-related processes.
Limitations of our analyses include the lack of control for informative missingness where the absence of information represents information in itself 89 . Translation from Chinese to English in the PIC database can cause information loss and inaccuracies because the Chinese ICD-10 codes are seven characters long compared to the five-character English codes. Incompleteness of databases, such as the lack of radiology images in the PIC database, low sample sizes, underrepresentation of non-White ancestries and participant self-selection, cannot be accounted for and limit generalizability. This restricts deeper phenotyping of, for example, all ‘unspecified pneumonia’ cases with respect to their survival, which could be overcome by the use of multiple databases. Our causal inference use case is limited by unrecorded variables, such as Sequential Organ Failure Assessment (SOFA) scores, and pneumonia-related pathogens that are missing in the causal graph due to dataset constraints, such as high sparsity and substantial missing data, which risk overfitting and can lead to overinterpretation. We counterbalanced this by employing several refutation methods that statistically reject the causal hypothesis, such as a placebo treatment, a random common cause or an unobserved common cause. The longer hospital stays associated with penicillins and cephalosporins may be dataset specific and stem from higher antibiotic resistance, their use as first-line treatments, more severe initial cases, comorbidities and hospital-specific protocols.
Most analysis steps can introduce algorithmic biases where results are misleading or unfavorably affect specific groups. This is particularly relevant in the context of missing data 22 where determining the type of missing data is necessary to handle it correctly. ehrapy includes an implementation of Little’s test 90 , which tests whether data are distributed MCAR to discern missing data types. For MCAR data single-imputation approaches, such as mean, median or mode, imputation can suffice, but these methods are known to reduce variability 91 , 92 . Multiple imputation strategies, such as Multiple Imputation by Chained Equations (MICE) 93 and MissForest 85 , as implemented in ehrapy, are effective for both MCAR and MAR data 22 , 94 , 95 . MNAR data require pattern-mixture or shared-parameter models that explicitly incorporate the mechanism by which data are missing 96 . Because MNAR involves unobserved data, the assumptions about the missingness mechanism cannot be directly verified, making sensitivity analysis crucial 21 . ehrapy’s wide range of normalization functions and grouping functionality enables to account for intrinsic variability within subgroups, and its compatibility with Fairlearn 83 can potentially mitigate predictor biases. Generally, we recommend to assess all pre-processing in an iterative manner with respect to downstream applications, such as patient stratification. Moreover, sensitivity analysis can help verify the robustness of all inferred knowledge 97 .
These diverse use cases illustrate ehrapy’s potential to sufficiently address the need for a computationally efficient, extendable, reproducible and easy-to-use framework. ehrapy is compatible with major standards, such as Observational Medical Outcomes Partnership (OMOP), Common Data Model (CDM) 47 , HL7, FHIR or openEHR, with flexible support for common tabular data formats. Once loaded into an AnnData object, subsequent sharing of analysis results is made easy because AnnData objects can be stored and read platform independently. ehrapy’s rich documentation of the application programming interface (API) and extensive hands-on tutorials make EHR analysis accessible to both novices and experienced analysts.
As ehrapy remains under active development, users can expect ehrapy to continuously evolve. We are improving support for the joint analysis of EHR, genetics and molecular data where ehrapy serves as a bridge between the EHR and the omics communities. We further anticipate the generation of EHR-specific reference datasets, so-called atlases 98 , to enable query-to-reference mapping where new datasets get contextualized by transferring annotations from the reference to the new dataset. To promote the sharing and collective analysis of EHR data, we envision adapted versions of interactive single-cell data explorers, such as CELLxGENE 99 or the UCSC Cell Browser 100 , for EHR data. Such web interfaces would also include disparity dashboards 20 to unveil trends of preferential outcomes for distinct patient groups. Additional modules specifically for high-frequency time-series data, natural language processing and other data types are currently under development. With the widespread availability of code-generating large language models, frameworks such as ehrapy are becoming accessible to medical professionals without coding expertise who can leverage its analytical power directly. Therefore, ehrapy, together with a lively ecosystem of packages, has the potential to enhance the scientific discovery pipeline to shape the era of EHR analysis.
All datasets that were used during the development of ehrapy and the use cases were used according to their terms of use as indicated by each provider.
Design and implementation of ehrapy
A unified pipeline as provided by our ehrapy framework streamlines the analysis of EHR data by providing an efficient, standardized approach, which reduces the complexity and variability in data pre-processing and analysis. This consistency ensures reproducibility of results and facilitates collaboration and sharing within the research community. Additionally, the modular structure allows for easy extension and customization, enabling researchers to adapt the pipeline to their specific needs while building on a solid foundational framework.
ehrapy was designed from the ground up as an open-source effort with community support. The package, as well as all associated tutorials and dataset preparation scripts, are open source. Development takes place publicly on GitHub where the developers discuss feature requests and issues directly with users. This tight interaction between both groups ensures that we implement the most pressing needs to cater the most important use cases and can guide users when difficulties arise. The open-source nature, extensive documentation and modular structure of ehrapy are designed for other developers to build upon and extend ehrapy’s functionality where necessary. This allows us to focus ehrapy on the most important features to keep the number of dependencies to a minimum.
ehrapy was implemented in the Python programming language and builds upon numerous existing numerical and scientific open-source libraries, specifically matplotlib 101 , seaborn 102 , NumPy 103 , numba 104 , Scipy 105 , scikit-learn 53 and Pandas 106 . Although taking considerable advantage of all packages implemented, ehrapy also shares the limitations of these libraries, such as a lack of GPU support or small performance losses due to the translation layer cost for operations between the Python interpreter and the lower-level C language for matrix operations. However, by building on very widely used open-source software, we ensure seamless integration and compatibility with a broad range of tools and platforms to promote community contributions. Additionally, by doing so, we enhance security by allowing a larger pool of developers to identify and address vulnerabilities 107 . All functions are grouped into task-specific modules whose implementation is complemented with additional dependencies.
Data preparation
Dataloaders.
ehrapy is compatible with any type of vectorized data, where vectorized refers to the data being stored in structured tables in either on-disk or database form. The input and output module of ehrapy provides readers for common formats, such as OMOP, CSV tables or SQL databases through Pandas. When reading in such datasets, the data are stored in the appropriate slots in a new AnnData 46 object. ehrapy’s data module provides access to more than 20 public EHR datasets that feature diseases, including, but not limited to, Parkinson’s disease, breast cancer, chronic kidney disease and more. All dataloaders return AnnData objects to allow for immediate analysis.
AnnData for EHR data
Our framework required a versatile data structure capable of handling various matrix formats, including Numpy 103 for general use cases and interoperability, Scipy 105 sparse matrices for efficient storage, Dask 108 matrices for larger-than-memory analysis and Awkward array 109 for irregular time-series data. We needed a single data structure that not only stores data but also includes comprehensive annotations for thorough contextual analysis. It was essential for this structure to be widely used and supported, which ensures robustness and continual updates. Interoperability with other analytical packages was a key criterion to facilitate seamless integration within existing tools and workflows. Finally, the data structure had to support both in-memory operations and on-disk storage using formats such as HDF5 (ref. 110 ) and Zarr 111 , ensuring efficient handling and accessibility of large datasets and the ability to easily share them with collaborators.
All of these requirements are fulfilled by the AnnData format, which is a popular data structure in single-cell genomics. At its core, an AnnData object encapsulates diverse components, providing a holistic representation of data and metadata that are always aligned in dimensions and easily accessible. A data matrix (commonly referred to as ‘ X ’) stands as the foundational element, embodying the measured data. This matrix can be dense (as Numpy array), sparse (as Scipy sparse matrix) or ragged (as Awkward array) where dimensions do not align within the data matrix. The AnnData object can feature several such data matrices stored in ‘layers’. Examples of such layers can be unnormalized or unencoded data. These data matrices are complemented by an observations (commonly referred to as ‘obs’) segment where annotations on the level of patients or visits are stored. Patients’ age or sex, for instance, are often used as such annotations. The variables (commonly referred to as ‘var’) section complements the observations, offering supplementary details about the features in the dataset, such as missing data rates. The observation-specific matrices (commonly referred to as ‘obsm’) section extends the capabilities of the AnnData structure by allowing the incorporation of observation-specific matrices. These matrices can represent various types of information at the individual cell level, such as principal component analysis (PCA) results, t-distributed stochastic neighbor embedding (t-SNE) coordinates or other dimensionality reduction outputs. Analogously, AnnData features a variables-specific variables (commonly referred to as ‘varm’) component. The observation-specific pairwise relationships (commonly referred to as ‘obsp’) segment complements the ‘obsm’ section by accommodating observation-specific pairwise relationships. This can include connectivity matrices, indicating relationships between patients. The inclusion of an unstructured annotations (commonly referred to as ‘uns’) component further enhances flexibility. This segment accommodates unstructured annotations or arbitrary data that might not conform to the structured observations or variables categories. Any AnnData object can be stored on disk in h5ad or Zarr format to facilitate data exchange.
ehrapy natively interfaces with the scientific Python ecosystem via Pandas 112 and Numpy 103 . The development of deep learning models for EHR data 113 is further accelerated through compatibility with pathml 114 , a unified framework for whole-slide image analysis in pathology, and scvi-tools 115 , which provides data loaders for loading tensors from AnnData objects into PyTorch 116 or Jax arrays 117 to facilitate the development of generalizing foundational models for medical artificial intelligence 118 .
Feature annotation
After AnnData creation, any metadata can be mapped against ontologies using Bionty ( https://github.com/laminlabs/bionty-base ). Bionty provides access to the Human Phenotype, Phecodes, Phenotype and Trait, Drug, Mondo and Human Disease ontologies.
Key medical terms stored in an AnnData object in free text can be extracted using the Medical Concept Annotation Toolkit (MedCAT) 119 .
Data processing
Cohort tracking.
ehrapy provides a CohortTracker tool that traces all filtering steps applied to an associated AnnData object. To calculate cohort summary statistics, the implementation makes use of tableone 120 and can subsequently be plotted as bar charts together with flow diagrams 121 that visualize the order and reasoning of filtering operations.
Basic pre-processing and quality control
ehrapy encompasses a suite of functionalities for fundamental data processing that are adopted from scanpy 52 but adapted to EHR data:
Regress out: To address unwanted sources of variation, a regression procedure is integrated, enhancing the dataset’s robustness.
Subsample: Selects a specified fraction of observations.
Balanced sample: Balances groups in the dataset by random oversampling or undersampling.
Highly variable features: The identification and annotation of highly variable features following the ‘highly variable genes’ function of scanpy is seamlessly incorporated, providing users with insights into pivotal elements influencing the dataset.
To identify and minimize quality issues, ehrapy provides several quality control functions:
Basic quality control: Determines the relative and absolute number of missing values per feature and per patient.
Winsorization: For data refinement, ehrapy implements a winsorization process, creating a version of the input array less susceptible to extreme values.
Feature clipping: Imposes limits on features to enhance dataset reliability.
Detect biases: Computes pairwise correlations between features, standardized mean differences for numeric features between groups of sensitive features, categorical feature value count differences between groups of sensitive features and feature importances when predicting a target variable.
Little’s MCAR test: Applies Little’s MCAR test whose null hypothesis is that data are MCAR. Rejecting the null hypothesis may not always mean that data are not MCAR, nor is accepting the null hypothesis a guarantee that data are MCAR. For more details, see Schouten et al. 122 .
Summarize features: Calculates statistical indicators per feature, including minimum, maximum and average values. This can be especially useful to reduce complex data with multiple measurements per feature per patient into sets of columns with single values.
Imputation is crucial in data analysis to address missing values, ensuring the completeness of datasets that can be required for specific algorithms. The ‘ehrapy’ pre-processing module offers a range of imputation techniques:
Explicit Impute: Replaces missing values, in either all columns or a user-specified subset, with a designated replacement value.
Simple Impute: Imputes missing values in numerical data using mean, median or the most frequent value, contributing to a more complete dataset.
KNN Impute: Uses k -nearest neighbor imputation to fill in missing values in the input AnnData object, preserving local data patterns.
MissForest Impute: Implements the MissForest strategy for imputing missing data, providing a robust approach for handling complex datasets.
MICE Impute: Applies the MICE algorithm for imputing data. This implementation is based on the miceforest ( https://github.com/AnotherSamWilson/miceforest ) package.
Data encoding can be required if categoricals are a part of the dataset to obtain numerical values only. Most algorithms in ehrapy are compatible only with numerical values. ehrapy offers two encoding algorithms based on scikit-learn 53 :
One-Hot Encoding: Transforms categorical variables into binary vectors, creating a binary feature for each category and capturing the presence or absence of each category in a concise representation.
Label Encoding: Assigns a unique numerical label to each category, facilitating the representation of categorical data as ordinal values and supporting algorithms that require numerical input.
To ensure that the distributions of the heterogeneous data are aligned, ehrapy offers several normalization procedures:
Log Normalization: Applies the natural logarithm function to the data, useful for handling skewed distributions and reducing the impact of outliers.
Max-Abs Normalization: Scales each feature by its maximum absolute value, ensuring that the maximum absolute value for each feature is 1.
Min-Max Normalization: Transforms the data to a specific range (commonly (0, 1)) by scaling each feature based on its minimum and maximum values.
Power Transformation Normalization: Applies a power transformation to make the data more Gaussian like, often useful for stabilizing variance and improving the performance of models sensitive to distributional assumptions.
Quantile Normalization: Aligns the distributions of multiple variables, ensuring that their quantiles match, which can be beneficial for comparing datasets or removing batch effects.
Robust Scaling Normalization: Scales data using the interquartile range, making it robust to outliers and suitable for datasets with extreme values.
Scaling Normalization: Standardizes data by subtracting the mean and dividing by the standard deviation, creating a distribution with a mean of 0 and a standard deviation of 1.
Offset to Positive Values: Shifts all values by a constant offset to make all values non-negative, with the lowest negative value becoming 0.
Dataset shifts can be corrected using the scanpy implementation of the ComBat 123 algorithm, which employs a parametric and non-parametric empirical Bayes framework for adjusting data for batch effects that is robust to outliers.
Finally, a neighbors graph can be efficiently computed using scanpy’s implementation.
To obtain meaningful lower-dimensional embeddings that can subsequently be visualized and reused for downstream algorithms, ehrapy provides the following algorithms based on scanpy’s implementation:
t-SNE: Uses a probabilistic approach to embed high-dimensional data into a lower-dimensional space, emphasizing the preservation of local similarities and revealing clusters in the data.
UMAP: Embeds data points by modeling their local neighborhood relationships, offering an efficient and scalable technique that captures both global and local structures in high-dimensional data.
Force-Directed Graph Drawing: Uses a physical simulation to position nodes in a graph, with edges representing pairwise relationships, creating a visually meaningful representation that emphasizes connectedness and clustering in the data.
Diffusion Maps: Applies spectral methods to capture the intrinsic geometry of high-dimensional data by modeling diffusion processes, providing a way to uncover underlying structures and patterns.
Density Calculation in Embedding: Quantifies the density of observations within an embedding, considering conditions or groups, offering insights into the concentration of data points in different regions and aiding in the identification of densely populated areas.
ehrapy further provides algorithms for clustering and trajectory inference based on scanpy:
Leiden Clustering: Uses the Leiden algorithm to cluster observations into groups, revealing distinct communities within the dataset with an emphasis on intra-cluster cohesion.
Hierarchical Clustering Dendrogram: Constructs a dendrogram through hierarchical clustering based on specified group by categories, illustrating the hierarchical relationships among observations and facilitating the exploration of structured patterns.
Feature ranking
ehrapy provides two ways of ranking feature contributions to clusters and target variables:
Statistical tests: To compare any obtained clusters to obtain marker features that are significantly different between the groups, ehrapy extends scanpy’s ‘rank genes groups’. The original implementation, which features a t -test for numerical data, is complemented by a g -test for categorical data.
Feature importance: Calculates feature rankings for a target variable using linear regression, support vector machine or random forest models from scikit-learn. ehrapy evaluates the relative importance of each predictor by fitting the model and extracting model-specific metrics, such as coefficients or feature importances.
Dataset integration
Based on scanpy’s ‘ingest’ function, ehrapy facilitates the integration of labels and embeddings from a well-annotated reference dataset into a new dataset, enabling the mapping of cluster annotations and spatial relationships for consistent comparative analysis. This process ensures harmonized clinical interpretations across datasets, especially useful when dealing with multiple experimental diseases or batches.
Knowledge inference
Survival analysis.
ehrapy’s implementation of survival analysis algorithms is based on lifelines 124 :
Ordinary Least Squares (OLS) Model: Creates a linear regression model using OLS from a specified formula and an AnnData object, allowing for the analysis of relationships between variables and observations.
Generalized Linear Model (GLM): Constructs a GLM from a given formula, distribution and AnnData, providing a versatile framework for modeling relationships with nonlinear data structures.
Kaplan–Meier: Fits the Kaplan–Meier curve to generate survival curves, offering a visual representation of the probability of survival over time in a dataset.
Cox Hazard Model: Constructs a Cox proportional hazards model using a specified formula and an AnnData object, enabling the analysis of survival data by modeling the hazard rates and their relationship to predictor variables.
Log-Rank Test: Calculates the P value for the log-rank test, comparing the survival functions of two groups, providing statistical significance for differences in survival distributions.
GLM Comparison: Given two fit GLMs, where the larger encompasses the parameter space of the smaller, this function returns the P value, indicating the significance of the larger model and adding explanatory power beyond the smaller model.
Trajectory inference
Trajectory inference is a computational approach that reconstructs and models the developmental paths and transitions within heterogeneous clinical data, providing insights into the temporal progression underlying complex systems. ehrapy offers several inbuilt algorithms for trajectory inference based on scanpy:
Diffusion Pseudotime: Infers the progression of observations by measuring geodesic distance along the graph, providing a pseudotime metric that represents the developmental trajectory within the dataset.
Partition-based Graph Abstraction (PAGA): Maps out the coarse-grained connectivity structures of complex manifolds using a partition-based approach, offering a comprehensive visualization of relationships in high-dimensional data and aiding in the identification of macroscopic connectivity patterns.
Because ehrapy is compatible with scverse, further trajectory inference-based algorithms, such as CellRank, can be seamlessly applied.
Causal inference
ehrapy’s causal inference module is based on ‘dowhy’ 69 . It is based on four key steps that are all implemented in ehrapy:
Graphical Model Specification: Define a causal graphical model representing relationships between variables and potential causal effects.
Causal Effect Identification: Automatically identify whether a causal effect can be inferred from the given data, addressing confounding and selection bias.
Causal Effect Estimation: Employ automated tools to estimate causal effects, using methods such as matching, instrumental variables or regression.
Sensitivity Analysis and Testing: Perform sensitivity analysis to assess the robustness of causal inferences and conduct statistical testing to determine the significance of the estimated causal effects.
Patient stratification
ehrapy’s complete pipeline from pre-processing to the generation of lower-dimensional embeddings, clustering, statistical comparison between determined groups and more facilitates the stratification of patients.
Visualization
ehrapy features an extensive visualization pipeline that is customizable and yet offers reasonable defaults. Almost every analysis function is matched with at least one visualization function that often shares the name but is available through the plotting module. For example, after importing ehrapy as ‘ep’, ‘ep.tl.umap(adata)’ runs the UMAP algorithm on an AnnData object, and ‘ep.pl.umap(adata)’ would then plot a scatter plot of the UMAP embedding.
ehrapy further offers a suite of more generally usable and modifiable plots:
Scatter Plot: Visualizes data points along observation or variable axes, offering insights into the distribution and relationships between individual data points.
Heatmap: Represents feature values in a grid, providing a comprehensive overview of the data’s structure and patterns.
Dot Plot: Displays count values of specified variables as dots, offering a clear depiction of the distribution of counts for each variable.
Filled Line Plot: Illustrates trends in data with filled lines, emphasizing variations in values over a specified axis.
Violin Plot: Presents the distribution of data through mirrored density plots, offering a concise view of the data’s spread.
Stacked Violin Plot: Combines multiple violin plots, stacked to allow for visual comparison of distributions across categories.
Group Mean Heatmap: Creates a heatmap displaying the mean count per group for each specified variable, providing insights into group-wise trends.
Hierarchically Clustered Heatmap: Uses hierarchical clustering to arrange data in a heatmap, revealing relationships and patterns among variables and observations.
Rankings Plot: Visualizes rankings within the data, offering a clear representation of the order and magnitude of values.
Dendrogram Plot: Plots a dendrogram of categories defined in a group by operation, illustrating hierarchical relationships within the dataset.
Benchmarking ehrapy
We generated a subset of the UKB data selecting 261 features and 488,170 patient visits. We removed all features with missingness rates greater than 70%. To demonstrate speed and memory consumption for various scenarios, we subsampled the data to 20%, 30% and 50%. We ran a minimal ehrapy analysis pipeline on each of those subsets and the full data, including the calculation of quality control metrics, filtering of variables by a missingness threshold, nearest neighbor imputation, normalization, dimensionality reduction and clustering (Supplementary Table 1 ). We conducted our benchmark on a single CPU with eight threads and 60 GB of maximum memory.
ehrapy further provides out-of-core implementations using Dask 108 for many algorithms in ehrapy, such as our normalization functions or our PCA implementation. Out-of-core computation refers to techniques that process data that do not fit entirely in memory, using disk storage to manage data overflow. This approach is crucial for handling large datasets without being constrained by system memory limits. Because the principal components get reused for other computationally expensive algorithms, such as the neighbors graph calculation, it effectively enables the analysis of very large datasets. We are currently working on supporting out-of-core computation for all computationally expensive algorithms in ehrapy.
We demonstrate the memory benefits in a hosted tutorial where the in-memory pipeline for 50,000 patients with 1,000 features required about 2 GB of memory, and the corresponding out-of-core implementation required less than 200 MB of memory.
The code for benchmarking is available at https://github.com/theislab/ehrapy-reproducibility . The implementation of ehrapy is accessible at https://github.com/theislab/ehrapy together with extensive API documentation and tutorials at https://ehrapy.readthedocs.io .
PIC database analysis
Study design.
We collected clinical data from the PIC 43 version 1.1.0 database. PIC is a single-center, bilingual (English and Chinese) database hosting information of children admitted to critical care units at the Children’s Hospital of Zhejiang University School of Medicine in China. The requirement for individual patient consent was waived because the study did not impact clinical care, and all protected health information was de-identified. The database contains 13,499 distinct hospital admissions of 12,881 distinct pediatric patients. These patients were admitted to five ICU units with 119 total critical care beds—GICU, PICU, SICU, CICU and NICU—between 2010 and 2018. The mean age of the patients was 2.5 years, of whom 42.5% were female. The in-hospital mortality was 7.1%; the mean hospital stay was 17.6 d; the mean ICU stay was 9.3 d; and 468 (3.6%) patients were admitted multiple times. Demographics, diagnoses, doctors’ notes, laboratory and microbiology tests, prescriptions, fluid balances, vital signs and radiographics reports were collected from all patients. For more details, see the original publication of Zeng et al. 43 .
Study participants
Individuals older than 18 years were excluded from the study. We grouped the data into three distinct groups: ‘neonates’ (0–28 d of age; 2,968 patients), ‘infants’ (1–12 months of age; 4,876 patients) and ‘youths’ (13 months to 18 years of age; 6,097 patients). We primarily analyzed the ‘youths’ group with the discharge diagnosis ‘unspecified pneumonia’ (277 patients).
Data collection
The collected clinical data included demographics, laboratory and vital sign measurements, diagnoses, microbiology and medication information and mortality outcomes. The five-character English ICD-10 codes were used, whose values are based on the seven-character Chinese ICD-10 codes.
Dataset extraction and analysis
We downloaded the PIC database of version 1.1.0 from Physionet 1 to obtain 17 CSV tables. Using Pandas, we selected all information with more than 50% coverage rate, including demographics and laboratory and vital sign measurements (Fig. 2 ). To reduce the amount of noise, we calculated and added only the minimum, maximum and average of all measurements that had multiple values per patient. Examination reports were removed because they describe only diagnostics and not detailed findings. All further diagnoses and microbiology and medication information were included into the observations slot to ensure that the data were not used for the calculation of embeddings but were still available for the analysis. This ensured that any calculated embedding would not be divided into treated and untreated groups but, rather, solely based on phenotypic features. We imputed all missing data through k -nearest neighbors imputation ( k = 20) using the knn_impute function of ehrapy. Next, we log normalized the data with ehrapy using the log_norm function. Afterwards, we winsorized the data using ehrapy’s winsorize function to obtain 277 ICU visits ( n = 265 patients) with 572 features. Of those 572 features, 254 were stored in the matrix X and the remaining 318 in the ‘obs’ slot in the AnnData object. For clustering and visualization purposes, we calculated 50 principal components using ehrapy’s pca function. The obtained principal component representation was then used to calculate a nearest neighbors graph using the neighbors function of ehrapy. The nearest neighbors graph then served as the basis for a UMAP embedding calculation using ehrapy’s umap function.
We applied the community detection algorithm Leiden with resolution 0.6 on the nearest neighbor graph using ehrapy’s leiden function. The four obtained clusters served as input for two-sided t -tests for all numerical values and two-sided g -tests for all categorical values for all four clusters against the union of all three other clusters, respectively. This was conducted using ehrapy’s rank_feature_groups function, which also corrects P values for multiple testing with the Benjamini–Hochberg method 125 . We presented the four groups and the statistically significantly different features between the groups to two pediatricians who annotated the groups with labels.
Our determined groups can be confidently labeled owing to their distinct clinical profiles. Nevertheless, we could only take into account clinical features that were measured. Insightful features, such as lung function tests, are missing. Moreover, the feature representation of the time-series data is simplified, which can hide some nuances between the groups. Generally, deciding on a clustering resolution is difficult. However, more fine-grained clusters obtained via higher clustering resolutions may become too specific and not generalize well enough.
Kaplan–Meier survival analysis
We selected patients with up to 360 h of total stay for Kaplan–Meier survival analysis to ensure a sufficiently high number of participants. We proceeded with the AnnData object prepared as described in the ‘Patient stratification’ subsection to conduct Kaplan–Meier analysis among all four determined pneumonia groups using ehrapy’s kmf function. Significance was tested through ehrapy’s test_kmf_logrank function, which tests whether two Kaplan–Meier series are statistically significant, employing a chi-squared test statistic under the null hypothesis. Let h i (t) be the hazard ratio of group i at time t and c a constant that represents a proportional change in the hazard ratio between the two groups, then:
This implicitly uses the log-rank weights. An additional Kaplan–Meier analysis was conducted for all children jointly concerning the liver markers AST, ALT and GGT. To determine whether measurements were inside or outside the norm range, we used reference ranges (Supplementary Table 2 ). P values less than 0.05 were labeled significant.
Our Kaplan–Meier curve analysis depends on the groups being well defined and shares the same limitations as the patient stratification. Additionally, the analysis is sensitive to the reference table where we selected limits that generalize well for the age ranges, but, due to children of different ages being examined, they may not necessarily be perfectly accurate for all children.
Causal effect of mechanism of action on LOS
Although the dataset was not initially intended for investigating causal effects of interventions, we adapted it for this purpose by focusing on the LOS in the ICU, measured in months, as the outcome variable. This choice aligns with the clinical aim of stabilizing patients sufficiently for ICU discharge. We constructed a causal graph to explore how different drug administrations could potentially reduce the LOS. Based on consultations with clinicians, we included several biomarkers of liver damage (AST, ALT and GGT) and inflammation (CRP and PCT) in our model. Patient age was also considered a relevant variable.
Because several different medications act by the same mechanisms, we grouped specific medications by their drug classes This grouping was achieved by cross-referencing the drugs listed in the dataset with DrugBank release 5.1 (ref. 126 ), using Levenshtein distances for partial string matching. After manual verification, we extracted the corresponding DrugBank categories, counted the number of features per category and compiled a list of commonly prescribed medications, as advised by clinicians. This approach facilitated the modeling of the causal graph depicted in Fig. 4 , where an intervention is defined as the administration of at least one drug from a specified category.
Causal inference was then conducted with ehrapy’s ‘dowhy’ 69 -based causal inference module using the expert-curated causal graph. Medication groups were designated as causal interventions, and the LOS was the outcome of interest. Linear regression served as the estimation method for analyzing these causal effects. We excluded four patients from the analysis owing to their notably long hospital stays exceeding 90 d, which were deemed outliers. To validate the robustness of our causal estimates, we incorporated several refutation methods:
Placebo Treatment Refuter: This method involved replacing the treatment assignment with a placebo to test the effect of the treatment variable being null.
Random Common Cause: A randomly generated variable was added to the data to assess the sensitivity of the causal estimate to the inclusion of potential unmeasured confounders.
Data Subset Refuter: The stability of the causal estimate was tested across various random subsets of the data to ensure that the observed effects were not dependent on a specific subset.
Add Unobserved Common Cause: This approach tested the effect of an omitted variable by adding a theoretically relevant unobserved confounder to the model, evaluating how much an unmeasured variable could influence the causal relationship.
Dummy Outcome: Replaces the true outcome variable with a random variable. If the causal effect nullifies, it supports the validity of the original causal relationship, indicating that the outcome is not driven by random factors.
Bootstrap Validation: Employs bootstrapping to generate multiple samples from the dataset, testing the consistency of the causal effect across these samples.
The selection of these refuters addresses a broad spectrum of potential biases and model sensitivities, including unobserved confounders and data dependencies. This comprehensive approach ensures robust verification of the causal analysis. Each refuter provides an orthogonal perspective, targeting specific vulnerabilities in causal analysis, which strengthens the overall credibility of the findings.
UKB analysis
Study population.
We used information from the UKB cohort, which includes 502,164 study participants from the general UK population without enrichment for specific diseases. The study involved the enrollment of individuals between 2006 and 2010 across 22 different assessment centers throughout the United Kingdom. The tracking of participants is still ongoing. Within the UKB dataset, metabolomics, proteomics and retinal optical coherence tomography data are available for a subset of individuals without any enrichment for specific diseases. Additionally, EHRs, questionnaire responses and other physical measures are available for almost everyone in the study. Furthermore, a variety of genotype information is available for nearly the entire cohort, including whole-genome sequencing, whole-exome sequencing, genotyping array data as well as imputed genotypes from the genotyping array 44 . Because only the latter two are available for download, and are sufficient for polygenic risk score calculation as performed here, we used the imputed genotypes in the present study. Participants visited the assessment center up to four times for additional and repeat measurements and completed additional online follow-up questionnaires.
In the present study, we restricted the analyses to data obtained from the initial assessment, including the blood draw, for obtaining the metabolomics data and the retinal imaging as well as physical measures. This restricts the study population to 33,521 individuals for whom all of these modalities are available. We have a clear study start point for each individual with the date of their initial assessment center visit. The study population has a mean age of 57 years, is 54% female and is censored at age 69 years on average; 4.7% experienced an incident myocardial infarction; and 8.1% have prevalent type 2 diabetes. The study population comes from six of the 22 assessment centers due to the retinal imaging being performed only at those.
For the myocardial infarction endpoint definition, we relied on the first occurrence data available in the UKB, which compiles the first date that each diagnosis was recorded for a participant in a hospital in ICD-10 nomenclature. Subsequently, we mapped these data to phecodes and focused on phecode 404.1 for myocardial infarction.
The Framingham Risk Score was developed on data from 8,491 participants in the Framingham Heart Study to assess general cardiovascular risk 77 . It includes easily obtainable predictors and is, therefore, easily applicable in clinical practice, although newer and more specific risk scores exist and might be used more frequently. It includes age, sex, smoking behavior, blood pressure, total and low-density lipoprotein cholesterol as well as information on insulin, antihypertensive and cholesterol-lowering medications, all of which are routinely collected in the UKB and used in this study as the Framingham feature set.
The metabolomics data used in this study were obtained using proton NMR spectroscopy, a low-cost method with relatively low batch effects. It covers established clinical predictors, such as albumin and cholesterol, as well as a range of lipids, amino acids and carbohydrate-related metabolites.
The retinal optical coherence tomography–derived features were returned by researchers to the UKB 75 , 76 . They used the available scans and determined the macular volume, macular thickness, retinal pigment epithelium thickness, disc diameter, cup-to-disk ratio across different regions as well as the thickness between the inner nuclear layer and external limiting membrane, inner and outer photoreceptor segments and the retinal pigment epithelium across different regions. Furthermore, they determined a wide range of quality metrics for each scan, including the image quality score, minimum motion correlation and inner limiting membrane (ILM) indicator.
Data analysis
After exporting the data from the UKB, all timepoints were transformed into participant age entries. Only participants without prevalent myocardial infarction (relative to the first assessment center visit at which all data were collected) were included.
The data were pre-processed for retinal imaging and metabolomics subsets separately, to enable a clear analysis of missing data and allow for the k -nearest neighbors–based imputation ( k = 20) of missing values when less than 10% were missing for a given participant. Otherwise, participants were dropped from the analyses. The imputed genotypes and Framingham analyses were available for almost every participant and, therefore, not imputed. Individuals without them were, instead, dropped from the analyses. Because genetic risk modeling poses entirely different methodological and computational challenges, we applied a published polygenic risk score for coronary heart disease using 6.6 million variants 73 . This was computed using the plink2 score option on the imputed genotypes available in the UKB.
UMAP embeddings were computed using default parameters on the full feature sets with ehrapy’s umap function. For all analyses, the same time-to-event and event-indicator columns were used. The event indicator is a Boolean variable indicating whether a myocardial infarction was observed for a study participant. The time to event is defined as the timespan between the start of the study, in this case the date of the first assessment center visit. Otherwise, it is the timespan from the start of the study to the start of censoring; in this case, this is set to the last date for which EHRs were available, unless a participant died, in which case the date of death is the start of censoring. Kaplan–Meier curves and Cox proportional hazards models were fit using ehrapy’s survival analysis module and the lifelines 124 package’s Cox-PHFitter function with default parameters. For Cox proportional hazards models with multiple feature sets, individually imputed and quality-controlled feature sets were concatenated, and the model was fit on the resulting matrix. Models were evaluated using the C-index 127 as a metric. It can be seen as an extension of the common area under the receiver operator characteristic score to time-to-event datasets, in which events are not observed for every sample and which ranges from 0.0 (entirely false) over 0.5 (random) to 1.0 (entirely correct). CIs for the C-index were computed based on bootstrapping by sampling 1,000 times with replacement from all computed partial hazards and computing the C-index over each of these samples. The percentiles at 2.5% and 97.5% then give the upper and lower confidence bound for the 95% CIs.
In all UKB analyses, the unit of study for a statistical test or predictive model is always an individual study participant.
The generalizability of the analysis is limited as the UK Biobank cohort may not represent the general population, with potential selection biases and underrepresentation of the different demographic groups. Additionally, by restricting analysis to initial assessment data and censoring based on the last available EHR or date of death, our analysis does not account for longitudinal changes and can introduce follow-up bias, especially if participants lost to follow-up have different risk profiles.
In-depth quality control of retina-derived features
A UMAP plot of the retina-derived features indicating the assessment centers shows a cluster of samples that lie somewhat outside the general population and mostly attended the Birmingham assessment center (Fig. 5b ). To further investigate this, we performed Leiden clustering of resolution 0.3 (Extended Data Fig. 9a ) and isolated this group in cluster 5. When comparing cluster 5 to the rest of the population in the retina-derived feature space, we noticed that many individuals in cluster 5 showed overall retinal pigment epithelium (RPE) thickness measures substantially elevated over the rest of the population in both eyes (Extended Data Fig. 9b ), which is mostly a feature of this cluster (Extended Data Fig. 9c ). To investigate potential confounding, we computed ratios between cluster 5 and the rest of the population over the ‘obs’ DataFrame containing the Framingham features, diabetes-related phecodes and genetic principal components. Out of the top and bottom five highest ratios observed, six are in genetic principal components, which are commonly used to represent genetic ancestry in a continuous space (Extended Data Fig. 9d ). Additionally, diagnoses for type 1 and type 2 diabetes and antihypertensive use are enriched in cluster 5. Further investigating the ancestry, we computed log ratios for self-reported ancestries and absolute counts, which showed no robust enrichment and depletion effects.
A closer look at three quality control measures of the imaging pipeline revealed that cluster 5 was an outlier in terms of either image quality (Extended Data Fig. 9e ) or minimum motion correlation (Extended Data Fig. 9f ) and the ILM indicator (Extended Data Fig. 9g ), all of which can be indicative of artifacts in image acquisition and downstream processing 128 . Subsequently, we excluded 301 individuals from cluster 5 from all analyses.

COVID-19 chest-x-ray fate determination
Dataset overview.
We used the public BrixIA COVID-19 dataset, which contains 192 chest x-ray images annotated with BrixIA scores 82 . Hereby, six regions were annotated by a senior radiologist with more than 20 years of experience and a junior radiologist with a disease severity score ranging from 0 to 3. A global score was determined as the sum of all of these regions and, therefore, ranges from 0 to 18 (S-Global). S-Global scores of 0 were classified as normal. Images that only had severity values up to 1 in all six regions were classified as mild. Images with severity values greater than or equal to 2, but a S-Global score of less than 7, were classified as moderate. All images that contained at least one 3 in any of the six regions with a S-Global score between 7 and 10 were classified as severe, and all remaining images with S-Global scores greater than 10 with at least one 3 were labeled critical. The dataset and instructions to download the images can be found at https://github.com/ieee8023/covid-chestxray-dataset .
We first resized all images to 224 × 224. Afterwards, the images underwent a random affine transformation that involved rotation, translation and scaling. The rotation angle was randomly selected from a range of −45° to 45°. The images were also subject to horizontal and vertical translation, with the maximum translation being 15% of the image size in either direction. Additionally, the images were scaled by a factor ranging from 0.85 to 1.15. The purpose of applying these transformations was to enhance the dataset and introduce variations, ultimately improving the robustness and generalization of the model.
To generate embeddings, we used a pre-trained DenseNet model with weights densenet121-res224-all of TorchXRayVision 129 . A DenseNet is a convolutional neural network that makes use of dense connections between layers (Dense Blocks) where all layers (with matching feature map sizes) directly connect with each other. To maintain a feed-forward nature, every layer in the DenseNet architecture receives supplementary inputs from all preceding layers and transmits its own feature maps to all subsequent layers. The model was trained on the nih-pc- chex-mimic_ch-google-openi-rsna dataset 130 .
Next, we calculated 50 principal components on the feature representation of the DenseNet model of all images using ehrapy’s pca function. The principal component representation served as input for a nearest neighbors graph calculation using ehrapy’s neighbors function. This graph served as the basis for the calculation of a UMAP embedding with three components that was finally visualized using ehrapy.
We randomly picked a root in the group of images that was labeled ‘Normal’. First, we calculated so-called pseudotime by fitting a trajectory through the calculated UMAP space using diffusion maps as implemented in ehrapy’s dpt function 57 . Each image’s pseudotime value represents its estimated position along this trajectory, serving as a proxy for its severity stage relative to others in the dataset. To determine fates, we employed CellRank 58 , 59 with the PseudotimeKernel . This kernel computes transition probabilities for patient visits based on the connectivity of the k -nearest neighbors graph and the pseudotime values of patient visits, which resembles their progression through a process. Directionality is infused in the nearest neighbors graph in this process where the kernel either removes or downweights edges in the graph that contradict the directional flow of increasing pseudotime, thereby refining the graph to better reflect the developmental trajectory. We computed the transition matrix with a soft threshold scheme (Parameter of the PseudotimeKernel ), which downweights edges that point against the direction of increasing pseudotime. Finally, we calculated a projection on top of the UMAP embedding with CellRank using the plot_projection function of the PseudotimeKernel that we subsequently plotted.
This analysis is limited by the small dataset of 192 chest x-ray images, which may affect the model’s generalizability and robustness. Annotation subjectivity from radiologists can further introduce variability in severity scores. Additionally, the random selection of a root from ‘Normal’ images can introduce bias in pseudotime calculations and subsequent analyses.
Diabetes 130-US hospitals analysis
We used data from the Diabetes 130-US hospitals dataset that were collected between 1999 and 2008. It contains clinical care information at 130 hospitals and integrated delivery networks. The extracted database information pertains to hospital admissions specifically for patients diagnosed with diabetes. These encounters required a hospital stay ranging from 1 d to 14 d, during which both laboratory tests and medications were administered. The selection criteria focused exclusively on inpatient encounters with these defined characteristics. More specifically, we used a version that was curated by the Fairlearn team where the target variable ‘readmitted’ was binarized and a few features renamed or binned ( https://fairlearn.org/main/user_guide/datasets/diabetes_hospital_data.html ). The dataset contains 101,877 patient visits and 25 features. The dataset predominantly consists of White patients (74.8%), followed by African Americans (18.9%), with other racial groups, such as Hispanic, Asian and Unknown categories, comprising smaller percentages. Females make up a slight majority in the data at 53.8%, with males accounting for 46.2% and a negligible number of entries listed as unknown or invalid. A substantial majority of the patients are over 60 years of age (67.4%), whereas those aged 30–60 years represent 30.2%, and those 30 years or younger constitute just 2.5%.
All of the following descriptions start by loading the Fairlearn version of the Diabetes 130-US hospitals dataset using ehrapy’s dataloader as an AnnData object.
Selection and filtering bias
An overview of sensitive variables was generated using tableone. Subsequently, ehrapy’s CohortTracker was used to track the age, gender and race variables. The cohort was filtered for all Medicare recipients and subsequently plotted.
Surveillance bias
We plotted the HbA1c measurement ratios using ehrapy’s catplot .
Missing data and imputation bias
MCAR-type missing data for the number of medications variable (‘num_medications‘) were introduced by randomly setting 30% of the variables to be missing using Numpy’s choice function. We tested that the data are MCAR by applying ehrapy’s implementation of Little’s MCAR test, which returned a non-significant P value of 0.71. MAR data for the number of medications variable (‘num_medications‘) were introduced by scaling the ‘time_in_hospital’ variable to have a mean of 0 and a standard deviation of 1, adjusting these values by multiplying by 1.2 and subtracting 0.6 to influence overall missingness rate, and then using these values to generate MAR data in the ‘num_medications’ variable via a logistic transformation and binomial sampling. We verified that the newly introduced missing values are not MCAR with respect to the ‘time_in_hospital’ variable by applying ehrapy’s implementation of Little’s test, which was significant (0.01 × 10 −2 ). The missing data were imputed using ehrapy’s mean imputation and MissForest implementation.
Algorithmic bias
Variables ‘race’, ‘gender’, ‘age’, ‘readmitted’, ‘readmit_binary’ and ‘discharge_disposition_id’ were moved to the ‘obs’ slot of the AnnData object to ensure that they were not used for model training. We built a binary label ‘readmit_30_days’ indicating whether a patient had been readmitted in fewer than 30 d. Next, we combined the ‘Asian’ and ‘Hispanic’ categories into a single ‘Other’ category within the ‘race’ column of our AnnData object and then filtered out and discarded any samples labeled as ‘Unknown/Invalid’ under the ‘gender‘ column and subsequently moved the ‘gender’ data to the variable matrix X of the AnnData object. All categorical variables got encoded. The data were split into train and test groups with a test size of 50%. The data were scaled, and a logistic regression model was trained using scikit-learn, which was also used to determine the balanced accuracy score. Fairlearn’s MetricFrame function was used to inspect the target model performance against the sensitive variable ‘race’. We subsequently fit Fairlearn’s ThresholdOptimizer using the logistic regression estimator with balanced_accuracy_score as the target object. The algorithmic demonstration of Fairlearn’s abilities on this dataset is shown here: https://github.com/fairlearn/talks/tree/main/2021_scipy_tutorial .
Normalization bias
We one-hot encoded all categorical variables with ehrapy using the encode function. We applied ehrapy’s implementation of scaling normalization with and without the ‘Age group’ variable as group key to scale the data jointly and separately using ehrapy’s scale_norm function.
Reporting summary
Further information on research design is available in the Nature Portfolio Reporting Summary linked to this article.
Data availability
Physionet provides access to the PIC database 43 at https://physionet.org/content/picdb/1.1.0 for credentialed users. The BrixIA images 82 are available at https://github.com/BrixIA/Brixia-score-COVID-19 . The data used in this study were obtained from the UK Biobank 44 ( https://www.ukbiobank.ac.uk/ ). Access to the UK Biobank resource was granted under application number 49966. The data are available to researchers upon application to the UK Biobank in accordance with their data access policies and procedures. The Diabetes 130-US Hospitals dataset is available at https://archive.ics.uci.edu/dataset/296/diabetes+130-us+hospitals+for+years+1999-2008 .
Code availability
The ehrapy source code is available at https://github.com/theislab/ehrapy under an Apache 2.0 license. Further documentation, tutorials and examples are available at https://ehrapy.readthedocs.io . We are actively developing the software and invite contributions from the community.
Jupyter notebooks to reproduce our analysis and figures, including Conda environments that specify all versions, are available at https://github.com/theislab/ehrapy-reproducibility .
Goldberger, A. L. et al. PhysioBank, PhysioToolkit, and PhysioNet: components of a new research resource for complex physiologic signals. Circulation 101 , E215–E220 (2000).
Article CAS PubMed Google Scholar
Atasoy, H., Greenwood, B. N. & McCullough, J. S. The digitization of patient care: a review of the effects of electronic health records on health care quality and utilization. Annu. Rev. Public Health 40 , 487–500 (2019).
Article PubMed Google Scholar
Jamoom, E. W., Patel, V., Furukawa, M. F. & King, J. EHR adopters vs. non-adopters: impacts of, barriers to, and federal initiatives for EHR adoption. Health (Amst.) 2 , 33–39 (2014).
Google Scholar
Rajkomar, A. et al. Scalable and accurate deep learning with electronic health records. NPJ Digit. Med. 1 , 18 (2018).
Article PubMed PubMed Central Google Scholar
Wolf, A. et al. Data resource profile: Clinical Practice Research Datalink (CPRD) Aurum. Int. J. Epidemiol. 48 , 1740–1740g (2019).
Sudlow, C. et al. UK biobank: an open access resource for identifying the causes of a wide range of complex diseases of middle and old age. PLoS Med. 12 , e1001779 (2015).
Pollard, T. J. et al. The eICU Collaborative Research Database, a freely available multi-center database for critical care research. Sci. Data 5 , 180178 (2018).
Johnson, A. E. W. et al. MIMIC-III, a freely accessible critical care database. Sci. Data 3 , 160035 (2016).
Article CAS PubMed PubMed Central Google Scholar
Hyland, S. L. et al. Early prediction of circulatory failure in the intensive care unit using machine learning. Nat. Med. 26 , 364–373 (2020).
Rasmy, L. et al. Recurrent neural network models (CovRNN) for predicting outcomes of patients with COVID-19 on admission to hospital: model development and validation using electronic health record data. Lancet Digit. Health 4 , e415–e425 (2022).
Marcus, J. L. et al. Use of electronic health record data and machine learning to identify candidates for HIV pre-exposure prophylaxis: a modelling study. Lancet HIV 6 , e688–e695 (2019).
Kruse, C. S., Stein, A., Thomas, H. & Kaur, H. The use of electronic health records to support population health: a systematic review of the literature. J. Med. Syst. 42 , 214 (2018).
Sheikh, A., Jha, A., Cresswell, K., Greaves, F. & Bates, D. W. Adoption of electronic health records in UK hospitals: lessons from the USA. Lancet 384 , 8–9 (2014).
Sheikh, A. et al. Health information technology and digital innovation for national learning health and care systems. Lancet Digit. Health 3 , e383–e396 (2021).
Cord, K. A. M., Mc Cord, K. A. & Hemkens, L. G. Using electronic health records for clinical trials: where do we stand and where can we go? Can. Med. Assoc. J. 191 , E128–E133 (2019).
Article Google Scholar
Landi, I. et al. Deep representation learning of electronic health records to unlock patient stratification at scale. NPJ Digit. Med. 3 , 96 (2020).
Ayaz, M., Pasha, M. F., Alzahrani, M. Y., Budiarto, R. & Stiawan, D. The Fast Health Interoperability Resources (FHIR) standard: systematic literature review of implementations, applications, challenges and opportunities. JMIR Med. Inform. 9 , e21929 (2021).
Peskoe, S. B. et al. Adjusting for selection bias due to missing data in electronic health records-based research. Stat. Methods Med. Res. 30 , 2221–2238 (2021).
Haneuse, S. & Daniels, M. A general framework for considering selection bias in EHR-based studies: what data are observed and why? EGEMS (Wash. DC) 4 , 1203 (2016).
PubMed Google Scholar
Gallifant, J. et al. Disparity dashboards: an evaluation of the literature and framework for health equity improvement. Lancet Digit. Health 5 , e831–e839 (2023).
Sauer, C. M. et al. Leveraging electronic health records for data science: common pitfalls and how to avoid them. Lancet Digit. Health 4 , e893–e898 (2022).
Li, J. et al. Imputation of missing values for electronic health record laboratory data. NPJ Digit. Med. 4 , 147 (2021).
Rubin, D. B. Inference and missing data. Biometrika 63 , 581 (1976).
Scheid, L. M., Brown, L. S., Clark, C. & Rosenfeld, C. R. Data electronically extracted from the electronic health record require validation. J. Perinatol. 39 , 468–474 (2019).
Phelan, M., Bhavsar, N. A. & Goldstein, B. A. Illustrating informed presence bias in electronic health records data: how patient interactions with a health system can impact inference. EGEMS (Wash. DC). 5 , 22 (2017).
PubMed PubMed Central Google Scholar
Secondary Analysis of Electronic Health Records (ed MIT Critical Data) (Springer, 2016).
Jetley, G. & Zhang, H. Electronic health records in IS research: quality issues, essential thresholds and remedial actions. Decis. Support Syst. 126 , 113137 (2019).
McCormack, J. P. & Holmes, D. T. Your results may vary: the imprecision of medical measurements. BMJ 368 , m149 (2020).
Hobbs, F. D. et al. Is the international normalised ratio (INR) reliable? A trial of comparative measurements in hospital laboratory and primary care settings. J. Clin. Pathol. 52 , 494–497 (1999).
Huguet, N. et al. Using electronic health records in longitudinal studies: estimating patient attrition. Med. Care 58 Suppl 6 Suppl 1 , S46–S52 (2020).
Zeng, J., Gensheimer, M. F., Rubin, D. L., Athey, S. & Shachter, R. D. Uncovering interpretable potential confounders in electronic medical records. Nat. Commun. 13 , 1014 (2022).
Getzen, E., Ungar, L., Mowery, D., Jiang, X. & Long, Q. Mining for equitable health: assessing the impact of missing data in electronic health records. J. Biomed. Inform. 139 , 104269 (2023).
Tang, S. et al. Democratizing EHR analyses with FIDDLE: a flexible data-driven preprocessing pipeline for structured clinical data. J. Am. Med. Inform. Assoc. 27 , 1921–1934 (2020).
Dagliati, A. et al. A process mining pipeline to characterize COVID-19 patients’ trajectories and identify relevant temporal phenotypes from EHR data. Front. Public Health 10 , 815674 (2022).
Sun, Y. & Zhou, Y.-H. A machine learning pipeline for mortality prediction in the ICU. Int. J. Digit. Health 2 , 3 (2022).
Article CAS Google Scholar
Mandyam, A., Yoo, E. C., Soules, J., Laudanski, K. & Engelhardt, B. E. COP-E-CAT: cleaning and organization pipeline for EHR computational and analytic tasks. In Proc. of the 12th ACM Conference on Bioinformatics, Computational Biology, and Health Informatics. https://doi.org/10.1145/3459930.3469536 (Association for Computing Machinery, 2021).
Gao, C. A. et al. A machine learning approach identifies unresolving secondary pneumonia as a contributor to mortality in patients with severe pneumonia, including COVID-19. J. Clin. Invest. 133 , e170682 (2023).
Makam, A. N. et al. The good, the bad and the early adopters: providers’ attitudes about a common, commercial EHR. J. Eval. Clin. Pract. 20 , 36–42 (2014).
Amezquita, R. A. et al. Orchestrating single-cell analysis with Bioconductor. Nat. Methods 17 , 137–145 (2020).
Virshup, I. et al. The scverse project provides a computational ecosystem for single-cell omics data analysis. Nat. Biotechnol. 41 , 604–606 (2023).
Zou, Q. et al. Predicting diabetes mellitus with machine learning techniques. Front. Genet. 9 , 515 (2018).
Cios, K. J. & William Moore, G. Uniqueness of medical data mining. Artif. Intell. Med. 26 , 1–24 (2002).
Zeng, X. et al. PIC, a paediatric-specific intensive care database. Sci. Data 7 , 14 (2020).
Bycroft, C. et al. The UK Biobank resource with deep phenotyping and genomic data. Nature 562 , 203–209 (2018).
Lee, J. et al. Open-access MIMIC-II database for intensive care research. Annu. Int. Conf. Proc. IEEE Eng. Med. Biol. Soc. 2011 , 8315–8318 (2011).
Virshup, I., Rybakov, S., Theis, F. J., Angerer, P. & Alexander Wolf, F. anndata: annotated data. Preprint at bioRxiv https://doi.org/10.1101/2021.12.16.473007 (2021).
Voss, E. A. et al. Feasibility and utility of applications of the common data model to multiple, disparate observational health databases. J. Am. Med. Inform. Assoc. 22 , 553–564 (2015).
Vasilevsky, N. A. et al. Mondo: unifying diseases for the world, by the world. Preprint at medRxiv https://doi.org/10.1101/2022.04.13.22273750 (2022).
Harrison, J. E., Weber, S., Jakob, R. & Chute, C. G. ICD-11: an international classification of diseases for the twenty-first century. BMC Med. Inform. Decis. Mak. 21 , 206 (2021).
Köhler, S. et al. Expansion of the Human Phenotype Ontology (HPO) knowledge base and resources. Nucleic Acids Res. 47 , D1018–D1027 (2019).
Wu, P. et al. Mapping ICD-10 and ICD-10-CM codes to phecodes: workflow development and initial evaluation. JMIR Med. Inform. 7 , e14325 (2019).
Wolf, F. A., Angerer, P. & Theis, F. J. SCANPY: large-scale single-cell gene expression data analysis. Genome Biol. 19 , 15 (2018).
Pedregosa, F. et al. Scikit-learn: machine learning in Python. J. Mach. Learn. Res . 12 , 2825–2830 (2011).
de Haan-Rietdijk, S., de Haan-Rietdijk, S., Kuppens, P. & Hamaker, E. L. What’s in a day? A guide to decomposing the variance in intensive longitudinal data. Front. Psychol. 7 , 891 (2016).
Pedersen, E. S. L., Danquah, I. H., Petersen, C. B. & Tolstrup, J. S. Intra-individual variability in day-to-day and month-to-month measurements of physical activity and sedentary behaviour at work and in leisure-time among Danish adults. BMC Public Health 16 , 1222 (2016).
Roffey, D. M., Byrne, N. M. & Hills, A. P. Day-to-day variance in measurement of resting metabolic rate using ventilated-hood and mouthpiece & nose-clip indirect calorimetry systems. JPEN J. Parenter. Enter. Nutr. 30 , 426–432 (2006).
Haghverdi, L., Büttner, M., Wolf, F. A., Buettner, F. & Theis, F. J. Diffusion pseudotime robustly reconstructs lineage branching. Nat. Methods 13 , 845–848 (2016).
Lange, M. et al. CellRank for directed single-cell fate mapping. Nat. Methods 19 , 159–170 (2022).
Weiler, P., Lange, M., Klein, M., Pe'er, D. & Theis, F. CellRank 2: unified fate mapping in multiview single-cell data. Nat. Methods 21 , 1196–1205 (2024).
Zhang, S. et al. Cost of management of severe pneumonia in young children: systematic analysis. J. Glob. Health 6 , 010408 (2016).
Torres, A. et al. Pneumonia. Nat. Rev. Dis. Prim. 7 , 25 (2021).
Traag, V. A., Waltman, L. & van Eck, N. J. From Louvain to Leiden: guaranteeing well-connected communities. Sci. Rep. 9 , 5233 (2019).
Kamin, W. et al. Liver involvement in acute respiratory infections in children and adolescents—results of a non-interventional study. Front. Pediatr. 10 , 840008 (2022).
Shi, T. et al. Risk factors for mortality from severe community-acquired pneumonia in hospitalized children transferred to the pediatric intensive care unit. Pediatr. Neonatol. 61 , 577–583 (2020).
Dudnyk, V. & Pasik, V. Liver dysfunction in children with community-acquired pneumonia: the role of infectious and inflammatory markers. J. Educ. Health Sport 11 , 169–181 (2021).
Charpignon, M.-L. et al. Causal inference in medical records and complementary systems pharmacology for metformin drug repurposing towards dementia. Nat. Commun. 13 , 7652 (2022).
Grief, S. N. & Loza, J. K. Guidelines for the evaluation and treatment of pneumonia. Prim. Care 45 , 485–503 (2018).
Paul, M. Corticosteroids for pneumonia. Cochrane Database Syst. Rev. 12 , CD007720 (2017).
Sharma, A. & Kiciman, E. DoWhy: an end-to-end library for causal inference. Preprint at arXiv https://doi.org/10.48550/ARXIV.2011.04216 (2020).
Khilnani, G. C. et al. Guidelines for antibiotic prescription in intensive care unit. Indian J. Crit. Care Med. 23 , S1–S63 (2019).
Harris, L. K. & Crannage, A. J. Corticosteroids in community-acquired pneumonia: a review of current literature. J. Pharm. Technol. 37 , 152–160 (2021).
Dou, L. et al. Decreased hospital length of stay with early administration of oseltamivir in patients hospitalized with influenza. Mayo Clin. Proc. Innov. Qual. Outcomes 4 , 176–182 (2020).
Khera, A. V. et al. Genome-wide polygenic scores for common diseases identify individuals with risk equivalent to monogenic mutations. Nat. Genet. 50 , 1219–1224 (2018).
Julkunen, H. et al. Atlas of plasma NMR biomarkers for health and disease in 118,461 individuals from the UK Biobank. Nat. Commun. 14 , 604 (2023).
Ko, F. et al. Associations with retinal pigment epithelium thickness measures in a large cohort: results from the UK Biobank. Ophthalmology 124 , 105–117 (2017).
Patel, P. J. et al. Spectral-domain optical coherence tomography imaging in 67 321 adults: associations with macular thickness in the UK Biobank study. Ophthalmology 123 , 829–840 (2016).
D’Agostino Sr, R. B. et al. General cardiovascular risk profile for use in primary care: the Framingham Heart Study. Circulation 117 , 743–753 (2008).
Buergel, T. et al. Metabolomic profiles predict individual multidisease outcomes. Nat. Med. 28 , 2309–2320 (2022).
Xu, Y. et al. An atlas of genetic scores to predict multi-omic traits. Nature 616 , 123–131 (2023).
Saelens, W., Cannoodt, R., Todorov, H. & Saeys, Y. A comparison of single-cell trajectory inference methods. Nat. Biotechnol. 37 , 547–554 (2019).
Rousan, L. A., Elobeid, E., Karrar, M. & Khader, Y. Chest x-ray findings and temporal lung changes in patients with COVID-19 pneumonia. BMC Pulm. Med. 20 , 245 (2020).
Signoroni, A. et al. BS-Net: learning COVID-19 pneumonia severity on a large chest X-ray dataset. Med. Image Anal. 71 , 102046 (2021).
Bird, S. et al. Fairlearn: a toolkit for assessing and improving fairness in AI. https://www.microsoft.com/en-us/research/publication/fairlearn-a-toolkit-for-assessing-and-improving-fairness-in-ai/ (2020).
Strack, B. et al. Impact of HbA1c measurement on hospital readmission rates: analysis of 70,000 clinical database patient records. BioMed. Res. Int. 2014 , 781670 (2014).
Stekhoven, D. J. & Bühlmann, P. MissForest—non-parametric missing value imputation for mixed-type data. Bioinformatics 28 , 112–118 (2012).
Banerjee, A. et al. Identifying subtypes of heart failure from three electronic health record sources with machine learning: an external, prognostic, and genetic validation study. Lancet Digit. Health 5 , e370–e379 (2023).
Nagamine, T. et al. Data-driven identification of heart failure disease states and progression pathways using electronic health records. Sci. Rep. 12 , 17871 (2022).
Da Silva Filho, J. et al. Disease trajectories in hospitalized COVID-19 patients are predicted by clinical and peripheral blood signatures representing distinct lung pathologies. Preprint at bioRxiv https://doi.org/10.1101/2023.09.08.23295024 (2023).
Haneuse, S., Arterburn, D. & Daniels, M. J. Assessing missing data assumptions in EHR-based studies: a complex and underappreciated task. JAMA Netw. Open 4 , e210184 (2021).
Little, R. J. A. A test of missing completely at random for multivariate data with missing values. J. Am. Stat. Assoc. 83 , 1198–1202 (1988).
Jakobsen, J. C., Gluud, C., Wetterslev, J. & Winkel, P. When and how should multiple imputation be used for handling missing data in randomised clinical trials—a practical guide with flowcharts. BMC Med. Res. Methodol. 17 , 162 (2017).
Dziura, J. D., Post, L. A., Zhao, Q., Fu, Z. & Peduzzi, P. Strategies for dealing with missing data in clinical trials: from design to analysis. Yale J. Biol. Med. 86 , 343–358 (2013).
White, I. R., Royston, P. & Wood, A. M. Multiple imputation using chained equations: issues and guidance for practice. Stat. Med. 30 , 377–399 (2011).
Jäger, S., Allhorn, A. & Bießmann, F. A benchmark for data imputation methods. Front. Big Data 4 , 693674 (2021).
Waljee, A. K. et al. Comparison of imputation methods for missing laboratory data in medicine. BMJ Open 3 , e002847 (2013).
Ibrahim, J. G. & Molenberghs, G. Missing data methods in longitudinal studies: a review. Test (Madr.) 18 , 1–43 (2009).
Li, C., Alsheikh, A. M., Robinson, K. A. & Lehmann, H. P. Use of recommended real-world methods for electronic health record data analysis has not improved over 10 years. Preprint at bioRxiv https://doi.org/10.1101/2023.06.21.23291706 (2023).
Regev, A. et al. The Human Cell Atlas. eLife 6 , e27041 (2017).
Megill, C. et al. cellxgene: a performant, scalable exploration platform for high dimensional sparse matrices. Preprint at bioRxiv https://doi.org/10.1101/2021.04.05.438318 (2021).
Speir, M. L. et al. UCSC Cell Browser: visualize your single-cell data. Bioinformatics 37 , 4578–4580 (2021).
Hunter, J. D. Matplotlib: a 2D graphics environment. Comput. Sci. Eng. 9 , 90–95 (2007).
Waskom, M. seaborn: statistical data visualization. J. Open Source Softw. 6 , 3021 (2021).
Harris, C. R. et al. Array programming with NumPy. Nature 585 , 357–362 (2020).
Lam, S. K., Pitrou, A. & Seibert, S. Numba: a LLVM-based Python JIT compiler. In Proc. of the Second Workshop on the LLVM Compiler Infrastructure in HPC. https://doi.org/10.1145/2833157.2833162 (Association for Computing Machinery, 2015).
Virtanen, P. et al. SciPy 1.0: fundamental algorithms for scientific computing in Python. Nat. Methods 17 , 261–272 (2020).
McKinney, W. Data structures for statistical computing in Python. In Proc. of the 9th Python in Science Conference (eds van der Walt, S. & Millman, J.). https://doi.org/10.25080/majora-92bf1922-00a (SciPy, 2010).
Boulanger, A. Open-source versus proprietary software: is one more reliable and secure than the other? IBM Syst. J. 44 , 239–248 (2005).
Rocklin, M. Dask: parallel computation with blocked algorithms and task scheduling. In Proc. of the 14th Python in Science Conference. https://doi.org/10.25080/majora-7b98e3ed-013 (SciPy, 2015).
Pivarski, J. et al. Awkward Array. https://doi.org/10.5281/ZENODO.4341376
Collette, A. Python and HDF5: Unlocking Scientific Data (‘O’Reilly Media, Inc., 2013).
Miles, A. et al. zarr-developers/zarr-python: v2.13.6. https://doi.org/10.5281/zenodo.7541518 (2023).
The pandas development team. pandas-dev/pandas: Pandas. https://doi.org/10.5281/ZENODO.3509134 (2024).
Weberpals, J. et al. Deep learning-based propensity scores for confounding control in comparative effectiveness research: a large-scale, real-world data study. Epidemiology 32 , 378–388 (2021).
Rosenthal, J. et al. Building tools for machine learning and artificial intelligence in cancer research: best practices and a case study with the PathML toolkit for computational pathology. Mol. Cancer Res. 20 , 202–206 (2022).
Gayoso, A. et al. A Python library for probabilistic analysis of single-cell omics data. Nat. Biotechnol. 40 , 163–166 (2022).
Paszke, A. et al. PyTorch: an imperative style, high-performance deep learning library. In Advances in Neural Information Processing Systems 32 (eds Wallach, H. et al.). 8024–8035 (Curran Associates, 2019).
Frostig, R., Johnson, M. & Leary, C. Compiling machine learning programs via high-level tracing. https://cs.stanford.edu/~rfrostig/pubs/jax-mlsys2018.pdf (2018).
Moor, M. et al. Foundation models for generalist medical artificial intelligence. Nature 616 , 259–265 (2023).
Kraljevic, Z. et al. Multi-domain clinical natural language processing with MedCAT: the Medical Concept Annotation Toolkit. Artif. Intell. Med. 117 , 102083 (2021).
Pollard, T. J., Johnson, A. E. W., Raffa, J. D. & Mark, R. G. An open source Python package for producing summary statistics for research papers. JAMIA Open 1 , 26–31 (2018).
Ellen, J. G. et al. Participant flow diagrams for health equity in AI. J. Biomed. Inform. 152 , 104631 (2024).
Schouten, R. M. & Vink, G. The dance of the mechanisms: how observed information influences the validity of missingness assumptions. Sociol. Methods Res. 50 , 1243–1258 (2021).
Johnson, W. E., Li, C. & Rabinovic, A. Adjusting batch effects in microarray expression data using empirical Bayes methods. Biostatistics 8 , 118–127 (2007).
Davidson-Pilon, C. lifelines: survival analysis in Python. J. Open Source Softw. 4 , 1317 (2019).
Benjamini, Y. & Hochberg, Y. Controlling the false discovery rate: a practical and powerful approach to multiple testing. J. R. Stat. Soc. Ser. B Stat. Methodol. 57 , 289–300 (1995).
Wishart, D. S. et al. DrugBank: a comprehensive resource for in silico drug discovery and exploration. Nucleic Acids Res. 34 , D668–D672 (2006).
Harrell, F. E. Jr, Califf, R. M., Pryor, D. B., Lee, K. L. & Rosati, R. A. Evaluating the yield of medical tests. JAMA 247 , 2543–2546 (1982).
Currant, H. et al. Genetic variation affects morphological retinal phenotypes extracted from UK Biobank optical coherence tomography images. PLoS Genet. 17 , e1009497 (2021).
Cohen, J. P. et al. TorchXRayVision: a library of chest X-ray datasets and models. In Proc. of the 5th International Conference on Medical Imaging with Deep Learning (eds Konukoglu, E. et al.). 172 , 231–249 (PMLR, 2022).
Cohen, J.P., Hashir, M., Brooks, R. & Bertrand, H. On the limits of cross-domain generalization in automated X-ray prediction. In Proceedings of Machine Learning Research , Vol. 121 (eds Arbel, T. et al.) 136–155 (PMLR, 2020).
Download references
Acknowledgements
We thank M. Ansari who designed the ehrapy logo. The authors thank F. A. Wolf, M. Lücken, J. Steinfeldt, B. Wild, G. Rätsch and D. Shung for feedback on the project. We further thank L. Halle, Y. Ji, M. Lücken and R. K. Rubens for constructive comments on the paper. We thank F. Hashemi for her help in implementing the survival analysis module. This research was conducted using data from the UK Biobank, a major biomedical database ( https://www.ukbiobank.ac.uk ), under application number 49966. This work was supported by the German Center for Lung Research (DZL), the Helmholtz Association and the CRC/TRR 359 Perinatal Development of Immune Cell Topology (PILOT). N.H. and F.J.T. acknowledge support from the German Federal Ministry of Education and Research (BMBF) (LODE, 031L0210A), co-funded by the European Union (ERC, DeepCell, 101054957). A.N. is supported by the Konrad Zuse School of Excellence in Learning and Intelligent Systems (ELIZA) through the DAAD program Konrad Zuse Schools of Excellence in Artificial Intelligence, sponsored by the Federal Ministry of Education and Research. This work was also supported by the Chan Zuckerberg Initiative (CZIF2022-007488; Human Cell Atlas Data Ecosystem).
Open access funding provided by Helmholtz Zentrum München - Deutsches Forschungszentrum für Gesundheit und Umwelt (GmbH).
Author information
Authors and affiliations.
Institute of Computational Biology, Helmholtz Munich, Munich, Germany
Lukas Heumos, Philipp Ehmele, Tim Treis, Eljas Roellin, Lilly May, Altana Namsaraeva, Nastassya Horlava, Vladimir A. Shitov, Xinyue Zhang, Luke Zappia, Leon Hetzel, Isaac Virshup, Lisa Sikkema, Fabiola Curion & Fabian J. Theis
Institute of Lung Health and Immunity and Comprehensive Pneumology Center with the CPC-M bioArchive; Helmholtz Zentrum Munich; member of the German Center for Lung Research (DZL), Munich, Germany
Lukas Heumos, Niklas J. Lang, Herbert B. Schiller & Anne Hilgendorff
TUM School of Life Sciences Weihenstephan, Technical University of Munich, Munich, Germany
Lukas Heumos, Tim Treis, Nastassya Horlava, Vladimir A. Shitov, Lisa Sikkema & Fabian J. Theis
Health Data Science Unit, Heidelberg University and BioQuant, Heidelberg, Germany
Julius Upmeier zu Belzen & Roland Eils
Department of Mathematics, School of Computation, Information and Technology, Technical University of Munich, Munich, Germany
Eljas Roellin, Lilly May, Luke Zappia, Leon Hetzel, Fabiola Curion & Fabian J. Theis
Konrad Zuse School of Excellence in Learning and Intelligent Systems (ELIZA), Darmstadt, Germany
Altana Namsaraeva
Systems Medicine, Deutsches Zentrum für Neurodegenerative Erkrankungen (DZNE), Bonn, Germany
Rainer Knoll
Center for Digital Health, Berlin Institute of Health (BIH) at Charité – Universitätsmedizin Berlin, Berlin, Germany
Roland Eils
Research Unit, Precision Regenerative Medicine (PRM), Helmholtz Munich, Munich, Germany
Herbert B. Schiller
Center for Comprehensive Developmental Care (CDeCLMU) at the Social Pediatric Center, Dr. von Hauner Children’s Hospital, LMU Hospital, Ludwig Maximilian University, Munich, Germany
Anne Hilgendorff
You can also search for this author in PubMed Google Scholar
Contributions
L. Heumos and F.J.T. conceived the study. L. Heumos, P.E., X.Z., E.R., L.M., A.N., L.Z., V.S., T.T., L. Hetzel, N.H., R.K. and I.V. implemented ehrapy. L. Heumos, P.E., N.L., L.S., T.T. and A.H. analyzed the PIC database. J.U.z.B. and L. Heumos analyzed the UK Biobank database. X.Z. and L. Heumos analyzed the COVID-19 chest x-ray dataset. L. Heumos, P.E. and J.U.z.B. wrote the paper. F.J.T., A.H., H.B.S. and R.E. supervised the work. All authors read, corrected and approved the final paper.
Corresponding author
Correspondence to Fabian J. Theis .
Ethics declarations
Competing interests.
L. Heumos is an employee of LaminLabs. F.J.T. consults for Immunai Inc., Singularity Bio B.V., CytoReason Ltd. and Omniscope Ltd. and has ownership interest in Dermagnostix GmbH and Cellarity. The remaining authors declare no competing interests.
Peer review
Peer review information.
Nature Medicine thanks Leo Anthony Celi and the other, anonymous, reviewer(s) for their contribution to the peer review of this work. Primary handling editor: Lorenzo Righetto, in collaboration with the Nature Medicine team.
Additional information
Publisher’s note Springer Nature remains neutral with regard to jurisdictional claims in published maps and institutional affiliations.
Extended data
Extended data fig. 1 overview of the paediatric intensive care database (pic)..
The database consists of several tables corresponding to several data modalities and measurement types. All tables colored in green were selected for analysis and all tables in blue were discarded based on coverage rate. Despite the high coverage rate, we discarded the ‘OR_EXAM_REPORTS’ table because of the lack of detail in the exam reports.
Extended Data Fig. 2 Preprocessing of the Paediatric Intensive Care (PIC) dataset with ehrapy.
( a ) Heterogeneous data of the PIC database was stored in ‘data’ (matrix that is used for computations) and ‘observations’ (metadata per patient visit). During quality control, further annotations are added to the ‘variables’ (metadata per feature) slot. ( b ) Preprocessing steps of the PIC dataset. ( c ) Example of the function calls in the data analysis pipeline that resembles the preprocessing steps in (B) using ehrapy.
Extended Data Fig. 3 Missing data distribution for the ‘youths’ group of the PIC dataset.
The x-axis represents the percentage of missing values in each feature. The y-axis reflects the number of features in each bin with text labels representing the names of the individual features.
Extended Data Fig. 4 Patient selection during analysis of the PIC dataset.
Filtering for the pneumonia cohort of the youths filters out care units except for the general intensive care unit and the pediatric intensive care unit.
Extended Data Fig. 5 Feature rankings of stratified patient groups.
Scores reflect the z-score underlying the p-value per measurement for each group. Higher scores (above 0) reflect overrepresentation of the measurement compared to all other groups and vice versa. ( a ) By clinical chemistry. ( b ) By liver markers. ( c ) By medication type. ( d ) By infection markers.
Extended Data Fig. 6 Liver marker value progression for the ‘youths’ group and Kaplan-Meier curves.
( a ) Viral and severe pneumonia with co-infection groups display enriched gamma-glutamyl transferase levels in blood serum. ( b ) Aspartate transferase (AST) and Alanine transaminase (ALT) levels are enriched for severe pneumonia with co-infection during early ICU stay. ( c ) and ( d ) Kaplan-Meier curves for ALT and AST demonstrate lower survivability for children with measurements outside the norm.
Extended Data Fig. 7 Overview of medication categories used for causal inference.
( a ) Feature engineering process to group administered medications into medication categories using drugbank. ( b ) Number of medications per medication category. ( c ) Number of patients that received (dark blue) and did not receive specific medication categories (light blue).
Extended Data Fig. 8 UK-Biobank data overview and quality control across modalities.
( a ) UMAP plot of the metabolomics data demonstrating a clear gradient with respect to age at sampling, and ( b ) type 2 diabetes prevalence. ( c ) Analogously, the features derived from retinal imaging show a less pronounced age gradient, and ( d ) type 2 diabetes prevalence gradient. ( e ) Stratifying myocardial infarction risk by the type 2 diabetes comorbidity confirms vastly increased risk with a prior type 2 (T2D) diabetes diagnosis. Kaplan-Meier estimators with 95 % confidence intervals are shown. ( f ) Similarly, the polygenic risk score for coronary heart disease used in this work substantially enriches myocardial infarction risk in its top 5% percentile. Kaplan-Meier estimators with 95 % confidence intervals are shown. ( g ) UMAP visualization of the metabolomics features colored by the assessment center shows no discernable biases. (A-G) n = 29,216.
Extended Data Fig. 9 UK-Biobank retina derived feature quality control.
( a ) Leiden Clustering of retina derived feature space. ( b ) Comparison of ‘overall retinal pigment epithelium (RPE) thickness’ values between cluster 5 (n = 301) and the rest of the population (n = 28,915). ( c ) RPE thickness in the right eye outliers on the UMAP largely corresponds to cluster 5. ( d ) Log ratio of top and bottom 5 fields in obs dataframe between cluster 5 and the rest of the population. ( e ) Image Quality of the optical coherence tomography scan as reported in the UKB. ( f ) Minimum motion correlation quality control indicator. ( g ) Inner limiting membrane (ILM) quality control indicator. (D-G) Data are shown for the right eye only, comparable results for the left eye are omitted. (A-G) n = 29,216.
Extended Data Fig. 10 Bias detection and mitigation study on the Diabetes 130-US hospitals dataset (n = 101,766 hospital visits, one patient can have multiple visits).
( a ) Filtering to the visits of Medicare recipients results in an increase of Caucasians. ( b ) Proportion of visits where Hb1Ac measurements are recorded, stratified by admission type. Adjusted P values were calculated with Chi squared tests and Bonferroni correction (Adjusted P values: Emergency vs Referral 3.3E-131, Emergency vs Other 1.4E-101, Referral vs Other 1.6E-4.) ( c ) Normalizing feature distributions jointly vs. separately can mask distribution differences. ( d ) Imputing the number of medications for visits. Onto the complete data (blue), MCAR (30% missing data) and MAR (38% missing data) were introduced (orange), with the MAR mechanism depending on the time in hospital. Mean imputation (green) can reduce the variance of the distribution under MCAR and MAR mechanisms, and bias the center of the distribution under an MAR mechanism. Multiple imputation, such as MissForest imputation can impute meaningfully even in MAR cases, when having access to variables involved in the MAR mechanism. Each boxplot represents the IQR of the data, with the horizontal line inside the box indicating the median value. The left and right bounds of the box represent the first and third quartiles, respectively. The ‘whiskers’ extend to the minimum and maximum values within 1.5 times the IQR from the lower and upper quartiles, respectively. ( e ) Predicting the early readmission within 30 days after release on a per-stay level. Balanced accuracy can mask differences in selection and false negative rate between sensitive groups.
Supplementary information
Supplementary tables 1 and 2, reporting summary, rights and permissions.
Open Access This article is licensed under a Creative Commons Attribution 4.0 International License, which permits use, sharing, adaptation, distribution and reproduction in any medium or format, as long as you give appropriate credit to the original author(s) and the source, provide a link to the Creative Commons licence, and indicate if changes were made. The images or other third party material in this article are included in the article’s Creative Commons licence, unless indicated otherwise in a credit line to the material. If material is not included in the article’s Creative Commons licence and your intended use is not permitted by statutory regulation or exceeds the permitted use, you will need to obtain permission directly from the copyright holder. To view a copy of this licence, visit http://creativecommons.org/licenses/by/4.0/ .
Reprints and permissions
About this article
Cite this article.
Heumos, L., Ehmele, P., Treis, T. et al. An open-source framework for end-to-end analysis of electronic health record data. Nat Med (2024). https://doi.org/10.1038/s41591-024-03214-0
Download citation
Received : 11 December 2023
Accepted : 25 July 2024
Published : 12 September 2024
DOI : https://doi.org/10.1038/s41591-024-03214-0
Share this article
Anyone you share the following link with will be able to read this content:
Sorry, a shareable link is not currently available for this article.
Provided by the Springer Nature SharedIt content-sharing initiative
Quick links
- Explore articles by subject
- Guide to authors
- Editorial policies
Sign up for the Nature Briefing: Translational Research newsletter — top stories in biotechnology, drug discovery and pharma.

Impact Analysis of Cyberattacks in Electric Propulsion Systems for Hybrid Tracked Vehicles 2024-01-4114
In the age of advancing digitalization and integrating complex electronics within modern vehicles, hybrid tracked vehicles (HTVs) are increasingly becoming susceptible to cybersecurity threats. Particularly vulnerable are the control units, which have become prime targets for adversarial exploitation due to their pivotal role in vehicle functionality. To address these security concerns, this study demonstrates two distinct yet equally harmful scenarios using replay and DoS attacks to uncover and evaluate vulnerabilities within the Energy Management System (EMS) of HEVs. In the replay attack scenario, the adversary surreptitiously alters the SoC control messages emanating from the Battery Management System (BMS). The attack is calibrated to strategically time and sequence the message replays across various operational states using reinforcement learning, thereby maintaining apparent legitimacy within expected SoC ranges to evade detection. The DoS attack, however, presents a more direct threat by targeting the generator’s revolution speed sensors. By compromising these sensors, the attack disrupts the generator and causes subsequent engine shutdowns, compelling the battery to singularly meet the vehicle’s power supply demands. Formal attack models are developed and subsequently deployed on a simulated HTV platform within MATLAB/Simulink to analyze the impacts of these cyberattacks. The simulation results illustrate the impacts of the simulated cyberattacks, further reinforcing the importance of resilient and adaptive security measures for the control layer.
SAE MOBILUS
Subscribers can view annotate, and download all of SAE's content. Learn More »
Access SAE MOBILUS »
- Open access
- Published: 12 September 2024
Vaccination governance in protracted conflict settings: the case of northwest Syria
- Ronja Kitlope Baatz 1 na1 ,
- Abdulkarim Ekzayez 2 , 3 na1 ,
- Yasser Najib 4 ,
- Munzer Alkhalil 5 , 6 ,
- Mohammad Salem 4 ,
- Mohammed Ayman Alshiekh 7 &
- Preeti Patel 2
BMC Health Services Research volume 24 , Article number: 1056 ( 2024 ) Cite this article
Metrics details
Effective vaccination governance in conflict-affected regions poses unique challenges. This study evaluates the governance of vaccination programs in northwest Syria, focusing on effectiveness, efficiency, inclusiveness, data availability, vision, transparency, accountability, and sustainability.
Using a mixed-methods approach, and adapting Siddiqi’s framework for health governance, data were collected through 14 key informant interviews (KIIs), a validating workshop, and ethnographic observations. Findings were triangulated to provide a comprehensive understanding of vaccination governance.
The study highlights innovative approaches used to navigate the complex health governance landscape to deliver vaccination interventions, which strengthened sub-national vaccination structures such as The Syria Immunisation Group (SIG). The analysis revealed several key themes. Effectiveness and efficiency were demonstrated through cold-chain reliability and extensive outreach activities, though formal reports lacked detailed analysis of vaccine losses and linkage between disease outbreak data and coverage statistics. Key informants and workshop participants rated the vaccination strategy positively but identified inefficiencies due to irregular funding and bureaucracy. Inclusiveness and data availability were prioritised, with outreach activities targeting vulnerable groups. However, significant gaps in demographic data and reliance on paper-based systems hindered comprehensive coverage analysis. Digitalisation efforts were noted but require further support. The SIG demonstrated a clear strategic vision supported by international organizations such as the World Health Organization, yet limited partner participation in strategic planning raised concerns about broader ownership and engagement. While the SIG was perceived as approachable, the lack of public documentation and financial disclosure limited transparency. Internal information sharing was prevalent, but public communication strategies were insufficient. Accountability and sustainability faced challenges due to a decentralized structure and reliance on diverse donors. Despite stabilizing factors such as decentralization and financial continuity, fragmented oversight and reliance on donor funding remained significant concerns.
The study highlights the complexities of vaccination governance in conflict-affected areas. Comparisons with other conflict zones underscore the importance of local organisations and international support. The SIG’s role is pivotal, but its legitimacy, transparency, and inclusivity require improvement. The potential transition to early recovery in Syria poses additional challenges to SIG’s sustainability and integration into national programs.
The governance of vaccination in northwest Syria is multifaceted, involving multiple stakeholders and lacking a legitimate government. Enhancing transparency, local ownership, and participatory decision-making are crucial for improving governance. The role of international bodies is essential, emphasising the need for structured feedback mechanisms and transparent monitoring processes to ensure the program’s success and sustainability.
Key message
• A hybrid governance model that combines top-down and bottom-up approaches effectively improves immunisation programs in conflict settings and promotes local ownership.
• In conflict settings, immunisation programmes require strong and direct intervention from the WHO with central management and coordination of the vaccine activities.
• In conflict areas, when the government is a party to the conflict or has limited access to some areas, United Nations institutions must intervene to manage or support vaccine activities in partnership with local entities, regardless of notions of national sovereignty.
• In Syria, the reluctance of United Nations institutions to fill the void left by the state in areas outside its control and provide vaccines led to the emergence of many diseases, including polio in 2013 and measles in 2017.
Peer Review reports
Introduction
Immunisation services are essential for any health system to ensure protection against major transmissible diseases. Armed conflicts often influence the availability, quality, accessibility, and uptake of vaccination services, which can lead to the emergence of outbreaks and epidemics [ 1 , 2 ]. The restoration of regular immunisation services in emergency contexts has not been extensively studied, and protracted crises “underscore the need to consider matters beyond the emergency mindset” [ 3 ]. Furthermore, health partnerships remain largely centred on national governments [ 4 ], raising the question of how areas beyond state control can best organise routine vaccination services.
The Syrian conflict, which started in March 2011, has had a devastating impact on the health system of the country; with vaccination coverage dropping from more than 90% for the Diphtheria, Tetanus & Pertussis (DTP) vaccine pre-conflict, to less than 10% in some areas [ 5 , 6 ]. With the fall of some areas under opposition control, the Syrian government began to withhold vaccinations from these areas, while simultaneously attacking healthcare facilities and infrastructure [ 7 ]. The decline in vaccine coverage resulted in outbreaks of Vaccine Preventable Diseases (VPDs), including polio (2013, 2017) and measles (2017, 2018) [ 8 , 9 ]. This led to vaccination becoming a priority for the humanitarian sector following the outbreak of wild poliovirus in October 2013.
Syria is now roughly divided into three main areas of control: the self-administration region of northeast Syria controlled by Kurdish majority forces, the governmental areas in the central, coastal and southern regions, and various opposition forces in the northwest. These delineations are visually depicted in Fig. 1 , where the regions are represented by the colours yellow, red, and green, respectively [ 10 ]. Opposition controlled areas in northwest Syria has a population of about 4.5 million people, of whom over a third, 1.8 million, live in camps, which is the area of focus in this study [ 11 ]. According to The United Nations Office for the Coordination of Humanitarian Affairs (OCHA), about 90% of the population is dependent on donor aid for their subsistence, including for health care [ 12 ]. Northwest Syria is governed by two main forces, the opposition forces with Turkish support in northern Aleppo, and Hayat Tahrir Al-Sham (HTS) in Idlib Governorate [ 13 ]. HTS is listed as a terrorist organisation by the US, UN, EU and Turkey, preventing aid organisations from working with them [ 14 ]. As there is no recognised government in northwest Syria and no clear end in sight to the conflict, international aid organisations are facing a long-term problem of coordination, particularly in programmes which require stability and effective governance, such as routine immunisation. Humanitarian access to northwest Syria has been using border crossing points from Turkey under annually renewed Resolutions by the UN Security Council since July 2014 [ 15 ]. However, this crossing became limited to only one crossing point, the Bab el Hawa border in 2019, and later this crossing has expired with the failure to renew this UN Resolution after being vetoed by Russia and China. The Security Council’s failure to reauthorise the long-standing cross border humanitarian aid mechanism in July 2023, has laid bare the implications for the humanitarian situation in Syria coupled with a deepening divide on the Security Council’s engagement on the issue. There is now uncertainty about the future of the aid mechanism and other UN operations in the region [ 16 ].
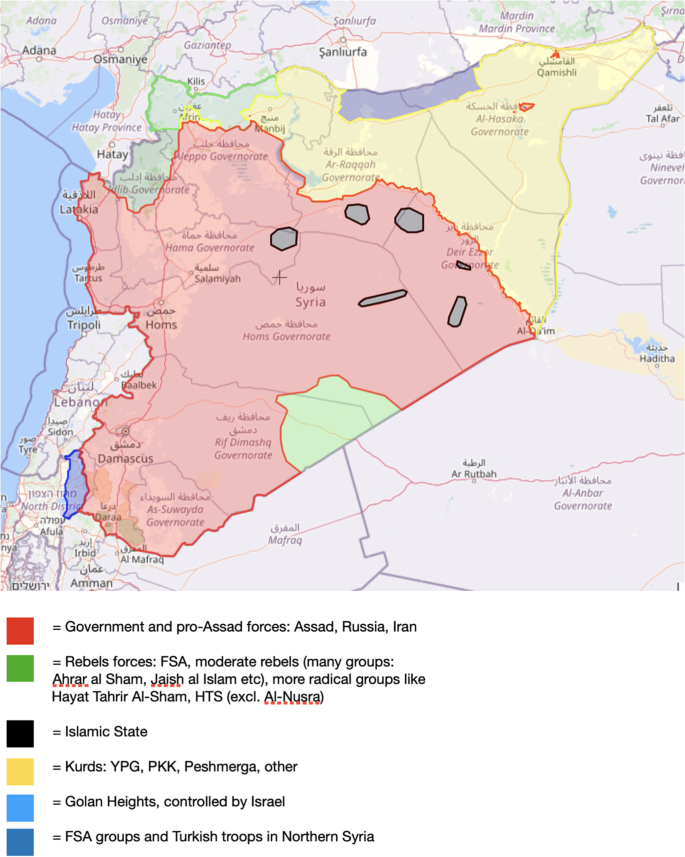
Areas of control in Syria as of April 2023. Source: Liveuamap, 2023
Prior to the conflict, Syria had advanced vaccination governance and high immunization coverage, with World Health Organization (WHO) and United Nations International Children’s Emergency Fund (UNICEF) estimating DTP vaccine coverage at over 89% [ 17 ]. During the conflict, vaccination activities faced significant challenges following the withdrawal of the Syrian Government from opposition-controlled territories in 2012. This led to disruptions in the supply chain, human resource shortages, and governance collapse, resulting in reduced vaccination coverage and outbreaks of diseases such as Polio and Measles [ 18 ]. Emergency vaccination campaigns were initiated by local and international actors to address these outbreaks, with the establishment of entities such as the Polio Task Force and Measles Task Force. Since 2016, vaccination efforts have been led by the Syria Immunisation Group (SIG), formed by local humanitarian actors and co-chaired by WHO and UNICEF. Please see Table 1 for the vaccination schedule in Syria before and after the conflict.
Despite Syria’s eligibility for Global Alliance for Vaccines and Immunization (GAVI) support in 2019, actual funding received remains lower than pledged, making it challenging to assess the total cost of vaccine activities [ 19 , 20 ]. The literature on vaccination governance in northwest Syria is scant, with limited distinction between northwest Syria and government-controlled areas. Comprehensive accounts of SIG’s work are rare, with the WHO 2020 report on Syria providing one notable exception [ 21 ]. This lack of literature may reflect the complex political economy context, as government withholding of vaccinations prompted alternative actors to facilitate vaccination and governance [ 22 ].
This study aims to explore the effectiveness and efficiency of vaccination governance in northwest Syria (NWS), its responsiveness, inclusivity, and informed decision-making processes, as well as its vision, strategy, transparency, and accountability. By examining these aspects, the research seeks to provide a comprehensive understanding of how vaccination programs operate in conflict-affected areas and the unique challenges they face.
Methodology
This study employed a mixed-methods approach consisting of semi-structured qualitative interviews, a validation workshop, and ethnographic observations to comprehensively investigate vaccination governance in northwest Syria.
Firstly, we adapted the Siddiqi framework for health governance [ 23 ] with modifications to accommodate the unique challenges and dynamics present in northwest Syria. Its six key principles offer a structured approach to assess governance effectiveness, inclusivity, transparency, and accountability, which were central to the study’s objectives. This adapted framework guided the data collection, analysis, and interpretation processes, providing a structured approach to examining vaccination governance from a health system perspective.
Secondly, we conducted 14 semi-structured qualitative Key Informant Interviews (KIIs) with key informants involved in vaccination governance in northwest Syria. Purposive sampling was used to select participants representing various stakeholders, including representatives from local health directorates, international organizations, and community leaders - please see Table 2 . Participants were identified based on their expertise and roles in vaccination delivery. We approached potential participants through email and phone calls, explaining the purpose of the study and inviting them to participate. Those who agreed to participate were scheduled for interviews at their convenience. The semi-structured interview guide (see Supplementary Material) aimed to explore participants’ experiences, perspectives, and challenges related to vaccination governance. The interviews were audio-recorded with participants’ consent and transcribed verbatim for analysis. Thematic analysis was conducted using both deductive and inductive approaches, with the Siddiqi framework guiding the thematic grouping and coding process. Notably, only two of the interviewees identified as female. This gender disparity reflects broader gender imbalances in leadership positions within the context of conflict-affected areas and may influence the perspectives and priorities discussed during the workshop.
Thirdly, a validation workshop was conducted in Gaziantep in November 2023 to validate the findings from the interviews and gather additional insights from stakeholders. The 15 participants in the workshop included key informants who had been interviewed, as well as other relevant stakeholders – please see Table 2 . An overview of the key findings per theme identified in the interviews was presented, followed by a discussion to validate and elaborate on these findings. The workshop facilitated a collaborative process to prioritize the main achievements and challenges identified in the interviews.
In addition, ethnographic observations were conducted alongside the field data collection to provide contextual insights into vaccination delivery and governance practices in northwest Syria. These observations involved daily immersion in the field, engaging in informal conversations with stakeholders, and documenting observations through field notes. This approach was used to build trust with key stakeholders, helping them understand the importance of our research and encouraging them to openly share their views and participate in research activities. The informal conversations and daily immersion provided rich qualitative data on the local context, practices, and challenges, which were crucial for interpreting the collected data. Additionally, relevant documents, such as reports and policy documents, were collected and analysed to complement the ethnographic data.
The three sets of data—interviews, workshop discussions, and ethnographic observations—were triangulated to enhance the validity and reliability of the findings. Triangulation was conducted through comparing and cross-referencing information from each data source. Initially, key themes and findings from the interviews were identified and categorised. These themes were then cross-checked against insights gathered from workshop discussions and ethnographic observations to identify common patterns, discrepancies, and unique contributions. Any discrepancies were further investigated through follow-up discussions or additional document analysis to resolve inconsistencies and confirm findings.
Ethical approval was obtained from the Institutional Review Board of King’s College London (MRA-22/23-34048) and, due to the sensitive nature of the subject, anonymity of participants was deemed critical. Informed consent was signed by all interviewees and interview records were deleted within two days after the interview, with notes being de-identified. All records and code-keys were stored on a password-protected secure drive.
This section presents five key themes that emerged from the data: effectiveness and efficiency, inclusiveness and data availability, clear vision with limited participatory strategy development, limited transparency, and accountability and sustainability. For each theme, findings are triangulated from interviews, workshop discussions, and ethnographic observations to provide a comprehensive understanding of vaccination governance in northwest Syria.
Effectiveness and efficiency
Field observations highlighted the operational success of the vaccination strategy, particularly in maintaining cold-chain reliability and conducting extensive outreach activities. Researchers noted that cold-chain facilities appeared well-maintained and outreach teams were active in various communities.
Document analysis corroborated these observations, although it revealed a lack of detailed analysis in formal reports regarding vaccine losses and linkage between disease outbreak data and coverage statistics. The annual report for 2021 noted the distribution of over 1.5 million routine vaccines and approximately 350,000 COVID-19 vaccines (SIG, 2021).
KIIs provided subjective assessments of effectiveness, with most participants rating the vaccination strategy very positively. For example, one key informant stated, “Cold-chain is very complicated, and (…) we have never faced gaps in the cold-chain. The outreach activities too, they are amazing in screening the whole community” (K-07). Another participant commented, “I think there are three successful entities in Syria. White Helmets, Early Warning and Response Network (EWARN) and SIG. Basically, they are performing governmental performance, without being a government” (K-10).
The workshop echoed these sentiments, emphasising the reliability of cold-chain logistics and the effectiveness of outreach programs. Participants highlighted the comprehensive knowledge outreach teams had about the communities, such as culture and health seeking behaviour, which facilitated high vaccine coverage.
Analysis suggests that while the subjective assessments are positive, the lack of detailed data in formal documents indicates a need for more robust quantitative evaluation mechanisms to fully substantiate these claims.
Efficiency was qualitatively explored through factors such as human resources, bureaucracy, corruption, and the non-governmental nature of the program. Field observations noted strong capacity among staff and stable governance structures.
Documents reviewed pointed to significant bureaucracy but suggested it was a necessary component to prevent corruption. KIIs reinforced this, with one participant noting, “You can’t do any humanitarian process without this paperwork, to be honest. It is the right way, because otherwise you are corrupted” (K-01). Another added that corruption was low due to the nature of the resources involved, stating, “There are few reasons for people to steal from this programme. It isn’t food baskets or money, it’s vaccines” (K-01).
Workshops confirmed these findings but also highlighted inefficiencies due to the lack of government services and irregular funding, which led to service discontinuations. One workshop participant explained, “The Expanded Programme for Immunisation (EPI) is continuous, it should be a 2 or 3 year project. For example, the first project ends by the end of May and the next project starts mid-June. So, there is a gap for staff, so they don’t receive their salaries” (W-02).
In conclusion, while the vaccination governance seems to be efficient with limited observed effectiveness, challenges remain in documentation and the impacts of funding irregularities, short termism and uncertainty.
Inclusiveness, responsiveness, and data availability
Field observations indicated that accessibility and inclusiveness are prioritized in vaccination efforts, with outreach activities playing a crucial role in reaching vulnerable groups. Researchers observed that outreach sessions outnumbered fixed sessions, reflecting the emphasis on inclusivity.
Document analysis revealed systematic data collection efforts to identify reasons for missed vaccinations to target vulnerable groups, including zero-dose children, people with disabilities, female-headed households, and those living in remote areas. However, significant gaps in demographic data and reliance on paper-based systems were noted, hindering comprehensive coverage analysis.
KIIs highlighted the challenges in data availability. One participant mentioned, “The most reliable approximations of vaccine coverage come from last year’s vaccination data and the door-to-door polio campaign” (K-05). Another added, “Alternative population data is available from OCHA, but it is considered inferior to the more comprehensive and up-to-date polio data” (K-06). This reliance on figures from previous Polio vaccination campaigns is confirmed by our document analysis. In 2021 the SIG vaccinated 134,083 children with Bacillus Calmette–Guérin (BCG). The Polio campaign in the previous year vaccinated a total of 155.378 children under 1. According to third party monitoring, the coverage rate of this polio campaign was 93%. Assuming that the age-distribution of the coverage is equal, this would make the total number of children under 1 in northwest Syria 167.073. Accordingly, the coverage rate for BCG would then be 80.3%. Similar statistics currently being used as coverage data, but these are suboptimal.
Workshop participants echoed these concerns, emphasizing the need for digitalization of medical and vaccination records. A participant remarked, “Paper vaccination cards are often lost, and manual data collection is prone to error. Digital systems are urgently needed” (W-03).
Our analysis indicates that while inclusivity is a stated priority and efforts are made to collect relevant data, the effectiveness of these efforts is limited by significant data availability challenges. Digitalization initiatives are a positive step but require more support and implementation.
Clear vision with limited participatory strategy development
Field observations showed the SIG’s active involvement in strategic planning, supported by WHO and GAVI. Researchers noted clear mission statements and detailed strategies in the SIG’s multi-year plan, though awareness among partners was limited.
Document analysis confirmed the existence of structured strategic plans but indicated fragmented decision-making processes involving multiple stakeholders, including donors, partners, and the SIG. The SIG was observed to function as a central coordination and mediation platform.
KIIs provided insights into the strategic planning processes, with participants acknowledging sufficient opportunities for input but noting limited participation from partners. One participant stated, “I don’t think the NGOs are participating in finding solutions. Mainly the SIG is doing this. The SIG is doing a good job, so we feel relaxed somehow, so we don’t want to interfere in the system” (K-11). Another added, “It is positive that the implementing partners are only implementing the central plans” (K-06).
Workshop participants supported these findings, expressing trust in the SIG’s strategic planning but also highlighting the lack of engagement from partners in the decision-making process. One participant noted, “The SIG maintains the strategy and the quality of the strategy. In humanitarian crises and the Syrian context, we operate as organizations, but we established a central team” (W-04).
Our analysis suggests that while the SIG has a clear vision and structured strategic plans, the limited participatory strategy development may hinder broader ownership and engagement from all partners.
Limited transparency
Field observations noted a general perception of the SIG being approachable, but with limited transparency in documentation. Researchers observed that information sharing was mostly internal, with minimal public disclosure.
Document analysis highlighted the lack of an internet presence, financial disclosure, and public availability of strategic plans and annual reports. Information was primarily disseminated through internal reports and meetings, limiting access for external stakeholders.
KIIs revealed a discrepancy between perceived and actual transparency. One participant commented, “A normal Ministry of Health would not separately publish their vaccination results in so much detail” (K-03). Another stated, “Partners funded through the WHO share their financial data with the SIG, but privately funded partners do not” (K-02).
Workshop participants emphasized the need for greater transparency, particularly for stakeholders not directly involved in the SIG’s network. A participant remarked, “It is difficult to obtain information about the topic if one is not part of the network. Only the WHO and the Assistant Coordination Unit (ACU) additionally report on selected aspects of vaccination” (W-01).
Our analysis indicates that while the SIG is considered transparent by partners due to its approachability, the lack of public documentation and financial disclosure limits overall transparency. Enhanced public communication strategies could improve transparency and accountability.
Accountability and sustainability
Field observations underscored the complex collaboration of stakeholders underpinning vaccine provision, with no single body having legitimate oversight. Filed researchers noted the decentralised structure and reliance on various donors.
Document analysis highlighted the lack of enforcement mechanisms for medical guidelines and protocols. The SIG’s Statement of Principle lacked enforceable standards, leaving de facto power with diverse donors. This patchwork funding approach posed challenges to accountability and sustainability.
KIIs pointed to the absence of a central governance body, with one participant noting, “The donors know that the SIG is not officially on the papers, but they know there is a body called SIG responsible for reaching the target, achieving the indicators, and supervising technically” (K-07). Another participant identified potential risks, stating, “The cut of funds, war, and lack of stability of the security situation. We have the scenario, but we don’t know what will happen” (K-08).
Workshop participants discussed stabilising factors such as the system’s size, decentralized structure, and financial continuity. One participant remarked, “The system grows and becomes a stable system. Everyone is aware of how the system is growing, and this assists the continuity” (W-05).
Our analysis concludes that while there are significant challenges to accountability and sustainability, including fragmented oversight and reliance on diverse donors, stabilizing factors such as decentralization and financial continuity offer some resilience against potential disruptions. Capacity building at district and governorate levels is crucial for ensuring long-term stability and effectiveness.
The primary themes under investigation in this study encompassed the effectiveness and efficiency of the vaccination governance in northwest Syria; its responsiveness, inclusivity, and informed decision-making; its vision and strategy; transparency; and accountability and sustainability.
The management and coordination of vaccination in conflict-affected areas pose significant challenges to effectiveness and efficiency. In regions like northwest Syria, where government control is limited, the discontinuation of routine vaccination services exacerbates these challenges. Comparisons with other conflict-affected areas, such as Myanmar and Somalia, highlight the role of local organizations and international support in filling governance gaps [ 24 , 25 ]. However, research on vaccination coordination in northwest Syria remains sparse, underscoring the need for a deeper understanding of local structures and operations.
Prior to 2016, the health governance model followed a bottom-up approach, with local entities playing significant roles in vaccination activities. With the establishment of SIG, a hybrid top-down and bottom-up model emerged, shifting the focus to international support and coordination while preserving field connection. This model change reflects the unique challenges of vaccination services in conflict-affected regions and underscores the need for a collaborative approach under the United Nations’ umbrella.
The Syria Immunisation Group (SIG) plays a pivotal role in vaccination governance in northwest Syria, aiming to address these challenges. While SIG has gained internal legitimacy through collaboration with health directorates (HDs) and external legitimacy through collaborating with WHO and UNICEF, concerns regarding accountability and inclusivity persist. The lack of transparency and involvement of partners in strategic planning processes hinder informed decision-making. These finding are in line with a study by Alaref et al. in 2023 which evaluated six governance principles for central quasi-governmental institutions in northwest Syria, including SIG, and found that its legitimacy is fair and requires improvement, scoring 41–60% on a health system governance scale adapted for this paper. Accountability, transparency, effectiveness and efficiency were poor and required significant improvement, scoring 21–40%, while strategic vision was very poor or inactive, scoring 0–20% [ 26 ].
Despite having a strategic plan and receiving support from international organisations like the WHO and GAVI, SIG faces contradictions in its effectiveness and efficiency. The transition from emergency task forces to SIG was marked by power dynamics and challenges to local ownership, raising questions about sustainability and integration into national vaccination programs [ 9 ]. The potential transition of WHO operations further complicates the future of SIG, posing a key challenge to early recovery in Syria.
These findings raise questions about the future of the SIG body in light of the political and military changes in the region and the constant threat associated with cross-border operations. What would happen if the WHO ceased operations in Gaziantep and moved to Damascus, where a national vaccine program has been in place for decades? In such a scenario, would the SIG continue to carry out its activities in northwest Syria, or would it become a part of the national vaccine program? This is a key challenge for the transition to early recovery in Syria.
In conclusion, the governance of vaccination in conflict-affected areas of northwest Syria is complex, with multiple stakeholders involved and a lack of a legitimate government to fulfil essential functions. The success of the vaccination program heavily relies on the efforts of the Syria Immunisation Group (SIG), which acts as a trusted mediator between various stakeholders. However, the lack of transparency and accountability hinders the ability to assess the program’s effectiveness and efficiency. This calls for a push towards more localised ownership and transparency, with a hybrid top-down and bottom-up approach that addresses the unique context of conflict settings. Engaging local partners in decision-making and capacity building can improve sustainability and address issues surrounding legitimacy. Moreover, the responsibility to protect public health goes beyond national sovereignty, and the role of international bodies like the WHO becomes crucial in conflict areas. Inaction or delayed action can have catastrophic consequences, as witnessed in Syria with the emergence of diseases like polio and measles. It is essential to implement a structured feedback mechanism and transparent monitoring and evaluation processes to address challenges and foster trust among stakeholders and the community. Ultimately, the findings of this study inform debates around health governance in conflict settings, highlighting the need for more inclusive, transparent, and context-sensitive approaches to ensure the success and sustainability of vaccination programs.
Data availability
The datasets generated and/or analysed during the current study are not publicly available due to the sensitive nature of the data, but are available from the corresponding author on reasonable request.
Abbreviations
Syria Immunisation Group
Early Warning and Response Network
Expanded Programme on Immunization
World Health Organization
Global Alliance for Vaccines and Immunization
United Nations Office for the Coordination of Humanitarian Affairs
Health Directorates
Global Alliance for Vaccines and Immunisation
Key Informant Interviews
United Nations International Children’s Emergency Fund
Sato R. Effect of armed conflict on vaccination: evidence from the Boko Haram insurgency in northeastern Nigeria. Confl Health. 2019;13(1):1–10.
Article Google Scholar
Ngo NV, Pemunta NV, Muluh NE, Adedze M, Basil N, Agwale S. Armed conflict, a neglected determinant of childhood vaccination: some children are left behind. 2019;16(6):1454–63. https://doi.org/10.1080/2164551520191688043
Lam E, McCarthy A, Brennan M. Vaccine-preventable diseases in humanitarian emergencies among refugee and internally-displaced populations. Hum Vaccin Immunother. 2015;11(11):2627–36.
Article PubMed PubMed Central Google Scholar
Kennedy J, Michailidou D. Civil war, contested sovereignty and the limits of global health partnerships: a case study of the Syrian polio outbreak in 2013. Health Policy Plan. 2017;32(5):690–8.
Article PubMed Google Scholar
Pereira A, de Southgate L, Ahmed R, O’Connor H, Cramond P, Lenglet V. A. Infectious Disease Risk and Vaccination in Northern Syria after 5 years of Civil War: the MSF experience. PLoS Curr. 2018;10.
Tajaldin B, Almilaji K, Langton P, Sparrow A. Defining polio: closing the gap in global surveillance. Ann Glob Health. 2015;81(3):386–95.
Ahmad B, Bhattacharya S. Polio eradication in Syria. Lancet Infect Dis. 2014;14(7):547–8.
Meiqari L, Hoetjes M, Baxter L, Lenglet A. Impact of war on child health in northern Syria: the experience of Médecins sans Frontières. Eur J Pediatr. 2018;177(3):371–80.
Initiative GPE. In. Syrian Arab Republic. 2021. p. 191–191.
Alkhalil M, Alaref M, Mkhallalati H, Alzoubi Z, Ekzayez A. An analysis of humanitarian and health aid alignment over a decade (2011–2019) of the Syrian conflict. Confl Health. 2022.
Alkhalil M, Ekzayez A, Rayes D, Abbara A. Inequitable access to aid after the devastating earthquake in Syria. Lancet Glob Health. 2023;0(0).
OCHA. Northwest Syria Humanitarian Readiness and Response Plan. 2020.
Zulfiqar ABBC, Reality C. 2020 [cited 2020 May 2]. Syria: Who’s in control of Idlib? - BBC News. https://www.bbc.co.uk/news/world-45401474
EUAA. 1.3. Anti-government armed groups | European Union Agency for Asylum [Internet]. 2020 [cited 2023 Sep 10]. https://euaa.europa.eu/country-guidance-syria/13-anti-government-armed-groups
Alkhalil M, Alaref M, Ekzayez A, Mkhallalati H, El Achi N, Alzoubi Z, et al. Health aid displacement during a decade of conflict (2011–19) in Syria: an exploratory analysis. BMC Public Health. 2023;23(1):1–16.
Security Council Report. In. Hindsight: the demise of the Syria cross-border aid mechanism, August 2023 Monthly Forecast. Security Council Report; 2023.
WHO, Unicef. Immunization Summary: A statistical reference containing data through 2010. Vol. 2011. 2011.
Ekzayez A, Alkhalil M, Patel P, Bowsher G. Pandemic governance and community mobilization in conflict: a case study of Idlib, Syria. Inoculating cities: Case studies of the Urban response to the COVID-19 pandemic. 2024;61–80.
OECD. Creditor Reporting System (CRS) [Internet]. 2023 [cited 2023 Dec 1]. https://stats.oecd.org/Index.aspx?DataSetCode=CRS1
Kaddar M, Saxenian H, Senouci K, Mohsni E, Sadr-Azodi N. Vaccine procurement in the Middle East and North Africa region: challenges and ways of improving program efficiency and fiscal space. Vaccine. 2019;37(27):3520–8.
World Health Organization. World Health Organization Syrian Arab Republic [Internet]. 2020 [cited 2023 Sep 10]. http://apps.who.int/bookorders
ACU. Annual report 2019. Vol. 5, AIMS Mathematics. 2019.
Siddiqi S, Masud TI, Nishtar S, Peters DH, Sabri B, Bile KM, et al. Framework for assessing governance of the health system in developing countries: gateway to good governance. Health Policy. 2009;90(1):13–25.
Hugh Guan T, Htut HN, Davison CM, Sebastian S, Bartels SA, Aung SM, et al. Implementation of a neonatal hepatitis B immunization program in rural Karenni State, Myanmar: a mixed-methods study. PLoS ONE. 2021;16(12 December):e0261470.
Hugh Guan T, Htut HN, Davison CM, Sebastian S, Bartels SA, Aung SM et al. Implementation of a neonatal hepatitis B immunization program in rural Karenni State, Myanmar: A mixed-methods study. PLoS One [Internet]. 2021 Dec 1 [cited 2023 May 9];16(12 December):e0261470. https://journals.plos.org/plosone/article?id=10.1371/journal.pone.0261470
Alaref M, Al-Abdulla O, Al Zoubi Z, Al Khalil M, Ekzayez A. Health system governance assessment in protracted crisis settings: Northwest Syria. Health Res Policy Syst. 2023;21(1):1–13.
Download references
Acknowledgements
The authors acknowledge invaluable contributions of several staff based in Turkey and Syria for their input, access and support. We also wish to acknowledge in particularly contribution from Dr. Mahmoud Daher, then Head of the Gaziantep (Turkey) Office. Furthermore, appreciation is expressed for the contributions of the Assistance Coordination Unit staff, for the documents they made available for this study and their input in the analysis.
This publication is funded through the National Institute for Health Research (NIHR) 131207, Research for Health Systems Strengthening in northern Syria (R4HSSS), using UK aid from the UK Government to support global health research. The views expressed in this publication are those of the author(s) and do not necessarily reflect those of the NIHR or the UK government.
Author information
Ronja Kitlope Baatz and Abdulkarim Ekzayez are equal contributors to this work and designated as co-first authors.
Authors and Affiliations
Deventer Hospital, Deventer, Netherlands
Ronja Kitlope Baatz
Research for Health System Strengthening in northern Syria (R4HSSS), The Centre for Conflict & Health Research (CCHR), King’s College London, Strand, WC2R 2LS, London, UK
Abdulkarim Ekzayez & Preeti Patel
Syria Development Centre (SyriaDev), London, UK
Abdulkarim Ekzayez
Syria Immunisation Group (SIG), Gaziantep, Turkey
Yasser Najib & Mohammad Salem
Syria Public Health Network, London, UK
Munzer Alkhalil
Research for Health System Strengthening in Northern Syria (R4HSSS), UOSSM, Gaziantep, Turkey
Vascular Senior Clinical Fellow, Manchester Royal Infirmary, Manchester, UK
Mohammed Ayman Alshiekh
You can also search for this author in PubMed Google Scholar
Contributions
The initial framing, literature review, data collection and drafting of the study were carried out by RB and AE. AE contributed to the design, supervision, data collection, data analysis, and multiple rounds of editing. YN contributed to access to data, data collection, and data analysis. MS contributed to access to data and data analysis. PP contributed to analysis and multiple rounds of editing. Mohammed Ayman Alshiekh (MA) contributed to analysis and multiple rounds of editing. Munzer Alkhalil contributed to analysis and multiple rounds of editing. All authors read and approved the final manuscript. All authors read and approved the final manuscript.
Corresponding authors
Correspondence to Ronja Kitlope Baatz or Abdulkarim Ekzayez .
Ethics declarations
Ethics approval and consent to participate.
Ethical approval was obtained from the Institutional Review Board of King’s College London, under the approval number MRA-22/23-34048. Informed consent was obtained from all participants involved in the study. Participants were provided with detailed information regarding the study’s objectives, procedures, potential risks, and benefits. They were assured of their right to withdraw from the study at any time without any repercussions. All data collected were anonymised to ensure the confidentiality and privacy of the participants.
Consent for publication
Not applicable.
Competing interests
The authors declare no competing interests.
Additional information
Publisher’s note.
Springer Nature remains neutral with regard to jurisdictional claims in published maps and institutional affiliations.
Electronic supplementary material
Below is the link to the electronic supplementary material.
Supplementary Material 1
Rights and permissions.
Open Access This article is licensed under a Creative Commons Attribution 4.0 International License, which permits use, sharing, adaptation, distribution and reproduction in any medium or format, as long as you give appropriate credit to the original author(s) and the source, provide a link to the Creative Commons licence, and indicate if changes were made. The images or other third party material in this article are included in the article’s Creative Commons licence, unless indicated otherwise in a credit line to the material. If material is not included in the article’s Creative Commons licence and your intended use is not permitted by statutory regulation or exceeds the permitted use, you will need to obtain permission directly from the copyright holder. To view a copy of this licence, visit http://creativecommons.org/licenses/by/4.0/ .
Reprints and permissions
About this article
Cite this article.
Baatz, R.K., Ekzayez, A., Najib, Y. et al. Vaccination governance in protracted conflict settings: the case of northwest Syria. BMC Health Serv Res 24 , 1056 (2024). https://doi.org/10.1186/s12913-024-11413-1
Download citation
Received : 16 January 2024
Accepted : 07 August 2024
Published : 12 September 2024
DOI : https://doi.org/10.1186/s12913-024-11413-1
Share this article
Anyone you share the following link with will be able to read this content:
Sorry, a shareable link is not currently available for this article.
Provided by the Springer Nature SharedIt content-sharing initiative
- Immunisation
- Vaccination
- Health governance
- Conflict setting
- Localisation
BMC Health Services Research
ISSN: 1472-6963
- General enquiries: [email protected]
A Review on Power System Security Issues in the High Renewable Energy Penetration Environment
- Original Article
- Published: 12 September 2024
Cite this article
- Dwi Riana Aryani ORCID: orcid.org/0000-0002-2687-5580 1 &
- Hwachang Song ORCID: orcid.org/0000-0002-6313-2719 1
As one of the efforts to overcome the problem of climate change, increasing the share of renewable energy (RE) in the national energy mix has become intensive in many countries, especially after the ratification of the Paris Agreement in 2015. Although this effort can effectively reduce carbon emissions, challenges to the security of power systems with increasing RE penetration are also emerging. This paper aims to provide an overview of several security issues on power systems, along with challenges arising from the impact of inertial reduction, RE fluctuations, RE prediction errors, and fault response, addressed to researchers as a reference for further studies. Case studies of security issues experienced by several system operators (SOs) when RE penetration is high in their electrical grids are discussed as a lesson for modern power systems operations. Moreover, measures to prevent and overcome these problems are proposed, including the need for changes and development in security assessment, protection and control schemes, and more relevant services for facing system security challenges in the future.
This is a preview of subscription content, log in via an institution to check access.
Access this article
Subscribe and save.
- Get 10 units per month
- Download Article/Chapter or eBook
- 1 Unit = 1 Article or 1 Chapter
- Cancel anytime
Price includes VAT (Russian Federation)
Instant access to the full article PDF.
Rent this article via DeepDyve
Institutional subscriptions
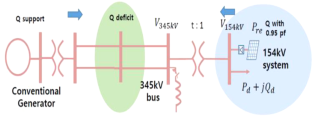
Similar content being viewed by others
Technical Challenges, Security and Risk in Grid Integration of Renewable Energy
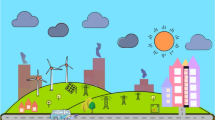
Impact of intermittent renewable energy generation penetration on the power system networks – A review
Grid Stability: High Penetration Levels of Renewable Energies
Explore related subjects.
- Artificial Intelligence
Anderson PM, Fouad AA (2003) Institute of Electrical, and Electronics Engineers. Power System Control and Stability. IEEE Press power engineering series Power system control and stability. Wiley, ISBN 9780471238621
Li W. Operation Reliability of Power Systems, pages 1–16. John Wiley and, Sons L (2012) ISBN 9780471346081. https://doi.org/10.1002/047134608X.W8187 . URL https://onlinelibrary.wiley.com/doi/abs/10.1002/047134608X.W8187
Juri Belikov and Yoash Levron (2018) Uses and misuses of quasi-static time-varying phasor models in power systems. IEEE Trans Power Delivery 33(6):3263–3266. https://doi.org/10.1109/TPWRD.2018.2852950
Article Google Scholar
Ili´c M, Zaborszky J. Dynamics and Control of Large Electric Power Systems. Wiley IEEE., Wiley (2000) ISBN 9780471298588. URL https://books.google.co.kr/books?id=i1JjQgAACAAJ
Morison K, Wang L, Kundur P (2004) Power system security assessment. IEEE Power Energ Mag 2(5):30–39. https://doi.org/10.1109/MPAE.2004.1338120
Mattavelli P, Stankovic AM, Verghese GC (1999) Ssr analysis with dynamic phasor model of thyristor-controlled series capacitor. IEEE Trans Power Syst 14(1):200–208. https://doi.org/10.1109/59.744524
Stefanov PC, Stankovic AM (2002) Modeling of upfc operation under unbalanced conditions with dynamic phasors. IEEE Trans Power Syst 17(2):395–403. https://doi.org/10.1109/TPWRS.2002.1007909
Kundur P, Paserba J, Ajjarapu V, Andersson G, Bose A, Canizares C, Hatziargyriou N, Hill D, Stankovic A, Taylor C, Van Cutsem T, Vittal V (2004) Definition and classification of power system stability ieee/cigre joint task force on stability terms and definitions. IEEE Trans Power Syst 19(3):1387–1401. https://doi.org/10.1109/TPWRS.2004.825981
Gu Y, Timothy C (2023) Green. Power system stability with a high penetration of inverter-based resources. Proceedings of the IEEE, 111(7):832– 853, https://doi.org/10.1109/JPROC.2022.3179826
Nikos Hatziargyriou J, Milanovic C, Rahmann V, Ajjarapu C, Canizares I, Erlich D, Hill I, Hiskens I, Kamwa B, Pal P, Pourbeik (2021) Juan Sanchez-Gasca, Aleksandar Stankovic, Thierry Van Cutsem, Vijay Vittal, and Costas Vournas. Definition and classification of power system stability– revisited & extended. IEEE Trans Power Syst 36(4):3271–3281. https://doi.org/10.1109/TPWRS.2020.3041774
Pieter Tielens and Dirk Van Hertem. The relevance of inertia in power systems. Renewable and Sustainable Energy Reviews, 55:999–1009 (2016) ISSN 1364– 0321. https://doi.org/10.1016/j.rser.2015.11.016 . URL https://www.sciencedirect.com/science/article/pii/S136403211501268X
Wenjuan Du J, Bi, Wang HF (2017) Small-signal angular stability of power system as affected by grid-connected variable speed wind generators- a survey of recent representative works. CSEE J Power Energy Syst 3(3):223. https://doi.org/10.17775/CSEEJPES.2016.00640
Bendong Tan J, Zhao M, Netto V, Krishnan V, Terzija, Zhang Y (2022) Power system inertia estimation: Review of methods and the impacts of converter-interfaced generations. International Journal of Electrical Power & Energy Systems, 134:107362, ISSN 0142–0615. https://doi.org/10.1016/j.ijepes.2021.107362 . URL https://www.sciencedirect.com/science/article/pii/S0142061521006013
Wang Y, Silva V, Winckels A (2014) Impact of high penetration of wind and pv generation on frequency dynamics in the continental europe interconnected system. 10:11. https://doi.org/10.1049/iet-rpg.2015.0141
Aleksandar Boriˇci´c Jos´eL, Rueda Torres, Popov M (2021) Comprehensive review of short-term voltage stability evaluation methods in modern power systems. Energies, 14(14), ISSN 1996– 1073. https://doi.org/10.3390/en14144076 . URL https://www.mdpi.com/1996-1073/14/14/4076
Aleksandar Boriˇci´c JLR, Torres, Popov M (2021) Fundamental study on the influence of dynamic load and distributed energy resources on power system short-term voltage stability. International Journal of Electrical Power & Energy Systems, 131:107141, ISSN 0142–0615. https://doi.org/10.1016/j.ijepes.2021.107141 . URL https://www.sciencedirect.com/science/article/pii/S014206152100380X
Panagiotis Mandoulidis T, Souxes, Vournas C (2021) Impact of converter interfaced generators on power system long-term voltage stability monitoring and control. Electric Power Systems Research, 199:107438, ISSN 0378–7796. https://doi.org/10.1016/j.epsr.2021.107438 . URL https://www.sciencedirect.com/science/article/pii/S0378779621004193
Ela E, Milligan M, Kirby B Operating reserves and variable generation. doi: 10.2172/ 1023095. URL https://www.osti.gov/biblio/1023095
April Sagan Y, Liu, Bernstein A (2021) Decentralized low-rank state estimation for power distribution systems. IEEE Trans Smart Grid 12(4):3097–3106. https://doi.org/10.1109/TSG.2021.3058609
Patrick S, Sauter P, Karg M, Kluwe, Hohmann S (2018) Load forecasting in distribution grids with high renewable energy penetration for predictive energy management systems. In 2018 IEEE PES Innovative Smart Grid Technologies Conference Europe (ISGT-Europe), pages 1– 6, https://doi.org/10.1109/ISGTEurope.2018.8571524
Milligan M, Frew B, Zhou E, Douglas J Arent. Advancing system flexibility for high penetration renewable integration. doi: 10.2172/1225920. URL https://www.osti.gov/biblio/1225920
Lasantha Meegahapola T, Littler, Perera S (2013) Capability curve based enhanced reactive power control strategy for stability enhancement and network voltage management. International Journal of Electrical Power & Energy Systems, 52:96–106, ISSN 0142–0615. https://doi.org/10.1016/j.ijepes.2013.03.036 . URL https://www.sciencedirect.com/science/article/pii/S0142061513001531
Jufri FH, Widiputra V, Jung J (2019) State-of-the-art review on power grid resilience to extreme weather events: Definitions, frameworks, quantitative assessment methodologies, and enhancement strategies. Applied Energy, 239:1049–1065, ISSN 0306–2619. https://doi.org/10.1016/j.apenergy.2019.02.017 . URL https://www.sciencedirect.com/science/article/pii/S0306261919303071
AEMO (2017) Black system south australia 28 september 2016. Technical report, 03 AEMO.
Ruifeng Y, Masood N-A, Saha TK, Bai F, Huajie Gu (2018) The anatomy of the 2016 south Australia blackout: a catastrophic event in a high renewable network. IEEE Trans Power Syst 33(5):5374–5388. https://doi.org/10.1109/TPWRS.2018.2820150
Joint NERC, Staff WECC (2022) Multiple solar pv disturbances in caiso. Technical report, 04. URL https://www.nerc.com/pa/rrm/ea/Documents/NERC_2021_California_Solar_PV_Disturbances_Report.pdf
Huang S-H, Schmall J, Conto J, Adams J, Zhang Y, Carter C (2012) Voltage control challenges on weak grids with high penetration of wind generation: Ercot experience. In 2012 IEEE Power and Energy Society General Meeting, pages 1–7, https://doi.org/10.1109/PESGM.2012.6344713
DESNZ Renewable energy planning database (repd): January 2024. Technical report
Diane Palmer E, Koubli T, Betts, and Ralph Gottschalg (2017). The uk solar farm fleet: A challenge for the national grid?. Energies, 10(8), ISSN 1996– 1073. https://doi.org/10.3390/en10081220 . URL https://www.mdpi.com/1996-1073/10/8 / 1220
Industry Ministry of Trade and Energy (MOTIE) Multiple solar pv disturbances in caiso. Technical report, 01 2023. URL https://motie.go.kr
Jeju Special Self-Governing Province (2019) URL https://www.jejuenergy.or.kr/index.php/ board?act = down&seq = 1941&serial = 3&bd_bcid = gallery_energy&page=
Korea Power Exchange and One Energy Island (2024) Securing power system reliability during renewable energy expansion: In light of south korea power system operation. Technical report, Smart KPX
Ricardo Bessa C, Moreira B, Silva, Manuel, Matos (2014) Handling renewable energy variability and uncertainty in power systems operation. WIREs Energy and Environment, 3 (2):156–178, https://doi.org/10.1002/wene.76 . URL https://wires.onlinelibrary.wiley.com/doi/abs/10.1002/wene.76
Jonghwan Kwon Z, Zhou T, Levin, Botterud A (2020) Resource adequacy in electricity markets with renewable energy. IEEE Trans Power Syst 35(1):773–781. https://doi.org/10.1109/TPWRS.2019.2930934
Kim S, Oh B, Lee D (05 2023) Application of an operating reserve demand curve in ercot in the South Korean electricity market to accommodate high penetration levels of renewable energy. J Electr Eng Technol 18:1457–1468. https://doi.org/10.1007/s42835-023-01417-y
Erik Ela and Mark O’Malley (2012) Studying the variability and uncertainty impacts of variable generation at multiple timescales. IEEE Trans Power Syst 27(3):1324–1333. https://doi.org/10.1109/TPWRS.2012.2185816
Kang J-O, Oh S-W, Son H-G, Jang-geun K (07 2022) Analysis on the variability of renewable energy in 2034 and importance of gas turbine of South Korea. J Electr Eng Technol 17:2181–2186. https://doi.org/10.1007/s42835-022-01053-y
Sreena Sreekumar, Savier JS (09 2023) A novel comparative approach for estimating maximum penetration capacity of grid connected solar photovoltaic system in distribution network. J Electr Eng Technol 18:3489–3506. https://doi.org/10.1007/s42835-023-01439-6
Tianshu Bi B, Yang K, Jia L, Zheng Q, Liu, Yang Q (2022) Review on renewable energy source fault characteristics analysis. CSEE J Power Energy Syst 8(4):963–972. https://doi.org/10.17775/CSEEJPES.2021.06890
Line A, Roald D, Pozo A, Papavasiliou DK, Molzahn (2023) Jalal Kazempour, and Antonio Conejo. Power systems optimization under uncertainty: A review of methods and applications. Electric Power Systems Research, 214:108725, ISSN 0378–7796. https://doi.org/10.1016/j.epsr.2022.108725 . URL https://www.sciencedirect.com/science/article/pii/S0378779622007842
Semich Impram SV, Nese, Bu¨lent, Oral (2020) Challenges of renewable energy penetration on power system flexibility: A survey. Energy Strategy Reviews, 31:100539, ISSN 2211-467X. https://doi.org/10.1016/j.esr.2020.100539 . URL https://www.sciencedirect.com/science/article/pii/S2211467X20300924
Ge X, Qian J, Fu Y, Lee W-J, Mi Y (2022) Transient stability evaluation criterion of multi-wind farms integrated power system. IEEE Trans Power Syst 37(4):3137–3140. https://doi.org/10.1109/TPWRS.2022.3156430
Eknath Vittal MO’Malley, Keane A (2012) Rotor angle stability with high penetrations of wind generation. IEEE Trans Power Syst 27(1):353–362. https://doi.org/10.1109/TPWRS.2011.2161097
Zhang Y, Zhu S, Sparks R, Green I (2012) Impacts of solar pv generators on power system stability and voltage performance. In 2012 IEEE Power and Energy Society General Meeting, pages 1–7, https://doi.org/10.1109/PESGM.2012 . 6344990
ENTSO-E (2020) Inertia and rate of change of frequency (rocof). Technical Report Version 17, ENTSO-E
Saha S, Saleem MI, Roy TK (2023) Impact of high penetration of renewable energy sources on grid frequency behaviour. International Journal of Electrical Power and Energy Systems, 145:108701, ISSN 0142–0615. https://doi.org/10.1016/j.ijepes.2022.108701 . URL https://www.sciencedirect.com/science/article/pii/S0142061522006974
Jeremy Till S, You Y, Liu, Du P, Transmission IEEEPES, Conference D, Exposition (2020) Impact of high pv penetration on voltage stability. In (T&D), pages 1–5, 2020. https://doi.org/10.1109/TD39804.2020.9299973
Justin Katigbak D, Kang, Song H (2023) Analysis of overvoltage reactive power margin for future power systems with renewable energy generation. Energy Reports, 9:962–970, ISSN 2352–4847. https://doi.org/10.1016/j.egyr.2023.05.153 . URL https://www.sciencedirect.com/science/article/pii/S2352484723009058 . 2022 The 3rd International Conference on Power and Electrical Engineering
KEPCO (2021) Regulations on the use of electrical equipment for transmission and distribution. Technical report, KEPCO
Hassan W, Qazi P, Wall MV, Escudero C, Carville N, Cunniffe, Jon O’Sullivan (2022) Impacts of fault ride through behavior of wind farms on a low inertia system. IEEE Trans Power Syst 37(4):3190–3198. https://doi.org/10.1109/TPWRS.2020.3003470
Popovi´c DH, Hiskens IA, Hill DJ (1998) Stability analysis of induction motor networks. International Journal of Electrical Power & Energy Systems, 20(7):475–487, ISSN 0142–0615. https://doi.org/10.1016/S0142-0615(98)00011-8 . URL https://www.sciencedirect.com/science/article/pii/S0142061598000118
Nahid-Al-Masood N, Modi, Ruifeng, Yan (2016) Low inertia power systems: Frequency response challenges and a possible solution. In. Australasian Universities Power Engineering Conference (AUPEC), pages 1–6, 2016. 10.1109/ AUPEC.2016.7749335
Kundur P, Balu NJ, Lauby MG (1994) Power System Stability and Control. EPRI power system engineering series. McGraw-Hill Education, ISBN 9780070359581. URL https://books.google.co.kr/books?id=2cbvyf8Ly4AC
Atia Adrees PN, Papadopoulos (2016) and Jovica V. Milanovic. A framework to assess the effect of reduction in inertia on system frequency response. In 2016 IEEE Power and Energy Society General Meeting (PESGM), pages 1–5, https://doi.org/10.1109/PESGM.2016.7741695
Aryani DR, Song H, Yoon-Sung, Cho (2022) Operation strategy of battery energy storage systems for stability improvement of the korean power system. Journal of Energy Storage, 56:106091, ISSN 2352-152X. https://doi.org/10.1016/j.est.2022.106091 . URL https://www.sciencedirect.com/science/article/pii/S2352152X22020795
MOTIE (2020) Power system reliability and electricity quality criteria. Technical report, Sejong-si, Korea
Paul Denholm and Maureen Hand. Grid flexibility and storage required to achieve very high penetration of variable renewable electricity. Energy Policy, 39(3):1817–1830 (2011) ISSN 0301–4215. https://doi.org/10.1016/j.enpol.2011.01.019 . URL https://www.sciencedirect.com/science/article/pii/S0301421511000292
Han SSNJW, Cho MS, Song H (2022) A guide for the life of Ess battery stabilizing power system on the analysis of power system environment. Trans Korean Inst Electr Eng 72(3):374–380 ISSN 1975. https://doi.org/10.5370/KIEE.2023.72.3.374 . 8359
Brinkel NBG, Gerritsma MK, AlSkaif TA, Lampropoulos AM, van Voorden HA, Fidder, van Sark WGJHM Impact of rapid pv fluctuations on power quality in the low-voltage grid and mitigation strategies using electric vehicles. International Journal of Electrical Power and Energy Systems, 118:105741, 2020. ISSN 0142–0615. https://doi.org/10.1016/j.ijepes.2019.105741 . URL https://www.sciencedirect.com/science/article/pii/S0142061519319994
Mark Brian Dastas and Hwachang Song. Renewable energy generation assessment in terms of small-signal stability. Sustainability, 11 (24) (2019) ISSN 2071– 1050. https://doi.org/10.3390/su11247079 . URL https://www.mdpi.com/2071– 1050/11/24/7079
Negar Rahimi S, Park W, Choi B, Oh S, Kim Young-ho, Cho S, Ahn C, Chong D, Kim (02 2023) Cheong Jin, and Duehee Lee. A comprehensive review on ensemble solar power forecasting algorithms. J Electr Eng Technol 18:719–733. 10.1007/ s42835-023-01407-0
Kirsten D, Orwig ML, Ahlstrom V, Banunarayanan J, Sharp JM, Wilczak J, Freedman SE, Haupt J, Cline O, Bartholomy, Hendrik F, Hamann JL, Peterson (2015) David Maggio, and Melinda Marquis. Recent trends in variable generation forecasting and its value to the power system. IEEE Transactions on Sustainable Energy, 6(3): 924–933, https://doi.org/10.1109/TSTE.2014.2366118
Benti NE, Chaka MD, and Addisu Gezahegn Semie (2023). Forecasting renewable energy generation with machine learningdeep learning: Current advancesfuture prospects. Sustainability, 15(9), ISSN 2071– 1050. https://doi.org/10.3390/su15097087 . URL https://www.mdpi.com/2071-1050/15/9/7087
Prema V, Bhaskar MS, Almakhles D, Gowtham N (2022) Uma Rao. Critical review of data, models and performance metrics for wind and solar power forecast. IEEE Access 10:667–688. https://doi.org/10.1109/ACCESS.2021.3137419
Yibo Dou S, Tan, Xie D (2023) Comparison of machine learning and statistical methods in the field of renewable energy power generation forecasting: a mini review. Frontiers in Energy Research, 11, ISSN 2296-598X. https://doi.org/10.3389/fenrg.2023.1218603 . URL https://www.frontiersin.org/articles/10.3389/fenrg.2023.1218603
Yurong Wang D, Wang, Tang Y (2020) Clustered hybrid wind power prediction model based on arma, pso-svm, and clustering methods. IEEE Access 8:17071–17079. https://doi.org/10.1109/ACCESS.2020.2968390
Mohar Chattopadhyay and Debabrata Chattopadhyay. Renewable energy contingencies in power systems: Concept and case study. Energy for Sustainable Development, 54:25–35 (2020) ISSN 0973– 0826. https://doi.org/10.1016/j.esd.2019.10.006 . URL https://www.sciencedirect.com/science/article/pii/S0973082619312797
Shadi Goodarzi H, Niles Perera, Bunn D (2019) The impact of renewable energy forecast errors on imbalance volumes and electricity spot prices. Energy Policy, 134:110827, ISSN 0301–4215. https://doi.org/10.1016/j.enpol.2019.06.035 . URL https://www.sciencedirect.com/science/article/pii/S0301421519304057
Audun Botterud Z, Zhou J, Wang RJ, Bessa H, Keko (2012) Jean Sumaili, and Vladimiro Miranda. Wind power trading under uncertainty in lmp markets. IEEE Trans Power Syst 27(2):894–903. https://doi.org/10.1109/TPWRS.2011.2170442
Song P, Xu Z, Dong H, Cai H, Xie Z (2017) Security-constrained line loss minimization in distribution systems with high penetration of renewable energy using upfc. Journal of Modern Power Systems and Clean Energy, 5:876–886, URL https://api.semanticscholar.org/CorpusID:67283318
Xu Y, Dong ZY, Zhao JH, Zhang P, Kit Po Wong (2012) A reliable intelligent system for real-time dynamic security assessment of power systems. IEEE Trans Power Syst 27(3):1253–1263. https://doi.org/10.1109/TPWRS.2012.2183899
Ciapessoni E, Cirio D, Massucco S, Morini A, Pitto A, Silvestro F (2017) Risk-based dynamic security assessment for power system operation and operational planning. Energies, 10(4), ISSN 1996– 1073. https://doi.org/10.3390/en10040475 . URL https://www.mdpi.com/1996-1073/10/4/475
Ming Ni JD, McCalley V, Vittal, Tayyib T (2003) Online risk-based security assessment. IEEE Trans Power Syst 18(1):258–265. https://doi.org/10.1109/TPWRS.2002.807091
Keller J, Kroposki B (2010) Understanding fault characteristics of inverter-based distributed energy resources. Technical Report TP-550-46698, NREL
Belwin J, Brearley, Raja Prabu R (2017) A review on issues and approaches for microgrid protection. Renewable and Sustainable Energy Reviews, 67:988–997, ISSN 1364– 0321. https://doi.org/10.1016/j.rser.2016.09.047 . URL https://www.sciencedirect.com/science/article/pii/S1364032116305354
Vasileios Papaspiliotopoulos V, Kleftakis P, Kotsampopoulos (2014) George Korres, and Nikos Hatziargyriou. Hardware-in-the-loop simulation for protection blinding and sympathetic tripping in distribution grids with high penetration of distributed generation. In MedPower page 6 pp., 2014. https://doi.org/10.1049/cp.2014.1699
Bahram Shakerighadi S, Peyghami E, Ebrahimzadeh (2020) Frede Blaabjerg, and Claus Leth Back. A new guideline for security assessment of power systems with a high penetration of wind turbines. Applied Sciences, 10(9), ISSN 2076–3417. https://doi.org/10.3390/app10093190 . URL https://www.mdpi.com/2076-3417/10/9/3190
Rahmann JV-HC, Valencia F, Strunz K (2021) Analysis and application of quasi-static and dynamic phasor calculus for stability assessment of integrated power electric and electronic systems. IEEE Trans Power Syst 36(3):1750–1760. https://doi.org/10.1109/TPWRS.2020.3030225
Uros Kerin R, Balaurescu F, Lazar R, Krebs, and Florin Balasiu (2012). Dynamic security assessment in system operationplanning — first experiences. In 2012 IEEE PowerEnergy Society General Meeting, pages 1–6, https://doi.org/10.1109/PESGM.2012.6344888
ENTSO-E RG-CE System Protection & Dynamics Sub Group (2017) Spd dsa task force dynamic security assessment (dsa). Technical report, ENTSO-e
Tiantian Qian F, Shi K, Wang S, Yang J, Geng Y, Li, Wu Q (2022) N-1 static security assessment method for power grids with high penetration rate of renewable energy generation. Electric Power Systems Research, 211:108200, ISSN 0378–7796. https://doi.org/10.1016/j.epsr.2022.108200 . URL https://www.sciencedirect.com/science/article/pii/S0378779622004096
Hailu EA, Nyakoe GN, Maina C, Muriithi (2023) Techniques of power system static security assessment and improvement: A literature survey. Heliyon, 9(3):e14524, ISSN 2405–8440. https://doi.org/10.1016/j.heliyon.2023 . e14524. URL https://www.sciencedirect.com/science/article/pii/S2405844023017310
Antonio RS, Parmezan, Vinicius MA, Souza, Gustavo EAPA, Batista (2019) Evaluation of statistical and machine learning models for time series prediction: Identifying the state-of-the-art and the best conditions for the use of each model. Information Sciences, 484:302–337, ISSN 0020–0255. https://doi.org/10.1016/j.ins.2019.01.076 . URL https://www.sciencedirect.com/science/article/pii/S0020025519300945
Giorgi MGD, Ficarella A, Tarantino M (2011) Error analysis of short term wind power prediction models. Applied Energy, 88(4):1298–1311, ISSN 0306–2619. https://doi.org/10.1016/j.apenergy.2010.10.035 . URL https://www.sciencedirect.com/science/article/pii/S030626191000437X
Hossein Sangrody M, Sarailoo N, Zhou N, Tran M, Motalleb, Foruzan E (2017) Weather forecasting error in solar energy forecasting. IET Renew Power Gener 11:06. https://doi.org/10.1049/iet-rpg.2016.1043
Hasan KN, Preece R and Jovica V. Milanovi´c. Existing approaches and trends in uncertainty modelling and probabilistic stability analysis of power systems with renewable generation. Renewable and Sustainable Energy Reviews, 101:168–180, 2019. ISSN 1364– 0321. https://doi.org/10.1016/j.rser.2018.10.027 . URL https://www.sciencedirect.com/science/article/pii/S1364032118307317
Ramakrishnan Venkatraman SK, Khaitan, Ajjarapu V (2018) Impact of distribution generation penetration on power system dynamics considering voltage ride-through requirements. In 2018 IEEE Power & Energy Society General Meeting (PESGM), pages 1–5, https://doi.org/10.1109/PESGM.2018.8585776
Yadav M, Pal N, and Devender Kumar Saini (2023). Low voltage ride through capability for resilient electrical distribution system integrated with renewable energy resources. Energy Reports, 9:833–858, ISSN 2352–4847. https://doi.org/10.1016/j.egyr.2022.12.023 . URL https://www.sciencedirect.com/science/article/pii/S2352484722026233
Fay¸cal Benyamina A, Benrabah F, Khoucha MF, Zia (2021) Yahia Achour, and Mohamed Benbouzid. Online current limiting-based control to improve fault ride-through capability of grid-feeding inverters. Electric Power Systems Research, 201:107524, ISSN 0378–7796. https://doi.org/10.1016/j.epsr.2021.107524 . URL https://www.sciencedirect.com/science/article/pii/S0378779621005058
Ravikiran Hiremath and Tukaram Moger (2020) Comprehensive review on low voltage ride through capability of wind turbine generators. Int Trans Electr Energy Syst 30:07. https://doi.org/10.1002/2050-7038.12524
Rishabh Jain YN, Velaga K, Prabakar M, Baggu, Schneider K (2022) Modern trends in power system protection for distribution grid with high der penetration. e-Prime - Advances in Electrical Engineering, Electronics and Energy, 2:100080, ISSN 2772–6711. https://doi.org/10.1016/j.prime.2022.100080 . URL https://www.sciencedirect.com/science/article/pii/S2772671122000523
Ma K, Chen Z, Liu Z, Bak CL, Manuel, Castillo (2021) Protection collaborative fault control for power electronic-based power plants during unbalanced grid faults. International Journal of Electrical Power & Energy Systems, 130:107009, ISSN 0142–0615. https://doi.org/10.1016/j.ijepes.2021.107009 . URL https://www.sciencedirect.com/science/article/pii/S0142061521002490
Doyle MT (2002) Reviewing the impacts of distributed generation on distribution system protection. In IEEE Power Engineering Society Summer Meeting, volume 1, pages 103–105 vol.1, https://doi.org/10.1109/PESS.2002.1043186
Bo Wen D, Boroyevich R, Burgos P, Mattavelli, Shen Z (2016) Analysis of d-q small-signal impedance of grid-tied inverters. IEEE Trans Power Electron 31(1):675–687. https://doi.org/10.1109/TPEL.2015.2398192
Zhangxin Zhou W, Wang D, Ramasubramanian E, Farantatos, Garng M (2023) Huang. Small signal stability of phase locked loop based current-controlled inverter in 100. IEEE Trans Sustain Energy 14(3):1612–1623. https://doi.org/10.1109/TSTE.2023.3241094
Hossam S, Salama A, Bakeer G, Magdy, Vokony I (2021) Virtual inertia emulation through virtual synchronous generator based superconducting magnetic energy storage in modern power system. Journal of Energy Storage, 44:103466, ISSN 2352-152X. https://doi.org/10.1016/j.est.2021.103466 . URL https://www.sciencedirect.com/science/article/pii/S2352152X21011506
Chen Y, Wang W (2023) A novel improved droop control for grid-supporting inverter combined with the virtual synchronous generator control. Journal of Electrical Engineering & Technology, 18:1601–1611, ISSN 2093–7423. https://doi.org/10.1007/s42835-022-01297-8 . URL https://doi.org/10.1007/s42835-022-01297-8
Ngoc-Bao Lai A, Tarras´o GN, Baltas LV (2021) Marin Arevalo, and Pedro Rodriguez. External inertia emulation controller for grid-following power converter. IEEE Trans Ind Appl 57(6):6568–6576. https://doi.org/10.1109/TIA.2021.3108350
Federico Milano F, D¨orfler G, Hug DJ, Hill, Verbiˇc G (2018) Foundations and challenges of low-inertia systems (invited paper). In 2018 Power Systems Computation Conference (PSCC), pages 1–25, 2018. https://doi.org/10.23919/PSCC . 8450880
Suud Ademnur, Hasen (2024) O¨ mer Aydın, Saffet Ayasun, and S¸ahin S¨onmez. Impact of virtual inertia and damping control on stability delay margins of load frequency control systems with renewable energy sources. Electr Eng 106:323–341. https://doi.org/10.1007/s00202-023-01984-3
Baraa Mohandes MSE, Moursi N, Hatziargyriou, and Sameh El Khatib (2019). A review of power system flexibility with high penetration of renewables. IEEE Transactions on Power Systems, 34(4):3140–3155, doi: 10. 1109/TPWRS.2019.2897727
Ioannis D, Margaris SA, Papathanassiou ND, Hatziargyriou AD, Hansen, Sorensen P (2012) Frequency control in autonomous power systems with high wind power penetration. IEEE Trans Sustain Energy 3(2):189–199. https://doi.org/10.1109/TSTE.2011.2174660
Debra Lew G, Brinkman E, Ibanez BM, Hodge, King J (2012) The western wind and solar integration study phase 2 preprint. Technical report, 09
Wei Wang B, Yuan Q, Sun, Wennersten R (2022) Application of energy storage in integrated energy systems — a solution to fluctuation and uncertainty of renewable energy. J Energy Storage 52:104812. https://doi.org/10.1016/j.est.2022.104812.URL . https://www.sciencedirect.com/science/article/pii/S2352152X22008210 ISSN 2352-152X
Lee J, Yoon YT, Yong-Beum, Yoon (09 2023) Comparison of stabilization effects of variable pumping storage hydro (vpsh) and bess on renewable generation oriented power system. J Electr Eng Technol 18:3375–3389. 10.1007/ s42835-023-01404-3
Heesung Moon S, Chung, Jang G (02 2024) Analysis of power system flexibility considering power system ramp rate. J Electr Eng Technol 19:867–876. https://doi.org/10.1007/s42835-024-01815-w
Leila Bird M, Milligan, Lew D (2013) Integrating variable renewable energy: Challenges and solutions. Technical report, 09
Safayet A, Fajri P, Husain I (2017) Reactive power management for overvoltage prevention at high pv penetration in a low-voltage distribution system. IEEE Trans Ind Appl 53(6):5786–5794. https://doi.org/10.1109/TIA.2017.2741925
Niu F, Xu G, Zhang J, Liu Y, Wang W (2022) Research on active and reactive power coordination control strategy for overvoltage of pv distribution network in high penetration area. In 2022 5th International Conference on Power and Energy Applications (ICPEA), pages 13–18, https://doi.org/10.1109/ICPEA56363.2022.10052609
Lee Y, Song H (2019) A reactive power compensation strategy for voltage stability challenges in the korean power system with dynamic loads. Sustainability, 11(2), ISSN 2071– 1050. https://doi.org/10.3390/su11020326 . URL https://www.mdpi.com/2071-1050/11/2/326
Hossain MJ, Pota HR, Mahmud MA, Rodrigo A, Ramos (2012) Investigation of the impacts of large-scale wind power penetration on the angle and voltage stability of power systems. IEEE Syst J 6(1):76–84. https://doi.org/10.1109/JSYST.2011.2162991
Faraedoon Ahmed DA, Kez Se´an, McLoone RJ, Best (2023) Ch´e Cameron, and Aoife Foley. Dynamic grid stability in low carbon power systems with minimum inertia. Renewable Energy, 210:486–506, ISSN 0960–1481. https://doi.org/10.1016/j.renene.2023.03.082 . URL https://www.sciencedirect.com/science/article/pii/S0960148123003774
Soni EirGrid (2016) Rocof alternative & complementary solutions project, phase 2 study report. Technical report, Tech. rep., Eirgrid
Daniel Fern´andez-Mun˜oz JI, P´erez-D´ıaz I, Guis´andez Manuel Chazarra, and A´lvaroFern´andez-Espina. Fast frequency control ancillary services: An international review. Renewable and Sustainable Energy Reviews, 120:109662, 2020. ISSN 1364– 0321. https://doi.org/10.1016/j.rser.2019.109662 . URL https://www.sciencedirect.com/science/article/pii/S1364032119308676
Download references
Acknowledgements
This work has been supported by Seoul National University of Science and Technology.
Author information
Authors and affiliations.
Department of Electrical and Information Engineering, Seoul National University of Science and Technology, Nowon-gu, Seoul, 01811, Republic of Korea
Dwi Riana Aryani & Hwachang Song
You can also search for this author in PubMed Google Scholar
Corresponding author
Correspondence to Hwachang Song .
Ethics declarations
Competing interests.
On behalf of all authors, the corresponding author states that there is no conflict of interest.
Additional information
Publisher’s note.
Springer Nature remains neutral with regard to jurisdictional claims in published maps and institutional affiliations.
Rights and permissions
Springer Nature or its licensor (e.g. a society or other partner) holds exclusive rights to this article under a publishing agreement with the author(s) or other rightsholder(s); author self-archiving of the accepted manuscript version of this article is solely governed by the terms of such publishing agreement and applicable law.
Reprints and permissions
About this article
Aryani, D.R., Song, H. A Review on Power System Security Issues in the High Renewable Energy Penetration Environment. J. Electr. Eng. Technol. (2024). https://doi.org/10.1007/s42835-024-02028-x
Download citation
Received : 06 May 2024
Revised : 10 August 2024
Accepted : 19 August 2024
Published : 12 September 2024
DOI : https://doi.org/10.1007/s42835-024-02028-x
Share this article
Anyone you share the following link with will be able to read this content:
Sorry, a shareable link is not currently available for this article.
Provided by the Springer Nature SharedIt content-sharing initiative
- High renewable energy penetration
- Low inertia
- Uncertainty
- Find a journal
- Publish with us
- Track your research

IMAGES
VIDEO
COMMENTS
Acknowledgments Parts of these course notes are loosely based on lecture notes by Professors Daniel Liberzon, Sean Meyn, and Mark Spong (University of Illinois), on notes by Professors Daniel Davison and Daniel Miller (University of Waterloo), and on parts of the textbook Feedback Control of Dynamic Systems (5th edition) by Franklin, Powell and Emami-Naeini. I claim credit for all typos and ...
In this chapter on complete case studies, we will employ the methods of the preceding chapters to design the following: Design for the positioning system of a tracking radar which illustrates both linear and nonlinear design considerations jointly. Design of the angular control system for a robot's joint. State-variable design for the controller and full-order estimator for a space satellite ...
This chapter gives a concise presentation of expert control on the basis of a generic architecture that involves the operator, the expert system, the control algorithm, auxiliary units (parameter estimator, state estimator, fault detector) and the plant under control. Particular aspects covered are knowledge representation, knowledge ...
Case study analysis of how fixing a blind spot in the process design helped user requirements for a heat exchanger control system.
Drawing on the growing literature that has addressed the role of Management Control Systems in innovation and the literature on institutional theory, this study explores the case of Amorim Cork Composites to analyse how the situated rationalities within the company get reflected in the management control practices in use, and then how these practices are used to communicate and provide ...
Case Studies in Control presents a framework to facilitate the use of advanced control concepts in real systems based on two decades of research and over 150 successful applications for industrial end-users from various backgrounds. In successive parts the text approaches the problem of putting the theory to work from both ends, theoretical and ...
About this book. "Robust Control Systems" gives a self-contained introduction to modern Control Theory. It thus adds a textbook to the existing research-oriented literature on Robust Control. The author lays emphasis on the modern aspects of the design of controllers with prescribed performance and robustness properties.
This longitudinal case study traces the experience of a real estate fund management company in addressing the need to make sense of increasing external complexity and its effort to design a management control system to support top management in this task.
Hands-on experimentation has widely demonstrated its efficacy in engineering training, especially in control formation, since experimentation using computer-aided control system design (CACSD) tools is essential for future engineers. In this context, this article describes a case study for Control Engineering formation, based on a new lab practice for the linear and angular velocity control ...
Management Control Systems: Mini Cases For his course on Implementing Strategy: Management Control Systems, Vijay Govindarajan uses mini-cases to illustrate how the design and implementation of ongoing management systems are used to plan and control a company's performance.
Case Study: Designing Centralized Protection and Control Systems for a Distribution Substation at Duke Energy Stephen B. Ladd, Taylor I. Raffield, and Ethan Haithcox
Case study: Commissioning a solar power plant Commissioning an integrated security system for solar power plant in Saudi Arabia was successful despite the challenges…
PDF | On Dec 1, 2019, Goh Chin Fei and others published A Case Study of Management Control Systems in Two Japanese Overseas R&D Organizations | Find, read and cite all the research you need on ...
Case studies can be used as an aid in teaching control systems to undergraduate students. A number of case studies, of differing styles, are described. Wherever possible the case studies make use of readily available software packages as analysis and design tools. A major case study considers the plastic injection moulding process, as one which ...
System Controller. Must take inputs from all the Cab controllers, floor control panels. It has both hard and soft real-time tasks. It must monitor all the moving elevators to make sure that they stop properly. Choose which elevator to dispatch to a request. Each elevator has a point-to-point connect to system controller.
This paper addresses the latter area where case studies focusing on control law design and evaluation, including experimental results in one case, are reported. These demonstrate that movement towards the actual deployment of multidimensional control systems is increasing.
Control System Case Studies From automotive and food processing to oil rigs and cranes, we've installed best-in-class electrical control systems into a wide range of industry sectors. Check out our case studies to find out more about some of the recent projects we've worked on.
Abstract Qualitative case study methodology enables researchers to conduct an in-depth exploration of intricate phenomena within some specific context. By keeping in mind research students, this article presents a systematic step-by-step guide to conduct a case study in the business discipline. Research students belonging to said discipline face issues in terms of clarity, selection, and ...
Abstract. This case study presented the design of an Industrial Control System (ICS). The design focused on network security using network segmentation and redundancy. The objective was to relate ...
Incorporating a series of analytical steps, from data extraction and quality control to the generation of low-dimensional representations and to longitudinal analyses, an open-source software is ...
Impact Analysis of Cyberattacks in Electric Propulsion Systems for Hybrid Tracked Vehicles 2024-01-4114. ... Particularly vulnerable are the control units, which have become prime targets for adversarial exploitation due to their pivotal role in vehicle functionality. ... this study demonstrates two distinct yet equally harmful scenarios using ...
We performed a case-control study, including 46 women with PCOS and 48 controls. Genotypes of VDR genes were determined using the PCR-RFLP (restriction fragment length polymorphism) method. In all women, the waist circumference, parameters of lipid and glucose metabolism were evaluated.
Background Effective vaccination governance in conflict-affected regions poses unique challenges. This study evaluates the governance of vaccination programs in northwest Syria, focusing on effectiveness, efficiency, inclusiveness, data availability, vision, transparency, accountability, and sustainability. Methods Using a mixed-methods approach, and adapting Siddiqi's framework for health ...
This paper aims to provide an overview of several security issues on power systems, along with challenges arising from the impact of inertial reduction, RE fluctuations, RE prediction errors, and fault response, addressed to researchers as a reference for further studies. Case studies of security issues experienced by several system operators ...