- Publications
- Conferences & Events
- Professional Learning
- Science Standards
- Awards & Competitions
- Instructional Materials
- Free Resources
- For Preservice Teachers
- NCCSTS Case Collection
- Science and STEM Education Jobs
- Interactive eBooks+
- Digital Catalog
- Regional Product Representatives
- e-Newsletters
- Browse All Titles
- Bestselling Books
- Latest Books
- Popular Book Series
- Submit Book Proposal
- Web Seminars
- National Conference • New Orleans 24
- Leaders Institute • New Orleans 24
- National Conference • Philadelphia 25
- Exhibits & Sponsorship
- Submit a Proposal
- Conference Reviewers
- Past Conferences
- Latest Resources
- Professional Learning Units & Courses
- For Districts
- Online Course Providers
- Schools & Districts
- College Professors & Students
- The Standards
- Teachers and Admin
- eCYBERMISSION
- Toshiba/NSTA ExploraVision
- Junior Science & Humanities Symposium
- Teaching Awards
- Climate Change
- Earth & Space Science
- New Science Teachers
- Early Childhood
- Middle School
- High School
- Postsecondary
- Informal Education
- Journal Articles
- Lesson Plans
- e-newsletters
- Science & Children
- Science Scope
- The Science Teacher
- Journal of College Sci. Teaching
- Connected Science Learning
- NSTA Reports
- Next-Gen Navigator
- Science Update
- Teacher Tip Tuesday
- Trans. Sci. Learning

MyNSTA Community
- My Collections
All Case Studies
Cases (only) are freely accessible; subscription is required for access to teaching notes and answer keys..
Suggested Keywords, to help with your search (besides selecting subjects): clicker cases, directed cases, interrupted cases, discussion cases, intimate debate cases. As a reminder, all cases may be adjusted to meet the needs of your student level. See our case use guidelines.
Grade level filtering: enter desired grade levels in the Keywords box and click Apply .
How Failing in Science Helped Solve the Coho Salmon Mystery
By Meghan Ward, Krystal Nunes, Nicole Laliberté, Fiona Rawle
Nia’s Painful Breast
By Jenna Ridlen, Sharifah Albraiki , Sarah Ellis, Jocelyn A. Moore, Anusree Mukherjee
An Anaphylaxis Praxis
By Hollie L. Leavitt
Pituitary Perplexity
By Marian Leal
The Anti-Cancer Fight with the Wellness Menu
By Michelle Sue, Kenneth W. Yip
Suminoe Oysters Redux
By Matthew L. Simon
Forests for Lemurs
By Ariadna Mondragon-Botero, Susan M. Galatowitsch
Beaker Has a UTI
By Melissa S. Kosinski-Collins, Kene Piasta, Martin Samuels, Ariana Hinckley-Boltax
The Organic Chemistry of Drug Design in a Case of Schizophrenia
By Brahmadeo Dewprashad, Vishnu Tiwari
A Long Recovery Road for Norrie
By Melissa S. Kosinski-Collins , Caitlin M. Hepps Keeney, Ariana L. Hinckley-Boltax
Dystrophin Stability and Cardiomyopathy
By Richard J. Kwak, Joyce A. Horton, Zyan Davis, Kristy J. Wilson
A Bioinformatic Investigation of a Mysterious Meningoencephalitis
By Sari Matar, Dyan Anore, Basma Galal, Shawn Xiong
Diabetic Ketoacidosis Upon Diagnosis
By Ali Chaari, Aisha Kafoud
The Name’s Bond, Chemical Bond
By Katie McShea, Kari Fleuriet, Fatmah Alamoudi, Deana Jaber
The Baby Who Had No Baby Fat
By Sheri L. Boyce
Using Case Studies with Large Classes
Why Use Case Studies?
Case studies are powerful tools for teaching. They explore the story behind scientific research to understand the phenomenon being studied, the question the scientist asked, the thinking they used to investigate it, and the data they collected to help students better understand the process and content of science.
A strength of this approach is that it gives students the chance to consider how they would investigate a topic. Their answers are often similar to what the researchers being studied did. But students also come up with novel perspectives and unique approaches to the problems.
Many BioInteractive resources lend themselves to a case study approach. In most instances, what I ultimately decide is to convert the resource into a case study. For example, the video Animated Life: Mary Leakey is an excellent tool to get students thinking about the logic scientists use to study fossils and extinct species. Data Point resources are also a rich source of figures and questions that can be copied and pasted into a presentation to provide a brief case study that introduces a topic.
The Challenge for Large Classes
Many BioInteractive activities are structured in a way that they are particularly useful for smaller groups and classes. And by smaller, I am thinking of fewer than 50 students. To some colleagues, that may seem to be a large class size. Indeed, in many instances, it probably is more than is optimal.
However, when I refer to large classes, what I am thinking of are the large introductory classes encountered in many colleges and universities in which enrollment can range from 100 to 500 or more depending on the institution. Classes of this size present instructors with the dual challenges of not just numbers but also anonymity. It’s logistically unmanageable to share and distribute printed copies of handouts or worksheets.
How to Scale Up
So how can an instructor promote the interaction that is essential to the success of these types of case study activities in such a large group? These are issues I grappled with when I went from teaching at a small liberal arts college where my classes were smaller than 30 to teaching at a large university with classes of several hundreds. I have found what I think are four parts to an effective solution.
1. Define a learning objective.
First and foremost, whether I have 30 or 300 students, I try to think about why I want to use a particular BioInteractive resource. I consider what it is that I want the students to do or think about while using the resource. How do I want them to be different after completing the assignment? In essence, I define the learning objective so I can determine the most effective platform and approach to deliver the lesson utilized in the resource.
2. Create presentations with strategic pause points.
PowerPoint is a common tool for delivering material in large classrooms. It is quite easy to take images and questions from BioInteractive resource PDFs and insert them into slides. After reading the teaching notes and text in the student handouts, it’s relatively simple to develop the story that weaves the slides together in an interrupted case study. This is a style of case study that progressively leads students through the information with carefully planned “reveals” of information and strategically placed questions as stopping points to ponder the material along the way.
Videos are also fabulous resources to use during interrupted case studies in class. For example, I regularly use the video Niche Partitioning and Species Coexistence , which describes Dr. Rob Pringle’s work on niche partitioning in the savanna, as the core of a video case study in class. After the class watches the video for a few minutes, I stop and ask students about the phenomenon being studied and approaches that could be used to answer different questions.
I often use the following questions/prompts:
- Why would anyone care about factors shaping species presence or absence?
- Think about what factors could be important influences on shaping species richness in a community.
How can we use modern techniques to study what an animal is eating when we can’t watch the animal eat? The video does an excellent job of addressing these topics and showing how researchers developed a creative approach to applying molecular techniques to answer ecological questions. How awesome is it that one video can help students tie together the central dogma, ecological theory, and community concepts! Depending on how much an instructor wants to structure the video case study in advance, it is even possible to embed small video clips and questions directly into a PowerPoint presentation.
3. Have students use clickers.
How should we tell the scientific story to large numbers of students and engage them in it? Clickers are a particularly helpful tool for asking questions about experiments, concepts, or results, because they present students with a specific moment when they need to choose among different options for a survey of their opinion or decide among right and wrong answers in a multiple-choice question.
For example, I typically start a case study with survey questions asking students to identify what they think is the most important item on a list of potential phenomena or to give their feedback about an issue in a Likert-scale response. Later, as the case study develops, I ask more specific questions about the experiment that require students to predict experimental outcomes or interpret a figure. For example, when I use the video The Effects of Fungicides on Bumble Bee Colonies , I show students several bar graphs with possible outcomes for the experiment and have them pick which they think the researchers will observe. After revealing the actual results, I ask them questions about interpreting the results and whether the results support the experimental hypothesis. I always allow students to talk and help one another during clicker questions to enhance their interaction and give them a choice to go along with a group opinion or answer based on their individual thinking.
4. Flip the classroom.
Another effective way to use BioInteractive resources in large classes is to use videos to flip a class session. BioInteractive animations and short films are rich with information that can pique interest, start discussions, or provide fundamental information. For example, I recently had my students watch the Genes as Medicine short film outside of class time. I asked them to then imagine they were an alien that found this video clip and to consider what information it would give them about life on Earth. This sparked a lively discussion about what life is to start the next class meeting that was more interesting than me going through a checklist of terms and definitions. Students had to uncover the characteristics of life from the video for themselves.
Benefits and Takeaways
What I hope these hints and suggestions from my own experiences show is how relatively simple it can be to scale up these resources to engage a class of any size. When they first encounter case studies, students can be a little unsure about this approach that requires them to talk to one another in a setting where they are expecting to be a face in the crowd. However, after they experience one or two case studies, I can see groups of students talking and exchanging ideas about the case. They are no longer passive listeners sitting in a room but instead have become active problem solvers seeking answers together. I can leave the stage and mingle through the room to listen to their discussions and encourage them as they develop their answers. This also gives me an opportunity to interact with students besides those sitting in the front row and to further develop a sense of community and connection, solving one of the challenges with big classes: anonymity.
It has been my experience that students quickly adapt to and begin to enjoy this approach. Rather than sitting in class watching yet another series of PowerPoint slides flash by, they are thinking and talking about science with one another. After my students talk things through with their neighbors and “shoulder buddies” during a case study, I find that they are more likely to speak up in class during the case study and at other points during the course.
At the beginning of the semester, I can barely get anyone to answer a question. After a few case studies, students begin asking and answering questions (even when we aren’t doing case studies), and the level of participation by different students in the room is noticeably higher. So in addition to case studies being a more interesting way for me as the teacher to present material to students and explore different biological topics, this approach also has the added benefits of helping build confidence within individual students and community among students, which makes a more rewarding and exciting learning environment for everyone.
Educational case studies based on examples of simulated or real research data can engage students in the process of thinking like a scientist, even when it is not possible to get into the field or laboratory to actually run an experiment. They can help overcome the challenges of data analysis and interpretation that are at the core of science education experiences. The collections of different resources available through HHMI BioInteractive provide a menu of modules for instructors to choose from that do just that. They get students to explore important biological topics from a variety of different approaches and look at the world through the lenses of different scientists. Regardless of what the actual format of a resource is when I encounter it, I know that it is possible to scale it up in some way to meet the needs of my classes.
Come join a conversation about this blog post at our Facebook group!
Phil Gibson is a professor at the University of Oklahoma, where he enjoys teaching his students that learning a little botany never hurt anyone and is probably good for them in the long run. When he’s not thinking about new resources to use in class, he enjoys hiking with his family, listening to music, and cooking outrageously large breakfasts on the weekends.
Related Articles
Cindy Gay presents BioInteractive's short video clip demonstrating the fruit fly courtship dance and its role in selection. She describes how she uses the clip in her AP® Biology curriculum, and how it connects to topics ranging from animal behavior to sympatric speciation.
In this video Educator Voices post, hear from St. John Fisher College professor Kaitlin Bonner about how she uses a publicly available data set, along with BioInteractive’s elephant resources, to have her students investigate data.
Kim Parfitt describes two activities (now merged into the activity “Scientific Inquiry and Data Analysis Using WildCam Gorongosa”) associated with the WildCam Gorongosa project. She also discusses a short film on lion populations in Gorongosa that she uses to introduce the topic.
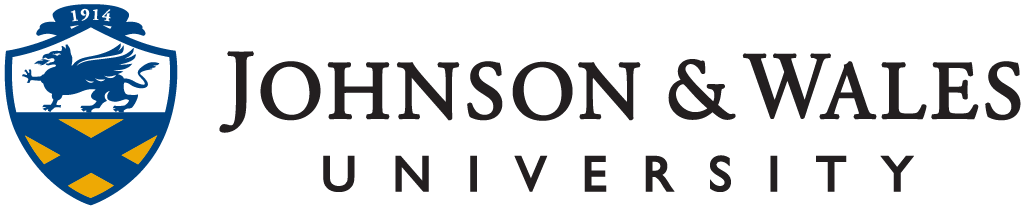
Want to create or adapt books like this? Learn more about how Pressbooks supports open publishing practices.
8 2.1 Case Study: Why Should You Study Human Biology?
Created by CK-12/Adapted by Christine Miller
Case Study: Our Invisible Inhabitants
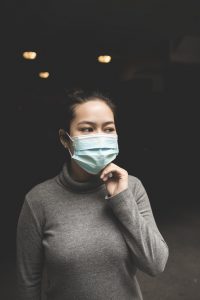
Lanying is suffering from a fever, body aches, and a painful sore throat that feels worse when she swallows. She visits her doctor, who examines her and performs a throat culture. When the results come back, he tells her that she has strep throat, which is caused by the bacteria Streptococcus pyogenes . He prescribes an antibiotic that will either kill the bacteria or stop it from reproducing, and advises her to take the full course of the treatment even if she is feeling better earlier. Stopping early can cause an increase in bacteria that are resistant to antibiotics.
Lanying takes the antibiotic as prescribed. Toward the end of the course, her throat is feeling much better — but she can’t say the same for other parts of her body! She has developed diarrhea and an itchy vaginal yeast infection. She calls her doctor, who suspects that the antibiotic treatment has caused both the digestive distress and the yeast infection. He explains that our bodies are home to many different kinds of microorganisms, some of which are actually beneficial to us because they help us digest our food and minimize the population of harmful microorganisms. When we take an antibiotic, many of these “good” bacteria are killed along with the “bad,” disease-causing bacteria, which can result in diarrhea and yeast infections.
Lanying’s doctor prescribes an antifungal medication for her yeast infection. He also recommends that she eat yogurt with live cultures, which will help replace the beneficial bacteria in her gut. Our bodies contain a delicate balance of inhabitants that are invisible without a microscope, and changes in that balance can cause unpleasant health effects.
What Is Human Biology?
As you read the rest of this book, you’ll learn more amazing facts about the human organism, and you’ll get a better sense of how biology relates to your health. Human biology is the scientific study of the human species, which includes the fascinating story of human evolution and a detailed account of our genetics, anatomy, physiology, and ecology. In short, the study focuses on how we got here, how we function, and the role we play in the natural world. This helps us to better understand human health, because we can learn how to stay healthy and how diseases and injuries can be treated. Human biology should be of personal interest to you to the extent that it can benefit your own health, as well as the health of your friends and family. This branch of science also has broader implications for society and the human species as a whole.
Chapter Overview: Living Organisms and Human Biology
In the rest of this chapter, you’ll learn about the traits shared by all living things, the basic principles that underlie all of biology, the vast diversity of living organisms, what it means to be human, and our place in the animal kingdom. Specifically, you’ll learn:
- The seven traits shared by all living things: homeostasis , or the maintenance of a more-or-less constant internal environment; multiple levels of organization consisting of one or more cells ; the use of energy and metabolism ; the ability to grow and develop; the ability to evolve adaptations to the environment; the ability to detect and respond to environmental stimuli; and the ability to reproduce .
- The basic principles that unify all fields of biology, including gene theory, homeostasis, and evolutionary theory.
- The diversity of life (including the different kinds of biodiversity), the definition of a species, the classification and naming systems for living organisms, and how evolutionary relationships can be represented through diagrams, such as phylogenetic trees.
- How the human species is classified and how we’ve evolved from our close relatives and ancestors.
- The physical traits and social behaviors that humans share with other primates.
As you read this chapter, consider the following questions about Lanying’s situation:
- What do single-celled organisms (such as the bacteria and yeast living in and on Lanying) have in common with humans?
- How are bacteria, yeast, and humans classified?
- How do the concepts of homeostasis and biodiversity apply to Lanying’s situation?
- Why can stopping antibiotics early cause the development of antibiotic-resistant bacteria?
Attribution
Figure 2.1.1
Photo (face mask) by Michael Amadeus , on Unsplash is used under the Unsplash license (https://unsplash.com/license).
Mayo Clinic Staff (n.d.). Strep throat [online article]. MayoClinic.org. https://www.mayoclinic.org/diseases-conditions/strep-throat/symptoms-causes/syc-20350338
The ability of an organism to maintain constant internal conditions despite external changes.
The smallest unit of life, consisting of at least a membrane, cytoplasm, and genetic material.
The chemical processes that occur in a living organism to sustain life.
The production of offspring by sexual or asexual process.
The variety of life in the world, ecosystem, or in a particular habitat.
Human Biology Copyright © 2020 by Christine Miller is licensed under a Creative Commons Attribution-NonCommercial 4.0 International License , except where otherwise noted.
Share This Book
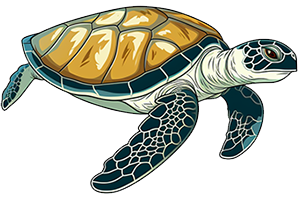
The Biology Corner
Biology Teaching Resources

Case Study – A Tiny Heart (old version)
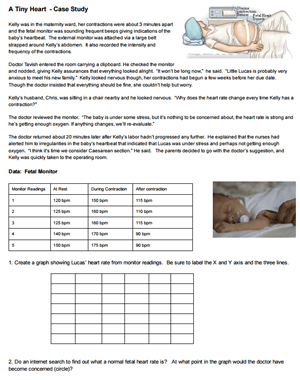
This case study was revised in 2023, get the NEW VERSION !
This case study focuses on a baby boy who was born with a problem with his heart. The story is based on a real scenario, though some of the names have been changed, and the parents gave permission to include photos of the infant.
Students will read about symptoms that occur when a baby is born with stenosis, or a narrowing of the artery. Students consider treatment options and compare the circulation of a fetus to that of an adult. Finally, the Ross Procedure is described where a valve from the pulmonary artery is moved to the aorta.
This case study was made for a high school anatomy class, and may not be appropriate for younger audiences. Students should have already completed the chapter on the circulatory system and have a strong foundation in how the circulatory system works. Case studies are designed to be completed in small groups so that students can have discussions and help each other with difficult vocabulary.
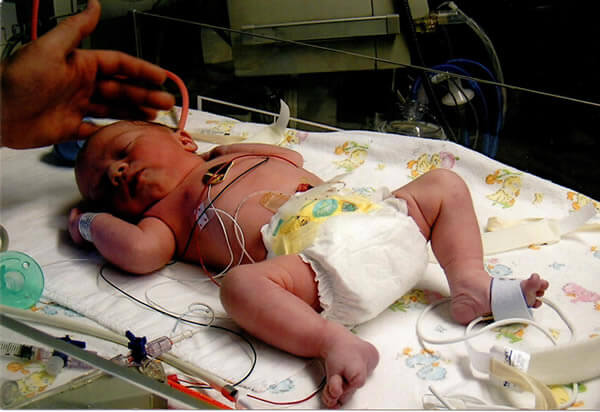
HS-LS1-2 Develop and use a model to illustrate the hierarchical organization of interacting systems that provide specific functions within multicellular organisms
Shannan Muskopf
Comparative case study on NAMs: towards enhancing specific target organ toxicity analysis
- Regulatory Toxicology
- Open access
- Published: 29 August 2024
Cite this article
You have full access to this open access article
- Kristina Jochum 1 ,
- Andrea Miccoli 1 , 2 , 5 ,
- Cornelia Sommersdorf 3 ,
- Oliver Poetz 3 , 4 ,
- Albert Braeuning 5 ,
- Tewes Tralau 1 &
- Philip Marx-Stoelting ORCID: orcid.org/0000-0002-6487-2153 1
Traditional risk assessment methodologies in toxicology have relied upon animal testing, despite concerns regarding interspecies consistency, reproducibility, costs, and ethics. New Approach Methodologies (NAMs), including cell culture and multi-level omics analyses, hold promise by providing mechanistic information rather than assessing organ pathology. However, NAMs face limitations, like lacking a whole organism and restricted toxicokinetic interactions. This is an inherent challenge when it comes to the use of omics data from in vitro studies for the prediction of organ toxicity in vivo. One solution in this context are comparative in vitro–in vivo studies as they allow for a more detailed assessment of the transferability of the respective NAM data. Hence, hepatotoxic and nephrotoxic pesticide active substances were tested in human cell lines and the results subsequently related to the biology underlying established effects in vivo. To this end, substances were tested in HepaRG and RPTEC/tERT1 cells at non-cytotoxic concentrations and analyzed for effects on the transcriptome and parts of the proteome using quantitative real-time PCR arrays and multiplexed microsphere-based sandwich immunoassays, respectively. Transcriptomics data were analyzed using three bioinformatics tools. Where possible, in vitro endpoints were connected to in vivo observations. Targeted protein analysis revealed various affected pathways, with generally fewer effects present in RPTEC/tERT1. The strongest transcriptional impact was observed for Chlorotoluron in HepaRG cells (increased CYP1A1 and CYP1A2 expression). A comprehensive comparison of early cellular responses with data from in vivo studies revealed that transcriptomics outperformed targeted protein analysis, correctly predicting up to 50% of in vivo effects.
Similar content being viewed by others
Comparison of base-line and chemical-induced transcriptomic responses in heparg and rptec/tert1 cells using tempo-seq.
Omics in Toxicology
Avoid common mistakes on your manuscript.
Introduction
Given the at times heated discussions about regulatory toxicology in the political and public domain, the quite remarkable track record of toxicological health protection sometimes tends to go unnoticed. Not only are chemical scares such as the chemically induced massive acute health impacts in the 1950ies, 60ies and 70ies a thing of the past (Herzler et al. 2021 ), but in many parts of the world, there are now regulatory frameworks in place which aim at the early identification of potential health risks from chemicals. Within Europe, the most notable in terms of impact are probably REACH (EC 2006 ) and the regulations on pesticides (EC 2009 ) both of which still overwhelmingly rely on animal data for their risk assessments. This has manifold reasons, one being the historical reliability of animal-based systems for the prediction of adversity in humans. However, there are a number of challenges to this traditional approach. These comprise capacity issues when it comes to the testing of thousands of new or hitherto untested substances, the testing of mixtures, the ever-daunting question of species specificity or the limitation of current in vivo studies regarding less accessible endpoints such as for example immunotoxicity or developmental neurotoxicity.
Over recent years, so-called New Approach Methodologies (NAMs) have thus attracted increased attention and importance for regulatory toxicology. The United States Environmental Protection Agency (US EPA 2018 ) defines NAM as ‘…a broadly descriptive reference to any technology, methodology, approach, or combination thereof that can be used to provide information on chemical hazard and risk assessment that avoids the use of intact animals… ’. One instance of an attempt to replace an animal test with an in vitro test system is the embryonic stem cell test in the area of developmental toxicology (Buesen et al. 2004 ; Seiler et al. 2006 ). This stand-alone test was first evaluated for assessing the embryotoxic potential of chemicals as early on as 2004 (Genschow et al. 2004 ). While its establishment as a regulatory prediction model took several more years, one major outcome was the realization that the use of NAMs in general is greatly improved when used as part of a biologically and toxicologically meaningful testing battery (Marx-Stoelting et al. 2009 ; Schenk et al. 2010 ). It should be noted that despite all the potential of such testing batteries a tentative one to one replacement of animal studies is neither practical nor straight forward. The reason is not only the complexity of the endpoints in question but also practical constraints. This was recently exemplified by Landsiedel et al. who pointed out that with the number of different organs and tissues tested during one sub-chronic rodent study, and assuming that 5 NAMs are needed to address the adverse outcomes in any of those organs, it would take decades just to replace this one study. Any regulatory use of NAMs should hence preferably rely on their direct use (Landsiedel et al. 2022 ).
An example from the field of hepatotoxicity testing is the in vitro toolbox for steatosis that was developed by Luckert et al. ( 2018 ) based on the adverse outcome pathway (AOP) concept by Vinken ( 2015 ). The authors employed five assays covering relevant key events from the AOP in HepaRG cells after incubation with the test substance Cyproconazole. Concomitantly, transcript and protein marker patterns for the identification of steatotic compounds were established in HepaRG cells (Lichtenstein et al. 2020 ). The findings were subsequently brought together in a proposed protocol for AOP-based analysis of liver steatosis in vitro (Karaca et al. 2023a ).
One promising use for such cell-based systems is their combination with multi-level omics. In conjunction with sufficient biological and mechanistic knowledge, the wealth of information provided by multi-omics data should potentially allow some prediction of substance-induced adversity. That said any such prediction can of course only be reliable within the established limits of such systems such as the lack of a whole organism and incomplete toxicokinetics and restrictions on adequately capturing the effects of long-term exposure (Schmeisser et al. 2023 ). Regulatory use and trust in cell-based systems will, therefore, strongly rely on how they compare to the outcome of studies based on systemic data (Schmeisser et al. 2023 ).
Pesticide active substances are a group of compounds with profound in vivo data. Some examples for active substances commonly used in PPPs are the fungicides Cyproconazole, Fluxapyroxad, Azoxystrobin and Thiabendazole, as well as the herbicide Chlorotoluron and the multi-purpose substance 2-Phenylphenol. For these compounds, several short- and long-term studies in rodents have been conducted and multiple adverse effects in target organs like liver or kidneys were observed (see Table 1 ). Liver steatosis, as one potential adverse health outcome, has been associated with triazole fungicides, such as Cyproconazole, but other active substances such as Azoxystrobin are suspected to interfere with the lipid metabolism as well (Gao et al. 2014 ; Luckert et al. 2018 ). Potential modes of action for adverse effects include the activation of nuclear receptors, such as the constitutive androstane receptor (CAR), which has been shown for Cyproconazole and Fluxapyroxad (Marx-Stoelting et al. 2017 ; Tamura et al. 2013 ; Zahn et al. 2018 ). Notably, even when an active substance is considered to be of low acute toxicity, e.g. Chlorotoluron, Thiabendazole and 2-Phenylphenol (EC 2015 ; US EPA 2002 ; WHO 1996 ), they might still exhibit adverse chronic effects (Mizutani et al. 1990 ; WHO 1996 ). This is the reason why pesticide active substances and plant protection products (PPP) are assessed extensively before their placing on the market (EC 2009 ).
The target organs most frequently affected by pesticide active ingredients are the liver and kidneys (Nielsen et al. 2012 ). Hence, an in vitro test system aimed at the prediction of pesticide organ toxicity should be able to model effects on these two target organs. One of the best options currently available for hepatotoxicity studies in vitro is the cell line HepaRG (Ashraf et al. 2018 ). Before their use in toxicological assays, the cells undergo a differentiation process resulting in CYP-dependent activities close to the levels in primary human hepatocytes (Andersson et al. 2012 ; Hart et al. 2010 ). They also feature the capability to induce or inhibit a variety of CYP enzymes (Antherieu et al. 2010 ; Hartman et al. 2020 ) and the expression of phase II enzymes, membrane transporters and transcription factors (Aninat et al. 2006 ). Antherieu et al. ( 2012 ) demonstrated that HepaRG cells can sustain various types of chemically induced hepatotoxicity following acute and repeated exposure. Hence, HepaRG cells have the potential to replace the use of primary human hepatocytes in the study of acute and chronic effects of xenobiotics in the liver. In 2012, the European Commission Joint Research Centre’s European Union Reference Laboratory for Alternatives to Animal Testing (EURL ECVAM) coordinated a validation study finding differentiated HepaRG cells as a reliable and relevant tool for CYP enzyme activity studies (EURL ECVAM 2012 ). This led to the proposal of a respective draft test guideline by the OECD in 2019 (OECD 2019 ). Additionally, as part of the US EPA Tox21 project, HepaRG cells were used for an assay assessing toxicogenomics (Franzosa et al. 2021 ).
A promising test system for investigations of nephrotoxicity is the tERT1 immortalized renal proximal tubular epithelial cell line RPTEC/tERT1 (further referred to as RPTEC). These non-cancerous cells have been found to closely resemble primary counterparts showing typical morphology and functionality (Shah et al. 2017 ; Wieser et al. 2008 ). Aschauer et al. ( 2015 ) demonstrated the applicability of RPTEC for investigation of repeated-dose nephrotoxicity using a transcriptomic-based approach. Simon et al. ( 2014 ) showed similar toxicological responses of RPTEC and the target tissue to exposure to benzo[ a ]pyrene and cadmium. Conclusively, RPTEC can be a useful tool for toxicological studies.
In the present study, six pesticide active substances were analyzed in two cell lines, namely the liver cell line HepaRG and the kidney cell line RPTEC. Assays were performed following exposure to the highest non-cytotoxic concentration and comprised targeted protein and transcriptomics analysis. Triggered pathways were identified and compared with established results from in vivo experiments.
Materials and methods
All test substances were purchased in analytical grade (purity ≥ 98.0%) from Sigma-Aldrich, Pestanal® (Taufkirchen, Germany): Cyproconazole, CAS no. 94361–06-5, catalog no. 46068, batch no. BCCD4066; Fluxapyroxad, CAS no. 907204–31-3, catalog no. 37047, batch no. BCCF6749; Azoxystrobin, CAS no. 131860–33-8, catalog no. 31697, batch no. BCCF6593; Chlorotoluron, CAS no. 15545–48-9, catalog no. 45400, batch no. BCBW1414; Thiabendazole, CAS no. 148–79-8, catalog no. 45684, batch no. BCBV5436; 2-Phenylphenol, CAS no. 90–43-7, catalog no. 45529, batch no. BCCF1784. William’s E medium, fetal calf serum (FCS) good forte (catalog no. P40-47500, batch no. P131102), recombinant human insulin and l -glutamine were acquired from PAN-Biotech GmbH (Aidenbach, Germany), FCS superior (catalog no. S0615, batch no. 0001659021) from Bio&Sell (Feucht bei Nürnberg, Germany). Dimethyl sulfoxide (DMSO, purity ≥ 99.8%), hydrocortisone-hemisuccinate (HC/HS), hydrocortisone, epidermal growth factor (EGF) and neutral red (NR) were purchased from Sigma-Aldrich (Taufkirchen, Germany). Dulbecco’s modified eagle medium (DMEM) and Ham’s F Nutrition mix were obtained from Gibco® Life Technologies (Karlsruhe, Germany), trypsin–EDTA, Penicillin–Streptomycin and insulin-transferrin-selenium from Capricorn Scientific GmbH (Ebsdorfergrund, Germany).
Cell culture
HepaRG cells were obtained from Biopredic International (Sant Grégoire, France) and kept in 75 cm 2 flasks under humid conditions at 37 °C and 5% CO 2 . Cells were grown in proliferation medium consisting of William’s E medium with 2 mM l -glutamine, supplemented with 10% FCS good forte, 100 U mL −1 penicillin, 100 µg mL −1 streptomycin, 0.05% human insulin and 50 µM HC/HS for 2 weeks. Then, HepaRG cells were passaged using trypsin–EDTA solution and seeded in 75 cm 2 flasks, 6-well, 12-well and 96-well plates at a density of 20 000 cells per cm 2 . Cells in cell culture dishes were maintained in proliferation medium for another 2 weeks before the medium was changed to differentiation medium (i.e., proliferation medium supplemented by 1.7% DMSO) and cells were cultured for another 2 weeks. Thereafter, cells were used in experiments within 4 weeks, while media was changed to treatment media (i.e., proliferation media supplemented by 0.5% DMSO and 2% FCS) 2 days prior to the experiments.
The RPTEC cell line was obtained from Evercyte GmbH (Vienna, Austria) and cultivated as previously described (Aschauer et al. 2013 ; Wieser et al. 2008 ). Cells were grown in a 1:1 mixture of DMEM and Ham’s F-12 Nutrient Mix, supplemented with 2.5% FCS superior, 100 U mL −1 penicillin, 100 µg mL −1 streptomycin, 2 mM l -glutamine, 36 ng mL −1 hydrocortisone, 10 ng mL −1 EGF, 5 µg mL −1 insulin, 5 µg mL −1 transferrin and 5 ng mL −1 selenium. RPTEC were cultivated in 75 cm 2 flasks until they reached near confluence. Then, cells were passaged using trypsin–EDTA and seeded at 30% density in 75 cm 2 flasks for further sub-cultivation and 6-well, 12-well and 96-well plates for experiments. To obtain complete differentiation, cells in cell culture dishes were maintained for 14 days before they were used in experiments.
Test concentrations
All substances were dissolved in DMSO and diluted in the respective medium to a final DMSO concentration of 0.5% before incubation. HepaRG treatment medium and 0.5% DMSO in RPTEC medium served as solvent controls for HepaRG cells and RPTEC, respectively. At least 3 biological replicates, i.e., independent experiments, were performed for each assay.
Cell viability
Cell viability was investigated with the WST-1 assay (Immunservice, Hamburg, Germany), according to the manufacturer’s protocol and subsequent NR uptake assay according to Repetto et al. ( 2008 ). HepaRG cells and RPTEC were seeded in 96-well plates and incubated with the test substances for 72 h. Triton X-100 (0.01%, Thermo Fisher Scientific, Darmstadt, Germany) was used as positive control for reduced cell viability. At the end of the incubation period, 10 µL WST-1 solution was added to each well and incubated for 30 min at 37 °C. The tetrazolium salt WST-1 is metabolized by cellular mitochondrial dehydrogenases of living cells to a formazan derivative, the absorbance of which was measured at 450 nm with an Infinite M200 PRO plate reader (Tecan, Maennedorf, Switzerland). The reading of each well was related to the absorbance value at the reference wavelength of 620 nm, and blank values were subtracted before the relation to the solvent control.
Afterwards the NR uptake assay was performed, where incorporation of NR into lysosomes of viable cells is measured. One day prior to the assay, NR medium was prepared by diluting a 4 mg mL −1 NR stock solution in PBS 1:100 with the respective cell culture medium for HepaRG cells and RPTEC, and incubated at 37 °C over night. After the WST-1 measurement, the incubation medium was removed and cells were washed twice with PBS. Subsequently, 100 µL NR medium, previously centrifuged for 10 min at 600 × g , was added and incubated for 2 h. Afterwards, cells were washed twice with PBS, and 100 µL destaining solution (49.5:49.5:1 ethanol absolute, distilled water, glacial acetic acid) per well was added. Plates were shaken at 500 rotations min −1 for 10 min and fluorescence of NR was measured with an Infinite M200 PRO plate reader (Tecan, Maennedorf, Switzerland) at 530 nm excitation and 645 nm emission. Each reading was subtracted by the blank value and normalized to the solvent control.
Multiplexed microsphere-based sandwich immunoassays
Marker proteins and protein modifications were analyzed by Signatope GmbH (Tübingen, Germany) with a multiplexed microsphere-based sandwich immunoassay. Cells were seeded in 6-well plates and incubated with the test substances for 36 and 72 h. Protein extraction was performed by adding 250 µL pre-cooled extraction buffer, supplied by the company, to the cells in each well and subsequent incubation for 30 min at 4 °C. Cell lysates were transferred to 1.5 mL reaction tubes and centrifuged for 30 min at 4 °C and 15 000 × g . The supernatant was aliquoted in 60 µL batches and stored at -80 °C until shipment. After thawing, aliquots were directly used and not frozen again. Samples were analyzed for 8 proteins and protein modifications, each representing a marker for a certain form of toxicity (Table 2 ).
Quantitative real-time PCR and PCR profiler arrays
RT-qPCR was conducted to ensure well performing RNA for subsequent PCR profiler arrays. Cells were seeded in 12-well plates and incubated with the test substances for 36 h. RNA extraction was performed with the RNA easy Mini Kit (Qiagen, Venlo, Netherlands) according to the manufacturer’s manual. Yield RNA concentration and purity were analyzed with a Nanodrop spectrometer (NanoDrop 2000, Thermo Fischer Scientific, Darmstadt, Germany) and RNA samples were stored at -80 °C until further use. Reverse transcription to cDNA was conducted using the High-Capacity cDNA Reverse Transcription Kit (Applied Biosystems, Waltham, MA, USA) according to the manufacturer’s protocol with a GeneAmp ® PCR System 9700 (Applied Biosystems, Darmstadt, Germany) and cDNA samples were stored at – 20 °C. RT-qPCR was performed with Maxima SYBR Green/ROX Master Mix (Thermo Fisher Scientific, Darmstadt, Germany) according to manufacturer’s protocol. In brief, 9 µL master mix, consisting of 5 µL Maxima SYBR Green/ROX qPCR Master Mix, 0.6 µL each of forward and reverse primers (2.5 µM) and 2.8 µL nuclease-free water, was added to each well of a 384-well plate. Primer sequences are shown in Online Resource 1. Subsequently, 20 ng cDNA was added to each well to a final volume of 10 µL and RT-qPCR was performed with an ABI 7900HT Fast Real-Time PCR system instrument (Applied Biosystems, Darmstadt, Germany). In brief, activation took place at 95 °C for 15 min, followed by 40 cycles of 15 s at 95 °C and 60 s at 60 °C, followed by 15 min at 60 °C and default melting curve analysis. Data were processed using 7900 software v241 and Microsoft Excel 2021. Threshold cycle (C T ) was set to 0.5, melting curve was checked and manual baseline correction was performed for each gene individually. Yield C T -values were extracted to Microsoft Excel 2021 and relative gene expression was obtained with the 2 −ΔΔCt method according to Livak and Schmittgen ( 2001 ). GUSB and HPRT1 served as endogenous control genes for HepaRG cells, GUSB and GAPDH were used for RPTEC. Primer efficiency was tested beforehand according to Schmittgen and Livak ( 2008 ). Only RNA samples showing amplification in RT-qPCR were used for further analysis with PCR profiler arrays. For quality control purposes, yield 2 −ΔΔCt values from RT-qPCR and PCR profiler arrays were compared and had to be within the same range (Online Resource 1).
For performing the PCR profiler array, cDNA was synthesized from 1 µg RNA using the RT 2 First Strand Kit (Qiagen, Venlo, Netherlands) according to the manufacturer’s protocol with a GeneAmp® PCR System 9700 (Applied Biosystems, Darmstadt, Germany). Subsequently, the RT 2 Profiler™ PCR Array Human Molecular Toxicology Pathway Finder or Nephrotoxicity (Qiagen, Venlo, Netherlands) was conducted with RT 2 SYBR ® Green ROX qPCR Mastermix (Qiagen, Venlo, Netherlands) according to the manufacturer’s protocol. RT-qPCR was performed with an ABI 7900HT Fast Real-Time PCR system instrument (Applied Biosystems, Darmstadt, Germany), where activation of polymerase took place for 10 min at 95 °C, followed by 40 cycles of 15 s at 95 °C and 60 s at 60 °C and default melting curve analysis. Data were analyzed using 7900 software v241 and Excel 2021. C T was set to 0.2, melting curve was checked and manual baseline correction was performed. Yield C T -values were extracted and further analyzed.
- Pathway analysis
Further evaluation of PCR array data was performed with functional class scoring methods such as Gene Ontology (GO) enrichment and Kyoto Encyclopedia of Genes and Genomes (KEGG), as well as with the bioinformatics analysis and search tool Ingenuity Pathway Analysis Software (IPA). Following the manufacturer’s instructions, yield C T -values were uploaded to the Qiagen Gene Globe Webportal Footnote 1 and analyzed using the standard ΔΔC T method referring to an untreated control. A cut-off C T was set to 35, all 5 built-in housekeeping genes were manually selected as reference genes and their arithmetic mean used for normalization. Means of fold regulation and p-values were calculated and further evaluated with the bioinformatics tools following the protocol provided in Online Resource 2. The processed results from HepaRG cells and RPTEC were used as input data individually, as well as combined. For the combined analysis, duplicate genes that were present on both arrays were removed.
To generate a first overview, the percentage of differentially expressed genes (DEG) per pathway was determined as previously published (Heise et al. 2018 ). Genes were assorted to pathways as suggested on the manufacturer’s web page. Footnote 2 The percentage of DEG was calculated as number of genes whose expression significantly differed by a fold change of 2, as determined by Student’s t- test (p < 0.05), related to the total number of genes in the pathway.
GO enrichment and KEGG analysis
The freely available web tools GOrilla Footnote 3 and ShinyGO 0.80 Footnote 4 were used for GO enrichment and KEGG analysis, respectively (Eden et al. 2007 , 2009 ; Ge et al. 2020 ). Detailed protocols are provided in Online Resource 2 together with the R code for determining DEG and background genes (see Data availability), which was adapted from Feiertag et al. ( 2023 ).
Ingenuity pathway analysis
In addition to GO enrichment and KEGG analysis, further evaluation of PCR array data was performed with the bioinformatics analysis and search tool IPA (Qiagen, Hilden, Germany, analysis date: Nov. 2023) as previously published (Karaca et al. 2023b ). IPA is a commercial bioinformatics tool for analyzing RNA data, predicting pathway activation and functional interrelations using a curated pathway database. Using Fisher’s exact test, IPA identifies overrepresented pathways by measuring significant overlaps between user-provided gene lists and predefined gene sets. Means of fold regulation and p -values were uploaded to IPA following the protocol provided in Online Resource 2. Cut-off was set to – 1.5 and + 1.5 for fold regulation and 0.05 for the p -value. Fold regulation represents fold change results in a biologically meaningful way. In case the fold change is greater than 1, the fold regulation is equal to the fold change. For fold change values less than 1, the fold regulation is the negative inverse of the fold change. No further filtering was applied and an IPA core analysis was run. One Excel spread sheet per substance was obtained including all predicted diseases or functions annotations, the associated categories, the p-value of overlap as well as the number and names of the DEG found in the respective annotation (Online Resource 3). Predicted effects on other organs than the liver or the kidneys, such as heart or lungs, were discarded. For further comparison with in vivo data only the categories were used, combined with the p-value of the annotation, which was the highest.
Comparison with animal studies
The data obtained from targeted protein and transcriptomics analyses were compared with known in vivo observations from Draft Assessment Reports (DARs) of the pesticide active substances required for pesticide legislation. To facilitate the comparison of the data, the in vitro data was transformed into a more comprehensible form by applying evaluation matrices as shown in Table 3 .
The in vivo effects attributed to the pesticide active substances were taken from the publication by Nielsen et al. ( 2012 ). Additionally, the DARs of the two substances not reported in Nielsen et al . were analyzed and assigned accordingly. All in vivo effects identified by the authors for liver and kidneys can be found in Online Resource 1. Based on expert knowledge, descriptions of in vitro outcomes were combined with in vivo observations (see Tables 4 and 5 ).
Based on the combination of the in vitro and the in vivo data, it was possible to draw conclusions on the concordance of the predictions. In order to establish optimized thresholds for regarding an effect as in vitro positive, the analyses were performed by considering at least medium effects, strong and very strong effects, or very strong effects only (see Table 3 ) and comparing these to the corresponding in vivo effect. In case multiple in vitro predictors were connected to the same in vivo observation, a positive prediction from one was sufficient to be considered in vitro positive. For protein analyses, the comparison was performed for the data from HepaRG cells and RPTEC individually, as well as combined, where a positive prediction from one of the cell lines was considered sufficient and compared to hepatotoxic and nephrotoxic in vivo effects. For the gene transcription analysis, the categories obtained by IPA were compared to in vivo observations from DARs. A further evaluation integrating protein and transcriptional data was conducted, wherein a positive result from either data type was sufficient to classify a sample as in vitro positive. Online Resource 1 shows the combination of the results in detail. The percentage of concordance between in vitro prediction and in vivo observation was calculated. Indicative concordance was defined as percentage of in vivo positive observations that were predicted to be positive by the in vitro test system.
Statistical analysis
Statistical analysis was performed using R 4.2.1 and RStudio 2023.09.1 + 494. Data evaluation was done with Microsoft Excel 2021.
All experiments were performed in at least three independent biological replicates. Technical replicates, when applicable, were averaged and subsequently mean and standard deviation values were calculated from biological replicates. For targeted protein analysis, statistical significance was calculated with bootstrap technique using R package boot (Canty and Ripley 2016 ; Davidson and Hinkley 1997 ) to account for the high variability that results when the protein expression is affected. Data visualization was done using ggplot2 package (Wickham 2016 ). Calculation of statistical significance of altered gene transcription was performed using Student’s t -test, and R package ComplexHeatmaps was used for data visualization (Gu 2022 ). All R scripts can be found using the link provided in the Data availability section.
Impairment of cell viability
Each substance was tested for its effect on the viability of HepaRG cells and RPTEC. Based on these results, the highest non-cytotoxic concentration was determined and employed in further experiments together with a second concentration (i.e., 0.33 × highest non-cytotoxic concentration). For HepaRG cells, published data were used as a starting point for cytotoxicity testing and confirmed with WST-1 and NR uptake assays. The highest non-cytotoxic concentration, defined as the concentration determining a cell viability greater than 80%, is shown in Table 6 .
For RPTEC, a relatively new cell line, little data was available. At least 3 biological replicates were performed in technical triplicates to determine the highest non-cytotoxic concentrations (Table 6 ). The bar graphs in Online Resource 4 depict the concentration-dependent course of all tested concentrations per substance limited by solubility. Online Resource 1 provides a table with calculated approximations of substance concentrations in the target organ at LOAEL or NOEAL level based on in vivo toxicokinetic results from DARs. These approximations can be compared with the selected in vitro concentrations based on cytotoxicity experiments.
Effects on marker proteins
The result from multiplex microsphere-based sandwich immunoassays of treated HepaRG cells and RPTEC are shown in Figs. 1 and 2 , respectively. In HepaRG cells, incubation with the highest non-cytotoxic concentrations of Azoxystrobin, Chlorotoluron and Thiabendazole increased the expression of total LC3B, an indicator of autophagy, after 36 h (all three compounds) and 72 h (Chlorotoluron and Thiabendazole). Strong effects were observed on cleaved PARP, an indicator of apoptosis, after 36 h of incubation with 120 µM Cyproconazole (247 ± 147%) and 300 µM Thiabendazole (359 ± 204%). However, after 72 h incubation with 120 µM Cyproconazole, the level of cleaved PARP was strongly reduced. Expression of HIF 1-alpha, an indicator of hypoxia, was significantly increased after 36 h incubation with 45 µM Azoxystrobin (214 ± 24%). Fluxapyroxad and 2-Phenylphenol did not significantly increase the expression of any of the protein analytes.
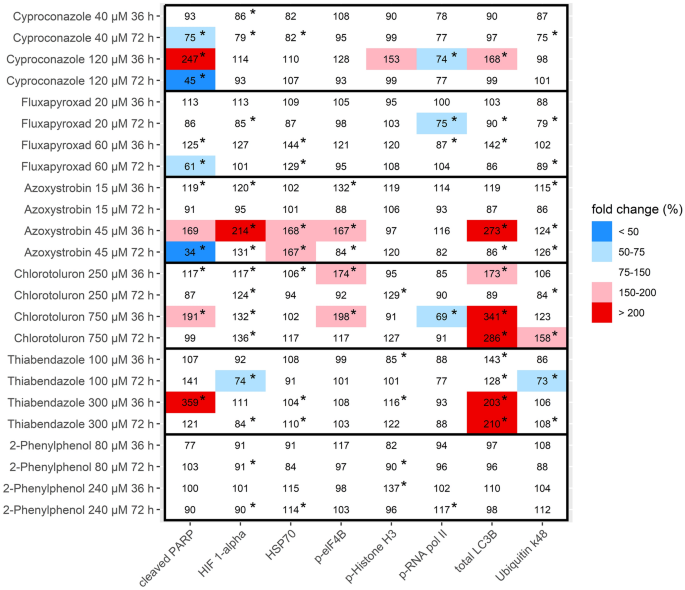
Effects on protein abundance and protein modification of key proteins observed in HepaRG cells after 36 and 72 h of incubation with the test substances using a multiplexed microsphere-based sandwich immunoassay panel. Results are shown as means of 3 independent experiments, normalized to solvent controls. Statistical differences to the solvent control were calculated with bootstrapping (* p < 0.05)
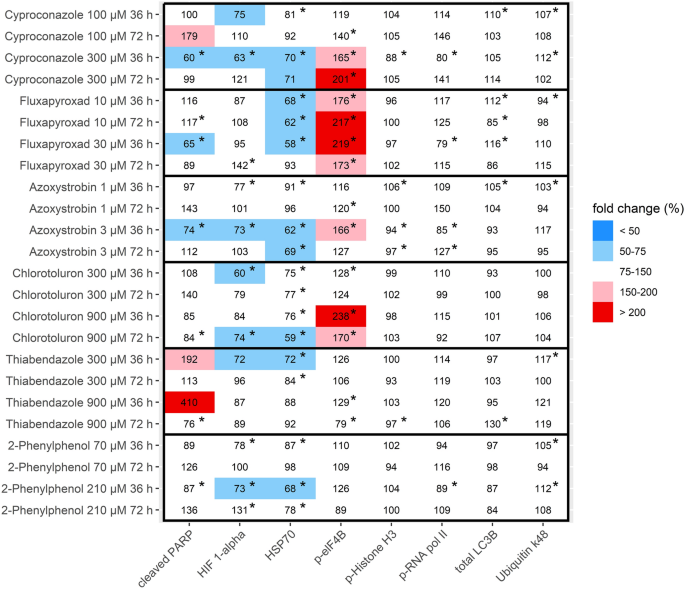
Effects on protein abundance and protein modification of key proteins in RPTEC after 36 and 72 h of incubation with the test substances using a multiplexed microsphere-based sandwich immunoassay panel. Results are shown as means of 3 independent experiments, normalized to solvent controls. Statistical differences to the solvent control were calculated with bootstrapping (* p < 0.05)
In RPTEC, the abundance of p-elF4B, involved in eukaryotic translation initiation, was increased after 36 and 72 h incubation with 300 µM Cyproconazole (165 ± 45% and 201 ± 51%, respectively), all conditions of Fluxapyroxad, incubation with 3 µM Azoxystrobin for 36 h (166 ± 56%) and incubation with 900 µM Chlorotoluron for 36 and 72 h (238 ± 59% and 170 ± 44%, respectively). Thiabendazole exposure for 36 h resulted in an increase of cleaved PARP at both tested concentrations. Due to the high standard deviation, these results were not statistically significant.
Comparing the results from HepaRG cells and RPTEC, fewer effects were observed in RPTEC than in HepaRG cells. Effects of Azoxystrobin and Chlorotoluron on p-elF4B were observed in both cell lines, as well as increased levels of cleaved PARP after Thiabendazole exposure; yet these results were only significant in HepaRG cells. 2-Phenylphenol did not increase the expression of any of the tested proteins in either cell line, while Fluxapyroxad only affected p-elF4B in RPTEC.
A graphical representation of all data points from HepaRG and RPTEC including means and standard deviations can be found in Online Resource 4.
Changes at the gene transcription level
Changes at the protein level are often preceded by changes at the gene expression level. These were analyzed by RT 2 Profiler™ PCR arrays. Figures 3 and 4 show the results from HepaRG cells and RPTEC, respectively. The genes included in the array were assigned to certain pathways according to the information provided on the manufacturer’s web page. For data interpretation, the percentage of DEG was calculated. In HepaRG cells, most DEG were observed following the exposure to Chlorotoluron. Overall, genes categorized as CYPs and phase I were predominantly affected. Cyproconazole and Chlorotoluron exerted effects on genes associated with fatty acid metabolism (10 and 55%, respectively). Of all steatosis-associated genes, 47% were altered by Chlorotoluron. With regards to individual genes, the strongest increase was observed for CYP1A1 and CYP1A2 , both in the group of CYPs and phase I, after exposure to Chlorotoluron (479-fold and 57-fold, respectively) and Thiabendazole (330-fold and 215-fold, respectively).
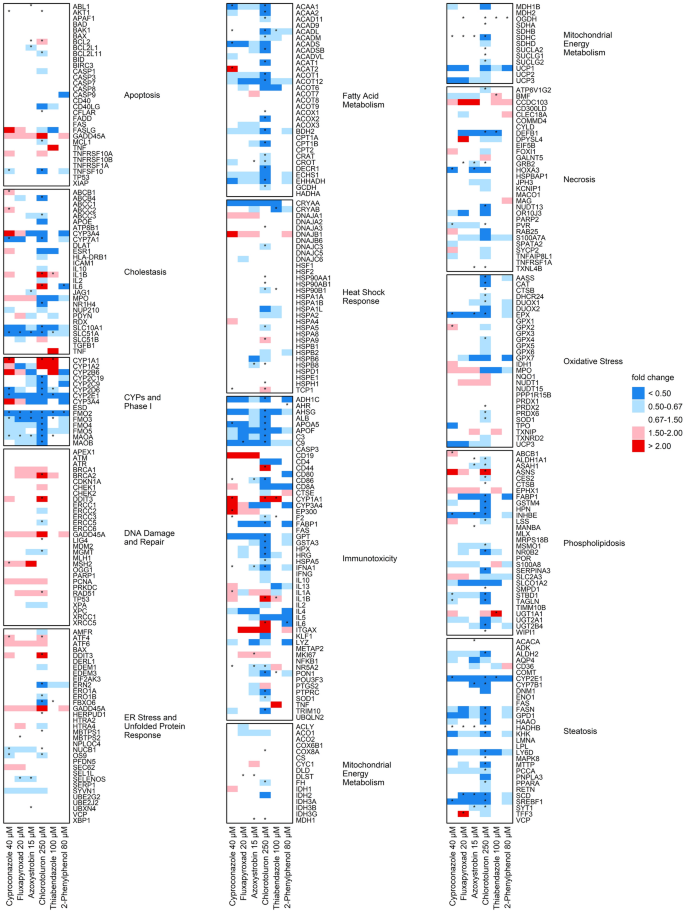
Relative quantities of mRNA transcript levels observed after 36 h exposure of HepaRG cells to non-cytotoxic concentrations of the test substances using the Human Molecular Toxicology Pathway Finder RT 2 Profiler™ PCR Array. Data evaluation was performed using the 2 −∆∆ Ct method, according to Livak and Schmittgen ( 2001 ). All target genes were normalized to 5 housekeeping genes. Results are shown as mean of 3 biological replicates and statistical analysis was performed by one sample Student’s t -test (* p < 0.05)
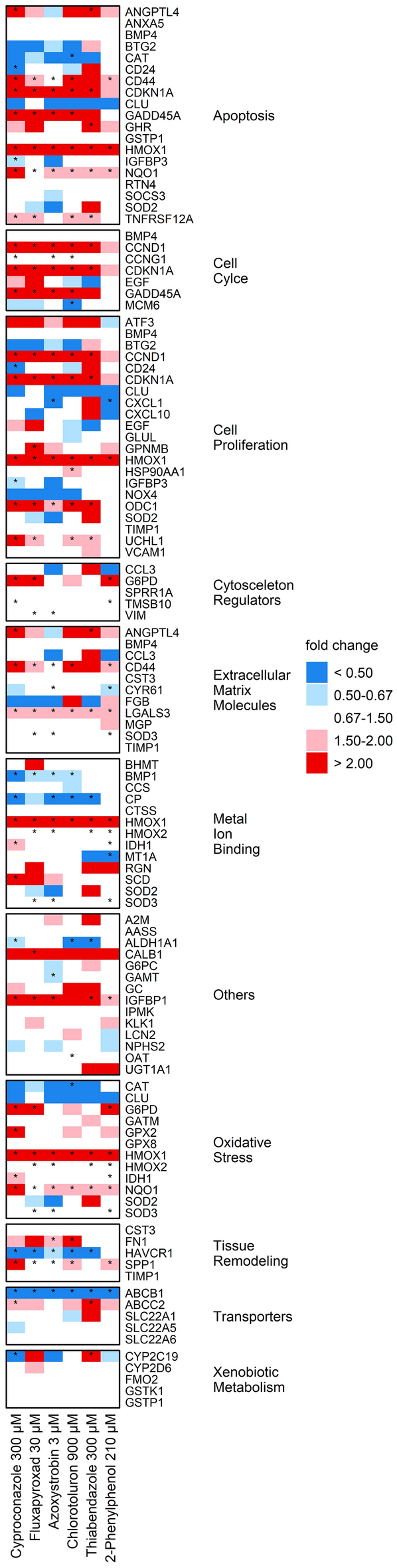
Relative quantities of mRNA transcript levels observed after 36 h exposure of RPTEC to non-cytotoxic concentrations of the test substances using the Human Nephrotoxicity RT 2 Profiler™ PCR Array. Data evaluation was performed using the 2 −∆∆ Ct method, according to Livak and Schmittgen ( 2001 ). All target genes were normalized to 5 housekeeping genes. Results are shown as mean of 3 biological replicates and statistical analysis was performed by one sample Student’s t -test (* p < 0.05)
In RPTEC, the cluster encompassing most of the DEG was that associated with regulation of the cell cycle. Here, Cyproconazole, Fluxapyroxad, Azoxystrobin, and Chlorotoluron affected the expression of over 40% of the associated genes. Genes associated with apoptosis were altered following the exposure to all substances, particularly Cyproconazole and Chlorotoluron (47 and 37%, respectively). Cyproconazole additionally showed pronounced effects on genes encoding for extracellular matrix and tissue remodeling molecules (27 and 40%, respectively). All substances affected about 20% of all genes contained in the group of genes related to cell proliferation. Cyproconazole, Chlorotoluron and 2-Phenylphenol affected 25% of all oxidative stress-associated genes. In comparison to HepaRG cells, where CYPs and phase I was the most impacted group, in RPTEC only one of the DEG established for any of the substances belonged to the group of xenobiotic metabolism. At the level of individual genes, HMOX1, a nephrotoxicity marker, was induced over twofold after incubation with all substances, but highest for Cyproconazole (eightfold). Of all genes, the strongest induction was observed for IGFBP1 , a member of the insulin-like growth factor-binding protein family, which was increased 53-fold by incubation with Cyproconazole and over 52-fold after incubation with Chlorotoluron.
A graphical representation of all data points including means and standard deviations can be found in Online Resource 4 for HepaRG and RPTEC results.
Data analysis with GO enrichment and KEGG analysis
Gene expression results were analyzed with GO enrichment and KEGG analysis. All effects obtained in the analyses can be found in Online Resource 3.
The GO enrichment analysis of HepaRG DEG from the incubation with Cyproconazole pointed at changes in secondary and xenobiotic metabolic processes , and the combined analysis additionally resulted in significant enrichment of response to estrogen . DEG modulated by the exposure to Chlorotoluron were involved in 16 ontologies including metabolic, biosynthetic, and catabolic processes , with lipid metabolic process and organic hydroxyl compound metabolic process being the most statistically supported (i.e., p-value: 9.2 × 10 –8 and 7.7 × 10 –7 , respectively). In RPTEC, nucleic acid metabolic process was the only significantly enriched GO term for Chlorotoluron, while the combined analysis revealed a total of 23. Analysis of DEG from incubation with Thiabendazole resulted, among others, in hits for xenobiotic, terpenoid, and isoprenoid metabolic process in HepaRG and combined results. Although analysis of DEG from incubation with 2-Phenylphenol did not result in significantly enriched GO terms from the HepaRG or the RPTEC data; the combined data set showed 5 enriched terms with NADP metabolic process and myeloid leukocyte migration having the lowest p-values (6.9 × 10 –4 , both).
For KEGG analysis, the HepaRG data set for Fluxapyroxad and Chlorotoluron showed enrichment of drug metabolism-cytochrome P450 , as well as taurine and hypotaurine metabolism (Fluxapyroxad) and metabolic pathways (Chlorotoluron). Thiabendazole data revealed enrichment of steroid hormone biosynthesis , metabolism of xenobiotics by cytochrome P450 and chemical carcinogenesis-DNA adducts . RPTEC data set for Azoxystrobin and Chlorotoluron showed multiple cancer-related pathways. The combined data set only resulted in few pathways: hepatocellular carcinoma for Azoxystrobin, metabolic pathways for Chlorotoluron and mineral absorption for 2-Phenylphenol. All other analyses did not result in any significant enrichment.
Data analysis with ingenuity pathway analysis software
Gene expression data were further analyzed with the IPA software. In total 32 different categories of diseases or functions were predicted. Figure 5 shows the ten most frequently resulting categories. Liver Hyperplasia/Hyperproliferation is the only common category across all cell lines and substances. The statistical confidence of the pathway analysis was strongest for Chlorotoluron, which also induced most DEG. Comparing the three methodologies of input data, lower p-values were observed for HepaRG and combined analysis and most categories of diseases or functions were predicted by the combined analysis. Evidently, effects on the kidney were predicted from the input data from liver cells and vice versa.
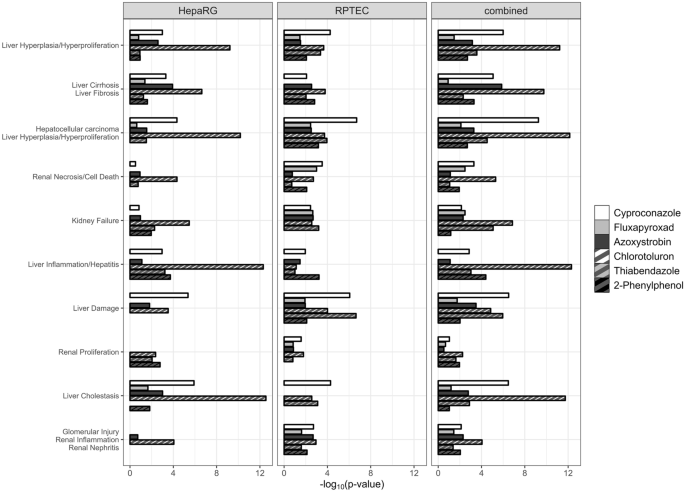
Results obtained by analysis of transcriptomics data with Qiagen Ingenuity Pathway Analysis. The 10 categories most affected are represented. The x-axis shows the -log 10 value of the p-value obtained for the respective effect
In a final step, the data acquired from targeted protein and transcriptomics analyses were compared with known in vivo observations. Given that the comparison focused on aligning the responses from human cell lines with whole animal data, the analysis focused on the extent to which the omics-responses were indicative of the respective biological response in vivo (indicative concordance). To establish an optimized threshold for the evaluation of in vitro predictions, the in vitro data were transformed by applying evaluation matrices as shown in Table 3 . Based on that, activated key proteins and thus cellular functions were identified for each substance from targeted protein analyses. For the evaluation of gene transcripts, the p-values for the categories obtained by IPA were considered. Indicative concordance with known in vivo results is shown in Table 7 .
For the protein analysis, the indicative concordance ranged from 18 to 47% for the single cell lines and their combination, respectively. In contrast to the results from targeted protein analyses, the indicative concordance for the transcriptomic response was much stronger with greatest values of 55, 63 and 76% for the single cell lines and their combination, respectively. Likewise, for those cases where no effect was seen in vivo, no adverse indications were seen in vitro in 80, 91 and 78% of cases, respectively. For protein analysis, this value ranged from 78 to 86% and was 50% for the combined analysis of protein and transcriptional data. It should be noted, however, that these values decreased when the evaluation criteria were less strict (medium or strong instead of very strong).
In the present study, the pathways triggered by non-cytotoxic concentrations of six pesticide active substances were examined, employing targeted protein and transcriptomics analyses in the liver cell line HepaRG and the kidney cell line RPTEC. Utilizing evaluation matrices and prediction software tools, the observed cellular responses were interpreted and compared with outcomes from established in vivo experiments, in order to assess the relevance of our in vitro model systems in predicting the impact of pesticide exposure on human hepatic and renal cellular function. The primary emphasis of this investigation did not lie in delineating discrete effects attributable to individual substances; rather, it centered on discerning the predictive capacity of the system and serving as a case study to highlight the current challenges in the regulatory adoption of NAMs.
When targeted protein data were used to predict in vivo impacts in rodents, the best result was achieved by the combined analysis and setting the evaluation criteria to medium effects (47%). Regarding the indicative concordance based on transcriptional data, medium effects in HepaRG cells seemed the most promising resulting in a 55% match. This is notable given the systemic as well as species differences between the corresponding test systems. It also highlights that the “gold standard”, i.e., the reference standard used for comparison, is in fact not necessarily indisputable (Trevethan 2017 ). Various studies pointed at the shortcomings of traditional animal studies, such as interspecies concordance, poor reproducibility and unsatisfactory extrapolation to humans (Goodman 2018 ; Karmaus et al. 2022 ; Luijten et al. 2020 ; Ly Pham et al. 2020; Smirnova et al. 2018 ; Wang and Gray 2015 ). One example illustrating the difficulties in extrapolating data from rodents to humans is the question whether Cyproconazole causes neoplasms in the liver. Here, animal studies with CD-1 mice showed statistically significant positive trends for hepatocellular adenomas and combined tumors in male mice (EFSA 2010 ; Hester et al. 2012 ). Ensuing studies identified CAR activation by Cyproconazole as the underlying Mode of Action (MoA) (Peffer et al. 2007 ). Marx-Stoelting et al. ( 2017 ) investigated effects of Cyproconazole in mice with humanized CAR and PXR and demonstrated increased sensitivity of rodents to CAR agonist-induced effects, compared to humanized mice. In line with these observations the Joint FAO/WHO Meeting on Pesticide Residues (JMPR) concluded that Cyproconazole is unlikely to pose a carcinogenic risk to humans (JMPR 2010 ). Likewise, Cyproconazole was not considered to cause neoplasms in the liver when analyzed for this study. However, such detailed analysis of a substance’s MoA is scarce.
Another important factor impeding the comparison of in vitro and in vivo data are the different ontologies. The need for harmonized ontologies and reporting formats of in vivo data has been expressed by many researchers in the field of in silico toxicology and has been addressed in multiple projects (Hardy et al. 2012 ; Sanz et al. 2017 ). For example, uncertainty arises as to the reason if and why an effect for a particular organ is possibly not reported. Depending on the case and study in question, this might be because absent effects were simply not explicitly reported as negative, or because other organ toxicities occurred at lower doses and hence data for the remaining organs were omitted or not assessed, or because the focus of the study was another organ (Smirnova et al. 2018 ). While this does not pose a problem for when such studies are used for risk assessment, it does affect the comparison with in vitro results. Another major obstacle is the retrospective conclusive combination of large and comprehensive sets of mechanistic data in vitro with systemic and histopathological observations in vivo. This issue has recently been picked up by on-going European ONTOX project Footnote 5 (“ontology-driven and artificial intelligence-based repeated dose toxicity testing of chemicals for next generation risk assessment “) and has led the consortium to reverse the strategy and build NAMs to predict systemic repeated dose toxicity effects to enable human risk assessment when combined with exposure assessment (Vinken et al. 2021 ). A recent publication by Jiang et al. ( 2023 ) as part of the ONTOX project identified transcriptomic signatures of drug-induced intrahepatic cholestasis with potential future use as prediction model. However, not all pathologies have been analyzed so far, and those that have were often only studied for a limited number of chemicals, limiting their transferability. Hence, this study relied on the use of computational tools such as IPA, GO enrichment and KEGG analysis, to draw functional conclusions from transcriptomics data. While IPA results in categorized diseases or functions annotations, KEGG and GO analyses display enriched ontologies. Therefore, while KEGG and GO results were too ambiguous to be related to distinct in vivo observations, it was feasible to combine IPA results with in vivo observations. It is noteworthy that even though GO enrichment and KEGG analysis seem fairly similar, the results varied widely between the predictions from the various software tools. Soh et al. ( 2010 ) analyzed consistency, comprehensiveness, and compatibility of pathway databases and made several crucial findings such as the inconsistency of associated genes across different databases pertaining to the same biological pathway. Furthermore, common biological pathways shared across different databases were frequently labeled with names that provided limited indication of their interrelationships. Chen et al. ( 2023 ) demonstrated that using the same gene list with different analysis methods may result in non-concordant overrepresented, enriched or perturbed pathways. Taken together, these considerations may explain the divergent findings from the different transcriptomics analyses in the present study. Additionally, these findings underscore the challenges associated with integrating pathway data from diverse sources and emphasize the need for standardized and cohesive representation of biological pathways in databases.
Compared to the transcriptomic data, protein analyses from HepaRG cells and RPTEC cells resulted in a comparatively low indicative concordance. This challenges the notion that protein analysis may be superior in prediction (Wu et al. 2023 ). One likely explanation is that proteins often reflect molecular functions and adverse effects more accurately, and diseases frequently involve dysregulated post-translational modifications, which are challenging to detect and may be poorly correlated with mRNA levels (Kannaiyan and Mahadevan 2018 ; Kelly et al. 2010 ; Zhao et al. 2020 ). However, due to the relatively low number of protein markers as compared to the number of mRNA markers, the targeted transcriptomics analysis is associated with a higher likelihood of finding a match. In the gene transcription analysis with ensuing IPA evaluation, 370 genes were analysed for HepaRG. In contrast, the protein analysis conducted in this study focussed on 8 proteins or modifications, each indicative of a particular cellular function, that were analysed at two time points after incubation of cells with two concentrations of the test substances. Consequently, a cellular response to a stressor over time can be observed, such as the different levels of cleaved PARP after 36 h and 72 h of incubation with Cyproconazole in HepaRG cells. While elevated levels of this apoptosis indicator were noted after 36 h, reduced levels were observed after 72 h. Possible explanations for this include a cellular feedback mechanism or an advanced stage of apoptosis.
Another central observation is that combination of cell lines and methods significantly increases indicative concordance (up to 88%). In the case of targeted protein analysis, combination of results led to an overall value of 47%, compared to approximately 20% for each cell line. Similar trends were observed for transcriptomic data with 76% indicative concordance for combined results, albeit decreasing the cases where an in vivo negative effect corresponded to no adverse indication seen in vitro , as the total number of positive in vitro effects was increased. Nonetheless, the idea that including omics data in regulatory process will unreasonably increase positive findings and lead to overprotectiveness can be challenged as strengthening the evaluation criteria lead to a reversion of this trend. The shortcomings of stand-alone in vitro tests to replace animal experiments have long been known. For example, single tests do not cover all possible outcomes of interest or all modes of action possibly causing a toxicological effect (Hartung et al. 2013 ; Rovida et al. 2015 ). In the present study, reported in vivo effects such as lesions of biliary epithelium or inflammation of the liver may not be fully represented by a single hepatic cell line. Hence, regulatory toxicologists strive to implement so-called integrated testing strategies (ITS) (Caloni et al. 2022 ). Results from projects in the fields of embryonic, developmental and reproductive, or acute oral toxicity have shown that test batteries increase the predictive value over individual assays (Piersma et al. 2013 ; Prieto et al. 2013 ; Sogorb et al. 2014 ). To share these novel methodologies in ITS for safety evaluations in the regulatory context, the OECD Integrated Approaches for Testing and Assessment (IATA) Case Studies Project offers a platform where comprehensive information on case studies, such as consideration documents capturing learnings and lessons from the review experience, can be found. Footnote 6
While this publication’s scope did not extend to establishing a conclusive ITS for liver and kidney toxicity, it serves as a valuable starting point for future analyses in this direction and offers ongoing assistance and insights. Moving forward, it could prove beneficial when exploring testing protocols that integrate protein and transcriptomics analyses, enhancing the comprehensiveness of safety evaluations in this domain.
Data availability
The data sets generated during the current study are available in the Jochum-et-al-2024 GitHub repository, https://github.com/KristinaJochum/Jochum-et-al-2024 .
https://geneglobe.qiagen.com/de/analyze , accessed last: 23.02.2024.
https://geneglobe.qiagen.com/us/product-groups/rt2-profiler-pcr-arrays , accessed last: 23.02.2024.
https://cbl-gorilla.cs.technion.ac.il/ , accessed last: 16.01.2024.
http://bioinformatics.sdstate.edu/go/ , accessed last: 16.01.2024.
https://ontox-project.eu/ , accessed last 26.02.2024.
https://www.oecd.org/chemicalsafety/risk-assessment/iata/ , accessed last: 26.04.2024.
Andersson TB, Kanebratt KP, Kenna JG (2012) The HepaRG cell line: a unique in vitro tool for understanding drug metabolism and toxicology in human. Expert Opin Drug Metab Toxicol 8(7):909–920. https://doi.org/10.1517/17425255.2012.685159
Article CAS PubMed Google Scholar
Andonegui-Elguera MA, Caceres-Gutierrez RE, Lopez-Saavedra A et al (2022) The Roles of Histone Post-Translational Modifications in the Formation and Function of a Mitotic Chromosome. Int J Mol Sci 23(15):8704. https://doi.org/10.3390/ijms23158704
Article CAS PubMed PubMed Central Google Scholar
Aninat C, Piton A, Glaise D et al (2006) Expression of cytochromes P450, conjugating enzymes and nuclear receptors in human hepatoma HepaRG cells. Drug Metab Dispos 34(1):75–83. https://doi.org/10.1124/dmd.105.006759
Antherieu S, Chesne C, Li R et al (2010) Stable expression, activity, and inducibility of cytochromes P450 in differentiated HepaRG cells. Drug Metab Dispos 38(3):516–525. https://doi.org/10.1124/dmd.109.030197
Antherieu S, Chesne C, Li R, Guguen-Guillouzo C, Guillouzo A (2012) Optimization of the HepaRG cell model for drug metabolism and toxicity studies. Toxicol in Vitro 26(8):1278–1285. https://doi.org/10.1016/j.tiv.2012.05.008
Aschauer L, Gruber LN, Pfaller W et al (2013) Delineation of the key aspects in the regulation of epithelial monolayer formation. Mol Cell Biol 33(13):2535–2550. https://doi.org/10.1128/MCB.01435-12
Aschauer L, Limonciel A, Wilmes A, et al. (2015) Application of RPTEC/TERT1 cells for investigation of repeat dose nephrotoxicity: A transcriptomic study. Toxicol In Vitro 30(1 Pt A):106–16 https://doi.org/10.1016/j.tiv.2014.10.005
Ashraf M, Asghar M, Rong Y, Doschak M, Kiang T (2018) Advanced In Vitro HepaRG Culture Systems for Xenobiotic Metabolism and Toxicity Characterization. Eur J Drug Metab Pharmacokinet 44:437–458. https://doi.org/10.1007/s13318-018-0533-3
Article CAS Google Scholar
Buesen R, Visan A, Genschow E, Slawik B, Spielmann H, Seiler A (2004) Trends in improving the embryonic stem cell test (EST): an overview. Altex 21(1):15–22
PubMed Google Scholar
Caloni F, De Angelis I, Hartung T (2022) Replacement of animal testing by integrated approaches to testing and assessment (IATA): a call for in vivitrosi. Arch Toxicol 96(7):1935–1950. https://doi.org/10.1007/s00204-022-03299-x
Canty A, Ripley B (2016) boot: Bootstrap R (S-Plus) Functions. R Package Version 1:3–18
Google Scholar
Chen JW, Shrestha L, Green G, Leier A, Marquez-Lago TT (2023) The hitchhikers' guide to RNA sequencing and functional analysis. Brief Bioinform 24(1):bbac529 https://doi.org/10.1093/bib/bbac529
Davidson AC, Hinkley DV (1997) Bootstrap Methods and Their Applications. Cambridge University Press, Cambridge
Book Google Scholar
Duncan RF, Hershey JW (1989) Protein synthesis and protein phosphorylation during heat stress, recovery, and adaptation. J Cell Biol 109(4 Pt 1):1467–1481. https://doi.org/10.1083/jcb.109.4.1467
EC (2006) Regulation (EC) No 1907/2006 concerning the Registration, Evaluation, Authorisation and Restriction of Chemicals (REACH), establishing a European Chemicals Agency. In: Parliament E (ed) 1907/2006. Official Journal of the European Union, p 396/1
EC (2009) Regulation (EC) No 1107/2009 of the European Parliament and of the council of 21 October 2009 concerning the placing of plant protection products on the market and repealing Council Directives 79/117/EEC and 91/414/EEC. In: EC (ed) 1107/2009. Official Journal of the European Union
EC (2015) Opinion on o-Phenylphenol, Sodium o-phenylphenate and Potassium o-phenylphenate. European Commission, Directorate-General for Health, Food Safety
EURL ECVAM (2012) Multi-study validation trial for cytochrome P450 induction providing a reliable human metabolically competent standard model or method using the human cryopreserved primary hepatocytes and the human cryopreserved HepaRG cell line. European commission joint research center, p 164
Eden E, Lipson D, Yogev S, Yakhini Z (2007) Discovering motifs in ranked lists of DNA sequences. PLoS Comput Biol 3(3):e39. https://doi.org/10.1371/journal.pcbi.0030039
Eden E, Navon R, Steinfeld I, Lipson D, Yakhini Z (2009) GOrilla: a tool for discovery and visualization of enriched GO terms in ranked gene lists. BMC Bioinformatics 10:48. https://doi.org/10.1186/1471-2105-10-48
Article PubMed PubMed Central Google Scholar
EFSA (2010) Conclusion on the peer review of the pesticide risk assessment of the active substance cyproconazole. EFSA J 8(11):1897. https://doi.org/10.2903/j.efsa.2010.1897
US EPA (2002) Reregistration Eligibility Decision Thiabendazole. In: US EPA OP, Pesticides and Toxic Substances (ed) Prevention, Pesticides and Toxic Substances. Washington D.C.
US EPA (2018) Strategic Plan to Promote the Development and Implementation of Alternative Test Methods Within the TSCA Program. In: U.S. Environmental Protection Agency OoCSaP, Prevention (eds). Washington, DC
Feiertag K, Karaca M, Fischer B, et al. (2023) Mixture effects of co-formulants and two plant protection products in a liver cell line. EXCLI J 22:221–236 https://doi.org/10.17179/excli2022-5648
Franzosa JA, Bonzo JA, Jack J et al (2021) High-throughput toxicogenomic screening of chemicals in the environment using metabolically competent hepatic cell cultures. NPJ Syst Biol Appl 7(1):7. https://doi.org/10.1038/s41540-020-00166-2
French ME, Koehler CF, Hunter T (2021) Emerging functions of branched ubiquitin chains. Cell Discov 7(1):6. https://doi.org/10.1038/s41421-020-00237-y
Gao A-H, Fu Y-Y, Zhang K-Z et al (1840) (2014) Azoxystrobin, a mitochondrial complex III Qo site inhibitor, exerts beneficial metabolic effects in vivo and in vitro. Biochim Biophys Acta 7:2212–2221. https://doi.org/10.1016/j.bbagen.2014.04.002
Ge SX, Jung D, Yao R (2020) ShinyGO: a graphical gene-set enrichment tool for animals and plants. Bioinformatics 36(8):2628–2629. https://doi.org/10.1093/bioinformatics/btz931
Genschow E, Spielmann H, Scholz G et al (2004) Validation of the embryonic stem cell test in the international ECVAM validation study on three in vitro embryotoxicity tests. Altern Lab Anim 32(3):209–244. https://doi.org/10.1177/026119290403200305
Goodman JI (2018) Goodbye to the Bioassay Toxicol Res (camb) 7(4):558–564. https://doi.org/10.1039/c8tx00004b
Gu Z (2022) Complex heatmap visualization. iMeta 1(3) https://doi.org/10.1002/imt2.43
Hardy B, Apic G, Carthew P, et al. (2012) Toxicology Ontology Perspectives. ALTEX https://doi.org/10.14573/altex.2012.2.139
Hart SN, Li Y, Nakamoto K, Subileau EA, Steen D, Zhong XB (2010) A comparison of whole genome gene expression profiles of HepaRG cells and HepG2 cells to primary human hepatocytes and human liver tissues. Drug Metab Dispos 38(6):988–994. https://doi.org/10.1124/dmd.109.031831
Hartman GD, Kuduk SD, Espiritu C, Lam AM (2020) P450s under Restriction (PURE) Screen Using HepaRG and Primary Human Hepatocytes for Discovery of Novel HBV Antivirals. ACS Med Chem Lett 11(10):1919–1927. https://doi.org/10.1021/acsmedchemlett.9b00630
Hartung T, Luechtefeld T, Maertens A, Kleensang A (2013) Integrated testing strategies for safety assessments. ALTEX 30(1):3–18 https://doi.org/10.14573/altex.2013.1.003
Heise T, Schmidt F, Knebel C et al (2018) Hepatotoxic combination effects of three azole fungicides in a broad dose range. Arch Toxicol 92(2):859–872. https://doi.org/10.1007/s00204-017-2087-6
Herzler M, Marx-Stoelting P, Pirow R et al (2021) The “EU chemicals strategy for sustainability” questions regulatory toxicology as we know it: is it all rooted in sound scientific evidence? Arch Toxicol 95(7):2589–2601. https://doi.org/10.1007/s00204-021-03091-3
Hester S, Moore T, Padgett WT, Murphy L, Wood CE, Nesnow S (2012) The hepatocarcinogenic conazoles: cyproconazole, epoxiconazole, and propiconazole induce a common set of toxicological and transcriptional responses. Toxicol Sci 127(1):54–65. https://doi.org/10.1093/toxsci/kfs086
Jackson RJ, Hellen CU, Pestova TV (2010) The mechanism of eukaryotic translation initiation and principles of its regulation. Nat Rev Mol Cell Biol 11(2):113–127. https://doi.org/10.1038/nrm2838
Jiang J, van Ertvelde J, Ertaylan G et al (2023) Unraveling the mechanisms underlying drug-induced cholestatic liver injury: identifying key genes using machine learning techniques on human in vitro data sets. Arch Toxicol 97(11):2969–2981. https://doi.org/10.1007/s00204-023-03583-4
JMPR (2010) Pesticide residues in food 2010. Report of the Joint Meeting of the FAO Panel of Experts on Pesticide Residues in Food and the Environment and the WHO Core Assessment Group on Pesticide Residues FAO Plant Production and Protection Paper. vol 200, Rome
Kannaiyan R, Mahadevan D (2018) A comprehensive review of protein kinase inhibitors for cancer therapy. Expert Rev Anticancer Ther 18(12):1249–1270. https://doi.org/10.1080/14737140.2018.1527688
Karaca M, Fritsche K, Lichtenstein D et al (2023a) Adverse outcome pathway-based analysis of liver steatosis in vitro using human liver cell lines. STAR Protoc 4(3):102500. https://doi.org/10.1016/j.xpro.2023.102500
Karaca M, Willenbockel CT, Tralau T, Bloch D, Marx-Stoelting P (2023b) Toxicokinetic and toxicodynamic mixture effects of plant protection products: A case study. Regul Toxicol Pharmacol 141:105400. https://doi.org/10.1016/j.yrtph.2023.105400
Karmaus AL, Mansouri K, To KT et al (2022) Evaluation of Variability Across Rat Acute Oral Systemic Toxicity Studies. Toxicol Sci 188(1):34–47. https://doi.org/10.1093/toxsci/kfac042
Kelly TK, De Carvalho DD, Jones PA (2010) Epigenetic modifications as therapeutic targets. Nat Biotechnol 28(10):1069–1078. https://doi.org/10.1038/nbt.1678
Kiang JG, Tsokos GC (1998) Heat shock protein 70 kDa: molecular biology, biochemistry, and physiology. Pharmacol Ther 80(2):183–201. https://doi.org/10.1016/s0163-7258(98)00028-x
Landsiedel R, Birk B, Funk-Weyer D (2022) The Evolution of Regulatory Toxicology: Where is the Gardener? Altern Lab Anim 50(4):255–262. https://doi.org/10.1177/02611929221107617
Article PubMed Google Scholar
Lee KA, Roth RA, LaPres JJ (2007) Hypoxia, drug therapy and toxicity. Pharmacol Ther 113(2):229–246. https://doi.org/10.1016/j.pharmthera.2006.08.001
Lichtenstein D, Mentz A, Schmidt FF et al (2020) Transcript and protein marker patterns for the identification of steatotic compounds in human HepaRG cells. Food Chem Toxicol 145:111690. https://doi.org/10.1016/j.fct.2020.111690
Lichtenstein D, Mentz A, Sprenger H et al (2021) A targeted transcriptomics approach for the determination of mixture effects of pesticides. Toxicology 460:152892. https://doi.org/10.1016/j.tox.2021.152892
Livak KJ, Schmittgen TD (2001) Analysis of relative gene expression data using real-time quantitative PCR and the 2(-Delta Delta C(T)) Method. Methods 25(4):402–408. https://doi.org/10.1006/meth.2001.1262
Luckert C, Braeuning A, de Sousa G et al (2018) Adverse Outcome Pathway-Driven Analysis of Liver Steatosis in Vitro: A Case Study with Cyproconazole. Chem Res Toxicol 31(8):784–798. https://doi.org/10.1021/acs.chemrestox.8b00112
Luijten M, Corvi R, Mehta J et al (2020) A comprehensive view on mechanistic approaches for cancer risk assessment of non-genotoxic agrochemicals. Regul Toxicol Pharmacol 118:104789. https://doi.org/10.1016/j.yrtph.2020.104789
Ly Pham L, Watford S, Pradeep P et al (2020) Variability in in vivo studies: defining the upper limit of performance for predictions of systemic effect levels. Comput Toxicol 15:1–100126. https://doi.org/10.1016/j.comtox.2020.100126
Marx-Stoelting P, Ganzenberg K, Knebel C et al (2017) Hepatotoxic effects of cyproconazole and prochloraz in wild-type and hCAR/hPXR mice. Arch Toxicol 91(8):2895–2907. https://doi.org/10.1007/s00204-016-1925-2
Marx-Stoelting P, Adriaens E, Ahr HJ, et al. (2009) A review of the implementation of the embryonic stem cell test (EST). The report and recommendations of an ECVAM/ReProTect Workshop. Altern Lab Anim 37(3):313–328 https://doi.org/10.1177/026119290903700314
Mennecozzi M, Landesmann B, Harris GA, Liska R, Whelan M (2012) Hepatotoxicity screening taking a mode-of-action approach using HepaRG cells and HCA. Altex Proc 1(12):193–204 https://doi.org/10.58847/ap.1201
Mizutani T, Ito K, Nomura H, Nakanishi K (1990) Nephrotoxicity of Thiabendazole in mice depleted of glutathione by treatment with DL-buthionine sulphoximine. Food Chem Toxicol 28:169–177. https://doi.org/10.1016/0278-6915(90)90005-8
Muniz L, Nicolas E, Trouche D (2021) RNA polymerase II speed: a key player in controlling and adapting transcriptome composition. EMBO J 40(15):e105740 https://doi.org/10.15252/embj.2020105740
Nelson DM, Ye X, Hall C et al (2002) Coupling of DNA synthesis and histone synthesis in S phase independent of cyclin/cdk2 activity. Mol Cell Biol 22(21):7459–7472. https://doi.org/10.1128/MCB.22.21.7459-7472.2002
Nielsen E, Nørhede P, Boberg J, et al. (2012) Identification of Cumulative Assessment Groups of Pesticides. EFSA Support Publ 9(4) https://doi.org/10.2903/sp.efsa.2012.EN-269
OECD (2019) Determination of Cytochrome P450 (CYP) enzyme activity induction using differentiated human hepatic cells.
Ohtake F (2022) Branched ubiquitin code: from basic biology to targeted protein degradation. J Biochem 171(4):361–366. https://doi.org/10.1093/jb/mvac002
Oliver FJ, de la Rubia G, Rolli V, Ruiz-Ruiz MC, de Murcia G, Murcia JM (1998) Importance of poly(ADP-ribose) polymerase and its cleavage in apoptosis. Lesson from an uncleavable mutant. J Biol Chem 273(50):33533–9 https://doi.org/10.1074/jbc.273.50.33533
Ozawa S, Ohta K, Miyajima A et al (2000) Metabolic activation of o-phenylphenol to a major cytotoxic metabolite, phenylhydroquinone: role of human CYP1A2 and rat CYP2C11/CYP2E1. Xenobiotica 30(10):1005–1017. https://doi.org/10.1080/00498250050200159
Peffer RC, Moggs JG, Pastoor T et al (2007) Mouse liver effects of cyproconazole, a triazole fungicide: role of the constitutive androstane receptor. Toxicol Sci 99(1):315–325. https://doi.org/10.1093/toxsci/kfm154
Piersma AH, Bosgra S, van Duursen MB et al (2013) Evaluation of an alternative in vitro test battery for detecting reproductive toxicants. Reprod Toxicol 38:53–64. https://doi.org/10.1016/j.reprotox.2013.03.002
Prieto P, Kinsner-Ovaskainen A, Stanzel S et al (2013) The value of selected in vitro and in silico methods to predict acute oral toxicity in a regulatory context: results from the European Project ACuteTox. Toxicol in Vitro 27(4):1357–1376. https://doi.org/10.1016/j.tiv.2012.07.013
Reggiori F, Klionsky DJ (2002) Autophagy in the eukaryotic cell. Eukaryot Cell 1(1):11–21. https://doi.org/10.1128/EC.01.1.11-21.2002
Repetto G, del Peso A, Zurita JL (2008) Neutral red uptake assay for the estimation of cell viability/cytotoxicity. Nat Protoc 3(7):1125–1131. https://doi.org/10.1038/nprot.2008.75
Rovida C, Alepee N, Api AM, et al. (2015) Integrated Testing Strategies (ITS) for safety assessment. ALTEX 32(1):25–40 https://doi.org/10.14573/altex.1411011
Sanz F, Pognan F, Steger-Hartmann T et al (2017) Legacy data sharing to improve drug safety assessment: the eTOX project. Nat Rev Drug Discov 16(12):811–812. https://doi.org/10.1038/nrd.2017.177
Schenk B, Weimer M, Bremer S et al (2010) The ReProTect Feasibility Study, a novel comprehensive in vitro approach to detect reproductive toxicants. Reprod Toxicol 30(1):200–218. https://doi.org/10.1016/j.reprotox.2010.05.012
Schmeisser S, Miccoli A, von Bergen M et al (2023) New approach methodologies in human regulatory toxicology - Not if, but how and when! Environ Int 178:108082. https://doi.org/10.1016/j.envint.2023.108082
Schmittgen TD, Livak KJ (2008) Analyzing real-time PCR data by the comparative C(T) method. Nat Protoc 3(6):1101–1108. https://doi.org/10.1038/nprot.2008.73
Seiler AE, Buesen R, Visan A, Spielmann H (2006) Use of murine embryonic stem cells in embryotoxicity assays: the embryonic stem cell test. Methods Mol Biol 329:371–395. https://doi.org/10.1385/1-59745-037-5:371
Shah H, Patel M, Shrivastava N (2017) Gene expression study of phase I and II metabolizing enzymes in RPTEC/TERT1 cell line: application in in vitro nephrotoxicity prediction. Xenobiotica 47(10):837–843. https://doi.org/10.1080/00498254.2016.1236299
Simon BR, Wilson MJ, Wickliffe JK (2014) The RPTEC/TERT1 cell line models key renal cell responses to the environmental toxicants, benzo[a]pyrene and cadmium. Toxicol Rep 1:231–242. https://doi.org/10.1016/j.toxrep.2014.05.010
Smirnova L, Kleinstreuer N, Corvi R, Levchenko A, Fitzpatrick SC, Hartung T (2018) 3S - Systematic, systemic, and systems biology and toxicology. ALTEX 35(2):139–162 https://doi.org/10.14573/altex.1804051
Sogorb MA, Pamies D, de Lapuente J, Estevan C, Estevez J, Vilanova E (2014) An integrated approach for detecting embryotoxicity and developmental toxicity of environmental contaminants using in vitro alternative methods. Toxicol Lett 230(2):356–367. https://doi.org/10.1016/j.toxlet.2014.01.037
Soh D, Dong D, Guo Y, Wong L (2010) Consistency, comprehensiveness, and compatibility of pathway databases. BMC Bioinformatics 11:449. https://doi.org/10.1186/1471-2105-11-449
Tamura K, Inoue K, Takahashi M et al (2013) Dose-response involvement of constitutive androstane receptor in mouse liver hypertrophy induced by triazole fungicides. Toxicol Lett 221(1):47–56. https://doi.org/10.1016/j.toxlet.2013.05.011
Trevethan R (2017) Sensitivity, Specificity, and Predictive Values: Foundations, Pliabilities, and Pitfalls in Research and Practice. Front Public Health 5:307. https://doi.org/10.3389/fpubh.2017.00307
Vinken M (2015) Adverse Outcome Pathways and Drug-Induced Liver Injury Testing. Chem Res Toxicol 28(7):1391–1397. https://doi.org/10.1021/acs.chemrestox.5b00208
Vinken M, Benfenati E, Busquet F et al (2021) Safer chemicals using less animals: kick-off of the European ONTOX project. Toxicology 458:152846. https://doi.org/10.1016/j.tox.2021.152846
Wang B, Gray G (2015) Concordance of Noncarcinogenic Endpoints in Rodent Chemical Bioassays. Risk Anal 35(6):1154–1166. https://doi.org/10.1111/risa.12314
WHO (1996) Guidlines for drinking-water quality. In: Water S, Hygiene and Health (ed) Health criteria and other supporting information. vol 2, 2 edn, Geneva
Wickham H (2016) ggplot2: Elegant Graphics for Data Analysis, 2nd edn. Springer Cham, New York
Wieser M, Stadler G, Jennings P et al (2008) hTERT alone immortalizes epithelial cells of renal proximal tubules without changing their functional characteristics. Am J Physiol Renal Physiol 295(5):F1365–F1375. https://doi.org/10.1152/ajprenal.90405.2008
Wu Y, Liu Q, Xie L (2023) Hierarchical multi-omics data integration and modeling predict cell-specific chemical proteomics and drug responses. Cell Rep Methods 3(4):100452. https://doi.org/10.1016/j.crmeth.2023.100452
Zahn E, Wolfrum J, Knebel C et al (2018) Mixture effects of two plant protection products in liver cell lines. Food Chem Toxicol 112:299–309. https://doi.org/10.1016/j.fct.2017.12.067
Zhao W, Li J, Chen MM, et al. (2020) Large-Scale Characterization of Drug Responses of Clinically Relevant Proteins in Cancer Cell Lines. Cancer Cell 38(6):829–843 e4 https://doi.org/10.1016/j.ccell.2020.10.008
Download references
Open Access funding enabled and organized by Projekt DEAL. This project was supported by BfR grant no. 1322–794.
Author information
Authors and affiliations.
Department of Pesticides Safety, German Federal Institute for Risk Assessment, Berlin, Germany
Kristina Jochum, Andrea Miccoli, Tewes Tralau & Philip Marx-Stoelting
Institute for Marine Biological Resources and Biotechnology (IRBIM), National Research Council, Ancona, Italy
Andrea Miccoli
Signatope GmbH, Tübingen, Germany
Cornelia Sommersdorf & Oliver Poetz
NMI Natural and Medical Sciences Institute at the University of Tübingen, Reutlingen, Germany
Oliver Poetz
Department of Food Safety, German Federal Institute for Risk Assessment, Berlin, Germany
Andrea Miccoli & Albert Braeuning
You can also search for this author in PubMed Google Scholar
Contributions
Conceptualization: Oliver Poetz, Albert Braeuning, Philip Marx-Stoelting, Tewes Tralau; methodology: Kristina Jochum, Philip Marx-Stoelting, Oliver Poetz; formal analysis and investigation: Kristina Jochum, Andrea Miccoli, Cornelia Sommersdorf; writing—original draft preparation: Kristina Jochum, Philip Marx-Stoelting; writing—review and editing: Andrea Miccoli, Cornelia Sommersdorf, Oliver Poetz, Albert Braeuning, Tewes Tralau, Philip Marx-Stoelting; funding acquisition: Tewes Tralau, Philip Marx-Stoelting; resources: Tewes Tralau, Philip Marx-Stoelting, Oliver Poetz.
Corresponding author
Correspondence to Philip Marx-Stoelting .
Ethics declarations
Conflict of interest.
Oliver Poetz is a shareholder of SIGNATOPE GmbH. Cornelia Sommersdorf is an employee at SIGNATOPE GmbH. SIGNATOPE offers assay development and service using immunoassay technology.
Additional information
Publisher's note.
Springer Nature remains neutral with regard to jurisdictional claims in published maps and institutional affiliations.
Supplementary Information
Below is the link to the electronic supplementary material.
Supplementary file1 (PDF 828 KB)
Supplementary file2 (pdf 250 kb), supplementary file3 (xlsx 295 kb), supplementary file4 (pdf 4573 kb), rights and permissions.
Open Access This article is licensed under a Creative Commons Attribution 4.0 International License, which permits use, sharing, adaptation, distribution and reproduction in any medium or format, as long as you give appropriate credit to the original author(s) and the source, provide a link to the Creative Commons licence, and indicate if changes were made. The images or other third party material in this article are included in the article's Creative Commons licence, unless indicated otherwise in a credit line to the material. If material is not included in the article's Creative Commons licence and your intended use is not permitted by statutory regulation or exceeds the permitted use, you will need to obtain permission directly from the copyright holder. To view a copy of this licence, visit http://creativecommons.org/licenses/by/4.0/ .
Reprints and permissions
About this article
Jochum, K., Miccoli, A., Sommersdorf, C. et al. Comparative case study on NAMs: towards enhancing specific target organ toxicity analysis. Arch Toxicol (2024). https://doi.org/10.1007/s00204-024-03839-7
Download citation
Received : 03 July 2024
Accepted : 08 August 2024
Published : 29 August 2024
DOI : https://doi.org/10.1007/s00204-024-03839-7
Share this article
Anyone you share the following link with will be able to read this content:
Sorry, a shareable link is not currently available for this article.
Provided by the Springer Nature SharedIt content-sharing initiative
- Gene enrichment
- Find a journal
- Publish with us
- Track your research

An official website of the United States government
The .gov means it’s official. Federal government websites often end in .gov or .mil. Before sharing sensitive information, make sure you’re on a federal government site.
The site is secure. The https:// ensures that you are connecting to the official website and that any information you provide is encrypted and transmitted securely.
- Publications
- Account settings
Preview improvements coming to the PMC website in October 2024. Learn More or Try it out now .
- Advanced Search
- Journal List
- CBE Life Sci Educ
- v.15(4); Winter 2016
A Case Study Documenting the Process by Which Biology Instructors Transition from Teacher-Centered to Learner-Centered Teaching
Gili marbach-ad.
† College of Computer, Mathematical and Natural Sciences, University of Maryland, College Park, MD 20742
Carly Hunt Rietschel
‡ College of Education, University of Maryland, College Park, MD 20742
Associated Data
A case study approach was used to obtain an in-depth understanding of the change process of two university instructors who were involved with redesigning a biology course to implement learner-centered teaching. Implications for instructors wishing to transform their teaching and for administrators who wish to support them are provided.
In this study, we used a case study approach to obtain an in-depth understanding of the change process of two university instructors who were involved with redesigning a biology course. Given the hesitancy of many biology instructors to adopt evidence-based, learner-centered teaching methods, there is a critical need to understand how biology instructors transition from teacher-centered (i.e., lecture-based) instruction to teaching that focuses on the students. Using the innovation-decision model for change, we explored the motivation, decision-making, and reflective processes of the two instructors through two consecutive, large-enrollment biology course offerings. Our data reveal that the change process is somewhat unpredictable, requiring patience and persistence during inevitable challenges that arise for instructors and students. For example, the change process requires instructors to adopt a teacher-facilitator role as opposed to an expert role, to cover fewer course topics in greater depth, and to give students a degree of control over their own learning. Students must adjust to taking responsibility for their own learning, working collaboratively, and relinquishing the anonymity afforded by lecture-based teaching. We suggest implications for instructors wishing to change their teaching and administrators wishing to encourage adoption of learner-centered teaching at their institutions.
This is the analogy I thought of, the first semester was where you drop a ball on a hard floor, and at first it bounces really high, then the next bounce is a little lower, hopefully it’s going to be a dampened thing, where we make fewer and fewer changes. Alex
It seems to take a village to send a course in a new direction!! Julie

INTRODUCTION
This study documents the process by which instructors transition from teacher-centered instruction to emphasizing learner-centered teaching in an introductory biology course. Weimer (2013 ) defines teacher-centered instruction as lecture-based teaching wherein students are “passive recipients of knowledge” (p. 64). She characterizes learner-centered teaching as “teaching focused on learning—what the students are doing is the central concern of the teacher” (p. 15). Weimer delineates five principles of learner-centered teaching, which are 1) to engage students in their learning, 2) to motivate and empower students by providing them some control over their own learning, 3) to encourage collaboration and foster a learning community, 4) to guide students to reflect on what and how they learn, and 5) to explicitly teach students skills on how to learn. Of note, various terms are used in the literature to refer to strategies that are related to learner-centered teaching (e.g., active learning, student-centered teaching).
The literature suggests that teacher-centered instruction as opposed to learner-centered teaching promotes memorization ( Hammer, 1994 ) rather than desired competencies like knowledge application, conceptual understanding, and critical thinking emphasized in national reports (American Association for the Advancement of Science [AAAS], 2011). Further, lecture-based teaching fails to promote understanding of the collaborative, interdisciplinary nature of scientific inquiry ( Handelsman et al ., 2007 ). Notably, female and minority students have expressed feelings of alienation and disenfranchisement in classrooms using teacher-centered instruction ( Okebukola, 1986 ; Seymour and Hewitt, 1997 ).
A recommended practice that can support implementation of learner-centered teaching is the use of the backward design ( Wiggins and McTighe, 2005 ). The backward design model involves articulation of learning goals, designing an assessment that measures achievement of the learning goals, and developing activities that are aligned with the assessment and learning goals.
Despite robust evidence documenting the superiority of learner-centered teaching over teacher-centered instruction (as reviewed by Freeman et al ., 2014 ), instructors continue to adhere to teacher-centered instruction. A recent study showed that the majority of faculty members participating in professional development programs designed to help them adopt learner-centered teaching practices continue to rely on lecture-based pedagogy as indicated by classroom observational data ( Ebert-May et al ., 2011 ). Possible reasons for such loyalty to lecturing include the following: 1) instructors’ own personal experiences with lecture as undergraduates ( Baldwin, 2009 ); 2) personal beliefs that transmission of knowledge to students through lecture is the best way to teach ( Wieman et al ., 2010 ); 3) the perception that lecture preparation is more time-effective than preparing learner-centered activities ( Dancy and Henderson, 2010 ); 4) student resistance to active learning ( Henderson and Dancy, 2007 ; Seidel and Tanner, 2013 ; Bourrie et al ., 2014 ); 5) initial difficulties are often encountered when transitioning to learner-centered teaching, requiring several iterations to perfect a new teaching style; 6) learner-centered teaching encourages instructors to cover fewer topics in greater depth to promote meaningful learning ( Weimer, 2013 ), and many instructors are uncomfortable with such loss of content coverage ( Fink, 2013 ); and 7) the learner-centered instructor must change his/her role from an expert who delivers knowledge to a “teacher-facilitator,” giving a degree of control over the learning process to students, and many instructors are uncomfortable with the unpredictability and vulnerability that comes with relinquishing control in the classroom ( Weimer, 2013 ). Further, universities oftentimes fail to incentivize and encourage faculty members to prioritize teaching to a similar degree as research ( Fairweather et al ., 1996 ). It has been argued that the professional culture of science assigns higher status to research over teaching, encouraging scientists to adopt a professional identity based on research that typically ignores teaching ( Brownell and Tanner, 2012 ).
Given that many instructors face challenges and intimidation while implementing learner-centered teaching in their classrooms, there is a need to explore their experiences and learn what support instructors need as they engage in the process of transforming their courses. Science education researchers have recently emphasized the critical need “to better understand the process by which undergraduate biology instructors decide to incorporate active learning teaching strategies, sustain use of these strategies, and implement them in a way that improves student outcomes” ( Andrews and Lemons, 2015 , p. 1).
Case studies have been shown as a useful tool to understand change processes ( Yin, 2003 ). A case study approach represents a qualitative method of inquiry that allows for in-depth description and understanding of the experience of one or more individuals ( Creswell, 2003 ; Merriam, 2009 ). Yin (2003 , p. 42) provides a rationale for using single, longitudinal case studies that document participants’ perspectives at two or more occasions to show how conditions and processes change over time. In this study, we used a case study approach to obtain an in-depth understanding of the change process of two university instructors (Julie and Alex) who were involved with redesigning a biology course. The instructors sought to transform the course from a teacher-centered, lecture-style class to one that incorporated learner-centered teaching. We interviewed the two instructors on multiple occasions; we also interviewed a graduate teaching assistant (GTA) and an undergraduate learning assistant (ULA) to gain their perspectives on teaching the course. We explored the motivation, challenges, and thought processes of the instructors during the interviews. We used several data sources in addition to the interviews to build the case study, including class observations by external observers and student feedback data.
Given that faculty members have difficulty changing their teaching, there are recommendations to use theoretical models of change to examine processes of change ( Connolly and Seymour, 2015 ). We looked for theoretical models of change ( Ellsworth, 2000 ; Rogers, 2003 ; Kezar et al ., 2015 ) and found that the innovation-decision model ( Rogers, 2003 ) has recently been used by science education researchers ( Henderson, 2005 ; Bourrie et al ., 2014 ; Andrews and Lemons, 2015 ). Therefore, we decided to use this model to theoretically approach our data. Specifically, we decided to use the adapted model developed by Andrews and Lemons (2015) , which they modified to represent the change process that biology instructors experience when redesigning a course. This model includes the following stages: 1) knowledge, in which the instructor learns about the innovation and how it functions; 2) persuasion/decision, in which the instructor develops an attitude, positive or negative, toward the innovation and decides whether or not to adopt the innovation; 3) implementation, when the instructor behaviorally implements the innovation; and 4) reflection, in which instructor considers the benefits and challenges of using the innovation. On the basis of reflection, an instructor decides to stay with the present version of the implementation or to start the process once again in an iterative manner by seeking new knowledge (see Figure 1 ). According to Rogers (2003) , a condition to begin the change process is that an instructor must be dissatisfied with his or her current teaching approach. Such dissatisfaction is one contributing factor leading an instructor to begin seeking new knowledge about new teaching strategies. Other external and internal factors usually influence an instructor’s decision to change his or her teaching, including release time, institutional commitment, and instructor attitude ( Andrews and Lemons, 2015 ).

Innovation-decision model adapted from Rogers (2003) , Henderson (2005) , and Andrews and Lemons (2015) .
Context of the Study
This study was conducted at a research-intensive university on the East Coast of the United States. The instructors cotaught Principles of Biology III: Organismal Biology (BSCI207). BSCI207 follows two prerequisite courses, BSCI105 and BSCI106. BSCI105 covers molecular and cellular biology, while BSCI106 covers ecology, evolution, and diversity. BSCI207 requires students to synthesize concepts and principles taught in prerequisite courses, apply them across contexts in biology, and generally engage in higher-order learning (e.g., interdisciplinarity, conceptual understanding, quantitative reasoning). The course enrolls between 100 and 200 students per semester.
In Fall 2013, the provost’s office distributed a call for grant proposals encouraging instructors to redesign their courses to incorporate evidence-based teaching approaches. The call specifically required applicants to design experimental studies to evaluate their course redesign approaches in comparison with their usual teaching approaches. Julie and Alex applied for the grant and were funded. Their proposed evidence-based teaching approach was to incorporate a series of small-group active-engagement (GAE) exercises throughout the semester. The traditional section would retain the usual three 50-minute lectures per week schedule. The experimental section would replace one 50-minute lecture with a shortened 20-minute lecture followed by a 30-minute GAE exercise with content matched to the traditional class occurring that day.
The instructors designed the GAEs to accomplish a series of learning goals that were consistent with Weimar’s five principles of learner-centered teaching. For example, one of the GAE goals was to foster collaboration among students in order to mimic the scientific process of inquiry. This goal was in accord with Weimer’s (2013 ) learner-centered teaching principle of collaboration, creating a learning community with a shared learning agenda, and modeling how experts learn. To accomplish this goal, the instructors implemented the GAEs in a small-group setting and required students to exchange ideas and achieve consensus on a single worksheet.
A second goal, which accords with Weimer’s (2013 ) framework, was to engage students in their learning and motivate them to take responsibility and control over their learning process. For example, one of the GAEs asked students to complete a humorous, fictional case study involving a spaceship captain and deadly neurotoxins. In this activity, students needed to use mathematical equations to calculate membrane potentials and to create simulations of conditions that impact membrane potential. Another activity was to collaboratively create a plot of ion transport rate versus concentration. Students were given a computer simulation that they used to generate data; they then entered the data into a Google documents Excel spreadsheet. This created a classroom database that was used to build the plot, which the instructor displayed using the lecture hall projector at the end of class. This activity involved multiple components of learner-centered teaching, including collaboration, student engagement, and student responsibility for learning. Detailed descriptions of a selection of GAEs are published elsewhere ( Carleton et al ., in press, 2017 ; Haag and Marbach-Ad, in press, 2017 ).
The provost grant offered funding that could be used for various purposes. The instructors decided to use the funding for summer salary to develop GAEs and to pay for support from a science education expert. Grant awardees were required to participate in Faculty Learning Communities (FLCs) and teaching workshops arranged by the campus teaching and learning center.
In Fall 2014, the instructors started to implement their experiment. Jeffrey, a third instructor, joined Alex and Julie to teach both sections; each of them was responsible for teaching several topics associated with their specific research expertise. In the GAE class, students were divided into small groups to complete a learning activity pertaining to the course topic. In total, 12 GAE sessions were held during the semester. Both GAE and traditional classes were taught in large auditoriums. For each GAE session, students self-selected into groups of three to five students. Four GTAs circulated among the groups to facilitate group work. Students were asked to leave empty rows around their respective groups to allow GTAs to move throughout the groups. This same topic was covered only by lecture format in the traditional class.
In Fall 2015, the instructors no longer conducted a comparative experiment while teaching. Julie and Alex continued to coteach the course with the GAE format with many modifications to the activities and other aspects of the course (see Results ). Jeffrey continued to teach a different section of the course independently. Henceforth, we will describe the experience of Julie and Alex in their process of transforming the course.
Teaching Staff
Julie and Alex are associate professors. Lisa is a doctoral-level teaching assistant (TA) in the biology department. Lisa was a GTA in the Fall 2014 and Fall 2015 semesters. Jason was a freshman student in the GAE section of the Fall 2014 semester. In Fall 2015, Jason served as a guided study session (GSS) peer leader in BSCI207. GSS leaders are students who have taken a course on implementing evidence-based teaching approaches, and who have also completed the course they are tutoring with a high grade. GSS students are expected to facilitate small-group discussions outside class. Jason also volunteered to attend all GAE sessions to help facilitate.
Data Collection Instruments
Yin (2003) notes that multiple data sources are important in building case studies. As such, we use interview data, class observations, student feedback on the course, and information written in the grant proposal.
Interview Protocol.
Julie and Alex were interviewed independently immediately following Fall 2014 for 20 minutes each. Julie was also interviewed independently in the beginning of Fall 2015 for 1 hour. Julie and Alex were interviewed together immediately following Fall 2015 for approximately 1 hour. Lisa and Jason were also interviewed following Fall 2015 for 20–30 minutes each. We used semistructured interview protocols (see the Supplemental Material) with additional questions to probe for clarification. The questions probed participants’ motivation for change, attitudes toward change, barriers and challenges, administrative supports, details about the implementation, and teaching philosophies.
Class Observations.
Two independent raters conducted class observations. Each year, raters attended six classes. In Fall 2014, they observed GAE class sessions and the parallel, content-matched class sessions that took place in the traditional class (overall 12 sessions). This procedure allowed the raters to compare the class sessions covering the same material but with differing teaching approaches (i.e., learner-centered vs. teacher-centered instruction). The two raters attended each class session together. Once in the class, the raters used a rubric to evaluate the class. In Fall 2014, raters used a rubric based on a previously constructed rubric that was created by the biology department for peer observations ( http://extras.springer.com/2015/978-3-319-01651-1 , in SM-Evaluation of teaching performance.pdf). In Fall 2015, to better document group work, the raters used the rubric developed by Shekhar and colleagues (2015) .
Student Feedback.
Students were invited to reflect on GAEs by providing anonymous written feedback on note cards following the activity. We use some of these data in the present study.
Data Analysis
Interviews were conducted by a science education researcher, audiotaped, and transcribed. A science education researcher and a doctoral student in counseling psychology separately analyzed the interviews and the note cards to define emergent themes. Then, they negotiated the findings until they could agree upon the themes ( Maykut and Morehouse, 1994 ). The instructors were shown the interpretation of data to verify accuracy of interpretations. We present the results in accordance with the adapted Rogers (2003) model presented in Andrews and Lemons (2015) . We slightly adapted the Andrews and Lemons (2015) model to the iterative process through which our instructors progressed to modify the course (see Figure 1 ).
Motivation for Change
Before 2014, the traditional BSCI207 class as taught was a three-credit course with three 50-minute lectures per week. Alex described the traditional course:
Before the GAEs came into being, we taught in the very standard, traditional lecture. We used mostly PowerPoint to show text and images, occasionally we would bring a prop in, like sometimes I would bring a piece of a tree to gesture towards as I was lecturing about water transport or something like that. But it was basically standard lecture.
The instructors were dissatisfied with the traditional lecture format for the following reasons:
- Evidence for inferiority of teacher-centered instruction compared with learner-centered teaching . The instructors expressed awareness of the empirical data documenting the superiority of learner-centered teaching over teacher-centered instruction, “There’s a lot of research that suggests that [teacher-centered instruction] may not be the best way to help the students understand what we’re trying to get them to understand” (Alex).
- Lecture hinders understanding of the process of science. The instructors also expressed a desire to get students to learn the process of science early in their education, rather than to passively receive information. “We are being asked as science professors more and more to try and get our students to understand that science is a process, earlier and earlier in their career, and to model what real science is like in their education” (Alex).
- Lecture promotes overreliance on memorization. The instructors discussed a goal to modify the course so as to decrease focus on memorization and increase emphasis on problem solving and conceptual understanding. Julie described: “BSCI207 is the biology majors’ class, and it’s a lot of what the pre meds are taking, and so, critical thinking I think [is important], we’re constantly trying to get them to not just memorize and regurgitate but to put the ideas together.”
We also rearranged the material. So they [the lectures] used to be in a taxonomic orientation, I would give a whole lecture titled the biology of fungi, and the students complained that this taxonomic focus seemed to resemble the structure of BSCI106 [the prerequisite course]. I decided to explode those taxonomic lectures, and take the bits of content that I still thought were valuable, and spread them into other parts. So for example the stuff on mating types, which is wacky and interesting to me, and I hope to the students, is now in a lecture on sex. And they don’t realize half the lecture is on fungi. So they’re susceptible to packaging I think, and we don’t get the complaint any more that the course is redundant to BSCI106 (Alex).
Organisms don’t care about our disciplinary boundaries of research. The organism doesn’t understand that there’s biophysics, and biochemistry, and evolutionary biology, and ecology, and genetics. All these attributes of their biology have to function simultaneously on several different spatial and temporal scales … if we think they do, then we continually miss things that otherwise would fall out naturally if we were a little less wedded to our disciplines.
Relatedly, the instructors noted that most students enrolled in BSCI207 without having taken introductory physics or chemistry, which they thought was preventing students from drawing upon highly relevant concepts (e.g., thermodynamics) from these courses for biology.
- Underrepresented groups do poorly in traditional classes. The instructors quantitatively examined student performance for specific student subgroups (i.e., underrepresented minority students, female students) in previous BSCI207 semesters. They observed that there were disproportionate D/F/W grades for underrepresented students. Coupled with the science education literature documenting the ability of active learning to help underrepresented groups ( Preszler, 2009 ; Haak et al ., 2011 ; Eddy and Hogan, 2014 ), the instructors speculated that adding active learning to the traditional class might help underrepresented students.
In Fall 2014, the instructors went through the process of course revision that follows the adapted model by Rogers (2003) and Andrews and Lemons (2015 ; see Figure 1 ). In the following sections, we discuss their progression through the innovation-decision model. Table 1 shows a summary of the change process for the Fall 2014 semester.
First Iteration of the instructors’ change process
Knowledge | Decision/persuasion | Implementation | Reflection | |
---|---|---|---|---|
Traditional (before Fall 2014) → traditional active comparison (Fall 2014) | Design an experiment to: | Fall 2014 |
Before the Fall 2014 semester, the instructors engaged in several efforts to increase knowledge about evidence-based teaching approaches to modify the course. The knowledge sources were as follows:
I will go ask [physics education professional] questions. When something doesn’t go well I’ll meet with the postdocs [from physics education research group (PERG)] over there and say, what are they not getting here, how can we make this better, so I’m always trying to get resources to help.
- Reading the science education literature. As a new instructor, Julie participated in the college workshop for new instructors. The workshop was led by the director of the teaching and learning center, who provided several resources for using evidence-based teaching approaches, including an article giving an overview of learning styles ( Felder, 1993 ), a book on teaching tips ( McKeachie and Svinicki, 2006 ), and the book Scientific Teaching ( Handelsman et al ., 2007 ). In her interview, Julie commented, “So I read a lot of books,… I think it was getting students to think about math, I read one of the books [that the director of the college teaching and learning center] had given me [ Scientific Teaching ].”
- Observing other instructors teaching. The instructors had observed another instructor who implemented evidence-based teaching approaches in a small class of BSCI207 (<40 students). This pilot implementation was successful, and the instructors were interested in investigating whether the learner-centered teaching model used could be scaled up to a large-enrollment class.
Persuasion/Decision.
Following the knowledge-generation phase, the instructors felt prepared to change their teaching to a more learner-centered teaching style. They decided to conduct a comparative experiment during the first implementation of the GAEs (i.e., traditional vs. GAE classes; see Marbach-Ad et al ., in press, 2017 ). Although the instructors were aware of the literature documenting the effectiveness of learner-centered teaching, they had several reasons to execute the experiment:
- Obtain evidence for overall effectiveness. The instructors were unsure whether their activities were the best way to change the course (e.g., they were unsure of the challenges that would emerge, how the intervention would impact students). The instructors also wished to explore cost-effectiveness, since they knew that changing the course would require a high instructor time commitment.
- Convince colleagues to adopt learner-centered teaching approaches. The instructors noted that faculty in the department were unconvinced of the superiority of learner-centered teaching approaches, and they thought that a comparison study bringing empirical evidence might demonstrate that changing one’s teaching style is worthwhile. Alex stated, “[A] lot of my motivation for this experiment was to try to provide some evidence that these approaches were worth the effort, and because there is resistance clearly, from some of our colleagues who have been teaching the course for a long time.”
- Respond to grant award requirements. As mentioned earlier, the institution announced a call for proposals for instructors to revise their teaching. The instructions required applicants to propose comparative experiments during course revision to document effectiveness.
Implementation.
As proposed in the provost grant application, the instructors executed the comparison study. In the traditional class, instructors delivered a 50-minute lecture three times per week. In the GAE class, one lecture was replaced with a GAE. The GAE consisted of a brief 20-minute introductory lecture (a short version of the lecture presented to traditional class students) and a 30-minute group activity. As scientists, the instructors wished to manipulate the addition of the GAE day only and to keep remaining variables constant across classes. Therefore, homework assignments, examinations, optional computer tutorials, and office hours availability were consistent in both classes (see Table 2 ).
Fall 2014 class comparison
GAE class | Traditional class | |
---|---|---|
Weekly class sessions | One GAE session and two lectures | Three lectures |
Weekly homework | Homework problems graded for effort | Homework problems graded for effort |
Tutorials | Optional | Optional |
TAs | Four GTAs | Four GTAs |
Class size | 136 students | 198 students |
Assessments | Pretest, three tests, final exam | Pretest, three tests, final exam |
Room setting | Large auditorium | Large auditorium |
In the GAE class, on the day of the GAEs, students were instructed to sit with groups of three to five students (of their own choosing) and to leave empty rows between groups. Students were asked to have at least one laptop per group. As discussed previously, the GAEs were designed to be more learner centered relative to traditional lecture classes. To illustrate this here, we give Alex’s description of the membrane transport GAE: “The students had a little computer simulation, and they used that to generate data that they then entered into a Google docs spreadsheet in real time in the class, and there were enough students in the class that their responses produced this beautiful textbook plot of transport rate versus concentration. They built that relationship in a way that otherwise I would have just told them.”
Reflection.
Following the Fall 2014 semester, the instructors reflected on the various pros and cons of the learner-centered teaching intervention in the interviews. Observers and students also provided feedback that was used by the instructors to reflect on both sections of the course and on the comparative experiment. Several themes emerged from these data:
It’s much less about my spouting facts, it’s about my thinking ahead of time to get them to draw conclusions and get them to cement ideas. My role was partly just to control the chaos sometimes, and to control that the TAs had the information they needed so they could provide guidance to the students.
Importantly, observers noted that the instructors were very actively engaged with student groups throughout the GAEs, helping students to work through problems and understand concepts. Julie also commented that teaching with GAEs requires greater proficiency with material than lecturing: “To use these activities, you have to know the material better than if you’re going to straight lecture. And I think some instructors are maybe still learning BSCI207, what is all the material in it. And until you teach it straight a couple of times you probably don’t have the background to really understand.”
We spent less time talking about dating the origins of life using various methods (fossil record, carbon dating); we got rid of a lecture on prokaryotes and had to shrink some of the nutrient assimilation information from two lectures to one.
The instructors explained that, in order to minimize loss of content coverage, they decided to have a GAE class only once per week and to pick GAEs corresponding to lecture topics for which “there was the least amount of lost material by focusing on a particular exercise” (Alex). An additional solution was to move in-class lectures to online, preclass lectures. Julie described this change: “We also ask students to review some of the material that is lost during lecture time into the prep slides they review ahead of time.” However, Julie wondered whether students would benefit from online lectures to the same degree as in-person lectures: “I am still worried they don’t get so much out of those [online lectures] and so miss much of that information.”
- Engagement in learning. Overall, the instructors reflected that most GAEs provided a space for students to interact with one another, TAs, and instructors: Julie added, “I think it was nice to see the energy in the class and the way the students took to the activities, it was different for them.” Observers noted that the GAE class treatment condition was usually associated with increased student interactivity. Specifically, they noted that students in the GAE class were not only more engaged in the GAEs, but that they also tended to raise more questions during the PowerPoint presentations relative to students in the traditional class. Students reflected on their note cards following GAEs, and in the end-of-semester survey, noting that they felt that many of the GAEs were engaging (see Marbach-Ad et al ., in press, 2017 ).
- Giving students control over learning. The instructors noted, “The GAEs represented a chance to turn the class over to the students for some part of the time, where they could do something actively, instead of just sitting there listening to us” (Alex).
It’s actually a bit more how real science works, right, even as somebody who runs a lab, I don’t go into my lab and sit there and talk to my graduate students for four hours, I mean we have a brief conversation about how they should tackle something, and then they go off and work more on it. So it’s more of a checking in and then separating again. That’s kind of how this class works, the GAEs do give the students a little more of a feel of how collaborative real science works, and how no one person is sort of dictating everything, everyone needs to be a bit independent. … I think that this active model gives the students, for the first time, a real taste of how a real scientist would approach a problem.
Students commented on the opportunity afforded by GAEs to take an active role in their learning: “I learned how to apply what we learn in lecture class to actual problems”; “I kind of felt like a real scientist since I was put in a situation in which I had to make a hypothesis myself.”
- Disengagement. The instructors noted that, for some GAEs, students were disengaged. For example, in the GAE on stress and strain, two students were doing measurements in front of the class for 10–15 minutes, and the remaining students were instructed to input data into Excel files. These data were then used to make calculations. Students also expressed their dissatisfaction with this activity on the note cards that they handed in to the instructors: “I feel I understood the concept well once Dr. Julie wrote the plots on the board. This activity was more tedious and like busy work”; “ We could have easily compared values without experimentally finding them. I didn’t feel this deepened my understanding of concepts.”
- Insufficient time for reflection. The instructors noted that most exercises were too long, which did not leave sufficient time for reflection. Alex noted, “Well I think also making sure that if we get the exercise done in the right, short amount of time, then that does give us time to add a reflection at the end. Connecting the results of our exercise back to some larger idea.”
- Student preparation. The instructors felt that students would gain more from the exercise, if they were to come to GAE classes with better understanding of concepts relevant to the GAE. Then, more time could also be allotted for summary and reflection on important concepts. Alex commented, “We probably will need the students to do a bit of preparation before they come in to these active exercises, so that we can spend less time setting it up, and more time summing it up.”
- Assessments and grading misaligned with GAEs. In this implementation, instructors kept the same assessment plan for both the traditional class and the GAE class in order to compare achievement across classes. This resulted in a mismatch between the course activities and the assessments in the GAE section. For example, there were no final examination questions specifically covering GAE material. Of note, the instructors analyzed their final examination questions before conducting the experiment and saw that the questions required students to demonstrate high levels of thinking ( Bloom and Krathwohl, 1956 ; e.g., knowledge application, quantitative analysis), and they believed the GAEs would improve students’ abilities in these areas. Further, the instructors did not count GAE participation toward final grades, which instructors and observers believed had a detrimental effect on GAE attendance. Julie noted that “on the GAE days, only 60% of the students would come. That was partly because they wouldn’t get any credit for it, and they weren’t seeing that it was helping them learn the material better.” Analyses showed that students with higher grade point averages (GPAs) were those who chose to attend on the GAE days (see Marbach-Ad et al ., in press, 2017 ). Given this, the instructors felt that attendance should be incentivized in future implementations of the learner-centered teaching intervention to motivate and benefit a wider range of students.
- Resistance to learner-centered activities. The instructors felt that students’ low attendance specifically on GAE days may also have been because the students did not perceive the benefit of GAEs for their learning. “I feel sort of parental here, maybe the GAEs are like broccoli and brussels sprouts, they need them, they just don’t know it yet” (Alex).
- Group dysfunction. The instructors and observers noted several issues with the groups. Some groups were not engaged, and some students were not participating within their groups (e.g., one student would be left out). In some activities, some groups would finish the activity very quickly and would subsequently appear bored and waiting for further summary or instruction. Julie was frustrated with these occurrences and noted, “People would be sitting there on their phones.” One reason for student disengagement could be that students groups were unassigned and could include different students each week: students “would sit and associate with whoever was around them” (Julie).
- Auditorium-setting challenges. The instructors commented on the difficulty of doing GAEs in the large auditorium: “It’s still tricky to think about how you actually stage all of this, there is a bit of theater to running a large class with 200 students, how you move from one aspect of the process to another [lecture to group activities] quickly, without losing people, without too much noise and disturbance” (Alex).
- Little impact on grade distributions. Alex and Julie were hopeful that the GAEs would lead to large improvements in students’ grades as compared with traditional learning. However, the effect of GAEs was very small. Alex commented, “This was the biggest outcome from my perspective, and it drove much of the revisions for 2015. This is interesting, as it shows that even though we were unable to realize a big payoff in the first year, we nevertheless saw something that we thought was worth keeping and hopefully improving upon.”
- TA training required. The instructors reflected that they did not provide adequate TA preparation for the GAEs: “We hadn’t really prepared the GAEs enough ahead of time so that we could talk about them with the TAs. The TAs at times were really clueless about what was supposed to be happening” (Julie). TAs, although instructed to guide and facilitate groups, apparently lacked the skills to engage students, as observers noted that most of them passively waited for students to ask questions rather than actively approaching students with questions, instructions, etc.
On the basis of their reflection, Julie and Alex decided to continue teaching with GAEs and to seek new knowledge to improve GAEs. In the following sections, we discuss their continued progression through the innovation-decision model (see Figure 1 ). A summary of the change process in Fall 2015 is shown in Table 3 .
Second iteration of the instructors’ change process
Knowledge | Decision/persuasion | Implementation | Reflection | |
---|---|---|---|---|
Traditional GAE comparison (Fall 2014) → GAE only (Fall 2015) | Fall 2015 |
- Learn about methods to form successful groups. The instructors reviewed the literature and consulted with the director of the teaching and learning center and other faculty members in the department to form new strategies on building effective groups in auditorium settings. The literature shows that groups work best when they are permanent and students are held accountable to other group members ( Michaelsen and Black, 1994 ; Michaelsen et al ., 2004 , 2008). The literature also shows that taking student diversity into account is important in creating successful groups ( Watson et al ., 1993 ). For example, Watson and colleagues (1993) reported that, although it takes time, heterogeneous groups outperformed homogeneous groups on several performance measures, including generating perspectives and alternative solutions. The instructors also learned from the director of the teaching and learning center about the Pogil method ( pogil.org ), in which students are assigned different roles during group work (e.g., recorder, facilitator). They weighed the pros and cons of implementing this method in the classroom.
- Learn about methods to flip courses. The instructors learned from models of flipped classes ( Hamdan et al ., 2013 ; Jensen et al ., 2015 ), which highlight how to capitalize on out-of-class time to cover material to prepare for face-to-face active learning. In this regard, instructors sought assistance from the information technology office about presentation software (i.e., Camtasia) that can deliver automated lectures effectively.
- Seek expert guidance. During the summer, the instructors again consulted with science education experts to enhance the GAEs. For example, they consulted with a science education expert on how to revise the concept map assignment. Julie described how this guidance helped her “leave the activity a bit more free form and get the students to make a graphic organizer of their own design rather than trying to fill in some pre-designed boxes.” As another example, the science educator recommended strategies about how to streamline GAEs to maximize time spent on developing conceptual understanding and minimize time spent on the mechanics of exercises.
- Learn about strategies to enhance TA support. The instructors wished to decrease student to TA ratio. However, GTAs require departmental funding, which was unavailable. The teaching and learning center director and the biological sciences administration offered to involve ULAs who are unpaid but receive alternative benefits, such as leadership and teaching experience and undergraduate course credit. This model was reported to be successful in our university ( Schalk et al ., 2009 ) and in other institutions ( Otero et al ., 2010 ).
Following reflection on the comparative experiment, instructors sought to keep improving the course and decided to make several changes:
- Teach all sections with learner-centered teaching. Although the instructors reported that keeping the GAE class format requires more time to prepare relative to lecturing and takes time from their research (“fine tuning the GAEs—that took weeks” [Julie]), they decided to implement the GAEs in all sections and to work to improve them.
[Last semester] I had a couple of students up front doing the experiment, and everyone else was kind of twiddling their thumbs while we gathered the data. We talked about the data but we didn’t really have time [to do data analysis and summarize concepts]. I think this year I’m just going to give them last year’s data, and have each group do some analysis.
As another way to modify GAEs, instructors decided to utilize more outside resources such as published, case-based activities. Julie described, “I’d love to come up with some more case studies that we could do. You know the Buffalo site [ http://sciencecases.lib.buffalo.edu/cs/collection ] has all the case studies for all the science classes. So I’m constantly perusing that. A couple of the GAEs that I developed actually come from there.”
I haven’t figured out what the best prep work is. What Alex has been doing is taking the slides he showed last year and just posting them online. I’m not sure that’s the best, or really enough.… But, then they just read. I mean he tries to put more words on them. I tried to find some videos that I thought were appropriate, and I’m not sure that’s any better. I was going to do some of these with Camtasia. In this way you can actually have the slides and actually talk over them and record. But I couldn’t make the software work. I haven’t really gone there yet, I will have to figure that out.
To encourage students to prepare for the GAEs, the instructors decided to give a preclass quiz covering the out-of-class preparatory materials. Julie described,
We’re also doing a quiz this time, we’re giving that preparatory information, they have to have done it by the morning before, they have to take a little 2-point quiz [before class] to show that they’ve covered that material. Then we have the whole class time [for the GAE] so that we’re not so rushed in trying to do to many things at one time.
- Train the TAs better, add ULAs, and involve both teams in the process of GAE development. The instructors decided to expand the team of assistants to decrease the ratio between students and TAs. Julie described the change from Fall 2014 to Fall 2015: “We have a bigger team. We have two of these ULAs, and then we have three UTAs, and two GTAs. So a team of seven helpers, and each person has a different job. The ULAs are specifically supposed to be trying out the GAEs ahead of time. So we kind of run things past them. And then we meet with all the TAs, and then talk through the GAEs beforehand. They have an assigned part of the class, where each of them is hopefully seeing the same students over and over, and hopefully getting to work with them to develop a rapport, and they go in the middle of the activity, so kind of checking in, so what do you think, kind of getting students to verbalize.” The benefit of this new format, where each TA was responsible for a subsection of the large class, was that it approximated a smaller class discussion session in which students could get to know their TAs more personally.
- Revise group structure. On the basis of the literature and their previous experiences, the instructors decided to assign permanent, diverse groups of four at the beginning of the semester. They also decided to instruct students on how to sit in the auditorium with their groups (in two rows rather than in a single line, to enhance group communication) and to award points for completing group work exercises.
In the Fall 2015 implementation, there were several changes to the course (for a comparison of 2014 and 2015 GAE classes, see Table 4 ).
GAE class comparison between Fall 2014 and Fall 2015
GAE class (2014) | GAE class (2015) | |
---|---|---|
Preparation | · In-class lecture (∼20 minutes) before GAE | · Online lecture slides + graded, preclass quiz |
Homework | · Homework problems graded for effort | · Homework problems graded for effort |
Activity duration | · ∼30 minutes | · 50-minute class period |
TAs | · Four GTAs | · Two ULAs, three undergraduate TAs, two GTAs (seven total helpers) |
Exams | · Exams did not include specific questions from GAE | · Exams included questions from GAEs |
Grading | · Homework assignments | · Homework assignments |
· Exams | · Prequiz | |
· GAEs | ||
· Exams | ||
Student groups | · Not assigned/impermanent | · Assigned/permanent |
· Three to five students | · Four students | |
· Free auditorium seating | · Specific auditorium seating |
- Modify the activities. The instructors devoted a full weekly class period to the GAE instead of 30 minutes. On the basis of their experiences in the previous semester, they revised some GAEs and adapted them to the time frame. Although they had more time for the GAEs, they wished to make them more efficient and interactive: “I think we had to cut some, with the GAEs, because they were taking way too long, but I think in a few cases we simplified them, took out 1/3 of them or something” (Alex). Instead of the 20-minute pre-GAE lecture that was presented in the Fall 2014 implementation, students were asked to prepare for activities at home by watching videos, reviewing lecture slides, and reading textbook materials. In contrast with Fall 2014, the students were awarded three points for participating in the GAE activity and two points for completing a quiz covering preparatory materials that was due before the GAE class. The instructors wished to assign points to these activities in order to “really give them weight” (Julie). “[The activities] formed a large part of the exams as well. So making the activities more integral to the class was a big change” (Julie).
So the next time I drew a map in the room, [which showed] two students in the front, and two students in the back. When you have a very formal auditorium, you have to try and help them assort with each other and talk with each other. The other thing we did was, we were giving each group two copies of the assignment, so they didn’t each have one. So that kind of helped, that kind of had them sharing things.
Finally, TAs and ULAs were assigned to stay with one section of the lecture hall throughout the semester. Thus, TAs and ULAs developed a rapport with a large group of students throughout the semester and were able to learn their names, which facilitated communication.
- Add more and better-trained TAs. Before Fall 2015, the instructors trained the TAs to better engage with student groups in class. Class observation data showed that, in Fall 2014, some TAs were lacking in their ability to engage actively with students. One observer described, “When I observed the classes last year [Fall 2014], they [TAs] were standing in the side [of the auditorium], and sometimes they got to students, but just students that raised their hands. They weren’t active. They were very passive, most of them, because they didn’t know what to do.” Following the implementation in Fall 2015, the observer noticed a change in TA involvement: “Now, it’s more about instruction, they circulate between groups and encourage them to ask questions, they encourage students that aren’t participating … it’s not enough to throw them [the TAs] in the classroom.”
Overall, instructors noticed improvements in the areas that they targeted to improve, and they also felt there were areas that they wished to continue improving.
- Student preparation. Julie described that although new techniques were put in place to increase student preparation, students often seemed unprepared for the activities: “And my data for that is essentially for the first 20 minutes of the GAE they would spend saying, what are we doing? There was a lot of flailing. It took them a lot longer to get going with the GAE than I thought, and I’m not sure if that’s because the preparatory material is not really preparing them, or that they just took the online quiz and didn’t really go through the preparatory material.” Julie thought about changing the nature of the preparatory lectures, “I would still like to explore turning those into little online lectures rather than having them read the slides.”
- Student attendance . Alex commented that the strategy of assigning points to participating in GAEs “made a big difference in attendance […] by incentivizing their attendance, at least on GAE days, they were coming.” The instructors commented that incentivizing participation in the GAEs and the preactivity quizzes increased the amount of student–instructor interaction regarding point grabbing. Alex stated, “The downside of associating points with everything is that I think we spent the largest fraction of our student interaction time dealing with the points related to the GAEs, excused absences, non-excused absences, anxiety about the points, I mean these are tiny amounts of points, but the students took it very seriously. But I think it was one of the top 3 issues that students came up with this semester.”
- Mechanics of exercises. Julie was very frustrated with how students could not effectively operate Excel software: “And they still don’t know Excel. My biggest frustration was that I thought Excel would make their lives easier, and it made their lives harder. I’m almost ready to go back to pencil and paper, just to get them to plot things and think about things, because they’re not getting back to the scientific inquiry and hard thinking, they’re just so stuck in which box do I click.” Alex added, “My issue is that the preparatory materials do a good job preparing them intellectually for what’s the point, but then they do get stuck on the mechanics, what they’re doing with their hands.”
- Allocating time for reflection. The instructors described that they improved substantially in the area of summarizing major concepts and timing activities: “I think we did a pretty good job of every 15 or 20 minutes bringing them back together and saying ok, you would have done this by now. There were a couple that worked really well, and a couple where we were still pressed for time. I think that generally it was far improved” (Julie). Because the instructors had the full class period to devote to the GAE and did not need to compare learner-centered teaching with teacher-centered instruction, they felt that the timing of the activities was much improved. Julie noted, however, “I always overestimate what students can do. I’m still adjusting.”
- Technical issues. There were difficulties with connecting to the wireless Internet in the lecture hall, particularly among students who failed to download the appropriate tools before coming to class. Further, students have different types of computers and software programs and knowledge of software programs required for the course.
The organized approach helped students see the material as well as make a few friends, in fact, I remember coming onto my dorm floor and seeing four people from my class working together, and they were actually in that GAE group, they had made a study group because they were used to working together. One of the aims of this project gets students communicating instead of competing.
Julie commented that there is still room for improvement in the student groups: “I saw a number of groups where at least one person would be left out. I don’t know if that’s a physical orientation, if we could point them toward each other it would be better. Next year one thing we talked about is going to groups of 3, because with 3 you can always get across each other and be more … everybody can talk to each other.” The instructors considered the benefits of the Pogil. Julie explained that they tried to appoint a different group member to act as the scribe each week during GAE activities as a way to increase student participation in groups. The instructors did not strictly enforce this policy, as they were not sure it was beneficial.
And we also had some undergraduates this year, … and I think they were really helpful because they understand what the students are capable of, more than we do … a lot of times they can give you some insight into what’s going on or what classes undergraduates are most likely taking at the same time. It was very helpful.
Finally, Lisa felt that the level of engagement among the teaching staff was higher than for a standard lecture course: “Everyone was very engaged, it’s a unique class to TA for, because I feel like the TAs and the professors are far more engaged than in a standard lecture course, so it was kind of nice.” Alex reflected that, in the future, “It would be even better,” since they will have “a whole floor of ULAs that had us for 207,” and they “will be well-positioned” to assist in the redesigned course.
This case study examines instructor change processes when moving from teacher-centered instruction toward learner-centered teaching. In this study, we examined the change process through the lens of the innovation-decision model ( Rogers, 2003 ; Andrews and Lemons, 2015 ), which recognizes several stages of change: knowledge, decision/persuasion, implementation, and reflection. The model is iterative, recognizing that transforming courses may require multiple revisions as instructors reflect on the inherent challenges and imperfections that arise when changing a course ( Henderson, 2005 ). Consistent with this literature, the first implementation of learner-centered course revision was fraught with imperfections, and the instructors persisted through two rounds of course revision before gaining satisfaction with their teaching approach, although they plan to continue enhancing the course with each semester.
Andrews and Lemons (2015) note that dissatisfaction with one’s current teaching approach is an important motivator leading instructors to change their teaching. Our instructors were dissatisfied with the lecture mode of teaching in their courses due to personal dislike for it, and the sense that it encouraged student reliance on memorization and hindered interdisciplinary thinking. Other motivators for change included 1) awareness of national recommendations to use learner-centered teaching ( AAAS, 2011 ); 2) a hope that underrepresented students would benefit from learner-centered instruction, based on education literature documenting such benefits ( Okebukola, 1986 ; Seymour and Hewitt, 1997 ); and 3) institutional support (i.e., a provost office grant initiative).
These motivations led the instructors to seek new knowledge about learner-centered teaching approaches and how to implement them, which, according to the adapted innovation-decision model ( Andrews and Lemons, 2015 ), is a first step toward changing a biology course. In the present study, knowledge-seeking strategies included consultation with science education experts and information technology experts, reading the empirical literature, observing other faculty members who had adopted evidence-based teaching practices, and involvement with a discipline-based FLC. Following the knowledge stage, the instructors progressed through the decision/persuasion and implementation stages of change. In the reflection stage, the instructors discussed what worked well, challenges, and areas they wished to improve in the subsequent iteration. We present here implications from this study for instructors seeking to change their courses, and also for administrators wishing to promote learner-centered instruction at their institutions.
IMPLICATIONS FOR INSTRUCTORS
Weimer (2013) noted that engaging students in their own learning is messy, unpredictable, and challenging as compared with teacher-centered instruction. The process can be difficult for the faculty members who want to change as well as for the students. First, the instructor must adopt a new role as “instructor-facilitator” ( Weimer, 2013 ), giving up a degree of control to the students to take responsibility for their own learning. Relating to their new role, our instructors reported that, on the one hand, the instructor-facilitator role felt like controlling chaos at times, particularly in the beginning, but that it was markedly beneficial for student learning and for their own teaching. For instance, it gave students an opportunity to be independent learners and to engage with their peers in collaborative problem solving, more closely modeling the process of science. Thus, although it may be intimidating to share control over the learning process with students, it appears that there are benefits for both students and instructors.
Second, learner-centered teaching encourages instructors to cover fewer topics in greater depth, as opposed to more topics in less depth ( Weimer, 2013 ). Despite being uncomfortable with losing content coverage due to the function of BSCI207 as a preparation course for the MCAT and a prerequisite, our instructors decided to remove some course topics and consolidate others into shorter units. Next, they implemented several solutions to the necessary loss of content coverage. First, they moved lecture content to required preclass, online lectures that substituted for in-class content coverage. Second, they were strategic about which course topics they used to redesign as GAEs. Specifically, they selected course topics that were historically conceptually challenging for students (e.g., membrane transport). Our faculty members’ transition process provides an example of how faculty members can identify and implement solutions for concerns about loss of content coverage.
Third, a fundamental principle of learner-centered teaching is to encourage collaboration in the classroom ( Weimer, 2013 ). To this end, our instructors implemented GAEs, a series of group work–based activities. Student collaboration is important, because it promotes sharing of the learning agenda ( Johnson et al ., 1984 ; Weimer, 2013 ), and collaboration is a skill that is essential for the workplace ( Hart Research Associates, 2015 ). Group work is a common and accessible strategy that instructors can use to increase learner-centered teaching in their classrooms. Our instructors experienced various challenges and implemented several revisions to group work activities throughout their change process. The most successful strategies for optimizing group work included 1) increasing the number of TAs and the amount of TA training; 2) creating diverse and permanent student groups to increase accountability ( Michaelsen et al ., 2004 ); 3) assigning grades and preparation assignments for group work activities; and 4) restructuring group work activities to provide more time for whole-class summary and reflection on concepts. Group work is just one type of teaching strategy that can increase learner-centered teaching. Each instructor needs to discover what kinds of approaches are most suitable to increase their level of learner-centered teaching. When selecting and implementing new teaching strategies, it is highly recommended to seek guidance from experts, more experienced faculty members, or from a teaching and learning community.
Transitioning away from lecture-based instruction to learner-centered instruction can be challenging for students as well as instructors. The literature has shown that students resist many learner-centered approaches that require them to engage in the classroom rather than sit anonymously in lecture ( Michaelsen et al ., 2008 ; Shekhar et al ., 2015 ). Our instructors learned about student resistance through several means: 1) student feedback that was collected on note cards at the end of GAE classes, 2) end-of-semester surveys asking students to reflect on each activity, and 3) low attendance on GAE days as compared with lecture class days. It is important for instructors transitioning their courses to monitor student resistance and satisfaction, as our instructors used these data to modify the activities from the first to second iteration.
The instructors used several strategies to reduce student resistance. First, through student feedback, instructors learned that they needed to provide students with better explanations for the purpose of doing GAEs as opposed to sitting in lecture class. Weimer (2013) emphasizes the importance of providing students explicit instruction on how to best learn. Therefore, at the second iteration of the learner-centered implementation, the instructors were explicit about the rationale for the GAEs. At various points throughout the semester, the instructors explained how the GAEs were helpful in enhancing skills (e.g., critical thinking, problem solving, collaboration, understanding the interdisciplinary nature of science, relating course material to everyday life and to scientific research) that are recommended by national organizations ( AAAS, 2011 ) and employers ( Hart Research Associates, 2015 ). Second, instructors awarded class participation points for completing GAE exercises and grades for completing the preclass online quiz. This strategy resulted in better alignment between requirements of students and course assessments, which accords with Wiggins and McTighe’s (2005) backward design theory. This method of GAE grading resulted in much higher student attendance as compared with the first iteration. Third, instructors used evidence-based strategies to reduce resistance within student groups, including creating permanent, diverse groups at the start of the semester. Fourth, instructors took student feedback into account with regard to their satisfaction with specific activities and modified activities with the goal of maximizing student engagement.
IMPLICATIONS FOR ADMINISTRATORS
Given that changing one’s teaching from teacher-centered instruction to learner-centered teaching is challenging, there must be administrative support for these efforts.
First, administrators can play a key role in acknowledging the importance of learner-centered teaching. Historically, universities have failed to encourage faculty members to prioritize teaching to a similar degree as research ( Fairweather et al ., 1996 ). Unfortunately, many tenure-track faculty members at research-intensive universities fear that they may be penalized for investing the time to adopt learner-centered teaching. Research-oriented universities should prioritize teaching in order to support more widespread adoption of evidence-based teaching approaches. Julie reflected on her frustration with the university’s message that teaching is devalued relative to research:
I think for assistant professors, I was actually scolded for putting time into teaching and trying to participate in teaching improvements and so, I think it’s discouraged, perhaps rightly so, because they’re not going to value it, so if that’s going to take away from what’s required to get tenure, to get promoted, they want you to know that. So they’re just being honest perhaps.
As part of a university culture that values learner-centered teaching, administrators (e.g., chairs, promotion committees) should acknowledge instructors who are making the effort to transition their courses and understand if their teaching evaluations are lower during the initial semesters of transition.
Second, as evidenced by our study and by others in the literature, transitioning from lecture-based teaching to learner-centered teaching requires a large time commitment from instructors. Thus, funding and release time are valuable supports that administrators can provide to improve the quality of teaching at their institutions. The provost grant was a fundamental support contributing to our instructors’ success in transitioning a core biology course. Further, the fact that teaching fellowships were awarded from the university provost shows that our research-intensive university is beginning to value faculty members’ adoption of learner-centered teaching. Alex commented on these fellowships:
The message comes through that the university values teaching, otherwise we wouldn’t have these fellowships from the Provost, that’s about as high up as it gets, I mean there is this signal, a voice that says, great, please do this. But then when the rubber meets the road, are you going to get promoted? It is not considered a substitute for quality research productivity as a research-active faculty.
Third, learner-centered instruction requires more human resources relative to teacher-centered instruction (e.g., for grading, facilitating small-group discussions, demonstrations, assisting in revising course activities). Administrators should consider ways to assign more TAs to courses that use learner-centered teaching. TAs and/or ULAs could be compensated through financial means or through other methods like course credit. Our university, for example, has developed a training program for undergraduate TAs, in which they receive training in how to facilitate small groups.
Fourth, in universities where there are state-of-the art facilities for teaching and learning, there should be a priority for courses that adopt innovative teaching approaches. In our university, such facilities are in a state of development, and administrators are planning to incentivize faculty who are using evidence-based teaching approaches by giving them priority to teach in the new, state-of-the art teaching and learning facility, which includes classrooms with round tables, movable seats, and advanced technology.
Finally, universities should provide support for a campus teaching and learning expert and an FLC. These resources were fundamental in the transition process of our faculty members. FLCs may be discipline-based ( Marbach-Ad et al ., 2010 ) or campus-wide ( Cox, 2001 ). FLCs and teaching and learning experts can provide pedagogical and curricular guidance, as well as emotional support for the stressors associated with teaching.
Supplementary Material
Acknowledgments.
This work has been approved by the University of Maryland Institutional Review Board (IRB protocol 601750-2). We thank our teaching team members who participated in the study.
- American Association for the Advancement of Science. Vision and Change in Undergraduate Biology Education: A Call to Action. Washington, DC: 2011. http://visionandchange.org/files/2011/03/Revised-Vision-and-Change-Final-Report.pdf (accessed 8 November 2016) [ Google Scholar ]
- Andrews TC, Lemons PP. It’s personal: biology instructors prioritize personal evidence over empirical evidence in teaching decisions. CBE Life Sci Educ. 2015; 14 :ar7. [ PMC free article ] [ PubMed ] [ Google Scholar ]
- Baldwin RG. The climate for undergraduate teaching and learning in STEM fields. New Dir Teach Learn. 2009; 117 :9–17. [ Google Scholar ]
- Bloom BS, Krathwohl DR. Taxonomy of Educational Objectives: The Classification of Educational Goals, Handbook 1: Cognitive Domain. New York: Longmans; 1956. [ Google Scholar ]
- Bourrie DM, Cegielski CG, Jones-Farmer LA, Sankar CS. Identifying characteristics of dissemination success using an expert panel. Decision Sci J Innov Educ. 2014; 12 :357–380. [ Google Scholar ]
- Brownell SE, Tanner KD. Barriers to faculty pedagogical change: lack of training, time, incentives, and… tensions with professional identity. CBE Life Sci Educ. 2012; 11 :339–346. [ PMC free article ] [ PubMed ] [ Google Scholar ]
- Carleton KL, Rietschel CH, Marbach-Ad G. Group active engagements using quantitative modeling of physiology concepts in large-enrollment biology classes. J Microbiol Biol Educ. 2017; 17 ( in press ) [ PMC free article ] [ PubMed ] [ Google Scholar ]
- Connolly MR, Seymour E. 2015. Why Theories of Change Matter (WCER Working Paper No. 2015-2). www.wcer.wisc.edu/publications/workingPapers/papers.php (accessed 8 November 2016)
- Cox MD. Faculty learning communities: change agents for transforming institutions into learning organizations. To Improve the Academy. 2001; 19 :69–93. [ Google Scholar ]
- Creswell JW. Research Design: Qualitative, Quantitative, and Mixed Methods Approaches, 2nd ed. Thousand Oaks, CA: Sage; 2003. [ Google Scholar ]
- Dancy M, Henderson C. Pedagogical practices and instructional change of physics faculty. Am J Phys. 2010; 78 :1056–1063. [ Google Scholar ]
- Ebert-May D, Derting TL, Hodder J, Momsen JL, Long TM, Jardeleza SE. What we say is not what we do: effective evaluation of faculty professional development programs. BioScience. 2011; 61 :550–558. [ Google Scholar ]
- Eddy S, Hogan K. Getting under the hood: how and for whom does increasing course structure work. CBE Life Sci Educ. 2014; 13 :453–468. [ PMC free article ] [ PubMed ] [ Google Scholar ]
- Ellsworth JB. Surviving Change: A Survey of Educational Change Models. Washington, DC: Office of Educational Research and Improvement; 2000. [ Google Scholar ]
- Fairweather J, Colbeck C, Paulson K, Campbell C, Bjorklund S, Malewski E. Engineering Coalition of Schools for Excellence and Leadership (ECSEL): Year 6. University Park: Center for the Study of Higher Education, Penn State University; 1996. [ Google Scholar ]
- Felder RM. Reaching the second tier. J Coll Sci Teach. 1993; 23 :286–290. [ Google Scholar ]
- Fink LD. Creating Significant Learning Experiences: An Integrated Approach to Designing College Courses. Hoboken, NJ: Wiley; 2013. [ Google Scholar ]
- Freeman S, Eddy S, McDonough M, Smith MK, Okoroafor N, Jordt H, Wenderoth MP. Active learning increases student performance in science, engineering, and mathematics. Proc Natl Acad Sci USA. 2014; 111 :8410–8415. [ PMC free article ] [ PubMed ] [ Google Scholar ]
- Haag ES, Marbach-Ad G. Quantitative modeling of membrane transport and anisogamy by small groups within a large-enrollment organismal biology course. J Microbiol Biol Educ. 2017; 17 ( in press ) [ PMC free article ] [ PubMed ] [ Google Scholar ]
- Haak DC, HilleRisLambers J, Pitre E, Freeman S. Increased structure and active learning reduce the achievement gap in introductory biology. Science. 2011; 332 :1213–1216. [ PubMed ] [ Google Scholar ]
- Hamdan N, McKnight P, McKnight K, Arfstrom KM. A review of flipped learning. 2013. Flipped Learning Network. flippedlearning.org/wp-content/uploads/2016/07/LitReview_FlippedLearning.pdf (accessed 8 November 2016)
- Hammer D. Epistemological beliefs in introductory physics. Cogn Instr. 1994; 12 :151–183. [ Google Scholar ]
- Handelsman J, Miller S, Pfund C. Scientific Teaching. New York: Freeman; 2007. [ PubMed ] [ Google Scholar ]
- Hart Research Associates. Falling Short? College Learning and Career Success. Washington, DC: 2015. [ Google Scholar ]
- Henderson C. The challenges of instructional change under the best of circumstances: a case study of one college physics instructor. Am J Phys. 2005; 73 :778–786. [ Google Scholar ]
- Henderson C, Dancy MH. Barriers to the use of research-based instructional strategies: the influence of both individual and situational characteristics. Phys Rev Spec Top Phys Educ Res. 2007; 3 :020102. [ Google Scholar ]
- Jensen JL, Kummer TA, Godoy PD. Improvements from a flipped classroom may simply be the fruits of active learning. CBE Life Sci Educ. 2015; 14 :ar5. [ PMC free article ] [ PubMed ] [ Google Scholar ]
- Johnson DW, Johnson RT, Holubec EJ, Roy P. Circles of Learning: Cooperation in the Classroom. Alexandria, VA: Association for Supervision and Curriculum Development; 1984. [ Google Scholar ]
- Kezar A, Gehrke S, Elrod S. Implicit theories of change as a barrier to change on college campuses: an examination of STEM reform. Rev High Educ. 2015; 38 :479–506. [ Google Scholar ]
- Marbach-Ad G, McAdams KC, Benson S, Briken V, Cathcart L, Chase M, El-Sayed NM, Frauwirth K, Fredericksen B, Joseph SW, Lee V. A model for using a concept inventory as a tool for students’ assessment and faculty professional development. CBE Life Sci Educ. 2010; 9 :408–416. [ PMC free article ] [ PubMed ] [ Google Scholar ]
- Marbach-Ad G, Rietschel C, Saluja N, Carleton KL, Haag E. The use of group activities in introductory biology supports learning gains and uniquely benefits high-achieving students. J Microbiol Biol Educ. 2017; 17 ( in press ) [ PMC free article ] [ PubMed ] [ Google Scholar ]
- Maykut P, Morehouse R. Beginning Qualitative Research: A Philosophic and Practical Approach. Bristol, PA: Falmer; 1994. [ Google Scholar ]
- McKeachie W, Svinicki M. McKeachie’s Teaching Tips: Strategies, Research, and Theory for College and University Teachers. Boston. MA: Houghton Mifflin; 2006. [ Google Scholar ]
- Merriam SB. Qualitative Research: A Guide to Design and Implementation. San Francisco, CA: Jossey-Bass; 2009. [ Google Scholar ]
- Michaelsen LK, Black RH. In: Collaborative Learning: A Sourcebook for Higher Education, vol. 2. University Park: National Center on Postsecondary Teaching, Learning, and Assessment, Pennsylvania State University; 1994. Building learning teams: the key to harnessing the power of small groups in education; pp. 65–81. [ Google Scholar ]
- Michaelsen LK, Knight AB, Fink LD. Team-Based Learning: A Transformative Use of Small Groups in College Teaching. Sterling, VA: Stylus; 2004. [ Google Scholar ]
- Michaelsen LK, Sweet M, Parmelee DX (eds.), editors. Team-Based Learning: Small Group Learning’s Next Big Step, New Directions for Teaching and Learning, Number 116. Hoboken, NJ: Wiley; 2008. [ Google Scholar ]
- Okebukola PA. Cooperative learning and students’ attitudes to laboratory work. School Sci Math. 1986; 86 :582–590. [ Google Scholar ]
- Otero V, Pollock S, Finkelstein N. A physics department’s role in preparing physics teachers: the Colorado learning assistant model. Am J Phys. 2010; 78 :1218–1224. [ Google Scholar ]
- Preszler RW. Replacing lecture with peer-led workshops improves student learning. CBE Life Sci Educ. 2009; 8 :182–192. [ PMC free article ] [ PubMed ] [ Google Scholar ]
- Rogers EM. Diffusion of Innovations, 5th ed. New York: The Free Press; 2003. [ Google Scholar ]
- Schalk KA, McGinnis JR, Harring JR, Hendrickson A, Smith AC. The undergraduate teaching assistant experience offers opportunities similar to the undergraduate research experience. J Microbiol Educ. 2009; 10 :32–42. [ PMC free article ] [ PubMed ] [ Google Scholar ]
- Seidel SB, Tanner KD. What if students revolt?—Considering student resistance: origins, options, and opportunities for investigation. CBE Life Sci Educ. 2013; 12 :586–595. [ PMC free article ] [ PubMed ] [ Google Scholar ]
- Seymour E, Hewitt NM. Talking about Leaving: Why Undergraduates Leave the Sciences. Boulder, CO: Westview; 1997. [ Google Scholar ]
- Shekhar P, Demonbrun M, Borrego M, Finelli C, Prince M, Henderson C, Waters C. Development of an observation protocol to study undergraduate engineering student resistance to active learning. Int J Eng Educ. 2015; 31 :597–609. [ Google Scholar ]
- Watson WE, Kumar K, Michaelsen LK. Cultural diversity’s impact on interaction process and performance: comparing homogeneous and diverse task groups. Acad Manage J. 1993; 36 :590–602. [ Google Scholar ]
- Weimer M. Learner-Centered Teaching. San Francisco: Jossey-Bass; 2013. [ Google Scholar ]
- Wieman C, Perkins K, Gilbert S. Transforming science education at large research universities: a case study in progress. Change. 2010; 42 :6–14. [ Google Scholar ]
- Wiggins GP, McTighe J. Understanding by Design. Alexandria, VA: Association for Supervision and Curriculum Development; 2005. [ Google Scholar ]
- Yin RK. Case Study Research: Design and Methods, 3rd ed. Thousand Oaks, CA: Sage; 2003. [ Google Scholar ]
Thank you for visiting nature.com. You are using a browser version with limited support for CSS. To obtain the best experience, we recommend you use a more up to date browser (or turn off compatibility mode in Internet Explorer). In the meantime, to ensure continued support, we are displaying the site without styles and JavaScript.
- View all journals
- Explore content
- About the journal
- Publish with us
- Sign up for alerts
- Open access
- Published: 02 September 2024
Enabling target-aware molecule generation to follow multi objectives with Pareto MCTS
- Yaodong Yang ORCID: orcid.org/0000-0001-8660-2376 1 ,
- Guangyong Chen ORCID: orcid.org/0000-0002-5892-8608 2 ,
- Jinpeng Li 1 ,
- Junyou Li 2 ,
- Odin Zhang 3 ,
- Xujun Zhang 3 ,
- Lanqing Li ORCID: orcid.org/0000-0003-1998-4022 2 ,
- Jianye Hao ORCID: orcid.org/0000-0002-0422-8235 4 ,
- Ercheng Wang ORCID: orcid.org/0000-0003-2074-4077 2 &
- Pheng-Ann Heng 1
Communications Biology volume 7 , Article number: 1074 ( 2024 ) Cite this article
Metrics details
- Computational models
- Pharmaceutics
Target-aware drug discovery has greatly accelerated the drug discovery process to design small-molecule ligands with high binding affinity to disease-related protein targets. Conditioned on targeted proteins, previous works utilize various kinds of deep generative models and have shown great potential in generating molecules with strong protein-ligand binding interactions. However, beyond binding affinity, effective drug molecules must manifest other essential properties such as high drug-likeness, which are not explicitly addressed by current target-aware generative methods. In this article, aiming to bridge the gap of multi-objective target-aware molecule generation in the field of deep learning-based drug discovery, we propose ParetoDrug, a Pareto Monte Carlo Tree Search (MCTS) generation algorithm. ParetoDrug searches molecules on the Pareto Front in chemical space using MCTS to enable synchronous optimization of multiple properties. Specifically, ParetoDrug utilizes pretrained atom-by-atom autoregressive generative models for the exploration guidance to desired molecules during MCTS searching. Besides, when selecting the next atom symbol, a scheme named ParetoPUCT is proposed to balance exploration and exploitation. Benchmark experiments and case studies demonstrate that ParetoDrug is highly effective in traversing the large and complex chemical space to discover novel compounds with satisfactory binding affinities and drug-like properties for various multi-objective target-aware drug discovery tasks.
Similar content being viewed by others
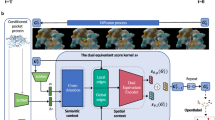
A dual diffusion model enables 3D molecule generation and lead optimization based on target pockets
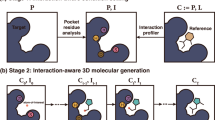
3D molecular generative framework for interaction-guided drug design
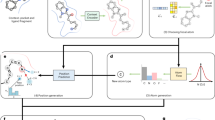
PocketFlow is a data-and-knowledge-driven structure-based molecular generative model
Introduction.
The rational design of molecules to act as clinical drugs remains a significant challenge in biopharmaceutical research, especially concerning the attainment of favorable physiochemical and pharmacological properties. In support of such endeavors, target-based drug discovery aims to identify small-molecule ligands that exhibit high affinity and specificity for a particular protein pocket structure 1 . Traditionally, target-based drug discovery has been approached through either high-throughput experimental methods or virtual screening of extensive chemical databases 2 , 3 targeted at specific biomolecular targets 4 , 5 . Subsequently, the screening of bioanalytical indicators through elaborate clinical experiments is conducted to evaluate drug-like properties. This pursuit contributes to the conventional 10-year drug development cycle and staggering research and development costs of approximately 2.8 billion USD, coupled with a remarkably high failure rate. The predetermined selection of compounds for screening further constrains the exploration of chemical space, tethering it to historical knowledge derived from previously investigated molecules. This ultimately leads to a fervent industry focus on popular drug targets, resulting in the challenge that the molecules selected through screening are unable to avoid patent restrictions. In contrast, recent advancements in target-aware molecule generation, particularly the development of generative models trained on extensive datasets, present a promising paradigm shift. These models, rooted in deep learning, offer an innovative approach to expedite ligand discovery and optimization. They achieve this by generating entirely novel and diverse molecules capable of binding to a specified protein target, starting from scratch 6 . This transformative approach holds great potential to overcome the limitations associated with traditional methods, offering a more efficient and expansive exploration of the entire chemical space.
Since the first inception of an autoencoder model conditioned on targeted proteins in 2018 7 , there has been rapid progress in deep learning-based target-aware molecule generation methods. Various works take advantage of conditional generative models, such as the autoencoder 7 , 8 , 9 , generative adversarial network 10 , and diffusion model 11 , 12 , to infer entire molecules through a one-time feedforward process, incorporating binding site information as input. Moreover, to enhance structural representation, convolutional neural networks 7 and graph convolutional networks 8 are employed. In the meantime, some approaches utilize voxelized representations 10 or atomic density grids 13 to characterize compound-receptor complexes. Another pivotal category of deep learning-based target-aware drug discovery involves autoregressive generative models, which predict the next atom (and its position) sequentially conditioned on the molecular fragment and binding site information. To model the conditioned intermediate context, diverse network architectures like transformers 14 , 15 , recurrent neural networks 16 , 17 , and flow models 18 are introduced as the context encoder. Additionally, graph neural networks 18 , 19 , 20 are widely utilized to extract chemical and geometrical features of ligands and protein pockets. However, these efforts are not yet integrated into mainstream drug discovery practices, and a significant obstacle lies in the inherent multi-objective optimization nature of drug discovery 21 . Beyond strong binding affinity to the targeted protein, drug molecules must exhibit other desirable properties, such as high drug-likeness and low toxicity. Presently, existing deep learning-based target-aware generative methods predominantly focus on the single objective of optimizing binding affinity. The multi-objective nature of drug molecules, with sometimes conflicting demands, necessitates ongoing development of novel multi-objective target-aware drug discovery techniques to enhance the overall success rates of drug discovery.
Conversely, numerous studies have explored the domain of general multi-objective drug discovery. Certain approaches, such as MolGPT 22 , fall within the ligand-based methodology, aspiring to generate novel compounds with favorable physicochemical properties. However, these methods fall short in incorporating protein information, thus lacking assurance that the generated molecules can effectively bind to specified protein targets. Concurrently, other methodologies like MCMG 21 , RationaleRL 23 , MolSearch 24 , and GENERA 25 aim to optimize not only the binding affinity objective but also other property objectives. Specifically, these methodologies leverage optimization techniques such as reinforcement learning 26 and genetic algorithms 27 to enhance the binding affinity objective predicted by machine learning-based or simulation-based docking score functions. However, a notable drawback is their failure to explicitly incorporate target protein information when constructing generative models. The absence of protein information renders the optimization of the binding affinity objective inefficient, and the resulting generative models from these target-scoring-based methods cannot be readily generalized to other protein targets. In contrast to ligand-based and target-scoring-based approaches, a recent development is CProMG 28 , designed to generate molecules that meet multiple property constraints with an enhanced representation of protein structure information. CProMG treats this task as a multi-constraint molecule generation problem, with each property constraint set to exceed a predefined threshold. However, CProMG does not attempt to maximize molecule properties through optimization techniques for a comprehensive exploration of the chemical space. A more in-depth discussion is provided in the Discussion section.
Similar challenges also exist in natural language generation tasks, where models predicting the next token often express unintended behaviors, such as making up facts, generating biased or toxic text, or not following user instructions. To address this issue, OpenAI focuses on fine-tuning approaches to align language models. Specifically, they employ reinforcement learning from human feedback (RLHF) to fine-tune GPT-3 29 to follow a broad class of written instructions 30 . In contrast to the fuzzy, hard-to-quantify human values in natural language tasks, we can explicitly calculate multiple molecular metrics in the context of drug development.
In this study, we explore the use of an autoregressive Pareto Monte Carlo Tree Search (MCTS) generation algorithm named ParetoDrug for the design of drug molecules to address the existing gap in multi-objective target-aware drug discovery within the domain of deep learning-based drug discovery. This algorithm effectively facilitates the simultaneous optimization of multiple molecule properties. In its operation, ParetoDrug first explores molecules on the Pareto Front within the chemical space. It achieves this by maintaining a global pool comprising Pareto optimal molecules, each of which is not surpassed by another molecule in the same pool across every property objective. During the exploration process, ParetoDrug leverages existing pretrained autoregressive target-aware molecule generation models to guide the search for the next atom symbol, facilitating the identification of molecules with high binding affinity to protein targets. Additionally, in the selection of the next atom symbol, ParetoDrug introduces a scheme named ParetoPUCT. This scheme is designed to balance the exploration of chemical space and the exploitation of the pretrained autoregressive generative model. Through these strategies, ParetoDrug owns the ability to generate molecules with multiple desirable properties, including binding affinity. Computational evaluations on the benchmark dataset and case studies, including multi-objective target-aware drug discovery tasks for known drugs (e.g., Tropifexor and Copanlisib), a multi-target drug discovery task for HIV-related disease targets, and a multi-target multi-objective drug discovery task for a dual-inhibitor Lapatinib, demonstrate the high effectiveness of ParetoDrug. The algorithm exhibits proficiency in discovering small-molecule drug candidates possessing multiple required properties, particularly including binding affinities to specified protein targets.
In this section, we first conduct the experiments on a benchmark to demonstrate ParetoDrug’s remarkable ability to generate molecules with multiple desired properties including the binding affinity and drug-like properties when compared with various baselines. Meanwhile, we also give the statistical analysis of the generated molecules of ParetoDrug. Then we use ParetoDrug to perform the case studies for the multi-objective target-aware drug discovery task, multi-target drug discovery task, and multi-target multi-objective drug discovery task respectively. In these case studies, ParetoDrug is able to generate the Pareto Dominate molecules over the known drug ligands in terms of the specified molecule property objectives, which exhibits the promising molecule discovery potential of ParetoDrug.
Benchmark experiments
In the benchmark experiments, we follow the settings as Qian et al. 15 where there are 100 protein targets sampled from the public database of protein-ligand pairs BindingDB 31 as the test set. For each test protein target, we generate 10 candidate molecules for evaluation. All 1000 candidate molecules are evaluated by a set of molecule property metrics, and the scores are averaged for an overall comparison. Please refer to Supplementary Information A and B for a detailed experimental and hyperparameter setup. We use several important metrics to evaluate the generated molecules, including docking score, uniqueness, LogP, QED, SA score, and NP-likeness described as follows.
Docking score . Binding energy is regarded as a general indicator to describe the binding affinity between molecule ligands and target proteins. Specifically, we utilize a free and widely used tool called smina 32 to compute the binding affinity. We use the negative value of the output by smina as the docking score. The higher the docking score is, the better the molecule is docked into the target protein.
Uniqueness . Drug design models should be able to generate different molecules conditioning on different target proteins. The higher the uniqueness value is, the more sensitive the model is to the specified target protein. This metric is computed as follows:
where \({{\mathbb{S}}}_{{{{{\rm{p}}}}}}\) indicates the set of test proteins, \({M}_{{S}_{{{{{\rm{p}}}}}}}\) denotes the collection of generated molecules for the target protein \({S}_{{{{{\rm{p}}}}}}\in {{\mathbb{S}}}_{{{{{\rm{p}}}}}}\) , # counts the number of molecules, and Set is an operator to remove the repeated molecules in the given set.
LogP . A large LogP value indicates the substance is lipophilic, while a small LogP value means it is easy to dissolve in water. According to Ghose filter 33 , the LogP value of a druggable molecule should range from −0.4 to +5.6.
QED . This score measures the drug-likeness and ranges from 0 to 1. A higher QED score indicates that a molecule is more likely to be a potential drug-like compound, with the desired molecular properties such as hydrogen bond acceptor, hydrogen bond donor, and polar molecular surface area 34 .
SA score . The synthetic accessibility (SA) score indicates how difficult one molecule is to synthesize, which is calculated based on a combination of fragment contributions and a complexity penalty 35 . The range of the estimated SA metric is from 1 (easy to make) to 10 (very difficult to make).
NP-likeness . Natural products play an important role in the history of drug discovery. Many drugs are natural products and their derivatives. The higher the score is, the more likely the molecule is to be a natural product. The calculated NP-likeness is typically in the range from -5 to 5 36 .
The reported results of “Known ligands”, SBMolGen, LiGANN, SBDD-3D, and BeamLmser are from AlphaDrug 15 . The “Known ligands” indicates the original molecules binding to protein targets in the database. The results of LiGANN 10 were collected on the web-based application provided in the original paper. SBMolGen 37 is developed from ChemTS 38 for target-specific molecular generation. The results of SBDD-3D 18 were based on the released codes and trained model published by the authors. BeamLmser applies the beam search on the pretrained Lmser Transformer 15 . The beam size of BeamLmser is set at 10 to collect 10 molecules for each test protein target. Besides the above representative baselines, we also test three recent advanced methods. The first is Pocket2Mol 20 , which uses the equivariant generative network and autoregressive sampling scheme to generate three-dimensional molecules. For Pocket2Mol, we utilize the official codes and trained model for sampling molecules. The second is TargetDiff 12 , which develops a three-dimensional equivariant diffusion model to sample molecules. For TargetDiff, we also use the officially released trained model and codes for sampling. We keep the sample numbers of Pocket2Mol and TargetDiff at 100 for each test protein, which is the default configuration to ensure the quality of generated molecules. To make a fair comparison with other methods, for each test protein target, we randomly select 10 molecules from the generated 100 molecules of Pocket2Mol and TargetDiff for the evaluation. The third is CProMG 28 , which proposes a multi-constraint autoregressive model to generate small molecules with controllable properties. We use the official codes and default configurations of CProMG to generate 10 molecules for each test protein with the pretrained CProMG-VQSLT model, which is trained to control multiple property metrics including the docking score, LogP, QED, and SA score that are evaluated here.
Besides the above basic generative models, there also emerges another kind of fundamental approach that integrates the powerful MCTS-based searching technique to better control the molecule generation procedure of the pretrained autoregressive generative models with the simulation feedback, and AlphaDrug and the proposed ParetoDrug fall into this kind. For AlphaDrug 15 which utilizes MCTS with the pretrained Lmser Transformer model to generate molecules based on given protein targets, we run the official codes and set iteration times (IT) at 150 when selecting the next atom symbol in MCTS. For ParetoDrug which conducts Pareto MCTS with the same pretrained Lmser Transformer model, we also set IT at 150 and let it optimize all objectives (docking score, LogP, QED, SA score, and NP-likeness) synchronously except the unoptimizable Uniqueness, which is a statistic metric for all generated molecules. In addition, we set the metric value of LogP as 1 if the molecule’s LogP value is in the range of [ − 0.4, 5.6], and 0 otherwise. After each Pareto MCTS, ParetoDrug obtains a global pool of Pareto optimal molecules. We choose the molecule with the largest reward vector summation value from the pool, which means this molecule has top rankings in each property metric. When testing, we collect 10 generated molecules for each test protein target from AlphaDrug and ParetoDrug.
Additionally, we compare a multi-objective drug discovery algorithm REINVENT 4 39 while its generation model is not conditioned on the protein information. It uses a reinforcement learning algorithm to generate optimized molecules compliant with a user-defined property profile defined as a multi-component score. We let REINVENT 4 optimize the docking score, LogP, QED, SA, and NP while setting their weights in the property profile all at 0.2. For each test protein target, we collected 10 molecules with the highest multi-component scores during the training process of REINVENT 4.
The results are shown in Table 1 and the direction of the arrow in the table means a better property score. The 95% confidence intervals for property scores of RL/MCTS are included. As we see, in terms of the docking score, ParetoDrug demonstrates superiority over all baselines except AlphaDrug. However, AlphaDrug is a single-objective target-aware drug discovery method that only optimizes the binding affinity. As AlphaDrug and ParetoDrug have the same iteration budgets (IT=150) for each atom symbol in sequence but ParetoDrug needs to optimize multiple objectives including the binding affinity, it is expected that ParetoDrug has a lower docking score than AlphaDrug. Meanwhile, although the docking score of ParetoDrug decreases slightly, other metrics including QED, SA score, and NP-likeness are improved significantly compared with AlphaDrug. Notably, QED changes from 0.4 to 0.6 (50% improvement) while NP-likeness changes from -0.9 to -0.4 (55.6% improvement). For the special LogP metric, although the average LogP value of AlphaDrug falls into the druggable molecule range, only 52.7% generated molecules of AlphaDrug satisfy the LogP range constraint if tested individually. On the contrary, 96.5% (83.1% improvement over AlphaDrug) generated molecules of ParetoDrug satisfy the LogP range constraint. These impressive results demonstrate that ParetoDrug is able to address the multi-objective target-aware drug discovery task by discovering novel compounds that possess multiple satisfactory properties including the binding affinity. On the other hand, we observe that the pretrained autoregressive Lmser Transformer with beam search (BeamLmser) cannot generate molecules with higher docking scores than the most recent TargetDiff. But with MCTS replacing beam search, AlphaDrug greatly boosts Lmser Transformer’s performance to find molecules with stronger binding affinity than BeamLmser even with the same docking time budgets 15 . Furthermore, ParetoDrug proposes the multi-objective Pareto MCTS to replace the MCTS used in AlphaDrug. With the same iteration times, ParetoDrug significantly improves multiple molecule properties compared with AlphaDrug while maintaining the docking score at the same level. Additionally, when compared with the multi-constraint conditional generation method CProMG, ParetoDrug has advantages in docking score, Uniqueness, SA score, and NP-likeness. In addition, the Uniqueness of CProMG is only 26.9% as it generates the same molecules for different protein targets, which is undesirable in de novo target-aware drug discovery tasks. Lastly, for REINVENT 4 which does not belong to the kind of target-aware drug discovery methods, we could see although it achieves superior performance in some metrics such as QED and NP, its docking score is much lower than ParetoDrug as it does not encode the protein-ligand prior to its generation model. This also indicates the importance of incorporating the protein target information into the molecule generation process as in the generative target-aware drug discovery methods.
Next, we conduct the statistical analysis with kernel density estimate 40 , which is analogous to a histogram but endowed with benefits such as smoothness and continuity. The property distributions of molecules generated by TargetDiff, AlphaDrug, and ParetoDrug are shown in Fig. 1 . For TargetDiff, here we use 10 molecules with the highest docking scores among the generated 100 molecules for each test protein to make an aligned comparison. We can see that although the docking score distributions of the three methods are similar while AlphaDrug is slightly better, other property distributions present differently. For LogP, ParetoDrug satisfies the range constraint of [ − 0.4, 5.6] while TargetDiff tends to generate more molecules with LogP values below the lower bound and AlphaDrug tends to generate more molecules with LogP values above the upper bound. Besides, ParetoDrug is able to generate more molecules with high QED values than the other two methods especially when QED is larger than 0.8 that molecules are very likely to be potential initiators of a drug candidate. Meanwhile, TargetDiff’s molecules are with significantly higher SA values than ParetoDrug, which means that TargetDiff’s molecules are much harder to synthesize. These statistical findings demonstrate that ParetoDrug has better molecule distributions than AlphaDrug and TargetDiff when taking multiple properties into account. More comparisons of computational efficacy, score distributions of a specific target, and the diversity of generated molecules between ParetoDrug and other methods could be referred to Supplementary Information C (and Supplementary Table 1 ), D (and Supplementary Fig. 1 ), and E (and Supplementary Table 2 ).
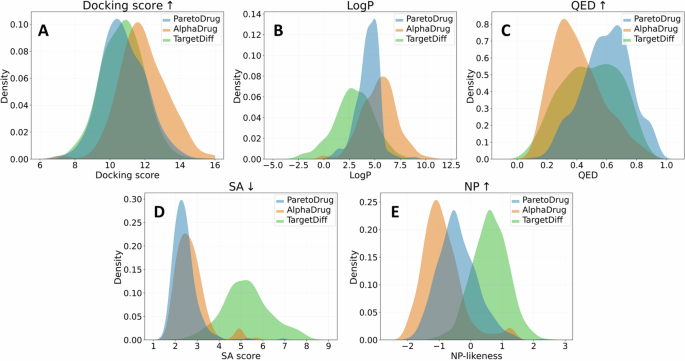
A The docking score (kcal ⋅ mol −1 ) distributions of each method. B The LogP value distributions of each method. C The QED value distributions of each method. D The SA score distributions of each method. E The NP-likeness distributions of each method.
Case studies for multi-objective target-aware drug discovery
Here we use two case studies of disease protein targets to show the molecule discovery ability of ParetoDrug for the multi-objective target-aware drug discovery tasks. The molecule objectives optimized by ParetoDrug are the docking score, LogP, QED, SA score, and NP-likeness. Additionally, the binding affinity is further validated by MM-GBSA 41 , 42 , which is a more accurate metric than docking scores but computationally expensive. For the analysis of the protein-ligand interactions, we use PLIP 43 and detailed can be referred to Supplementary Information F .
Case 1: targeting FXR
Non-alcoholic fatty liver disease (NAFLD) is defined as the excessive and abnormal intracellular accumulation of lipids in the liver, primarily in the form of triglycerides 44 , 45 . Currently, NAFLD has been the most common cause of chronic liver disease, especially in Western countries, and the estimated prevalence of NAFLD is approximately 30% in the general population 46 , 47 . One of the best-known drugs for NAFLD is Tropifexor, which acts as an agonist of the farnesoid X receptor (FXR). The structural basis of Tropifexor as a potent and selective agonist of FXR is shown in Fig. 2 A (PDB ID: 7D42) 48 . In this case study, we use ParetoDrug to discover potential drug molecules with desired computational properties for FXR. Using ParetoDrug, we collect 10 molecules and find four Pareto Dominate molecules compared with Tropifexor. The chemical structures of Tropifexor and the discovered ligands by ParetoDrug for FXR are shown in Fig. 2 B. Table 2 shows the property metrics of different ligands. Our ParetoDrug model discovers multiple ligands that outperform Tropifexor on all the optimized properties. Especially, the SA scores of the new ligands are much lower than Tropifexor, which means that they are easier to synthesize. We also run AlphaDrug and TargetDiff to collect molecules for FXR, however, no Pareto Dominate molecule over Tropifexor is found for the two methods. For example, the best molecule from AlphaDrug (with the most number of better properties than Tropifexor) has the Docking score at 12.9, LogP at 4.1, QED at 0.3, SA at 2.6, and NP at -1.59. Compared with it, Compound 4 generated by ParetoDrug has 3 better properties (Docking score, QED, and NP) and 1 worse property (SA). Meanwhile, the best molecule from TargetDiff (with the most number of better properties than Tropifexor) has the Docking score at 12.8, LogP at 4.9, QED at 0.48, SA at 7.7, and NP at 1.01. Compared with it, Compound 4 generated by ParetoDrug has 3 better properties (Docking score, QED, and SA) and 1 worse property (NP). As shown in Fig. 2 C, the docked poses and interactions of these four discovered compounds are quite different compared with Tropifexor. More specifically, one hydrogen bond forms between Tropifexor and the amino-acid residue MET265. At the same time, Compounds 1, 3, and 4 with new scaffolds form new hydrogen bonds with other residues (Compound 1 with THR288 and TYR369, Compound 3 with HIS294, and Compound 4 with THR288) while no hydrogen bond forms between Compound 2 and FXR.
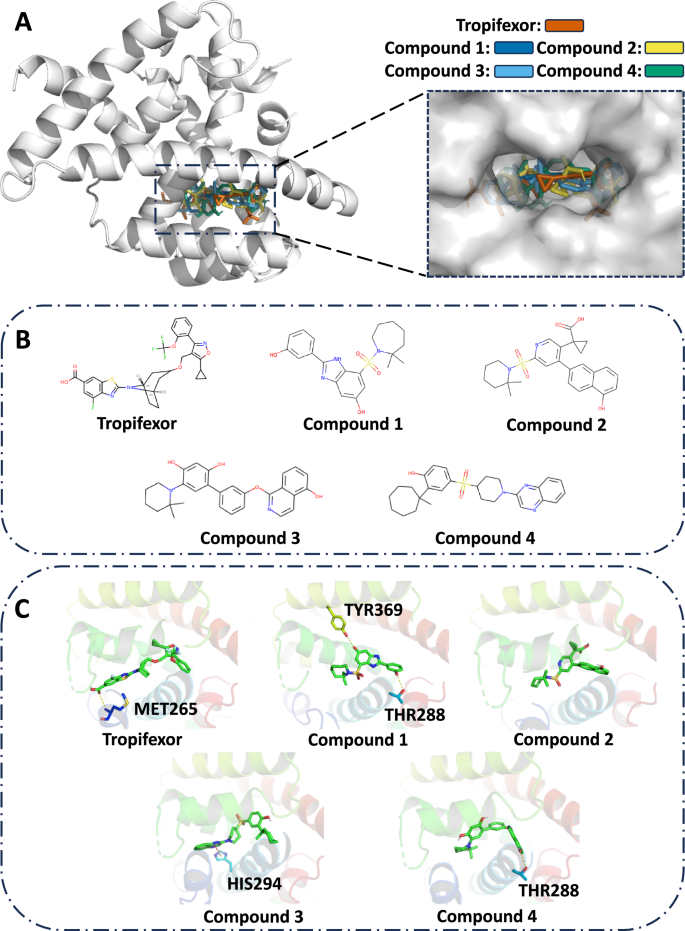
Tropifexor is an agonist of FXR and Compounds 1–4 are found by ParetoDrug. Hydrogen bonds are displayed in yellow dashed lines and π - π interactions are in red. A Solvent-accessible surfaces of the binding pocket of FXR for Tropifexor and Compounds 1 to 4. B Chemical structures of Tropifexor and Compounds 1–4. C The binding poses of Tropifexor and Compounds 1–4.
Besides the docking score, MM-GBSA rescoring based on molecular dynamics simulations is used to further computationally validate the discovered compounds 41 . MM-GBSA uses molecular mechanics with generalized Born surface area to determine highly potential inhibitors for targets. The detailed settings of MM-GBSA are provided in Supplementary Information G . In this case, we use MM-GBSA to further validate the generated Pareto optimal molecules with promising docking scores. As shown in Table 2 , the MM-GBSA scores indicate that the generated molecules have the same level of binding free energies as the known drug Tropifexor. Surprisingly, although two hydrogen bonds formed between Compound 1 and FXR, the MM-GBSA scores show no significant difference. Possibly because hydrogen bonding interaction is ignored in the MM-GBSA calculation.
Case 2: targeting PI3K- γ
Follicular lymphoma (FL) is a systemic neoplasm of the lymphoid tissue displaying germinal center B-cell differentiation, which belongs to a cancer that involves certain types of white blood cells known as lymphocytes. FL represents 5% of all hematological neoplasms and about 20-25% of all new non-Hodgkin lymphoma diagnoses in Western countries 49 . One of the best-known drugs for FL is Copanlisib, which has been shown to affect the survival and spread of cancerous B-cells. The structural basis of the PI3K- γ related to FL in complex with Copanlisib is shown in Fig. 3 A (PDB ID: 5G2N) 50 . Here we use ParetoDrug to discover potential drug molecules with desired computational properties for PI3K- γ . We collect 10 molecules and find one Pareto Dominate molecule (Compound 5) compared with Copanlisib (Fig. 3 B). As shown in Fig. 3 C, Compound 5 found by ParetoDrug has three hydrogen bonds with surrounding residues (VAL882, ASP836, and LYS833) and two π - π stackings with surrounding residues (TRP812 and TYR867). Notably, the hydrogen bonds to VAL882 and LYS833 as well as π - π stacking to TYR867 also appear in Copanlisib’s docking interactions. Meanwhile, Table 3 shows the computational metric values of Copanlisib and Compound 5. Compound 5 is better than Copanlisib in terms of the optimized molecule metric objectives. However, the MM-GBSA scores indicate that the binding strength of Compound 5 decreases compared with Copanlisib (-46.48 kcal ⋅ mol −1 vs. -55.51 kcal ⋅ mol −1 ). The possible reason is that hydrogen bonding and π - π interactions are not considered in the energy terms of MM-GBSA. We also run AlphaDrug and TargetDiff to collect molecules for PI3K- γ and no Pareto Dominate molecule over Copanlisib is found by the two methods. For example, the best molecule from AlphaDrug (with the most number of better properties than Copanlisib) has the Docking score at 12.4, LogP at 4.35, QED at 0.41, SA at 5.0, and NP at 1.29. Meanwhile, the best molecule from TargetDiff (with the most number of better properties than Copanlisib) has the Docking score at 11.6, LogP at 3.2, QED at 0.56, SA at 3.9, and NP at 0.18. Although with some good properties, the two top molecules from AlphaDrug and TargetDiff cannot dominate the drug Copanlisib with worse SA.
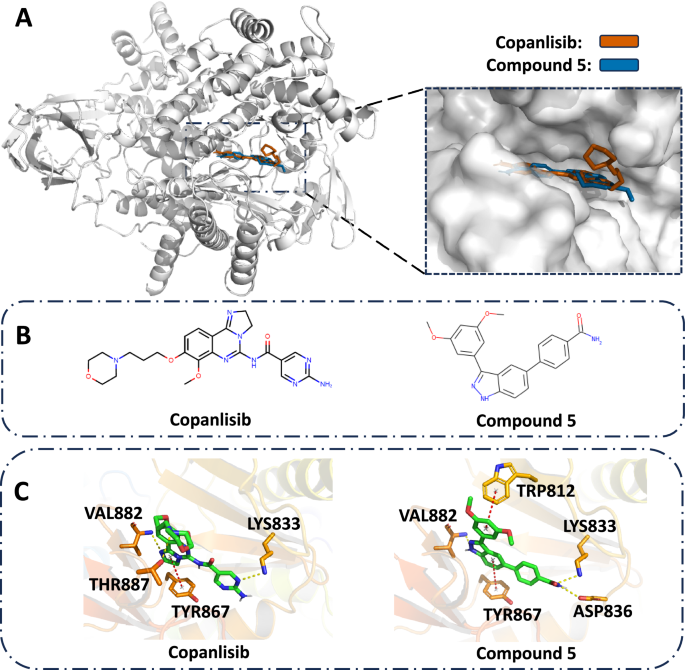
Copanlisib is a drug binding to PI3K- γ and Compound 5 is found by ParetoDrug. Hydrogen bonds are displayed in yellow dashed lines and π - π interactions are in red. A Solvent-accessible surfaces of the binding pocket of PI3K- γ for Copanlisib and Compound 5. B Chemical structures of Copanlisib and Compound 5. C The binding poses of Copanlisib and Compound 5.
While the in silico computational metrics of molecules discovered by ParetoDrug show promise in comparison to existing drugs, it is crucial to acknowledge that these molecules are still far from being drugs. Drug discovery is an extremely complicated process, and the current metrics for molecules cannot perfectly reflect the physicochemical properties required for a compound to be a drug. Nevertheless, we clearly see ParetoDrug’s promising potential in addressing multi-objective target-aware drug discovery tasks.
Case study for multi-target drug discovery
Multi-target drug discovery can be considered a special case of multi-objective drug discovery where each protein target is going to be regarded as an objective to optimize. Until now, the study of multi-target target-aware drug discovery remains underexplored as it is challenging to consider the information of multiple protein targets at the same time to derive one ligand that could bind to all these given targets. Meanwhile, previous generative target-aware drug discovery works mainly focus on the single-target situation as there lack data sets to train the multi-target conditioned generative models. In this case study, we use ParetoDrug to perform a multi-target target-aware drug discovery task to design dual-functional inhibitors for both the HIV protease (HIV-PR) and HIV reverse transcriptase (HIV-RT). ParetoDrug is slightly modified to be compatible with this kind of task, and details are given in the Method section.
The crystal structures of HIV-PR and HIV-RT used here are 3A2O and 4G1Q 51 . Both structures are complexes with potent inhibitors solved at high resolution. We compare with LigBuilder V3 51 , the first de novo multi-target drug design program, and the variants of Pocket2Mol and TargetDiff extended by combining with screening. There are three different strategies in LigBuilder V3, including multi-target de novo design, multi-target growing, and multi-target linking. The best molecules for each strategy from the original paper are reported here. For the variants of Pocket2Mol and TargetDiff, we use each method to generate 100 molecules for each target. Then we use smina to screen the generated 200 molecules of each method to find the best molecule that has the best docking scores for both targets. We call the variants as Pocket2Mol-screen and TargetDiff-screen, and report the best molecules of each variant.
Results of both the docking scores and MM-GBSA scores for each method’s best molecule are shown in Table 4 . For ParetoDrug, we additionally report another two top molecules (Compound 7 and Compound 8) which are almost the same good as Compound 6 in terms of the docking scores. As shown in Table 4 , the promising docking scores and MM-GBSA scores of Compounds 6–8 demonstrate that they are potential strong dual inhibitors to the given two protein targets in this task. Additionally, Fig. 4 shows Compounds 6–8 and their docking poses and interactions with HIV-PR (PDB ID: 3A2O) and HIV-RT (PDB ID: 4G1Q). Interestingly, the similar structures of Compounds 6 to 8 in Fig. 4 B indicate that ParetoDrug found a chemical subspace of strong inhibitors for both the HIV-PR and HIV-RT targets.
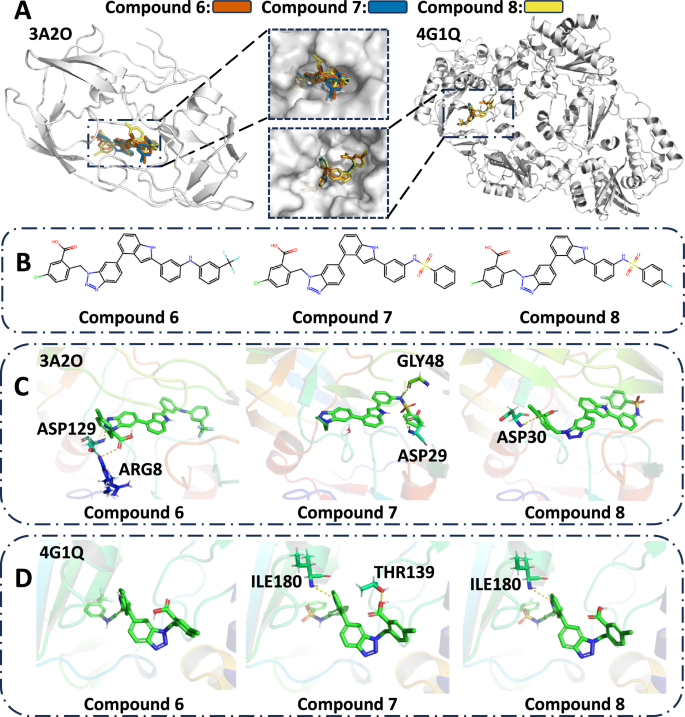
Compounds 6–8 are found by ParetoDrug. Hydrogen bonds are displayed in yellow dashed lines. A Solvent-accessible surfaces of the binding pockets of HIV-PR (left) and HIV-RT (right) for Compounds 6–8. B Chemical structures of Compounds 6–8. C The binding poses of Compounds 6 to 8 with HIV-PR. D The binding poses of Compounds 6–8 with HIV-RT.
Case study for multi-target multi-objective drug discovery
We have shown ParetoDrug’s promising ability for both the multi-objective target-aware drug discovery and multi-target drug discovery tasks separately. Naturally, a more attractive and challenging task is the multi-target multi-objective drug discovery task where the generated molecules need to bind to a given set of protein targets while manifesting other desired computational molecule properties. To the best of our knowledge, there are no published works yet to specifically address this kind of task. Here we use Lapatinib as a case study to evaluate ParetoDrug’s ability for the multi-target multi-objective drug discovery task. Lapatinib is a dual tyrosine kinase inhibitor that interrupts the EGFR pathway and inhibits HER4/ErbB4 Kinase for the treatment of breast cancer 52 , 53 . 1XKK and 3BBT are respectively the PDB IDs of crystal structures for Lapatinib binding to EGFR 54 and the HER4/ErbB4 kinase 55 . In this task, we configure ParetoDrug to bind to both protein targets while optimizing LogP, QED, SA, and NP metrics synchronously with IT at 150. We compare the generated molecules in the global Pareto pool with the known dual-inhibitor drug Lapatinib. Impressively, plenty of Pareto Dominate molecules over Lapatinib are discovered by ParetoDrug, as shown in Fig. 5 . Furthermore, the QED values of Compound 12 and Compound 14 are greater than 0.8, which indicates the two molecules are potential initiators for a drug.
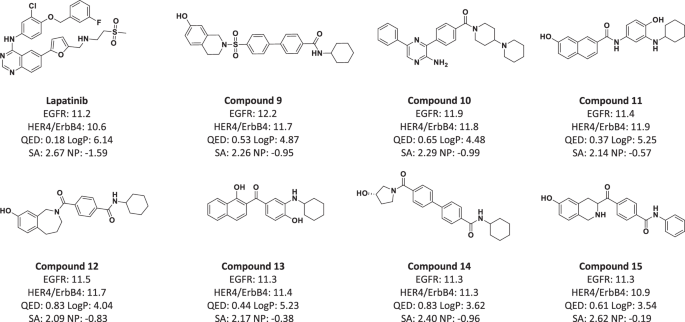
EGFR means the ligand’s docking score to EGFR (PDB ID: 1XKK) while HER4/ErbB4 means the ligand’s docking score to HER4/ErbB4 kinase (PDB ID: 3BBT), and the unit for the two docking scores is kcal ⋅ mol −1 .
In this work, we divide multi-objective drug discovery methods into three kinds based on whether they use the target protein information. The representative works of each kind of method and their comparisons are provided in Table 5 . The first kind is the ligand-based method such as MolGPT 22 , which utilizes the conditional transformer to generate molecules that satisfy multiple inputting property constraints. However, the ligand-based method does not consider protein information and thus cannot guarantee to generate molecules with high binding affinity to a given protein target.
The second kind is the target-scoring-based method that employs a docking scoring function to predict the binding affinity of the generated molecules to the given protein target. In this way, although the target-scoring-based method also does not explicitly consider the target protein information, the binding affinity scores could be optimized by optimization techniques such as reinforcement learning and genetic algorithm. For example, Wang et al., propose MCMG 21 to combine conditional transformer, knowledge distillation, and reinforcement learning to generate molecules that satisfy multiple constraints including binding to targets such as GSK3 β and JNK3. However, MCMG does not incorporate the target protein information into the generation process of its model and needs to design a reward that is a linear combination of each metric. Recently, MolSearch 24 is proposed to use multi-objective MCTS to generate molecules based on molecule fragments. However, MolSearch is a pure search-based method with predefined massive rules to modify molecules and also does not consider the target protein information. Furthermore, REINVENT 4 39 uses a reinforcement learning algorithm to generate optimized molecules compliant with a user-defined property profile defined as a multi-component score. However, it is not a generative target-aware method although it optimizes multiple objectives while treating the binding affinity as a standard optimizing objective. The lack of specific protein information in these target-scoring-based methods makes the optimization of binding affinity objective inefficient and the trained models cannot be generalized to other target proteins. Therefore, this kind of method is different from the mainstream target-aware molecule generation in that the protein-ligand interactions are modeled in the molecule generation process. Meanwhile, most of them are only evaluated on several case studies which limits the assessment of their generality on various target proteins. For example, RationaleRL 23 , MCMG 21 , and MolSearch 24 optimize the molecule’s binding affinity to GSK3 β and JNK3, which is predicted by random forest models pretrained on the data sets 56 that contain samples of positive and negative compounds to the GSK3 β and JNK3 targets, and are not available for most protein targets.
The third kind of method is the multi-objective target-aware molecule generation, which models the protein-ligand interactions to generate the molecules with high binding affinity to the inputting protein target. Recently, CProMG 28 is proposed to use the conditional multi-constraint autoregressive framework to generate molecules owning desired property constraints in a controllable manner. However, the ability of CProMG largely depends on the quality of data used to train the model and it does not involve an optimization process for a comprehensive searching in the chemical space. Compared with CProMG, ParetoDrug does not use a multi-constraint generative model. Instead, ParetoDrug employs the Pareto MCTS to optimize multiple objectives synchronously by searching desired molecules with the guidance of the pretrained autoregressive molecule generative model. Also as shown in the benchmark experiment, ParetoDrug achieves better multi-objective metrics of the generated molecules when compared with CProMG on all the property objectives except QED.
In conclusion, in this work, we propose ParetoDrug to fulfill the gap of multi-objective target-aware drug discovery in the field of deep learning-based drug discovery. ParetoDrug is an autoregressive Pareto MCTS algorithm that integrates the pretrained autoregressive generative model to search desired multi-objective molecules in an atom-by-atom way with the help of Pareto MCTS. We perform the evaluation of ParetoDrug on a standard benchmark setting with various baselines. The benchmark results show that ParetoDrug achieves multiple satisfactory molecule properties including binding affinity while previous single-objective methods cannot. We further conduct the case studies of the multi-objective target-aware drug discovery tasks for two known drugs, the multi-target drug discovery task for HIV-related disease targets, and the multi-target multi-objective drug discovery task for a dual inhibitor. In these case studies, new molecules discovered by ParetoDrug exhibit high potentials that Pareto Dominate the known drugs of the disease targets on all required property objectives. In conclusion, ParetoDrug demonstrates its ability to handle the challenging multi-objective target-aware drug discovery tasks and its superiority in searching in the large and complex chemical space for novel compounds that possess multiple promising properties including binding affinity.
For future work, on the one hand, making ParetoDrug compatible with more recent advanced autoregressive molecule generative models such as the Diffusion model is highly promising. On the other hand, extending ParetoDrug into the multi-objective design of protein, polypeptide, and nucleic acid drugs also holds significant potential.
In this section, we first formulate the target-aware drug discovery task as a Markov decision process. Then we introduce the concepts of Pareto Dominate and Pareto Front in the multi-objective optimization domain. Finally, we propose the framework of ParetoDrug designed for the multi-objective target-aware drug discovery task and the multi-target target-aware drug discovery task.
Problem definition
Target-aware molecule generation can be formulated as a Markov decision process (MDP) 57 given that the next atom to be chosen only depends on the generated molecule fragment and the protein target. The MDP can be defined as M = ( S , A , P , R ) where S denotes the set of states that describe the current molecule fragment and the protein, A denotes the set of actions that indicate the chosen atom symbol to be added to the current molecule fragment, and P : S × A → S is the state transition function where the molecule fragment incorporates the chosen atom symbol to grow up to a new molecule fragment. \(R:S\to {{\mathbb{R}}}^{d}\) is the reward function based on the current state. In target-aware molecule generation, the reward to evaluate the generated molecule is usually available at the terminal state, which is a typical sparse-reward setting. If d > 1, multiple reward objectives are considered such as strong binding affinity, high drug-likeness, and low toxicity in drug discovery. The goal is to take the action that maximizes the expected episodic reward \(\overline{R}(s,a)\) , which can be approximated under repeated rollouts 58 as
where N ( s ) denotes the rollout times starting from state s and N ( s , a ) is the times that action a has been taken from state s . \({{\mathbb{I}}}_{j}(s,a)\) is an indicator function with value 1 if action a is selected from state s at the j th rollout round, 0 otherwise. r j ( s ) is the final reward to evaluate the final generated molecule at the terminal state for the j th rollout round starting from state s . A larger \(\overline{R}(s,a)\) value indicates a higher expected reward by taking action a from state s .
Multi-objective optimization
Multi-objective optimization (also known as Pareto optimization) is concerned with optimization problems involving more than one objective function to be optimized simultaneously 59 , which has been applied in many fields. In multi-objective optimization, there does not typically exist a feasible solution that maximizes all objective functions at the same time. Therefore, attention is paid to Pareto optimal solutions 60 , which cannot be improved in any of the objectives without degrading at least one of the other objectives. In mathematical terms, a feasible vector \({{{{\bf{X}}}}}\in {{\mathbb{R}}}^{d}\) is said to Pareto Dominate another vector \({{{{{\bf{X}}}}}}^{{\prime} }\in {{\mathbb{R}}}^{d}\) is defined as below 61 .
Definition 1
Pareto Dominate. Given two vectors X = ( x 1 , …, x d ) and \({{{{{\bf{X}}}}}}^{{\prime} }=({x}_{1}^{{\prime} },\ldots ,{x}_{d}^{{\prime} })\) , X is said to dominate \({{{{{\bf{X}}}}}}^{{\prime} }\) , i.e., \({{{{\bf{X}}}}}\succcurlyeq {{{{{\bf{X}}}}}}^{{\prime} }\) if and only if \({x}_{i}\ge {x}_{i}^{{\prime} },\forall i=1,\ldots ,d\) . X is said to strictly dominate \({{{{{\bf{X}}}}}}^{{\prime} }\) , i.e., \({{{{\bf{X}}}}}\succ {{{{{\bf{X}}}}}}^{{\prime} }\) if and only if \({{{{\bf{X}}}}}\succcurlyeq {{{{{\bf{X}}}}}}^{{\prime} }\) and ∃ i such that \({x}_{i} > {x}_{i}^{{\prime} }\) .
A vector \({{{{{\bf{X}}}}}}^{* }\in {{\mathbb{R}}}^{d}\) is called Pareto optimal if there does not exist another vector that Pareto Dominates it. The set of Pareto optimal vectors \({{\mathbb{X}}}^{* }\) , called Pareto Front, is defined as below.
Definition 2
Pareto Front. Given a set of vectors \({\mathbb{X}}\subset {{\mathbb{R}}}^{d}\) , the non-dominant set \({{\mathbb{X}}}^{* }\in {\mathbb{X}}\) is defined as \({{\mathbb{X}}}^{* }=\{{{{{\bf{X}}}}}\in {\mathbb{X}}:\nexists {{{{{\bf{X}}}}}}^{{\prime} }\in {\mathbb{X}}\,s.t.\,{{{{{\bf{X}}}}}}^{{\prime} }\succ {{{{\bf{X}}}}}\}\) .
In drug molecule design, the optimization or constraint of multiple properties is a pervasive requirement. For instance, for a new drug to be successful, it must simultaneously be potent, bioavailable, safe, and synthesizable, with these properties being often competing 62 . As Pareto optimization is capable of discovering a set of solutions that reveal trade-offs among objectives and relies on no prior measure of the importance of competing objectives, it is believed as the most robust approach to multi-objective drug discovery 62 . Next, we introduce how to utilize the concepts of Pareto Dominate and Pareto Front to construct the ParetoDrug framework for multi-objective target-aware drug discovery with the help of MCTS and the pretrained autoregressive generative model.
To solve the challenging multi-objective target-aware drug discovery task, which requires generating molecules with multiple desired properties including the strong binding affinity to specified protein targets, we propose an autoregressive Pareto MCTS generation algorithm called ParetoDrug. First, ParetoDrug employs existing pretrained autoregressive generative models to provide exploration guidance toward desired molecules during searching. Based on both the protein context and intermediate molecule fragment, the pretrained autoregressive model predicts the probability of the next atom symbol to be added to the current molecule fragment. Second, with exploration guidance from the pretrained autoregressive model, ParetoDrug performs Pareto MCTS to progressively find Pareto optimal molecules with multiple desired properties. Third, to achieve the exploration-exploitation balance during searching, we propose the ParetoPUCT selection criterion to determine the next atom symbol in the Selection step of Pareto MCTS. Through these three key components, ParetoDrug is able to generate high-quality molecules for multi-objective target-aware drug discovery tasks. The overall framework of ParetoDrug is shown in Fig. 6 . Next, we explain the details including Pareto MCTS, ParetoPUCT, and how to extend ParetoDrug into the case of multi-target target-aware molecule generation.
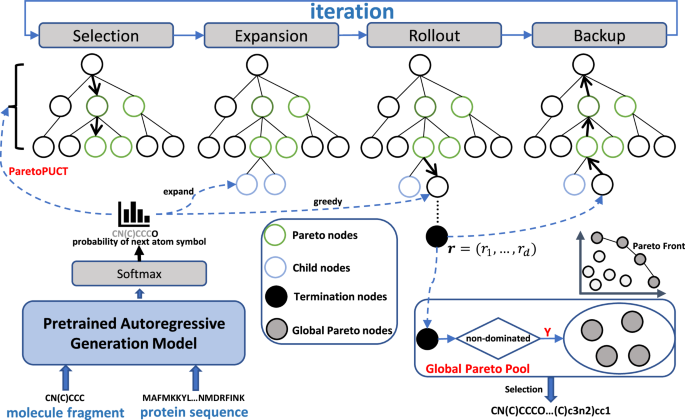
The pretrained autoregressive generative model gives the probability distribution of the next atom symbol. The ParetoPUCT balances the exploration and exploitation when searching for the next atom symbol in the Selection step. ParetoDrug maintains a global pool of Pareto optimal molecules, which are updated during Pareto MCTS.
Pareto MCTS
In MCTS, pretrained neural networks based on the expert data could be used for the guidance of action selection 63 and this idea has been extended into single-objective target-aware drug discovery 15 . Similarly, to enable the exploration guidance to desired molecules with strong binding affinity to specified protein targets, ParetoDrug employs an existing pretrained autoregressive generative model 15 to predict the next atom symbol given the protein target and current molecule fragment. The protein target is represented by the amino acid sequence. The molecule fragment is based on SMILES 64 , which describes molecules with short ASCII strings. The autoregressive generative model includes a protein encoder based on the protein’s amino acid sequence and a ligand molecule decoder. At each step, the protein encoder receives the target protein sequence and outputs the protein embedding into the ligand decoder. Next, the ligand decoder predicts the probability of the next atom symbol based on both the protein embedding and intermediate molecule fragment from the last step. This autoregressive generative model is pretrained on the protein-ligand data set and used in MCTS.
When generating molecules with the pretrained autoregressive generative model, although we could obtain the ligand molecule in a greedy manner by taking the next atom symbol with the maximum probability, it is prone to be stuck in a local optimum due to the unpredictable complexity of the chemical space. At the same time, the predicted atom with the maximum probability does not mean that it must be in the optimal molecule that satisfies multiple required properties as the pretrained model is not optimized for these properties. To address the above difficulties for the multi-objective target-aware drug discovery, we propose ParetoDrug, which employs the Pareto MCTS to enable a synchronous optimization of multiple properties together with the help of a pretrained autoregressive model for selection guidance. Next, we introduce Pareto MCTS.
Pareto MCTS 65 extends basic MCTS 66 to optimize multiple objectives, which adopts a tree structure to perform simulation iterations and estimates action values to guide searching. The Pareto MCTS procedure consists of four steps per iteration:
Selection . Each iteration starts from the current root node a τ and the best child is recursively selected until a leaf node a τ + l after l selections, i.e., a node that has not been expanded or terminated, is reached. For each selection t ∈ [1, l ], we need a selection criterion to determine which child node is the best to be chosen. This criterion balances between exploitation and exploration to avoid being trapped in local optimums and is given in Eq. ( 6 ).
Expansion . Given a selected leaf node a τ + l , the probability P ( a ∣ C τ + l ) for each expandable atom symbol a ∈ A is computed by the pretrained autoregressive generative model. C τ + l = { S p , m τ + l } is the state context with the target protein sequence S p and the current simulated intermediate molecule fragment m τ + l = a 1 ⋯ a τ a τ +1 ⋯ a τ + l . Here A is the legal action space, i.e. the SMILES vocabulary of molecules, under the given state context. The expanded child nodes of a τ + l are added to the tree and initialized immediately.
Rollout . The value of the reached leaf node a τ + l is evaluated by a fast rollout. From the leaf node, MCTS recursively generates the next state until termination and receives the reward of the final molecule at the termination state. During the rollout, each atom symbol is selected in a greedy manner according to the predicted probability given by the pretrained autoregressive network until a terminal symbol a τ + L is generated or the tree reaches a maximum depth. The path from the initial atom symbol to the terminal atom symbol forms a complete molecule m = a 1 ⋯ a τ a τ +1 ⋯ a τ + L . The reward r of the final molecule m is then evaluated based on the molecule property metrics. Specifically, the binding affinity is computed by the docking function f ( S p , m ) such as smina 32 . The reward r is calculated as defined in Eq. ( 3 ) by normalizing the property metric in each dimension.
Backup . The reward is backpropagated along the visited nodes to update their statistics until the root node. The detailed updating process for tree nodes is elaborated in Eq. ( 4 ) with the defined reward vector r for nodes.
When performing Pareto MCTS, we maintain a global pool of all the Pareto optimal molecules found so far to represent the molecule Pareto Front as defined by Definition 2. We update the global pool of Pareto optimal molecules by adding newly generated Pareto optimal molecules and removing invalid ones if they are Pareto Dominated by the global pool’s new coming molecules. The molecule comparison is based on the reward vector r defined as follows. For each generated molecule in the rollout with the property metric vector \({{{{\bf{h}}}}}=({h}_{1},\ldots ,{h}_{d})\in {{\mathbb{R}}}^{d}\) , the reward vector \({{{{\bf{r}}}}}=({r}_{1},\ldots ,{r}_{d})\in {{\mathbb{R}}}^{d}\) of this molecule is defined as
where N P is the number of Pareto optimal molecules in the global pool, \({h}_{i}^{k}\) is the i th property metric of the k th Pareto optimal molecule, and h i is the i th property metric of the current generated molecule to be compared. \({\mathbb{I}}\) is the indicator function with value 1 if the condition \({h}_{i} > {h}_{i}^{k}\) is satisfied, 0 otherwise. The calculation of reward r treats each dimension separately, regardless of their scale difference, which gains an advantage over methods that aggregate all dimensions into one score using predefined weights 24 . With the reward vector, the Backup step is performed as
where N a is the total times that node a has been selected and W a is the cumulative reward vector of node a . For each selection t ∈ [1, l ], the statistics of node a τ + t are updated by adding the reward vector of the node a τ + l ’s rollout result to W a and increasing the visiting times N a by 1.
The most important step of MCTS is the Selection step where a criterion is needed to select the next child node by comparing all child nodes. The most commonly used criterion is the upper confidence bound 67 in which a child node is selected to maximize
where N is the total times of iterations and N a is the times of node a being selected. U is a scalar used to select the best child node with the largest value. However, in the multi-objective target-aware drug discovery, the reward becomes a vector and U is not applicable for the comparison of vectors. At the same time, we also want to utilize the pretrained autoregressive generative model to provide the exploration guidance in the chemical space 15 , 68 when selecting the next child node in the Selection step.
Therefore, here we propose ParetoPUCT that extends the scalar predictor upper confidence bound applied to trees (PUCT) selection criterion 69 with the concepts of Pareto Dominate and Pareto Front into a vectorial selection criterion for the multi-objective MCTS 65 . At each selection t , we first compute a selection score vector for each candidate child node as
where c is a constant that controls the degree of exploration. Here W a is the cumulative reward vector for node a . The \(\frac{\sqrt{N}}{1+{N}_{a}}\) part guides MCTS to initially prefer to visit the nodes with a low number of visits. At the same time, the P ( a ∣ C τ + t −1 ) part tends to visit the atom nodes that are probably to produce a molecule with strong binding affinity to the protein target indicated by the pretrained autoregressive generative model. Furthermore, the \(\frac{{{{{{\bf{W}}}}}}_{a}}{{N}_{a}}\) makes ParetoDrug exploit the nodes with multiple high property metrics while c balances the exploitation and exploration. As the U p score is in the vector from for each child node a , to determine which child node to be selected, ParetoPUCT constructs a Pareto Front for those child nodes that are not Pareto Dominated by other child nodes by comparing their U p score vectors. Each child node in the resulting Pareto Front cannot be replaced by a better child node and thus becomes the candidate node for the selection. Finally, ParetoPUCT selects a node from the Pareto Front of the candidate child nodes uniformly at random.
Modifications of ParetoDrug for multi-target target-aware molecule generation
As the multi-target target-aware molecule generation involves multiple protein targets, we modify the ParetoPUCT node selection criterion to handle multiple predictions from the pretrained autoregressive generative model for different protein targets. Therefore, we propose the Multi-Target ParetoPUCT (M-ParetoPUCT) defined as
where there are m predictions for the next node a and c is a constant to control the exploration degree. For the prediction fusing function f ( P 1 ( a ∣ C 1, τ + t −1 ), …, P m ( a ∣ C m , τ + t −1 )), each prediction is a distribution of the next atom symbol with the inputting of the molecule’s SMILES string representation 64 and specified target protein’s amino acid sequence. Here P i ( a ∣ C i , τ + t −1 ) is the neural network prediction for the i th target pretrained on the protein-ligand data set. As the distributions are on the same action set, we use the mean-pooling operation for f as
This mean-pooling operation keeps the probabilities of the possible next atom symbols for each protein target. Meanwhile, it enhances the probabilities of the next atom symbol if it is predicted to be preferred by all the given protein targets.
After a leaf node a τ + l is selected, we need to expand it. The probability for each expandable atom symbol is computed the same as Eq. ( 8 ) from multiple predictions of the pretrained autoregressive model on multiple protein targets. Each child node a of a τ + l is initialized to \(\{{N}_{a}=0,{{{{{\bf{W}}}}}}_{a}={{{{\bf{0}}}}},\frac{\mathop{\sum }_{i = 1}^{m}{P}_{i}(a| {C}_{i,\tau +t-1})}{m}\}\) .
Statistics and reproducibility
Data manipulation and processing analyses were conducted using the packages Python (version 3.7), Biopython (version 1.79), Pandas (version 1.3.4), MMseqs2 (version 13.45111), RDKit (version 2020.09.5), PyTorch (version 1.13.1), and Openbabel (version 3.1.1). We used PyMOL (version 2.6.0a0) to analyze the protein structures. We used AMBER22 package to calculate the MM-GBSA scores (Supplementary Information G). We used PLIP 2021 to analyze protein-ligand Interactions Supplementary Information F. We use smina (version 2020.12.10) to calculate the docking score. The molecule property distributions are drawn by Matplotlib (version 3.4.3) and Seaborn (version 0.12.2), where the function “kdeplot” is called for kernel density estimate. The T test and p value calculation in this article are conducted with SciPy (version 1.9.1).
Data availability
The training and testing data of the autoregressive generative model used in ParetoDrug is processed from BindingDB and PDBbind , and is the same as in AlphaDrug and could be obtained from https://github.com/CMACH508/AlphaDrug . For the multi-objective target-aware drug discovery and multi-target drug discovery case studies, the PDB and ligand files of 7D42, 5G2N, 3A2O, 4G1Q, 1XKK, and 3BBT are downloaded from RCSB Protein Data Bank .
Code availability
The source code of this study is publicly available from the GitHub repository: https://github.com/CNDOTA/ParetoDrug . We also provide the Google Colab version of ParetoDrug , which could be directly run online. The numerical source data for graphs and charts in this article is provided in Figshare with DOI 70 . We also deposit the data and codes of ParetoDrug in Figshare with DOI 71 .
Anderson, A. C. The process of structure-based drug design. Chem. Biol. 10 , 787–797 (2003).
Article CAS PubMed Google Scholar
Shoichet, B. K. Virtual screening of chemical libraries. Nature 432 , 862–865 (2004).
Article CAS PubMed PubMed Central Google Scholar
Gorgulla, C. et al. An open-source drug discovery platform enables ultra-large virtual screens. Nature 580 , 663–668 (2020).
Blundell, T. L. Structure-based drug design. Nature 384 , 23–26 (1996).
CAS PubMed Google Scholar
Macarron, R. et al. Impact of high-throughput screening in biomedical research. Nat. Rev. Drug Discov. 10 , 188–195 (2011).
Cheng, Y., Gong, Y., Liu, Y., Song, B. & Zou, Q. Molecular design in drug discovery: a comprehensive review of deep generative models. Brief. Bioinforma. 22 , bbab344 (2021).
Article Google Scholar
Skalic, M., Varela-Rial, A., Jiménez, J., Martínez-Rosell, G. & De Fabritiis, G. LigVoxel: inpainting binding pockets using 3D-convolutional neural networks. Bioinformatics 35 , 243–250 (2019).
Aumentado-Armstrong, T. Latent molecular optimization for targeted therapeutic design ArXiv:1809.02032 [cs, q-bio] (2018).
Long, S., Zhou, Y., Dai, X. & Zhou, H. Zero-Shot 3D Drug design by sketching and generating. In Oh, A. H., Agarwal, A., Belgrave, D. & Cho, K. (eds.) Advances in Neural Information Processing Systems (2022).
Skalic, M., Sabbadin, D., Sattarov, B., Sciabola, S. & De Fabritiis, G. From target to drug: Generative modeling for the multimodal structure-based ligand design. Mol. Pharmaceutics 16 , 4282–4291 (2019).
Article CAS Google Scholar
Schneuing, A. et al. Structure-based drug design with equivariant diffusion models ArXiv:2210.13695 [cs, q-bio] (2022).
Guan, J. et al. 3D Equivariant diffusion for target-aware molecule generation and affinity prediction. In The Eleventh International Conference on Learning Representations (2023).
Ragoza, M., Masuda, T. & Koes, D. R. Generating 3D molecules conditional on receptor binding sites with deep generative models. Chem. Sci. 13 , 2701–2713 (2022).
Grechishnikova, D. Transformer neural network for protein-specific de novo drug generation as a machine translation problem. Sci. Rep. 11 , 321 (2021).
Qian, H., Lin, C., Zhao, D., Tu, S. & Xu, L. AlphaDrug: Protein target specific de novo molecular generation. PNAS Nexus 1 , pgac227 (2022).
Article PubMed PubMed Central Google Scholar
Xu, M., Ran, T. & Chen, H. De novo molecule design through the molecular generative model conditioned by 3D information of protein binding sites. J. Chem. Inf. Model. 61 , 3240–3254 (2021).
Zhang, J. & Chen, H. De novo molecule design using molecular generative models constrained by ligand-protein interactions. J. Chem. Inf. Model. 62 , 3291–3306 (2022).
Liu, M., Luo, Y., Uchino, K., Maruhashi, K. & Ji, S. Generating 3D molecules for target protein binding. In Chaudhuri, K. et al. (eds.) Proceedings of the 39th International Conference on Machine Learning , 162 , 13912–13924 (2022).
Luo, S., Guan, J., Ma, J. & Peng, J. A 3D generative model for structure-based drug design. In Ranzato, M., Beygelzimer, A., Dauphin, Y., Liang, P. S. & Vaughan, J. W. (eds.) Advances in Neural Information Processing Systems , 34 , 6229–6239 (2021).
Peng, X. et al. Pocket2Mol: Efficient molecular sampling based on 3D protein pockets. In Proceedings of the 39th International Conference on Machine Learning , 162 , 17644–17655 (2022).
Wang, J. et al. Multi-constraint molecular generation based on conditional transformer, knowledge distillation and reinforcement learning. Nat. Mach. Intell. 3 , 914–922 (2021).
Bagal, V., Aggarwal, R., Vinod, P. K. & Priyakumar, U. D. MolGPT: Molecular generation using a transformer-decoder model. J. Chem. Inf. Model. 62 , 2064–2076 (2022).
Jin, W., Barzilay, D. & Jaakkola, T. Multi-objective molecule generation using interpretable substructures. In Proceedings of the 37th International Conference on Machine Learning , 119 of Proceedings of Machine Learning Research , 4849–4859 (2020).
Sun, M. et al. MolSearch: Search-based multi-objective molecular generation and property optimization. In Proceedings of the 28th ACM SIGKDD Conference on Knowledge Discovery and Data Mining , 4724–4732 (2022).
Lamanna, G. et al. GENERA: A combined genetic/deep-learning algorithm for multiobjective target-oriented de novo design. J. Chem. Inf. Model. 63 , 5107–5119 (2023).
Sutton, R. S. & Barto, A. G. Reinforcement learning: an introduction . Adaptive computation and machine learning (MIT Press, Cambridge, Mass, 1998).
Mitchell, M. An introduction to genetic algorithms . Complex adaptive systems (MIT Press, Cambridge, Mass, 1996).
Li, J.-N., Yang, G., Zhao, P.-C., Wei, X.-X. & Shi, J.-Y. CProMG: Controllable protein-oriented molecule generation with desired binding affinity and drug-like properties. Bioinformatics 39 , i326–i336 (2023).
Brown, T. et al. Language Models are Few-Shot Learners. In Larochelle, H., Ranzato, M., Hadsell, R., Balcan, M. F. & Lin, H. (eds.) Advances in Neural Information Processing Systems , 33 , 1877–1901 (2020).
Ouyang, L. et al. Training language models to follow instructions with human feedback. In Koyejo, S. et al. (eds.) Advances in Neural Information Processing Systems , 35 , 27730–27744 (2022).
Liu, T., Lin, Y., Wen, X., Jorissen, R. N. & Gilson, M. K. BindingDB: A web-accessible database of experimentally determined protein-ligand binding affinities. Nucleic Acids Res. 35 , D198–D201 (2007).
Koes, D. R., Baumgartner, M. P. & Camacho, C. J. Lessons learned in empirical scoring with smina from the CSAR 2011 benchmarking exercise. J. Chem. Inf. Model. 53 , 1893–1904 (2013).
Ghose, A. K., Viswanadhan, V. N. & Wendoloski, J. J. A knowledge-based approach in designing combinatorial or medicinal chemistry libraries for drug discovery. 1. A Qualitative and quantitative characterization of known drug databases. J. Comb. Chem. 1 , 55–68 (1999).
Bickerton, G. R., Paolini, G. V., Besnard, J., Muresan, S. & Hopkins, A. L. Quantifying the chemical beauty of drugs. Nat. Chem. 4 , 90–98 (2012).
Ertl, P. & Schuffenhauer, A. Estimation of synthetic accessibility score of drug-like molecules based on molecular complexity and fragment contributions. J. Cheminf. 1 , 8 (2009).
Ertl, P., Roggo, S. & Schuffenhauer, A. Natural product-likeness score and its application for prioritization of compound libraries. J. Chem. Inf. Model. 48 , 68–74 (2008).
Ma, B. et al. Structure-based de novo molecular generator combined with artificial intelligence and docking simulations. J. Chem. Inf. Model. 61 , 3304–3313 (2021).
Yang, X., Zhang, J., Yoshizoe, K., Terayama, K. & Tsuda, K. ChemTS: an efficient python library for de novo molecular generation. Sci. Technol. Adv. Mater. 18 , 972–976 (2017).
Loeffler, H. H. et al. Reinvent 4: Modern AI-driven generative molecule design. J. Cheminf. 16 , 20 (2024).
Chen, Y.-C. A tutorial on kernel density estimation and recent advances. Biostat. Epidemiol. 1 , 161–187 (2017).
Rastelli, G., Rio, A. D., Degliesposti, G. & Sgobba, M. Fast and accurate predictions of binding free energies using MM-PBSA and MM-GBSA. J. Comput. Chem. 31 , 797–810 (2010).
Wang, E. et al. End-point binding free energy calculation with MM/PBSA and MM/GBSA: Strategies and applications in drug design. Chem. Rev. 119 , 9478–9508 (2019).
Salentin, S., Schreiber, S., Haupt, V. J., Adasme, M. F. & Schroeder, M. PLIP: fully automated protein-ligand interaction profiler. Nucleic Acids Res. 43 , W443–W447 (2015).
Angulo, P. Nonalcoholic fatty liver disease. N. Engl. J. Med. 346 , 1221–1231 (2002).
Adams, L. A. et al. The natural history of nonalcoholic fatty liver disease: A population-based cohort study. Gastroenterology 129 , 113–121 (2005).
Article PubMed Google Scholar
Bellentani, S., Bedogni, G., Miglioli, L. & Tiribelli, C. The epidemiology of fatty liver. Eur. J. Gastroenterol. Hepatol. 16 , 1087–1093 (2004).
Browning, J. D. et al. Prevalence of hepatic steatosis in an urban population in the United States: Impact of ethnicity. Hepatology 40 , 1387–1395 (2004).
Jiang, L. et al. Structural basis of tropifexor as a potent and selective agonist of farnesoid X receptor. Biochem. Biophys. Res. Commun. 534 , 1047–1052 (2021).
Carbone, A. et al. Follicular lymphoma. Nat. Rev. Dis. Prim. 5 , 83 (2019).
Scott, W. J. et al. Discovery and SAR of Novel 2,3-Dihydroimidazo[1,2- c]quinazoline PI3K Inhibitors: Identification of Copanlisib (BAY 80-6946). ChemMedChem 11 , 1517–1530 (2016).
Yuan, Y., Pei, J. & Lai, L. LigBuilder V3: A multi-target de novo drug design approach. Front. Chem. 8 , 142 (2020).
Higa, G. M. & Abraham, J. Lapatinib in the treatment of breast cancer. Expert Rev. Anticancer Ther. 7 , 1183–1192 (2007).
El-Gamal, M. I. et al. A review of HER4 (ErbB4) kinase, its impact on cancer, and its inhibitors. Molecules 26 , 7376 (2021).
Wood, E. R. et al. A unique structure for epidermal growth factor receptor bound to GW572016 (Lapatinib). Cancer Res. 64 , 6652–6659 (2004).
Qiu, C. et al. Mechanism of activation and inhibition of the HER4/ErbB4 kinase. Structure 16 , 460–467 (2008).
Li, Y., Zhang, L. & Liu, Z. Multi-objective de novo drug design with conditional graph generative model. J. Cheminf. 10 , 33 (2018).
Bellman, R. A Markovian decision process. J. Math. Mech. 6 , 679–684 (1957).
Google Scholar
Gelly, S. & Silver, D. Monte-Carlo tree search and rapid action value estimation in computer Go. Artif. Intell. 175 , 1856–1875 (2011).
Miettinen, K. Nonlinear multiobjective optimization. No. 12 in International series in operations research & management science (1999).
Luc, D. T. Pareto Optimality. In Pardalos, P. M., Chinchuluun, A., Pardalos, P. M., Migdalas, A. & Pitsoulis, L. (eds.) Pareto Optimality, Game Theory And Equilibria , 17 , 481–515 (2008).
Wang, W. & Sebag, M. Multi-objective Monte-Carlo Tree Search. In Proceedings of the Asian Conference on Machine Learning , 25 , 507–522 (Singapore Management University, Singapore, 2012).
Fromer, J. C. & Coley, C. W. Computer-aided multi-objective optimization in small molecule discovery. Patterns 4 , 100678 (2023).
Silver, D. et al. Mastering the game of Go without human knowledge. Nature 550 , 354–359 (2017).
Weininger, D. SMILES, a chemical language and information system. 1. Introduction to methodology and encoding rules. J. Chem. Inf. Comput. Sci. 28 , 31–36 (1988).
Chen, W. & Liu, L. Pareto Monte Carlo Tree Search for Multi-Objective Informative Planning. In Proceedings of Robotics: Science and Systems (FreiburgimBreisgau, Germany, 2019).
Browne, C. B. et al. A Survey of Monte Carlo tree search methods. IEEE Trans. Comput. Intell. AI Games 4 , 1–43 (2012).
Auer, P. Using confidence bounds for exploitation-exploration trade-offs. J. Mach. Learn. Res. 3 , 397–422 (2003).
Schrittwieser, J. et al. Mastering Atari, Go, chess and shogi by planning with a learned model. Nature 588 , 604–609 (2020).
Rosin, C. D. Multi-armed bandits with episode context. Ann. Math. Artif. Intell. 61 , 203–230 (2011).
Yang, Y. ParetoDrug numerical source data https://figshare.com/articles/dataset/ParetoDrug_numerical_source_data/26304124 (2024).
Yang, Y. ParetoDrug codes and data https://figshare.com/articles/dataset/ParetoDrug_codes_and_data_zip/26309932 (2024).
Prasanna, S. & Doerksen, R. Topological polar surface area: A useful descriptor in 2D-QSAR. Curr. Med. Chem. 16 , 21–41 (2009).
Olivecrona, M., Blaschke, T., Engkvist, O. & Chen, H. Molecular de-novo design through deep reinforcement learning. J. Cheminf. 9 , 48 (2017).
Korb, O., Stützle, T. & Exner, T. E. PLANTS: Application of ant colony optimization to structure-based drug design. In Ant Colony Optimization and Swarm Intelligence , 4150 , 247–258 (Springer Berlin Heidelberg, Berlin, Heidelberg, 2006).
Friesner, R. A. et al. Glide: A new approach for rapid, accurate docking and scoring. 1. Method and assessment of docking accuracy. J. Med. Chem. 47 , 1739–1749 (2004).
Trott, O. & Olson, A. J. AutoDock Vina: Improving the speed and accuracy of docking with a new scoring function, efficient optimization, and multithreading. J. Comput. Chem. 31 , 455–461 (2010).
Download references
Acknowledgements
The work described in this article was supported by a grant from the Hong Kong Innovation and Technology Fund (Project No. ITS/241/21) and a grant from the National Natural Science Foundation of China (22377111).
Author information
Authors and affiliations.
Department of Computer Science and Engineering, The Chinese University of Hong Kong, Hong Kong, China
Yaodong Yang, Jinpeng Li & Pheng-Ann Heng
Zhejiang Lab, Hangzhou, China
Guangyong Chen, Junyou Li, Lanqing Li & Ercheng Wang
Zhejiang University, Hangzhou, China
Odin Zhang & Xujun Zhang
Noah’s Ark Lab, Huawei, Shenzhen, China
You can also search for this author in PubMed Google Scholar
Contributions
Pheng-Ann Heng and Guangyong Chen conceived the research topic. Yaodong Yang designed and developed the method and carried out the computational benchmark experiments. Odin Zhang and Ercheng Wang provided the necessary domain background and helped with experiments. Yaodong Yang, Guangyong Chen, and Ercheng Wang designed and performed the case studies. Odin Zhang, Junyou Li, and Xujun Zhang contributed to the computational analysis. Yaodong Yang, Ercheng Wang, Jinpeng Li, Guangyong Chen, Lanqing Li, and Jianye Hao participated in the writing of the paper. Guangyong Chen, Ercheng Wang, Jianye Hao, and Pheng-Ann Heng supervised the work. All the co-authors participated in the discussions and agreed with the contents of this work.
Corresponding authors
Correspondence to Guangyong Chen , Jianye Hao or Ercheng Wang .
Ethics declarations
Competing interests.
The authors declare no competing interests.
Peer review
Peer review information.
Communications Biology thanks the anonymous reviewers for their contribution to the peer review of this work. Primary Handling Editor: Laura Rodríguez Pérez.
Additional information
Publisher’s note Springer Nature remains neutral with regard to jurisdictional claims in published maps and institutional affiliations.
Supplementary information
Supplementary information, rights and permissions.
Open Access This article is licensed under a Creative Commons Attribution 4.0 International License, which permits use, sharing, adaptation, distribution and reproduction in any medium or format, as long as you give appropriate credit to the original author(s) and the source, provide a link to the Creative Commons licence, and indicate if changes were made. The images or other third party material in this article are included in the article’s Creative Commons licence, unless indicated otherwise in a credit line to the material. If material is not included in the article’s Creative Commons licence and your intended use is not permitted by statutory regulation or exceeds the permitted use, you will need to obtain permission directly from the copyright holder. To view a copy of this licence, visit http://creativecommons.org/licenses/by/4.0/ .
Reprints and permissions
About this article
Cite this article.
Yang, Y., Chen, G., Li, J. et al. Enabling target-aware molecule generation to follow multi objectives with Pareto MCTS. Commun Biol 7 , 1074 (2024). https://doi.org/10.1038/s42003-024-06746-w
Download citation
Received : 03 April 2024
Accepted : 16 August 2024
Published : 02 September 2024
DOI : https://doi.org/10.1038/s42003-024-06746-w
Share this article
Anyone you share the following link with will be able to read this content:
Sorry, a shareable link is not currently available for this article.
Provided by the Springer Nature SharedIt content-sharing initiative
By submitting a comment you agree to abide by our Terms and Community Guidelines . If you find something abusive or that does not comply with our terms or guidelines please flag it as inappropriate.
Quick links
- Explore articles by subject
- Guide to authors
- Editorial policies
Sign up for the Nature Briefing: Translational Research newsletter — top stories in biotechnology, drug discovery and pharma.


IMAGES
VIDEO
COMMENTS
The NCCSTS Case Collection, created and curated by the National Center for Case Study Teaching in Science, on behalf of the University at Buffalo, contains over a thousand peer-reviewed case studies on a variety of topics in all areas of science. Cases (only) are freely accessible; subscription is required for access to teaching notes and ...
National Science Teaching Association 405 E Laburnum Avenue Ste 3 Richmond, VA 23222 (T) 703.524.3646 (F) 703.243.7177
Students in my anatomy class complete many case studies throughout the year focused on body system units. Case studies are a way to add a personal story to (sometimes) technical information about physiology. For my high school students, I try to find cases that are about younger people or even children, cases like " A Tiny Heart ," which ...
HHMI Biointeractive has many interactive resources, case studies, and data analysis. Here is a list of my favorites: Interactive Case Study For Studying Elephant Communication. Effects of Fungicides on Bumble Bee Colonies. Human Skin Color: Evidence for Selection. Lactase Persistence: Evidence for Selection. Exploring Trophic Cascades.
Cases (only) are freely accessible; subscription is required for access to teaching notes and answer keys. Suggested Keywords, to help with your search (besides selecting subjects): clicker cases, directed cases, interrupted cases, discussion cases, intimate debate cases. As a reminder, all cases may be adjusted to meet the needs of your ...
I always allow students to talk and help one another during clicker questions to enhance their interaction and give them a choice to go along with a group opinion or answer based on their individual thinking. 4. Flip the classroom. Another effective way to use BioInteractive resources in large classes is to use videos to flip a class session.
These four case studies are interactivities based on actual scientific research projects carried out by leading teams in the field. Each case study takes the participant through a series of steps in a research project — just follow the step-by-step instructions to test a hypothesis or analyze data. The case studies provide an interactive ...
Case Studies in Biology: Climate and Health Exploration Course. This is an online course offered in the summer and open to current high school students. As scientists, we take a lot of STEM classes, including biology, chemistry, physics, and math. But we often don't have time to connect all of this information together.
Case Study - Mitosis, Cancer, and the HPV Vaccine. Students in my anatomy class get a quick review of the cell and mitosis. This activity on HPV shows how the cell cycle relates to overall health. In fact, many of the chapters in anatomy have anchoring phenomena on diseases and health. For example, cystic fibrosis is a cellular transport ...
AP Biology Case Studies Primary Literature and Featured Scientists. Fall Term 2022 Investigation 1 - Circadian Rhythms in Hibernating Bats Hope, P. R. & Jones, G. (2013). An entrained circadian cycle of peak activity in a population of hibernating bats. Journal of Mammology, 94(2), 497-505. Essay Question 1:
The interrupted case study consists of four major parts: 1) an opening hook activity to capture students' attention and introduce the antibiotic crisis, 2) a jigsaw activity to research different classes of antibiotic targets and the resistance mechanisms that can arise, 3) a discussion of antibiotic resistance in real-time using the MEGA ...
case study based on the "medical. clues" provided in the narrative. Thus, each student had to evaluate, critique, and attempt to solve at least. The writer of this case study was a. 20-year-old Vietnamese-American stu. eighteen other case studies. Students dent who had been living in the United.
The case study, "Coat Proteins and Vesicle Transport" (Scales SJ, Pepperkok R, Kreis TE. Visualization of ER-to-Golgi transport in living cells reveals a sequential mode of action for COPII and COP I. Cell 1997; 90: 1137-1148), examines the role of COPI and COPII in protein transport from the RER to the Golgi complex.
Although case studies were considered a novel method of science education just 20 years ago, the case study teaching method has gained popularity in recent years among an array of scientific disciplines such as biology, chemistry, nursing, and psychology (5-7, 9, 11, 13, 15-17, 21, 22, 24).
This book provides case studies that can be used in Systems Biology related classes. Each case study has the same structure which answers the following questions: What is the biological problem and why is it interesting? What are the relevant details with regard to cell physiology and molecular mechanisms?
Human biology is the scientific study of the human species, which includes the fascinating story of human evolution and a detailed account of our genetics, anatomy, physiology, and ecology. In short, the study focuses on how we got here, how we function, and the role we play in the natural world. This helps us to better understand human health ...
Summary. There are 180 currently recognised species of RNA virus that can infect humans and, on average, 2 new species are added every year. RNA viruses are routinely exchanged between humans and other hosts (particularly other mammals and sometimes birds) over both epidemiological and evolutionary time: 89% of human-infective species are ...
Using case studies to support active, inquiry-based approaches can be especially effective [13, 14].Case study pedagogies are well suited to supporting the development of CT skills because of their sustained focus on a theme with applications in a specific setting and the opportunity to emphasize distinct steps in the processes of understanding and analyzing issues that comprise essential CT ...
Each of the following cases involves an important research tool in molecular biology, and each was chosen to illustrate a form of protection of intellectual property and a pattern of development involving both the public and the private sector. For each case, we present background material and a summary of the discussion that raised issues peculiar to the case.
This case study was revised in 2023, get the NEW VERSION! This case study focuses on a baby boy who was born with a problem with his heart. The story is based on a real scenario, though some of the names have been changed, and the parents gave permission to include photos of the infant. Students will read about symptoms that occur when a baby ...
Traditional risk assessment methodologies in toxicology have relied upon animal testing, despite concerns regarding interspecies consistency, reproducibility, costs, and ethics. New Approach Methodologies (NAMs), including cell culture and multi-level omics analyses, hold promise by providing mechanistic information rather than assessing organ pathology. However, NAMs face limitations, like ...
In this study, we used a case study approach to obtain an in-depth understanding of the change process of two university instructors (Julie and Alex) who were involved with redesigning a biology course. The instructors sought to transform the course from a teacher-centered, lecture-style class to one that incorporated learner-centered teaching.
In these case studies, ParetoDrug is able to generate the Pareto Dominate molecules over the known drug ligands in terms of the specified molecule property objectives, which exhibits the promising ...