

The Future of AI Research: 20 Thesis Ideas for Undergraduate Students in Machine Learning and Deep Learning for 2023!
A comprehensive guide for crafting an original and innovative thesis in the field of ai..
By Aarafat Islam on 2023-01-11
“The beauty of machine learning is that it can be applied to any problem you want to solve, as long as you can provide the computer with enough examples.” — Andrew Ng
This article provides a list of 20 potential thesis ideas for an undergraduate program in machine learning and deep learning in 2023. Each thesis idea includes an introduction , which presents a brief overview of the topic and the research objectives . The ideas provided are related to different areas of machine learning and deep learning, such as computer vision, natural language processing, robotics, finance, drug discovery, and more. The article also includes explanations, examples, and conclusions for each thesis idea, which can help guide the research and provide a clear understanding of the potential contributions and outcomes of the proposed research. The article also emphasized the importance of originality and the need for proper citation in order to avoid plagiarism.
1. Investigating the use of Generative Adversarial Networks (GANs) in medical imaging: A deep learning approach to improve the accuracy of medical diagnoses.
Introduction: Medical imaging is an important tool in the diagnosis and treatment of various medical conditions. However, accurately interpreting medical images can be challenging, especially for less experienced doctors. This thesis aims to explore the use of GANs in medical imaging, in order to improve the accuracy of medical diagnoses.
2. Exploring the use of deep learning in natural language generation (NLG): An analysis of the current state-of-the-art and future potential.
Introduction: Natural language generation is an important field in natural language processing (NLP) that deals with creating human-like text automatically. Deep learning has shown promising results in NLP tasks such as machine translation, sentiment analysis, and question-answering. This thesis aims to explore the use of deep learning in NLG and analyze the current state-of-the-art models, as well as potential future developments.
3. Development and evaluation of deep reinforcement learning (RL) for robotic navigation and control.
Introduction: Robotic navigation and control are challenging tasks, which require a high degree of intelligence and adaptability. Deep RL has shown promising results in various robotics tasks, such as robotic arm control, autonomous navigation, and manipulation. This thesis aims to develop and evaluate a deep RL-based approach for robotic navigation and control and evaluate its performance in various environments and tasks.
4. Investigating the use of deep learning for drug discovery and development.
Introduction: Drug discovery and development is a time-consuming and expensive process, which often involves high failure rates. Deep learning has been used to improve various tasks in bioinformatics and biotechnology, such as protein structure prediction and gene expression analysis. This thesis aims to investigate the use of deep learning for drug discovery and development and examine its potential to improve the efficiency and accuracy of the drug development process.
5. Comparison of deep learning and traditional machine learning methods for anomaly detection in time series data.
Introduction: Anomaly detection in time series data is a challenging task, which is important in various fields such as finance, healthcare, and manufacturing. Deep learning methods have been used to improve anomaly detection in time series data, while traditional machine learning methods have been widely used as well. This thesis aims to compare deep learning and traditional machine learning methods for anomaly detection in time series data and examine their respective strengths and weaknesses.
Photo by Joanna Kosinska on Unsplash
6. Use of deep transfer learning in speech recognition and synthesis.
Introduction: Speech recognition and synthesis are areas of natural language processing that focus on converting spoken language to text and vice versa. Transfer learning has been widely used in deep learning-based speech recognition and synthesis systems to improve their performance by reusing the features learned from other tasks. This thesis aims to investigate the use of transfer learning in speech recognition and synthesis and how it improves the performance of the system in comparison to traditional methods.
7. The use of deep learning for financial prediction.
Introduction: Financial prediction is a challenging task that requires a high degree of intelligence and adaptability, especially in the field of stock market prediction. Deep learning has shown promising results in various financial prediction tasks, such as stock price prediction and credit risk analysis. This thesis aims to investigate the use of deep learning for financial prediction and examine its potential to improve the accuracy of financial forecasting.
8. Investigating the use of deep learning for computer vision in agriculture.
Introduction: Computer vision has the potential to revolutionize the field of agriculture by improving crop monitoring, precision farming, and yield prediction. Deep learning has been used to improve various computer vision tasks, such as object detection, semantic segmentation, and image classification. This thesis aims to investigate the use of deep learning for computer vision in agriculture and examine its potential to improve the efficiency and accuracy of crop monitoring and precision farming.
9. Development and evaluation of deep learning models for generative design in engineering and architecture.
Introduction: Generative design is a powerful tool in engineering and architecture that can help optimize designs and reduce human error. Deep learning has been used to improve various generative design tasks, such as design optimization and form generation. This thesis aims to develop and evaluate deep learning models for generative design in engineering and architecture and examine their potential to improve the efficiency and accuracy of the design process.
10. Investigating the use of deep learning for natural language understanding.
Introduction: Natural language understanding is a complex task of natural language processing that involves extracting meaning from text. Deep learning has been used to improve various NLP tasks, such as machine translation, sentiment analysis, and question-answering. This thesis aims to investigate the use of deep learning for natural language understanding and examine its potential to improve the efficiency and accuracy of natural language understanding systems.
Photo by UX Indonesia on Unsplash
11. Comparing deep learning and traditional machine learning methods for image compression.
Introduction: Image compression is an important task in image processing and computer vision. It enables faster data transmission and storage of image files. Deep learning methods have been used to improve image compression, while traditional machine learning methods have been widely used as well. This thesis aims to compare deep learning and traditional machine learning methods for image compression and examine their respective strengths and weaknesses.
12. Using deep learning for sentiment analysis in social media.
Introduction: Sentiment analysis in social media is an important task that can help businesses and organizations understand their customers’ opinions and feedback. Deep learning has been used to improve sentiment analysis in social media, by training models on large datasets of social media text. This thesis aims to use deep learning for sentiment analysis in social media, and evaluate its performance against traditional machine learning methods.
13. Investigating the use of deep learning for image generation.
Introduction: Image generation is a task in computer vision that involves creating new images from scratch or modifying existing images. Deep learning has been used to improve various image generation tasks, such as super-resolution, style transfer, and face generation. This thesis aims to investigate the use of deep learning for image generation and examine its potential to improve the quality and diversity of generated images.
14. Development and evaluation of deep learning models for anomaly detection in cybersecurity.
Introduction: Anomaly detection in cybersecurity is an important task that can help detect and prevent cyber-attacks. Deep learning has been used to improve various anomaly detection tasks, such as intrusion detection and malware detection. This thesis aims to develop and evaluate deep learning models for anomaly detection in cybersecurity and examine their potential to improve the efficiency and accuracy of cybersecurity systems.
15. Investigating the use of deep learning for natural language summarization.
Introduction: Natural language summarization is an important task in natural language processing that involves creating a condensed version of a text that preserves its main meaning. Deep learning has been used to improve various natural language summarization tasks, such as document summarization and headline generation. This thesis aims to investigate the use of deep learning for natural language summarization and examine its potential to improve the efficiency and accuracy of natural language summarization systems.
Photo by Windows on Unsplash
16. Development and evaluation of deep learning models for facial expression recognition.
Introduction: Facial expression recognition is an important task in computer vision and has many practical applications, such as human-computer interaction, emotion recognition, and psychological studies. Deep learning has been used to improve facial expression recognition, by training models on large datasets of images. This thesis aims to develop and evaluate deep learning models for facial expression recognition and examine their performance against traditional machine learning methods.
17. Investigating the use of deep learning for generative models in music and audio.
Introduction: Music and audio synthesis is an important task in audio processing, which has many practical applications, such as music generation and speech synthesis. Deep learning has been used to improve generative models for music and audio, by training models on large datasets of audio data. This thesis aims to investigate the use of deep learning for generative models in music and audio and examine its potential to improve the quality and diversity of generated audio.
18. Study the comparison of deep learning models with traditional algorithms for anomaly detection in network traffic.
Introduction: Anomaly detection in network traffic is an important task that can help detect and prevent cyber-attacks. Deep learning models have been used for this task, and traditional methods such as clustering and rule-based systems are widely used as well. This thesis aims to compare deep learning models with traditional algorithms for anomaly detection in network traffic and analyze the trade-offs between the models in terms of accuracy and scalability.
19. Investigating the use of deep learning for improving recommender systems.
Introduction: Recommender systems are widely used in many applications such as online shopping, music streaming, and movie streaming. Deep learning has been used to improve the performance of recommender systems, by training models on large datasets of user-item interactions. This thesis aims to investigate the use of deep learning for improving recommender systems and compare its performance with traditional content-based and collaborative filtering approaches.
20. Development and evaluation of deep learning models for multi-modal data analysis.
Introduction: Multi-modal data analysis is the task of analyzing and understanding data from multiple sources such as text, images, and audio. Deep learning has been used to improve multi-modal data analysis, by training models on large datasets of multi-modal data. This thesis aims to develop and evaluate deep learning models for multi-modal data analysis and analyze their potential to improve performance in comparison to single-modal models.
I hope that this article has provided you with a useful guide for your thesis research in machine learning and deep learning. Remember to conduct a thorough literature review and to include proper citations in your work, as well as to be original in your research to avoid plagiarism. I wish you all the best of luck with your thesis and your research endeavors!
Continue Learning
Are we building ai systems that learned to lie to us.
DeepFakes = DeepLearning + Fake
MidJourney Lighting Guide: Tips and Advice
Role of artificial intelligence in metaverse.
Exploring the Saga of Metaverse with AI
All You Need To Know About Face Swap and Face Swap AI Tools
Top 10 trending ai/ml research papers you must read, wondershare virbo reviewed: the best ai video creator.

Research Topics & Ideas
Artifical Intelligence (AI) and Machine Learning (ML)
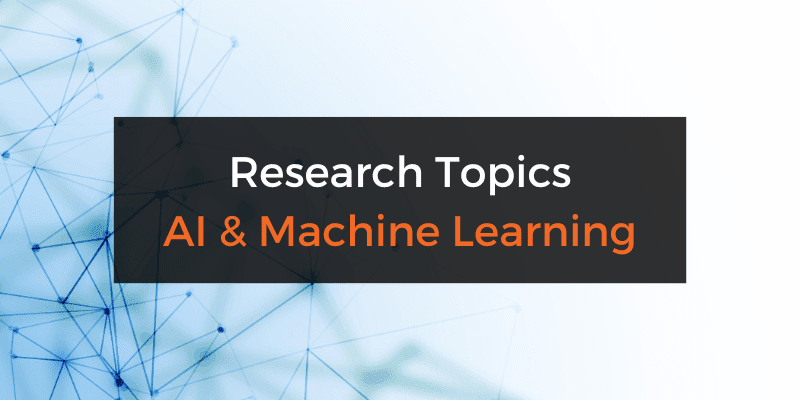
If you’re just starting out exploring AI-related research topics for your dissertation, thesis or research project, you’ve come to the right place. In this post, we’ll help kickstart your research topic ideation process by providing a hearty list of research topics and ideas , including examples from past studies.
PS – This is just the start…
We know it’s exciting to run through a list of research topics, but please keep in mind that this list is just a starting point . To develop a suitable research topic, you’ll need to identify a clear and convincing research gap , and a viable plan to fill that gap.
If this sounds foreign to you, check out our free research topic webinar that explores how to find and refine a high-quality research topic, from scratch. Alternatively, if you’d like hands-on help, consider our 1-on-1 coaching service .
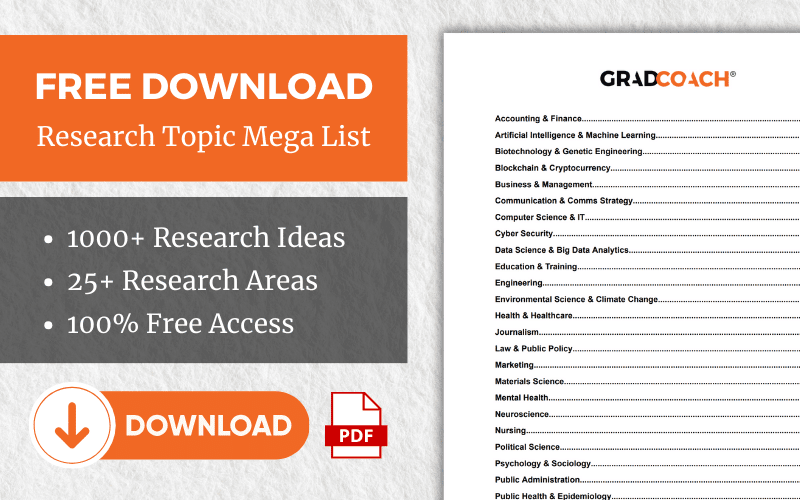
AI-Related Research Topics & Ideas
Below you’ll find a list of AI and machine learning-related research topics ideas. These are intentionally broad and generic , so keep in mind that you will need to refine them a little. Nevertheless, they should inspire some ideas for your project.
- Developing AI algorithms for early detection of chronic diseases using patient data.
- The use of deep learning in enhancing the accuracy of weather prediction models.
- Machine learning techniques for real-time language translation in social media platforms.
- AI-driven approaches to improve cybersecurity in financial transactions.
- The role of AI in optimizing supply chain logistics for e-commerce.
- Investigating the impact of machine learning in personalized education systems.
- The use of AI in predictive maintenance for industrial machinery.
- Developing ethical frameworks for AI decision-making in healthcare.
- The application of ML algorithms in autonomous vehicle navigation systems.
- AI in agricultural technology: Optimizing crop yield predictions.
- Machine learning techniques for enhancing image recognition in security systems.
- AI-powered chatbots: Improving customer service efficiency in retail.
- The impact of AI on enhancing energy efficiency in smart buildings.
- Deep learning in drug discovery and pharmaceutical research.
- The use of AI in detecting and combating online misinformation.
- Machine learning models for real-time traffic prediction and management.
- AI applications in facial recognition: Privacy and ethical considerations.
- The effectiveness of ML in financial market prediction and analysis.
- Developing AI tools for real-time monitoring of environmental pollution.
- Machine learning for automated content moderation on social platforms.
- The role of AI in enhancing the accuracy of medical diagnostics.
- AI in space exploration: Automated data analysis and interpretation.
- Machine learning techniques in identifying genetic markers for diseases.
- AI-driven personal finance management tools.
- The use of AI in developing adaptive learning technologies for disabled students.
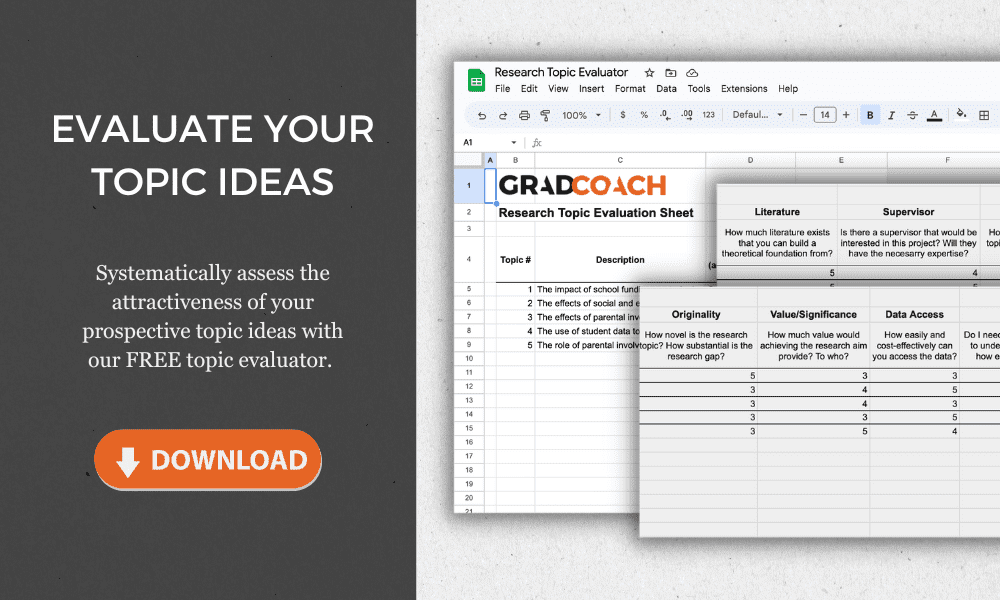
AI & ML Research Topic Ideas (Continued)
- Machine learning in cybersecurity threat detection and response.
- AI applications in virtual reality and augmented reality experiences.
- Developing ethical AI systems for recruitment and hiring processes.
- Machine learning for sentiment analysis in customer feedback.
- AI in sports analytics for performance enhancement and injury prevention.
- The role of AI in improving urban planning and smart city initiatives.
- Machine learning models for predicting consumer behaviour trends.
- AI and ML in artistic creation: Music, visual arts, and literature.
- The use of AI in automated drone navigation for delivery services.
- Developing AI algorithms for effective waste management and recycling.
- Machine learning in seismology for earthquake prediction.
- AI-powered tools for enhancing online privacy and data protection.
- The application of ML in enhancing speech recognition technologies.
- Investigating the role of AI in mental health assessment and therapy.
- Machine learning for optimization of renewable energy systems.
- AI in fashion: Predicting trends and personalizing customer experiences.
- The impact of AI on legal research and case analysis.
- Developing AI systems for real-time language interpretation for the deaf and hard of hearing.
- Machine learning in genomic data analysis for personalized medicine.
- AI-driven algorithms for credit scoring in microfinance.
- The use of AI in enhancing public safety and emergency response systems.
- Machine learning for improving water quality monitoring and management.
- AI applications in wildlife conservation and habitat monitoring.
- The role of AI in streamlining manufacturing processes.
- Investigating the use of AI in enhancing the accessibility of digital content for visually impaired users.
Recent AI & ML-Related Studies
While the ideas we’ve presented above are a decent starting point for finding a research topic in AI, they are fairly generic and non-specific. So, it helps to look at actual studies in the AI and machine learning space to see how this all comes together in practice.
Below, we’ve included a selection of AI-related studies to help refine your thinking. These are actual studies, so they can provide some useful insight as to what a research topic looks like in practice.
- An overview of artificial intelligence in diabetic retinopathy and other ocular diseases (Sheng et al., 2022)
- HOW DOES ARTIFICIAL INTELLIGENCE HELP ASTRONOMY? A REVIEW (Patel, 2022)
- Editorial: Artificial Intelligence in Bioinformatics and Drug Repurposing: Methods and Applications (Zheng et al., 2022)
- Review of Artificial Intelligence and Machine Learning Technologies: Classification, Restrictions, Opportunities, and Challenges (Mukhamediev et al., 2022)
- Will digitization, big data, and artificial intelligence – and deep learning–based algorithm govern the practice of medicine? (Goh, 2022)
- Flower Classifier Web App Using Ml & Flask Web Framework (Singh et al., 2022)
- Object-based Classification of Natural Scenes Using Machine Learning Methods (Jasim & Younis, 2023)
- Automated Training Data Construction using Measurements for High-Level Learning-Based FPGA Power Modeling (Richa et al., 2022)
- Artificial Intelligence (AI) and Internet of Medical Things (IoMT) Assisted Biomedical Systems for Intelligent Healthcare (Manickam et al., 2022)
- Critical Review of Air Quality Prediction using Machine Learning Techniques (Sharma et al., 2022)
- Artificial Intelligence: New Frontiers in Real–Time Inverse Scattering and Electromagnetic Imaging (Salucci et al., 2022)
- Machine learning alternative to systems biology should not solely depend on data (Yeo & Selvarajoo, 2022)
- Measurement-While-Drilling Based Estimation of Dynamic Penetrometer Values Using Decision Trees and Random Forests (García et al., 2022).
- Artificial Intelligence in the Diagnosis of Oral Diseases: Applications and Pitfalls (Patil et al., 2022).
- Automated Machine Learning on High Dimensional Big Data for Prediction Tasks (Jayanthi & Devi, 2022)
- Breakdown of Machine Learning Algorithms (Meena & Sehrawat, 2022)
- Technology-Enabled, Evidence-Driven, and Patient-Centered: The Way Forward for Regulating Software as a Medical Device (Carolan et al., 2021)
- Machine Learning in Tourism (Rugge, 2022)
- Towards a training data model for artificial intelligence in earth observation (Yue et al., 2022)
- Classification of Music Generality using ANN, CNN and RNN-LSTM (Tripathy & Patel, 2022)
As you can see, these research topics are a lot more focused than the generic topic ideas we presented earlier. So, in order for you to develop a high-quality research topic, you’ll need to get specific and laser-focused on a specific context with specific variables of interest. In the video below, we explore some other important things you’ll need to consider when crafting your research topic.
Get 1-On-1 Help
If you’re still unsure about how to find a quality research topic, check out our Research Topic Kickstarter service, which is the perfect starting point for developing a unique, well-justified research topic.
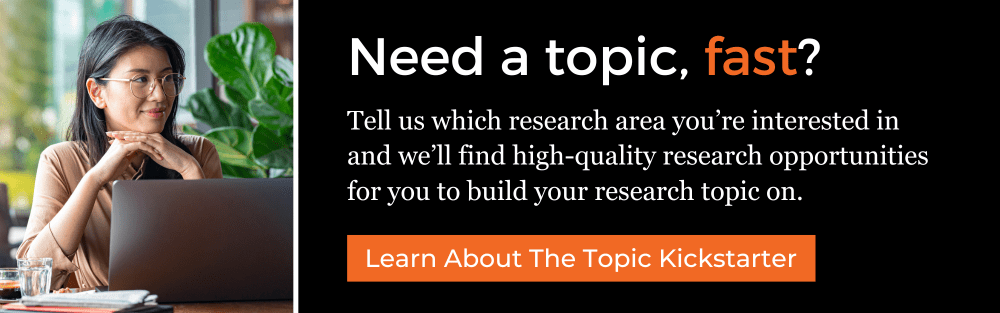
can one come up with their own tppic and get a search
can one come up with their own title and get a search
Submit a Comment Cancel reply
Your email address will not be published. Required fields are marked *
Save my name, email, and website in this browser for the next time I comment.
- Print Friendly
- Interesting
- Scholarships
- UGC-CARE Journals
50 Deep Learning Research Ideas
Deep learning Research and Project Ideas
Deep learning is a branch of artificial intelligence that uses algorithms to model high-level abstractions in data by using multiple layers of processing. It is a subset of machine learning , which is a broader field of artificial intelligence that uses algorithms to learn from data.
Deep learning algorithms are used to recognize patterns in large datasets and make predictions based on those patterns. Deep learning research focuses on developing algorithms that can learn from data in an unsupervised manner, allowing them to learn complex representations of data without relying on explicit instructions from humans.
Deep learning research also focuses on developing methods for improving the accuracy and speed of deep learning algorithms. Additionally, deep learning research explores ways to make deep learning algorithms more efficient and effective in a variety of applications.
In this article, ilovephd listed the 50 interesting research and project ideas in deep learning
Deep Learning Research Ideas
1. Developing a deep learning model to detect and classify objects in images.
2. Developing a deep learning model to detect and classify objects in videos.
3. Developing a deep learning model to detect and classify objects in 3D scenes.
4. Developing a deep learning model to detect and classify objects in audio.
5. Developing a deep learning model to detect and classify objects in text.
6. Develop a deep learning model to generate new images from a given set of images.
7. Develop a deep learning model to generate new videos from a given set of videos.
8. Develop a deep learning model to generate new 3D scenes from a given set of 3D scenes.
9. Developing a deep learning model to generate new audio from a given set of audio.
10. Developing a deep learning model to generate new text from a given set of text.
11. Developing a deep learning model to detect and classify emotions in images.
12. Developing a deep learning model to detect and classify emotions in videos.
13. Developing a deep learning model to detect and classify emotions in audio.
14. Developing a deep learning model to detect and classify emotions in text.
15. Developing a deep learning model to detect and classify objects in medical images.
16. Developing a deep learning model to detect and classify objects in medical videos.
17. Developing a deep learning model to detect and classify objects in medical audio.
18. Developing a deep learning model to detect and classify objects in medical text.
19. Developing a deep learning model to detect and classify objects in satellite images.
20. Developing a deep learning model to detect and classify objects in aerial videos.
21. Developing a deep learning model to detect and classify objects in aerial audio.
22. Developing a deep learning model to detect and classify objects in aerial text.
23. Developing a deep learning model to detect and classify objects in street view images.
24. Developing a deep learning model to detect and classify objects in street view videos.
25. Developing a deep learning model to detect and classify objects in street view audio.
26. Developing a deep learning model to detect and classify objects in street view text.
27. Developing a deep learning model to detect and classify objects in industrial images.
28. Developing a deep learning model to detect and classify objects in industrial videos.
29. Developing a deep learning model to detect and classify objects in industrial audio.
30. Developing a deep learning model to detect and classify objects in industrial text.
31. Developing a deep learning model to detect and classify objects in autonomous vehicle images.
32. Developing a deep learning model to detect and classify objects in autonomous vehicle videos.
33. Developing a deep learning model to detect and classify objects in autonomous vehicle audio.
34. Developing a deep learning model to detect and classify objects in autonomous vehicle text.
35. Developing a deep learning model to detect and classify objects in robotics images.
36. Developing a deep learning model to detect and classify objects in robotics videos.
37. Developing a deep learning model to detect and classify objects in robotics audio.
38. Developing a deep learning model to detect and classify objects in robotics text.
39. Developing a deep learning model to detect and classify objects in natural language processing.
40. Developing a deep learning model to detect and classify objects in computer vision.
41. Developing a deep learning model to detect and classify objects in speech recognition.
42. Developing a deep learning model to detect and classify objects in natural language understanding.
43. Developing a deep learning model to detect and classify objects in facial recognition.
44. Developing a deep learning model to detect and classify objects in gesture recognition.
45. Developing a deep learning model to detect and classify objects in sentiment analysis.
46. Developing a deep learning model to detect and classify objects in time series analysis.
47. Developing a deep learning model to detect and classify objects in anomaly detection.
48. Developing a deep learning model to detect and classify objects in recommender systems.
49. Developing a deep learning model to detect and classify objects in medical diagnosis.
50. Developing a deep learning model to detect and classify objects in fraud detection.
I hope, this article would help you know various Deep Learning research ideas and project ideas.
- Deep Learning
- Machine Learning
- Research Ideas
What is a PhD? A Comprehensive Guide for Indian Scientists and Aspiring Researchers
Fellowships in india 2024 -comprehensive guide, working sci-hub proxy links 2024: access research papers easily, most popular, the nippon foundation fellowship programme 2025, agi in research: unraveling the future of artificial intelligence, abstract template for research paper, 10 types of plagiarism – every academic writer should know – updated, the harsh reality: why revoked graduate degrees aren’t easily reclaimed, top 50 research institutions in india: nirf rankings 2024, top 35 scopus indexed journals in english literature, best for you, 24 best online plagiarism checker free – 2024, popular posts, newly accepted scopus indexed journals june 2024, top 10 scopus indexed agronomy and crop science journals, popular category.
- POSTDOC 317
- Interesting 257
- Journals 235
- Fellowship 134
- Research Methodology 102
- All Scopus Indexed Journals 93
Mail Subscription
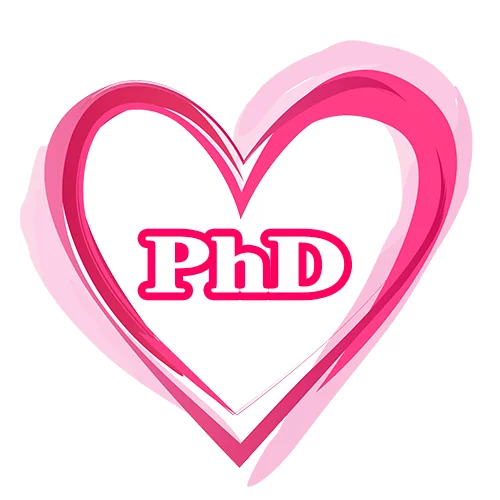
iLovePhD is a research education website to know updated research-related information. It helps researchers to find top journals for publishing research articles and get an easy manual for research tools. The main aim of this website is to help Ph.D. scholars who are working in various domains to get more valuable ideas to carry out their research. Learn the current groundbreaking research activities around the world, love the process of getting a Ph.D.
Contact us: [email protected]
Google News
Copyright © 2024 iLovePhD. All rights reserved
- Artificial intelligence
Available Master's thesis topics in machine learning
Main content.
Here we list topics that are available. You may also be interested in our list of completed Master's theses .
Learning and inference with large Bayesian networks
Most learning and inference tasks with Bayesian networks are NP-hard. Therefore, one often resorts to using different heuristics that do not give any quality guarantees.
Task: Evaluate quality of large-scale learning or inference algorithms empirically.
Advisor: Pekka Parviainen
Sum-product networks
Traditionally, probabilistic graphical models use a graph structure to represent dependencies and independencies between random variables. Sum-product networks are a relatively new type of a graphical model where the graphical structure models computations and not the relationships between variables. The benefit of this representation is that inference (computing conditional probabilities) can be done in linear time with respect to the size of the network.
Potential thesis topics in this area: a) Compare inference speed with sum-product networks and Bayesian networks. Characterize situations when one model is better than the other. b) Learning the sum-product networks is done using heuristic algorithms. What is the effect of approximation in practice?
Bayesian Bayesian networks
The naming of Bayesian networks is somewhat misleading because there is nothing Bayesian in them per se; A Bayesian network is just a representation of a joint probability distribution. One can, of course, use a Bayesian network while doing Bayesian inference. One can also learn Bayesian networks in a Bayesian way. That is, instead of finding an optimal network one computes the posterior distribution over networks.
Task: Develop algorithms for Bayesian learning of Bayesian networks (e.g., MCMC, variational inference, EM)
Large-scale (probabilistic) matrix factorization
The idea behind matrix factorization is to represent a large data matrix as a product of two or more smaller matrices.They are often used in, for example, dimensionality reduction and recommendation systems. Probabilistic matrix factorization methods can be used to quantify uncertainty in recommendations. However, large-scale (probabilistic) matrix factorization is computationally challenging.
Potential thesis topics in this area: a) Develop scalable methods for large-scale matrix factorization (non-probabilistic or probabilistic), b) Develop probabilistic methods for implicit feedback (e.g., recommmendation engine when there are no rankings but only knowledge whether a customer has bought an item)
Bayesian deep learning
Standard deep neural networks do not quantify uncertainty in predictions. On the other hand, Bayesian methods provide a principled way to handle uncertainty. Combining these approaches leads to Bayesian neural networks. The challenge is that Bayesian neural networks can be cumbersome to use and difficult to learn.
The task is to analyze Bayesian neural networks and different inference algorithms in some simple setting.
Deep learning for combinatorial problems
Deep learning is usually applied in regression or classification problems. However, there has been some recent work on using deep learning to develop heuristics for combinatorial optimization problems; see, e.g., [1] and [2].
Task: Choose a combinatorial problem (or several related problems) and develop deep learning methods to solve them.
References: [1] Vinyals, Fortunato and Jaitly: Pointer networks. NIPS 2015. [2] Dai, Khalil, Zhang, Dilkina and Song: Learning Combinatorial Optimization Algorithms over Graphs. NIPS 2017.
Advisors: Pekka Parviainen, Ahmad Hemmati
Estimating the number of modes of an unknown function
Mode seeking considers estimating the number of local maxima of a function f. Sometimes one can find modes by, e.g., looking for points where the derivative of the function is zero. However, often the function is unknown and we have only access to some (possibly noisy) values of the function.
In topological data analysis, we can analyze topological structures using persistent homologies. For 1-dimensional signals, this can translate into looking at the birth/death persistence diagram, i.e. the birth and death of connected topological components as we expand the space around each point where we have observed our function. These observations turn out to be closely related to the modes (local maxima) of the function. A recent paper [1] proposed an efficient method for mode seeking.
In this project, the task is to extend the ideas from [1] to get a probabilistic estimate on the number of modes. To this end, one has to use probabilistic methods such as Gaussian processes.
[1] U. Bauer, A. Munk, H. Sieling, and M. Wardetzky. Persistence barcodes versus Kolmogorov signatures: Detecting modes of one-dimensional signals. Foundations of computational mathematics17:1 - 33, 2017.
Advisors: Pekka Parviainen , Nello Blaser
Causal Abstraction Learning
We naturally make sense of the world around us by working out causal relationships between objects and by representing in our minds these objects with different degrees of approximation and detail. Both processes are essential to our understanding of reality, and likely to be fundamental for developing artificial intelligence. The first process may be expressed using the formalism of structural causal models, while the second can be grounded in the theory of causal abstraction [1]. This project will consider the problem of learning an abstraction between two given structural causal models. The primary goal will be the development of efficient algorithms able to learn a meaningful abstraction between the given causal models. [1] Rubenstein, Paul K., et al. "Causal consistency of structural equation models." arXiv preprint arXiv:1707.00819 (2017).
Advisor: Fabio Massimo Zennaro
Causal Bandits
"Multi-armed bandit" is an informal name for slot machines, and the formal name of a large class of problems where an agent has to choose an action among a range of possibilities without knowing the ensuing rewards. Multi-armed bandit problems are one of the most essential reinforcement learning problems where an agent is directly faced with an exploitation-exploration trade-off. This project will consider a class of multi-armed bandits where an agent, upon taking an action, interacts with a causal system [1]. The primary goal will be the development of learning strategies that takes advantage of the underlying causal system in order to learn optimal policies in a shortest amount of time. [1] Lattimore, Finnian, Tor Lattimore, and Mark D. Reid. "Causal bandits: Learning good interventions via causal inference." Advances in neural information processing systems 29 (2016).
Causal Modelling for Battery Manufacturing
Lithium-ion batteries are poised to be one of the most important sources of energy in the near future. Yet, the process of manufacturing these batteries is very hard to model and control. Optimizing the different phases of production to maximize the lifetime of the batteries is a non-trivial challenge since physical models are limited in scope and collecting experimental data is extremely expensive and time-consuming [1]. This project will consider the problem of aggregating and analyzing data regarding a few stages in the process of battery manufacturing. The primary goal will be the development of algorithms for transporting and integrating data collected in different contexts, as well as the use of explainable algorithms to interpret them. [1] Niri, Mona Faraji, et al. "Quantifying key factors for optimised manufacturing of Li-ion battery anode and cathode via artificial intelligence." Energy and AI 7 (2022): 100129.
Advisor: Fabio Massimo Zennaro , Mona Faraji Niri
Reinforcement Learning for Computer Security
The field of computer security presents a wide variety of challenging problems for artificial intelligence and autonomous agents. Guaranteeing the security of a system against attacks and penetrations by malicious hackers has always been a central concern of this field, and machine learning could now offer a substantial contribution. Security capture-the-flag simulations are particularly well-suited as a testbed for the application and development of reinforcement learning algorithms [1]. This project will consider the use of reinforcement learning for the preventive purpose of testing systems and discovering vulnerabilities before they can be exploited. The primary goal will be the modelling of capture-the-flag challenges of interest and the development of reinforcement learning algorithms that can solve them. [1] Erdodi, Laszlo, and Fabio Massimo Zennaro. "The Agent Web Model--Modelling web hacking for reinforcement learning." arXiv preprint arXiv:2009.11274 (2020).
Advisor: Fabio Massimo Zennaro , Laszlo Tibor Erdodi
Approaches to AI Safety
The world and the Internet are more and more populated by artificial autonomous agents carrying out tasks on our behalf. Many of these agents are provided with an objective and they learn their behaviour trying to achieve their objective as better as they can. However, this approach can not guarantee that an agent, while learning its behaviour, will not undertake actions that may have unforeseen and undesirable effects. Research in AI safety tries to design autonomous agent that will behave in a predictable and safe way [1]. This project will consider specific problems and novel solution in the domain of AI safety and reinforcement learning. The primary goal will be the development of innovative algorithms and their implementation withing established frameworks. [1] Amodei, Dario, et al. "Concrete problems in AI safety." arXiv preprint arXiv:1606.06565 (2016).
Reinforcement Learning for Super-modelling
Super-modelling [1] is a technique designed for combining together complex dynamical models: pre-trained models are aggregated with messages and information being exchanged in order synchronize the behavior of the different modles and produce more accurate and reliable predictions. Super-models are used, for instance, in weather or climate science, where pre-existing models are ensembled together and their states dynamically aggregated to generate more realistic simulations.
This project will consider how reinforcement learning algorithms may be used to solve the coordination problem among the individual models forming a super-model. The primary goal will be the formulation of the super-modelling problem within the reinforcement learning framework and the study of custom RL algorithms to improve the overall performance of super-models.
[1] Schevenhoven, Francine, et al. "Supermodeling: improving predictions with an ensemble of interacting models." Bulletin of the American Meteorological Society 104.9 (2023): E1670-E1686.
Advisor: Fabio Massimo Zennaro , Francine Janneke Schevenhoven
Multilevel Causal Discovery
Modelling causal relationships between variables of interest is a crucial step in understanding and controlling a system. A common approach is to represent such relations using graphs with directed arrows discriminating causes from effects.
While causal graphs are often built relying on expert knowledge, a more interesting challenge is to learn them from data. In particular, we want to consider the case where data might have been collected at multiple levels, for instance, with sensor with different resolutions. In this project we want to explore how these heterogeneous data can help the process of inferring causal structures.
[1] Anand, Tara V., et al. "Effect identification in cluster causal diagrams." Proceedings of the 37th AAAI Conference on Artificial Intelligence. Vol. 82. 2023.
Advisor: Fabio Massimo Zennaro , Pekka Parviainen
Manifolds of Causal Models
Modelling causal relationships is fundamental in order to understand real-world systems. A common formalism is offered by structural causal models (SCMs) which represent these relationships graphical. However, SCMs are complex mathematical objects entailing collections of different probability distributions. In this project we want to explore a differential geometric perspective on structural causal models [1]. We will model an SCM and the probability distributions it generates in terms of manifold, and we will study how this modelling encodes causal properties of interest and how relevant quantities may be computed in this framework. [1] Dominguez-Olmedo, Ricardo, et al. "On data manifolds entailed by structural causal models." International Conference on Machine Learning. PMLR, 2023.
Advisor: Fabio Massimo Zennaro , Nello Blaser
Topological Data Analysis on Simulations
Complex systems and dynamics may be hard to formalize in a closed form, and they can often be better studied through simulations. Social systems, for instance, may be reproduced by instantiating simple agents whose interactions generate complex and emergent dynamics. Still, analyzing the behaviours arising from these interactions is not trivial. In this project we will consider the use of topological data analysis for categorizing and understanding the behaviour of agents in agent-based models [1]. We will analyze the insights and the limitations of exisiting algorithms, as well as consider what dynamical information may be glimpsed through such an analysis.
[1] Swarup, Samarth, and Reza Rezazadegan. "Constructing an Agent Taxonomy from a Simulation Through Topological Data Analysis." Multi-Agent-Based Simulation XX: 20th International Workshop, MABS 2019, Montreal, QC, Canada, May 13, 2019, Revised Selected Papers 20. Springer International Publishing, 2020.
Abstraction for Epistemic Logic
Weighted Kripke models constitute a powerful formalism to express the evolving knowledge of an agent; it allows to express known facts and beliefs, and to recursively model the knowledge of an agent about another agent. Moreover, such relations of knowledge can be given a graphical expression using suitable diagrams on which to perform reasoning. Unfortunately, such graphs can quickly become very large and inefficient to process.
This project consider the reduction of epistemic logic graph using ideas from causal abstraction [1]. The primary goal will be the development of ML models that can learn to output small epistemic logic graph still satisfying logical and consistency constraints.
[1] Zennaro, Fabio Massimo, et al. "Jointly learning consistent causal abstractions over multiple interventional distributions." Conference on Causal Learning and Reasoning. PMLR, 2023
Advisor: Fabio Massimo Zennaro , Rustam Galimullin
Optimal Transport for Public Transportation
Modelling public transportation across cities is critical in order to improve viability, provide reliable services and increase reliance on greener form of mass transport. Yet cities and transportation networks are complex systems and modelling often has to rely on incomplete and uncertain data.
This project will start from considering a concrete challenge in modelling commuter flows across the city of Bergen. In particular, it will consider the application of the mathematical framework of optimal transport [1] to recover statistical patterns in the usage of the main transportation lines across different periods.
[1] Peyré, Gabriel, and Marco Cuturi. "Computational optimal transport: With applications to data science." Foundations and Trends in Machine Learning 11.5-6 (2019): 355-607.
Finalistic Models
The behavior of an agent may be explained both in causal terms (what has caused a certain behavior) or in finalistic terms (what aim justifies a certain behaviour). While causal reasoning is well explained by different mathematical formalism (e.g., structural causal models), finalistic reasoning is still object of research.
In this project we want to explore how a recently-proposed framework for finalistic reasoning [1] may be used to model intentions and counterfactuals in a causal bandit setting, or how it could be used to enhance inverse reinforcement learning.
[1] Compagno, Dario. "Final models: A finalistic interpretation of statistical correlation." arXiv preprint arXiv:2310.02272 (2023).
Advisor: Fabio Massimo Zennaro , Dario Compagno
Automatic hyperparameter selection for isomap
Isomap is a non-linear dimensionality reduction method with two free hyperparameters (number of nearest neighbors and neighborhood radius). Different hyperparameters result in dramatically different embeddings. Previous methods for selecting hyperparameters focused on choosing one optimal hyperparameter. In this project, you will explore the use of persistent homology to find parameter ranges that result in stable embeddings. The project has theoretic and computational aspects.
Advisor: Nello Blaser
Topological Ancombs quartet
This topic is based on the classical Ancombs quartet and families of point sets with identical 1D persistence ( https://arxiv.org/abs/2202.00577 ). The goal is to generate more interesting datasets using the simulated annealing methods presented in ( http://library.usc.edu.ph/ACM/CHI%202017/1proc/p1290.pdf ). This project is mostly computational.
Persistent homology vectorization with cycle location
There are many methods of vectorizing persistence diagrams, such as persistence landscapes, persistence images, PersLay and statistical summaries. Recently we have designed algorithms to in some cases efficiently detect the location of persistence cycles. In this project, you will vectorize not just the persistence diagram, but additional information such as the location of these cycles. This project is mostly computational with some theoretic aspects.
Divisive covers
Divisive covers are a divisive technique for generating filtered simplicial complexes. They original used a naive way of dividing data into a cover. In this project, you will explore different methods of dividing space, based on principle component analysis, support vector machines and k-means clustering. In addition, you will explore methods of using divisive covers for classification. This project will be mostly computational.
Learning Acquisition Functions for Cost-aware Bayesian Optimization
This is a follow-up project of an earlier Master thesis that developed a novel method for learning Acquisition Functions in Bayesian Optimization through the use of Reinforcement Learning. The goal of this project is to further generalize this method (more general input, learned cost-functions) and apply it to hyperparameter optimization for neural networks.
Advisors: Nello Blaser , Audun Ljone Henriksen
Stable updates
This is a follow-up project of an earlier Master thesis that introduced and studied empirical stability in the context of tree-based models. The goal of this project is to develop stable update methods for deep learning models. You will design sevaral stable methods and empirically compare them (in terms of loss and stability) with a baseline and with one another.
Advisors: Morten Blørstad , Nello Blaser
Multimodality in Bayesian neural network ensembles
One method to assess uncertainty in neural network predictions is to use dropout or noise generators at prediction time and run every prediction many times. This leads to a distribution of predictions. Informatively summarizing such probability distributions is a non-trivial task and the commonly used means and standard deviations result in the loss of crucial information, especially in the case of multimodal distributions with distinct likely outcomes. In this project, you will analyze such multimodal distributions with mixture models and develop ways to exploit such multimodality to improve training. This project can have theoretical, computational and applied aspects.
Wet area segmentation for rivers
NORCE LFI is working on digitizing wetted areas in rivers. You will apply different machine learning techniques for distinguishing water bodies (rivers) from land based on drone aerial (RGB) pictures. This is important for water management and assessing effects of hydropower on river ecosystems (residual flow, stranding of fish and spawning areas). We have a database of approximately 100 rivers (aerial pictures created from totally ca. 120.000 single pictures with Structure from Motion, single pictures available as well) and several of these rivers are flown at 2-4 different discharges, taken in different seasons and with different weather patterns. For ca. 50 % of the pictures the wetted area is digitized for training (GIS shapefile), most (>90 % of single pictures) cover water surface and land. Possible challenges include shading, reflectance from the water surface, different water/ground colours and wet surfaces on land. This is an applied topic, where you will try many different machine learning techniques to find the best solution for the mapping tasks by NORCE LFI.
Advisor: Nello Blaser , Sebastian Franz Stranzl
Learning a hierarchical metric
Often, labels have defined relationships to each other, for instance in a hierarchical taxonomy. E.g. ImageNet labels are derived from the WordNet graph, and biological species are taxonomically related, and can have similarities depending on life stage, sex, or other properties.
ArcFace is an alternative loss function that aims for an embedding that is more generally useful than softmax. It is commonly used in metric learning/few shot learning cases.
Here, we will develop a metric learning method that learns from data with hierarchical labels. Using multiple ArcFace heads, we will simultaneously learn to place representations to optimize the leaf label as well as intermediate labels on the path from leaf to root of the label tree. Using taxonomically classified plankton image data, we will measure performance as a function of ArcFace parameters (sharpness/temperature and margins -- class-wise or level-wise), and compare the results to existing methods.
Advisor: Ketil Malde ( [email protected] )
Self-supervised object detection in video
One challenge with learning object detection is that in many scenes that stretch off into the distance, annotating small, far-off, or blurred objects is difficult. It is therefore desirable to learn from incompletely annotated scenes, and one-shot object detectors may suffer from incompletely annotated training data.
To address this, we will use a region-propsal algorithm (e.g. SelectiveSearch) to extract potential crops from each frame. Classification will be based on two approaches: a) training based on annotated fish vs random similarly-sized crops without annotations, and b) using a self-supervised method to build a representation for crops, and building a classifier for the extracted regions. The method will be evaluated against one-shot detectors and other training regimes.
If successful, the method will be applied to fish detection and tracking in videos from baited and unbaited underwater traps, and used to estimate abundance of various fish species.
See also: Benettino (2016): https://link.springer.com/chapter/10.1007/978-3-319-48881-3_56
Representation learning for object detection
While traditional classifiers work well with data that is labeled with disjoint classes and reasonably balanced class abundances, reality is often less clean. An alternative is to learn a vectors space embedding that reflects semantic relationships between objects, and deriving classes from this representation. This is especially useful for few-shot classification (ie. very few examples in the training data).
The task here is to extend a modern object detector (e.g. Yolo v8) to output an embedding of the identified object. Instead of a softmax classifier, we can learn the embedding either in a supervised manner (using annotations on frames) by attaching an ArcFace or other supervised metric learning head. Alternatively, the representation can be learned from tracked detections over time using e.g. a contrastive loss function to keep the representation for an object (approximately) constant over time. The performance of the resulting object detector will be measured on underwater videos, targeting species detection and/or indiviual recognition (re-ID).
Time-domain object detection
Object detectors for video are normally trained on still frames, but it is evident (from human experience) that using time domain information is more effective. I.e., it can be hard to identify far-off or occluded objects in still images, but movement in time often reveals them.
Here we will extend a state of the art object detector (e.g. yolo v8) with time domain data. Instead of using a single frame as input, the model will be modified to take a set of frames surrounding the annotated frame as input. Performance will be compared to using single-frame detection.
Large-scale visualization of acoustic data
The Institute of Marine Research has decades of acoustic data collected in various surveys. These data are in the process of being converted to data formats that can be processed and analyzed more easily using packages like Xarray and Dask.
The objective is to make these data more accessible to regular users by providing a visual front end. The user should be able to quickly zoom in and out, perform selection, export subsets, apply various filters and classifiers, and overlay annotations and other relevant auxiliary data.
Learning acoustic target classification from simulation
Broadband echosounders emit a complex signal that spans a large frequency band. Different targets will reflect, absorb, and generate resonance at different amplitudes and frequencies, and it is therefore possible to classify targets at much higher resolution and accuracy than before. Due to the complexity of the received signals, deriving effective profiles that can be used to identify targets is difficult.
Here we will use simulated frequency spectra from geometric objects with various shapes, orientation, and other properties. We will train ML models to estimate (recover) the geometric and material properties of objects based on these spectra. The resulting model will be applied to read broadband data, and compared to traditional classification methods.
Online learning in real-time systems
Build a model for the drilling process by using the Virtual simulator OpenLab ( https://openlab.app/ ) for real-time data generation and online learning techniques. The student will also do a short survey of existing online learning techniques and learn how to cope with errors and delays in the data.
Advisor: Rodica Mihai
Building a finite state automaton for the drilling process by using queries and counterexamples
Datasets will be generated by using the Virtual simulator OpenLab ( https://openlab.app/ ). The student will study the datasets and decide upon a good setting to extract a finite state automaton for the drilling process. The student will also do a short survey of existing techniques for extracting finite state automata from process data. We present a novel algorithm that uses exact learning and abstraction to extract a deterministic finite automaton describing the state dynamics of a given trained RNN. We do this using Angluin's L*algorithm as a learner and the trained RNN as an oracle. Our technique efficiently extracts accurate automata from trained RNNs, even when the state vectors are large and require fine differentiation.arxiv.org
Machine Learning for Drug Repositioning in Parkinson’s Disease
Background : Parkinson’s Disease (PD) is a major neurological condition with a complex etiology that tends to affect the elderly population. Understanding the risk factors associated with PD, including drug usage patterns across different demographics, can provide insights into its management and prevention. The Norwegian Prescribed Drug Registry (NorPD) provides comprehensive data on prescriptions dispensed from 2004, making it an excellent resource for such an analysis.
Objective : This project seeks to investigate how well machine learning techniques can predict PD risk, using the individual histories of drug usage along with demographic variables like gender and age.
Methodology :
- Exploratory Data Analysis and Data Preprocessing: Although the dataset is clean and structured, specific preprocessing steps will be required to tailor the data for the chosen methods.
- Predictive Modeling: Apply standard machine learning models such as Random Forest for handling large, imbalanced, sparse dataset, to find the best model or ensemble models to robust prediction. The predictive model will be employed to discern patterns in drug usage and demographic factors that correlate with PD risk.
- Feature Analysis: Conduct a detailed analysis to understand the importance of different features, such as specific drugs, gender, and age, in predicting PD risk and explore complex dependencies between features.
- Evaluation Metrics: Explore different metrics, such as F1-score and AUC-ROC to evaluate the performance of the predictive models.
Expected Outcomes : The project aims to study and develop predictive models that can accurately identify individuals at increased risk of developing PD based on their prescription history and demographic data.
Ethical Considerations : Data privacy and confidentiality will be strictly maintained by conducting all analyses on the SAFE server, following ethical guidelines for handling sensitive health data. The approval from regional ethics committee (REK) is already in place, as the project will be part of DRONE ( https://www.uib.no/en/epistat/139849/drone-drug-repurposing-neurological-diseases ).
Project Benefits .
- The student practices working with a huge and rich set of real data and working with experts from epidemiology group at MED faculty.
- Utilizing different ML methods in real data
- The possibility of publication if the results are promising.
Advisors : Asieh Abolpour Mofrad , Samaneh Abolpour Mofrad , Julia Romanowska , Jannicke Igland
Exploring Graph Neural Networks for Analyzing Prescription Data to Predict Parkinson’s Disease Risk
Background : Parkinson’s Disease (PD) significantly impacts the elderly, necessitating advanced computational approaches to predict and understand its risk factors better. The Norwegian Prescribed Drug Registry (NorPD) provides comprehensive data on prescriptions dispensed from 2004, presents an excellent opportunity to employ graph neural networks (GNNs), especially to analyze the temporal dynamics of prescription data.
Objective . The project aims to investigate the effectiveness of GNNs in analyzing time-dependent prescription data, focusing on various graph structures to understand how drug interactions and patient demographics influence PD risk over time.
- Exploratory Data Analysis and Data Preprocessing: Prepare the prescription data for GNN analysis by investigating different structures to represent the data as a graph. This step is a challenging step; we must investigate what is the best structure for a graph based on the existing GNN and temporal GNN methods. For instance, one might assign a graph to each individual and consider classification approaches, or defining a graph for all participants, and investigating the GNN methods for clustering or predicting nodes and edges.
Incorporate demographic features, such as age, gender, and education, into the graph. Additionally, explore how to integrate time-dependent features to reflect the dynamic nature of the prescription data effectively.
- Graph Neural Network Implementation: Apply graph neural network models such as Graph Convolutional Networks (GCNs) or Graph Attention Networks (GATs) that can process temporal graph data, based on the structure of our defined graph.
- Feature Analysis: Perform an in-depth analysis of the learned embeddings and node features to identify significant patterns and influential factors related to increased, decreased PD risk.
- Evaluation Metrics: Explore different metrics to evaluate the performance of the predictive models.
Expected Outcomes :
The project aims to study how graph neural networks (GNNs) can be utilized to analyze complex, time-dependent prescription data.
Ethical Considerations . All analyses will adhere to strict privacy protocols by conducting research on the SAFE server, ensuring that all individual data remains confidential and secure in compliance with ethical healthcare data management practices. The approval from regional ethics committee (REK) is already in place, as the project will be part of DRONE ( https://www.uib.no/en/epistat/139849/drone-drug-repurposing-neurological-diseases )
Project Benefits :
- Get familiar with GNNs as advanced ML methods and utilize them in real data.
Advisors : Samaneh Abolpour Mofrad , Asieh Abolpour Mofrad , Julia Romanowska , Jannicke Igland
Scaling Laws for Language Models in Generative AI
Large Language Models (LLM) power today's most prominent language technologies in Generative AI like ChatGPT, which, in turn, are changing the way that people access information and solve tasks of many kinds.
A recent interest on scaling laws for LLMs has shown trends on understanding how well they perform in terms of factors like the how much training data is used, how powerful the models are, or how much computational cost is allocated. (See, for example, Kaplan et al. - "Scaling Laws for Neural Language Models”, 2020.)
In this project, the task will consider to study scaling laws for different language models and with respect with one or multiple modeling factors.
Advisor: Dario Garigliotti
Applications of causal inference methods to omics data
Many hard problems in machine learning are directly linked to causality [1]. The graphical causal inference framework developed by Judea Pearl can be traced back to pioneering work by Sewall Wright on path analysis in genetics and has inspired research in artificial intelligence (AI) [1].
The Michoel group has developed the open-source tool Findr [2] which provides efficient implementations of mediation and instrumental variable methods for applications to large sets of omics data (genomics, transcriptomics, etc.). Findr works well on a recent data set for yeast [3].
We encourage students to explore promising connections between the fiels of causal inference and machine learning. Feel free to contact us to discuss projects related to causal inference. Possible topics include: a) improving methods based on structural causal models, b) evaluating causal inference methods on data for model organisms, c) comparing methods based on causal models and neural network approaches.
References:
1. Schölkopf B, Causality for Machine Learning, arXiv (2019): https://arxiv.org/abs/1911.10500
2. Wang L and Michoel T. Efficient and accurate causal inference with hidden confounders from genome-transcriptome variation data. PLoS Computational Biology 13:e1005703 (2017). https://journals.plos.org/ploscompbiol/article?id=10.1371/journal.pcbi.1005703
3. Ludl A and and Michoel T. Comparison between instrumental variable and mediation-based methods for reconstructing causal gene networks in yeast. arXiv:2010.07417 https://arxiv.org/abs/2010.07417
Advisors: Adriaan Ludl , Tom Michoel
Space-Time Linkage of Fish Distribution to Environmental Conditions
Conditions in the marine environment, such as, temperature and currents, influence the spatial distribution and migration patterns of marine species. Hence, understanding the link between environmental factors and fish behavior is crucial in predicting, e.g., how fish populations may respond to climate change. Deriving this link is challenging because it requires analysis of two types of datasets (i) large environmental (currents, temperature) datasets that vary in space and time, and (ii) sparse and sporadic spatial observations of fish populations.
Project goal
The primary goal of the project is to develop a methodology that helps predict how spatial distribution of two fish stocks (capelin and mackerel) change in response to variability in the physical marine environment (ocean currents and temperature). The information can also be used to optimize data collection by minimizing time spent in spatial sampling of the populations.
The project will focus on the use of machine learning and/or causal inference algorithms. As a first step, we use synthetic (fish and environmental) data from analytic models that couple the two data sources. Because the ‘truth’ is known, we can judge the efficiency and error margins of the methodologies. We then apply the methodologies to real world (empirical) observations.
Advisors: Tom Michoel , Sam Subbey .
Towards precision medicine for cancer patient stratification
On average, a drug or a treatment is effective in only about half of patients who take it. This means patients need to try several until they find one that is effective at the cost of side effects associated with every treatment. The ultimate goal of precision medicine is to provide a treatment best suited for every individual. Sequencing technologies have now made genomics data available in abundance to be used towards this goal.
In this project we will specifically focus on cancer. Most cancer patients get a particular treatment based on the cancer type and the stage, though different individuals will react differently to a treatment. It is now well established that genetic mutations cause cancer growth and spreading and importantly, these mutations are different in individual patients. The aim of this project is use genomic data allow to better stratification of cancer patients, to predict the treatment most likely to work. Specifically, the project will use machine learning approach to integrate genomic data and build a classifier for stratification of cancer patients.
Advisor: Anagha Joshi
Unraveling gene regulation from single cell data
Multi-cellularity is achieved by precise control of gene expression during development and differentiation and aberrations of this process leads to disease. A key regulatory process in gene regulation is at the transcriptional level where epigenetic and transcriptional regulators control the spatial and temporal expression of the target genes in response to environmental, developmental, and physiological cues obtained from a signalling cascade. The rapid advances in sequencing technology has now made it feasible to study this process by understanding the genomewide patterns of diverse epigenetic and transcription factors as well as at a single cell level.
Single cell RNA sequencing is highly important, particularly in cancer as it allows exploration of heterogenous tumor sample, obstructing therapeutic targeting which leads to poor survival. Despite huge clinical relevance and potential, analysis of single cell RNA-seq data is challenging. In this project, we will develop strategies to infer gene regulatory networks using network inference approaches (both supervised and un-supervised). It will be primarily tested on the single cell datasets in the context of cancer.
Developing a Stress Granule Classifier
To carry out the multitude of functions 'expected' from a human cell, the cell employs a strategy of division of labour, whereby sub-cellular organelles carry out distinct functions. Thus we traditionally understand organelles as distinct units defined both functionally and physically with a distinct shape and size range. More recently a new class of organelles have been discovered that are assembled and dissolved on demand and are composed of liquid droplets or 'granules'. Granules show many properties characteristic of liquids, such as flow and wetting, but they can also assume many shapes and indeed also fluctuate in shape. One such liquid organelle is a stress granule (SG).
Stress granules are pro-survival organelles that assemble in response to cellular stress and important in cancer and neurodegenerative diseases like Alzheimer's. They are liquid or gel-like and can assume varying sizes and shapes depending on their cellular composition.
In a given experiment we are able to image the entire cell over a time series of 1000 frames; from which we extract a rough estimation of the size and shape of each granule. Our current method is susceptible to noise and a granule may be falsely rejected if the boundary is drawn poorly in a small majority of frames. Ideally, we would also like to identify potentially interesting features, such as voids, in the accepted granules.
We are interested in applying a machine learning approach to develop a descriptor for a 'classic' granule and furthermore classify them into different functional groups based on disease status of the cell. This method would be applied across thousands of granules imaged from control and disease cells. We are a multi-disciplinary group consisting of biologists, computational scientists and physicists.
Advisors: Sushma Grellscheid , Carl Jones
Machine Learning based Hyperheuristic algorithm
Develop a Machine Learning based Hyper-heuristic algorithm to solve a pickup and delivery problem. A hyper-heuristic is a heuristics that choose heuristics automatically. Hyper-heuristic seeks to automate the process of selecting, combining, generating or adapting several simpler heuristics to efficiently solve computational search problems [Handbook of Metaheuristics]. There might be multiple heuristics for solving a problem. Heuristics have their own strength and weakness. In this project, we want to use machine-learning techniques to learn the strength and weakness of each heuristic while we are using them in an iterative search for finding high quality solutions and then use them intelligently for the rest of the search. Once a new information is gathered during the search the hyper-heuristic algorithm automatically adjusts the heuristics.
Advisor: Ahmad Hemmati
Machine learning for solving satisfiability problems and applications in cryptanalysis
Advisor: Igor Semaev
Hybrid modeling approaches for well drilling with Sintef
Several topics are available.
"Flow models" are first-principles models simulating the flow, temperature and pressure in a well being drilled. Our project is exploring "hybrid approaches" where these models are combined with machine learning models that either learn from time series data from flow model runs or from real-world measurements during drilling. The goal is to better detect drilling problems such as hole cleaning, make more accurate predictions and correctly learn from and interpret real-word data.
The "surrogate model" refers to a ML model which learns to mimic the flow model by learning from the model inputs and outputs. Use cases for surrogate models include model predictions where speed is favoured over accuracy and exploration of parameter space.
Surrogate models with active Learning
While it is possible to produce a nearly unlimited amount of training data by running the flow model, the surrogate model may still perform poorly if it lacks training data in the part of the parameter space it operates in or if it "forgets" areas of the parameter space by being fed too much data from a narrow range of parameters.
The goal of this thesis is to build a surrogate model (with any architecture) for some restricted parameter range and implement an active learning approach where the ML requests more model runs from the flow model in the parts of the parameter space where it is needed the most. The end result should be a surrogate model that is quick and performs acceptably well over the whole defined parameter range.
Surrogate models trained via adversarial learning
How best to train surrogate models from runs of the flow model is an open question. This master thesis would use the adversarial learning approach to build a surrogate model which to its "adversary" becomes indistinguishable from the output of an actual flow model run.
GPU-based Surrogate models for parameter search
While CPU speed largely stalled 20 years ago in terms of working frequency on single cores, multi-core CPUs and especially GPUs took off and delivered increases in computational power by parallelizing computations.
Modern machine learning such as deep learning takes advantage this boom in computing power by running on GPUs.
The SINTEF flow models in contrast, are software programs that runs on a CPU and does not happen to utilize multi-core CPU functionality. The model runs advance time-step by time-step and each time step relies on the results from the previous time step. The flow models are therefore fundamentally sequential and not well suited to massive parallelization.
It is however of interest to run different model runs in parallel, to explore parameter spaces. The use cases for this includes model calibration, problem detection and hypothesis generation and testing.
The task of this thesis is to implement an ML-based surrogate model in such a way that many surrogate model outputs can be produced at the same time using a single GPU. This will likely entail some trade off with model size and maybe some coding tricks.
Uncertainty estimates of hybrid predictions (Lots of room for creativity, might need to steer it more, needs good background literature)
When using predictions from a ML model trained on time series data, it is useful to know if it's accurate or should be trusted. The student is challenged to develop hybrid approaches that incorporates estimates of uncertainty. Components could include reporting variance from ML ensembles trained on a diversity of time series data, implementation of conformal predictions, analysis of training data parameter ranges vs current input, etc. The output should be a "traffic light signal" roughly indicating the accuracy of the predictions.
Transfer learning approaches
We're assuming an ML model is to be used for time series prediction
It is possible to train an ML on a wide range of scenarios in the flow models, but we expect that to perform well, the model also needs to see model runs representative of the type of well and drilling operation it will be used in. In this thesis the student implements a transfer learning approach, where the model is trained on general model runs and fine-tuned on a most representative data set.
(Bonus1: implementing one-shot learning, Bonus2: Using real-world data in the fine-tuning stage)
ML capable of reframing situations
When a human oversees an operation like well drilling, she has a mental model of the situation and new data such as pressure readings from the well is interpreted in light of this model. This is referred to as "framing" and is the normal mode of work. However, when a problem occurs, it becomes harder to reconcile the data with the mental model. The human then goes into "reframing", building a new mental model that includes the ongoing problem. This can be seen as a process of hypothesis generation and testing.
A computer model however, lacks re-framing. A flow model will keep making predictions under the assumption of no problems and a separate alarm system will use the deviation between the model predictions and reality to raise an alarm. This is in a sense how all alarm systems work, but it means that the human must discard the computer model as a tool at the same time as she's handling a crisis.
The student is given access to a flow model and a surrogate model which can learn from model runs both with and without hole cleaning and is challenged to develop a hybrid approach where the ML+flow model continuously performs hypothesis generation and testing and is able to "switch" into predictions of a hole cleaning problem and different remediations of this.
Advisor: Philippe Nivlet at Sintef together with advisor from UiB
Explainable AI at Equinor
In the project Machine Teaching for XAI (see https://xai.w.uib.no ) a master thesis in collaboration between UiB and Equinor.
Advisor: One of Pekka Parviainen/Jan Arne Telle/Emmanuel Arrighi + Bjarte Johansen from Equinor.
Explainable AI at Eviny
In the project Machine Teaching for XAI (see https://xai.w.uib.no ) a master thesis in collaboration between UiB and Eviny.
Advisor: One of Pekka Parviainen/Jan Arne Telle/Emmanuel Arrighi + Kristian Flikka from Eviny.
If you want to suggest your own topic, please contact Pekka Parviainen , Fabio Massimo Zennaro or Nello Blaser .
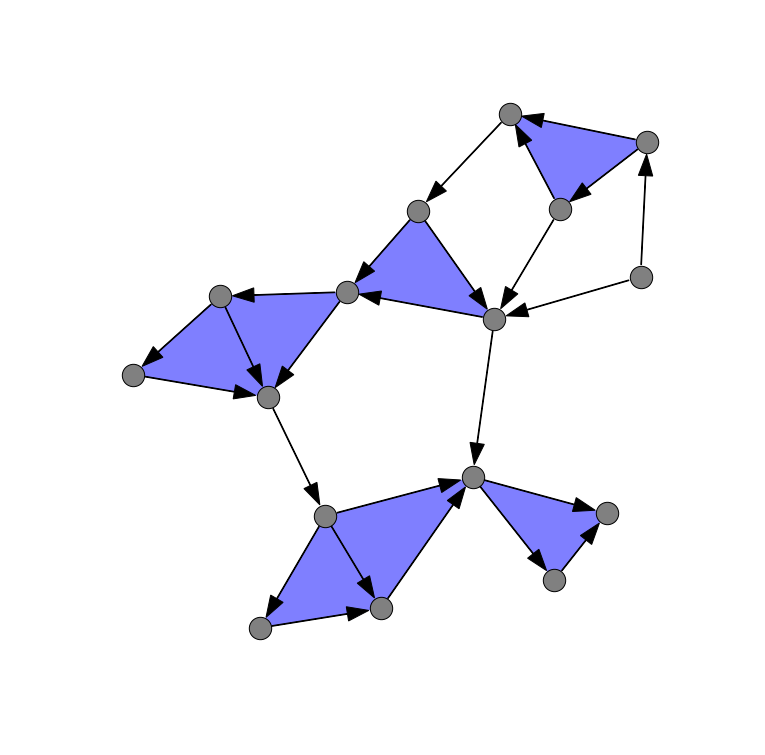

Exploring 250+ Machine Learning Research Topics

In recent years, machine learning has become super popular and grown very quickly. This happened because technology got better, and there’s a lot more data available. Because of this, we’ve seen lots of new and amazing things happen in different areas. Machine learning research is what makes all these cool things possible. In this blog, we’ll talk about machine learning research topics, why they’re important, how you can pick one, what areas are popular to study, what’s new and exciting, the tough problems, and where you can find help if you want to be a researcher.
Whether you’re delving into popular areas or tackling tough problems, our ‘ ‘ service is here to support your research journey.” |
Why Does Machine Learning Research Matter?
Table of Contents
Machine learning research is at the heart of the AI revolution. It underpins the development of intelligent systems capable of making predictions, automating tasks, and improving decision-making across industries. The importance of this research can be summarized as follows:
Advancements in Technology
The growth of machine learning research has led to the development of powerful algorithms, tools, and frameworks. Numerous industries, including healthcare, banking, autonomous cars, and natural language processing, have found use for these technology.
As researchers continue to push the boundaries of what’s possible, we can expect even more transformative technologies to emerge.
Real-world Applications
Machine learning research has brought about tangible changes in our daily lives. Voice assistants like Siri and Alexa, recommendation systems on streaming platforms, and personalized healthcare diagnostics are just a few examples of how this research impacts our world.
By working on new research topics, scientists can further refine these applications and create new ones.
Economic and Industrial Impacts
The economic implications of machine learning research are substantial. Companies that harness the power of machine learning gain a competitive edge in the market.
This creates a demand for skilled machine learning researchers, driving job opportunities and contributing to economic growth.
How to Choose the Machine Learning Research Topics?
Selecting the right machine learning research topics is crucial for your success as a machine learning researcher. Here’s a guide to help you make an informed decision:
- Understanding Your Interests
Start by considering your personal interests. Machine learning is a broad field with applications in virtually every sector. By choosing a topic that aligns with your passions, you’ll stay motivated and engaged throughout your research journey.
- Reviewing Current Trends
Stay updated on the latest trends in machine learning. Attend conferences, read research papers, and engage with the community to identify emerging research topics. Current trends often lead to exciting breakthroughs.
- Identifying Gaps in Existing Research
Sometimes, the most promising research topics involve addressing gaps in existing knowledge. These gaps may become evident through your own experiences, discussions with peers, or in the course of your studies.
- Collaborating with Experts
Collaboration is key in research. Working with experts in the field can help you refine your research topic and gain valuable insights. Seek mentors and collaborators who can guide you.
250+ Machine Learning Research Topics: Category-wise
Supervised learning.
- Explainable AI for Decision Support
- Few-shot Learning Methods
- Time Series Forecasting with Deep Learning
- Handling Imbalanced Datasets in Classification
- Regression Techniques for Non-linear Data
- Transfer Learning in Supervised Settings
- Multi-label Classification Strategies
- Semi-Supervised Learning Approaches
- Novel Feature Selection Methods
- Anomaly Detection in Supervised Scenarios
- Federated Learning for Distributed Supervised Models
- Ensemble Learning for Improved Accuracy
- Automated Hyperparameter Tuning
- Ethical Implications in Supervised Models
- Interpretability of Deep Neural Networks.
Unsupervised Learning
- Unsupervised Clustering of High-dimensional Data
- Semi-Supervised Clustering Approaches
- Density Estimation in Unsupervised Learning
- Anomaly Detection in Unsupervised Settings
- Transfer Learning for Unsupervised Tasks
- Representation Learning in Unsupervised Learning
- Outlier Detection Techniques
- Generative Models for Data Synthesis
- Manifold Learning in High-dimensional Spaces
- Unsupervised Feature Selection
- Privacy-Preserving Unsupervised Learning
- Community Detection in Complex Networks
- Clustering Interpretability and Visualization
- Unsupervised Learning for Image Segmentation
- Autoencoders for Dimensionality Reduction.
Reinforcement Learning
- Deep Reinforcement Learning in Real-world Applications
- Safe Reinforcement Learning for Autonomous Systems
- Transfer Learning in Reinforcement Learning
- Imitation Learning and Apprenticeship Learning
- Multi-agent Reinforcement Learning
- Explainable Reinforcement Learning Policies
- Hierarchical Reinforcement Learning
- Model-based Reinforcement Learning
- Curriculum Learning in Reinforcement Learning
- Reinforcement Learning in Robotics
- Exploration vs. Exploitation Strategies
- Reward Function Design and Ethical Considerations
- Reinforcement Learning in Healthcare
- Continuous Action Spaces in RL
- Reinforcement Learning for Resource Management.
Natural Language Processing (NLP)
- Multilingual and Cross-lingual NLP
- Contextualized Word Embeddings
- Bias Detection and Mitigation in NLP
- Named Entity Recognition for Low-resource Languages
- Sentiment Analysis in Social Media Text
- Dialogue Systems for Improved Customer Service
- Text Summarization for News Articles
- Low-resource Machine Translation
- Explainable NLP Models
- Coreference Resolution in NLP
- Question Answering in Specific Domains
- Detecting Fake News and Misinformation
- NLP for Healthcare: Clinical Document Understanding
- Emotion Analysis in Text
- Text Generation with Controlled Attributes.
Computer Vision
- Video Action Recognition and Event Detection
- Object Detection in Challenging Conditions (e.g., low light)
- Explainable Computer Vision Models
- Image Captioning for Accessibility
- Large-scale Image Retrieval
- Domain Adaptation in Computer Vision
- Fine-grained Image Classification
- Facial Expression Recognition
- Visual Question Answering
- Self-supervised Learning for Visual Representations
- Weakly Supervised Object Localization
- Human Pose Estimation in 3D
- Scene Understanding in Autonomous Vehicles
- Image Super-resolution
- Gaze Estimation for Human-Computer Interaction.
Deep Learning
- Neural Architecture Search for Efficient Models
- Self-attention Mechanisms and Transformers
- Interpretability in Deep Learning Models
- Robustness of Deep Neural Networks
- Generative Adversarial Networks (GANs) for Data Augmentation
- Neural Style Transfer in Art and Design
- Adversarial Attacks and Defenses
- Neural Networks for Audio and Speech Processing
- Explainable AI for Healthcare Diagnosis
- Automated Machine Learning (AutoML)
- Reinforcement Learning with Deep Neural Networks
- Model Compression and Quantization
- Lifelong Learning with Deep Learning Models
- Multimodal Learning with Vision and Language
- Federated Learning for Privacy-preserving Deep Learning.
Explainable AI
- Visualizing Model Decision Boundaries
- Saliency Maps and Feature Attribution
- Rule-based Explanations for Black-box Models
- Contrastive Explanations for Model Interpretability
- Counterfactual Explanations and What-if Analysis
- Human-centered AI for Explainable Healthcare
- Ethics and Fairness in Explainable AI
- Explanation Generation for Natural Language Processing
- Explainable AI in Financial Risk Assessment
- User-friendly Interfaces for Model Interpretability
- Scalability and Efficiency in Explainable Models
- Hybrid Models for Combined Accuracy and Explainability
- Post-hoc vs. Intrinsic Explanations
- Evaluation Metrics for Explanation Quality
- Explainable AI for Autonomous Vehicles.
Transfer Learning
- Zero-shot Learning and Few-shot Learning
- Cross-domain Transfer Learning
- Domain Adaptation for Improved Generalization
- Multilingual Transfer Learning in NLP
- Pretraining and Fine-tuning Techniques
- Lifelong Learning and Continual Learning
- Domain-specific Transfer Learning Applications
- Model Distillation for Knowledge Transfer
- Contrastive Learning for Transfer Learning
- Self-training and Pseudo-labeling
- Dynamic Adaption of Pretrained Models
- Privacy-Preserving Transfer Learning
- Unsupervised Domain Adaptation
- Negative Transfer Avoidance in Transfer Learning.
Federated Learning
- Secure Aggregation in Federated Learning
- Communication-efficient Federated Learning
- Privacy-preserving Techniques in Federated Learning
- Federated Transfer Learning
- Heterogeneous Federated Learning
- Real-world Applications of Federated Learning
- Federated Learning for Edge Devices
- Federated Learning for Healthcare Data
- Differential Privacy in Federated Learning
- Byzantine-robust Federated Learning
- Federated Learning with Non-IID Data
- Model Selection in Federated Learning
- Scalable Federated Learning for Large Datasets
- Client Selection and Sampling Strategies
- Global Model Update Synchronization in Federated Learning.
Quantum Machine Learning
- Quantum Neural Networks and Quantum Circuit Learning
- Quantum-enhanced Optimization for Machine Learning
- Quantum Data Compression and Quantum Principal Component Analysis
- Quantum Kernels and Quantum Feature Maps
- Quantum Variational Autoencoders
- Quantum Transfer Learning
- Quantum-inspired Classical Algorithms for ML
- Hybrid Quantum-Classical Models
- Quantum Machine Learning on Near-term Quantum Devices
- Quantum-inspired Reinforcement Learning
- Quantum Computing for Quantum Chemistry and Drug Discovery
- Quantum Machine Learning for Finance
- Quantum Data Structures and Quantum Databases
- Quantum-enhanced Cryptography in Machine Learning
- Quantum Generative Models and Quantum GANs.
Ethical AI and Bias Mitigation
- Fairness-aware Machine Learning Algorithms
- Bias Detection and Mitigation in Real-world Data
- Explainable AI for Ethical Decision Support
- Algorithmic Accountability and Transparency
- Privacy-preserving AI and Data Governance
- Ethical Considerations in AI for Healthcare
- Fairness in Recommender Systems
- Bias and Fairness in NLP Models
- Auditing AI Systems for Bias
- Societal Implications of AI in Criminal Justice
- Ethical AI Education and Training
- Bias Mitigation in Autonomous Vehicles
- Fair AI in Financial and Hiring Decisions
- Case Studies in Ethical AI Failures
- Legal and Policy Frameworks for Ethical AI.
Meta-Learning and AutoML
- Neural Architecture Search (NAS) for Efficient Models
- Transfer Learning in NAS
- Reinforcement Learning for NAS
- Multi-objective NAS
- Automated Data Augmentation
- Neural Architecture Optimization for Edge Devices
- Bayesian Optimization for AutoML
- Model Compression and Quantization in AutoML
- AutoML for Federated Learning
- AutoML in Healthcare Diagnostics
- Explainable AutoML
- Cost-sensitive Learning in AutoML
- AutoML for Small Data
- Human-in-the-Loop AutoML.
AI for Healthcare and Medicine
- Disease Prediction and Early Diagnosis
- Medical Image Analysis with Deep Learning
- Drug Discovery and Molecular Modeling
- Electronic Health Record Analysis
- Predictive Analytics in Healthcare
- Personalized Treatment Planning
- Healthcare Fraud Detection
- Telemedicine and Remote Patient Monitoring
- AI in Radiology and Pathology
- AI in Drug Repurposing
- AI for Medical Robotics and Surgery
- Genomic Data Analysis
- AI-powered Mental Health Assessment
- Explainable AI in Healthcare Decision Support
- AI in Epidemiology and Outbreak Prediction.
AI in Finance and Investment
- Algorithmic Trading and High-frequency Trading
- Credit Scoring and Risk Assessment
- Fraud Detection and Anti-money Laundering
- Portfolio Optimization with AI
- Financial Market Prediction
- Sentiment Analysis in Financial News
- Explainable AI in Financial Decision-making
- Algorithmic Pricing and Dynamic Pricing Strategies
- AI in Cryptocurrency and Blockchain
- Customer Behavior Analysis in Banking
- Explainable AI in Credit Decisioning
- AI in Regulatory Compliance
- Ethical AI in Financial Services
- AI for Real Estate Investment
- Automated Financial Reporting.
AI in Climate Change and Sustainability
- Climate Modeling and Prediction
- Renewable Energy Forecasting
- Smart Grid Optimization
- Energy Consumption Forecasting
- Carbon Emission Reduction with AI
- Ecosystem Monitoring and Preservation
- Precision Agriculture with AI
- AI for Wildlife Conservation
- Natural Disaster Prediction and Management
- Water Resource Management with AI
- Sustainable Transportation and Urban Planning
- Climate Change Mitigation Strategies with AI
- Environmental Impact Assessment with Machine Learning
- Eco-friendly Supply Chain Optimization
- Ethical AI in Climate-related Decision Support.
Data Privacy and Security
- Differential Privacy Mechanisms
- Federated Learning for Privacy-preserving AI
- Secure Multi-Party Computation
- Privacy-enhancing Technologies in Machine Learning
- Homomorphic Encryption for Machine Learning
- Ethical Considerations in Data Privacy
- Privacy-preserving AI in Healthcare
- AI for Secure Authentication and Access Control
- Blockchain and AI for Data Security
- Explainable Privacy in Machine Learning
- Privacy-preserving AI in Government and Public Services
- Privacy-compliant AI for IoT and Edge Devices
- Secure AI Models Sharing and Deployment
- Privacy-preserving AI in Financial Transactions
- AI in the Legal Frameworks of Data Privacy.
Global Collaboration in Research
- International Research Partnerships and Collaboration Models
- Multilingual and Cross-cultural AI Research
- Addressing Global Healthcare Challenges with AI
- Ethical Considerations in International AI Collaborations
- Interdisciplinary AI Research in Global Challenges
- AI Ethics and Human Rights in Global Research
- Data Sharing and Data Access in Global AI Research
- Cross-border Research Regulations and Compliance
- AI Innovation Hubs and International Research Centers
- AI Education and Training for Global Communities
- Humanitarian AI and AI for Sustainable Development Goals
- AI for Cultural Preservation and Heritage Protection
- Collaboration in AI-related Global Crises
- AI in Cross-cultural Communication and Understanding
- Global AI for Environmental Sustainability and Conservation.
Emerging Trends and Hot Topics in Machine Learning Research
The landscape of machine learning research topics is constantly evolving. Here are some of the emerging trends and hot topics that are shaping the field:
As AI systems become more prevalent, addressing ethical concerns and mitigating bias in algorithms are critical research areas.
Interpretable and Explainable Models
Understanding why machine learning models make specific decisions is crucial for their adoption in sensitive areas, such as healthcare and finance.
Meta-learning algorithms are designed to enable machines to learn how to learn, while AutoML aims to automate the machine learning process itself.
Machine learning is revolutionizing the healthcare sector, from diagnostic tools to drug discovery and patient care.
Algorithmic trading, risk assessment, and fraud detection are just a few applications of AI in finance, creating a wealth of research opportunities.
Machine learning research is crucial in analyzing and mitigating the impacts of climate change and promoting sustainable practices.
Challenges and Future Directions
While machine learning research has made tremendous strides, it also faces several challenges:
- Data Privacy and Security: As machine learning models require vast amounts of data, protecting individual privacy and data security are paramount concerns.
- Scalability and Efficiency: Developing efficient algorithms that can handle increasingly large datasets and complex computations remains a challenge.
- Ensuring Fairness and Transparency: Addressing bias in machine learning models and making their decisions transparent is essential for equitable AI systems.
- Quantum Computing and Machine Learning: The integration of quantum computing and machine learning has the potential to revolutionize the field, but it also presents unique challenges.
- Global Collaboration in Research: Machine learning research benefits from collaboration on a global scale. Ensuring that researchers from diverse backgrounds work together is vital for progress.
Resources for Machine Learning Researchers
If you’re looking to embark on a journey in machine learning research topics, there are various resources at your disposal:
- Journals and Conferences
Journals such as the “Journal of Machine Learning Research” and conferences like NeurIPS and ICML provide a platform for publishing and discussing research findings.
- Online Communities and Forums
Platforms like Stack Overflow, GitHub, and dedicated forums for machine learning provide spaces for collaboration and problem-solving.
- Datasets and Tools
Open-source datasets and tools like TensorFlow and PyTorch simplify the research process by providing access to data and pre-built models.
- Research Grants and Funding Opportunities
Many organizations and government agencies offer research grants and funding for machine learning projects. Seek out these opportunities to support your research.
Machine learning research is like a superhero in the world of technology. To be a part of this exciting journey, it’s important to choose the right machine learning research topics and keep up with the latest trends.
Machine learning research makes our lives better. It powers things like smart assistants and life-saving medical tools. It’s like the force driving the future of technology and society.
But, there are challenges too. We need to work together and be ethical in our research. Everyone should benefit from this technology. The future of machine learning research is incredibly bright. If you want to be a part of it, get ready for an exciting adventure. You can help create new solutions and make a big impact on the world.
Related Posts

Tips on How To Tackle A Machine Learning Project As A Beginner
Here in this blog, CodeAvail experts will explain to you tips on how to tackle a machine learning project as a beginner step by step…

Artificial Intelligence and Machine Learning Basics for Beginners
Here in this blog, CodeAvail experts will explain to you Artificial Intelligence and Machine Learning basics for beginners in detail step by step. What is…
Navigation Menu
Search code, repositories, users, issues, pull requests..., provide feedback.
We read every piece of feedback, and take your input very seriously.
Saved searches
Use saved searches to filter your results more quickly.
To see all available qualifiers, see our documentation .
master-thesis
Here are 95 public repositories matching this topic..., johnmartinsson / bird-species-classification.
Using convolutional neural networks to build and train a bird species classifier on bird song data with corresponding species labels.
- Updated Oct 11, 2023
Tim-HW / HW-BlueRov2-Sonar-based-SLAM
This project will evaluate simultaneous localisation and mapping (SLAM) algorithms for fusing sonar with DVL and IMU to produce maps for autonomous underwater vehicle (AUV) navigation for underwater ROV
- Updated Jan 24, 2024
meurissemax / autonomous-drone
Master's thesis about autonomous navigation of a drone in indoor environments carried out to obtain the degree of Master of Science in Computer Science Engineering (University of Liège, academic year 2020-2021).
- Updated Jul 13, 2021
dpuljic01 / financial-dashboard
Masters Thesis - Fintech Dashboard
- Updated Sep 10, 2023
LasseRegin / master-thesis-deep-learning
Code for my master thesis in Deep Learning: "Generating answers to medical questions using recurrent neural networks"
- Updated Jul 16, 2017
RealityNet / McAFuse
Toolset to analyze disks encrypted with McAFee FDE technology
- Updated Mar 11, 2021
harshildarji / thesis
Master's thesis, Uni Passau
- Updated Mar 21, 2022
Boren / MasterThesis
Deep Convolutional Neural Networks for Semantic Segmentation of Multi-Band Satellite Images
- Updated May 30, 2018
thomasSve / Msc_Multi_label_ZeroShot
Code for master thesis on Zero-Shot Learning in multi-label scenarios
- Updated Mar 28, 2018
kdevo / chaos-rrs
Chaos - a first of its kind framework for researching Reciprocal Recommender Systems (RRS).
- Updated Nov 7, 2021
Josef-Djarf / sEMG-Sim
Source code for multiple parameter modelling of synthetic electromyography data.
- Updated Feb 21, 2024
JanPokorny / speed-climbing-mapping
Mapping videos of speed climbers onto a virtual wall using ML, OpenCV, and maths. Implementation of my master's thesis.
- Updated Jul 20, 2023
danielathome19 / Form-NN
Master thesis project - a hybrid Neural Network-Decision Tree system and dataset for classical music form recognition and analysis.
- Updated Jul 29, 2024

KyleOng / starreco
State-of-The-Art Rating-based RECOmmendation system: pytorch lightning implementation
- Updated Oct 10, 2023
lukaselmer / hierarchical-paragraph-vectors
Hierarchical Paragraph Vectors
- Updated Sep 21, 2015
rand-asswad / muallef
Study of Music Information Retrieval (MIR) methods for multi-pitch estimation and onset detection.
- Updated Dec 8, 2022
lcebear / memoryDialogueBot
Master Thesis Project: A memory based dialogue agent
- Updated Dec 20, 2020
develooper1994 / MasterThesis
My Master Thesis experimentation source codes
- Updated Apr 6, 2021
EivindArvesen / master_code
Various code from my master's project
- Updated Jan 9, 2019
jrmak / FNNR-ABM-Primate
An agent-based model (with a web simulation) for Guizhou "golden" monkey population and movements using the Mesa Python framework; thesis project + human/GTGP expansion; Summer 2018-Winter 2019
- Updated Mar 22, 2020
Improve this page
Add a description, image, and links to the master-thesis topic page so that developers can more easily learn about it.
Curate this topic
Add this topic to your repo
To associate your repository with the master-thesis topic, visit your repo's landing page and select "manage topics."
Machine Learning - CMU

PhD Dissertations
[all are .pdf files].
Neural processes underlying cognitive control during language production (unavailable) Tara Pirnia, 2024
The Neurodynamic Basis of Real World Face Perception Arish Alreja, 2024
Towards More Powerful Graph Representation Learning Lingxiao Zhao, 2024
Robust Machine Learning: Detection, Evaluation and Adaptation Under Distribution Shift Saurabh Garg, 2024
UNDERSTANDING, FORMALLY CHARACTERIZING, AND ROBUSTLY HANDLING REAL-WORLD DISTRIBUTION SHIFT Elan Rosenfeld, 2024
Representing Time: Towards Pragmatic Multivariate Time Series Modeling Cristian Ignacio Challu, 2024
Foundations of Multisensory Artificial Intelligence Paul Pu Liang, 2024
Advancing Model-Based Reinforcement Learning with Applications in Nuclear Fusion Ian Char, 2024
Learning Models that Match Jacob Tyo, 2024
Improving Human Integration across the Machine Learning Pipeline Charvi Rastogi, 2024
Reliable and Practical Machine Learning for Dynamic Healthcare Settings Helen Zhou, 2023
Automatic customization of large-scale spiking network models to neuronal population activity (unavailable) Shenghao Wu, 2023
Estimation of BVk functions from scattered data (unavailable) Addison J. Hu, 2023
Rethinking object categorization in computer vision (unavailable) Jayanth Koushik, 2023
Advances in Statistical Gene Networks Jinjin Tian, 2023 Post-hoc calibration without distributional assumptions Chirag Gupta, 2023
The Role of Noise, Proxies, and Dynamics in Algorithmic Fairness Nil-Jana Akpinar, 2023
Collaborative learning by leveraging siloed data Sebastian Caldas, 2023
Modeling Epidemiological Time Series Aaron Rumack, 2023
Human-Centered Machine Learning: A Statistical and Algorithmic Perspective Leqi Liu, 2023
Uncertainty Quantification under Distribution Shifts Aleksandr Podkopaev, 2023
Probabilistic Reinforcement Learning: Using Data to Define Desired Outcomes, and Inferring How to Get There Benjamin Eysenbach, 2023
Comparing Forecasters and Abstaining Classifiers Yo Joong Choe, 2023
Using Task Driven Methods to Uncover Representations of Human Vision and Semantics Aria Yuan Wang, 2023
Data-driven Decisions - An Anomaly Detection Perspective Shubhranshu Shekhar, 2023
Applied Mathematics of the Future Kin G. Olivares, 2023
METHODS AND APPLICATIONS OF EXPLAINABLE MACHINE LEARNING Joon Sik Kim, 2023
NEURAL REASONING FOR QUESTION ANSWERING Haitian Sun, 2023
Principled Machine Learning for Societally Consequential Decision Making Amanda Coston, 2023
Long term brain dynamics extend cognitive neuroscience to timescales relevant for health and physiology Maxwell B. Wang, 2023
Long term brain dynamics extend cognitive neuroscience to timescales relevant for health and physiology Darby M. Losey, 2023
Calibrated Conditional Density Models and Predictive Inference via Local Diagnostics David Zhao, 2023
Towards an Application-based Pipeline for Explainability Gregory Plumb, 2022
Objective Criteria for Explainable Machine Learning Chih-Kuan Yeh, 2022
Making Scientific Peer Review Scientific Ivan Stelmakh, 2022
Facets of regularization in high-dimensional learning: Cross-validation, risk monotonization, and model complexity Pratik Patil, 2022
Active Robot Perception using Programmable Light Curtains Siddharth Ancha, 2022
Strategies for Black-Box and Multi-Objective Optimization Biswajit Paria, 2022
Unifying State and Policy-Level Explanations for Reinforcement Learning Nicholay Topin, 2022
Sensor Fusion Frameworks for Nowcasting Maria Jahja, 2022
Equilibrium Approaches to Modern Deep Learning Shaojie Bai, 2022
Towards General Natural Language Understanding with Probabilistic Worldbuilding Abulhair Saparov, 2022
Applications of Point Process Modeling to Spiking Neurons (Unavailable) Yu Chen, 2021
Neural variability: structure, sources, control, and data augmentation Akash Umakantha, 2021
Structure and time course of neural population activity during learning Jay Hennig, 2021
Cross-view Learning with Limited Supervision Yao-Hung Hubert Tsai, 2021
Meta Reinforcement Learning through Memory Emilio Parisotto, 2021
Learning Embodied Agents with Scalably-Supervised Reinforcement Learning Lisa Lee, 2021
Learning to Predict and Make Decisions under Distribution Shift Yifan Wu, 2021
Statistical Game Theory Arun Sai Suggala, 2021
Towards Knowledge-capable AI: Agents that See, Speak, Act and Know Kenneth Marino, 2021
Learning and Reasoning with Fast Semidefinite Programming and Mixing Methods Po-Wei Wang, 2021
Bridging Language in Machines with Language in the Brain Mariya Toneva, 2021
Curriculum Learning Otilia Stretcu, 2021
Principles of Learning in Multitask Settings: A Probabilistic Perspective Maruan Al-Shedivat, 2021
Towards Robust and Resilient Machine Learning Adarsh Prasad, 2021
Towards Training AI Agents with All Types of Experiences: A Unified ML Formalism Zhiting Hu, 2021
Building Intelligent Autonomous Navigation Agents Devendra Chaplot, 2021
Learning to See by Moving: Self-supervising 3D Scene Representations for Perception, Control, and Visual Reasoning Hsiao-Yu Fish Tung, 2021
Statistical Astrophysics: From Extrasolar Planets to the Large-scale Structure of the Universe Collin Politsch, 2020
Causal Inference with Complex Data Structures and Non-Standard Effects Kwhangho Kim, 2020
Networks, Point Processes, and Networks of Point Processes Neil Spencer, 2020
Dissecting neural variability using population recordings, network models, and neurofeedback (Unavailable) Ryan Williamson, 2020
Predicting Health and Safety: Essays in Machine Learning for Decision Support in the Public Sector Dylan Fitzpatrick, 2020
Towards a Unified Framework for Learning and Reasoning Han Zhao, 2020
Learning DAGs with Continuous Optimization Xun Zheng, 2020
Machine Learning and Multiagent Preferences Ritesh Noothigattu, 2020
Learning and Decision Making from Diverse Forms of Information Yichong Xu, 2020
Towards Data-Efficient Machine Learning Qizhe Xie, 2020
Change modeling for understanding our world and the counterfactual one(s) William Herlands, 2020
Machine Learning in High-Stakes Settings: Risks and Opportunities Maria De-Arteaga, 2020
Data Decomposition for Constrained Visual Learning Calvin Murdock, 2020
Structured Sparse Regression Methods for Learning from High-Dimensional Genomic Data Micol Marchetti-Bowick, 2020
Towards Efficient Automated Machine Learning Liam Li, 2020
LEARNING COLLECTIONS OF FUNCTIONS Emmanouil Antonios Platanios, 2020
Provable, structured, and efficient methods for robustness of deep networks to adversarial examples Eric Wong , 2020
Reconstructing and Mining Signals: Algorithms and Applications Hyun Ah Song, 2020
Probabilistic Single Cell Lineage Tracing Chieh Lin, 2020
Graphical network modeling of phase coupling in brain activity (unavailable) Josue Orellana, 2019
Strategic Exploration in Reinforcement Learning - New Algorithms and Learning Guarantees Christoph Dann, 2019 Learning Generative Models using Transformations Chun-Liang Li, 2019
Estimating Probability Distributions and their Properties Shashank Singh, 2019
Post-Inference Methods for Scalable Probabilistic Modeling and Sequential Decision Making Willie Neiswanger, 2019
Accelerating Text-as-Data Research in Computational Social Science Dallas Card, 2019
Multi-view Relationships for Analytics and Inference Eric Lei, 2019
Information flow in networks based on nonstationary multivariate neural recordings Natalie Klein, 2019
Competitive Analysis for Machine Learning & Data Science Michael Spece, 2019
The When, Where and Why of Human Memory Retrieval Qiong Zhang, 2019
Towards Effective and Efficient Learning at Scale Adams Wei Yu, 2019
Towards Literate Artificial Intelligence Mrinmaya Sachan, 2019
Learning Gene Networks Underlying Clinical Phenotypes Under SNP Perturbations From Genome-Wide Data Calvin McCarter, 2019
Unified Models for Dynamical Systems Carlton Downey, 2019
Anytime Prediction and Learning for the Balance between Computation and Accuracy Hanzhang Hu, 2019
Statistical and Computational Properties of Some "User-Friendly" Methods for High-Dimensional Estimation Alnur Ali, 2019
Nonparametric Methods with Total Variation Type Regularization Veeranjaneyulu Sadhanala, 2019
New Advances in Sparse Learning, Deep Networks, and Adversarial Learning: Theory and Applications Hongyang Zhang, 2019
Gradient Descent for Non-convex Problems in Modern Machine Learning Simon Shaolei Du, 2019
Selective Data Acquisition in Learning and Decision Making Problems Yining Wang, 2019
Anomaly Detection in Graphs and Time Series: Algorithms and Applications Bryan Hooi, 2019
Neural dynamics and interactions in the human ventral visual pathway Yuanning Li, 2018
Tuning Hyperparameters without Grad Students: Scaling up Bandit Optimisation Kirthevasan Kandasamy, 2018
Teaching Machines to Classify from Natural Language Interactions Shashank Srivastava, 2018
Statistical Inference for Geometric Data Jisu Kim, 2018
Representation Learning @ Scale Manzil Zaheer, 2018
Diversity-promoting and Large-scale Machine Learning for Healthcare Pengtao Xie, 2018
Distribution and Histogram (DIsH) Learning Junier Oliva, 2018
Stress Detection for Keystroke Dynamics Shing-Hon Lau, 2018
Sublinear-Time Learning and Inference for High-Dimensional Models Enxu Yan, 2018
Neural population activity in the visual cortex: Statistical methods and application Benjamin Cowley, 2018
Efficient Methods for Prediction and Control in Partially Observable Environments Ahmed Hefny, 2018
Learning with Staleness Wei Dai, 2018
Statistical Approach for Functionally Validating Transcription Factor Bindings Using Population SNP and Gene Expression Data Jing Xiang, 2017
New Paradigms and Optimality Guarantees in Statistical Learning and Estimation Yu-Xiang Wang, 2017
Dynamic Question Ordering: Obtaining Useful Information While Reducing User Burden Kirstin Early, 2017
New Optimization Methods for Modern Machine Learning Sashank J. Reddi, 2017
Active Search with Complex Actions and Rewards Yifei Ma, 2017
Why Machine Learning Works George D. Montañez , 2017
Source-Space Analyses in MEG/EEG and Applications to Explore Spatio-temporal Neural Dynamics in Human Vision Ying Yang , 2017
Computational Tools for Identification and Analysis of Neuronal Population Activity Pengcheng Zhou, 2016
Expressive Collaborative Music Performance via Machine Learning Gus (Guangyu) Xia, 2016
Supervision Beyond Manual Annotations for Learning Visual Representations Carl Doersch, 2016
Exploring Weakly Labeled Data Across the Noise-Bias Spectrum Robert W. H. Fisher, 2016
Optimizing Optimization: Scalable Convex Programming with Proximal Operators Matt Wytock, 2016
Combining Neural Population Recordings: Theory and Application William Bishop, 2015
Discovering Compact and Informative Structures through Data Partitioning Madalina Fiterau-Brostean, 2015
Machine Learning in Space and Time Seth R. Flaxman, 2015
The Time and Location of Natural Reading Processes in the Brain Leila Wehbe, 2015
Shape-Constrained Estimation in High Dimensions Min Xu, 2015
Spectral Probabilistic Modeling and Applications to Natural Language Processing Ankur Parikh, 2015 Computational and Statistical Advances in Testing and Learning Aaditya Kumar Ramdas, 2015
Corpora and Cognition: The Semantic Composition of Adjectives and Nouns in the Human Brain Alona Fyshe, 2015
Learning Statistical Features of Scene Images Wooyoung Lee, 2014
Towards Scalable Analysis of Images and Videos Bin Zhao, 2014
Statistical Text Analysis for Social Science Brendan T. O'Connor, 2014
Modeling Large Social Networks in Context Qirong Ho, 2014
Semi-Cooperative Learning in Smart Grid Agents Prashant P. Reddy, 2013
On Learning from Collective Data Liang Xiong, 2013
Exploiting Non-sequence Data in Dynamic Model Learning Tzu-Kuo Huang, 2013
Mathematical Theories of Interaction with Oracles Liu Yang, 2013
Short-Sighted Probabilistic Planning Felipe W. Trevizan, 2013
Statistical Models and Algorithms for Studying Hand and Finger Kinematics and their Neural Mechanisms Lucia Castellanos, 2013
Approximation Algorithms and New Models for Clustering and Learning Pranjal Awasthi, 2013
Uncovering Structure in High-Dimensions: Networks and Multi-task Learning Problems Mladen Kolar, 2013
Learning with Sparsity: Structures, Optimization and Applications Xi Chen, 2013
GraphLab: A Distributed Abstraction for Large Scale Machine Learning Yucheng Low, 2013
Graph Structured Normal Means Inference James Sharpnack, 2013 (Joint Statistics & ML PhD)
Probabilistic Models for Collecting, Analyzing, and Modeling Expression Data Hai-Son Phuoc Le, 2013
Learning Large-Scale Conditional Random Fields Joseph K. Bradley, 2013
New Statistical Applications for Differential Privacy Rob Hall, 2013 (Joint Statistics & ML PhD)
Parallel and Distributed Systems for Probabilistic Reasoning Joseph Gonzalez, 2012
Spectral Approaches to Learning Predictive Representations Byron Boots, 2012
Attribute Learning using Joint Human and Machine Computation Edith L. M. Law, 2012
Statistical Methods for Studying Genetic Variation in Populations Suyash Shringarpure, 2012
Data Mining Meets HCI: Making Sense of Large Graphs Duen Horng (Polo) Chau, 2012
Learning with Limited Supervision by Input and Output Coding Yi Zhang, 2012
Target Sequence Clustering Benjamin Shih, 2011
Nonparametric Learning in High Dimensions Han Liu, 2010 (Joint Statistics & ML PhD)
Structural Analysis of Large Networks: Observations and Applications Mary McGlohon, 2010
Modeling Purposeful Adaptive Behavior with the Principle of Maximum Causal Entropy Brian D. Ziebart, 2010
Tractable Algorithms for Proximity Search on Large Graphs Purnamrita Sarkar, 2010
Rare Category Analysis Jingrui He, 2010
Coupled Semi-Supervised Learning Andrew Carlson, 2010
Fast Algorithms for Querying and Mining Large Graphs Hanghang Tong, 2009
Efficient Matrix Models for Relational Learning Ajit Paul Singh, 2009
Exploiting Domain and Task Regularities for Robust Named Entity Recognition Andrew O. Arnold, 2009
Theoretical Foundations of Active Learning Steve Hanneke, 2009
Generalized Learning Factors Analysis: Improving Cognitive Models with Machine Learning Hao Cen, 2009
Detecting Patterns of Anomalies Kaustav Das, 2009
Dynamics of Large Networks Jurij Leskovec, 2008
Computational Methods for Analyzing and Modeling Gene Regulation Dynamics Jason Ernst, 2008
Stacked Graphical Learning Zhenzhen Kou, 2007
Actively Learning Specific Function Properties with Applications to Statistical Inference Brent Bryan, 2007
Approximate Inference, Structure Learning and Feature Estimation in Markov Random Fields Pradeep Ravikumar, 2007
Scalable Graphical Models for Social Networks Anna Goldenberg, 2007
Measure Concentration of Strongly Mixing Processes with Applications Leonid Kontorovich, 2007
Tools for Graph Mining Deepayan Chakrabarti, 2005
Automatic Discovery of Latent Variable Models Ricardo Silva, 2005
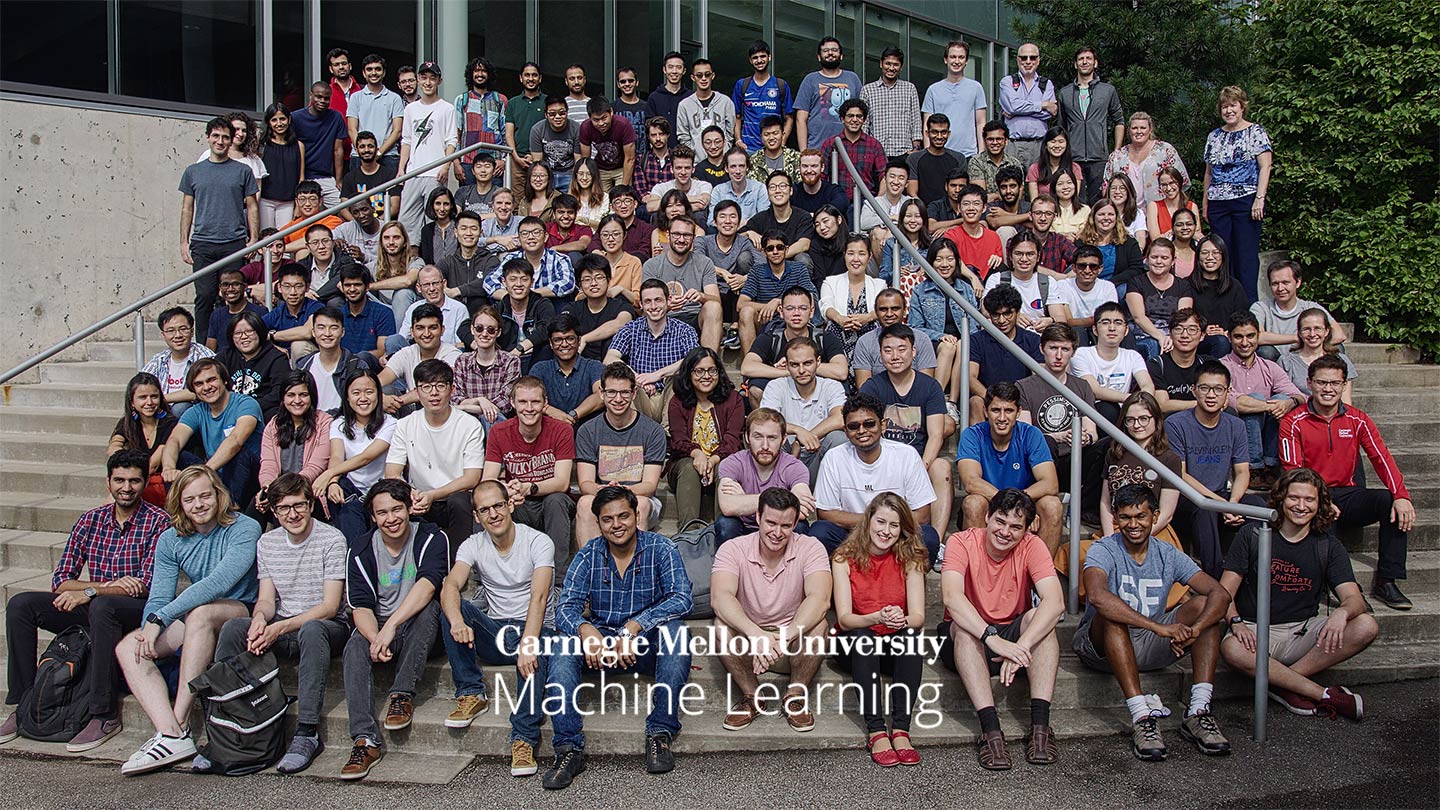
Advertisement
Deep Learning: A Comprehensive Overview on Techniques, Taxonomy, Applications and Research Directions
- Review Article
- Published: 18 August 2021
- Volume 2 , article number 420 , ( 2021 )
Cite this article
- Iqbal H. Sarker ORCID: orcid.org/0000-0003-1740-5517 1 , 2
230k Accesses
882 Citations
24 Altmetric
Explore all metrics
Deep learning (DL), a branch of machine learning (ML) and artificial intelligence (AI) is nowadays considered as a core technology of today’s Fourth Industrial Revolution (4IR or Industry 4.0). Due to its learning capabilities from data, DL technology originated from artificial neural network (ANN), has become a hot topic in the context of computing, and is widely applied in various application areas like healthcare, visual recognition, text analytics, cybersecurity, and many more. However, building an appropriate DL model is a challenging task, due to the dynamic nature and variations in real-world problems and data. Moreover, the lack of core understanding turns DL methods into black-box machines that hamper development at the standard level. This article presents a structured and comprehensive view on DL techniques including a taxonomy considering various types of real-world tasks like supervised or unsupervised. In our taxonomy, we take into account deep networks for supervised or discriminative learning , unsupervised or generative learning as well as hybrid learning and relevant others. We also summarize real-world application areas where deep learning techniques can be used. Finally, we point out ten potential aspects for future generation DL modeling with research directions . Overall, this article aims to draw a big picture on DL modeling that can be used as a reference guide for both academia and industry professionals.
Similar content being viewed by others
Deep Learning: History and State-of-the-Arts
Machine Learning and Deep Learning: A Comparative Review
Future and discussions, explore related subjects.
- Artificial Intelligence
Avoid common mistakes on your manuscript.
Introduction
In the late 1980s, neural networks became a prevalent topic in the area of Machine Learning (ML) as well as Artificial Intelligence (AI), due to the invention of various efficient learning methods and network structures [ 52 ]. Multilayer perceptron networks trained by “Backpropagation” type algorithms, self-organizing maps, and radial basis function networks were such innovative methods [ 26 , 36 , 37 ]. While neural networks are successfully used in many applications, the interest in researching this topic decreased later on. After that, in 2006, “Deep Learning” (DL) was introduced by Hinton et al. [ 41 ], which was based on the concept of artificial neural network (ANN). Deep learning became a prominent topic after that, resulting in a rebirth in neural network research, hence, some times referred to as “new-generation neural networks”. This is because deep networks, when properly trained, have produced significant success in a variety of classification and regression challenges [ 52 ].
Nowadays, DL technology is considered as one of the hot topics within the area of machine learning, artificial intelligence as well as data science and analytics, due to its learning capabilities from the given data. Many corporations including Google, Microsoft, Nokia, etc., study it actively as it can provide significant results in different classification and regression problems and datasets [ 52 ]. In terms of working domain, DL is considered as a subset of ML and AI, and thus DL can be seen as an AI function that mimics the human brain’s processing of data. The worldwide popularity of “Deep learning” is increasing day by day, which is shown in our earlier paper [ 96 ] based on the historical data collected from Google trends [ 33 ]. Deep learning differs from standard machine learning in terms of efficiency as the volume of data increases, discussed briefly in Section “ Why Deep Learning in Today's Research and Applications? ”. DL technology uses multiple layers to represent the abstractions of data to build computational models. While deep learning takes a long time to train a model due to a large number of parameters, it takes a short amount of time to run during testing as compared to other machine learning algorithms [ 127 ].
While today’s Fourth Industrial Revolution (4IR or Industry 4.0) is typically focusing on technology-driven “automation, smart and intelligent systems”, DL technology, which is originated from ANN, has become one of the core technologies to achieve the goal [ 103 , 114 ]. A typical neural network is mainly composed of many simple, connected processing elements or processors called neurons, each of which generates a series of real-valued activations for the target outcome. Figure 1 shows a schematic representation of the mathematical model of an artificial neuron, i.e., processing element, highlighting input ( \(X_i\) ), weight ( w ), bias ( b ), summation function ( \(\sum\) ), activation function ( f ) and corresponding output signal ( y ). Neural network-based DL technology is now widely applied in many fields and research areas such as healthcare, sentiment analysis, natural language processing, visual recognition, business intelligence, cybersecurity, and many more that have been summarized in the latter part of this paper.
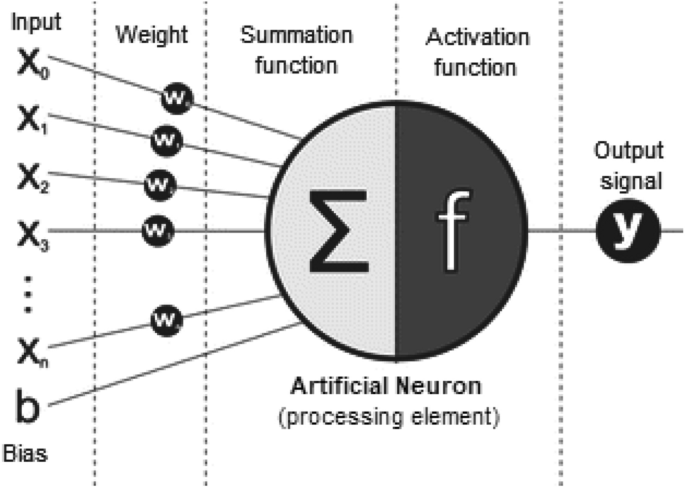
Schematic representation of the mathematical model of an artificial neuron (processing element), highlighting input ( \(X_i\) ), weight ( w ), bias ( b ), summation function ( \(\sum\) ), activation function ( f ) and output signal ( y )
Although DL models are successfully applied in various application areas, mentioned above, building an appropriate model of deep learning is a challenging task, due to the dynamic nature and variations of real-world problems and data. Moreover, DL models are typically considered as “black-box” machines that hamper the standard development of deep learning research and applications. Thus for clear understanding, in this paper, we present a structured and comprehensive view on DL techniques considering the variations in real-world problems and tasks. To achieve our goal, we briefly discuss various DL techniques and present a taxonomy by taking into account three major categories: (i) deep networks for supervised or discriminative learning that is utilized to provide a discriminative function in supervised deep learning or classification applications; (ii) deep networks for unsupervised or generative learning that are used to characterize the high-order correlation properties or features for pattern analysis or synthesis, thus can be used as preprocessing for the supervised algorithm; and (ii) deep networks for hybrid learning that is an integration of both supervised and unsupervised model and relevant others. We take into account such categories based on the nature and learning capabilities of different DL techniques and how they are used to solve problems in real-world applications [ 97 ]. Moreover, identifying key research issues and prospects including effective data representation, new algorithm design, data-driven hyper-parameter learning, and model optimization, integrating domain knowledge, adapting resource-constrained devices, etc. is one of the key targets of this study, which can lead to “Future Generation DL-Modeling”. Thus the goal of this paper is set to assist those in academia and industry as a reference guide, who want to research and develop data-driven smart and intelligent systems based on DL techniques.
The overall contribution of this paper is summarized as follows:
This article focuses on different aspects of deep learning modeling, i.e., the learning capabilities of DL techniques in different dimensions such as supervised or unsupervised tasks, to function in an automated and intelligent manner, which can play as a core technology of today’s Fourth Industrial Revolution (Industry 4.0).
We explore a variety of prominent DL techniques and present a taxonomy by taking into account the variations in deep learning tasks and how they are used for different purposes. In our taxonomy, we divide the techniques into three major categories such as deep networks for supervised or discriminative learning, unsupervised or generative learning, as well as deep networks for hybrid learning, and relevant others.
We have summarized several potential real-world application areas of deep learning, to assist developers as well as researchers in broadening their perspectives on DL techniques. Different categories of DL techniques highlighted in our taxonomy can be used to solve various issues accordingly.
Finally, we point out and discuss ten potential aspects with research directions for future generation DL modeling in terms of conducting future research and system development.
This paper is organized as follows. Section “ Why Deep Learning in Today's Research and Applications? ” motivates why deep learning is important to build data-driven intelligent systems. In Section“ Deep Learning Techniques and Applications ”, we present our DL taxonomy by taking into account the variations of deep learning tasks and how they are used in solving real-world issues and briefly discuss the techniques with summarizing the potential application areas. In Section “ Research Directions and Future Aspects ”, we discuss various research issues of deep learning-based modeling and highlight the promising topics for future research within the scope of our study. Finally, Section “ Concluding Remarks ” concludes this paper.
Why Deep Learning in Today’s Research and Applications?
The main focus of today’s Fourth Industrial Revolution (Industry 4.0) is typically technology-driven automation, smart and intelligent systems, in various application areas including smart healthcare, business intelligence, smart cities, cybersecurity intelligence, and many more [ 95 ]. Deep learning approaches have grown dramatically in terms of performance in a wide range of applications considering security technologies, particularly, as an excellent solution for uncovering complex architecture in high-dimensional data. Thus, DL techniques can play a key role in building intelligent data-driven systems according to today’s needs, because of their excellent learning capabilities from historical data. Consequently, DL can change the world as well as humans’ everyday life through its automation power and learning from experience. DL technology is therefore relevant to artificial intelligence [ 103 ], machine learning [ 97 ] and data science with advanced analytics [ 95 ] that are well-known areas in computer science, particularly, today’s intelligent computing. In the following, we first discuss regarding the position of deep learning in AI, or how DL technology is related to these areas of computing.
The Position of Deep Learning in AI
Nowadays, artificial intelligence (AI), machine learning (ML), and deep learning (DL) are three popular terms that are sometimes used interchangeably to describe systems or software that behaves intelligently. In Fig. 2 , we illustrate the position of deep Learning, comparing with machine learning and artificial intelligence. According to Fig. 2 , DL is a part of ML as well as a part of the broad area AI. In general, AI incorporates human behavior and intelligence to machines or systems [ 103 ], while ML is the method to learn from data or experience [ 97 ], which automates analytical model building. DL also represents learning methods from data where the computation is done through multi-layer neural networks and processing. The term “Deep” in the deep learning methodology refers to the concept of multiple levels or stages through which data is processed for building a data-driven model.
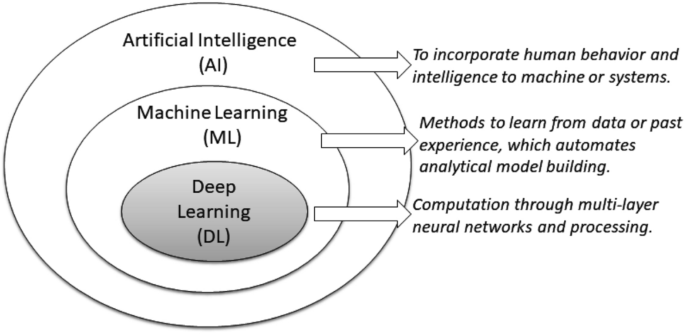
An illustration of the position of deep learning (DL), comparing with machine learning (ML) and artificial intelligence (AI)
Thus, DL can be considered as one of the core technology of AI, a frontier for artificial intelligence, which can be used for building intelligent systems and automation. More importantly, it pushes AI to a new level, termed “Smarter AI”. As DL are capable of learning from data, there is a strong relation of deep learning with “Data Science” [ 95 ] as well. Typically, data science represents the entire process of finding meaning or insights in data in a particular problem domain, where DL methods can play a key role for advanced analytics and intelligent decision-making [ 104 , 106 ]. Overall, we can conclude that DL technology is capable to change the current world, particularly, in terms of a powerful computational engine and contribute to technology-driven automation, smart and intelligent systems accordingly, and meets the goal of Industry 4.0.
Understanding Various Forms of Data
As DL models learn from data, an in-depth understanding and representation of data are important to build a data-driven intelligent system in a particular application area. In the real world, data can be in various forms, which typically can be represented as below for deep learning modeling:
Sequential Data Sequential data is any kind of data where the order matters, i,e., a set of sequences. It needs to explicitly account for the sequential nature of input data while building the model. Text streams, audio fragments, video clips, time-series data, are some examples of sequential data.
Image or 2D Data A digital image is made up of a matrix, which is a rectangular array of numbers, symbols, or expressions arranged in rows and columns in a 2D array of numbers. Matrix, pixels, voxels, and bit depth are the four essential characteristics or fundamental parameters of a digital image.
Tabular Data A tabular dataset consists primarily of rows and columns. Thus tabular datasets contain data in a columnar format as in a database table. Each column (field) must have a name and each column may only contain data of the defined type. Overall, it is a logical and systematic arrangement of data in the form of rows and columns that are based on data properties or features. Deep learning models can learn efficiently on tabular data and allow us to build data-driven intelligent systems.
The above-discussed data forms are common in the real-world application areas of deep learning. Different categories of DL techniques perform differently depending on the nature and characteristics of data, discussed briefly in Section “ Deep Learning Techniques and Applications ” with a taxonomy presentation. However, in many real-world application areas, the standard machine learning techniques, particularly, logic-rule or tree-based techniques [ 93 , 101 ] perform significantly depending on the application nature. Figure 3 also shows the performance comparison of DL and ML modeling considering the amount of data. In the following, we highlight several cases, where deep learning is useful to solve real-world problems, according to our main focus in this paper.
DL Properties and Dependencies
A DL model typically follows the same processing stages as machine learning modeling. In Fig. 4 , we have shown a deep learning workflow to solve real-world problems, which consists of three processing steps, such as data understanding and preprocessing, DL model building, and training, and validation and interpretation. However, unlike the ML modeling [ 98 , 108 ], feature extraction in the DL model is automated rather than manual. K-nearest neighbor, support vector machines, decision tree, random forest, naive Bayes, linear regression, association rules, k-means clustering, are some examples of machine learning techniques that are commonly used in various application areas [ 97 ]. On the other hand, the DL model includes convolution neural network, recurrent neural network, autoencoder, deep belief network, and many more, discussed briefly with their potential application areas in Section 3 . In the following, we discuss the key properties and dependencies of DL techniques, that are needed to take into account before started working on DL modeling for real-world applications.
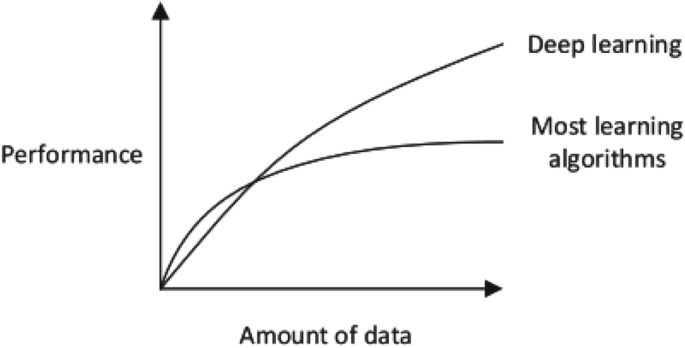
An illustration of the performance comparison between deep learning (DL) and other machine learning (ML) algorithms, where DL modeling from large amounts of data can increase the performance
Data Dependencies Deep learning is typically dependent on a large amount of data to build a data-driven model for a particular problem domain. The reason is that when the data volume is small, deep learning algorithms often perform poorly [ 64 ]. In such circumstances, however, the performance of the standard machine-learning algorithms will be improved if the specified rules are used [ 64 , 107 ].
Hardware Dependencies The DL algorithms require large computational operations while training a model with large datasets. As the larger the computations, the more the advantage of a GPU over a CPU, the GPU is mostly used to optimize the operations efficiently. Thus, to work properly with the deep learning training, GPU hardware is necessary. Therefore, DL relies more on high-performance machines with GPUs than standard machine learning methods [ 19 , 127 ].
Feature Engineering Process Feature engineering is the process of extracting features (characteristics, properties, and attributes) from raw data using domain knowledge. A fundamental distinction between DL and other machine-learning techniques is the attempt to extract high-level characteristics directly from data [ 22 , 97 ]. Thus, DL decreases the time and effort required to construct a feature extractor for each problem.
Model Training and Execution time In general, training a deep learning algorithm takes a long time due to a large number of parameters in the DL algorithm; thus, the model training process takes longer. For instance, the DL models can take more than one week to complete a training session, whereas training with ML algorithms takes relatively little time, only seconds to hours [ 107 , 127 ]. During testing, deep learning algorithms take extremely little time to run [ 127 ], when compared to certain machine learning methods.
Black-box Perception and Interpretability Interpretability is an important factor when comparing DL with ML. It’s difficult to explain how a deep learning result was obtained, i.e., “black-box”. On the other hand, the machine-learning algorithms, particularly, rule-based machine learning techniques [ 97 ] provide explicit logic rules (IF-THEN) for making decisions that are easily interpretable for humans. For instance, in our earlier works, we have presented several machines learning rule-based techniques [ 100 , 102 , 105 ], where the extracted rules are human-understandable and easier to interpret, update or delete according to the target applications.
The most significant distinction between deep learning and regular machine learning is how well it performs when data grows exponentially. An illustration of the performance comparison between DL and standard ML algorithms has been shown in Fig. 3 , where DL modeling can increase the performance with the amount of data. Thus, DL modeling is extremely useful when dealing with a large amount of data because of its capacity to process vast amounts of features to build an effective data-driven model. In terms of developing and training DL models, it relies on parallelized matrix and tensor operations as well as computing gradients and optimization. Several, DL libraries and resources [ 30 ] such as PyTorch [ 82 ] (with a high-level API called Lightning) and TensorFlow [ 1 ] (which also offers Keras as a high-level API) offers these core utilities including many pre-trained models, as well as many other necessary functions for implementation and DL model building.
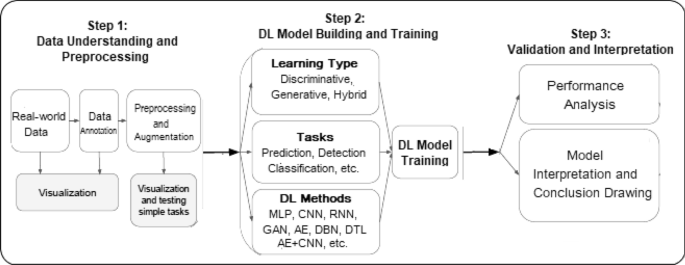
A typical DL workflow to solve real-world problems, which consists of three sequential stages (i) data understanding and preprocessing (ii) DL model building and training (iii) validation and interpretation
Deep Learning Techniques and Applications
In this section, we go through the various types of deep neural network techniques, which typically consider several layers of information-processing stages in hierarchical structures to learn. A typical deep neural network contains multiple hidden layers including input and output layers. Figure 5 shows a general structure of a deep neural network ( \(hidden \; layer=N\) and N \(\ge\) 2) comparing with a shallow network ( \(hidden \; layer=1\) ). We also present our taxonomy on DL techniques based on how they are used to solve various problems, in this section. However, before exploring the details of the DL techniques, it’s useful to review various types of learning tasks such as (i) Supervised: a task-driven approach that uses labeled training data, (ii) Unsupervised: a data-driven process that analyzes unlabeled datasets, (iii) Semi-supervised: a hybridization of both the supervised and unsupervised methods, and (iv) Reinforcement: an environment driven approach, discussed briefly in our earlier paper [ 97 ]. Thus, to present our taxonomy, we divide DL techniques broadly into three major categories: (i) deep networks for supervised or discriminative learning; (ii) deep networks for unsupervised or generative learning; and (ii) deep networks for hybrid learning combing both and relevant others, as shown in Fig. 6 . In the following, we briefly discuss each of these techniques that can be used to solve real-world problems in various application areas according to their learning capabilities.
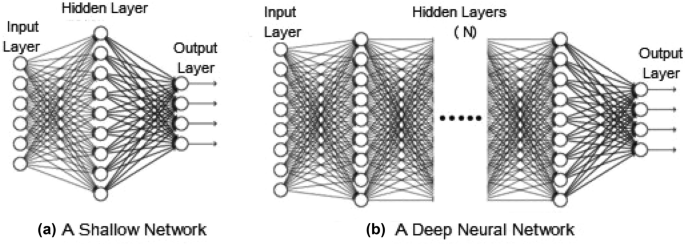
A general architecture of a a shallow network with one hidden layer and b a deep neural network with multiple hidden layers
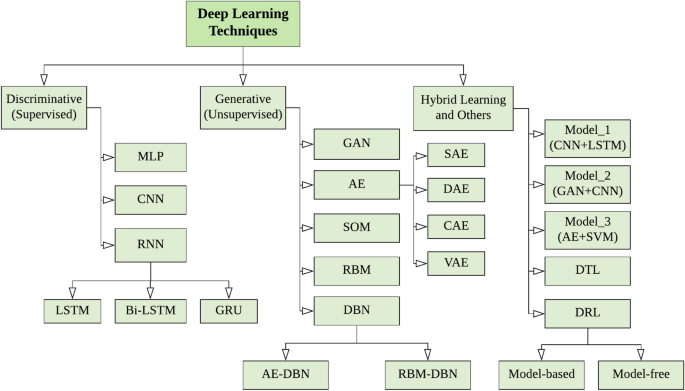
A taxonomy of DL techniques, broadly divided into three major categories (i) deep networks for supervised or discriminative learning, (ii) deep networks for unsupervised or generative learning, and (ii) deep networks for hybrid learning and relevant others
Deep Networks for Supervised or Discriminative Learning
This category of DL techniques is utilized to provide a discriminative function in supervised or classification applications. Discriminative deep architectures are typically designed to give discriminative power for pattern classification by describing the posterior distributions of classes conditioned on visible data [ 21 ]. Discriminative architectures mainly include Multi-Layer Perceptron (MLP), Convolutional Neural Networks (CNN or ConvNet), Recurrent Neural Networks (RNN), along with their variants. In the following, we briefly discuss these techniques.
Multi-layer Perceptron (MLP)
Multi-layer Perceptron (MLP), a supervised learning approach [ 83 ], is a type of feedforward artificial neural network (ANN). It is also known as the foundation architecture of deep neural networks (DNN) or deep learning. A typical MLP is a fully connected network that consists of an input layer that receives input data, an output layer that makes a decision or prediction about the input signal, and one or more hidden layers between these two that are considered as the network’s computational engine [ 36 , 103 ]. The output of an MLP network is determined using a variety of activation functions, also known as transfer functions, such as ReLU (Rectified Linear Unit), Tanh, Sigmoid, and Softmax [ 83 , 96 ]. To train MLP employs the most extensively used algorithm “Backpropagation” [ 36 ], a supervised learning technique, which is also known as the most basic building block of a neural network. During the training process, various optimization approaches such as Stochastic Gradient Descent (SGD), Limited Memory BFGS (L-BFGS), and Adaptive Moment Estimation (Adam) are applied. MLP requires tuning of several hyperparameters such as the number of hidden layers, neurons, and iterations, which could make solving a complicated model computationally expensive. However, through partial fit, MLP offers the advantage of learning non-linear models in real-time or online [ 83 ].
Convolutional Neural Network (CNN or ConvNet)
The Convolutional Neural Network (CNN or ConvNet) [ 65 ] is a popular discriminative deep learning architecture that learns directly from the input without the need for human feature extraction. Figure 7 shows an example of a CNN including multiple convolutions and pooling layers. As a result, the CNN enhances the design of traditional ANN like regularized MLP networks. Each layer in CNN takes into account optimum parameters for a meaningful output as well as reduces model complexity. CNN also uses a ‘dropout’ [ 30 ] that can deal with the problem of over-fitting, which may occur in a traditional network.
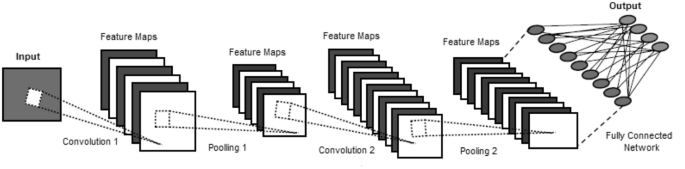
An example of a convolutional neural network (CNN or ConvNet) including multiple convolution and pooling layers
CNNs are specifically intended to deal with a variety of 2D shapes and are thus widely employed in visual recognition, medical image analysis, image segmentation, natural language processing, and many more [ 65 , 96 ]. The capability of automatically discovering essential features from the input without the need for human intervention makes it more powerful than a traditional network. Several variants of CNN are exist in the area that includes visual geometry group (VGG) [ 38 ], AlexNet [ 62 ], Xception [ 17 ], Inception [ 116 ], ResNet [ 39 ], etc. that can be used in various application domains according to their learning capabilities.
Recurrent Neural Network (RNN) and its Variants
A Recurrent Neural Network (RNN) is another popular neural network, which employs sequential or time-series data and feeds the output from the previous step as input to the current stage [ 27 , 74 ]. Like feedforward and CNN, recurrent networks learn from training input, however, distinguish by their “memory”, which allows them to impact current input and output through using information from previous inputs. Unlike typical DNN, which assumes that inputs and outputs are independent of one another, the output of RNN is reliant on prior elements within the sequence. However, standard recurrent networks have the issue of vanishing gradients, which makes learning long data sequences challenging. In the following, we discuss several popular variants of the recurrent network that minimizes the issues and perform well in many real-world application domains.
Long short-term memory (LSTM) This is a popular form of RNN architecture that uses special units to deal with the vanishing gradient problem, which was introduced by Hochreiter et al. [ 42 ]. A memory cell in an LSTM unit can store data for long periods and the flow of information into and out of the cell is managed by three gates. For instance, the ‘Forget Gate’ determines what information from the previous state cell will be memorized and what information will be removed that is no longer useful, while the ‘Input Gate’ determines which information should enter the cell state and the ‘Output Gate’ determines and controls the outputs. As it solves the issues of training a recurrent network, the LSTM network is considered one of the most successful RNN.
Bidirectional RNN/LSTM Bidirectional RNNs connect two hidden layers that run in opposite directions to a single output, allowing them to accept data from both the past and future. Bidirectional RNNs, unlike traditional recurrent networks, are trained to predict both positive and negative time directions at the same time. A Bidirectional LSTM, often known as a BiLSTM, is an extension of the standard LSTM that can increase model performance on sequence classification issues [ 113 ]. It is a sequence processing model comprising of two LSTMs: one takes the input forward and the other takes it backward. Bidirectional LSTM in particular is a popular choice in natural language processing tasks.
Gated recurrent units (GRUs) A Gated Recurrent Unit (GRU) is another popular variant of the recurrent network that uses gating methods to control and manage information flow between cells in the neural network, introduced by Cho et al. [ 16 ]. The GRU is like an LSTM, however, has fewer parameters, as it has a reset gate and an update gate but lacks the output gate, as shown in Fig. 8 . Thus, the key difference between a GRU and an LSTM is that a GRU has two gates (reset and update gates) whereas an LSTM has three gates (namely input, output and forget gates). The GRU’s structure enables it to capture dependencies from large sequences of data in an adaptive manner, without discarding information from earlier parts of the sequence. Thus GRU is a slightly more streamlined variant that often offers comparable performance and is significantly faster to compute [ 18 ]. Although GRUs have been shown to exhibit better performance on certain smaller and less frequent datasets [ 18 , 34 ], both variants of RNN have proven their effectiveness while producing the outcome.
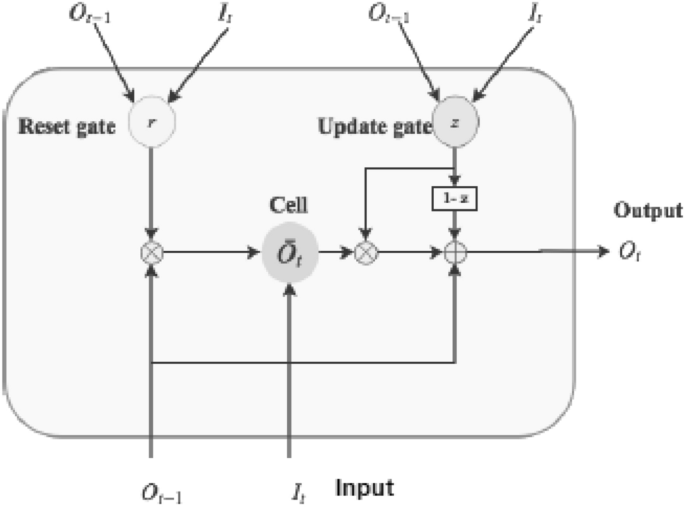
Basic structure of a gated recurrent unit (GRU) cell consisting of reset and update gates
Overall, the basic property of a recurrent network is that it has at least one feedback connection, which enables activations to loop. This allows the networks to do temporal processing and sequence learning, such as sequence recognition or reproduction, temporal association or prediction, etc. Following are some popular application areas of recurrent networks such as prediction problems, machine translation, natural language processing, text summarization, speech recognition, and many more.
Deep Networks for Generative or Unsupervised Learning
This category of DL techniques is typically used to characterize the high-order correlation properties or features for pattern analysis or synthesis, as well as the joint statistical distributions of the visible data and their associated classes [ 21 ]. The key idea of generative deep architectures is that during the learning process, precise supervisory information such as target class labels is not of concern. As a result, the methods under this category are essentially applied for unsupervised learning as the methods are typically used for feature learning or data generating and representation [ 20 , 21 ]. Thus generative modeling can be used as preprocessing for the supervised learning tasks as well, which ensures the discriminative model accuracy. Commonly used deep neural network techniques for unsupervised or generative learning are Generative Adversarial Network (GAN), Autoencoder (AE), Restricted Boltzmann Machine (RBM), Self-Organizing Map (SOM), and Deep Belief Network (DBN) along with their variants.
Generative Adversarial Network (GAN)
A Generative Adversarial Network (GAN), designed by Ian Goodfellow [ 32 ], is a type of neural network architecture for generative modeling to create new plausible samples on demand. It involves automatically discovering and learning regularities or patterns in input data so that the model may be used to generate or output new examples from the original dataset. As shown in Fig. 9 , GANs are composed of two neural networks, a generator G that creates new data having properties similar to the original data, and a discriminator D that predicts the likelihood of a subsequent sample being drawn from actual data rather than data provided by the generator. Thus in GAN modeling, both the generator and discriminator are trained to compete with each other. While the generator tries to fool and confuse the discriminator by creating more realistic data, the discriminator tries to distinguish the genuine data from the fake data generated by G .
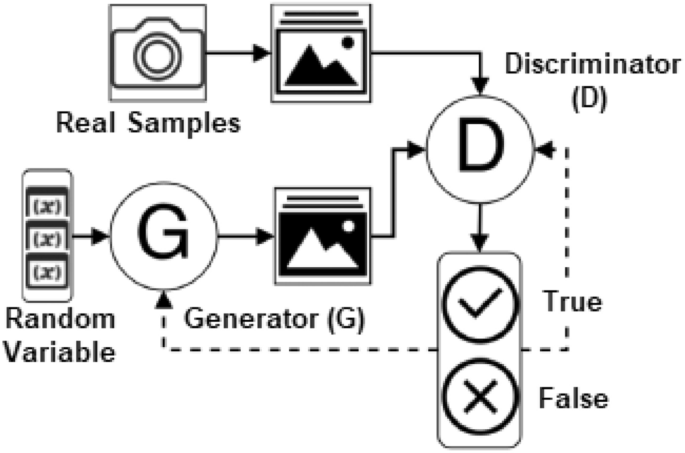
Schematic structure of a standard generative adversarial network (GAN)
Generally, GAN network deployment is designed for unsupervised learning tasks, but it has also proven to be a better solution for semi-supervised and reinforcement learning as well depending on the task [ 3 ]. GANs are also used in state-of-the-art transfer learning research to enforce the alignment of the latent feature space [ 66 ]. Inverse models, such as Bidirectional GAN (BiGAN) [ 25 ] can also learn a mapping from data to the latent space, similar to how the standard GAN model learns a mapping from a latent space to the data distribution. The potential application areas of GAN networks are healthcare, image analysis, data augmentation, video generation, voice generation, pandemics, traffic control, cybersecurity, and many more, which are increasing rapidly. Overall, GANs have established themselves as a comprehensive domain of independent data expansion and as a solution to problems requiring a generative solution.
Auto-Encoder (AE) and Its Variants
An auto-encoder (AE) [ 31 ] is a popular unsupervised learning technique in which neural networks are used to learn representations. Typically, auto-encoders are used to work with high-dimensional data, and dimensionality reduction explains how a set of data is represented. Encoder, code, and decoder are the three parts of an autoencoder. The encoder compresses the input and generates the code, which the decoder subsequently uses to reconstruct the input. The AEs have recently been used to learn generative data models [ 69 ]. The auto-encoder is widely used in many unsupervised learning tasks, e.g., dimensionality reduction, feature extraction, efficient coding, generative modeling, denoising, anomaly or outlier detection, etc. [ 31 , 132 ]. Principal component analysis (PCA) [ 99 ], which is also used to reduce the dimensionality of huge data sets, is essentially similar to a single-layered AE with a linear activation function. Regularized autoencoders such as sparse, denoising, and contractive are useful for learning representations for later classification tasks [ 119 ], while variational autoencoders can be used as generative models [ 56 ], discussed below.
Sparse Autoencoder (SAE) A sparse autoencoder [ 73 ] has a sparsity penalty on the coding layer as a part of its training requirement. SAEs may have more hidden units than inputs, but only a small number of hidden units are permitted to be active at the same time, resulting in a sparse model. Figure 10 shows a schematic structure of a sparse autoencoder with several active units in the hidden layer. This model is thus obliged to respond to the unique statistical features of the training data following its constraints.
Denoising Autoencoder (DAE) A denoising autoencoder is a variant on the basic autoencoder that attempts to improve representation (to extract useful features) by altering the reconstruction criterion, and thus reduces the risk of learning the identity function [ 31 , 119 ]. In other words, it receives a corrupted data point as input and is trained to recover the original undistorted input as its output through minimizing the average reconstruction error over the training data, i.e, cleaning the corrupted input, or denoising. Thus, in the context of computing, DAEs can be considered as very powerful filters that can be utilized for automatic pre-processing. A denoising autoencoder, for example, could be used to automatically pre-process an image, thereby boosting its quality for recognition accuracy.
Contractive Autoencoder (CAE) The idea behind a contractive autoencoder, proposed by Rifai et al. [ 90 ], is to make the autoencoders robust of small changes in the training dataset. In its objective function, a CAE includes an explicit regularizer that forces the model to learn an encoding that is robust to small changes in input values. As a result, the learned representation’s sensitivity to the training input is reduced. While DAEs encourage the robustness of reconstruction as discussed above, CAEs encourage the robustness of representation.
Variational Autoencoder (VAE) A variational autoencoder [ 55 ] has a fundamentally unique property that distinguishes it from the classical autoencoder discussed above, which makes this so effective for generative modeling. VAEs, unlike the traditional autoencoders which map the input onto a latent vector, map the input data into the parameters of a probability distribution, such as the mean and variance of a Gaussian distribution. A VAE assumes that the source data has an underlying probability distribution and then tries to discover the distribution’s parameters. Although this approach was initially designed for unsupervised learning, its use has been demonstrated in other domains such as semi-supervised learning [ 128 ] and supervised learning [ 51 ].
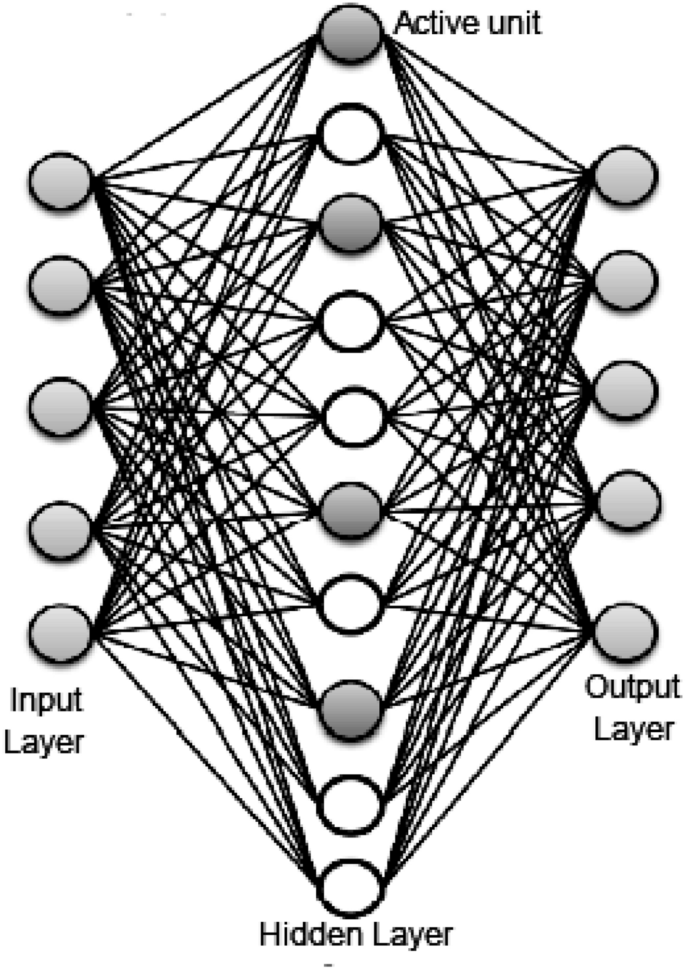
Schematic structure of a sparse autoencoder (SAE) with several active units (filled circle) in the hidden layer
Although, the earlier concept of AE was typically for dimensionality reduction or feature learning mentioned above, recently, AEs have been brought to the forefront of generative modeling, even the generative adversarial network is one of the popular methods in the area. The AEs have been effectively employed in a variety of domains, including healthcare, computer vision, speech recognition, cybersecurity, natural language processing, and many more. Overall, we can conclude that auto-encoder and its variants can play a significant role as unsupervised feature learning with neural network architecture.
Kohonen Map or Self-Organizing Map (SOM)
A Self-Organizing Map (SOM) or Kohonen Map [ 59 ] is another form of unsupervised learning technique for creating a low-dimensional (usually two-dimensional) representation of a higher-dimensional data set while maintaining the topological structure of the data. SOM is also known as a neural network-based dimensionality reduction algorithm that is commonly used for clustering [ 118 ]. A SOM adapts to the topological form of a dataset by repeatedly moving its neurons closer to the data points, allowing us to visualize enormous datasets and find probable clusters. The first layer of a SOM is the input layer, and the second layer is the output layer or feature map. Unlike other neural networks that use error-correction learning, such as backpropagation with gradient descent [ 36 ], SOMs employ competitive learning, which uses a neighborhood function to retain the input space’s topological features. SOM is widely utilized in a variety of applications, including pattern identification, health or medical diagnosis, anomaly detection, and virus or worm attack detection [ 60 , 87 ]. The primary benefit of employing a SOM is that this can make high-dimensional data easier to visualize and analyze to understand the patterns. The reduction of dimensionality and grid clustering makes it easy to observe similarities in the data. As a result, SOMs can play a vital role in developing a data-driven effective model for a particular problem domain, depending on the data characteristics.
Restricted Boltzmann Machine (RBM)
A Restricted Boltzmann Machine (RBM) [ 75 ] is also a generative stochastic neural network capable of learning a probability distribution across its inputs. Boltzmann machines typically consist of visible and hidden nodes and each node is connected to every other node, which helps us understand irregularities by learning how the system works in normal circumstances. RBMs are a subset of Boltzmann machines that have a limit on the number of connections between the visible and hidden layers [ 77 ]. This restriction permits training algorithms like the gradient-based contrastive divergence algorithm to be more efficient than those for Boltzmann machines in general [ 41 ]. RBMs have found applications in dimensionality reduction, classification, regression, collaborative filtering, feature learning, topic modeling, and many others. In the area of deep learning modeling, they can be trained either supervised or unsupervised, depending on the task. Overall, the RBMs can recognize patterns in data automatically and develop probabilistic or stochastic models, which are utilized for feature selection or extraction, as well as forming a deep belief network.
Deep Belief Network (DBN)
A Deep Belief Network (DBN) [ 40 ] is a multi-layer generative graphical model of stacking several individual unsupervised networks such as AEs or RBMs, that use each network’s hidden layer as the input for the next layer, i.e, connected sequentially. Thus, we can divide a DBN into (i) AE-DBN which is known as stacked AE, and (ii) RBM-DBN that is known as stacked RBM, where AE-DBN is composed of autoencoders and RBM-DBN is composed of restricted Boltzmann machines, discussed earlier. The ultimate goal is to develop a faster-unsupervised training technique for each sub-network that depends on contrastive divergence [ 41 ]. DBN can capture a hierarchical representation of input data based on its deep structure. The primary idea behind DBN is to train unsupervised feed-forward neural networks with unlabeled data before fine-tuning the network with labeled input. One of the most important advantages of DBN, as opposed to typical shallow learning networks, is that it permits the detection of deep patterns, which allows for reasoning abilities and the capture of the deep difference between normal and erroneous data [ 89 ]. A continuous DBN is simply an extension of a standard DBN that allows a continuous range of decimals instead of binary data. Overall, the DBN model can play a key role in a wide range of high-dimensional data applications due to its strong feature extraction and classification capabilities and become one of the significant topics in the field of neural networks.
In summary, the generative learning techniques discussed above typically allow us to generate a new representation of data through exploratory analysis. As a result, these deep generative networks can be utilized as preprocessing for supervised or discriminative learning tasks, as well as ensuring model accuracy, where unsupervised representation learning can allow for improved classifier generalization.
Deep Networks for Hybrid Learning and Other Approaches
In addition to the above-discussed deep learning categories, hybrid deep networks and several other approaches such as deep transfer learning (DTL) and deep reinforcement learning (DRL) are popular, which are discussed in the following.
Hybrid Deep Neural Networks
Generative models are adaptable, with the capacity to learn from both labeled and unlabeled data. Discriminative models, on the other hand, are unable to learn from unlabeled data yet outperform their generative counterparts in supervised tasks. A framework for training both deep generative and discriminative models simultaneously can enjoy the benefits of both models, which motivates hybrid networks.
Hybrid deep learning models are typically composed of multiple (two or more) deep basic learning models, where the basic model is a discriminative or generative deep learning model discussed earlier. Based on the integration of different basic generative or discriminative models, the below three categories of hybrid deep learning models might be useful for solving real-world problems. These are as follows:
Hybrid \(Model\_1\) : An integration of different generative or discriminative models to extract more meaningful and robust features. Examples could be CNN+LSTM, AE+GAN, and so on.
Hybrid \(Model\_2\) : An integration of generative model followed by a discriminative model. Examples could be DBN+MLP, GAN+CNN, AE+CNN, and so on.
Hybrid \(Model\_3\) : An integration of generative or discriminative model followed by a non-deep learning classifier. Examples could be AE+SVM, CNN+SVM, and so on.
Thus, in a broad sense, we can conclude that hybrid models can be either classification-focused or non-classification depending on the target use. However, most of the hybrid learning-related studies in the area of deep learning are classification-focused or supervised learning tasks, summarized in Table 1 . The unsupervised generative models with meaningful representations are employed to enhance the discriminative models. The generative models with useful representation can provide more informative and low-dimensional features for discrimination, and they can also enable to enhance the training data quality and quantity, providing additional information for classification.
Deep Transfer Learning (DTL)
Transfer Learning is a technique for effectively using previously learned model knowledge to solve a new task with minimum training or fine-tuning. In comparison to typical machine learning techniques [ 97 ], DL takes a large amount of training data. As a result, the need for a substantial volume of labeled data is a significant barrier to address some essential domain-specific tasks, particularly, in the medical sector, where creating large-scale, high-quality annotated medical or health datasets is both difficult and costly. Furthermore, the standard DL model demands a lot of computational resources, such as a GPU-enabled server, even though researchers are working hard to improve it. As a result, Deep Transfer Learning (DTL), a DL-based transfer learning method, might be helpful to address this issue. Figure 11 shows a general structure of the transfer learning process, where knowledge from the pre-trained model is transferred into a new DL model. It’s especially popular in deep learning right now since it allows to train deep neural networks with very little data [ 126 ].
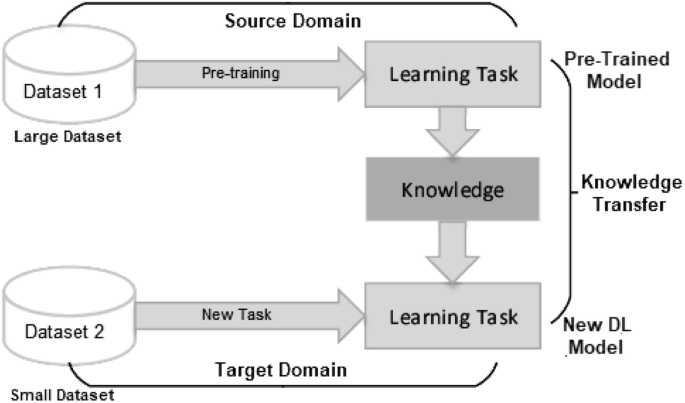
A general structure of transfer learning process, where knowledge from pre-trained model is transferred into new DL model
Transfer learning is a two-stage approach for training a DL model that consists of a pre-training step and a fine-tuning step in which the model is trained on the target task. Since deep neural networks have gained popularity in a variety of fields, a large number of DTL methods have been presented, making it crucial to categorize and summarize them. Based on the techniques used in the literature, DTL can be classified into four categories [ 117 ]. These are (i) instances-based deep transfer learning that utilizes instances in source domain by appropriate weight, (ii) mapping-based deep transfer learning that maps instances from two domains into a new data space with better similarity, (iii) network-based deep transfer learning that reuses the partial of network pre-trained in the source domain, and (iv) adversarial based deep transfer learning that uses adversarial technology to find transferable features that both suitable for two domains. Due to its high effectiveness and practicality, adversarial-based deep transfer learning has exploded in popularity in recent years. Transfer learning can also be classified into inductive, transductive, and unsupervised transfer learning depending on the circumstances between the source and target domains and activities [ 81 ]. While most current research focuses on supervised learning, how deep neural networks can transfer knowledge in unsupervised or semi-supervised learning may gain further interest in the future. DTL techniques are useful in a variety of fields including natural language processing, sentiment classification, visual recognition, speech recognition, spam filtering, and relevant others.
Deep Reinforcement Learning (DRL)
Reinforcement learning takes a different approach to solving the sequential decision-making problem than other approaches we have discussed so far. The concepts of an environment and an agent are often introduced first in reinforcement learning. The agent can perform a series of actions in the environment, each of which has an impact on the environment’s state and can result in possible rewards (feedback) - “positive” for good sequences of actions that result in a “good” state, and “negative” for bad sequences of actions that result in a “bad” state. The purpose of reinforcement learning is to learn good action sequences through interaction with the environment, typically referred to as a policy.
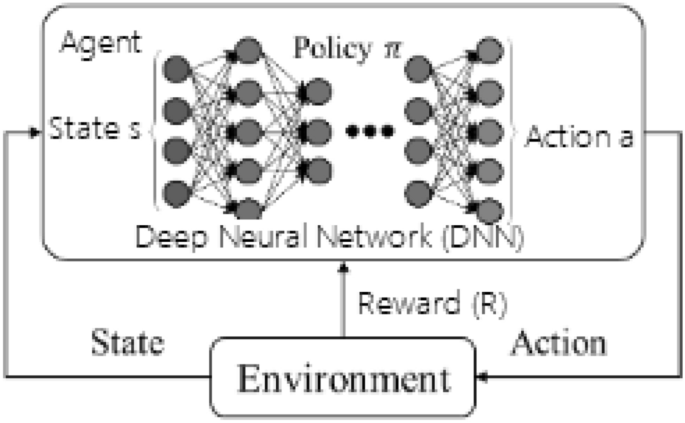
Schematic structure of deep reinforcement learning (DRL) highlighting a deep neural network
Deep reinforcement learning (DRL or deep RL) [ 9 ] integrates neural networks with a reinforcement learning architecture to allow the agents to learn the appropriate actions in a virtual environment, as shown in Fig. 12 . In the area of reinforcement learning, model-based RL is based on learning a transition model that enables for modeling of the environment without interacting with it directly, whereas model-free RL methods learn directly from interactions with the environment. Q-learning is a popular model-free RL technique for determining the best action-selection policy for any (finite) Markov Decision Process (MDP) [ 86 , 97 ]. MDP is a mathematical framework for modeling decisions based on state, action, and rewards [ 86 ]. In addition, Deep Q-Networks, Double DQN, Bi-directional Learning, Monte Carlo Control, etc. are used in the area [ 50 , 97 ]. In DRL methods it incorporates DL models, e.g. Deep Neural Networks (DNN), based on MDP principle [ 71 ], as policy and/or value function approximators. CNN for example can be used as a component of RL agents to learn directly from raw, high-dimensional visual inputs. In the real world, DRL-based solutions can be used in several application areas including robotics, video games, natural language processing, computer vision, and relevant others.
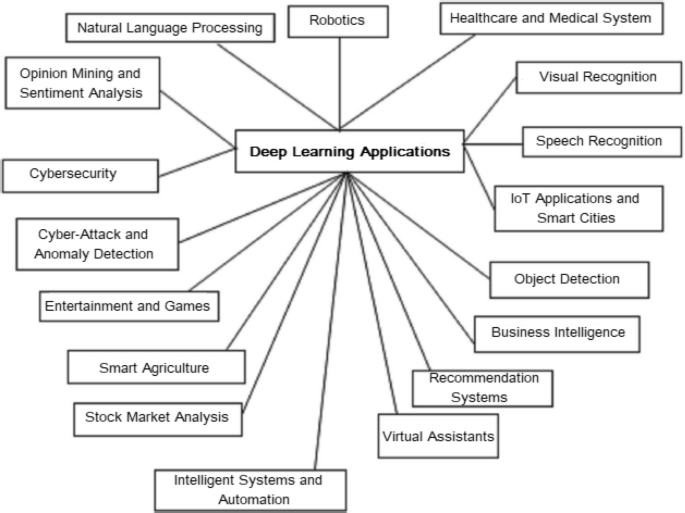
Several potential real-world application areas of deep learning
Deep Learning Application Summary
During the past few years, deep learning has been successfully applied to numerous problems in many application areas. These include natural language processing, sentiment analysis, cybersecurity, business, virtual assistants, visual recognition, healthcare, robotics, and many more. In Fig. 13 , we have summarized several potential real-world application areas of deep learning. Various deep learning techniques according to our presented taxonomy in Fig. 6 that includes discriminative learning, generative learning, as well as hybrid models, discussed earlier, are employed in these application areas. In Table 1 , we have also summarized various deep learning tasks and techniques that are used to solve the relevant tasks in several real-world applications areas. Overall, from Fig. 13 and Table 1 , we can conclude that the future prospects of deep learning modeling in real-world application areas are huge and there are lots of scopes to work. In the next section, we also summarize the research issues in deep learning modeling and point out the potential aspects for future generation DL modeling.
Research Directions and Future Aspects
While existing methods have established a solid foundation for deep learning systems and research, this section outlines the below ten potential future research directions based on our study.
Automation in Data Annotation According to the existing literature, discussed in Section 3 , most of the deep learning models are trained through publicly available datasets that are annotated. However, to build a system for a new problem domain or recent data-driven system, raw data from relevant sources are needed to collect. Thus, data annotation, e.g., categorization, tagging, or labeling of a large amount of raw data, is important for building discriminative deep learning models or supervised tasks, which is challenging. A technique with the capability of automatic and dynamic data annotation, rather than manual annotation or hiring annotators, particularly, for large datasets, could be more effective for supervised learning as well as minimizing human effort. Therefore, a more in-depth investigation of data collection and annotation methods, or designing an unsupervised learning-based solution could be one of the primary research directions in the area of deep learning modeling.
Data Preparation for Ensuring Data Quality As discussed earlier throughout the paper, the deep learning algorithms highly impact data quality, and availability for training, and consequently on the resultant model for a particular problem domain. Thus, deep learning models may become worthless or yield decreased accuracy if the data is bad, such as data sparsity, non-representative, poor-quality, ambiguous values, noise, data imbalance, irrelevant features, data inconsistency, insufficient quantity, and so on for training. Consequently, such issues in data can lead to poor processing and inaccurate findings, which is a major problem while discovering insights from data. Thus deep learning models also need to adapt to such rising issues in data, to capture approximated information from observations. Therefore, effective data pre-processing techniques are needed to design according to the nature of the data problem and characteristics, to handling such emerging challenges, which could be another research direction in the area.
Black-box Perception and Proper DL/ML Algorithm Selection In general, it’s difficult to explain how a deep learning result is obtained or how they get the ultimate decisions for a particular model. Although DL models achieve significant performance while learning from large datasets, as discussed in Section 2 , this “black-box” perception of DL modeling typically represents weak statistical interpretability that could be a major issue in the area. On the other hand, ML algorithms, particularly, rule-based machine learning techniques provide explicit logic rules (IF-THEN) for making decisions that are easier to interpret, update or delete according to the target applications [ 97 , 100 , 105 ]. If the wrong learning algorithm is chosen, unanticipated results may occur, resulting in a loss of effort as well as the model’s efficacy and accuracy. Thus by taking into account the performance, complexity, model accuracy, and applicability, selecting an appropriate model for the target application is challenging, and in-depth analysis is needed for better understanding and decision making.
Deep Networks for Supervised or Discriminative Learning: According to our designed taxonomy of deep learning techniques, as shown in Fig. 6 , discriminative architectures mainly include MLP, CNN, and RNN, along with their variants that are applied widely in various application domains. However, designing new techniques or their variants of such discriminative techniques by taking into account model optimization, accuracy, and applicability, according to the target real-world application and the nature of the data, could be a novel contribution, which can also be considered as a major future aspect in the area of supervised or discriminative learning.
Deep Networks for Unsupervised or Generative Learning As discussed in Section 3 , unsupervised learning or generative deep learning modeling is one of the major tasks in the area, as it allows us to characterize the high-order correlation properties or features in data, or generating a new representation of data through exploratory analysis. Moreover, unlike supervised learning [ 97 ], it does not require labeled data due to its capability to derive insights directly from the data as well as data-driven decision making. Consequently, it thus can be used as preprocessing for supervised learning or discriminative modeling as well as semi-supervised learning tasks, which ensure learning accuracy and model efficiency. According to our designed taxonomy of deep learning techniques, as shown in Fig. 6 , generative techniques mainly include GAN, AE, SOM, RBM, DBN, and their variants. Thus, designing new techniques or their variants for an effective data modeling or representation according to the target real-world application could be a novel contribution, which can also be considered as a major future aspect in the area of unsupervised or generative learning.
Hybrid/Ensemble Modeling and Uncertainty Handling According to our designed taxonomy of DL techniques, as shown in Fig 6 , this is considered as another major category in deep learning tasks. As hybrid modeling enjoys the benefits of both generative and discriminative learning, an effective hybridization can outperform others in terms of performance as well as uncertainty handling in high-risk applications. In Section 3 , we have summarized various types of hybridization, e.g., AE+CNN/SVM. Since a group of neural networks is trained with distinct parameters or with separate sub-sampling training datasets, hybridization or ensembles of such techniques, i.e., DL with DL/ML, can play a key role in the area. Thus designing effective blended discriminative and generative models accordingly rather than naive method, could be an important research opportunity to solve various real-world issues including semi-supervised learning tasks and model uncertainty.
Dynamism in Selecting Threshold/ Hyper-parameters Values, and Network Structures with Computational Efficiency In general, the relationship among performance, model complexity, and computational requirements is a key issue in deep learning modeling and applications. A combination of algorithmic advancements with improved accuracy as well as maintaining computational efficiency, i.e., achieving the maximum throughput while consuming the least amount of resources, without significant information loss, can lead to a breakthrough in the effectiveness of deep learning modeling in future real-world applications. The concept of incremental approaches or recency-based learning [ 100 ] might be effective in several cases depending on the nature of target applications. Moreover, assuming the network structures with a static number of nodes and layers, hyper-parameters values or threshold settings, or selecting them by the trial-and-error process may not be effective in many cases, as it can be changed due to the changes in data. Thus, a data-driven approach to select them dynamically could be more effective while building a deep learning model in terms of both performance and real-world applicability. Such type of data-driven automation can lead to future generation deep learning modeling with additional intelligence, which could be a significant future aspect in the area as well as an important research direction to contribute.
Lightweight Deep Learning Modeling for Next-Generation Smart Devices and Applications: In recent years, the Internet of Things (IoT) consisting of billions of intelligent and communicating things and mobile communications technologies have become popular to detect and gather human and environmental information (e.g. geo-information, weather data, bio-data, human behaviors, and so on) for a variety of intelligent services and applications. Every day, these ubiquitous smart things or devices generate large amounts of data, requiring rapid data processing on a variety of smart mobile devices [ 72 ]. Deep learning technologies can be incorporate to discover underlying properties and to effectively handle such large amounts of sensor data for a variety of IoT applications including health monitoring and disease analysis, smart cities, traffic flow prediction, and monitoring, smart transportation, manufacture inspection, fault assessment, smart industry or Industry 4.0, and many more. Although deep learning techniques discussed in Section 3 are considered as powerful tools for processing big data, lightweight modeling is important for resource-constrained devices, due to their high computational cost and considerable memory overhead. Thus several techniques such as optimization, simplification, compression, pruning, generalization, important feature extraction, etc. might be helpful in several cases. Therefore, constructing the lightweight deep learning techniques based on a baseline network architecture to adapt the DL model for next-generation mobile, IoT, or resource-constrained devices and applications, could be considered as a significant future aspect in the area.
Incorporating Domain Knowledge into Deep Learning Modeling Domain knowledge, as opposed to general knowledge or domain-independent knowledge, is knowledge of a specific, specialized topic or field. For instance, in terms of natural language processing, the properties of the English language typically differ from other languages like Bengali, Arabic, French, etc. Thus integrating domain-based constraints into the deep learning model could produce better results for such particular purpose. For instance, a task-specific feature extractor considering domain knowledge in smart manufacturing for fault diagnosis can resolve the issues in traditional deep-learning-based methods [ 28 ]. Similarly, domain knowledge in medical image analysis [ 58 ], financial sentiment analysis [ 49 ], cybersecurity analytics [ 94 , 103 ] as well as conceptual data model in which semantic information, (i.e., meaningful for a system, rather than merely correlational) [ 45 , 121 , 131 ] is included, can play a vital role in the area. Transfer learning could be an effective way to get started on a new challenge with domain knowledge. Moreover, contextual information such as spatial, temporal, social, environmental contexts [ 92 , 104 , 108 ] can also play an important role to incorporate context-aware computing with domain knowledge for smart decision making as well as building adaptive and intelligent context-aware systems. Therefore understanding domain knowledge and effectively incorporating them into the deep learning model could be another research direction.
Designing General Deep Learning Framework for Target Application Domains One promising research direction for deep learning-based solutions is to develop a general framework that can handle data diversity, dimensions, stimulation types, etc. The general framework would require two key capabilities: the attention mechanism that focuses on the most valuable parts of input signals, and the ability to capture latent feature that enables the framework to capture the distinctive and informative features. Attention models have been a popular research topic because of their intuition, versatility, and interpretability, and employed in various application areas like computer vision, natural language processing, text or image classification, sentiment analysis, recommender systems, user profiling, etc [ 13 , 80 ]. Attention mechanism can be implemented based on learning algorithms such as reinforcement learning that is capable of finding the most useful part through a policy search [ 133 , 134 ]. Similarly, CNN can be integrated with suitable attention mechanisms to form a general classification framework, where CNN can be used as a feature learning tool for capturing features in various levels and ranges. Thus, designing a general deep learning framework considering attention as well as a latent feature for target application domains could be another area to contribute.
To summarize, deep learning is a fairly open topic to which academics can contribute by developing new methods or improving existing methods to handle the above-mentioned concerns and tackle real-world problems in a variety of application areas. This can also help the researchers conduct a thorough analysis of the application’s hidden and unexpected challenges to produce more reliable and realistic outcomes. Overall, we can conclude that addressing the above-mentioned issues and contributing to proposing effective and efficient techniques could lead to “Future Generation DL” modeling as well as more intelligent and automated applications.
Concluding Remarks
In this article, we have presented a structured and comprehensive view of deep learning technology, which is considered a core part of artificial intelligence as well as data science. It starts with a history of artificial neural networks and moves to recent deep learning techniques and breakthroughs in different applications. Then, the key algorithms in this area, as well as deep neural network modeling in various dimensions are explored. For this, we have also presented a taxonomy considering the variations of deep learning tasks and how they are used for different purposes. In our comprehensive study, we have taken into account not only the deep networks for supervised or discriminative learning but also the deep networks for unsupervised or generative learning, and hybrid learning that can be used to solve a variety of real-world issues according to the nature of problems.
Deep learning, unlike traditional machine learning and data mining algorithms, can produce extremely high-level data representations from enormous amounts of raw data. As a result, it has provided an excellent solution to a variety of real-world problems. A successful deep learning technique must possess the relevant data-driven modeling depending on the characteristics of raw data. The sophisticated learning algorithms then need to be trained through the collected data and knowledge related to the target application before the system can assist with intelligent decision-making. Deep learning has shown to be useful in a wide range of applications and research areas such as healthcare, sentiment analysis, visual recognition, business intelligence, cybersecurity, and many more that are summarized in the paper.
Finally, we have summarized and discussed the challenges faced and the potential research directions, and future aspects in the area. Although deep learning is considered a black-box solution for many applications due to its poor reasoning and interpretability, addressing the challenges or future aspects that are identified could lead to future generation deep learning modeling and smarter systems. This can also help the researchers for in-depth analysis to produce more reliable and realistic outcomes. Overall, we believe that our study on neural networks and deep learning-based advanced analytics points in a promising path and can be utilized as a reference guide for future research and implementations in relevant application domains by both academic and industry professionals.
Abadi M, Barham P, Chen J, Chen Z, Davis A, Dean J, Devin Ma, Ghemawat S, Irving G, Isard M, et al. Tensorflow: a system for large-scale machine learning. In: 12th { USENIX } Symposium on operating systems design and implementation ({ OSDI } 16), 2016; p. 265–283.
Abdel-Basset M, Hawash H, Chakrabortty RK, Ryan M. Energy-net: a deep learning approach for smart energy management in iot-based smart cities. IEEE Internet of Things J. 2021.
Aggarwal A, Mittal M, Battineni G. Generative adversarial network: an overview of theory and applications. Int J Inf Manag Data Insights. 2021; p. 100004.
Al-Qatf M, Lasheng Y, Al-Habib M, Al-Sabahi K. Deep learning approach combining sparse autoencoder with svm for network intrusion detection. IEEE Access. 2018;6:52843–56.
Article Google Scholar
Ale L, Sheta A, Li L, Wang Y, Zhang N. Deep learning based plant disease detection for smart agriculture. In: 2019 IEEE Globecom Workshops (GC Wkshps), 2019; p. 1–6. IEEE.
Amarbayasgalan T, Lee JY, Kim KR, Ryu KH. Deep autoencoder based neural networks for coronary heart disease risk prediction. In: Heterogeneous data management, polystores, and analytics for healthcare. Springer; 2019. p. 237–48.
Anuradha J, et al. Big data based stock trend prediction using deep cnn with reinforcement-lstm model. Int J Syst Assur Eng Manag. 2021; p. 1–11.
Aqib M, Mehmood R, Albeshri A, Alzahrani A. Disaster management in smart cities by forecasting traffic plan using deep learning and gpus. In: International Conference on smart cities, infrastructure, technologies and applications. Springer; 2017. p. 139–54.
Arulkumaran K, Deisenroth MP, Brundage M, Bharath AA. Deep reinforcement learning: a brief survey. IEEE Signal Process Mag. 2017;34(6):26–38.
Aslan MF, Unlersen MF, Sabanci K, Durdu A. Cnn-based transfer learning-bilstm network: a novel approach for covid-19 infection detection. Appl Soft Comput. 2021;98:106912.
Bu F, Wang X. A smart agriculture iot system based on deep reinforcement learning. Futur Gener Comput Syst. 2019;99:500–7.
Chang W-J, Chen L-B, Hsu C-H, Lin C-P, Yang T-C. A deep learning-based intelligent medicine recognition system for chronic patients. IEEE Access. 2019;7:44441–58.
Chaudhari S, Mithal V, Polatkan Gu, Ramanath R. An attentive survey of attention models. arXiv preprint arXiv:1904.02874, 2019.
Chaudhuri N, Gupta G, Vamsi V, Bose I. On the platform but will they buy? predicting customers’ purchase behavior using deep learning. Decis Support Syst. 2021; p. 113622.
Chen D, Wawrzynski P, Lv Z. Cyber security in smart cities: a review of deep learning-based applications and case studies. Sustain Cities Soc. 2020; p. 102655.
Cho K, Van MB, Gulcehre C, Bahdanau D, Bougares F, Schwenk H, Bengio Y. Learning phrase representations using rnn encoder-decoder for statistical machine translation. arXiv preprint arXiv:1406.1078, 2014.
Chollet F. Xception: Deep learning with depthwise separable convolutions. In: Proceedings of the IEEE Conference on computer vision and pattern recognition, 2017; p. 1251–258.
Chung J, Gulcehre C, Cho KH, Bengio Y. Empirical evaluation of gated recurrent neural networks on sequence modeling. arXiv preprint arXiv:1412.3555, 2014.
Coelho IM, Coelho VN, da Eduardo J, Luz S, Ochi LS, Guimarães FG, Rios E. A gpu deep learning metaheuristic based model for time series forecasting. Appl Energy. 2017;201:412–8.
Da'u A, Salim N. Recommendation system based on deep learning methods: a systematic review and new directions. Artif Intel Rev. 2020;53(4):2709–48.
Deng L. A tutorial survey of architectures, algorithms, and applications for deep learning. APSIPA Trans Signal Inf Process. 2014; p. 3.
Deng L, Dong Yu. Deep learning: methods and applications. Found Trends Signal Process. 2014;7(3–4):197–387.
Article MathSciNet MATH Google Scholar
Deng S, Li R, Jin Y, He H. Cnn-based feature cross and classifier for loan default prediction. In: 2020 International Conference on image, video processing and artificial intelligence, volume 11584, page 115841K. International Society for Optics and Photonics, 2020.
Dhyani M, Kumar R. An intelligent chatbot using deep learning with bidirectional rnn and attention model. Mater Today Proc. 2021;34:817–24.
Donahue J, Krähenbühl P, Darrell T. Adversarial feature learning. arXiv preprint arXiv:1605.09782, 2016.
Du K-L, Swamy MNS. Neural networks and statistical learning. Berlin: Springer Science & Business Media; 2013.
MATH Google Scholar
Dupond S. A thorough review on the current advance of neural network structures. Annu Rev Control. 2019;14:200–30.
Google Scholar
Feng J, Yao Y, Lu S, Liu Y. Domain knowledge-based deep-broad learning framework for fault diagnosis. IEEE Trans Ind Electron. 2020;68(4):3454–64.
Garg S, Kaur K, Kumar N, Rodrigues JJPC. Hybrid deep-learning-based anomaly detection scheme for suspicious flow detection in sdn: a social multimedia perspective. IEEE Trans Multimed. 2019;21(3):566–78.
Géron A. Hands-on machine learning with Scikit-Learn, Keras. In: and TensorFlow: concepts, tools, and techniques to build intelligent systems. O’Reilly Media; 2019.
Goodfellow I, Bengio Y, Courville A, Bengio Y. Deep learning, vol. 1. Cambridge: MIT Press; 2016.
Goodfellow I, Pouget-Abadie J, Mirza M, Xu B, Warde-Farley D, Ozair S, Courville A, Bengio Y. Generative adversarial nets. In: Advances in neural information processing systems. 2014; p. 2672–680.
Google trends. 2021. https://trends.google.com/trends/ .
Gruber N, Jockisch A. Are gru cells more specific and lstm cells more sensitive in motive classification of text? Front Artif Intell. 2020;3:40.
Gu B, Ge R, Chen Y, Luo L, Coatrieux G. Automatic and robust object detection in x-ray baggage inspection using deep convolutional neural networks. IEEE Trans Ind Electron. 2020.
Han J, Pei J, Kamber M. Data mining: concepts and techniques. Amsterdam: Elsevier; 2011.
Haykin S. Neural networks and learning machines, 3/E. London: Pearson Education; 2010.
He K, Zhang X, Ren S, Sun J. Spatial pyramid pooling in deep convolutional networks for visual recognition. IEEE Trans Pattern Anal Mach Intell. 2015;37(9):1904–16.
He K, Zhang X, Ren S, Sun J. Deep residual learning for image recognition. In: Proceedings of the IEEE Conference on computer vision and pattern recognition, 2016; p. 770–78.
Hinton GE. Deep belief networks. Scholarpedia. 2009;4(5):5947.
Hinton GE, Osindero S, Teh Y-W. A fast learning algorithm for deep belief nets. Neural Comput. 2006;18(7):1527–54.
Hochreiter S, Schmidhuber J. Long short-term memory. Neural Comput. 1997;9(8):1735–80.
Huang C-J, Kuo P-H. A deep cnn-lstm model for particulate matter (pm2. 5) forecasting in smart cities. Sensors. 2018;18(7):2220.
Huang H-H, Fukuda M, Nishida T. Toward rnn based micro non-verbal behavior generation for virtual listener agents. In: International Conference on human-computer interaction, 2019; p. 53–63. Springer.
Hulsebos M, Hu K, Bakker M, Zgraggen E, Satyanarayan A, Kraska T, Demiralp Ça, Hidalgo C. Sherlock: a deep learning approach to semantic data type detection. In: Proceedings of the 25th ACM SIGKDD International Conference on knowledge discovery & data mining, 2019; p. 1500–508.
Imamverdiyev Y, Abdullayeva F. Deep learning method for denial of service attack detection based on restricted Boltzmann machine. Big Data. 2018;6(2):159–69.
Islam MZ, Islam MM, Asraf A. A combined deep cnn-lstm network for the detection of novel coronavirus (covid-19) using x-ray images. Inf Med Unlock. 2020;20:100412.
Ismail WN, Hassan MM, Alsalamah HA, Fortino G. Cnn-based health model for regular health factors analysis in internet-of-medical things environment. IEEE. Access. 2020;8:52541–9.
Jangid H, Singhal S, Shah RR, Zimmermann R. Aspect-based financial sentiment analysis using deep learning. In: Companion Proceedings of the The Web Conference 2018, 2018; p. 1961–966.
Kaelbling LP, Littman ML, Moore AW. Reinforcement learning: a survey. J Artif Intell Res. 1996;4:237–85.
Kameoka H, Li L, Inoue S, Makino S. Supervised determined source separation with multichannel variational autoencoder. Neural Comput. 2019;31(9):1891–914.
Karhunen J, Raiko T, Cho KH. Unsupervised deep learning: a short review. In: Advances in independent component analysis and learning machines. 2015; p. 125–42.
Kawde P, Verma GK. Deep belief network based affect recognition from physiological signals. In: 2017 4th IEEE Uttar Pradesh Section International Conference on electrical, computer and electronics (UPCON), 2017; p. 587–92. IEEE.
Kim J-Y, Seok-Jun B, Cho S-B. Zero-day malware detection using transferred generative adversarial networks based on deep autoencoders. Inf Sci. 2018;460:83–102.
Kingma DP, Welling M. Auto-encoding variational bayes. arXiv preprint arXiv:1312.6114, 2013.
Kingma DP, Welling M. An introduction to variational autoencoders. arXiv preprint arXiv:1906.02691, 2019.
Kiran PKR, Bhasker B. Dnnrec: a novel deep learning based hybrid recommender system. Expert Syst Appl. 2020.
Kloenne M, Niehaus S, Lampe L, Merola A, Reinelt J, Roeder I, Scherf N. Domain-specific cues improve robustness of deep learning-based segmentation of ct volumes. Sci Rep. 2020;10(1):1–9.
Kohonen T. The self-organizing map. Proc IEEE. 1990;78(9):1464–80.
Kohonen T. Essentials of the self-organizing map. Neural Netw. 2013;37:52–65.
Kök İ, Şimşek MU, Özdemir S. A deep learning model for air quality prediction in smart cities. In: 2017 IEEE International Conference on Big Data (Big Data), 2017; p. 1983–990. IEEE.
Krizhevsky A, Sutskever I, Hinton GE. Imagenet classification with deep convolutional neural networks. In: Advances in neural information processing systems. 2012; p. 1097–105.
Latif S, Rana R, Younis S, Qadir J, Epps J. Transfer learning for improving speech emotion classification accuracy. arXiv preprint arXiv:1801.06353, 2018.
LeCun Y, Bengio Y, Hinton G. Deep learning. Nature. 2015;521(7553):436–44.
LeCun Y, Bottou L, Bengio Y, Haffner P. Gradient-based learning applied to document recognition. Proc IEEE. 1998;86(11):2278–324.
Li B, François-Lavet V, Doan T, Pineau J. Domain adversarial reinforcement learning. arXiv preprint arXiv:2102.07097, 2021.
Li T-HS, Kuo P-H, Tsai T-N, Luan P-C. Cnn and lstm based facial expression analysis model for a humanoid robot. IEEE Access. 2019;7:93998–4011.
Liu C, Cao Y, Luo Y, Chen G, Vokkarane V, Yunsheng M, Chen S, Hou P. A new deep learning-based food recognition system for dietary assessment on an edge computing service infrastructure. IEEE Trans Serv Comput. 2017;11(2):249–61.
Liu W, Wang Z, Liu X, Zeng N, Liu Y, Alsaadi FE. A survey of deep neural network architectures and their applications. Neurocomputing. 2017;234:11–26.
López AU, Mateo F, Navío-Marco J, Martínez-Martínez JM, Gómez-Sanchís J, Vila-Francés J, Serrano-López AJ. Analysis of computer user behavior, security incidents and fraud using self-organizing maps. Comput Secur. 2019;83:38–51.
Lopez-Martin M, Carro B, Sanchez-Esguevillas A. Application of deep reinforcement learning to intrusion detection for supervised problems. Expert Syst Appl. 2020;141:112963.
Ma X, Yao T, Menglan H, Dong Y, Liu W, Wang F, Liu J. A survey on deep learning empowered iot applications. IEEE Access. 2019;7:181721–32.
Makhzani A, Frey B. K-sparse autoencoders. arXiv preprint arXiv:1312.5663, 2013.
Mandic D, Chambers J. Recurrent neural networks for prediction: learning algorithms, architectures and stability. Hoboken: Wiley; 2001.
Book Google Scholar
Marlin B, Swersky K, Chen B, Freitas N. Inductive principles for restricted boltzmann machine learning. In: Proceedings of the Thirteenth International Conference on artificial intelligence and statistics, p. 509–16. JMLR Workshop and Conference Proceedings, 2010.
Masud M, Muhammad G, Alhumyani H, Alshamrani SS, Cheikhrouhou O, Ibrahim S, Hossain MS. Deep learning-based intelligent face recognition in iot-cloud environment. Comput Commun. 2020;152:215–22.
Memisevic R, Hinton GE. Learning to represent spatial transformations with factored higher-order boltzmann machines. Neural Comput. 2010;22(6):1473–92.
Article MATH Google Scholar
Minaee S, Azimi E, Abdolrashidi AA. Deep-sentiment: sentiment analysis using ensemble of cnn and bi-lstm models. arXiv preprint arXiv:1904.04206, 2019.
Naeem M, Paragliola G, Coronato A. A reinforcement learning and deep learning based intelligent system for the support of impaired patients in home treatment. Expert Syst Appl. 2021;168:114285.
Niu Z, Zhong G, Hui Yu. A review on the attention mechanism of deep learning. Neurocomputing. 2021;452:48–62.
Pan SJ, Yang Q. A survey on transfer learning. IEEE Trans Knowl Data Eng. 2009;22(10):1345–59.
Paszke A, Gross S, Massa F, Lerer A, Bradbury J, Chanan G, Killeen T, Lin Z, Gimelshein N, Antiga L, et al. Pytorch: An imperative style, high-performance deep learning library. Adv Neural Inf Process Syst. 2019;32:8026–37.
Pedregosa F, Varoquaux G, Gramfort A, Michel V, Thirion B, Grisel O, Blondel M, Prettenhofer P, Weiss R, Dubourg V, et al. Scikit-learn: machine learning in python. J Mach Learn Res. 2011;12:2825–30.
MathSciNet MATH Google Scholar
Pi Y, Nath ND, Behzadan AH. Convolutional neural networks for object detection in aerial imagery for disaster response and recovery. Adv Eng Inf. 2020;43:101009.
Piccialli F, Giampaolo F, Prezioso E, Crisci D, Cuomo S. Predictive analytics for smart parking: A deep learning approach in forecasting of iot data. ACM Trans Internet Technol (TOIT). 2021;21(3):1–21.
Puterman ML. Markov decision processes: discrete stochastic dynamic programming. Hoboken: Wiley; 2014.
Qu X, Lin Y, Kai G, Linru M, Meng S, Mingxing K, Mu L, editors. A survey on the development of self-organizing maps for unsupervised intrusion detection. Mob Netw Appl. 2019; p. 1–22.
Rahman MW, Tashfia SS, Islam R, Hasan MM, Sultan SI, Mia S, Rahman MM. The architectural design of smart blind assistant using iot with deep learning paradigm. Internet of Things. 2021;13:100344.
Ren J, Green M, Huang X. From traditional to deep learning: fault diagnosis for autonomous vehicles. In: Learning control. Elsevier. 2021; p. 205–19.
Rifai S, Vincent P, Muller X, Glorot X, Bengio Y. Contractive auto-encoders: Explicit invariance during feature extraction. In: Icml, 2011.
Rosa RL, Schwartz GM, Ruggiero WV, Rodríguez DZ. A knowledge-based recommendation system that includes sentiment analysis and deep learning. IEEE Trans Ind Inf. 2018;15(4):2124–35.
Sarker IH. Context-aware rule learning from smartphone data: survey, challenges and future directions. J Big Data. 2019;6(1):1–25.
Article MathSciNet Google Scholar
Sarker IH. A machine learning based robust prediction model for real-life mobile phone data. Internet of Things. 2019;5:180–93.
Sarker IH. Cyberlearning: effectiveness analysis of machine learning security modeling to detect cyber-anomalies and multi-attacks. Internet of Things. 2021;14:100393.
Sarker IH. Data science and analytics: an overview from data-driven smart computing, decision-making and applications perspective. SN Comput Sci. 2021.
Sarker IH. Deep cybersecurity: a comprehensive overview from neural network and deep learning perspective. SN Computer. Science. 2021;2(3):1–16.
MathSciNet Google Scholar
Sarker IH. Machine learning: Algorithms, real-world applications and research directions. SN Computer. Science. 2021;2(3):1–21.
Sarker IH, Abushark YB, Alsolami F, Khan AI. Intrudtree: a machine learning based cyber security intrusion detection model. Symmetry. 2020;12(5):754.
Sarker IH, Abushark YB, Khan AI. Contextpca: Predicting context-aware smartphone apps usage based on machine learning techniques. Symmetry. 2020;12(4):499.
Sarker IH, Colman A, Han J. Recencyminer: mining recency-based personalized behavior from contextual smartphone data. J Big Data. 2019;6(1):1–21.
Sarker IH, Colman A, Han J, Khan AI, Abushark YB, Salah K. Behavdt: a behavioral decision tree learning to build user-centric context-aware predictive model. Mob Netw Appl. 2020;25(3):1151–61.
Sarker IH, Colman A, Kabir MA, Han J. Individualized time-series segmentation for mining mobile phone user behavior. Comput J. 2018;61(3):349–68.
Sarker IH, Furhad MH, Nowrozy R. Ai-driven cybersecurity: an overview, security intelligence modeling and research directions. SN Computer. Science. 2021;2(3):1–18.
Sarker IH, Hoque MM, Uddin MK. Mobile data science and intelligent apps: concepts, ai-based modeling and research directions. Mob Netw Appl. 2021;26(1):285–303.
Sarker IH, Kayes ASM. Abc-ruleminer: User behavioral rule-based machine learning method for context-aware intelligent services. J Netw Comput Appl. 2020;168:102762.
Sarker IH, Kayes ASM, Badsha S, Alqahtani H, Watters P, Ng A. Cybersecurity data science: an overview from machine learning perspective. J Big data. 2020;7(1):1–29.
Sarker IH, Kayes ASM, Watters P. Effectiveness analysis of machine learning classification models for predicting personalized context-aware smartphone usage. J Big Data. 2019;6(1):1–28.
Sarker IH, Salah K. Appspred: predicting context-aware smartphone apps using random forest learning. Internet of Things. 2019;8:100106.
Satt A, Rozenberg S, Hoory R. Efficient emotion recognition from speech using deep learning on spectrograms. In: Interspeec, 2017; p. 1089–1093.
Sevakula RK, Singh V, Verma NK, Kumar C, Cui Y. Transfer learning for molecular cancer classification using deep neural networks. IEEE/ACM Trans Comput Biol Bioinf. 2018;16(6):2089–100.
Sujay Narumanchi H, Ananya Pramod Kompalli Shankar A, Devashish CK. Deep learning based large scale visual recommendation and search for e-commerce. arXiv preprint arXiv:1703.02344, 2017.
Shao X, Kim CS. Multi-step short-term power consumption forecasting using multi-channel lstm with time location considering customer behavior. IEEE Access. 2020;8:125263–73.
Siami-Namini S, Tavakoli N, Namin AS. The performance of lstm and bilstm in forecasting time series. In: 2019 IEEE International Conference on Big Data (Big Data), 2019; p. 3285–292. IEEE.
Ślusarczyk B. Industry 4.0: are we ready? Pol J Manag Stud. 2018; p. 17
Sumathi P, Subramanian R, Karthikeyan VV, Karthik S. Soil monitoring and evaluation system using edl-asqe: enhanced deep learning model for ioi smart agriculture network. Int J Commun Syst. 2021; p. e4859.
Szegedy C, Liu W, Jia Y, Sermanet P, Reed S, Anguelov D, Erhan D, Vanhoucke V, Rabinovich A. Going deeper with convolutions. In: Proceedings of the IEEE Conference on computer vision and pattern recognition, 2015; p. 1–9.
Tan C, Sun F, Kong T, Zhang W, Yang C, Liu C. A survey on deep transfer learning. In: International Conference on artificial neural networks, 2018; p. 270–279. Springer.
Vesanto J, Alhoniemi E. Clustering of the self-organizing map. IEEE Trans Neural Netw. 2000;11(3):586–600.
Vincent P, Larochelle H, Lajoie I, Bengio Y, Manzagol P-A, Bottou L. Stacked denoising autoencoders: Learning useful representations in a deep network with a local denoising criterion. J Mach Learn Res. 2010;11(12).
Wang J, Liang-Chih Yu, Robert Lai K, Zhang X. Tree-structured regional cnn-lstm model for dimensional sentiment analysis. IEEE/ACM Trans Audio Speech Lang Process. 2019;28:581–91.
Wang S, Wan J, Li D, Liu C. Knowledge reasoning with semantic data for real-time data processing in smart factory. Sensors. 2018;18(2):471.
Wang W, Zhao M, Wang J. Effective android malware detection with a hybrid model based on deep autoencoder and convolutional neural network. J Ambient Intell Humaniz Comput. 2019;10(8):3035–43.
Wang X, Liu J, Qiu T, Chaoxu M, Chen C, Zhou P. A real-time collision prediction mechanism with deep learning for intelligent transportation system. IEEE Trans Veh Technol. 2020;69(9):9497–508.
Wang Y, Huang M, Zhu X, Zhao L. Attention-based lstm for aspect-level sentiment classification. In: Proceedings of the 2016 Conference on empirical methods in natural language processing, 2016; p. 606–615.
Wei P, Li Y, Zhang Z, Tao H, Li Z, Liu D. An optimization method for intrusion detection classification model based on deep belief network. IEEE Access. 2019;7:87593–605.
Weiss K, Khoshgoftaar TM, Wang DD. A survey of transfer learning. J Big data. 2016;3(1):9.
Xin Y, Kong L, Liu Z, Chen Y, Li Y, Zhu H, Gao M, Hou H, Wang C. Machine learning and deep learning methods for cybersecurity. Ieee access. 2018;6:35365–81.
Xu W, Sun H, Deng C, Tan Y. Variational autoencoder for semi-supervised text classification. In: Thirty-First AAAI Conference on artificial intelligence, 2017.
Xue Q, Chuah MC. New attacks on rnn based healthcare learning system and their detections. Smart Health. 2018;9:144–57.
Yousefi-Azar M, Hamey L. Text summarization using unsupervised deep learning. Expert Syst Appl. 2017;68:93–105.
Yuan X, Shi J, Gu L. A review of deep learning methods for semantic segmentation of remote sensing imagery. Expert Syst Appl. 2020;p. 114417.
Zhang G, Liu Y, Jin X. A survey of autoencoder-based recommender systems. Front Comput Sci. 2020;14(2):430–50.
Zhang X, Yao L, Huang C, Wang S, Tan M, Long Gu, Wang C. Multi-modality sensor data classification with selective attention. arXiv preprint arXiv:1804.05493, 2018.
Zhang X, Yao L, Wang X, Monaghan J, Mcalpine D, Zhang Y. A survey on deep learning based brain computer interface: recent advances and new frontiers. arXiv preprint arXiv:1905.04149, 2019; p. 66.
Zhang Y, Zhang P, Yan Y. Attention-based lstm with multi-task learning for distant speech recognition. In: Interspeech, 2017; p. 3857–861.
Download references
Author information
Authors and affiliations.
Swinburne University of Technology, Melbourne, VIC, 3122, Australia
Iqbal H. Sarker
Chittagong University of Engineering & Technology, Chittagong, 4349, Bangladesh
You can also search for this author in PubMed Google Scholar
Corresponding author
Correspondence to Iqbal H. Sarker .
Ethics declarations
Conflict of interest.
The author declares no conflict of interest.
Additional information
Publisher's note.
Springer Nature remains neutral with regard to jurisdictional claims in published maps and institutional affiliations.
This article is part of the topical collection “Advances in Computational Approaches for Artificial Intelligence, Image Processing, IoT and Cloud Applications” guest edited by Bhanu Prakash K. N. and M. Shivakumar.
Rights and permissions
Reprints and permissions
About this article
Sarker, I.H. Deep Learning: A Comprehensive Overview on Techniques, Taxonomy, Applications and Research Directions. SN COMPUT. SCI. 2 , 420 (2021). https://doi.org/10.1007/s42979-021-00815-1
Download citation
Received : 29 May 2021
Accepted : 07 August 2021
Published : 18 August 2021
DOI : https://doi.org/10.1007/s42979-021-00815-1
Share this article
Anyone you share the following link with will be able to read this content:
Sorry, a shareable link is not currently available for this article.
Provided by the Springer Nature SharedIt content-sharing initiative
- Deep learning
- Artificial neural network
- Artificial intelligence
- Discriminative learning
- Generative learning
- Hybrid learning
- Intelligent systems
- Find a journal
- Publish with us
- Track your research
- Latest News
.png)
- Cryptocurrencies
- White Papers
Top 10 Research and Thesis Topics for ML Projects in 2022
This article features the top 10 research and thesis topics for ml projects for students to try in 2022.
In this tech-driven world, selecting research and thesis topics in machine learning projects is the first choice of masters and Doctorate scholars. Selecting and working on a thesis topic in machine learning is not an easy task as machine learning uses statistical algorithms to make computers work in a certain way without being explicitly programmed. Achieving mastery over machine learning (ML) is becoming increasingly crucial for all the students in this field. Both artificial intelligence and machine learning complement each other. So, if you are a beginner, the best thing you can do is work on some ML projects. This article features the top 10 research and thesis topics for ML projects for students to try in 2022.
Text Mining and Text Classification
Text mining (also referred to as text analytics) is an artificial intelligence (AI) technology that uses natural language processing (NLP) to transform the free (unstructured) text in documents and databases into normalized, structured data suitable for analysis or to drive machine learning (ML) algorithms. Text classification tools categorize text by understanding its overall meaning, without predefined categories being explicitly present within the text. This is one of the best research and thesis topics for ML projects.
Image-Based Applications
An image-based test consists of a sequence of operations on UI elements in your tested application: clicks (for desktop and web applications), touches (for mobile applications), drag and drop operations, checkpoints, and so on. In image applications, one must first get familiar with masks, convolution, edge, and corner detection to be able to extract useful information from images and further use them for applications like image segmentation, keypoints extraction, and more.
Machine Vision
Using machine learning -based/mathematical techniques to enable machines to do specific tasks. For example, watermarking, face identification from datasets of images with rotation and different camera angles, criminals identification from surveillance cameras (video and series of images), handwriting and personal signature classification, object detection/recognition.
Clustering or cluster analysis is a machine learning technique, which groups the unlabeled dataset. It can be defined as "A way of grouping the data points into different clusters, consisting of similar data points. For example Graph clustering, data clustering, density-based clustering, and more. Clustering is one of the best research and thesis topics for ML projects.
Optimization
A) Population-based optimization inspired from a natural mechanism: Black-box optimization, multi/many-objective optimization, evolutionary methods (Genetic Algorithm, Genetic Programming, Memetic Programming), Metaheuristics (e.g., PSO, ABC, SA)
B) Exact/Mathematical Models: Convex optimization, Bi-Convex, and Semi-Convex optimization, Gradient Descent, Block Coordinate Descent, Manifold Optimization, and Algebraic Models
Voice Classification
Voice classification or sound classification can be referred to as the process of analyzing audio recordings. Voice and Speech Recognition, Signal Processing, Message Embedding, Message Extraction from Voice Encoded, and more are the best research and thesis topics for ML projects.
Sentiment Analysis
Sentiment analysis is one of the best Machine Learning projects well-known to uncover emotions in the text. By analyzing movie reviews, customer feedback, support tickets, companies may discover many interesting things. So learning how to build sentiment analysis models is quite a practical skill. There is no need to collect the data yourself. To train and test your model, use the biggest open-source database for sentiment analysis created by IMDb.
Recommendation Framework Project
This a rich dataset assortment containing a different scope of datasets accumulated from famous sites like Goodreads book audits, Amazon item surveys, online media, and so forth You will probably fabricate a recommendation engine (like the ones utilized by Amazon and Netflix) that can create customized recommendations for items, films, music, and so on, because of client inclinations, needs, and online conduct.
Mall Customers' Project
As the name suggests, the mall customers' dataset includes the records of people who visited the mall, such as gender, age, customer ID, annual income, spending score, etc. You will build a model that will use this data to segment the customers into different groups based on their behavior patterns. Such customer segmentation is a highly useful marketing tactic used by brands and marketers to boost sales and revenue while also increasing customer satisfaction.
Object Detection with Deep Learning
Object Detection with Deep Learning is one of the interesting machine learning projects to create. When it comes to image classification, Deep Neural Networks (DNNs) should be your go-to choice. While DNNs are already used in many real-world image classification applications, it is one of the best ML projects that aims to crank it up a notch. In this Machine Learning project, you will solve the problem of object detection by leveraging DNNs.
Disclaimer: Analytics Insight does not provide financial advice or guidance. Also note that the cryptocurrencies mentioned/listed on the website could potentially be scams, i.e. designed to induce you to invest financial resources that may be lost forever and not be recoverable once investments are made. You are responsible for conducting your own research (DYOR) before making any investments. Read more here.
Related Stories

M.Tech/Ph.D Thesis Help in Chandigarh | Thesis Guidance in Chandigarh
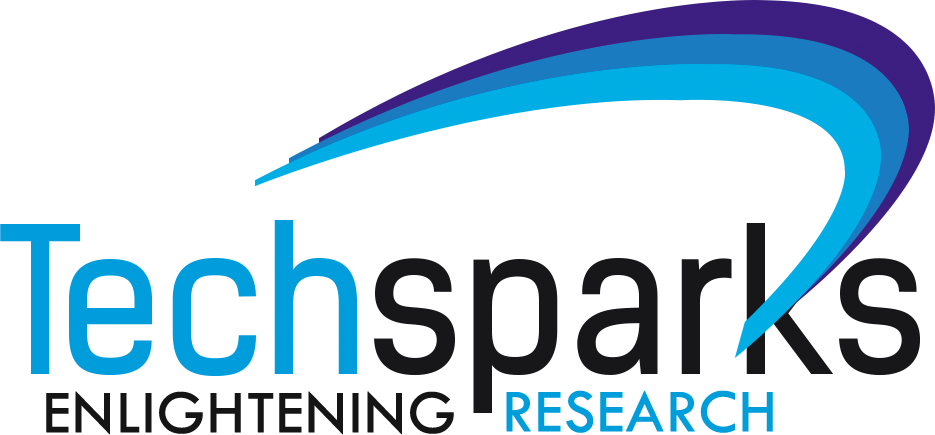

+91-9465330425

Latest thesis topics in Machine Learning for research scholars:
Choosing a research and thesis topics in Machine Learning is the first choice of masters and Doctorate scholars now a days. Though, choosing and working on a thesis topic in machine learning is not an easy task as Machine learning uses certain statistical algorithms to make computers work in a certain way without being explicitly programmed. The algorithms receive an input value and predict an output for this by the use of certain statistical methods. The main aim of machine learning is to create intelligent machines which can think and work like human beings. Achieving the above mentioned goals is surely not very easy because of which students who choose research topic in machine learning face difficult challenges and require professional thesis help in their thesis work.
Below is the list of the latest thesis topics in Machine learning for research scholars:
- The classification technique for the face spoof detection in artificial neural networks using concepts of machine learning .
- The iris detection and reorganization system using classification and glcm algorithm in machine learning.
- Using machine learning algorithms in the detection of pattern system using algorithm of textual feature analysis and classification
- The plant disease detection using glcm and KNN classification in neural networks merged with the concepts of machine learning
- Using the algorithms of machine learning to propose technique for the prediction analysis in data mining
- The sentiment analysis technique using SVM classifier in data mining using machine learning approach
- The heart disease prediction using technique of classification in machine learning using the concepts of data mining.
So let’s start with machine learning.
First of all…
What exactly is machine learning?
Find the link at the end to download the latest topics for thesis and research in Machine Learning
What is Machine Learning?
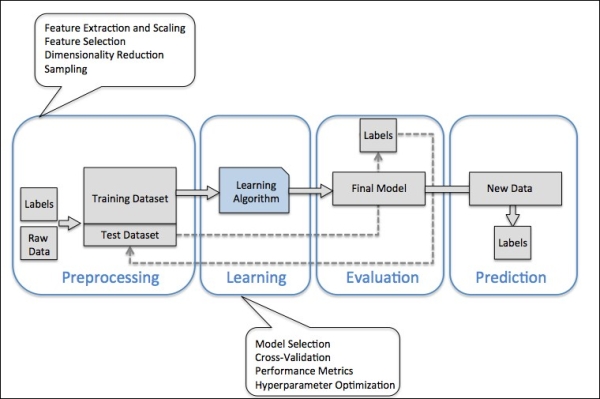
Machine Learning is a branch of artificial intelligence that gives systems the ability to learn automatically and improve themselves from the experience without being explicitly programmed or without the intervention of human. Its main aim is to make computers learn automatically from the experience.
Requirements of creating good machine learning systems
So what is required for creating such machine learning systems? Following are the things required in creating such machine learning systems:
Data – Input data is required for predicting the output.
Algorithms – Machine Learning is dependent on certain statistical algorithms to determine data patterns.
Automation – It is the ability to make systems operate automatically.
Iteration – The complete process is iterative i.e. repetition of process.
Scalability – The capacity of the machine can be increased or decreased in size and scale.
Modeling – The models are created according to the demand by the process of modeling.
Methods of Machine Learning
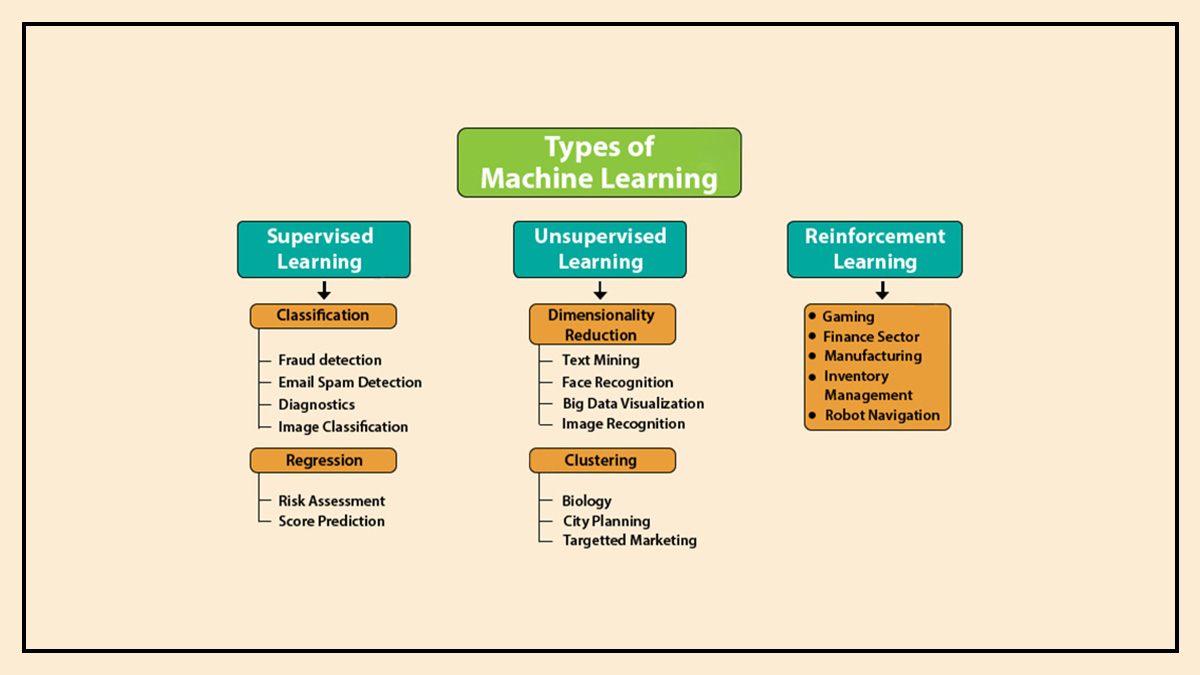
Machine Learning methods are classified into certain categories These are:
- Supervised Learning
- Unsupervised Learning
Reinforcement Learning
Supervised Learning – In this method, input and output is provided to the computer along with feedback during the training. The accuracy of predictions by the computer during training is also analyzed. The main goal of this training is to make computers learn how to map input to the output.
Unsupervised Learning – In this case, no such training is provided leaving computers to find the output on its own. Unsupervised learning is mostly applied on transactional data. It is used in more complex tasks. It uses another approach of iteration known as deep learning to arrive at some conclusions.
Reinforcement Learning – This type of learning uses three components namely – agent, environment, action. An agent is the one that perceives its surroundings, an environment is the one with which an agent interacts and acts in that environment. The main goal in reinforcement learning is to find the best possible policy.
How does machine learning work?
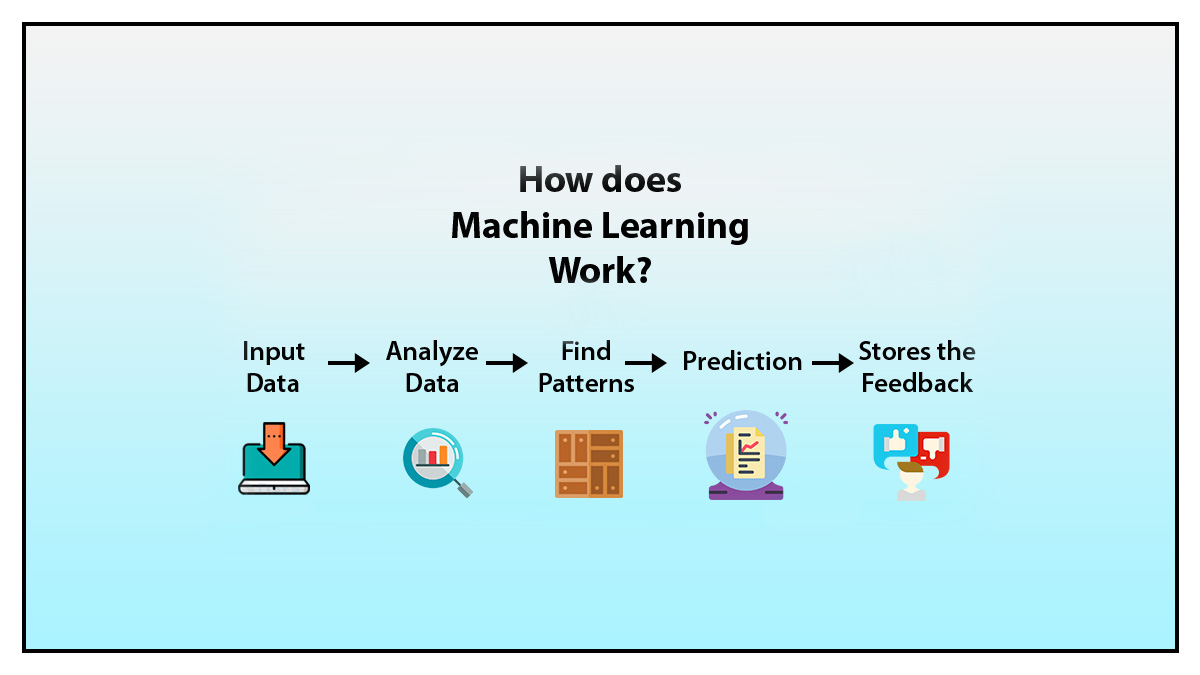
Machine learning makes use of processes similar to that of data mining. Machine learning algorithms are described in terms of target function(f) that maps input variable (x) to an output variable (y). This can be represented as:
There is also an error e which is the independent of the input variable x. Thus the more generalized form of the equation is:
In machine the mapping from x to y is done for predictions. This method is known as predictive modeling to make most accurate predictions. There are various assumptions for this function.
Benefits of Machine Learning
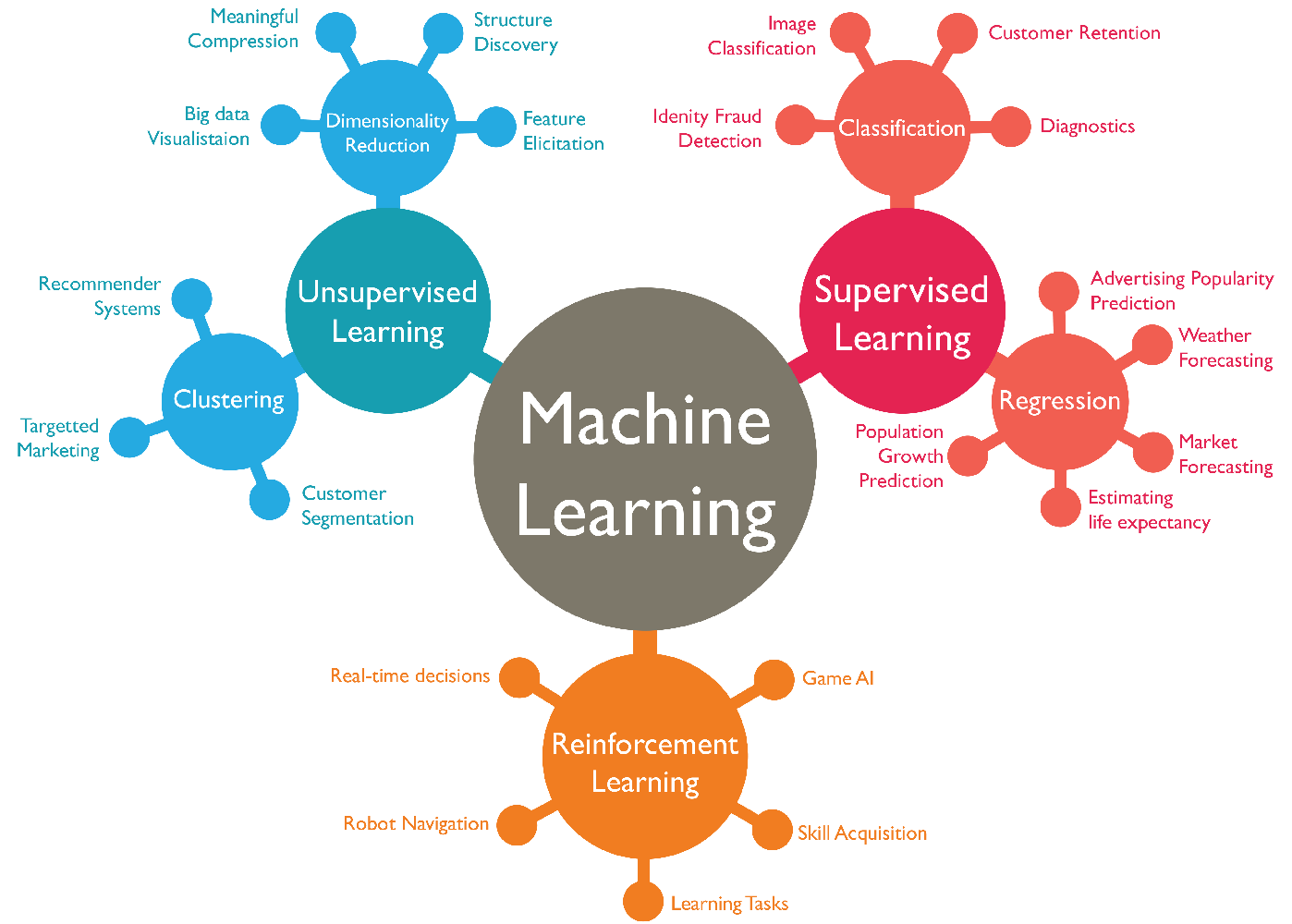
Everything is dependent on machine learning. Find out what are the benefits of machine learning.
Decision making is faster – Machine learning provides the best possible outcomes by prioritizing the routine decision-making processes.
Adaptability – Machine Learning provides the ability to adapt to new changing environment rapidly. The environment changes rapidly due to the fact that data is being constantly updated.
Innovation – Machine learning uses advanced algorithms that improve the overall decision-making capacity. This helps in developing innovative business services and models.
Insight – Machine learning helps in understanding unique data patterns and based on which specific actions can be taken.
Business growth – With machine learning overall business process and workflow will be faster and hence this would contribute to the overall business growth and acceleration.
Outcome will be good – With machine learning the quality of the outcome will be improved with lesser chances of error.
Branches of Machine Learning
- Computational Learning Theory
- Adversarial Machine Learning
- Quantum Machine Learning
- Robot Learning
- Meta-Learning
Computational Learning Theory – Computational learning theory is a subfield of machine learning for studying and analyzing the algorithms of machine learning. It is more or less similar to supervised learning.
Adversarial Machine Learning – Adversarial machine learning deals with the interaction of machine learning and computer security. The main aim of this technique is to look for safer methods in machine learning to prevent any form of spam and malware. It works on the following three principles:
Finding vulnerabilities in machine learning algorithms.
Devising strategies to check these potential vulnerabilities.
Implementing these preventive measures to improve the security of the algorithms.
Quantum Machine Learning – This area of machine learning deals with quantum physics. In this algorithm, the classical data set is translated into quantum computer for quantum information processing. It uses Grover’s search algorithm to solve unstructured search problems.
Predictive Analysis – Predictive Analysis uses statistical techniques from data modeling, machine learning and data mining to analyze current and historical data to predict the future. It extracts information from the given data. Customer relationship management(CRM) is the common application of predictive analysis.
Robot Learning – This area deals with the interaction of machine learning and robotics. It employs certain techniques to make robots to adapt to the surrounding environment through learning algorithms.
Grammar Induction – It is a process in machine learning to learn formal grammar from a given set of observations to identify characteristics of the observed model. Grammar induction can be done through genetic algorithms and greedy algorithms.
Meta-Learning – In this process learning algorithms are applied on meta-data and mainly deals with automatic learning algorithms.
Best Machine Learning Tools
Here is a list of artificial intelligence and machine learning tools for developers:
ai-one – It is a very good tool that provides software development kit for developers to implement artificial intelligence in an application.
Protege – It is a free and open-source framework and editor to build intelligent systems with the concept of ontology. It enables developers to create, upload and share applications.
IBM Watson – It is an open-API question answering system that answers questions asked in natural language. It has a collection of tools which can be used by developers and in business.
DiffBlue – It is another tool in artificial intelligence whose main objective is to locate bugs, errors and fix weaknesses in the code. All such things are done through automation.
TensorFlow – It is an open-source software library for machine learning. TensorFlow provides a library of numerical computations along with documentation, tutorials and other resources for support.
Amazon Web Services – Amazon has launched toolkits for developers along with applications which range from image interpretation to facial recognition.
OpenNN – It is an open-source, high-performance library for advanced analytics and is written in C++ programming language. It implements neural networks. It has a lot of tutorials and documentation along with an advanced tool known as Neural Designer.
Apache Spark – It is a framework for large-scale processing of data. It also provides a programming tool for deep learning on various machines.
Caffe – It is a framework for deep learning and is used in various industrial applications in the area of speech, vision and expression.
Veles – It is another deep learning platform written in C++ language and make use of python language for interaction between the nodes.
Machine Learning Applications
Following are some of the applications of machine learning:
Cognitive Services
Medical Services
Language Processing
Business Management
Image Recognition
Face Detection
Video Games
Computer Vision
Pattern Recognition
Machine Learning in Bioinformatics
Bioinformatics term is a combination of two terms bio, informatics. Bio means related to biology and informatics means information. Thus bioinformatics is a field that deals with processing and understanding of biological data using computational and statistical approach. Machine Learning has a number of applications in the area of bioinformatics. Machine Learning find its application in the following subfields of bioinformatics:
Genomics – Genomics is the study of DNA of organisms. Machine Learning systems can help in finding the location of protein-encoding genes in a DNA structure. Gene prediction is performed by using two types of searches named as extrinsic and intrinsic. Machine Learning is used in problems related to DNA alignment.
Proteomics – Proteomics is the study of proteins and amino acids. Proteomics is applied to problems related to proteins like protein side-chain prediction, protein modeling, and protein map prediction.
Microarrays – Microarrays are used to collect data about large biological materials. Machine learning can help in the data analysis, pattern prediction and genetic induction. It can also help in finding different types of cancer in genes.
System Biology – It deals with the interaction of biological components in the system. These components can be DNA, RNA, proteins and metabolites. Machine Learning help in modeling these interactions.
Text mining – Machine learning help in extraction of knowledge through natural language processing techniques.
Deep Learning
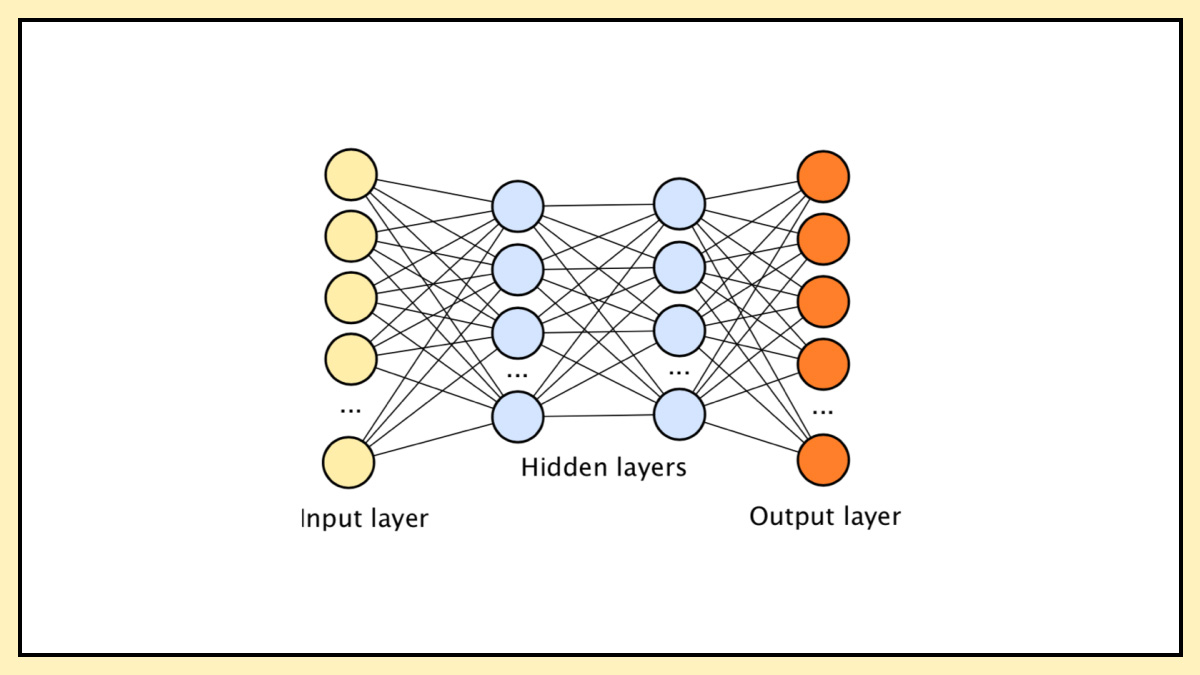
Deep Learning is a part of the broader field machine learning and is based on data representation learning. It is based on the interpretation of artificial neural network. Deep Learning algorithm uses many layers of processing. Each layer uses the output of previous layer as an input to itself. The algorithm used can be supervised algorithm or unsupervised algorithm. Deep Learning is mainly developed to handle complex mappings of input and output. It is another hot topic for M.Tech thesis and project along with machine learning.
Deep Neural Network
Deep Neural Network is a type of Artificial Neural Network with multiple layers which are hidden between the input layer and the output layer. This concept is known as feature hierarchy and it tends to increase the complexity and abstraction of data. This gives network the ability to handle very large, high-dimensional data sets having millions of parameters. The procedure of deep neural networks is as follows:
Consider some examples from a sample dataset.
Calculate error for this network.
Improve weight of the network to reduce the error.
Repeat the procedure.
Applications of Deep Learning
Here are some of the applications of Deep Learning:
Automatic Speech Recognition
Natural Language Processing
Customer Relationship Management
Bioinformatics
Mobile Advertising
Advantages of Deep Learning
Deep Learning helps in solving certain complex problems with high speed which were earlier left unsolved. Deep Learning is very useful in real world applications. Following are some of the main advantages of deep learning:
Eliminates unnecessary costs – Deep Learning helps to eliminate unnecessary costs by detecting defects and errors in the system.
Identifies defects which otherwise are difficult to detect – Deep Learning helps in identifying defects which left untraceable in the system.
Can inspect irregular shapes and patterns – Deep Learning can inspect irregular shapes and patterns which is difficult for machine learning to detect.
From this introduction, you must have known that why this topic is called as hot for your M.Tech thesis and projects. This was just the basic introduction to machine learning and deep learning. There is more to explore in these fields. You will get to know more once you start doing research on this topic for your M.Tech thesis. You can get thesis assistance and guidance on this topic from experts specialized in this field.
Research and Thesis Topics in Machine Learning
Here is the list of current research and thesis topics in Machine Learning :
Machine Learning Algorithms
Supervised Machine Learning
Unsupervised Machine Learning
Neural Networks
Predictive Learning
Bayesian Network
Data Mining
For starting with Machine Learning, you need to know some algorithms. Machine Learning algorithms are classified into three categories which provide the base for machine learning. These categories of algorithms are supervised learning, unsupervised learning, and reinforcement learning. The choice of algorithms depends upon the type of tasks you want to be done along with the type, quality, and nature of data present. The role of input data is crucial in machine learning algorithms.
Computer Vision is a field that deals with making systems that can read and interpret images. In simple terms, computer vision is a method of transmitting human intelligence and vision in machines. In computer vision, data is collected from images which are imparted to systems. The system will take action according to the information it interprets from what it sees.
It is a good topic for machine learning masters thesis. It is a type of machine learning algorithm in which makes predictions based on known data-sets. Input and output is provided to the system along with feedback. Supervised Learning is further classified into classification and regression problems. In the classification problem, the output is a category while in regression problem the output is a real value.
It is another category of machine learning algorithm in which input is known but the output is not known. Prior training is not provided to the system as in case of supervised learning. The main purpose of unsupervised learning is to model the underlying structure of data. Clustering and Association are the two types of unsupervised learning problems. k-means and Apriori algorithm are the examples of unsupervised learning algorithms.
Deep Learning is a hot topic in Machine Learning. It is already explained above. It is a part of the family of machine learning and deals with the functioning of the artificial neural network. Neural Networks are used to study the functioning of the human brain. It is one of the growing and exciting field. Deep learning has made it possible for the practical implementation of various machine learning applications.
Neural Networks are the systems to study the biological neural networks. It is an important application of machine learning and a good topic for masters thesis and research. The main purpose of Artificial Neural Network is to study how the human brain works. It finds its application in computer vision, speech recognition, machine translation etc. Artificial Neural Network is a collection of nodes which represent neurons.
Reinforcement Learning is a category of machine learning algorithms. Reinforcement Learning deals with software agents to study how these agents take actions in an environment in order to maximize their performance. Reinforcement Learning is different from supervised learning in the sense that correct input and output parameters are not provided.
Predictive Learning is another good topic for thesis in machine learning. In this technique, a model is built by an agent of its environment in which it performs actions. There is another field known as predictive analytics which is used to make predictions about future events which are unknown. For this, techniques like data mining, statistics, modeling, machine learning, and artificial intelligence are used.
It is a network that represents probabilistic relationships via Directed Acyclic Graph(DAG). There are algorithms in Bayesian Network for inference and learning. In the network, a probability function is there for each node which takes an input to give probability to the value associated with the node. Bayesian Network finds its application in bioinformatics, image processing, and computational biology.
Data Mining is the process of finding patterns from large data-sets to extract valuable information to make better decisions. It is a hot area of research. This technology use method from machine learning, statistics, and database systems for processing. There exist data mining techniques like clustering, association, decision trees, classification for the data mining process.
Click on the following link to download the latest thesis and research topics in Machine Learning
Latest Thesis and Research Topics on Machine Learning(pdf)
For more details Contact Us. You can call us on this number +91-9465330425 or drop an email at [email protected] for any type of dissertation help in India. You can also fill the query form on the website.
You can also visit our website Techsparks and follow us on Pinterest , Facebook , Twitter, YouTube and Linkedin for latest thesis blog.
Leave a Reply Cancel reply
Your email address will not be published. Required fields are marked *
Save my name, email, and website in this browser for the next time I comment.
Quick Enquiry
Get a quote, share your details to get free.
- ODSC EUROPE
- AI+ Training
- Speak at ODSC

- Data Analytics
- Data Engineering
- Data Visualization
- Deep Learning
- Generative AI
- Machine Learning
- NLP and LLMs
- Business & Use Cases
- Career Advice
- Write for us
- ODSC Community Slack Channel
- Upcoming Webinars

Best Deep Learning Research of 2021 So Far
Deep Learning Modeling Research posted by Daniel Gutierrez, ODSC August 2, 2021 Daniel Gutierrez, ODSC
The discipline of AI most often mentioned these days is deep learning (DL) along with its many incarnations implemented with deep neural networks. DL also is a rapidly accelerating area of research with papers being published at a fast clip by research teams from around the globe.
I enjoy keeping a pulse on deep learning research and so far in 2021 research innovations have propagated at a quick pace. Some of the top topical areas for deep learning research are: causality, explainability/interpretability, transformers, NLP, GPT, language models, GANs, deep learning for tabular data, and many others.
In this article, we’ll take a brief tour of my top picks for deep learning research (in no particular order) of papers that I found to be particularly compelling. I’m pretty attached to this leading-edge research. I’m known to carry a thick folder of recent research papers around in my backpack and consume all the great developments when I have a spare moment. Enjoy!
Check out my previous lists: Best Machine Learning Research of 2021 So Far , Best of Deep Reinforcement Learning Research of 2019 , Most Influential NLP Research of 2019 , and Most Influential Deep Learning Research of 2019 .
Cause and Effect: Concept-based Explanation of Neural Networks
In many scenarios, human decisions are explained based on some high-level concepts. This paper takes a step in the interpretability of neural networks by examining their internal representation or neuron’s activations against concepts. A concept is characterized by a set of samples that have specific features in common. A framework is proposed to check the existence of a causal relationship between a concept (or its negation) and task classes. While the previous methods focus on the importance of a concept to a task class, the paper goes further and introduces four measures to quantitatively determine the order of causality. Through experiments, the effectiveness of the proposed method is demonstrated in explaining the relationship between a concept and the predictive behavior of a neural network.
Pretrained Language Models for Text Generation: A Survey
Text generation has become one of the most important yet challenging tasks in natural language processing (NLP). The resurgence of deep learning has greatly advanced this field by neural generation models, especially the paradigm of pretrained language models (PLMs). This paper presents an overview of the major advances achieved in the topic of PLMs for text generation. As the preliminaries, the paper presents the general task definition and briefly describes the mainstream architectures of PLMs for text generation. As the core content, the deep learning research paper discusses how to adapt existing PLMs to model different input data and satisfy special properties in the generated text.
A Short Survey of Pre-trained Language Models for Conversational AI-A NewAge in NLP
Building a dialogue system that can communicate naturally with humans is a challenging yet interesting problem of agent-based computing. The rapid growth in this area is usually hindered by the long-standing problem of data scarcity as these systems are expected to learn syntax, grammar, decision making, and reasoning from insufficient amounts of task-specific data sets. The recently introduced pre-trained language models have the potential to address the issue of data scarcity and bring considerable advantages by generating contextualized word embeddings. These models are considered counterparts of ImageNet in NLP and have demonstrated the ability to capture different facets of language such as hierarchical relations, long-term dependency, and sentiment. This short survey paper discusses the recent progress made in the field of pre-trained language models.
TrustyAI Explainability Toolkit
AI is becoming increasingly more popular and can be found in workplaces and homes around the world. However, how do we ensure trust in these systems? Regulation changes such as the GDPR mean that users have a right to understand how their data has been processed as well as saved. Therefore if, for example, you are denied a loan you have the right to ask why. This can be hard if the method for working this out uses “black box” machine learning techniques such as neural networks. TrustyAI is a new initiative which looks into explainable artificial intelligence (XAI) solutions to address trustworthiness in ML as well as decision services landscapes. This deep learning research paper looks at how TrustyAI can support trust in decision services and predictive models. The paper investigates techniques such as LIME, SHAP and counterfactuals, benchmarking both LIME and counterfactual techniques against existing implementations.
Generative Adversarial Network: Some Analytical Perspectives
Ever since its debut, generative adversarial networks (GANs) have attracted tremendous amount of attention. Over the past years, different variations of GANs models have been developed and tailored to different applications in practice. Meanwhile, some issues regarding the performance and training of GANs have been noticed and investigated from various theoretical perspectives. This paper starts from an introduction of GANs from an analytical perspective, then moves onto the training of GANs via SDE approximations and finally discusses some applications of GANs in computing high dimensional MFGs as well as tackling mathematical finance problems.
PyTorch Tabular: A Framework for Deep Learning with Tabular Data
In spite of showing unreasonable effectiveness in modalities like Text and Image, deep learning has always lagged gradient boosting in tabular data – both in popularity and performance. But recently there have been newer models created specifically for tabular data, which is pushing the performance bar. But popularity is still a challenge because there is no easy, ready-to-use library like scikit-learn for deep learning. PyTorch Tabular is a new deep learning library which makes working with deep learning and tabular data easy and fast. It is a library built on top of PyTorch and PyTorch Lightning and works on Pandas dataframes directly. Many SOTA models like NODE and TabNet are already integrated and implemented in the library with a unified API. PyTorch Tabular is designed to be easily extensible for researchers, simple for practitioners, and robust in industrial deployments.
A Survey of Quantization Methods for Efficient Neural Network Inference
As soon as abstract mathematical computations were adapted to computation on digital computers, the problem of efficient representation, manipulation, and communication of the numerical values in those computations arose. Strongly related to the problem of numerical representation is the problem of quantization : in what manner should a set of continuous real-valued numbers be distributed over a fixed discrete set of numbers to minimize the number of bits required and also to maximize the accuracy of the attendant computations? This perennial problem of quantization is particularly relevant whenever memory and/or computational resources are severely restricted, and it has come to the forefront in recent years due to the remarkable performance of Neural Network models in computer vision, natural language processing, and related areas. Moving from floating-point representations to low-precision fixed integer values represented in four bits or less holds the potential to reduce the memory footprint and latency by a factor of 16x; and, in fact, reductions of 4x to 8x are often realized in practice in these applications. Thus, it is not surprising that quantization has emerged recently as an important and very active sub-area of research in the efficient implementation of computations associated with Neural Networks. This paper surveys approaches to the problem of quantizing the numerical values in deep Neural Network computations, covering the advantages/disadvantages of current methods.
How to decay your learning rate
Complex learning rate schedules have become an integral part of deep learning. This research finds empirically that common fine-tuned schedules decay the learning rate after the weight norm bounces. This leads to the proposal of ABEL : an automatic scheduler which decays the learning rate by keeping track of the weight norm. ABEL’s performance matches that of tuned schedules and is more robust with respect to its parameters. Through extensive experiments in vision, NLP, and RL, it is shown that if the weight norm does not bounce, it is possible to simplify schedules even further with no loss in performance. In such cases, a complex schedule has similar performance to a constant learning rate with a decay at the end of training.
GPT Understands, Too
While GPTs with traditional fine-tuning fail to achieve strong results on natural language understanding (NLU), this paper shows that GPTs can be better than or comparable to similar-sized BERTs on NLU tasks with a novel method P-tuning — which employs trainable continuous prompt embeddings. On the knowledge probing (LAMA) benchmark, the best GPT recovers 64% (P@1) of world knowledge without any additional text provided during test time, which substantially improves the previous best by 20+ percentage points. On the SuperGlue benchmark, GPTs achieve comparable and sometimes better performance to similar-sized BERTs in supervised learning. Importantly, it is found that P-tuning also improves BERTs’ performance in both few-shot and supervised settings while largely reducing the need for prompt engineering. Consequently, P-tuning outperforms the state-of-the-art approaches on the few-shot SuperGlue benchmark.
Understanding Robustness of Transformers for Image Classification
Deep Convolutional Neural Networks (CNNs) have long been the architecture of choice for computer vision tasks. Recently, Transformer-based architectures like Vision Transformer (ViT) have matched or even surpassed ResNets for image classification. However, details of the Transformer architecture — such as the use of non-overlapping patches — lead one to wonder whether these networks are as robust. This paper performs an extensive study of a variety of different measures of robustness of ViT models and compare the findings to ResNet baselines. Investigated is robustness to input perturbations as well as robustness to model perturbations. The paper finds that when pre-trained with a sufficient amount of data, ViT models are at least as robust as the ResNet counterparts on a broad range of perturbations. Also found is that Transformers are robust to the removal of almost any single layer, and that while activations from later layers are highly correlated with each other, they nevertheless play an important role in classification.
Improving DeepFake Detection Using Dynamic Face Augmentation
The creation of altered and manipulated faces has become more common due to the improvement of DeepFake generation methods. Simultaneously, we have seen the development of detection models for differentiating between a manipulated and original face from image or video content. We have observed that most publicly available DeepFake detection datasets have limited variations, where a single face is used in many videos, resulting in an oversampled training dataset. Due to this, deep neural networks tend to overfit to the facial features instead of learning to detect manipulation features of DeepFake content. As a result, most detection architectures perform poorly when tested on unseen data. This paper provides a quantitative analysis to investigate this problem and present a solution to prevent model overfitting due to the high volume of samples generated from a small number of actors.
An Evaluation of Edge TPU Accelerators for Convolutional Neural Networks
Edge TPUs are a domain of accelerators for low-power, edge devices and are widely used in various Google products such as Coral and Pixel devices. This paper first discusses the major microarchitectural details of Edge TPUs. This is followed by an extensive evaluation of three classes of Edge TPUs, covering different computing ecosystems that are either currently deployed in Google products or are the product pipeline. Building upon this extensive study, the paper discusses critical and interpretable microarchitectural insights about the studied classes of Edge TPUs. Mainly discussed is how Edge TPU accelerators perform across CNNs with different structures. Finally, the paper presents ongoing efforts in developing high-accuracy learned machine learning models to estimate the major performance metrics of accelerators such as latency and energy consumption. These learned models enable significantly faster (in the order of milliseconds) evaluations of accelerators as an alternative to time-consuming cycle-accurate simulators and establish an exciting opportunity for rapid hard-ware/software co-design.
Attention Models for Point Clouds in Deep Learning: A Survey
Recently, the advancement of 3D point clouds in deep learning has attracted intensive research in different application domains such as computer vision and robotic tasks. However, creating feature representation of robust, discriminative from unordered and irregular point clouds is challenging. The goal of this paper is to provide a comprehensive overview of the point clouds feature representation which uses attention models. More than 75+ key contributions in the recent three years are summarized in this survey, including the 3D objective detection, 3D semantic segmentation, 3D pose estimation, point clouds completion etc. Also provided are: a detailed characterization of (i) the role of attention mechanisms, (ii) the usability of attention models into different tasks, and (iii) the development trend of key technology.
Constrained Optimization for Training Deep Neural Networks Under Class Imbalance
Deep neural networks (DNNs) are notorious for making more mistakes for the classes that have substantially fewer samples than the others during training. Such class imbalance is ubiquitous in clinical applications and very crucial to handle because the classes with fewer samples most often correspond to critical cases (e.g., cancer) where misclassifications can have severe consequences. Not to miss such cases, binary classifiers need to be operated at high True Positive Rates (TPR) by setting a higher threshold but this comes at the cost of very high False Positive Rates (FPR) for problems with class imbalance. Existing methods for learning under class imbalance most often do not take this into account. This paper argues that prediction accuracy should be improved by emphasizing reducing FPRs at high TPRs for problems where misclassification of the positive samples are associated with higher cost. To this end, it’s posed the training of a DNN for binary classification as a constrained optimization problem and introduce a novel constraint that can be used with existing loss functions to enforce maximal area under the ROC curve (AUC). The resulting constrained optimization problem is solved using an Augmented Lagrangian method (ALM), where the constraint emphasizes reduction of FPR at high TPR. Results demonstrate that the proposed method almost always improves the loss functions it is used with by attaining lower FPR at high TPR and higher or equal AUC.
Deep Convolutional Neural Networks with Unitary Weights
While normalizations aim to fix the exploding and vanishing gradient problem in deep neural networks, they have drawbacks in speed or accuracy because of their dependency on the data set statistics. This paper is a comprehensive study of a novel method based on unitary synaptic weights derived from Lie Group to construct intrinsically stable neural systems. Here it’s shown that unitary convolutional neural networks deliver up to 32% faster inference speeds while maintaining competitive prediction accuracy. Unlike prior arts restricted to square synaptic weights, the paper expands the unitary networks to weights of any size and dimension.
TransGAN: Two Pure Transformers Can Make One Strong GAN, and That Can Scale Up
The recent explosive interest with transformers has suggested their potential to become powerful “universal” models for computer vision tasks, such as classification, detection, and segmentation. An important question is how much further transformers can go – are they ready to take some more notoriously difficult vision tasks, e.g., generative adversarial networks (GANs)? Driven by that curiosity, this paper conducts the first pilot study in building a GAN completely free of convolutions, using only pure transformer-based architectures. The proposed vanilla GAN architecture, dubbed TransGAN , consists of a memory-friendly transformer-based generator that progressively increases feature resolution while decreasing embedding dimension, and a patch-level discriminator that is also transformer-based. TransGAN is seen to notably benefit from data augmentations (more than standard GANs), a multi-task co-training strategy for the generator, and a locally initialized self-attention that emphasizes the neighborhood smoothness of natural images. Equipped with those findings, TransGAN can effectively scale up with bigger models and high-resolution image datasets. Specifically, the architecture achieves highly competitive performance compared to current state-of-the-art GANs based on convolutional backbones. The GitHub repo associated with this paper can be found HERE .

Deep Learning for Scene Classification: A Survey
Scene classification , aiming at classifying a scene image to one of the predefined scene categories by comprehending the entire image, is a longstanding, fundamental and challenging problem in computer vision. The rise of large-scale datasets, which constitute a dense sampling of diverse real-world scenes, and the renaissance of deep learning techniques, which learn powerful feature representations directly from big raw data, have been bringing remarkable progress in the field of scene representation and classification. To help researchers master needed advances in this field, the goal of this paper is to provide a comprehensive survey of recent achievements in scene classification using deep learning. More than 260 major publications are included in this survey covering different aspects of scene classification, including challenges, benchmark datasets, taxonomy, and quantitative performance comparisons of the reviewed methods. In retrospect of what has been achieved so far, this paper is concluded with a list of promising research opportunities.
Introducing and assessing the explainable AI (XAI) method: SIDU
Explainable Artificial Intelligence (XAI) has in recent years become a well-suited framework to generate human-understandable explanations of black box models. This paper presents a novel XAI visual explanation algorithm denoted SIDU that can effectively localize entire object regions responsible for prediction. The paper analyzes its robustness and effectiveness through various computational and human subject experiments. In particular, the SIDU algorithm is assessed using three different types of evaluations (Application, Human and Functionally-Grounded) to demonstrate its superior performance. The robustness of SIDU is further studied in presence of adversarial attack on black box models to better understand its performance.
Evolving Reinforcement Learning Algorithms
This paper proposes a method for meta-learning reinforcement learning algorithms by searching over the space of computational graphs which compute the loss function for a value-based model-free RL agent to optimize. The learned algorithms are domain-agnostic and can generalize to new environments not seen during training. The method can both learn from scratch and bootstrap off known existing algorithms, like DQN, enabling interpretable modifications which improve performance. Learning from scratch on simple classical control and gridworld tasks, the method rediscovers the temporal-difference (TD) algorithm. Bootstrapped from DQN, two learned algorithms are highlighted which obtain good generalization performance over other classical control tasks, gridworld type tasks, and Atari games. The analysis of the learned algorithm behavior shows resemblance to recently proposed RL algorithms that address overestimation in value-based methods.
RepVGG: Making VGG-style ConvNets Great Again
VGG-style ConvNets, although now considered a classic architecture, were attractive due to their simplicity. In contrast, ResNets have become popular due to their high accuracy but are more difficult to customize and display undesired inference drawbacks. To address these issues, Ding et al. propose RepVGG – the return of the VGG!
RepVGG is an efficient and simple architecture using plain VGG-style ConvNets. It decouples the inference-time and training-time architecture through a structural re-parameterization technique. The researchers report favorable speed-accuracy tradeoff compared to state-of-the-art models, such as EfficientNet and RegNet. RepVGG achieves 80% top-1 accuracy on ImageNet and is benchmarked as being 83% faster than ResNet-50. This research is part of a broader effort to build more efficient models using simpler architectures and operations. The GitHub repo associated with this paper can be found HERE .
Switch Transformers: Scaling to Trillion Parameter Models with Simple and Efficient Sparsity
In deep learning, models typically reuse the same parameters for all inputs. Mixture of Experts (MoE) defies this and instead selects different parameters for each incoming example. The result is a sparsely-activated model — with outrageous numbers of parameters — but a constant computational cost. However, despite several notable successes of MoE, widespread adoption has been hindered by complexity, communication costs and training instability — this paper addresses these with the Switch Transformer . The Google Brain researchers simplify the MoE routing algorithm and design intuitive improved models with reduced communication and computational costs. The proposed training techniques help wrangle the instabilities and it is shown that large sparse models may be trained, for the first time, with lower precision (bfloat16) formats. They design models based off T5-Base and T5-Large to obtain up to 7x increases in pre-training speed with the same computational resources. These improvements extend into multilingual settings to measure gains over the mT5-Base version across all 101 languages. Finally, the paper advances the current scale of language models by pre-training up to trillion parameter models on the “Colossal Clean Crawled Corpus” and achieve a 4x speedup over the T5-XXL model. The GitHub repo associated with this paper can be found HERE .
How to Learn More about Deep Learning Research
At our upcoming event this November 16th-18th in San Francisco, ODSC West 2021 will feature a plethora of talks, workshops, and training sessions on deep learning and deep learning research. You can register now for 60% off all ticket types before the discount drops to 40% in a few weeks. Some highlighted sessions on deep learning include:
Sessions on Deep Learning and Deep Learning Research:
- GANs: Theory and Practice, Image Synthesis With GANs Using TensorFlow: Ajay Baranwal | Center Director | Center for Deep Learning in Electronic Manufacturing, Inc
- Machine Learning With Graphs: Going Beyond Tabular Data: Dr. Clair J. Sullivan | Data Science Advocate | Neo4j
- Deep Dive into Reinforcement Learning with PPO using TF-Agents & TensorFlow 2.0: Oliver Zeigermann | Software Developer | embarc Software Consulting GmbH
- Get Started with Time-Series Forecasting using the Google Cloud AI Platform: Karl Weinmeister | Developer Relations Engineering Manager | Google
Sessions on Machine Learning:
- Towards More Energy-Efficient Neural Networks? Use Your Brain!: Olaf de Leeuw | Data Scientist | Dataworkz
- Practical MLOps: Automation Journey: Evgenii Vinogradov, PhD | Head of DHW Development | YooMoney
- Applications of Modern Survival Modeling with Python: Brian Kent, PhD | Data Scientist | Founder The Crosstab Kite
- Using Change Detection Algorithms for Detecting Anomalous Behavior in Large Systems: Veena Mendiratta, PhD | Adjunct Faculty, Network Reliability and Analytics Researcher | Northwestern University
Sessions on MLOps:
- Tuning Hyperparameters with Reproducible Experiments: Milecia McGregor | Senior Software Engineer | Iterative
- MLOps… From Model to Production: Filipa Peleja, PhD | Lead Data Scientist | Levi Strauss & Co
- Operationalization of Models Developed and Deployed in Heterogeneous Platforms: Sourav Mazumder | Data Scientist, Thought Leader, AI & ML Operationalization Leader | IBM
- Develop and Deploy a Machine Learning Pipeline in 45 Minutes with Ploomber: Eduardo Blancas | Data Scientist | Fidelity Investment

Daniel Gutierrez, ODSC
Daniel D. Gutierrez is a practicing data scientist who’s been working with data long before the field came in vogue. As a technology journalist, he enjoys keeping a pulse on this fast-paced industry. Daniel is also an educator having taught data science, machine learning and R classes at the university level. He has authored four computer industry books on database and data science technology, including his most recent title, “Machine Learning and Data Science: An Introduction to Statistical Learning Methods with R.” Daniel holds a BS in Mathematics and Computer Science from UCLA.

How AI Impacts Diversity in College Admissions
Business + Management posted by Zac Amos Aug 27, 2024 Higher education institutions are beginning to use artificial intelligence to review college applications. Where does this...

Scaling AI Initiatives in Retail
West 2024 Conferences posted by ODSC Community Aug 27, 2024 Editor’s note: Fatih is a speaker for ODSC West this October 29th-31st! Be sure to check...

Just Do Something with AI: Bridging the Business Communication Gap for ML Practitioners
West 2024 Career Insights posted by ODSC Community Aug 26, 2024 Editor’s note: Stephanie Kirmer is a speaker for ODSC West this October 29th-31st. Be sure to...

Deep learning for medical image interpretation
Abstract/contents, description.
Type of resource | text |
---|---|
Form | electronic resource; remote; computer; online resource |
Extent | 1 online resource. |
Place | California |
Place | [Stanford, California] |
Publisher | [Stanford University] |
Copyright date | 2021; ©2021 |
Publication date | 2021; 2021 |
Issuance | monographic |
Language | English |
Creators/Contributors
Author | Rajpurkar, Pranav Samir |
---|---|
Degree supervisor | Liang, Percy |
Thesis advisor | Liang, Percy |
Thesis advisor | Bernstein, Michael S, 1984- |
Degree committee member | Bernstein, Michael S, 1984- |
Associated with | Stanford University, Computer Science Department |
Genre | Theses |
---|---|
Genre | Text |
Bibliographic information
Statement of responsibility | Pranav Rajpurkar. |
---|---|
Note | Submitted to the Computer Science Department. |
Thesis | Thesis Ph.D. Stanford University 2021. |
Location |
Access conditions
Version 1 | May 9, 2024 | You are viewing this version | |
---|
Each version has a distinct URL, but you can use this PURL to access the latest version. https://purl.stanford.edu/jc097kx0188
Also listed in
Loading usage metrics...

- Current Members
- Off-Campus Students
- Robot Videos
- Funded Projects
- Publications by Year
- Publications by Type
- Robot Learning Lecture
- Robot Learning IP
- Humanoid Robotics Seminar
- Research Oberseminar
- New, Open Topics
- Ongoing Theses
- Completed Theses
- External Theses
- Advice for Thesis Students
- Thesis Checklist and Template
- Jobs and Open Positions
- Current Openings
- Information for Applicants
- Apply Here!
- TU Darmstadt Student Hiwi Jobs
- Contact Information
Currently Available Theses Topics
We offer these current topics directly for Bachelor and Master students at TU Darmstadt who can feel free to DIRECTLY contact the thesis advisor if you are interested in one of these topics. Excellent external students from another university may be accepted but are required to first email Jan Peters before contacting any other lab member for a thesis topic. Note that we cannot provide funding for any of these theses projects.
We highly recommend that you do either our robotics and machine learning lectures ( Robot Learning , Statistical Machine Learning ) or our colleagues ( Grundlagen der Robotik , Probabilistic Graphical Models and/or Deep Learning). Even more important to us is that you take both Robot Learning: Integrated Project, Part 1 (Literature Review and Simulation Studies) and Part 2 (Evaluation and Submission to a Conference) before doing a thesis with us.
In addition, we are usually happy to devise new topics on request to suit the abilities of excellent students. Please DIRECTLY contact the thesis advisor if you are interested in one of these topics. When you contact the advisor, it would be nice if you could mention (1) WHY you are interested in the topic (dreams, parts of the problem, etc), and (2) WHAT makes you special for the projects (e.g., class work, project experience, special programming or math skills, prior work, etc.). Supplementary materials (CV, grades, etc) are highly appreciated. Of course, such materials are not mandatory but they help the advisor to see whether the topic is too easy, just about right or too hard for you.
Only contact *ONE* potential advisor at the same time! If you contact a second one without first concluding discussions with the first advisor (i.e., decide for or against the thesis with her or him), we may not consider you at all. Only if you are super excited for at most two topics send an email to both supervisors, so that the supervisors are aware of the additional interest.
FOR FB16+FB18 STUDENTS: Students from other depts at TU Darmstadt (e.g., ME, EE, IST), you need an additional formal supervisor who officially issues the topic. Please do not try to arrange your home dept advisor by yourself but let the supervising IAS member get in touch with that person instead. Multiple professors from other depts have complained that they were asked to co-supervise before getting contacted by our advising lab member.
NEW THESES START HERE
Blending Deep Generative Models using Stochastic Optimization
Topic: This Master Thesis aims to explore the blending of deep generative models using stochastic optimization techniques [1], focusing on reactive motion generation for robotics. The research will encompass the training of deep generative models, such as Score-based [2], or Flow-based models, specifically utilizing the JAX framework for efficient computation. A significant part of the thesis will involve deriving a mixture of experts algorithm, which leverages these trained generative models in combination with other manually specified objectives to enhance the performance of the motion generator. This integration aims to create more adaptive and responsive robotic behaviors in dynamic environments, offering a substantial advancement over existing methods.
Requirements
- Strong Python programming skills
- Knowledge in Machine Learning
- Experience with deep learning libraries and JAX is a plus
Interested students can apply by sending an e-mail to [email protected] and attaching the documents mentioned below:
- Curriculum Vitae
- Motivation letter explaining why you would like to work on this topic and why you are the perfect candidate
References [1] Hansel, K.; Urain, J.; Peters, J.; Chalvatzaki, G. (2023). Hierarchical Policy Blending as Inference for Reactive Robot Control, 2023 IEEE International Conference on Robotics and Automation (ICRA), IEEE. [2] Urain, J.; Funk, N.; Peters, J.; Chalvatzaki G (2023). SE(3)-DiffusionFields: Learning smooth cost functions for joint grasp and motion optimization through diffusion, International Conference on Robotics and Automation (ICRA).
Self-supervised learning of a visual object-centric representation for robotic manipulation
Scope: External Master Thesis 🇫🇷 This master thesis will be conducted with our French partners at Ecole Centrale de Lyon . Possibility of ERASMUS scholarship. Advisor: Alexandre Chapin , Liming Chen , Emmanuel Dellandrea Added: 2024-07-15 Start: ASAP Topic:
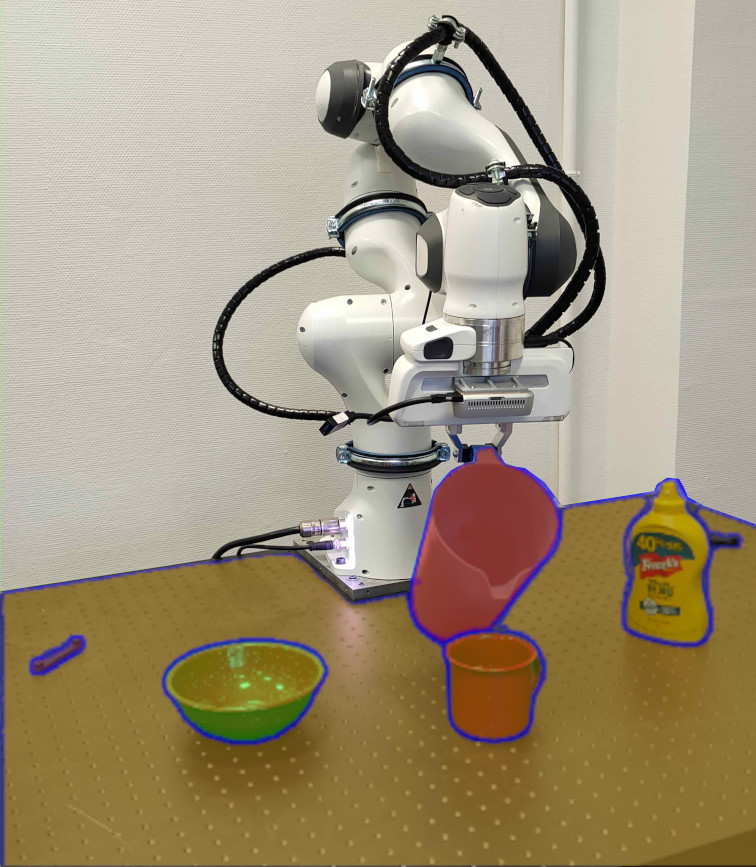
Vision-based learning for robotic manipulation often relies on holistic visual scene representations, where the environment is depicted as a single vector. This method is suboptimal for handling diverse scenes and objects in unconstrained environments. Better representations can improve generalization and data efficiency in robotic learning [1]. Inspired by human perception, object-centric representation has been developed to represent environments with multiple vectors, each corresponding to an object's properties [2]. However, these methods mainly use synthetic datasets [3, 4, 5] and struggle with real-world scenarios [6]. With advances in self-supervised learning for vision models [7, 8], which show promise for object discovery, we propose pre-training an object-centric representation using self-supervised methods to scale to real-world scenarios. This thesis will focus on: Developing and training an object-centric self-supervised model on a real-world dataset. Pre-training the model on a real-world robotic dataset. Applying the pre-trained model to visual-based robotic manipulation tasks.
Interested students can apply by sending the required documents to [email protected] and attaching the required documents mentioned below.
- Experience with the Pytorch library
Preferred Qualifications
- Prior experience in Computer Vision and/or Robotics is preferred
- Use of distributed environment for learning of models (SLURM)
- Knowledge on recent self-supervised learning methods for vision [7, 8]
Required Documents
References [1] O. Kroemer et al. “A review of robot learning for manipulation: Challenges, representations, and algorithms” (2019) [2] F. Locatello et al. “Object-centric learning with Slot Attention” (2020) [3] G. Singh et al. “Illiterate DALL-E learns to compose” (2021) [4] T. Kipf et al. “Conditional object-centric learning from video” (2022) [5] G. Singh et al. “Simple Unsupervised Object-Centric Learning for Complex and Naturalistic Videos” (2022) [6] Z. Wu et al. “SlotDiffusion: Object-Centric Generative Modeling with Diffusion Models” (2023) [7] M. Caron et al. “Emerging Properties in Self-Supervised Vision Transformers” (2021) [8] O. J. Hénaff et al. “Object discovery and representation networks” (2022)
Imitation Learning for High-Speed Robot Air Hockey
Scope: Master thesis Advisor: Puze Liu and Julen Urain De Jesus Start: ASAP Topic:
High-speed reactive motion is one of the fundamental capabilities of robots to achieve human-level behavior. Optimization-based methods suffer from real-time requirement when the problem is non-convex and contains constraints. Reinforcement learning requires extensive reward engineering to achieve the desired performance. Imitation learning, on the other hand, gathers human knowledge directly from data collection and enables robots to learn natural movements efficiently. In this paper, we explore how imitation learning can be performed in a complex robot Air Hockey Task. The robot needs to learn not only low-level skills, but also high-level tactics from human demonstrations.
- Knowledge in Machine Learning / Supervised Learning
- Good Knowledge in Robotics
- Experience with deep learning libraries is a plus
References * Chi, Cheng, et al. "Diffusion policy: Visuomotor policy learning via action diffusion." arXiv preprint arXiv:2303.04137 (2023). * Liu, Puze, et al. "Robot reinforcement learning on the constraint manifold." Conference on Robot Learning. PMLR (2022). * Pan, Yunpeng, et al. "Imitation learning for agile autonomous driving." The International Journal of Robotics Research 39.2-3 (2020). Interested students can apply by sending an e-mail to [email protected] and attaching the required documents mentioned above.
Walk your network: investigating neural network’s location in Q-learning methods.
Scope: Master thesis Advisor: Theo Vincent Start: Flexible Topic:
Q-learning methods are at the heart of Reinforcement Learning. They have been shown to outperform humans on some complex tasks such as playing video games [1]. In robotics, where the action space is in most cases continuous, actor-critic methods are relying on Q-learning methods to learn the critic [2]. Although Q-learning methods have been extensively studied in the past, little focus has been placed on the way the online neural network is exploring the space of Q functions. Most approaches focus on crafting a loss that would make the agent learn better policies [3]. Here, we offer a thesis that focuses on the position of the online Q neural network in the space of Q functions. The student will first investigate this idea on simple problems before comparing the performance to strong baselines such as DQN or REM [1, 4] on Atari games. Depending on the result, the student might as well get into MuJoCo and compare the results with SAC [2]. The student will be welcome to propose some ideas as well.
Highly motivated students can apply by sending an email to [email protected] . Please attach your CV, a grade sheet and clearly state why you are interested in this topic. Students who have followed the Reinforcement Learning or Robot Learning course will be prioritized.
- Knowledge in Reinforcement Learning
References [1] Mnih, Volodymyr, et al. "Human-level control through deep reinforcement learning." nature 518.7540 (2015): 529-533. [2] Haarnoja, Tuomas, et al. "Soft actor-critic: Off-policy maximum entropy deep reinforcement learning with a stochastic actor." International conference on machine learning. PMLR, 2018. [3] Hessel, Matteo, et al. "Rainbow: Combining improvements in deep reinforcement learning." Proceedings of the AAAI conference on artificial intelligence. Vol. 32. No. 1. 2018. [4] Agarwal, R., Schuurmans, D. & Norouzi, M.. (2020). An Optimistic Perspective on Offline Reinforcement Learning International Conference on Machine Learning (ICML).
Co-optimizing Hand and Action for Robotic Grasping of Deformable objects
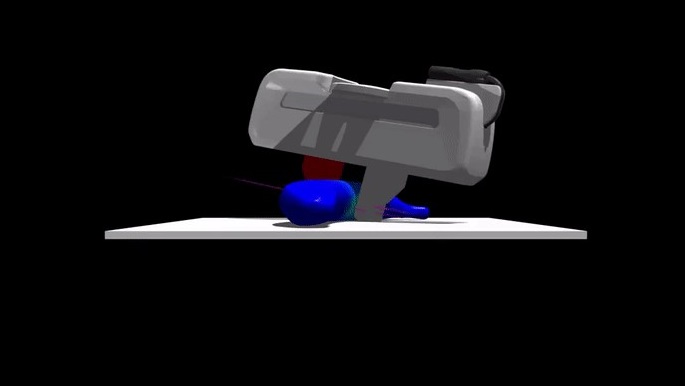
This project aims to advance deformable object manipulation by co-optimizing robot gripper morphology and control policies. The project will involve utilizing existing simulation environments for deformable object manipulation [2] and implementing a method to jointly optimize gripper morphology and grasp policies within the simulation.
Required Qualification:
- Familiarity with deep learning libraries such as PyTorch or Tensorflow
Preferred Qualification:
- Attendance of the lectures "Statistical Machine Learning", "Computational Engineering and Robotics" and "Robot Learning"
Application Requirements:
Interested students can apply by sending an e-mail to [email protected] and attaching the required documents mentioned above.
References: [1] Xu, Jie, et al. "An End-to-End Differentiable Framework for Contact-Aware Robot Design." Robotics: Science & Systems. 2021. [2] Huang, Isabella, et al. "DefGraspNets: Grasp Planning on 3D Fields with Graph Neural Nets." arXiv preprint arXiv:2303.16138 (2023).
Geometry-Aware Diffusion Models for Robotics
In this thesis, you will work on developing an imitation learning algorithm using diffusion models for robotic manipulation tasks, such as the ones in [2, 3, 4], but taking into account the geometry of the task space.
If this sounds interesting, please send an email to [email protected] and [email protected] , and possibly attach your CV, highlighting the relevant courses you took in robotics and machine learning.
What's in it for you:
- You get to work on an exciting topic at the intersection of deep-learning and robotics
- We will supervise you closely throughout your thesis
- Depending on the results, we will aim for an international conference publication
Requirements:
- Be motivated -- we will support you a lot, but we expect you to contribute a lot too
- Robotics knowledge
- Experience setting up deep learning pipelines -- from data collection, architecture design, training, and evaluation
- PyTorch -- especially experience writing good parallelizable code (i.e., runs fast in the GPU)
References: [1] https://arxiv.org/abs/2112.10752 [2] https://arxiv.org/abs/2308.01557 [3] https://arxiv.org/abs/2209.03855 [4] https://arxiv.org/abs/2303.04137 [5] https://arxiv.org/abs/2205.09991
Learning Latent Representations for Embodied Agents
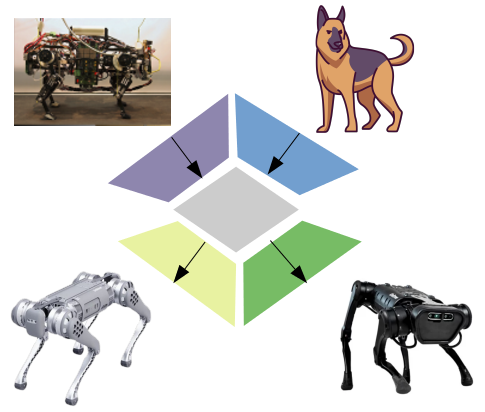
Interested students can apply by sending an E-Mail to [email protected] and attaching the required documents mentioned below.
- Experience with TensorFlow/PyTorch
- Familiarity with core Machine Learning topics
- Experience programming/controlling robots (either simulated or real world)
- Knowledgeable about different robot platforms (quadrupeds and bipedal robots)
- Resume / CV
- Cover letter explaining why this topic fits you well and why you are an ideal candidate
References: [1] Ho and Ermon. "Generative adversarial imitation learning" [2] Arenz, et al. "Efficient Gradient-Free Variational Inference using Policy Search"
Characterizing Fear-induced Adaptation of Balance by Inverse Reinforcement Learning
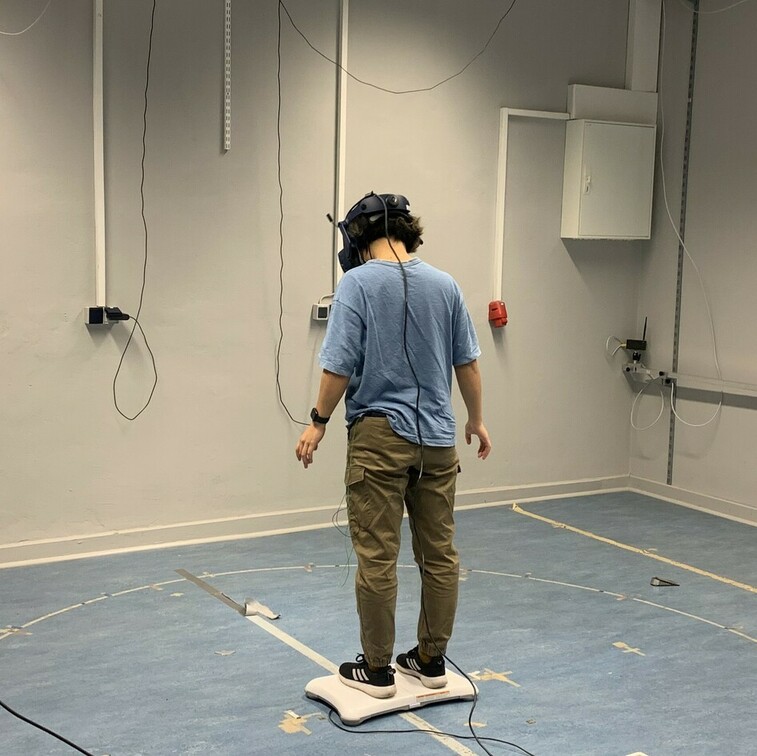
Interested students can apply by sending an E-Mail to [email protected] and attaching the required documents mentioned below.
- Basic knowledge of reinforcement learning
- Hand-on experience with reinforcement learning or inverse reinforcement learning
- Cognitive science background
References: [1] Maki, et al. "Fear of Falling and Postural Performance in the Elderly" [2] Davis et al. "The relationship between fear of falling and human postural control" [3] Ho and Ermon. "Generative adversarial imitation learning"
Timing is Key: CPGs for regularizing Quadruped Gaits learned with DRL
To tackle this problem we want to utilize Central Pattern Generators (CPGs), which can generate timings for ground contacts for the four feet. The policy gets rewarded for complying with the contact patterns of the CPGs. This leads to a straightforward way of regularizing and steering the policy to a natural gait without posing too strong restrictions on it. We first want to manually find fitting CPG parameters for different gait velocities and later move to learning those parameters in an end-to-end fashion.
Highly motivated students can apply by sending an E-Mail to [email protected] and attaching the required documents mentioned below.
Minimum Qualification:
- Good Python programming skills
- Basic knowledge of the PyTorch library
- Basic knowledge of Reinforcement Learning
- Good knowledge of the PyTorch library
- Basic knowledge of the MuJoCo simulator
References: [1] Cheng, Xuxin, et al. "Extreme Parkour with Legged Robots."
Damage-aware Reinforcement Learning for Deformable and Fragile Objects
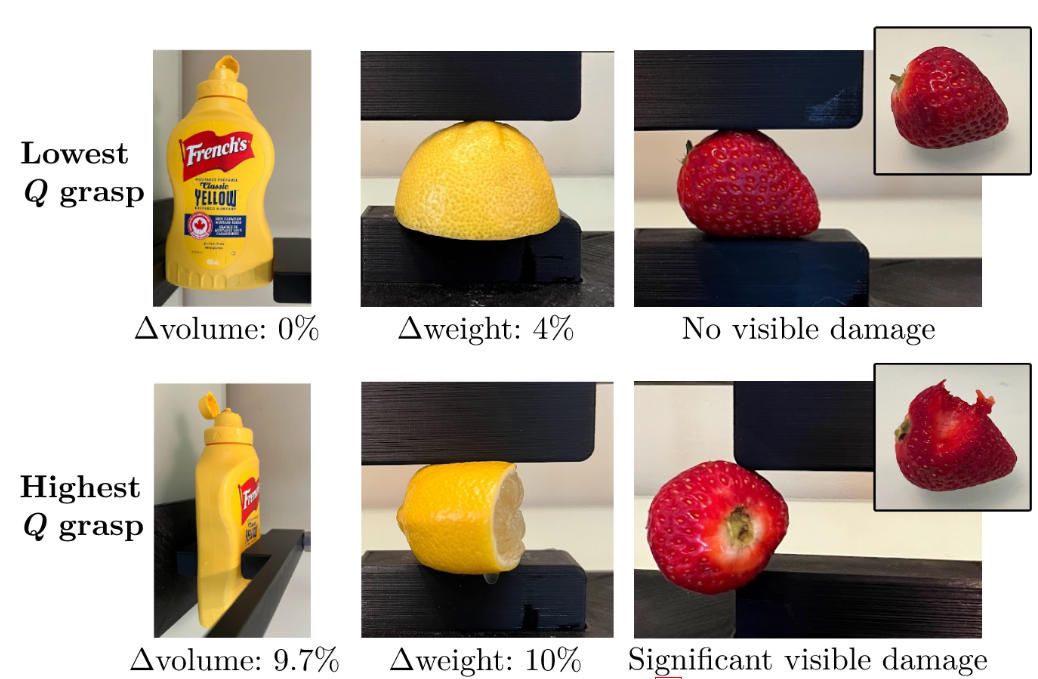
Goal of this thesis will be the development and application of a model-based reinforcement learning method on real robots. Your tasks will include: 1. Setting up a simulation environment for deformable object manipulation 2. Utilizing existing models for stress and deformability prediction[1] 3. Implementing a reinforcement learning method to work in simulation and, if possible, on the real robot methods.
If you are interested in this thesis topic and believe you possess the necessary skills and qualifications, please submit your application, including a resume and a brief motivation letter explaining your interest and relevant experience. Please send your application to [email protected].
Required Qualification :
- Enthusiasm for and experience in robotics, machine learning, and simulation
- Strong programming skills in Python
Desired Qualification :
- Attendance of the lectures "Statistical Machine Learning", "Computational Engineering and Robotics" and (optionally) "Robot Learning"
References: [1] Huang, I., Narang, Y., Bajcsy, R., Ramos, F., Hermans, T., & Fox, D. (2023). DefGraspNets: Grasp Planning on 3D Fields with Graph Neural Nets. arXiv preprint arXiv:2303.16138.
Imitation Learning meets Diffusion Models for Robotics
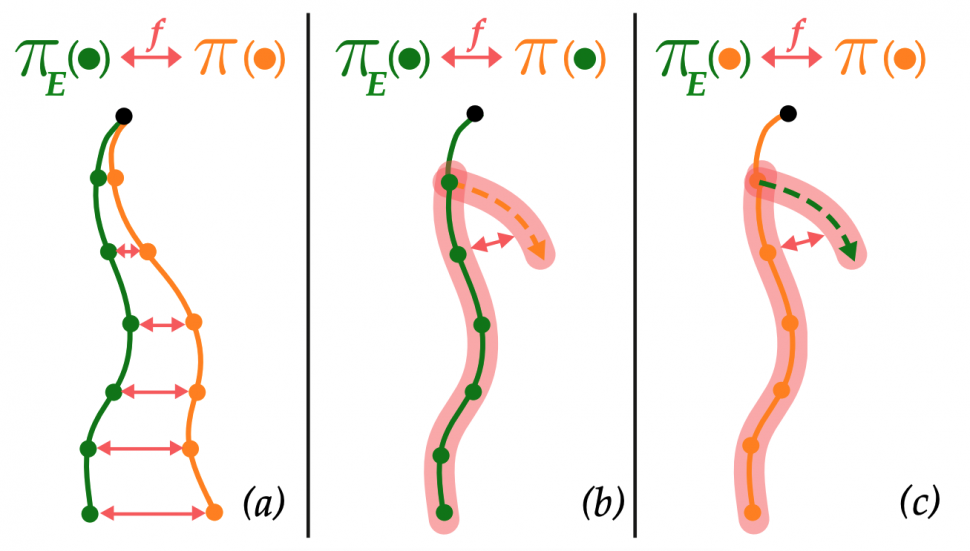
The objective of this thesis is to build upon prior research [2, 3] to establish a connection between Diffusion Models and Imitation Learning. We aim to explore how to exploit Diffusion Models and improve the performance of Imitation learning algorithms that interact with the world.
We welcome highly motivated students to apply for this opportunity by sending an email expressing their interest to Firas Al-Hafez ( [email protected] ) Julen Urain ( [email protected] ). Please attach your letter of motivation and CV, and clearly state why you are interested in this topic and why you are the ideal candidate for this position.
Required Qualification : 1. Strong Python programming skills 2. Basic Knowledge in Imitation Learning 3. Interest in Diffusion models, Reinforcement Learning
Desired Qualification : 1. Attendance of the lectures "Statistical Machine Learning", "Computational Engineering and Robotics" and/or "Reinforcement Learning: From Fundamentals to the Deep Approaches"
References: [1] Song, Yang, and Stefano Ermon. "Generative modeling by estimating gradients of the data distribution." Advances in neural information processing systems 32 (2019). [2] Ho, Jonathan, and Stefano Ermon. "Generative adversarial imitation learning." Advances in neural information processing systems 29 (2016). [3] Garg, D., Chakraborty, S., Cundy, C., Song, J., & Ermon, S. (2021). Iq-learn: Inverse soft-q learning for imitation. Advances in Neural Information Processing Systems, 34, 4028-4039. [4] Chen, R. T., & Lipman, Y. (2023). Riemannian flow matching on general geometries. arXiv preprint arXiv:2302.03660.
- Be extremely motivated -- we will support you a lot, but we expect you to contribute a lot too
Scaling Behavior Cloning to Humanoid Locomotion
Scope: Bachelor / Master thesis Advisor: Joe Watson Added: 2023-10-07 Start: ASAP Topic: In a previous project [1], I found that behavior cloning (BC) was a surprisingly poor baseline for imitating humanoid locomotion. I suspect the issue may lie in the challenges of regularizing high-dimensional regression.
The goal of this project is to investigate BC for humanoid imitation, understand the scaling issues present, and evaluate possible solutions, e.g. regularization strategies from the regression literature.
The project will be building off Google Deepmind's Acme library [2], which has BC algorithms and humanoid demonstration datasets [3] already implemented, and will serve as the foundation of the project.
To apply, email [email protected] , ideally with a CV and transcript so I can assess your suitability.
- Experience, interest and enthusiasm for the intersection of robot learning and machine learning
- Experience with Acme and JAX would be a benefit, but not necessary
References: [1] https://arxiv.org/abs/2305.16498 [2] https://github.com/google-deepmind/acme [3] https://arxiv.org/abs/2106.00672
Robot Gaze for Communicating Collision Avoidance Intent in Shared Workspaces
Scope: Bachelor/Master thesis Advisor: Alap Kshirsagar , Dorothea Koert Added: 2023-09-27 Start: ASAP
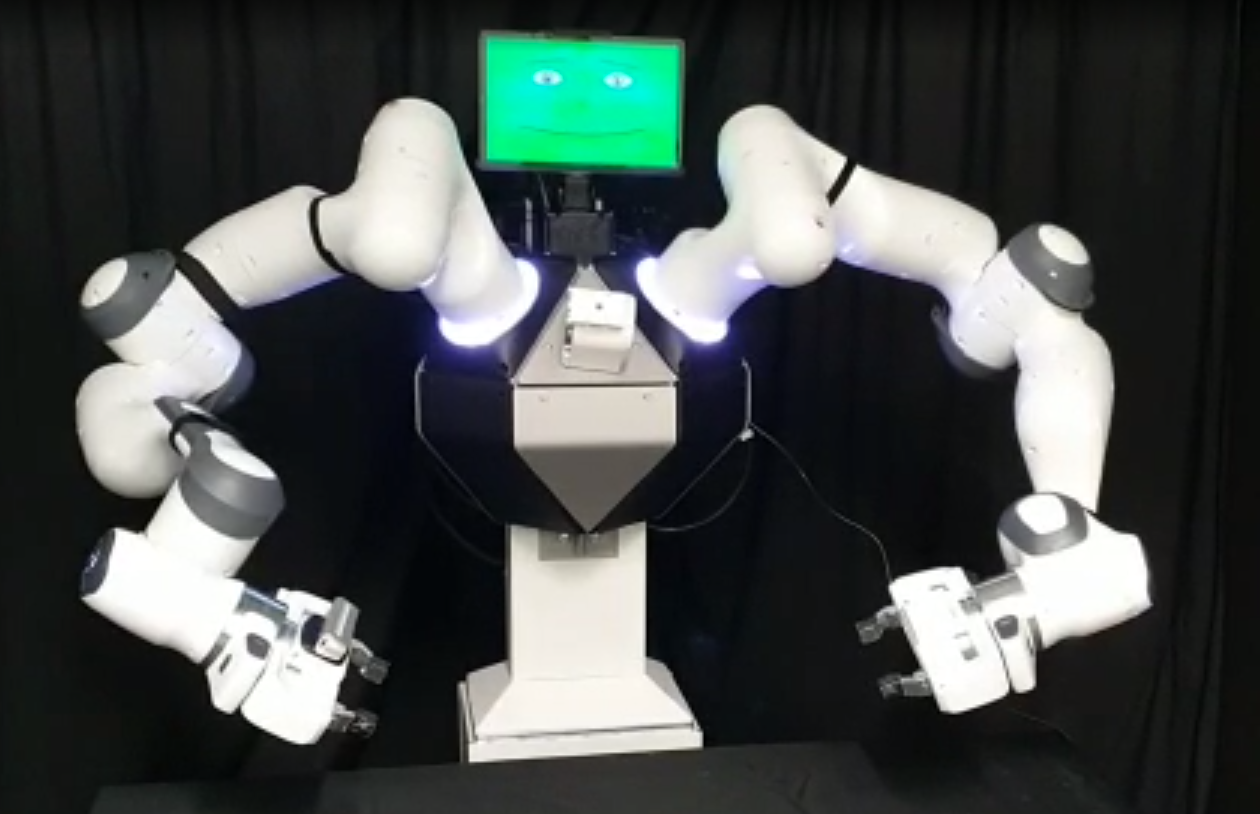
Topic: In order to operate close to non-experts, future robots require both an intuitive form of instruction accessible to lay users and the ability to react appropriately to a human co-worker. Instruction by imitation learning with probabilistic movement primitives (ProMPs) [1] allows capturing tasks by learning robot trajectories from demonstrations including the motion variability. However, appropriate responses to human co-workers during the execution of the learned movements are crucial for fluent task execution, perceived safety, and subjective comfort. To facilitate such appropriate responsive behaviors in human-robot interaction, the robot needs to be able to react to its human workspace co-inhabitant online during the execution. Also, the robot needs to communicate its motion intent to the human through non-verbal gestures such as eye and head gazes [2][3]. In particular for humanoid robots, combining motions of arms with expressive head and gaze directions is a promising approach that has not yet been extensively studied in related work.
Goals of the thesis:
- Develop a method to combine robot head/gaze motion with ProMPs for online collision avoidance
- Implement the method on a Franka-Emika Panda Robot
- Evaluate and compare the implemented behaviors in a study with human participants
Highly motivated students can apply by sending an email to [email protected]. Please attach your CV and transcript, and clearly state your prior experiences and why you are interested in this topic.
- Strong Programming Skills in python
- Prior experience with Robot Operating System (ROS) and user studies would be beneficial
- Strong motivation for human-centered robotics including design and implementation of a user study
References : [1] Koert, Dorothea, et al. "Learning intention aware online adaptation of movement primitives." IEEE Robotics and Automation Letters 4.4 (2019): 3719-3726. [2] Admoni, Henny, and Brian Scassellati. "Social eye gaze in human-robot interaction: a review." Journal of Human-Robot Interaction 6.1 (2017): 25-63. [3] Lemasurier, Gregory, et al. "Methods for expressing robot intent for human–robot collaboration in shared workspaces." ACM Transactions on Human-Robot Interaction (THRI) 10.4 (2021): 1-27.
Tactile Sensing for the Real World
Topic: Tactile sensing is a crucial sensing modality that allows humans to perform dexterous manipulation[1]. In recent years, the development of artificial tactile sensors has made substantial progress, with current models relying on cameras inside the fingertips to extract information about the points of contact [2]. However, robotic tactile sensing is still a largely unsolved topic despite these developments. A central challenge of tactile sensing is the extraction of usable representations of sensor readings, especially since these generally contain an incomplete view of the environment.
Recent model-based reinforcement learning methods like Dreamer [3] leverage latent state-space models to reason about the environment from partial and noisy observations. However, more work has yet to be done to apply such methods to real-world manipulation tasks. Hence, this thesis will explore whether Dreamer can solve challenging real-world manipulation tasks by leveraging tactile information. Initial results suggest that tasks like peg-in-a-hole can indeed be solved with Dreamer in simulation (see figure above), but the applicability of this method in the real world has yet to be shown.
In this work, you will work with state-of-the-art hardware and compute resources on a hot research topic with the option of publishing your work at a scientific conference.
Highly motivated students can apply by sending an email to [email protected]. Please attach a transcript of records and clearly state your prior experiences and why you are interested in this topic.
- Ideally experience with deep learning libraries like JAX or PyTorch
- Experience with reinforcement learning is a plus
- Experience with Linux
References [1] 2S Match Anest2, Roland Johansson Lab (2005), https://www.youtube.com/watch?v=HH6QD0MgqDQ [2] Gelsight Inc., Gelsight Mini, https://www.gelsight.com/gelsightmini/ [3] Hafner, D., Lillicrap, T., Ba, J., & Norouzi, M. (2019). Dream to control: Learning behaviors by latent imagination. arXiv preprint arXiv:1912.01603.
Large Vision-Language Neural Networks for Open-Vocabulary Robotic Manipulation
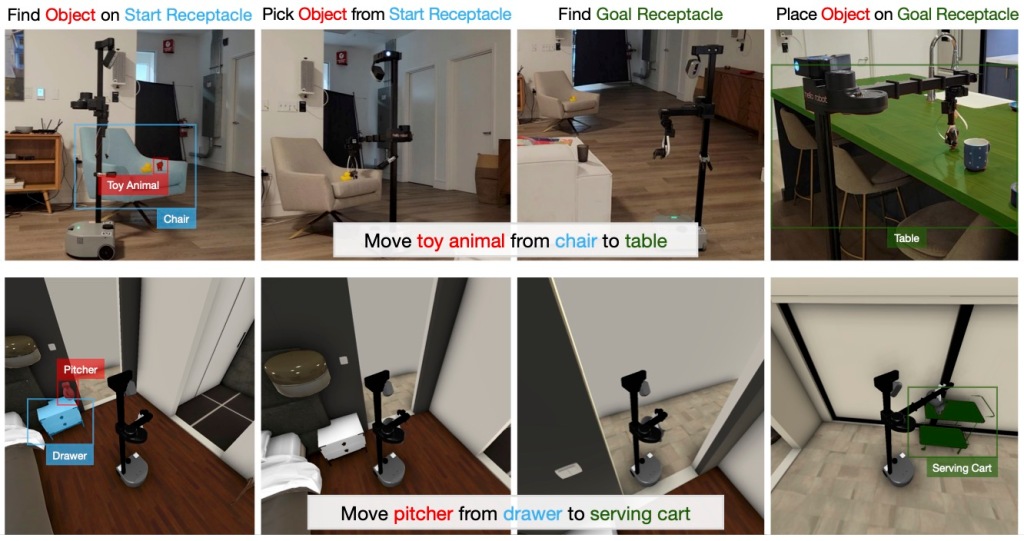
Robots are expected to soon leave their factory/laboratory enclosures and operate autonomously in everyday unstructured environments such as households. Semantic information is especially important when considering real-world robotic applications where the robot needs to re-arrange objects as per a set of language instructions or human inputs (as shown in the figure). Many sophisticated semantic segmentation networks exist [1]. However, a challenge when using such methods in the real world is that the semantic classes rarely align perfectly with the language input received by the robot. For instance, a human language instruction might request a ‘glass’ or ‘water’, but the semantic classes detected might be ‘cup’ or ‘drink’.
Nevertheless, with the rise of large language and vision-language models, we now have capable segmentation models that do not directly predict semantic classes but use learned associations between language queries and classes to give us ’open-vocabulary’ segmentation [2]. Some models are especially powerful since they can be used with arbitrary language queries.
In this thesis, we aim to build on advances in 3D vision-based robot manipulation and large open-vocabulary vision models [2] to build a full pick-and-place pipeline for real-world manipulation. We also aim to find synergies between scene reconstruction and semantic segmentation to determine if knowing the object semantics can aid the reconstruction of the objects and, in turn, aid manipulation.
Highly motivated students can apply by sending an e-mail expressing their interest to Snehal Jauhri (email: [email protected]) or Ali Younes (email: [email protected]), attaching your letter of motivation and possibly your CV.
Topic in detail : Thesis_Doc.pdf
Requirements: Enthusiasm, ambition, and a curious mind go a long way. There will be ample supervision provided to help the student understand basic as well as advanced concepts. However, prior knowledge of computer vision, robotics, and Python programming would be a plus.
References: [1] Y. Wu, A. Kirillov, F. Massa, W.-Y. Lo, and R. Girshick, “Detectron2”, https://github.com/facebookresearch/detectron2 , 2019. [2] F. Liang, B. Wu, X. Dai, K. Li, Y. Zhao, H. Zhang, P. Zhang, P. Vajda, and D. Marculescu, “Open-vocabulary semantic segmentation with mask-adapted clip,” in CVPR, 2023, pp. 7061–7070, https://github.com/facebookresearch/ov-seg
Dynamic Tiles for Deep Reinforcement Learning
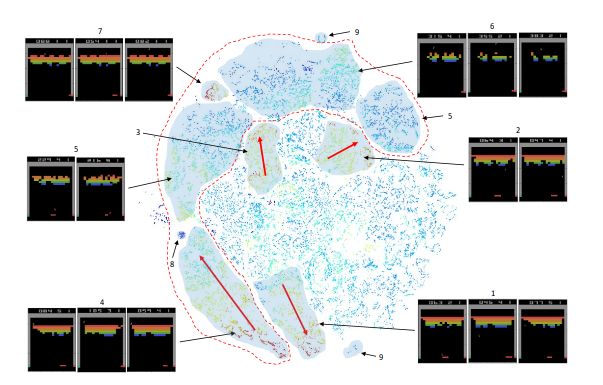
Linear approximators in Reinforcement Learning are well-studied and come with an in-depth theoretical analysis. However, linear methods require defining a set of features of the state to be used by the linear approximation. Unfortunately, the feature construction process is a particularly problematic and challenging task. Deep Reinforcement learning methods have been introduced to mitigate the feature construction problem: these methods do not require handcrafted features, as features are extracted automatically by the network during learning, using gradient descent techniques.
In simple reinforcement learning tasks, however, it is possible to use tile coding as features: Tiles are simply a convenient discretization of the state space that allows us to easily control the generalization capabilities of the linear approximator. The objective of this thesis is to design a novel algorithm for automatic feature extraction that generates a set of features similar to tile coding, but that can arbitrarily partition the state space and deal with arbitrary complex state space, such as images. The idea is to combine the feature extraction problem directly with Linear Reinforcement Learning methods, defining an algorithm that is able both to have the theoretical guarantees and good convergence properties of these methods and the flexibility of Deep Learning approaches.
- Curriculum Vitae (CV);
- A motivation letter explaining the reason for applying for this thesis and academic/career objectives.
Minimum knowledge
- Good Python programming skills;
- Basic knowledge of Reinforcement Learning.
Preferred knowledge
- Knowledge of the PyTorch library;
- Knowledge of the Atari environments (ale-py library).
- Knowledge of the MushroomRL library.
Accepted candidate will
- Define a generalization of tile coding working with an arbitrary input set (including images);
- Design a learning algorithm to adapt the tiles using data of interaction with the environment;
- Combine feature learning with standard linear methods for Reinforcement Learning;
- Verify the novel methodology in simple continuous state and discrete actions environments;
- (Optionally) Extend the experimental analysis to the Atari environment setting.
Deep Learning Meets Teleoperation: Constructing Learnable and Stable Inductive Guidance for Shared Control
This work considers policies as learnable inductive guidance for shared control. In particular, we use the class of Riemannian motion policies [3] and consider them as differentiable optimization layers [4]. We analyze (i) if RMPs can be pre-trained by learning from demonstrations [5] or reinforcement learning [6] given a specific context; (ii) and subsequently employed seamlessly for human-guided teleoperation thanks to their physically consistent properties, such as stability [3]. We believe this step eliminates the laborious process of constructing complex policies and leads to improved and generalizable shared control architectures.
Highly motivated students can apply by sending an e-mail expressing your interest to [email protected] and [email protected] , attaching your letter of motivation and possibly your CV.
- Experience with deep learning libraries (in particular Pytorch)
- Knowledge in reinforcement learning and/or machine learning
References: [1] Niemeyer, Günter, et al. "Telerobotics." Springer handbook of robotics (2016); [2] Selvaggio, Mario, et al. "Autonomy in physical human-robot interaction: A brief survey." IEEE RAL (2021); [3] Cheng, Ching-An, et al. "RMP flow: A Computational Graph for Automatic Motion Policy Generation." Springer (2020); [4] Jaquier, Noémie, et al. "Learning to sequence and blend robot skills via differentiable optimization." IEEE RAL (2022); [5] Mukadam, Mustafa, et al. "Riemannian motion policy fusion through learnable lyapunov function reshaping." CoRL (2020); [6] Xie, Mandy, et al. "Neural geometric fabrics: Efficiently learning high-dimensional policies from demonstration." CoRL (2023).
Dynamic symphony: Seamless human-robot collaboration through hierarchical policy blending
This work focuses on arbitration between the user and assistive policy, i.e., shared autonomy. Various works allow the user to influence the dynamic behavior explicitly and, therefore, could not satisfy stability guarantees [3]. We pursue the idea of formulating arbitration as a trajectory-tracking problem that implicitly considers the user's desired behavior as an objective [4]. Therefore, we extend the work of Hansel et al. [5], who employed probabilistic inference for policy blending in robot motion control. The proposed method corresponds to a sampling-based online planner that superposes reactive policies given a predefined objective. This method enables the user to implicitly influence the behavior without injecting energy into the system, thus satisfying stability properties. We believe this step leads to an alternative view of shared autonomy with an improved and generalizable framework.
Highly motivated students can apply by sending an e-mail expressing your interest to [email protected] or [email protected] , attaching your letter of motivation and possibly your CV.
References: [1] Niemeyer, Günter, et al. "Telerobotics." Springer handbook of robotics (2016); [2] Selvaggio, Mario, et al. "Autonomy in physical human-robot interaction: A brief survey." IEEE RAL (2021); [3] Dragan, Anca D., and Siddhartha S. Srinivasa. "A policy-blending formalism for shared control." IJRR (2013); [4] Javdani, Shervin, et al. "Shared autonomy via hindsight optimization for teleoperation and teaming." IJRR (2018); [5] Hansel, Kay, et al. "Hierarchical Policy Blending as Inference for Reactive Robot Control." IEEE ICRA (2023).
Feeling the Heat: Igniting Matches via Tactile Sensing and Human Demonstrations
In this thesis, we want to investigate the effectiveness of vision-based tactile sensors for solving dynamic tasks (igniting matches). Since the whole task is difficult to simulate, we directly collect real-world data to learn policies from the human demonstrations [2,3]. We believe that this work is an important step towards more advanced tactile skills.
Highly motivated students can apply by sending an e-mail expressing your interest to [email protected] and [email protected] , attaching your letter of motivation and possibly your CV.
- Good knowledge of Python
- Prior experience with real robots and Linux is a plus
References: [1] https://www.youtube.com/watch?v=HH6QD0MgqDQ [2] Learning Compliant Manipulation through Kinesthetic and Tactile Human-Robot Interaction; Klas Kronander and Aude Billard. [3] https://www.youtube.com/watch?v=jAtNvfPrKH8
Inverse Reinforcement Learning for Neuromuscular Control of Humanoids
Within this thesis, the problems of learning from observations and efficient exploration in overactued systems should be addressed. Regarding the former, novel methods incorporating inverse dynamics models into the inverse reinforcement learning problem [1] should be adapted and applied. To address the problem of efficient exploration in overactuted systems, two approaches should be implemented and compared. The first approach uses a handcrafted action space, which disables and modulates actions in different phases of the gait based on biomechanics knowledge [2]. The second approach uses a stateful policy to incorporate an inductive bias into the policy [3]. The thesis will be supervised in conjunction with Guoping Zhao ( [email protected] ) from the locomotion lab.
Highly motivated students can apply by sending an e-mail expressing their interest to Firas Al-Hafez ( [email protected] ), attaching your letter of motivation and possibly your CV. Try to make clear why you would like to work on this topic, and why you would be the perfect candidate for the latter.
Required Qualification : 1. Strong Python programming skills 2. Knowledge in Reinforcement Learning 3. Interest in understanding human locomotion
Desired Qualification : 1. Hands-on experience on robotics-related RL projects 2. Prior experience with different simulators 3. Attendance of the lectures "Statistical Machine Learning", "Computational Engineering and Robotics" and/or "Reinforcement Learning: From Fundamentals to the Deep Approaches"
References: [1] Al-Hafez, F.; Tateo, D.; Arenz, O.; Zhao, G.; Peters, J. (2023). LS-IQ: Implicit Reward Regularization for Inverse Reinforcement Learning, International Conference on Learning Representations (ICLR). [2] Ong CF; Geijtenbeek T.; Hicks JL; Delp SL (2019) Predicting gait adaptations due to ankle plantarflexor muscle weakness and contracture using physics-based musculoskeletal simulations. PLoS Computational Biology [3] Srouji, M.; Zhang, J:;Salakhutdinow, R. (2018) Structured Control Nets for Deep Reinforcement Learning, International Conference on Machine Learning (ICML)
Robotic Tactile Exploratory Procedures for Identifying Object Properties
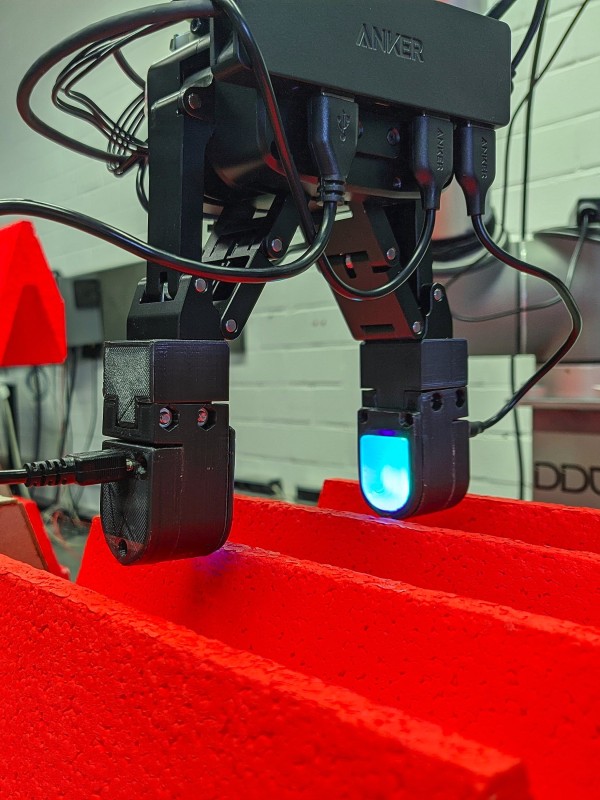
Goals of the thesis
- Literature review of robotic EPs for identifying object properties [2,3,4]
- Develop and implement robotic EPs for a Digit tactile sensor
- Compare performance of robotic EPs with human EPs
Desired Qualifications
- Interested in working with real robotic systems
- Python programming skills
Literature [1] Lederman and Klatzky, “Haptic perception: a tutorial” [2] Seminara et al., “Active Haptic Perception in Robots: A Review” [3] Chu et al., “Using robotic exploratory procedures to learn the meaning of haptic adjectives” [4] Kerzel et al., “Neuro-Robotic Haptic Object Classification by Active Exploration on a Novel Dataset”
Scaling learned, graph-based assembly policies
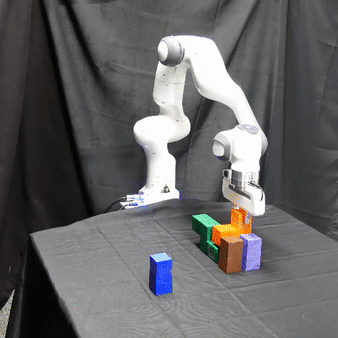
- scaling our previous methods to incorporate mobile manipulators or the Kobo bi-manual manipulation platform. The increased workspace of both would allow for handling a wider range of objects
- [2] has shown more powerful, yet, it includes running a MILP for every desired structure. Thus another idea could be to investigate approaches aiming to approximate this solution
- adapting the methods to handle more irregular-shaped objects / investigate curriculum learning
Highly motivated students can apply by sending an e-mail expressing your interest to [email protected] , attaching your letter of motivation and possibly your CV.
- Experience with deep learning libraries (in particular Pytorch) is a plus
- Experience with reinforcement learning / having taken Robot Learning is also a plus
References: [1] Learn2Assemble with Structured Representations and Search for Robotic Architectural Construction; Niklas Funk et al. [2] Graph-based Reinforcement Learning meets Mixed Integer Programs: An application to 3D robot assembly discovery; Niklas Funk et al. [3] Structured agents for physical construction; Victor Bapst et al.
Long-Horizon Manipulation Tasks from Visual Imitation Learning (LHMT-VIL): Algorithm
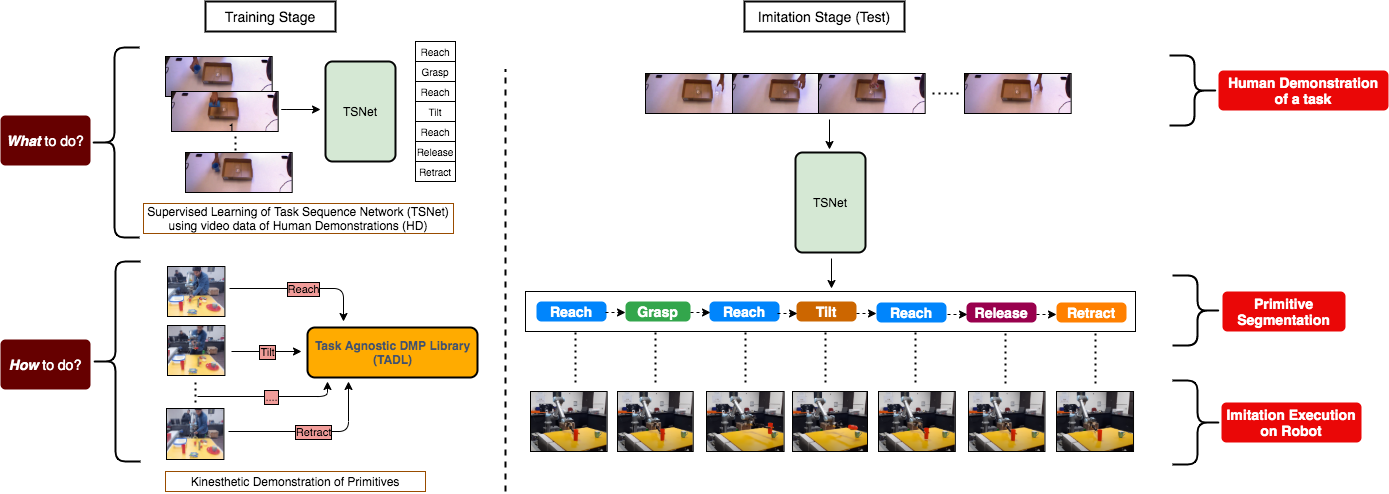
The proposed architecture can be broken down into the following sub-tasks: 1. Multi-object 6D pose estimation from video: Identify the object 6D poses in each video frame to generate the object trajectories 2. Action segmentation from video: Classify the action being performed in each video frame 3. High-level task representation learning: Learn the sequence of robotic movement primitives with the associated object poses such that the robot completes the demonstrated task 4. Low-level movement primitives: Create a database of low-level robotic movement primitives which can be sequenced to solve the long-horizon task
Desired Qualification: 1. Strong Python programming skills 2. Prior experience in Computer Vision and/or Robotics is preferred
Long-Horizon Manipulation Tasks from Visual Imitation Learning (LHMT-VIL): Dataset
During the project, we will create a large-scale dataset of videos of humans demonstrating industrial assembly sequences. The dataset will contain information of the 6D poses of the objects, the hand and body poses of the human, the action sequences among numerous other features. The dataset will be open-sourced to encourage further research on VIL.
[1] F. Sener, et al. "Assembly101: A Large-Scale Multi-View Video Dataset for Understanding Procedural Activities". CVPR 2022. [2] P. Sharma, et al. "Multiple Interactions Made Easy (MIME) : Large Scale Demonstrations Data for Imitation." CoRL, 2018.
Adaptive Human-Robot Interactions with Human Trust Maximization
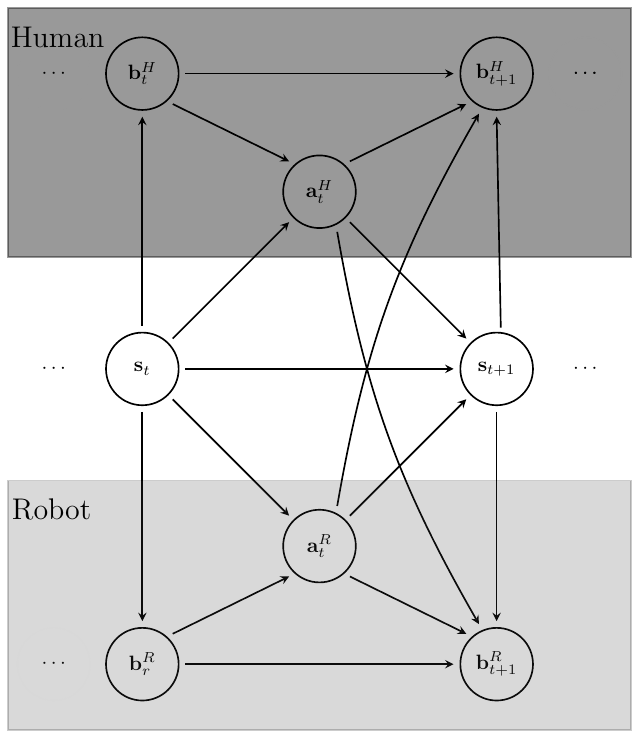
- Good knowledge of Python and/or C++;
- Good knowledge in Robotics and Machine Learning;
- Good knowledge of Deep Learning frameworks, e.g, PyTorch;
References: [1] Xu, Anqi, and Gregory Dudek. "Optimo: Online probabilistic trust inference model for asymmetric human-robot collaborations." ACM/IEEE HRI, IEEE, 2015; [2] Kwon, Minae, et al. "When humans aren’t optimal: Robots that collaborate with risk-aware humans." ACM/IEEE HRI, IEEE, 2020; [3] Chen, Min, et al. "Planning with trust for human-robot collaboration." ACM/IEEE HRI, IEEE, 2018; [4] Poole, Ben et al. “On variational bounds of mutual information”. ICML, PMLR, 2019.
Causal inference of human behavior dynamics for physical Human-Robot Interactions
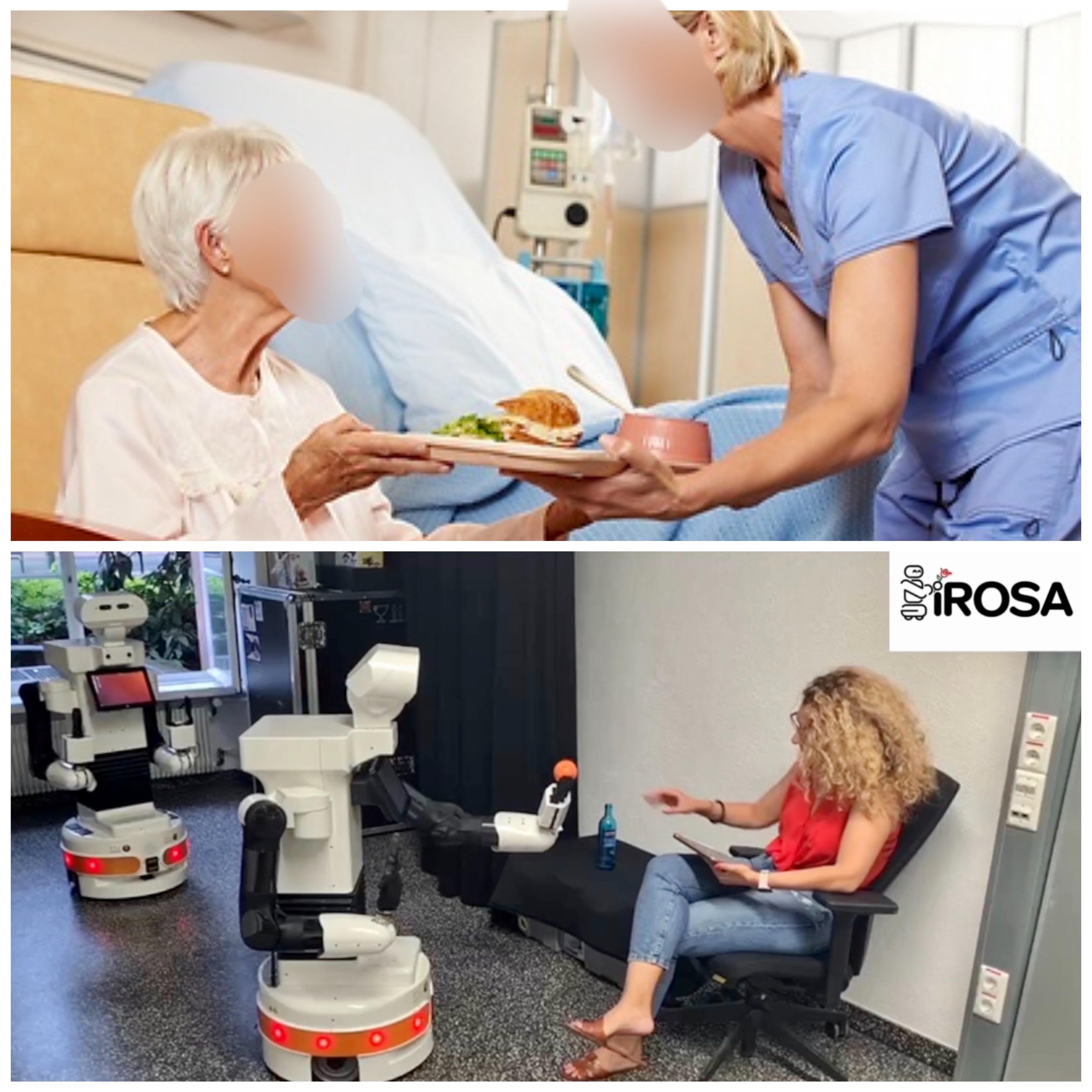
Highly motivated students can apply by sending an e-mail expressing your interest to [email protected] , attaching your a letter of motivation and possibly your CV.
- Good knowledge of Robotics;
- Good knowledge of Deep Learning frameworks, e.g, PyTorch
- Li, Q., Chalvatzaki, G., Peters, J., Wang, Y., Directed Acyclic Graph Neural Network for Human Motion Prediction, 2021 IEEE International Conference on Robotics and Automation (ICRA).
- Löwe, S., Madras, D., Zemel, R. and Welling, M., 2020. Amortized causal discovery: Learning to infer causal graphs from time-series data. arXiv preprint arXiv:2006.10833.
- Yang, W., Paxton, C., Mousavian, A., Chao, Y.W., Cakmak, M. and Fox, D., 2020. Reactive human-to-robot handovers of arbitrary objects. arXiv preprint arXiv:2011.08961.
Incorporating First and Second Order Mental Models for Human-Robot Cooperative Manipulation Under Partial Observability
Scope: Master Thesis Advisor: Dorothea Koert , Joni Pajarinen Added: 2021-06-08 Start: ASAP
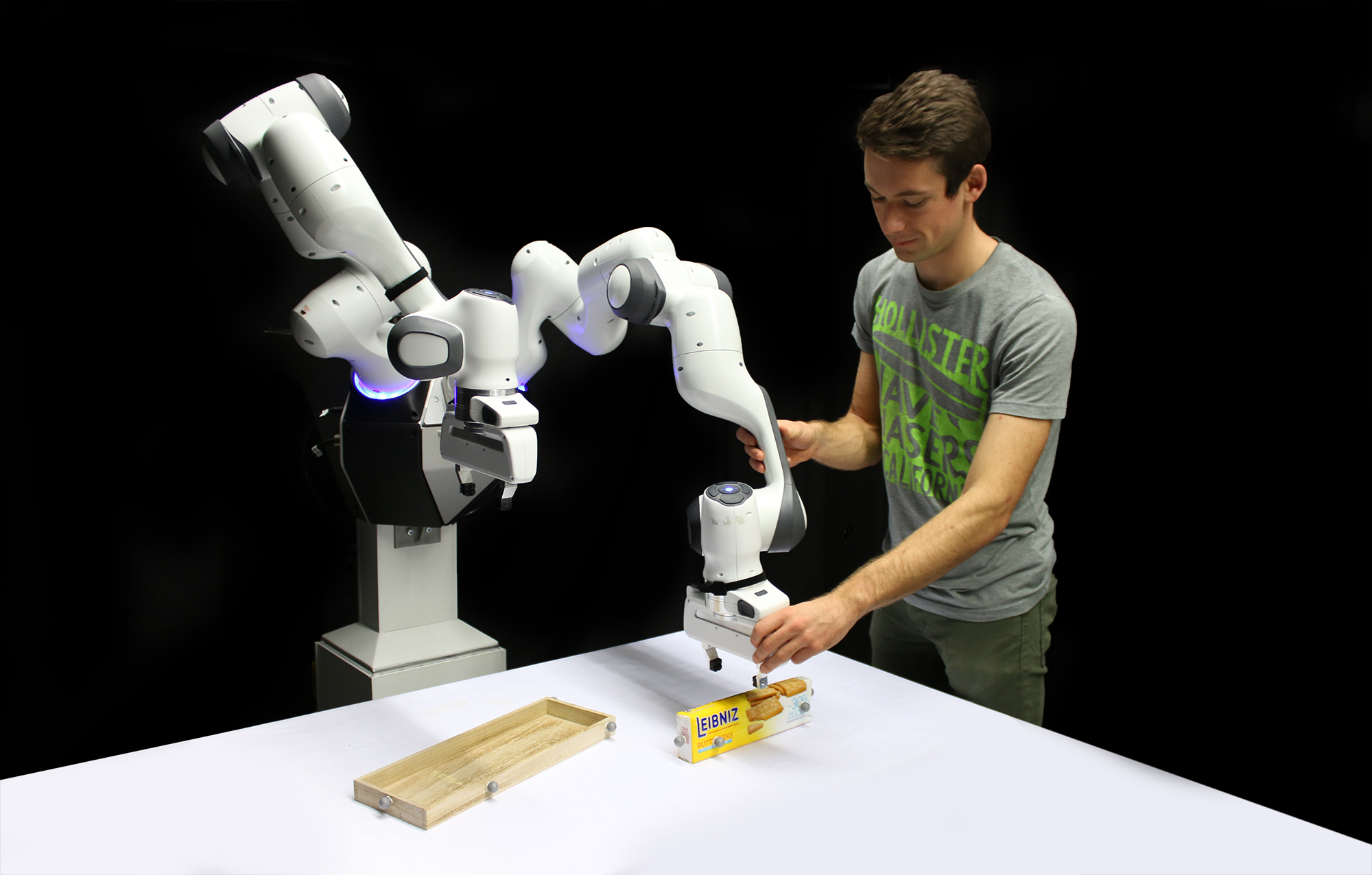
The ability to model the beliefs and goals of a partner is an essential part of cooperative tasks. While humans develop theory of mind models for this aim already at a very early age [1] it is still an open question how to implement and make use of such models for cooperative robots [2,3,4]. In particular, in shared workspaces human robot collaboration could potentially profit from the use of such models e.g. if the robot can detect and react to planned human goals or a human's false beliefs during task execution. To make such robots a reality, the goal of this thesis is to investigate the use of first and second order mental models in a cooperative manipulation task under partial observability. Partially observable Markov decision processes (POMDPs) and interactive POMDPs (I-POMDPs) [5] define an optimal solution to the mental modeling task and may provide a solid theoretical basis for modelling. The thesis may also compare related approaches from the literature and setup an experimental design for evaluation with the bi-manual robot platform Kobo.
Highly motivated students can apply by sending an e-mail expressing your interest to [email protected] attaching your CV and transcripts.
References:
- Wimmer, H., & Perner, J. Beliefs about beliefs: Representation and constraining function of wrong beliefs in young children's understanding of deception (1983)
- Sandra Devin and Rachid Alami. An implemented theory of mind to improve human-robot shared plans execution (2016)
- Neil Rabinowitz, Frank Perbet, Francis Song, Chiyuan Zhang, SM Ali Eslami,and Matthew Botvinick. Machine theory of mind (2018)
- Connor Brooks and Daniel Szafir. Building second-order mental models for human-robot interaction. (2019)
- Prashant Doshi, Xia Qu, Adam Goodie, and Diana Young. Modeling recursive reasoning by humans using empirically informed interactive pomdps. (2010)
Thesis Topic Proposals 2023-2024
Thesis information form.
Below you can see the thesis topics for 2023-2024. We offer 3 different thesis formats: - Format 1 : Regular thesis (fully supervised by KU Leuven) - Format 2 : Thesis in cooperation with a company (supervised by KU Leuven and the company) - Format 3 : Thesis with a company project within a company (supervised by the company)
NOTE: Additional late proposals are still added in October. Please regularly check this page for updates.
X = Thesis topic is taken
[NEW] = added less than a week ago
Company projects (format 3) for ECS or BDA
- A study on the accuracy and efficiency of savings simulations for households with flexible assets
- X Abandoned object detection and tracking in video data
- Accelerating the green transition by unraveling and forecasting energy market behaviour
- Assembling a set of representative energy profiles for simulation purposes
- Cleaning system automation using vision technology development on a combine harvester
- Comparison of Re-ID architectures in the context of plastic waste detection
- X Deep learning computer vision techniques for the removal and correction of interfering elements (e.g. shadows) from aerial images
- X Deep learning-based driving scenario detection on public road measurements
- X Deep Multimodal Fusion for Remote Sensing Applications
- Deep reinforcement learning for large-scale job shop scheduling problems
- Development of an Attention-Driven Approach for Remote Sensing Data Compression Considering Spatial and Temporal Correlations
- X Development of an Attention-Driven Approach for Remote Sensing Image Upscaling, Exploiting Temporal Variance and the Satellite’s Inherent Spatial Jittering
- Information Management
- GenAI Vision
- Digital Twins
- Fondant - open source
- Hybrid solution Note that when choosing one of these topics, the proposal and title still need to be decided in detail and approved by the program ([email protected]).
- X Domain adaptation for 3D lidar-based object detection using synthetic datasets
- Efficient Embedded Machine-Learning
- X Efficient Radiance Fields for View Synthesis with Depth Supervision : the information will be shared on Toledo [Master of Artifiicial Intelligence > Course documents > Confidential Thesis topics]
- Embedded neural network based modality detection for medical displays
- Enhancing Computer Vision Datasets through Bounding Box to Segmentation Conversion to Improve the Efficiency of the Waste Sorting and Recycling Processes
- Finetuning Segment Anything Model to custom Datasets
- Hyperparameter optimization of acoustic fault detection model(s)
- Intra-Oral-Scan (IOS) mesh restoration to remove scanning artifacts
- X LLM-based Customer Support
- Machine Learning-based anomaly detection on timeseries data (generated in the additive manufacturing context) for root cause analysis
- X Machine Learning-based Sound Event Localization, Detection and Tracking
- Multi-task transfer learning for sound event detection
- X Multimodal Document Classification and Information Extraction for Cross-Lingual Document Data
- Multimodal learning to measure chopping quality
- (P)Retrain and data sampling methods across various Medical Image Segmentation models
- X Probabilistic forecasting of real time electricity prices in the Belgian electricity market
- QualityNet - Speech Quality Estimation using Neural Networks
- X Robust Depth Estimation Algorithms for Automotive In-Cabin Safety Functions : the information will be shared on Toledo [Master of Artifiicial Intelligence > Course documents > Confidential Thesis topics]
- Scalable Audio Database Management System
- Simultaneous Enhancement of AI Model Performance and Data Quality to Improve the Efficiency of the Waste Sorting and Recycling Processes
- X Tackling data scarcity with transfer learning for wind noise detection in automobile end-of-line testing
- Topic related to AI act - title to be defined
- Topic related to federated learning - title to be defined
- Topic related to in Silico Testing - title to be defined
- Topic related to LLMOps - title to be defined
- Topic related to Model fraud monitoring - title to be defined
- Topic related to Optimization - title to be defined
- Topic related to Quantum computing - title to be defined
- Topic related to Sustainable AI - title to be defined
- Towards AI-agents self-healing supply chain
- Using remote sensing and sensor fusion to predict geospatial yield prior to harvest
Theses proposed by the Department of Computer Science
- A position and orientation-independent framework for human activity recognition using semi-supervised and transfer learning
- Approximate Knowledge Compilation for probabilistic reasoning
- Comparing FO(.) to ErgoAI
- X Detecting adversarial examples in neural network ensembles
- X FitBEAT – towards a cardiorespiratory fitness score for heart failure and chronic obstructive pulmonary disease using machine learning
- X From Binary Decisions to Sentences Extending variable ordering heuristics
- Symmetric Component Caching for Knowledge Compilation
- Case study in linguistically informed interpretability
- Case study in NLP for truly low-resource languages
- Continuous Style Control with Diffusion Models
- X Fostering a Holistic Understanding of Patients Afflicted by Amyotrophic Lateral Sclerosis through Person-Centric Graph Extraction
- Investigating the Inductive Biases of Linear Attention Models
- MuZero for dialog planning
- Pretrained Language Models for Code-Mixed Languages
Theses proposed by the Department of Electrical Engineering
- Industrial IoT Device Identification Using Reinforcement Learning
- X Predicting student grades enforcing privacy
- Privacy-preserving Federated Learning
- X Using AI to Attack the Blockchain
- X Deep learning for point and interval forecasts for electricity demand and prices
- Estimating calendar effects to detect operational issues in commercial buildings
- X GIS-enabled predictions for whole building energy demand and generation
- Safety for autonomous systems with machine learning
- Hierarchical transfer learning of surrogate model in mixed-signal circuit design
- Novel MEMS Devices Design with Machine Learning Algorithm
- Topology Optimization of MEMS devices for 5G with Machine Learning Algorithm
- X 3D facial growth curves using geometric deep learning
- X A deep learning approach for automatic statistical shape modelling from MRI images
- Accelerating reflection-aware neural radiance fields in the context of shiny objects
- X Autoencoder-based outlier detection of cardiac CT scans
- X Automatic Linguistic and Acoustic Analysis of Aphasia through Spontaneous Speech
- Bayesian Optimization for Hyperparameter Tuning Language Model
- CT reconstruction and image artifact removal based on neural network
- Estimating specular regions through multi-view appearance inconsistencies
- High-resolution Surface Smoothness Estimation and Quality Measurement
- Image Simulator to Model Shotcrete Deposition Process
- Join forces with the EMA (European Medicines Agency) The automation of data extraction and trend analysis of the pharmaceutical pipeline in Europe
- Learning Robust Shotcreting Policies in Dusty Conditions from Demonstration
- X Leveraging Active Learning and Semantic Segmentation for Early Detection of Construction Violations Near Public Waterways
- X Leveraging car-part segmentation masks to improve material estimation in NeRFbased
- Mars cartography From Orbital Data to Subpixel Surface Features
- Self, semi and fully supervised learning for anomaly detection
- [NEW] Self-supervised learning for histopathology using the Joint Embedding Predictive Architecture
- X Statistical shape modelling of 3D facial scans using graph neural networks
- Transforming a metric map to a topological map for Vision-Language Navigation
- Uncertainty Calibration in Active Learning
- Uncertainty Calibration under Dataset Shift
- Anomaly Detection with Deep Neural Networks using deep features calibrated with Stiefel-manifold Kernel Machines
- Clinical notes summarization using pre-trained models and large language models for length of stay prediction
- Communication-efficient Federated Kernel Learning
- X Comparison of advanced model architectures for end-to-end reinforcement learning applied to autonomous highway driving
- X Deep kernel learning for graphs extending Graph Convolutional Kernel Machines from convolutions to message passing
- X Early disease detection based on shallow whole genome sequencing (sWGS) of cell-free DNA (cfDNA) using federated learning
- Efficient Training of Restricted Kernel Machines via Dual Minibatching
- Generative models for gene regulatory network inference from single-cell RNA-seq data
- X Graph Representation Learning for Medical Vision
- Machine learning algorithms to detect and quantify stair climbing performance using accelerometry
- Multi-omic modeling of cell-free DNA samples with fragment-level kernel functions
- X Multi-view spectral clustering for unsupervised object discovery via Transformers
- X Neural correlates of auditory-motor coupling during walking to music and metronomes at different tempi in persons with cerebellar impairment and healthy controls
- Prediction of premature ventricular beats (PVB) and ablation location for paroxysmal supraventricular tachycardia based on electrocardiograms
WaveCORE, Networked Systems
- Detecting Cyber Attacks from the Energy Consumption of Internet of Things Networks
- Neural Networks for Green Next-Generation Communication Networks
Theses proposed by the Computational Neuroscience Research Group
- X Can vibrotactile stimulation facilitate finger movement imagery decoding from EEG?
- Decoding finger movements from non-invasive brain electrical signals via few-shot learning
- Neural correlates of auditory-motor coupling during walking to music and metronomes at different tempi in persons with cerebellar impairment and healthy controls
- Towards Future AR BCI Application Select Anything Using Your Mind
- Track dexterous finger movements via semi-invasive brain electrical signals and AI
- Unlocking Conversations in Virtual Reality Integrating Advanced Language Models with Traditional Brain-Computer Interfaces and Speech Synthesis
Theses proposed by the Centre for Computational Linguistics -- Faculty of Arts
- X Automated rating of Exams Dutch as a Foreign Language
- Automatical (verification of) dating of Dutch historical documents
- X Detection of Code Switching Points for Speech Recognition in a Video Corpus
- Enriching Open Dutch Wordnet through Knowledge Graph Completion
- Linguistic enrichment of historical Dutch using deep learning
- X Performing Low Resource Text Simplification for Dutch
Theses proposed by the Department of Mechanical Engineering
Students are welcome to contact prof. Herman Bruyninckx with a suggestion of their own, as long as it focusses on the integration of explicit formal "knowledge" with the sensor-based control of robots.
- X Context-Aware Intention Recognition for Shared-Control Grasping
- X Enhancing Multi Robot Assembly Skills through Computer Vision
- Epistemic Reinforcement Learning and application in Robotic
- Evaluate if the cost of deliberate destructive tests of machines for the sake of supervised training data can be justified
- Generative AI for vibro-acoustics examining the effect of pointwise reconstruction losses
- Imitation learning applied to an autonomous driving challenge
- X Self supervised learning for detecting machine faults
Theses proposed by Philosophy
Contemporary issues in Deontic Logic
Additionally, students are welcome to contact dr. Stef Frijters with a suggestion of their own, as long as it focusses on the use of formal logic within the domain of AI or on one of the following topics
- Epistemic logic
- Dynamic logic
- Bitstring semantics
- Temporal logic
- Formal semantics
Students are also welcome to contact prof. L ode Lauwaert with a suggestion of their own, as long as it focusses on one of the following topics
- Machine consciousness
- AI and the responsibility gap
- Singularity and AI
- Fairness in AI
- Agency of AI systems
- Value alignment
- Machine ethics
- Ethical algorithms
- Value by design
- AI and Existential Risks
Students are welcome to contact prof. Lorenz Demey to discuss potential thesis topics, which should fall withing the broad scope of formal logic and its applications in AI. Alghough any proposals are welcome, areas that are of particular interest include:
- Logical geometry (diagrammatic reasoning)
- epistemic logic
- deontic logic
- temporal logic
- dynamic logic
- digital topology
- formal semantics of natural language
Depending on the specific topic, the thesis project will be carried out in close collaboration with dr. Stef Frijters and/or drs. Alexander De Klerck as daily advisor. For a specific example, consider the proposal form "Contemporary Issues in Deontic Logic"
Thesis with prof. Demey are expected to be entirely theoretical in nature. The student is required to have a good understanding of classifcal logic (i.e., propositional logic and first-order/predicate logic). Some working knowledge of other logics (in particular, modal logic) will be useful, but can also acquired during the course of the thesis project, if necessary. For some of the aforementioned topics, a strong background in discrete mathematics (algebra, graph theory, combinatorics) will be a big plus as well.
OTHER THESIS TOPICS
Natural sciences.
Topics proposed by
Dept. of Astronomy
- X Finding exoplanets with the power of machine learning
- X Revealing faint signals from Supermassive Black Hole Binaries using Deep Neural Networks
- X Unsupervised machine learning to probe the internal physics of stars from asteroseismology
Cell Stress & Immunity laboratory
- X Recognition of immune communities in tissue images using spatial gene profiling
Dept. of Development and Regeneration
- [NEW] Quantitative Comparison of Deep Learning Segmentation Performance between Ultrasound and MRI Modalities for Medical Imaging Analysis
Dept. of Earth and Environmental Sciences - Forest, Nature & Landscape
- X Deep learning to model species distributions
- X Deepfaking ecosystem response to climate extremes
- X Emulating ice sheet models using graph networks
- Physics-Constrained Deep Learning for Downscaling Climate Data
- X Superresolution for spatiotemporally continuous rain-use efficiency
- Chicken call detector using machine learning techniques
- Chicken health monitoring by sneeze detection in noisy environments using deep learning
- Few-Shot Bird calls detection and classification as a pre-processing step for animal welfare monitoring through vocalization
- Multistate detection from physiological data: Development of a Stress Tolerance Zone for Occupational Drivers
Dept. of Mathematics - Plasma-astrophysics
- X Autoencoding of solar images and generation of artificial active regions
- Discovering physical laws from computer simulations of plasmas using machine learning
- X Unsupervised ML Classification of velocity distributions in space
Social Sciences
the laboratory of Experimental Psychology
- X AI-assisted drawing style conversion for comics - a deep learning approach
- Predicting aesthetic image assessment by Bayesian Hypernetwork
- X Towards human-like visual perception in AI Investigating Gestalt laws in deep convolutional neural networks
Faculty of Economics and Business - LIRIS
- X A Benchmark of Network Learning Techniques
- Enhancing the reliability of global neural point forecasting models
- X Market index prediction based on graph transformer
- X Pairs trading based on predictive analytics with feature selection
Public Governance Institute
- AI and Public Governance
Department of Movement Science, KU Leuven
- Estimation of ambulatory knee joint loading parameters from gait kinematics and subject anthropometrics
UZLeuven - the department of Laboratory Medicine
- X Classification of urinary sediment particles based on automatic microscope images
Propose your own thesis topic or company project
Students with other interests than the topics proposed by the programme can propose their own topic.
- Thesis topic proposal (Format 1 and 2) : The student can take contact with a lecturer of whom they expect that the topic lies in the expertise of this lecturer, or they can contact [email protected] to get help on finding a potential promotor for the topic. Typically, if a lecturer can be found who is interested in guiding the thesis topic, this will still require some discussion to polish the proposal, in order for it to reach a number of criteria (achievable goals, sufficient research questions, manageable, within the interests and expertise of the lecturer, sufficient AI dimension). If you found a promotor, you can fill out the following template and send it to [email protected] In case your proposal is in cooperation with a company, you can use this template and send it to [email protected]
- Company project proposal (Format 3) : The student can take contact with a company of their interest. Together with the company, you can fill in the project template and send it to [email protected]. If the proposed project is positively evaluated, an academic promotor will be sought by the program director. Please find the "requirements for the company" here . ! It is not allowed for working students to do a company project nor a thesis within their own company.
- Data Science
- Data Analysis
- Data Visualization
- Machine Learning
- Deep Learning
- Computer Vision
- Artificial Intelligence
- AI ML DS Interview Series
- AI ML DS Projects series
- Data Engineering
- Web Scrapping
8 Best Topics for Research and Thesis in Artificial Intelligence
Imagine a future in which intelligence is not restricted to humans!!! A future where machines can think as well as humans and work with them to create an even more exciting universe. While this future is still far away, Artificial Intelligence has still made a lot of advancement in these times. There is a lot of research being conducted in almost all fields of AI like Quantum Computing, Healthcare, Autonomous Vehicles, Internet of Things , Robotics , etc. So much so that there is an increase of 90% in the number of annually published research papers on Artificial Intelligence since 1996.
Keeping this in mind, if you want to research and write a thesis based on Artificial Intelligence, there are many sub-topics that you can focus on. Some of these topics along with a brief introduction are provided in this article. We have also mentioned some published research papers related to each of these topics so that you can better understand the research process.
Table of Content
1. Machine Learning
2. deep learning, 3. reinforcement learning, 4. robotics, 5. natural language processing (nlp), 6. computer vision, 7. recommender systems, 8. internet of things.
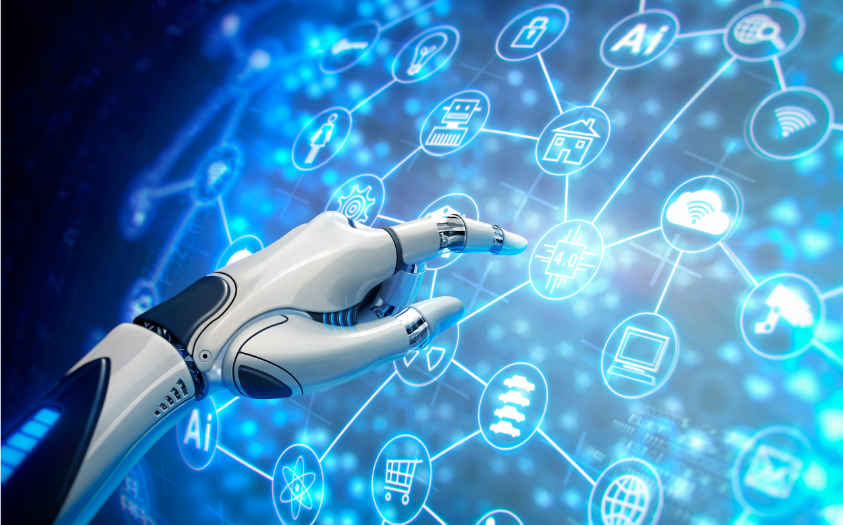
So without further ado, let’s see the different Topics for Research and Thesis in Artificial Intelligence!
Machine Learning involves the use of Artificial Intelligence to enable machines to learn a task from experience without programming them specifically about that task. (In short, Machines learn automatically without human hand holding!!!) This process starts with feeding them good quality data and then training the machines by building various machine learning models using the data and different algorithms. The choice of algorithms depends on what type of data do we have and what kind of task we are trying to automate.
However, generally speaking, Machine Learning Algorithms are generally divided into 3 types: Supervised Machine Learning Algorithms , Unsupervised Machine Learning Algorithms , and Reinforcement Machine Learning Algorithms . If you are interested in gaining practical experience and understanding these algorithms in-depth, check out the Data Science Live Course by us.
Deep Learning is a subset of Machine Learning that learns by imitating the inner working of the human brain in order to process data and implement decisions based on that data. Basically, Deep Learning uses artificial neural networks to implement machine learning. These neural networks are connected in a web-like structure like the networks in the human brain (Basically a simplified version of our brain!).
This web-like structure of artificial neural networks means that they are able to process data in a nonlinear approach which is a significant advantage over traditional algorithms that can only process data in a linear approach. An example of a deep neural network is RankBrain which is one of the factors in the Google Search algorithm.
Reinforcement Learning is a part of Artificial Intelligence in which the machine learns something in a way that is similar to how humans learn. As an example, assume that the machine is a student. Here the hypothetical student learns from its own mistakes over time (like we had to!!). So the Reinforcement Machine Learning Algorithms learn optimal actions through trial and error.
This means that the algorithm decides the next action by learning behaviors that are based on its current state and that will maximize the reward in the future. And like humans, this works for machines as well! For example, Google’s AlphaGo computer program was able to beat the world champion in the game of Go (that’s a human!) in 2017 using Reinforcement Learning.
Robotics is a field that deals with creating humanoid machines that can behave like humans and perform some actions like human beings. Now, robots can act like humans in certain situations but can they think like humans as well? This is where artificial intelligence comes in! AI allows robots to act intelligently in certain situations. These robots may be able to solve problems in a limited sphere or even learn in controlled environments.
An example of this is Kismet , which is a social interaction robot developed at M.I.T’s Artificial Intelligence Lab. It recognizes the human body language and also our voice and interacts with humans accordingly. Another example is Robonaut , which was developed by NASA to work alongside the astronauts in space.
It’s obvious that humans can converse with each other using speech but now machines can too! This is known as Natural Language Processing where machines analyze and understand language and speech as it is spoken (Now if you talk to a machine it may just talk back!). There are many subparts of NLP that deal with language such as speech recognition, natural language generation, natural language translation , etc. NLP is currently extremely popular for customer support applications, particularly the chatbot . These chatbots use ML and NLP to interact with the users in textual form and solve their queries. So you get the human touch in your customer support interactions without ever directly interacting with a human.
Some Research Papers published in the field of Natural Language Processing are provided here. You can study them to get more ideas about research and thesis on this topic.
The internet is full of images! This is the selfie age, where taking an image and sharing it has never been easier. In fact, millions of images are uploaded and viewed every day on the internet. To make the most use of this huge amount of images online, it’s important that computers can see and understand images. And while humans can do this easily without a thought, it’s not so easy for computers! This is where Computer Vision comes in.
Computer Vision uses Artificial Intelligence to extract information from images. This information can be object detection in the image, identification of image content to group various images together, etc. An application of computer vision is navigation for autonomous vehicles by analyzing images of surroundings such as AutoNav used in the Spirit and Opportunity rovers which landed on Mars.
When you are using Netflix, do you get a recommendation of movies and series based on your past choices or genres you like? This is done by Recommender Systems that provide you some guidance on what to choose next among the vast choices available online. A Recommender System can be based on Content-based Recommendation or even Collaborative Filtering.
Content-Based Recommendation is done by analyzing the content of all the items. For example, you can be recommended books you might like based on Natural Language Processing done on the books. On the other hand, Collaborative Filtering is done by analyzing your past reading behavior and then recommending books based on that.
Artificial Intelligence deals with the creation of systems that can learn to emulate human tasks using their prior experience and without any manual intervention. Internet of Things , on the other hand, is a network of various devices that are connected over the internet and they can collect and exchange data with each other.
Now, all these IoT devices generate a lot of data that needs to be collected and mined for actionable results. This is where Artificial Intelligence comes into the picture. Internet of Things is used to collect and handle the huge amount of data that is required by the Artificial Intelligence algorithms. In turn, these algorithms convert the data into useful actionable results that can be implemented by the IoT devices.
Please Login to comment...
Similar reads.
- AI-ML-DS Blogs
- How to Get a Free SSL Certificate
- Best SSL Certificates Provider in India
- Elon Musk's xAI releases Grok-2 AI assistant
- What is OpenAI SearchGPT? How it works and How to Get it?
- Content Improvement League 2024: From Good To A Great Article
Improve your Coding Skills with Practice
What kind of Experience do you want to share?
Information
- Author Services
Initiatives
You are accessing a machine-readable page. In order to be human-readable, please install an RSS reader.
All articles published by MDPI are made immediately available worldwide under an open access license. No special permission is required to reuse all or part of the article published by MDPI, including figures and tables. For articles published under an open access Creative Common CC BY license, any part of the article may be reused without permission provided that the original article is clearly cited. For more information, please refer to https://www.mdpi.com/openaccess .
Feature papers represent the most advanced research with significant potential for high impact in the field. A Feature Paper should be a substantial original Article that involves several techniques or approaches, provides an outlook for future research directions and describes possible research applications.
Feature papers are submitted upon individual invitation or recommendation by the scientific editors and must receive positive feedback from the reviewers.
Editor’s Choice articles are based on recommendations by the scientific editors of MDPI journals from around the world. Editors select a small number of articles recently published in the journal that they believe will be particularly interesting to readers, or important in the respective research area. The aim is to provide a snapshot of some of the most exciting work published in the various research areas of the journal.
Original Submission Date Received: .
- Active Journals
- Find a Journal
- Proceedings Series
- For Authors
- For Reviewers
- For Editors
- For Librarians
- For Publishers
- For Societies
- For Conference Organizers
- Open Access Policy
- Institutional Open Access Program
- Special Issues Guidelines
- Editorial Process
- Research and Publication Ethics
- Article Processing Charges
- Testimonials
- Preprints.org
- SciProfiles
- Encyclopedia
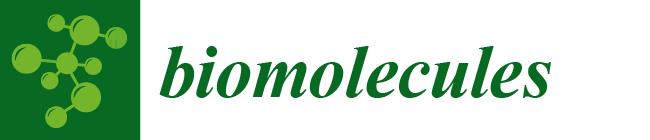
Article Menu

- Subscribe SciFeed
- Recommended Articles
- Google Scholar
- on Google Scholar
- Table of Contents
Find support for a specific problem in the support section of our website.
Please let us know what you think of our products and services.
Visit our dedicated information section to learn more about MDPI.
JSmol Viewer
Drpred: a novel deep learning-based predictor for multi-label mrna subcellular localization prediction by incorporating bayesian inferred prior label relationships.

1. Introduction
2. materials and methods, 2.1. datasets, 2.1.1. mrna sequences distribution and nucleotide composition analysis, 2.1.2. label correlation analysis, 2.2. network framework of drpred, 2.3. feature extraction, 2.4. extraction of label relationships, 2.5. multi-label prediction, 2.6. bidirectional long short-term memory, 2.7. performance evaluation metrics, 3. results and discussion, 3.1. comparison of different sequence coding schemes, 3.2. comparison of different network architectures, 3.3. performance comparison with existing state-of-the-art predictors, 4. conclusions, author contributions, institutional review board statement, informed consent statement, data availability statement, conflicts of interest.
- Aken, B.L.; Achuthan, P.; Akanni, W. Ensembl 2017. Nucleic Acids Res 2017 , 45 , D635–D642. [ Google Scholar ] [ CrossRef ] [ PubMed ]
- Meyer, C.; Garzia, A.; Tuschl, T. Simultaneous detection of the subcellular localization of RNAs and proteins in cultured cells by combined multicolor RNA-FISH and IF. Methods 2017 , 118–119 , 101–110. [ Google Scholar ] [ CrossRef ]
- Martin, K.C.; Ephrussi, A. mRNA localization: Gene expression in the spatial dimension. Cell 2009 , 136 , 719–730. [ Google Scholar ] [ CrossRef ]
- Tong, C.; Yin, Y. Localization of RNAs in the nucleus: Cis- and trans- regulation. RNA Biol. 2021 , 18 , 2073–2086. [ Google Scholar ] [ CrossRef ] [ PubMed ]
- Li, J.; Zou, Q.; Yuan, L. A review from biological mapping to computation-based subcellular localization. Mol. Ther. Nucleic Acids 2023 , 32 , 507–521. [ Google Scholar ] [ CrossRef ] [ PubMed ]
- Deng, J.; Xu, W.; Jie, Y.; Chong, Y. Subcellular localization and relevant mechanisms of human cancer-related micropeptides. FASEB J. 2023 , 37 , e23270. [ Google Scholar ] [ CrossRef ]
- Jung, H.; Gkogkas, C.G.; Sonenberg, N.; Holt, C.E. Remote control of gene function by local translation. Cell 2014 , 157 , 26–40. [ Google Scholar ] [ CrossRef ]
- Di Liegro, C.M.; Schiera, G.; Di Liegro, I. Regulation of mRNA transport, localization and translation in the nervous system of mammals (Review). Int. J. Mol. Med. 2014 , 33 , 747–762. [ Google Scholar ] [ CrossRef ]
- Bourke, A.M.; Schwarz, A.; Schuman, E.M. De-centralizing the Central Dogma: mRNA translation in space and time. Mol. Cell 2023 , 83 , 452–468. [ Google Scholar ] [ CrossRef ]
- Macdonald, P.M. mRNA localization: Assembly of transport complexes and their incorporation into particles. Curr. Opin. Genet. Dev. 2011 , 21 , 407–413. [ Google Scholar ] [ CrossRef ]
- Tian, L.; Chou, H.L.; Fukuda, M.; Kumamaru, T.; Okita, T.W. mRNA Localization in Plant Cells. Plant Physiol. 2020 , 182 , 97–109. [ Google Scholar ] [ CrossRef ]
- Medioni, C.; Mowry, K.; Besse, F. Principles and roles of mRNA localization in animal development. Development 2012 , 139 , 3263–3276. [ Google Scholar ] [ CrossRef ]
- Liu, D.; Li, G.; Zuo, Y. Function determinants of TET proteins: The arrangements of sequence motifs with specific codes. Brief. Bioinform. 2019 , 20 , 1826–1835. [ Google Scholar ] [ CrossRef ]
- Herbert, S.P.; Costa, G. Sending messages in moving cells: mRNA localization and the regulation of cell migration. Essays Biochem. 2019 , 63 , 595–606. [ Google Scholar ] [ PubMed ]
- Liu-Yesucevitz, L.; Bassell, G.J.; Gitler, A.D.; Hart, A.C.; Klann, E.; Richter, J.D.; Warren, S.T.; Wolozin, B. Local RNA translation at the synapse and in disease. J. Neurosci. 2011 , 3 , 16086–16093. [ Google Scholar ] [ CrossRef ]
- Cooper, T.A.; Wan, L.; Dreyfuss, G. RNA and disease. Cell 2009 , 13 , 777–793. [ Google Scholar ] [ CrossRef ] [ PubMed ]
- Cagnetta, R.; Flanagan, J.G.; Sonenberg, N. Control of Selective mRNA Translation in Neuronal Subcellular Compartments in Health and Disease. J. Neurosci. 2023 , 43 , 7247–7263. [ Google Scholar ] [ CrossRef ] [ PubMed ]
- Otis, J.P.; Mowry, K.L. Hitting the mark: Localization of mRNA and biomolecular condensates in health and disease. Wiley Interdiscip. Rev. RNA 2023 , 14 , e1807. [ Google Scholar ] [ CrossRef ] [ PubMed ]
- LaForce, G.R.; Philippidou, P.; Schaffer, A.E. mRNA isoform balance in neuronal development and disease. Wiley Interdiscip. Rev. RNA 2023 , 14 , e1762. [ Google Scholar ] [ CrossRef ]
- Lawrence, J.B.; Singer, R.H. Intracellular localization of messenger RNAs for cytoskeletal proteins. Cell 1986 , 45 , 407–415. [ Google Scholar ] [ CrossRef ]
- Le, P.; Ahmed, N.; Yeo, G.W. Illuminating RNA biology through imaging. Nat. Cell Biol. 2022 , 24 , 815–824. [ Google Scholar ] [ CrossRef ] [ PubMed ]
- Jiang, J. Fluorescence in situ hybridization in plants: Recent developments and future application. Chromosome Res. 2019 , 27 , 153–165. [ Google Scholar ] [ CrossRef ]
- Xia, C.; Fan, J.; Emanuel, G.; Hao, J.; Zhuang, X. Spatial transcriptome profiling by MERFISH reveals subcellular RNA compartmentalization and cell cycle-dependent gene expression. Proc. Natl. Acad. Sci. USA 2019 , 116 , 19490–19499. [ Google Scholar ] [ CrossRef ]
- Lefebvre, F.A.; Cody, N.A.L.; Bouvrette, L.P.B. CeFra-seq: Systematic mapping of RNA subcellular distribution properties through cell fractionation coupled to deep-sequencing. Methods 2017 , 126 , 138–148. [ Google Scholar ] [ CrossRef ]
- Kaewsapsak, P.; Shechner, D.M.; Mallard, W.; Rinn, J.L.; Ting, A.Y. Live-cell mapping of organelle-associated RNAs via proximity biotinylation combined with protein-RNA crosslinking. Elife 2017 , 6 , e29224. [ Google Scholar ] [ CrossRef ]
- Parton, R.M.; Davidson, A.; Davis, I.; Weil, T.T. Subcellular mRNA localisation at a glance. J. Cell Sci. 2014 , 127 , 2127–2133. [ Google Scholar ] [ CrossRef ]
- Buxbaum, A.R.; Wu, B.; Singer, R.H. Single β-actin mRNA detection in neurons reveals a mechanism for regulating its translatability. Science 2014 , 343 , 419–422. [ Google Scholar ] [ CrossRef ] [ PubMed ]
- Wu, B.; Chao, J.A.; Singer, R.H. Fluorescence fluctuation spectroscopy enables quantitative imaging of single mRNAs in living cells. Biophys. J. 2012 , 102 , 2936–2944. [ Google Scholar ] [ CrossRef ] [ PubMed ]
- Fagerberg, L.; Hallström, B.M.; Oksvold, P.; Kampf, C.; Djureinovic, D.; Odeberg, J.; Habuka, M.; Tahmasebpoor, S.; Danielsson, A.; Edlund, K.; et al. Analysis of the human tissue-specific expression by genome-wide integration of transcriptomics and antibody-based proteomics. Mol. Cell Proteom. 2014 , 13 , 397–406. [ Google Scholar ] [ CrossRef ]
- Fazal, F.M.; Han, S.; Parker, K.R.; Kaewsapsak, P.; Xu, J.; Boettiger, A.N.; Chang, H.Y.; Ting, A.Y. Atlas of Subcellular RNA Localization Revealed by APEX-Seq. Cell 2019 , 178 , 473–490.e26. [ Google Scholar ] [ CrossRef ]
- Giassa, I.C.; Alexiou, P. Bioinformatics and Machine Learning Approaches to Understand the Regulation of Mobile Genetic Elements. Biology 2021 , 10 , 896. [ Google Scholar ] [ CrossRef ]
- Jianrong, Y.; Min, Z. A Review About RNA-Protein-Binding Sites Prediction Based on Deep Learning. IEEE Access 2020 , 8 , 150929–150944. [ Google Scholar ]
- Yan, Z.; Lécuyer, E.; Blanchette, M. Prediction of mRNA subcellular localization using deep recurrent neural networks. Bioinformatics 2019 , 35 , i333–i342. [ Google Scholar ] [ CrossRef ]
- Garg, A.; Singhal, N.; Kumar, R.; Kumar, M. mRNALoc: A novel machine-learning based in-silico tool to predict mRNA subcellular localization. Nucleic Acids Res. 2020 , 48 , W239–W243. [ Google Scholar ] [ CrossRef ] [ PubMed ]
- Li, J.; Zhang, L.; He, S.; Guo, F.; Zou, Q. SubLocEP: A novel ensemble predictor of subcellular localization of eukaryotic mRNA based on machine learning. Brief. Bioinform. 2021 , 22 , bbaa401. [ Google Scholar ] [ CrossRef ]
- Tang, Q.; Nie, F.; Kang, J.; Chen, W. mRNALocater: Enhance the prediction accuracy of eukaryotic mRNA subcellular localization by using model fusion strategy. Mol. Ther. 2021 , 29 , 2617–2623. [ Google Scholar ] [ CrossRef ]
- Musleh, S.; Islam, M.T.; Qureshi, R.; Alajez, N.M.; Alam, T. MSLP: mRNA subcellular localization predictor based on machine learning techniques. BMC Bioinform. 2023 , 24 , 109. [ Google Scholar ]
- Zhang, Z.; Yang, Y.; Ding, H.; Wang, D.; Chen, W.; Lin, H. Design powerful predictor for mRNA subcellular location prediction in Homo sapiens. Brief. Bioinform. 2021 , 22 , 526–535. [ Google Scholar ] [ CrossRef ]
- Wang, D.; Zhang, Z.; Jiang, Y.; Mao, Z.; Wang, D.; Lin, H.; Xu, D. DM3Loc: Multi-label mRNA subcellular localization prediction and analysis based on multi-head self-attention mechanism. Nucleic Acids Res. 2021 , 49 , e46. [ Google Scholar ] [ CrossRef ] [ PubMed ]
- Bi, Y.; Li, F.; Guo, X.; Wang, Z.; Pan, T.; Guo, Y.; Webb, I.G.; Yao, J.; Jia, C.; Song, J. Clarion is a multi-label problem transformation method for identifying mRNA subcellular localizations. Brief. Bioinform. 2022 , 23 , bbac467. [ Google Scholar ] [ CrossRef ]
- Choudhury, S.; Bajiya, N.; Patiyal, S.; Raghava, G.P.S. MRSLpred—A hybrid approach for predicting multi-label subcellular localization of mRNA at the genome scale. Front. Bioinform. 2024 , 4 , 1341479. [ Google Scholar ] [ CrossRef ] [ PubMed ]
- Wang, H.; Ding, Y.; Tang, J.; Zou, Q.; Guo, F. Identify RNA-associated subcellular localizations based on multi-label learning using Chou’s 5-steps rule. BMC Genom. 2021 , 22 , 56. [ Google Scholar ] [ CrossRef ] [ PubMed ]
- Blasco, T.; Balzerani, F.; Valcárcel, L.V.; Larrañaga, P.; Bielza, C.; Francino, M.P.; Rufián-Henares, J.; Planes, F.J.; Pérez-Burillo, S. BN-BacArena: Bayesian network extension of BacArena for the dynamic simulation of microbial communities. Bioinformatics 2024 , 40 , btae266. [ Google Scholar ] [ CrossRef ] [ PubMed ]
- Ren, R.; Yin, C.; S-T Yau, S. kmer2vec: A Novel Method for Comparing DNA Sequences by word2vec Embedding. J. Comput. Biol. 2022 , 29 , 1001–1021. [ Google Scholar ] [ CrossRef ]
- Wan, C.; Jones, D.T. Protein function prediction is improved by creating synthetic feature samples with generative adversarial networks. Nat. Mach. Intell. 2020 , 2 , 540–550. [ Google Scholar ] [ CrossRef ]
Click here to enlarge figure
Subcellular Localization | Total | Training-Validation Set | Independent Test Set |
---|---|---|---|
Exosome | 31,448 | 28,304 | 3144 |
Nucleus | 21,439 | 19,301 | 2138 |
Nucleoplasm | 14,237 | 12,807 | 1430 |
Chromatin | 14,328 | 12,893 | 1435 |
Cytoplasm | 4016 | 3597 | 419 |
Nucleolus | 11,124 | 10,000 | 1124 |
Cytosol | 16,312 | 14,686 | 1626 |
Membrane | 6739 | 6047 | 692 |
Ribosome | 8680 | 7796 | 884 |
Subcellular Localization | Exosome | Nucleus | Nucleoplasm | Chromatin | Nucleolus | Membrane | Ribosome | Cytosol | Cytoplasm |
---|---|---|---|---|---|---|---|---|---|
Marginal Probability | 0.188 | 0.153 | 0.128 | 0.129 | 0.104 | 0.065 | 0.075 | 0.137 | 0.020 |
K-mer | Word2vec (k = 1) | Word2vec (k = 2) | Word2vec (k = 3) | |
---|---|---|---|---|
Acc | 0.649 | 0.654 | 0.662 | 0.667 |
Average Precision | 0.711 | 0.717 | 0.722 | 0.725 |
Coverage | 3.900 | 3.840 | 3.771 | 3.730 |
One-error | 0.556 | 0.550 | 0.544 | 0.536 |
Ranking Loss | 0.318 | 0.311 | 0.304 | 0.299 |
Hamming Loss | 0.175 | 0.173 | 0.170 | 0.169 |
LSTM | LSTM + Attention | BiLSTM + Attention | |
---|---|---|---|
Acc | 0.671 | 0.684 | 0.703 |
Average Precision | 0.727 | 0.739 | 0.753 |
Coverage | 3.690 | 3.624 | 3.477 |
One-error | 0.531 | 0.521 | 0.509 |
Ranking Loss | 0.293 | 0.261 | 0.239 |
Hamming Loss | 0.167 | 0.162 | 0.154 |
DM3Loc | MRSLpred | Clarion | DRpred | |
---|---|---|---|---|
Acc | 0.441 | 0.642 | 0.722 | 0.731 |
Average Precision | 0.618 | 0.712 | 0.769 | 0.771 |
Coverage | 3.957 | 3.736 | 3.019 | 2.998 |
One-error | 0.869 | 0.654 | 0.463 | 0.451 |
Ranking Loss | 0.533 | 0.423 | 0.204 | 0.182 |
Hamming Loss | 0.330 | 0.268 | 0.150 | 0.098 |
Wang’s | Clarion | DRpred | |
---|---|---|---|
Acc | 0.281 | 0.745 | 0.752 |
Average Precision | 0.679 | 0.767 | 0.767 |
Coverage | 4.516 | 3.127 | 3.069 |
One-error | 0.882 | 0.445 | 0.447 |
Ranking Loss | 0.716 | 0.241 | 0.194 |
Hamming Loss | 0.375 | 0.146 | 0.097 |
iLoc-mRNA | mRNALoc | mRNALocator | MSLP | DRpred | |
---|---|---|---|---|---|
Chromatin | - | - | - | - | 82.14% |
Cytoplasm | - | 54.88% | 38.90% | 84.05% | 93.02% |
Cytosol | - | - | - | 78.50% | 80.37% |
Exosome | - | - | - | 80.00% | 94.00% |
Membrane | - | - | - | - | 90.58% |
Nucleolus | - | - | - | - | 84.53% |
Nucleoplasm | - | - | - | - | 82.01% |
Nucleus | - | 55.18% | 57.42% | 85.40% | 79.71% |
Ribosome | 73.41% | - | - | 79.30% | 85.67% |
The statements, opinions and data contained in all publications are solely those of the individual author(s) and contributor(s) and not of MDPI and/or the editor(s). MDPI and/or the editor(s) disclaim responsibility for any injury to people or property resulting from any ideas, methods, instructions or products referred to in the content. |
Share and Cite
Wang, X.; Yang, L.; Wang, R. DRpred: A Novel Deep Learning-Based Predictor for Multi-Label mRNA Subcellular Localization Prediction by Incorporating Bayesian Inferred Prior Label Relationships. Biomolecules 2024 , 14 , 1067. https://doi.org/10.3390/biom14091067
Wang X, Yang L, Wang R. DRpred: A Novel Deep Learning-Based Predictor for Multi-Label mRNA Subcellular Localization Prediction by Incorporating Bayesian Inferred Prior Label Relationships. Biomolecules . 2024; 14(9):1067. https://doi.org/10.3390/biom14091067
Wang, Xiao, Lixiang Yang, and Rong Wang. 2024. "DRpred: A Novel Deep Learning-Based Predictor for Multi-Label mRNA Subcellular Localization Prediction by Incorporating Bayesian Inferred Prior Label Relationships" Biomolecules 14, no. 9: 1067. https://doi.org/10.3390/biom14091067
Article Metrics
Article access statistics, further information, mdpi initiatives, follow mdpi.
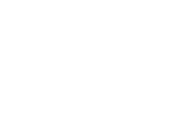
Subscribe to receive issue release notifications and newsletters from MDPI journals

IMAGES
COMMENTS
Deep learning models have been used for this task, and traditional methods such as clustering and rule-based systems are widely used as well. This thesis aims to compare deep learning models with traditional algorithms for anomaly detection in network traffic and analyze the trade-offs between the models in terms of accuracy and scalability. 19.
A comprehensive list of research topics ideas in the AI and machine learning area. Includes access to a free webinar and topic evaluator. ... If you're just starting out exploring AI-related research topics for your dissertation, thesis or research project, you've come to the ... The use of deep learning in enhancing the accuracy of weather ...
3. Developing a deep learning model to detect and classify objects in 3D scenes. 4. Developing a deep learning model to detect and classify objects in audio. 5. Developing a deep learning model to detect and classify objects in text. 6. Develop a deep learning model to generate new images from a given set of images. 7.
Potential thesis topics in this area: a) Compare inference speed with sum-product networks and Bayesian networks. Characterize situations when one model is better than the other. ... Deep learning is usually applied in regression or classification problems. However, there has been some recent work on using deep learning to develop heuristics ...
The importance of this research can be summarized as follows: Advancements in Technology. The growth of machine learning research has led to the development of powerful algorithms, tools, and frameworks. Numerous industries, including healthcare, banking, autonomous cars, and natural language processing, have found use for these technology.
Before diving into the millions of options for research papers for specific topics, I recommend checking these out to gain further exposure to the subject of deep learning. If you want to get notified about my articles as soon as they go up, check out the following link to subscribe for email recommendations.
If you can find a dissertation that aligns with your areas of interest, consuming the research is an excellent way to do a deep dive into the technology. After reviewing hundreds of recent theses from universities all over the country, I present 10 machine learning dissertations that I found compelling in terms of my own areas of interest.
The new model family introduced in this thesis is summarized under the term Recursive Deep Learning. The models in this family are variations and extensions of unsupervised and supervised recursive neural networks (RNNs) which generalize deep and feature learning ideas to hierarchical structures. The RNN models of this thesis
LasseRegin / master-thesis-deep-learning Star 18. Code Issues Pull requests Code for my master thesis in Deep Learning: "Generating answers to medical questions using recurrent neural networks" ... Add a description, image, and links to the master-thesis topic page so that developers can more easily learn about it. Curate this topic Add this ...
The goal of our research is to develop methods advancing automatic visual recognition. In order to predict the unique or multiple labels associated to an image, we study different kind of Deep Neural Networks architectures and methods for supervised features learning. We first draw up a state-of-the-art review of the Convolutional Neural Networks aiming to understand the history behind this ...
• Theoretical research in deep learning • Improvements to existing deep learning algorithms or models • Application of deep learning to solve a real-word problem • Development of deep learning software Finding a research problem to work on is considered as part of this thesis subject. Students should come with a concrete and well-de ned ...
PhD Dissertations [All are .pdf files] Neural processes underlying cognitive control during language production (unavailable) Tara Pirnia, 2024 The Neurodynamic Basis of Real World Face Perception Arish Alreja, 2024. Towards More Powerful Graph Representation Learning Lingxiao Zhao, 2024. Robust Machine Learning: Detection, Evaluation and Adaptation Under Distribution Shift Saurabh Garg, 2024
Deep learning (DL), a branch of machine learning (ML) and artificial intelligence (AI) is nowadays considered as a core technology of today's Fourth Industrial Revolution (4IR or Industry 4.0). Due to its learning capabilities from data, DL technology originated from artificial neural network (ANN), has become a hot topic in the context of computing, and is widely applied in various ...
most e cient statistical model for visual recognition. The goal of this work is to produce an analysis of the state-of-the-art methods to train such models, to explain their limitatio. se original idea to overcome the latter.ContributionsThis master's thesis introduces a number of c.
Selecting a thesis topic for ML Projects is not easy as Machine Learning uses statistical algorithms to make computers work. Here are the top 10 research and thesis topics for ML projects. ... Object Detection with Deep Learning is one of the interesting machine learning projects to create. When it comes to image classification, Deep Neural ...
Below you can see the thesis topics for 2022-2023. We offer 3 different thesis formats: - Format 1 : Regular thesis (fully supervised by KU Leuven) - Format 2 : Thesis in cooperation with a company (supervised by KU Leuven and the company) - Format 3 : Thesis with a company project within a company (supervised by the company) NOTE: Additional ...
Go 1.0 was released in March 2012 [22]. The focus of this thesis is to integrate GPU computation with the Go language for the purpose of developing deep learning models. This chapter includes a review of some of the packages that were developed for GPU computation with Go, the applications that use them, and other deep learning frameworks. 2.1 ...
Latest thesis topics in Machine Learning for research scholars: ... Deep Learning. Deep Learning is a hot topic in Machine Learning. It is already explained above. It is a part of the family of machine learning and deals with the functioning of the artificial neural network. Neural Networks are used to study the functioning of the human brain.
At our upcoming event this November 16th-18th in San Francisco, ODSC West 2021 will feature a plethora of talks, workshops, and training sessions on deep learning and deep learning research. You can register now for 60% off all ticket types before the discount drops to 40% in a few weeks.
Abstract. There have been rapid advances at the intersection of deep learning and medicine over the last few years, especially for the interpretation of medical images. In this thesis, I describe three key directions that present challenges and opportunities for the development of deep learning technologies for medical image interpretation.
Topic: Current model-free Deep Reinforcement Learning (DRL) approaches for quadruped locomotion can learn highly agile locomotion (like parkour [1]), while being far more flexible than model-based or optimal control approaches. But to achieve a natural-looking gait they rely on complex reward functions with up to 12 or more reward terms and ...
Below you can see the thesis topics for 2023-2024. We offer 3 different thesis formats: - Format 1 : Regular thesis (fully supervised by KU Leuven) ... X Deep learning for point and interval forecasts for electricity demand and prices; Estimating calendar effects to detect operational issues in commercial buildings; X ...
2. Deep Learning . Deep Learning is a subset of Machine Learning that learns by imitating the inner working of the human brain in order to process data and implement decisions based on that data. Basically, Deep Learning uses artificial neural networks to implement machine learning. These neural networks are connected in a web-like structure ...
The subcellular localization of RNA has become a very popular research topic in recent years, with the subcellular localization of ... "DRpred: A Novel Deep Learning-Based Predictor for Multi-Label mRNA Subcellular Localization Prediction by Incorporating Bayesian Inferred Prior Label Relationships" Biomolecules 14, no. 9: 1067. https://doi.org ...