Hypothesis Requirements
Hypotheses are a crucial part of the scientific thinking process, and most professional scientific endeavors are hypothesis-driven. That is, they seek to address a specific, measurable, and answerable question. A well-constructed hypothesis has several characteristics: it is clear, testable, falsifiable, and serves as the basis for constructing a clear set of experiments that will allow the student to discuss why it can be accepted or rejected based on the experiments. We believe that it is important for students who publish with JEI to practice rigorous scientific thinking through generating and testing hypotheses.
This means that manuscripts that merely introduce an invention, a computational method, a new machine/deep learning or AI algorithm, no matter how impressive they are, are not appropriate for JEI. Here are some common examples of unacceptable “hypotheses” relating to engineering projects:
- I hypothesize that my invention/method/machine learning model will work
- I hypothesize that I can build this invention/method/machine learning model
- I hypothesize that my machine/deep learning or AI model will be effective and yield accurate results
If your hypothesis boils down to one of the above hypotheses, your research is engineering-based. If your manuscript is related to engineering and/or computation algorithm development, please read our Guidelines for Engineering- and Machine Learning-Based Projects .
Additionally, review articles , where a review of the existing literature on a topic is presented, are not eligible for publication in JEI at this time .
This video goes over the general hypothesis requirements as they relate to research eligible for publication at JEI. It was created by one of our previous authors and current student advisory board members, Akshya Mahadevan!
When you assess whether your manuscript has a clear, well-constructed hypothesis, please ask whether it meets the following five criteria:

1. It IS NOT discovery or descriptive research
Some research is not hypothesis-driven. Terms used to describe non-hypothesis-driven research are ‘descriptive research,’ in which information is collected without a particular question in mind, and ‘discovery science,’ where large volumes of experimental data are analyzed with the goal of finding new patterns or correlations. These new observations can lead to hypothesis formation and other scientific methodologies. Some examples of discovery or descriptive research include an invention, explaining an engineered design like a program or an algorithm, mining large datasets for potential targets, or even characterizing a new species. However, if you have a pre-existing hypothesis and use large datasets to test it , this is acceptable for submission to JEI.
Another way to assess whether your research is hypothesis-driven is by analyzing the experimental setup. What variables in the experiment are independent, and which are dependent? Do the results of the dependent variable answer the scientific question? Are there positive and negative control groups?
2. It IS original
While your hypothesis does not have to be completely novel within the larger field of your research topic, it cannot be obvious to you, given the background information or experimental setup. You must have developed the hypothesis and designed experiments to test it yourself. This means that the experiments cannot be prescribed – an assigned project from an AP biology course, for example.
3. It IS NOT too general/global
Example 1: “Disease X results from the expression of virulence genes.” Instead the hypothesis should focus on the expression of a particular gene or a set of genes.
Example 2: “Quantifying X will provide significant increases in income for industry.” This is essentially untestable in an experimental setup and is really a potential outcome, not a hypothesis.
4. It IS NOT too complex
Hypothesis statements that contain words like “and” and “or” are ‘compound hypotheses’. This makes testing difficult, because while one part may be true the other may not be so. When your hypothesis has multiple parts, make sure that your experiments directly test the entire hypothesis. Possible further implications that you cannot test should be discussed in Discussion.
5. It DOES NOT misdirect to the researcher
The hypothesis should not address your capabilities. “Discovering the mechanism behind X will enable us to better detect the pathogen.” This example tests the ability of the researchers to take information and use it; this is a result of successful hypothesis-driven research, not a testable hypothesis. Instead, the hypothesis should focus on the experimental system. If it is difficult to state the hypothesis without misdirecting to the researcher, the focus of the research may be discovery science or invention-based, and should be edited to incorporate a properly formulated hypothesis.
Please contact the JEI Editorial Staff at [email protected] if you have any questions regarding the hypothesis of your research.
Loading metrics
Open Access
Perspective
Perspective: Dimensions of the scientific method
* E-mail: [email protected]
Affiliation Department of Biomedical Engineering, Georgia Institute of Technology and Emory University, Atlanta, Georgia, United States of America

- Eberhard O. Voit
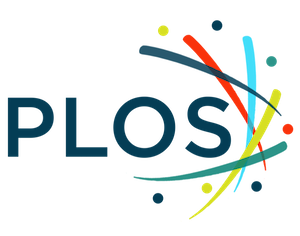
Published: September 12, 2019
- https://doi.org/10.1371/journal.pcbi.1007279
- Reader Comments
The scientific method has been guiding biological research for a long time. It not only prescribes the order and types of activities that give a scientific study validity and a stamp of approval but also has substantially shaped how we collectively think about the endeavor of investigating nature. The advent of high-throughput data generation, data mining, and advanced computational modeling has thrown the formerly undisputed, monolithic status of the scientific method into turmoil. On the one hand, the new approaches are clearly successful and expect the same acceptance as the traditional methods, but on the other hand, they replace much of the hypothesis-driven reasoning with inductive argumentation, which philosophers of science consider problematic. Intrigued by the enormous wealth of data and the power of machine learning, some scientists have even argued that significant correlations within datasets could make the entire quest for causation obsolete. Many of these issues have been passionately debated during the past two decades, often with scant agreement. It is proffered here that hypothesis-driven, data-mining–inspired, and “allochthonous” knowledge acquisition, based on mathematical and computational models, are vectors spanning a 3D space of an expanded scientific method. The combination of methods within this space will most certainly shape our thinking about nature, with implications for experimental design, peer review and funding, sharing of result, education, medical diagnostics, and even questions of litigation.
Citation: Voit EO (2019) Perspective: Dimensions of the scientific method. PLoS Comput Biol 15(9): e1007279. https://doi.org/10.1371/journal.pcbi.1007279
Editor: Jason A. Papin, University of Virginia, UNITED STATES
Copyright: © 2019 Eberhard O. Voit. This is an open access article distributed under the terms of the Creative Commons Attribution License , which permits unrestricted use, distribution, and reproduction in any medium, provided the original author and source are credited.
Funding: This work was supported in part by grants from the National Science Foundation ( https://www.nsf.gov/div/index.jsp?div=MCB ) grant NSF-MCB-1517588 (PI: EOV), NSF-MCB-1615373 (PI: Diana Downs) and the National Institute of Environmental Health Sciences ( https://www.niehs.nih.gov/ ) grant NIH-2P30ES019776-05 (PI: Carmen Marsit). The funders had no role in study design, data collection and analysis, decision to publish, or preparation of the manuscript.
Competing interests: The author has declared that no competing interests exist.
The traditional scientific method: Hypothesis-driven deduction
Research is the undisputed core activity defining science. Without research, the advancement of scientific knowledge would come to a screeching halt. While it is evident that researchers look for new information or insights, the term “research” is somewhat puzzling. Never mind the prefix “re,” which simply means “coming back and doing it again and again,” the word “search” seems to suggest that the research process is somewhat haphazard, that not much of a strategy is involved in the process. One might argue that research a few hundred years ago had the character of hoping for enough luck to find something new. The alchemists come to mind in their quest to turn mercury or lead into gold, or to discover an elixir for eternal youth, through methods we nowadays consider laughable.
Today’s sciences, in stark contrast, are clearly different. Yes, we still try to find something new—and may need a good dose of luck—but the process is anything but unstructured. In fact, it is prescribed in such rigor that it has been given the widely known moniker “scientific method.” This scientific method has deep roots going back to Aristotle and Herophilus (approximately 300 BC), Avicenna and Alhazen (approximately 1,000 AD), Grosseteste and Robert Bacon (approximately 1,250 AD), and many others, but solidified and crystallized into the gold standard of quality research during the 17th and 18th centuries [ 1 – 7 ]. In particular, Sir Francis Bacon (1561–1626) and René Descartes (1596–1650) are often considered the founders of the scientific method, because they insisted on careful, systematic observations of high quality, rather than metaphysical speculations that were en vogue among the scholars of the time [ 1 , 8 ]. In contrast to their peers, they strove for objectivity and insisted that observations, rather than an investigator’s preconceived ideas or superstitions, should be the basis for formulating a research idea [ 7 , 9 ].
Bacon and his 19th century follower John Stuart Mill explicitly proposed gaining knowledge through inductive reasoning: Based on carefully recorded observations, or from data obtained in a well-planned experiment, generalized assertions were to be made about similar yet (so far) unobserved phenomena [ 7 ]. Expressed differently, inductive reasoning attempts to derive general principles or laws directly from empirical evidence [ 10 ]. An example is the 19th century epigram of the physician Rudolf Virchow, Omnis cellula e cellula . There is no proof that indeed “every cell derives from a cell,” but like Virchow, we have made the observation time and again and never encountered anything suggesting otherwise.
In contrast to induction, the widely accepted, traditional scientific method is based on formulating and testing hypotheses. From the results of these tests, a deduction is made whether the hypothesis is presumably true or false. This type of hypotheticodeductive reasoning goes back to William Whewell, William Stanley Jevons, and Charles Peirce in the 19th century [ 1 ]. By the 20th century, the deductive, hypothesis-based scientific method had become deeply ingrained in the scientific psyche, and it is now taught as early as middle school in order to teach students valid means of discovery [ 8 , 11 , 12 ]. The scientific method has not only guided most research studies but also fundamentally influenced how we think about the process of scientific discovery.
Alas, because biology has almost no general laws, deduction in the strictest sense is difficult. It may therefore be preferable to use the term abduction, which refers to the logical inference toward the most plausible explanation, given a set of observations, although this explanation cannot be proven and is not necessarily true.
Over the decades, the hypothesis-based scientific method did experience variations here and there, but its conceptual scaffold remained essentially unchanged ( Fig 1 ). Its key is a process that begins with the formulation of a hypothesis that is to be rigorously tested, either in the wet lab or computationally; nonadherence to this principle is seen as lacking rigor and can lead to irreproducible results [ 1 , 13 – 15 ].
- PPT PowerPoint slide
- PNG larger image
- TIFF original image
The central concept of the traditional scientific method is a falsifiable hypothesis regarding some phenomenon of interest. This hypothesis is to be tested experimentally or computationally. The test results support or refute the hypothesis, triggering a new round of hypothesis formulation and testing.
https://doi.org/10.1371/journal.pcbi.1007279.g001
Going further, the prominent philosopher of science Sir Karl Popper argued that a scientific hypothesis can never be verified but that it can be disproved by a single counterexample. He therefore demanded that scientific hypotheses had to be falsifiable, because otherwise, testing would be moot [ 16 , 17 ] (see also [ 18 ]). As Gillies put it, “successful theories are those that survive elimination through falsification” [ 19 ]. Kelley and Scott agreed to some degree but warned that complete insistence on falsifiability is too restrictive as it would mark many computational techniques, statistical hypothesis testing, and even Darwin’s theory of evolution as nonscientific [ 20 ].
While the hypothesis-based scientific method has been very successful, its exclusive reliance on deductive reasoning is dangerous because according to the so-called Duhem–Quine thesis, hypothesis testing always involves an unknown number of explicit or implicit assumptions, some of which may steer the researcher away from hypotheses that seem implausible, although they are, in fact, true [ 21 ]. According to Kuhn, this bias can obstruct the recognition of paradigm shifts [ 22 ], which require the rethinking of previously accepted “truths” and the development of radically new ideas [ 23 , 24 ]. The testing of simultaneous alternative hypotheses [ 25 – 27 ] ameliorates this problem to some degree but not entirely.
The traditional scientific method is often presented in discrete steps, but it should really be seen as a form of critical thinking, subject to review and independent validation [ 8 ]. It has proven very influential, not only by prescribing valid experimentation, but also for affecting the way we attempt to understand nature [ 18 ], for teaching [ 8 , 12 ], reporting, publishing, and otherwise sharing information [ 28 ], for peer review and the awarding of funds by research-supporting agencies [ 29 , 30 ], for medical diagnostics [ 7 ], and even in litigation [ 31 ].
A second dimension of the scientific method: Data-mining–inspired induction
A major shift in biological experimentation occurred with the–omics revolution of the early 21st century. All of a sudden, it became feasible to perform high-throughput experiments that generated thousands of measurements, typically characterizing the expression or abundances of very many—if not all—genes, proteins, metabolites, or other biological quantities in a sample.
The strategy of measuring large numbers of items in a nontargeted fashion is fundamentally different from the traditional scientific method and constitutes a new, second dimension of the scientific method. Instead of hypothesizing and testing whether gene X is up-regulated under some altered condition, the leading question becomes which of the thousands of genes in a sample are up- or down-regulated. This shift in focus elevates the data to the supreme role of revealing novel insights by themselves ( Fig 2 ). As an important, generic advantage over the traditional strategy, this second dimension is free of a researcher’s preconceived notions regarding the molecular mechanisms governing the phenomenon of interest, which are otherwise the key to formulating a hypothesis. The prominent biologists Patrick Brown and David Botstein commented that “the patterns of expression will often suffice to begin de novo discovery of potential gene functions” [ 32 ].
Data-driven research begins with an untargeted exploration, in which the data speak for themselves. Machine learning extracts patterns from the data, which suggest hypotheses that are to be tested in the lab or computationally.
https://doi.org/10.1371/journal.pcbi.1007279.g002
This data-driven, discovery-generating approach is at once appealing and challenging. On the one hand, very many data are explored simultaneously and essentially without bias. On the other hand, the large datasets supporting this approach create a genuine challenge to understanding and interpreting the experimental results because the thousands of data points, often superimposed with a fair amount of noise, make it difficult to detect meaningful differences between sample and control. This situation can only be addressed with computational methods that first “clean” the data, for instance, through the statistically valid removal of outliers, and then use machine learning to identify statistically significant, distinguishing molecular profiles or signatures. In favorable cases, such signatures point to specific biological pathways, whereas other signatures defy direct explanation but may become the launch pad for follow-up investigations [ 33 ].
Today’s scientists are very familiar with this discovery-driven exploration of “what’s out there” and might consider it a quaint quirk of history that this strategy was at first widely chastised and ridiculed as a “fishing expedition” [ 30 , 34 ]. Strict traditionalists were outraged that rigor was leaving science with the new approach and that sufficient guidelines were unavailable to assure the validity and reproducibility of results [ 10 , 35 , 36 ].
From the view point of philosophy of science, this second dimension of the scientific method uses inductive reasoning and reflects Bacon’s idea that observations can and should dictate the research question to be investigated [ 1 , 7 ]. Allen [ 36 ] forcefully rejected this type of reasoning, stating “the thinking goes, we can now expect computer programs to derive significance, relevance and meaning from chunks of information, be they nucleotide sequences or gene expression profiles… In contrast with this view, many are convinced that no purely logical process can turn observation into understanding.” His conviction goes back to the 18th century philosopher David Hume and again to Popper, who identified as the overriding problem with inductive reasoning that it can never truly reveal causality, even if a phenomenon is observed time and again [ 16 , 17 , 37 , 38 ]. No number of observations, even if they always have the same result, can guard against an exception that would violate the generality of a law inferred from these observations [ 1 , 35 ]. Worse, Popper argued, through inference by induction, we cannot even know the probability of something being true [ 10 , 17 , 36 ].
Others argued that data-driven and hypothesis-driven research actually do not differ all that much in principle, as long as there is cycling between developing new ideas and testing them with care [ 27 ]. In fact, Kell and Oliver [ 34 ] maintained that the exclusive acceptance of hypothesis-driven programs misrepresents the complexities of biological knowledge generation. Similarly refuting the prominent rule of deduction, Platt [ 26 ] and Beard and Kushmerick [ 27 ] argued that repeated inductive reasoning, called strong inference, corresponds to a logically sound decision tree of disproving or refining hypotheses that can rapidly yield firm conclusions; nonetheless, Platt had to admit that inductive inference is not as certain as deduction, because it projects into the unknown. Lander compared the task of obtaining causality by induction to the problem of inferring the design of a microprocessor from input-output readings, which in a strict sense is impossible, because the microprocessor could be arbitrarily complicated; even so, inference often leads to novel insights and therefore is valuable [ 39 ].
An interesting special case of almost pure inductive reasoning is epidemiology, where hypothesis-driven reasoning is rare and instead, the fundamental question is whether data-based evidence is sufficient to associate health risks with specific causes [ 31 , 34 ].
Recent advances in machine learning and “big-data” mining have driven the use of inductive reasoning to unprecedented heights. As an example, machine learning can greatly assist in the discovery of patterns, for instance, in biological sequences [ 40 ]. Going a step further, a pithy article by Andersen [ 41 ] proffered that we may not need to look for causality or mechanistic explanations anymore if we just have enough correlation: “With enough data, the numbers speak for themselves, correlation replaces causation, and science can advance even without coherent models or unified theories.”
Of course, the proposal to abandon the quest for causality caused pushback on philosophical as well as mathematical grounds. Allen [ 10 , 35 ] considered the idea “absurd” that data analysis could enhance understanding in the absence of a hypothesis. He felt confident “that even the formidable combination of computing power with ease of access to data cannot produce a qualitative shift in the way that we do science: the making of hypotheses remains an indispensable component in the growth of knowledge” [ 36 ]. Succi and Coveney [ 42 ] refuted the “most extravagant claims” of big-data proponents very differently, namely by analyzing the theories on which machine learning is founded. They contrasted the assumptions underlying these theories, such as the law of large numbers, with the mathematical reality of complex biological systems. Specifically, they carefully identified genuine features of these systems, such as nonlinearities, nonlocality of effects, fractal aspects, and high dimensionality, and argued that they fundamentally violate some of the statistical assumptions implicitly underlying big-data analysis, like independence of events. They concluded that these discrepancies “may lead to false expectations and, at their nadir, even to dangerous social, economical and political manipulation.” To ameliorate the situation, the field of big-data analysis would need new strong theorems characterizing the validity of its methods and the numbers of data required for obtaining reliable insights. Succi and Coveney go as far as stating that too many data are just as bad as insufficient data [ 42 ].
While philosophical doubts regarding inductive methods will always persist, one cannot deny that -omics-based, high-throughput studies, combined with machine learning and big-data analysis, have been very successful [ 43 ]. Yes, induction cannot truly reveal general laws, no matter how large the datasets, but they do provide insights that are very different from what science had offered before and may at least suggest novel patterns, trends, or principles. As a case in point, if many transcriptomic studies indicate that a particular gene set is involved in certain classes of phenomena, there is probably some truth to the observation, even though it is not mathematically provable. Kepler’s laws of astronomy were arguably derived solely from inductive reasoning [ 34 ].
Notwithstanding the opposing views on inductive methods, successful strategies shape how we think about science. Thus, to take advantage of all experimental options while ensuring quality of research, we must not allow that “anything goes” but instead identify and characterize standard operating procedures and controls that render this emerging scientific method valid and reproducible. A laudable step in this direction was the wide acceptance of “minimum information about a microarray experiment” (MIAME) standards for microarray experiments [ 44 ].
A third dimension of the scientific method: Allochthonous reasoning
Parallel to the blossoming of molecular biology and the rapid rise in the power and availability of computing in the late 20th century, the use of mathematical and computational models became increasingly recognized as relevant and beneficial for understanding biological phenomena. Indeed, mathematical models eventually achieved cornerstone status in the new field of computational systems biology.
Mathematical modeling has been used as a tool of biological analysis for a long time [ 27 , 45 – 48 ]. Interesting for the discussion here is that the use of mathematical and computational modeling in biology follows a scientific approach that is distinctly different from the traditional and the data-driven methods, because it is distributed over two entirely separate domains of knowledge. One consists of the biological reality of DNA, elephants, and roses, whereas the other is the world of mathematics, which is governed by numbers, symbols, theorems, and abstract work protocols. Because the ways of thinking—and even the languages—are different in these two realms, I suggest calling this type of knowledge acquisition “allochthonous” (literally Greek: in or from a “piece of land different from where one is at home”; one could perhaps translate it into modern lingo as “outside one’s comfort zone”). De facto, most allochthonous reasoning in biology presently refers to mathematics and computing, but one might also consider, for instance, the application of methods from linguistics in the analysis of DNA sequences or proteins [ 49 ].
One could argue that biologists have employed “models” for a long time, for instance, in the form of “model organisms,” cell lines, or in vitro experiments, which more or less faithfully reflect features of the organisms of true interest but are easier to manipulate. However, this type of biological model use is rather different from allochthonous reasoning, as it does not leave the realm of biology and uses the same language and often similar methodologies.
A brief discussion of three experiences from our lab may illustrate the benefits of allochthonous reasoning. (1) In a case study of renal cell carcinoma, a dynamic model was able to explain an observed yet nonintuitive metabolic profile in terms of the enzymatic reaction steps that had been altered during the disease [ 50 ]. (2) A transcriptome analysis had identified several genes as displaying significantly different expression patterns during malaria infection in comparison to the state of health. Considered by themselves and focusing solely on genes coding for specific enzymes of purine metabolism, the findings showed patterns that did not make sense. However, integrating the changes in a dynamic model revealed that purine metabolism globally shifted, in response to malaria, from guanine compounds to adenine, inosine, and hypoxanthine [ 51 ]. (3) Data capturing the dynamics of malaria parasites suggested growth rates that were biologically impossible. Speculation regarding possible explanations led to the hypothesis that many parasite-harboring red blood cells might “hide” from circulation and therewith from detection in the blood stream. While experimental testing of the feasibility of the hypothesis would have been expensive, a dynamic model confirmed that such a concealment mechanism could indeed quantitatively explain the apparently very high growth rates [ 52 ]. In all three cases, the insights gained inductively from computational modeling would have been difficult to obtain purely with experimental laboratory methods. Purely deductive allochthonous reasoning is the ultimate goal of the search for design and operating principles [ 53 – 55 ], which strives to explain why certain structures or functions are employed by nature time and again. An example is a linear metabolic pathway, in which feedback inhibition is essentially always exerted on the first step [ 56 , 57 ]. This generality allows the deduction that a so far unstudied linear pathway is most likely (or even certain to be) inhibited at the first step. Not strictly deductive—but rather abductive—was a study in our lab in which we analyzed time series data with a mathematical model that allowed us to infer the most likely regulatory structure of a metabolic pathway [ 58 , 59 ].
A typical allochthonous investigation begins in the realm of biology with the formulation of a hypothesis ( Fig 3 ). Instead of testing this hypothesis with laboratory experiments, the system encompassing the hypothesis is moved into the realm of mathematics. This move requires two sets of ingredients. One set consists of the simplification and abstraction of the biological system: Any distracting details that seem unrelated to the hypothesis and its context are omitted or represented collectively with other details. This simplification step carries the greatest risk of the entire modeling approach, as omission of seemingly negligible but, in truth, important details can easily lead to wrong results. The second set of ingredients consists of correspondence rules that translate every biological component or process into the language of mathematics [ 60 , 61 ].
This mathematical and computational approach is distributed over two realms, which are connected by correspondence rules.
https://doi.org/10.1371/journal.pcbi.1007279.g003
Once the system is translated, it has become an entirely mathematical construct that can be analyzed purely with mathematical and computational means. The results of this analysis are also strictly mathematical. They typically consist of values of variables, magnitudes of processes, sensitivity patterns, signs of eigenvalues, or qualitative features like the onset of oscillations or the potential for limit cycles. Correspondence rules are used again to move these results back into the realm of biology. As an example, the mathematical result that “two eigenvalues have positive real parts” does not make much sense to many biologists, whereas the interpretation that “the system is not stable at the steady state in question” is readily explained. New biological insights may lead to new hypotheses, which are tested either by experiments or by returning once more to the realm of mathematics. The model design, diagnosis, refinements, and validation consist of several phases, which have been discussed widely in the biomathematical literature. Importantly, each iteration of a typical modeling analysis consists of a move from the biological to the mathematical realm and back.
The reasoning within the realm of mathematics is often deductive, in the form of an Aristotelian syllogism, such as the well-known “All men are mortal; Socrates is a man; therefore, Socrates is mortal.” However, the reasoning may also be inductive, as it is the case with large-scale Monte-Carlo simulations that generate arbitrarily many “observations,” although they cannot reveal universal principles or theorems. An example is a simulation randomly drawing numbers in an attempt to show that every real number has an inverse. The simulation will always attest to this hypothesis but fail to discover the truth because it will never randomly draw 0. Generically, computational models may be considered sets of hypotheses, formulated as equations or as algorithms that reflect our perception of a complex system [ 27 ].
Impact of the multidimensional scientific method on learning
Almost all we know in biology has come from observation, experimentation, and interpretation. The traditional scientific method not only offered clear guidance for this knowledge gathering, but it also fundamentally shaped the way we think about the exploration of nature. When presented with a new research question, scientists were trained to think immediately in terms of hypotheses and alternatives, pondering the best feasible ways of testing them, and designing in their minds strong controls that would limit the effects of known or unknown confounders. Shaped by the rigidity of this ever-repeating process, our thinking became trained to move forward one well-planned step at a time. This modus operandi was rigid and exact. It also minimized the erroneous pursuit of long speculative lines of thought, because every step required testing before a new hypothesis was formed. While effective, the process was also very slow and driven by ingenuity—as well as bias—on the scientist’s part. This bias was sometimes a hindrance to necessary paradigm shifts [ 22 ].
High-throughput data generation, big-data analysis, and mathematical-computational modeling changed all that within a few decades. In particular, the acceptance of inductive principles and of the allochthonous use of nonbiological strategies to answer biological questions created an unprecedented mix of successes and chaos. To the horror of traditionalists, the importance of hypotheses became minimized, and the suggestion spread that the data would speak for themselves [ 36 ]. Importantly, within this fog of “anything goes,” the fundamental question arose how to determine whether an experiment was valid.
Because agreed-upon operating procedures affect research progress and interpretation, thinking, teaching, and sharing of results, this question requires a deconvolution of scientific strategies. Here I proffer that the single scientific method of the past should be expanded toward a vector space of scientific methods, with spanning vectors that correspond to different dimensions of the scientific method ( Fig 4 ).
The traditional hypothesis-based deductive scientific method is expanded into a 3D space that allows for synergistic blends of methods that include data-mining–inspired, inductive knowledge acquisition, and mathematical model-based, allochthonous reasoning.
https://doi.org/10.1371/journal.pcbi.1007279.g004
Obviously, all three dimensions have their advantages and drawbacks. The traditional, hypothesis-driven deductive method is philosophically “clean,” except that it is confounded by preconceptions and assumptions. The data-mining–inspired inductive method cannot offer universal truths but helps us explore very large spaces of factors that contribute to a phenomenon. Allochthonous, model-based reasoning can be performed mentally, with paper and pencil, through rigorous analysis, or with a host of computational methods that are precise and disprovable [ 27 ]. At the same time, they are incomparable faster, cheaper, and much more comprehensive than experiments in molecular biology. This reduction in cost and time, and the increase in coverage, may eventually have far-reaching consequences, as we can already fathom from much of modern physics.
Due to its long history, the traditional dimension of the scientific method is supported by clear and very strong standard operating procedures. Similarly, strong procedures need to be developed for the other two dimensions. The MIAME rules for microarray analysis provide an excellent example [ 44 ]. On the mathematical modeling front, no such rules are generally accepted yet, but trends toward them seem to emerge at the horizon. For instance, it seems to be becoming common practice to include sensitivity analyses in typical modeling studies and to assess the identifiability or sloppiness of ensembles of parameter combinations that fit a given dataset well [ 62 , 63 ].
From a philosophical point of view, it seems unlikely that objections against inductive reasoning will disappear. However, instead of pitting hypothesis-based deductive reasoning against inductivism, it seems more beneficial to determine how the different methods can be synergistically blended ( cf . [ 18 , 27 , 34 , 42 ]) as linear combinations of the three vectors of knowledge acquisition ( Fig 4 ). It is at this point unclear to what degree the identified three dimensions are truly independent of each other, whether additional dimensions should be added [ 24 ], or whether the different versions could be amalgamated into a single scientific method [ 18 ], especially if it is loosely defined as a form of critical thinking [ 8 ]. Nobel Laureate Percy Bridgman even concluded that “science is what scientists do, and there are as many scientific methods as there are individual scientists” [ 8 , 64 ].
Combinations of the three spanning vectors of the scientific method have been emerging for some time. Many biologists already use inductive high-throughput methods to develop specific hypotheses that are subsequently tested with deductive or further inductive methods [ 34 , 65 ]. In terms of including mathematical modeling, physics and geology have been leading the way for a long time, often by beginning an investigation in theory, before any actual experiment is performed. It will benefit biology to look into this strategy and to develop best practices of allochthonous reasoning.
The blending of methods may take quite different shapes. Early on, Ideker and colleagues [ 65 ] proposed an integrated experimental approach for pathway analysis that offered a glimpse of new experimental strategies within the space of scientific methods. In a similar vein, Covert and colleagues [ 66 ] included computational methods into such an integrated approach. Additional examples of blended analyses in systems biology can be seen in other works, such as [ 43 , 67 – 73 ]. Generically, it is often beneficial to start with big data, determine patterns in associations and correlations, then switch to the mathematical realm in order to filter out spurious correlations in a high-throughput fashion. If this procedure is executed in an iterative manner, the “surviving” associations have an increased level of confidence and are good candidates for further experimental or computational testing (personal communication from S. Chandrasekaran).
If each component of a blended scientific method follows strict, commonly agreed guidelines, “linear combinations” within the 3D space can also be checked objectively, per deconvolution. In addition, guidelines for synergistic blends of component procedures should be developed. If we carefully monitor such blends, time will presumably indicate which method is best for which task and how the different approaches optimally inform each other. For instance, it will be interesting to study whether there is an optimal sequence of experiments along the three axes for a particular class of tasks. Big-data analysis together with inductive reasoning might be optimal for creating initial hypotheses and possibly refuting wrong speculations (“we had thought this gene would be involved, but apparently it isn’t”). If the logic of an emerging hypotheses can be tested with mathematical and computational tools, it will almost certainly be faster and cheaper than an immediate launch into wet-lab experimentation. It is also likely that mathematical reasoning will be able to refute some apparently feasible hypothesis and suggest amendments. Ultimately, the “surviving” hypotheses must still be tested for validity through conventional experiments. Deconvolving current practices and optimizing the combination of methods within the 3D or higher-dimensional space of scientific methods will likely result in better planning of experiments and in synergistic blends of approaches that have the potential capacity of addressing some of the grand challenges in biology.
Acknowledgments
The author is very grateful to Dr. Sriram Chandrasekaran and Ms. Carla Kumbale for superb suggestions and invaluable feedback.
- View Article
- Google Scholar
- 2. Gauch HGJ. Scientific Method in Brief. Cambridge, UK.: Cambridge University Press; 2012.
- 3. Gimbel S (Ed). Exploring the Scientific Method: Cases and Questions. Chicago, IL: The University of Chicago Press; 2011.
- PubMed/NCBI
- 8. McLelland CV. The nature of science and the scientific method. Boulder, CO: The Geological Society of America; 2006.
- 9. Ladyman J. Understanding Philosophy of Science. Abington, Oxon: Routledge; 2002.
- 16. Popper KR. Conjectures and Refutations: The Growth of Scientific Knowledge. Abingdon, Oxon: Routledge and Kegan Paul; 1963.
- 17. Popper KR. The Logic of Scientific Discovery. Abingdon, Oxon: Routledge; 2002.
- 21. Harding SE. Can theories be refuted?: Essays on the Duhem-Quine thesis. Dordrecht-Holland / Boston, MA: D. Reidel Publ. Co; 1976.
- 22. Kuhn TS. The Structure of Scientific Revolutions. Chicago, IL: University of Chicago Press; 1962.
- 37. Hume D. An enquiry concerning human understanding. Oxford, U.K.: Oxford University Press; 1748/1999.
- 38. Popper KR. Objective knowledge. An evolutionary approach. Oxford, U.K.: Oxford University Press; 1972.
- 47. von Bertalanffy L. General System Theory: Foundations, Development, Applications. New York: George Braziller; 1968.
- 48. May RM. Stability and Complexity in Model Ecosystems: Princeton University Press; 1973.
- 57. Savageau MA. Biochemical Systems Analysis: A Study of Function and Design in Molecular Biology. Reading, Mass: Addison-Wesley Pub. Co. Advanced Book Program (reprinted 2009); 1976.
- 60. Reither F. Über das Denken mit Analogien und Modellen. In: Schaefer G, Trommer G, editors. Denken in Modellen. Braunschweig, Germany: Georg Westermann Verlag; 1977.
- 61. Voit EO. A First Course in Systems Biology, 2nd Ed. New York, NY: Garland Science; 2018.
- 64. Bridgman PW. Reflections of a Physicist. New York, NY: reprinted by Kessinger Legacy Reprints, 2010; 1955.
Society Homepage About Public Health Policy Contact
Data-driven hypothesis generation in clinical research: what we learned from a human subject study, article sidebar.
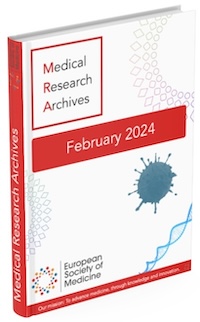
Submit your own article
Register as an author to reserve your spot in the next issue of the Medical Research Archives.
Join the Society
The European Society of Medicine is more than a professional association. We are a community. Our members work in countries across the globe, yet are united by a common goal: to promote health and health equity, around the world.
Join Europe’s leading medical society and discover the many advantages of membership, including free article publication.
Main Article Content
Hypothesis generation is an early and critical step in any hypothesis-driven clinical research project. Because it is not yet a well-understood cognitive process, the need to improve the process goes unrecognized. Without an impactful hypothesis, the significance of any research project can be questionable, regardless of the rigor or diligence applied in other steps of the study, e.g., study design, data collection, and result analysis. In this perspective article, the authors provide a literature review on the following topics first: scientific thinking, reasoning, medical reasoning, literature-based discovery, and a field study to explore scientific thinking and discovery. Over the years, scientific thinking has shown excellent progress in cognitive science and its applied areas: education, medicine, and biomedical research. However, a review of the literature reveals the lack of original studies on hypothesis generation in clinical research. The authors then summarize their first human participant study exploring data-driven hypothesis generation by clinical researchers in a simulated setting. The results indicate that a secondary data analytical tool, VIADS—a visual interactive analytic tool for filtering, summarizing, and visualizing large health data sets coded with hierarchical terminologies, can shorten the time participants need, on average, to generate a hypothesis and also requires fewer cognitive events to generate each hypothesis. As a counterpoint, this exploration also indicates that the quality ratings of the hypotheses thus generated carry significantly lower ratings for feasibility when applying VIADS. Despite its small scale, the study confirmed the feasibility of conducting a human participant study directly to explore the hypothesis generation process in clinical research. This study provides supporting evidence to conduct a larger-scale study with a specifically designed tool to facilitate the hypothesis-generation process among inexperienced clinical researchers. A larger study could provide generalizable evidence, which in turn can potentially improve clinical research productivity and overall clinical research enterprise.
Article Details
The Medical Research Archives grants authors the right to publish and reproduce the unrevised contribution in whole or in part at any time and in any form for any scholarly non-commercial purpose with the condition that all publications of the contribution include a full citation to the journal as published by the Medical Research Archives .
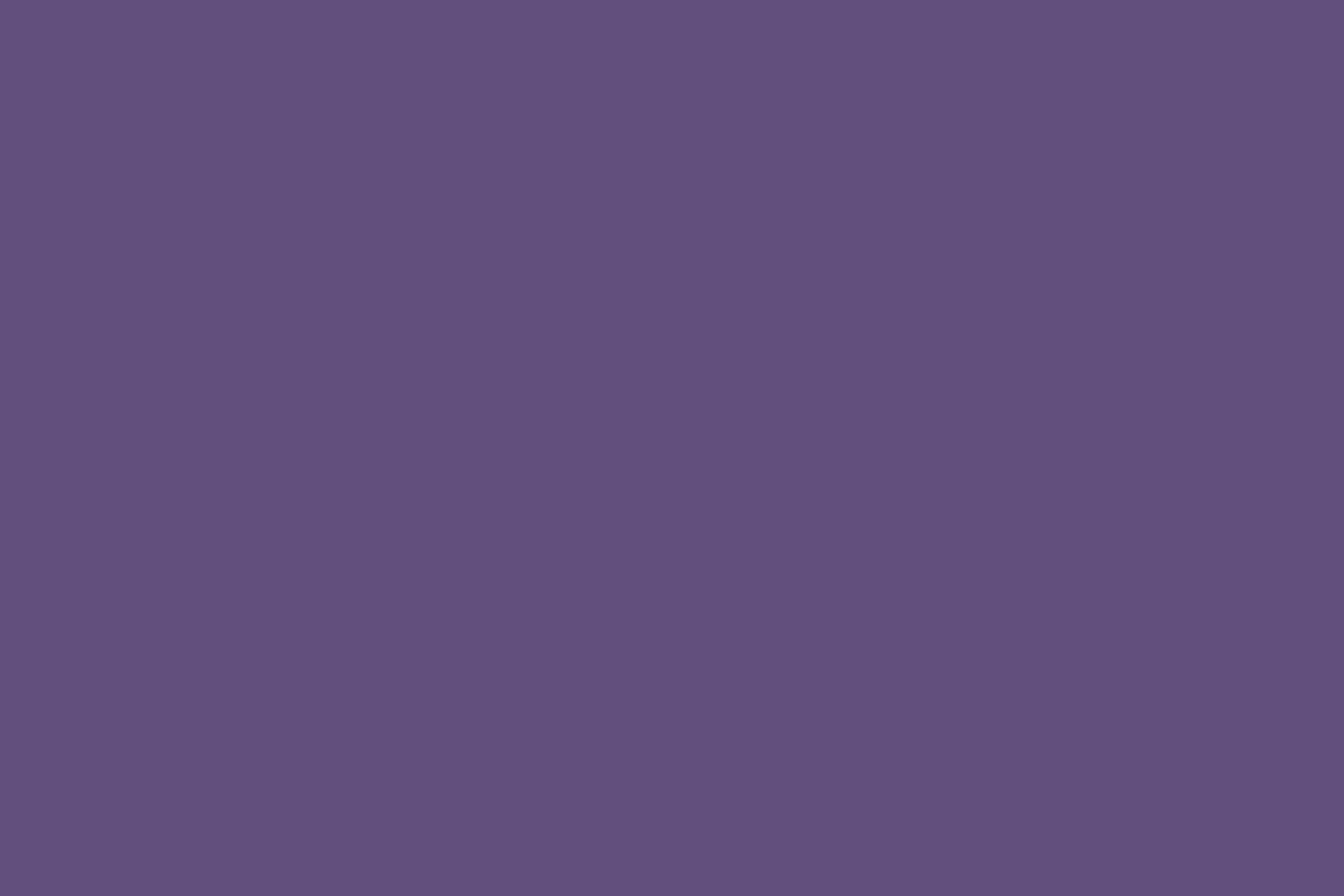
How to Implement Hypothesis-Driven Development
Remember back to the time when we were in high school science class. Our teachers had a framework for helping us learn – an experimental approach based on the best available evidence at hand. We were asked to make observations about the world around us, then attempt to form an explanation or hypothesis to explain what we had observed. We then tested this hypothesis by predicting an outcome based on our theory that would be achieved in a controlled experiment – if the outcome was achieved, we had proven our theory to be correct.
We could then apply this learning to inform and test other hypotheses by constructing more sophisticated experiments, and tuning, evolving or abandoning any hypothesis as we made further observations from the results we achieved.
Experimentation is the foundation of the scientific method, which is a systematic means of exploring the world around us. Although some experiments take place in laboratories, it is possible to perform an experiment anywhere, at any time, even in software development.
Practicing Hypothesis-Driven Development is thinking about the development of new ideas, products and services – even organizational change – as a series of experiments to determine whether an expected outcome will be achieved. The process is iterated upon until a desirable outcome is obtained or the idea is determined to be not viable.
We need to change our mindset to view our proposed solution to a problem statement as a hypothesis, especially in new product or service development – the market we are targeting, how a business model will work, how code will execute and even how the customer will use it.
We do not do projects anymore, only experiments. Customer discovery and Lean Startup strategies are designed to test assumptions about customers. Quality Assurance is testing system behavior against defined specifications. The experimental principle also applies in Test-Driven Development – we write the test first, then use the test to validate that our code is correct, and succeed if the code passes the test. Ultimately, product or service development is a process to test a hypothesis about system behaviour in the environment or market it is developed for.
The key outcome of an experimental approach is measurable evidence and learning.
Learning is the information we have gained from conducting the experiment. Did what we expect to occur actually happen? If not, what did and how does that inform what we should do next?
In order to learn we need use the scientific method for investigating phenomena, acquiring new knowledge, and correcting and integrating previous knowledge back into our thinking.
As the software development industry continues to mature, we now have an opportunity to leverage improved capabilities such as Continuous Design and Delivery to maximize our potential to learn quickly what works and what does not. By taking an experimental approach to information discovery, we can more rapidly test our solutions against the problems we have identified in the products or services we are attempting to build. With the goal to optimize our effectiveness of solving the right problems, over simply becoming a feature factory by continually building solutions.
The steps of the scientific method are to:
- Make observations
- Formulate a hypothesis
- Design an experiment to test the hypothesis
- State the indicators to evaluate if the experiment has succeeded
- Conduct the experiment
- Evaluate the results of the experiment
- Accept or reject the hypothesis
- If necessary, make and test a new hypothesis
Using an experimentation approach to software development
We need to challenge the concept of having fixed requirements for a product or service. Requirements are valuable when teams execute a well known or understood phase of an initiative, and can leverage well understood practices to achieve the outcome. However, when you are in an exploratory, complex and uncertain phase you need hypotheses.
Handing teams a set of business requirements reinforces an order-taking approach and mindset that is flawed.
Business does the thinking and ‘knows’ what is right. The purpose of the development team is to implement what they are told. But when operating in an area of uncertainty and complexity, all the members of the development team should be encouraged to think and share insights on the problem and potential solutions. A team simply taking orders from a business owner is not utilizing the full potential, experience and competency that a cross-functional multi-disciplined team offers.
Framing hypotheses
The traditional user story framework is focused on capturing requirements for what we want to build and for whom, to enable the user to receive a specific benefit from the system.
As A…. <role>
I Want… <goal/desire>
So That… <receive benefit>
Behaviour Driven Development (BDD) and Feature Injection aims to improve the original framework by supporting communication and collaboration between developers, tester and non-technical participants in a software project.
In Order To… <receive benefit>
As A… <role>
When viewing work as an experiment, the traditional story framework is insufficient. As in our high school science experiment, we need to define the steps we will take to achieve the desired outcome. We then need to state the specific indicators (or signals) we expect to observe that provide evidence that our hypothesis is valid. These need to be stated before conducting the test to reduce biased interpretations of the results.
If we observe signals that indicate our hypothesis is correct, we can be more confident that we are on the right path and can alter the user story framework to reflect this.
Therefore, a user story structure to support Hypothesis-Driven Development would be;
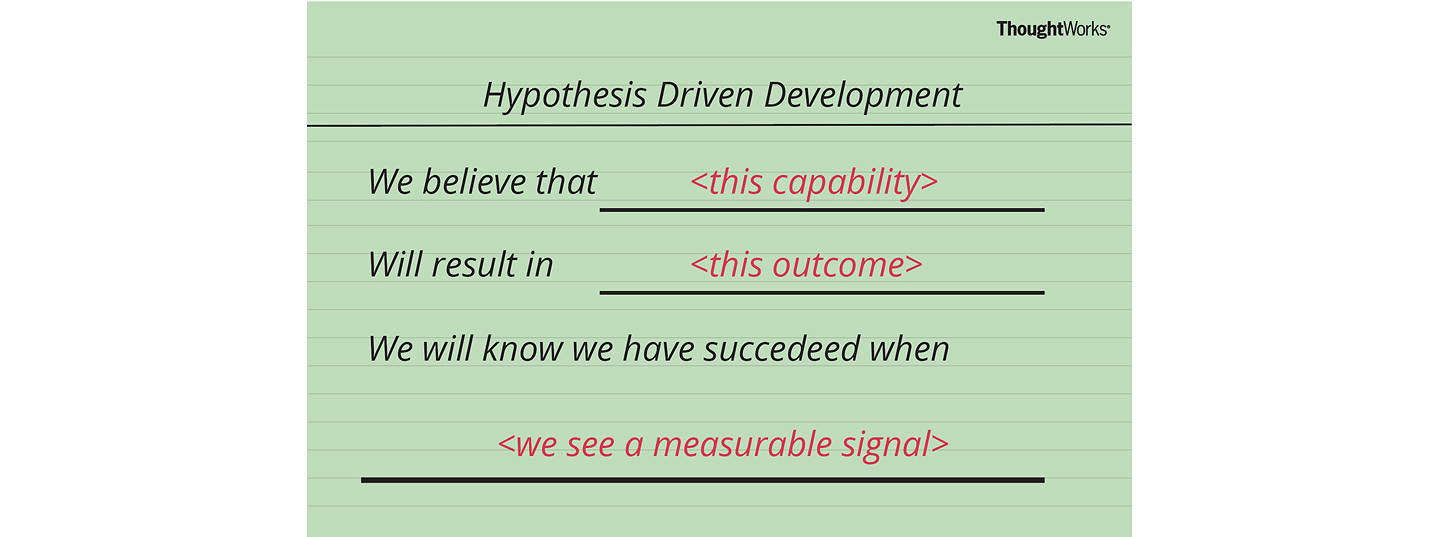
We believe < this capability >
What functionality we will develop to test our hypothesis? By defining a ‘test’ capability of the product or service that we are attempting to build, we identify the functionality and hypothesis we want to test.
Will result in < this outcome >
What is the expected outcome of our experiment? What is the specific result we expect to achieve by building the ‘test’ capability?
We will know we have succeeded when < we see a measurable signal >
What signals will indicate that the capability we have built is effective? What key metrics (qualitative or quantitative) we will measure to provide evidence that our experiment has succeeded and give us enough confidence to move to the next stage.
The threshold you use for statistically significance will depend on your understanding of the business and context you are operating within. Not every company has the user sample size of Amazon or Google to run statistically significant experiments in a short period of time. Limits and controls need to be defined by your organization to determine acceptable evidence thresholds that will allow the team to advance to the next step.
For example if you are building a rocket ship you may want your experiments to have a high threshold for statistical significance. If you are deciding between two different flows intended to help increase user sign up you may be happy to tolerate a lower significance threshold.
The final step is to clearly and visibly state any assumptions made about our hypothesis, to create a feedback loop for the team to provide further input, debate and understanding of the circumstance under which we are performing the test. Are they valid and make sense from a technical and business perspective?
Hypotheses when aligned to your MVP can provide a testing mechanism for your product or service vision. They can test the most uncertain areas of your product or service, in order to gain information and improve confidence.
Examples of Hypothesis-Driven Development user stories are;
Business story
We Believe That increasing the size of hotel images on the booking page
Will Result In improved customer engagement and conversion
We Will Know We Have Succeeded When we see a 5% increase in customers who review hotel images who then proceed to book in 48 hours.
It is imperative to have effective monitoring and evaluation tools in place when using an experimental approach to software development in order to measure the impact of our efforts and provide a feedback loop to the team. Otherwise we are essentially blind to the outcomes of our efforts.
In agile software development we define working software as the primary measure of progress.
By combining Continuous Delivery and Hypothesis-Driven Development we can now define working software and validated learning as the primary measures of progress.
Ideally we should not say we are done until we have measured the value of what is being delivered – in other words, gathered data to validate our hypothesis.
Examples of how to gather data is performing A/B Testing to test a hypothesis and measure to change in customer behaviour. Alternative testings options can be customer surveys, paper prototypes, user and/or guerrilla testing.
One example of a company we have worked with that uses Hypothesis-Driven Development is lastminute.com . The team formulated a hypothesis that customers are only willing to pay a max price for a hotel based on the time of day they book. Tom Klein, CEO and President of Sabre Holdings shared the story of how they improved conversion by 400% within a week.
Combining practices such as Hypothesis-Driven Development and Continuous Delivery accelerates experimentation and amplifies validated learning. This gives us the opportunity to accelerate the rate at which we innovate while relentlessly reducing cost, leaving our competitors in the dust. Ideally we can achieve the ideal of one piece flow: atomic changes that enable us to identify causal relationships between the changes we make to our products and services, and their impact on key metrics.
As Kent Beck said, “Test-Driven Development is a great excuse to think about the problem before you think about the solution”. Hypothesis-Driven Development is a great opportunity to test what you think the problem is, before you work on the solution.
How can you achieve faster growth?

What Is A Research (Scientific) Hypothesis? A plain-language explainer + examples
By: Derek Jansen (MBA) | Reviewed By: Dr Eunice Rautenbach | June 2020
If you’re new to the world of research, or it’s your first time writing a dissertation or thesis, you’re probably noticing that the words “research hypothesis” and “scientific hypothesis” are used quite a bit, and you’re wondering what they mean in a research context .
“Hypothesis” is one of those words that people use loosely, thinking they understand what it means. However, it has a very specific meaning within academic research. So, it’s important to understand the exact meaning before you start hypothesizing.
Research Hypothesis 101
- What is a hypothesis ?
- What is a research hypothesis (scientific hypothesis)?
- Requirements for a research hypothesis
- Definition of a research hypothesis
- The null hypothesis
What is a hypothesis?
Let’s start with the general definition of a hypothesis (not a research hypothesis or scientific hypothesis), according to the Cambridge Dictionary:
Hypothesis: an idea or explanation for something that is based on known facts but has not yet been proved.
In other words, it’s a statement that provides an explanation for why or how something works, based on facts (or some reasonable assumptions), but that has not yet been specifically tested . For example, a hypothesis might look something like this:
Hypothesis: sleep impacts academic performance.
This statement predicts that academic performance will be influenced by the amount and/or quality of sleep a student engages in – sounds reasonable, right? It’s based on reasonable assumptions , underpinned by what we currently know about sleep and health (from the existing literature). So, loosely speaking, we could call it a hypothesis, at least by the dictionary definition.
But that’s not good enough…
Unfortunately, that’s not quite sophisticated enough to describe a research hypothesis (also sometimes called a scientific hypothesis), and it wouldn’t be acceptable in a dissertation, thesis or research paper . In the world of academic research, a statement needs a few more criteria to constitute a true research hypothesis .
What is a research hypothesis?
A research hypothesis (also called a scientific hypothesis) is a statement about the expected outcome of a study (for example, a dissertation or thesis). To constitute a quality hypothesis, the statement needs to have three attributes – specificity , clarity and testability .
Let’s take a look at these more closely.
Need a helping hand?
Hypothesis Essential #1: Specificity & Clarity
A good research hypothesis needs to be extremely clear and articulate about both what’ s being assessed (who or what variables are involved ) and the expected outcome (for example, a difference between groups, a relationship between variables, etc.).
Let’s stick with our sleepy students example and look at how this statement could be more specific and clear.
Hypothesis: Students who sleep at least 8 hours per night will, on average, achieve higher grades in standardised tests than students who sleep less than 8 hours a night.
As you can see, the statement is very specific as it identifies the variables involved (sleep hours and test grades), the parties involved (two groups of students), as well as the predicted relationship type (a positive relationship). There’s no ambiguity or uncertainty about who or what is involved in the statement, and the expected outcome is clear.
Contrast that to the original hypothesis we looked at – “Sleep impacts academic performance” – and you can see the difference. “Sleep” and “academic performance” are both comparatively vague , and there’s no indication of what the expected relationship direction is (more sleep or less sleep). As you can see, specificity and clarity are key.
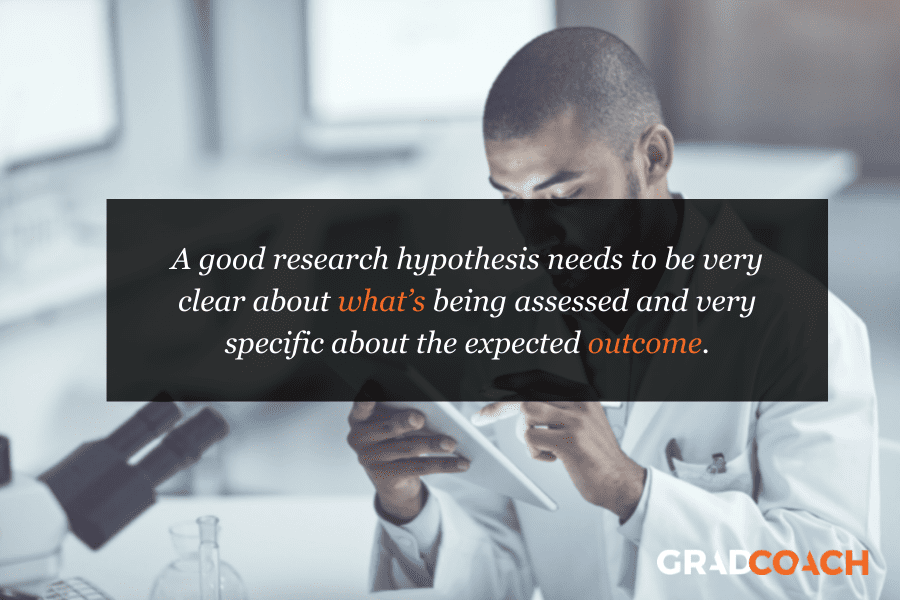
Hypothesis Essential #2: Testability (Provability)
A statement must be testable to qualify as a research hypothesis. In other words, there needs to be a way to prove (or disprove) the statement. If it’s not testable, it’s not a hypothesis – simple as that.
For example, consider the hypothesis we mentioned earlier:
Hypothesis: Students who sleep at least 8 hours per night will, on average, achieve higher grades in standardised tests than students who sleep less than 8 hours a night.
We could test this statement by undertaking a quantitative study involving two groups of students, one that gets 8 or more hours of sleep per night for a fixed period, and one that gets less. We could then compare the standardised test results for both groups to see if there’s a statistically significant difference.
Again, if you compare this to the original hypothesis we looked at – “Sleep impacts academic performance” – you can see that it would be quite difficult to test that statement, primarily because it isn’t specific enough. How much sleep? By who? What type of academic performance?
So, remember the mantra – if you can’t test it, it’s not a hypothesis 🙂
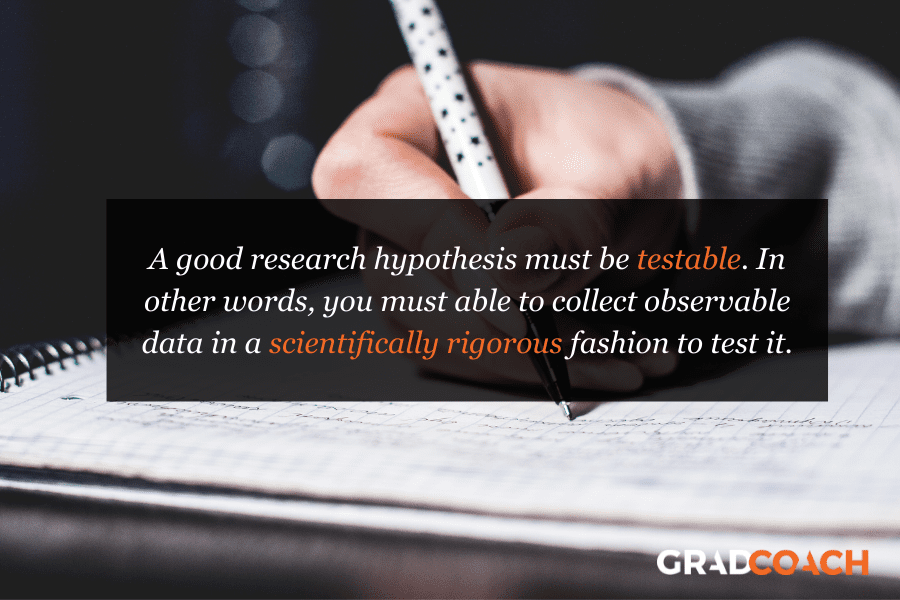
Defining A Research Hypothesis
You’re still with us? Great! Let’s recap and pin down a clear definition of a hypothesis.
A research hypothesis (or scientific hypothesis) is a statement about an expected relationship between variables, or explanation of an occurrence, that is clear, specific and testable.
So, when you write up hypotheses for your dissertation or thesis, make sure that they meet all these criteria. If you do, you’ll not only have rock-solid hypotheses but you’ll also ensure a clear focus for your entire research project.
What about the null hypothesis?
You may have also heard the terms null hypothesis , alternative hypothesis, or H-zero thrown around. At a simple level, the null hypothesis is the counter-proposal to the original hypothesis.
For example, if the hypothesis predicts that there is a relationship between two variables (for example, sleep and academic performance), the null hypothesis would predict that there is no relationship between those variables.
At a more technical level, the null hypothesis proposes that no statistical significance exists in a set of given observations and that any differences are due to chance alone.
And there you have it – hypotheses in a nutshell.
If you have any questions, be sure to leave a comment below and we’ll do our best to help you. If you need hands-on help developing and testing your hypotheses, consider our private coaching service , where we hold your hand through the research journey.
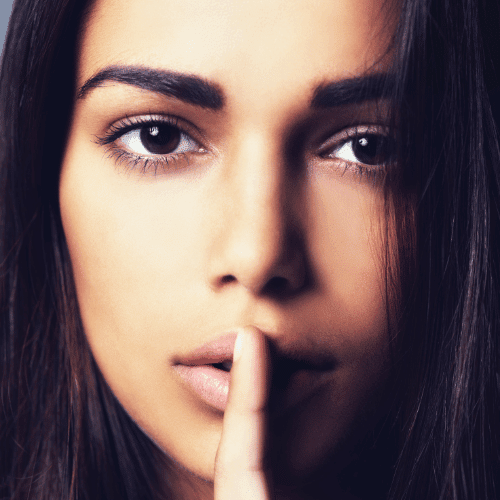
Psst... there’s more!
This post was based on one of our popular Research Bootcamps . If you're working on a research project, you'll definitely want to check this out ...
17 Comments
Very useful information. I benefit more from getting more information in this regard.
Very great insight,educative and informative. Please give meet deep critics on many research data of public international Law like human rights, environment, natural resources, law of the sea etc
In a book I read a distinction is made between null, research, and alternative hypothesis. As far as I understand, alternative and research hypotheses are the same. Can you please elaborate? Best Afshin
This is a self explanatory, easy going site. I will recommend this to my friends and colleagues.
Very good definition. How can I cite your definition in my thesis? Thank you. Is nul hypothesis compulsory in a research?
It’s a counter-proposal to be proven as a rejection
Please what is the difference between alternate hypothesis and research hypothesis?
It is a very good explanation. However, it limits hypotheses to statistically tasteable ideas. What about for qualitative researches or other researches that involve quantitative data that don’t need statistical tests?
In qualitative research, one typically uses propositions, not hypotheses.
could you please elaborate it more
I’ve benefited greatly from these notes, thank you.
This is very helpful
well articulated ideas are presented here, thank you for being reliable sources of information
Excellent. Thanks for being clear and sound about the research methodology and hypothesis (quantitative research)
I have only a simple question regarding the null hypothesis. – Is the null hypothesis (Ho) known as the reversible hypothesis of the alternative hypothesis (H1? – How to test it in academic research?
this is very important note help me much more
Hi” best wishes to you and your very nice blog”
Trackbacks/Pingbacks
- What Is Research Methodology? Simple Definition (With Examples) - Grad Coach - […] Contrasted to this, a quantitative methodology is typically used when the research aims and objectives are confirmatory in nature. For example,…
Submit a Comment Cancel reply
Your email address will not be published. Required fields are marked *
Save my name, email, and website in this browser for the next time I comment.
- Print Friendly
Thank you for visiting nature.com. You are using a browser version with limited support for CSS. To obtain the best experience, we recommend you use a more up to date browser (or turn off compatibility mode in Internet Explorer). In the meantime, to ensure continued support, we are displaying the site without styles and JavaScript.
- View all journals
- Explore content
- About the journal
- Publish with us
- Sign up for alerts
- Published: April 2009
Defining the scientific method
Nature Methods volume 6 , page 237 ( 2009 ) Cite this article
36k Accesses
32 Citations
21 Altmetric
Metrics details
The rise of 'omics' methods and data-driven research presents new possibilities for discovery but also stimulates disagreement over how science should be conducted and even how it should be defined.
Please visit methagora to view and post comments on this article.
Modern biological research methods are powerful tools in biologists' arsenal for investigating biology. But is the ability of these methods to amass extraordinary amounts of data altering the nature of scientific inquiry?
As schoolchildren we are taught that the scientific method involves a question and suggested explanation (hypothesis) based on observation, followed by the careful design and execution of controlled experiments, and finally validation, refinement or rejection of this hypothesis. Developed by thinkers including Bacon, Descartes and Pierce, this methodology has been credited with much of science's success. Modern philosophers such as Feyerabend have argued that this is not how most science is conducted, but up to now most modern scientists have subscribed to the hypothesis-centric scientific method.
Scientists' defense of this methodology has often been vigorous, likely owing to the historic success of predictive hypothesis-driven mechanistic theories in physics, the dangers inherent in 'fishing expeditions' and the likelihood of false correlations based on data from improperly designed experiments. For example, The Human Genome Project was considered by many at the time to be a serious break with the notion that proper biological research must be hypothesis-driven. But the project proceeded because others successfully argued that it would yield information vital for understanding human biology.
Methodological developments are now making it possible to obtain massive amounts of 'omics' data on a variety of biological constituents. These immense datasets allow biologists to generate useful predictions (for example, gene-finding and function or protein structure and function) using machine learning and statistics that do not take into account the underlying mechanisms that dictate design and function—considerations that would form the basis of a traditional hypothesis.
Now that the bias against data-driven investigation has weakened, the desire to simplify 'omics' data reuse has led to the establishment of minimal information requirements for different types of primary data. The hope is that this will allow new analyses and predictions using aggregated data from disparate experiments.
Last summer, the editor-in-chief of Wired , Chris Anderson, went so far as to argue that biology is too complex for hypotheses and models, and that the classical scientific method is dead. Instead, he called for these methods to be replaced by powerful correlative analyses of massive amounts of data gathered by new technologies similar to how Google Translate relies on only correlative analyses of documents on the internet.
“Hypotheses aren't simply useful tools in some potentially outmoded vision of science; they are the whole point.” Sean Carroll
This generated quite a response from the scientific community with California Institute of Technology physicist Sean Carroll arguing in Edge that “hypotheses aren't simply useful tools in some potentially outmoded vision of science; they are the whole point. Theory is understanding, and understanding our world is what science is all about.”
“Science, it turns out, is whatever scientists do.” David Goodstein
Is the generation of parts lists and correlations in the absence of functional models science? Based on the often accepted definition of the scientific method, the answer would be a qualified no. But not everyone would agree. Carroll's colleague, David Goodstein, previously stated in a Thesis article in Nature Physics that “science, it turns out, is whatever scientists do.” A philosopher would find this to be a circular and unfulfilling argument, but it is likely that many biologists who are more interested in the practical outcomes of their methods than their philosophical underpinnings would agree with this sentiment.
But the rise of methodologies that generate massive amounts of data does not dictate that biology should be data-driven. In a return to hypothesis-driven research, systems biologists are attempting to use the same 'omics' methods to generate data for use in quantitative biological models. Hypotheses are needed before data collection because model-driven quantitative analyses require rich dynamic data collected under defined conditions and stimuli.
So where does this leave us? It is likely that the high complexity of biology will actually make full biological understanding by purely correlative analysis impossible. This method works for Google because language has simple rules and low complexity. Biology has neither constraint. Correlations in large datasets may be able to provide some useful answers, but not all of them.
But 'omics' data can provide information on the size and composition of biological entities and thus determine the boundaries of the problem at hand. Biologists can then proceed to investigate function using classical hypothesis-driven experiments. It is still unclear whether even this marriage of the two methods will deliver a complete understanding of biology, but it arguably has a better chance than either method on its own.
Philosophers are free to argue whether one method is science and the other is not. Ultimately the public who funds the work and the biologists who conduct it want results that will materially impact the quality of life regardless of what the method is called.
Rights and permissions
Reprints and permissions
About this article
Cite this article.
Defining the scientific method. Nat Methods 6 , 237 (2009). https://doi.org/10.1038/nmeth0409-237
Download citation
Issue Date : April 2009
DOI : https://doi.org/10.1038/nmeth0409-237
Share this article
Anyone you share the following link with will be able to read this content:
Sorry, a shareable link is not currently available for this article.
Provided by the Springer Nature SharedIt content-sharing initiative
This article is cited by
Influence of the nutritional status on facial morphology in young japanese women.
- Chihiro Tanikawa
- Miki Kurata
- Kenji Takada
Scientific Reports (2022)
Big data in IBD: a look into the future
- Pablo Olivera
- Silvio Danese
- Laurent Peyrin-Biroulet
Nature Reviews Gastroenterology & Hepatology (2019)
Efficient modeling, simulation and coarse-graining of biological complexity with NFsim
- Michael W Sneddon
- James R Faeder
- Thierry Emonet
Nature Methods (2011)
Quick links
- Explore articles by subject
- Guide to authors
- Editorial policies
Sign up for the Nature Briefing newsletter — what matters in science, free to your inbox daily.

Hypothesis-driven science in large-scale studies: the case of GWAS
- Open access
- Published: 19 September 2021
- Volume 36 , article number 46 , ( 2021 )
Cite this article
You have full access to this open access article
- James Read ORCID: orcid.org/0000-0003-2226-0340 1 &
- Sumana Sharma ORCID: orcid.org/0000-0003-0598-2181 2
6892 Accesses
3 Citations
1 Altmetric
Explore all metrics
It is now well-appreciated by philosophers that contemporary large-scale ‘-omics’ studies in biology stand in non-trivial relationships to more orthodox hypothesis-driven approaches. These relationships have been clarified by Ratti ( 2015 ); however, there remains much more to be said regarding how an important field of genomics cited in that work—‘genome-wide association studies’ (GWAS)—fits into this framework. In the present article, we propose a revision to Ratti’s framework more suited to studies such as GWAS. In the process of doing so, we introduce to the philosophical literature novel exploratory experiments in (phospho)proteomics, and demonstrate how these experiments interplay with the above considerations.
Similar content being viewed by others
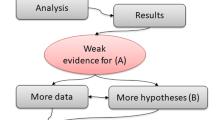
Raiders of the lost HARK: a reproducible inference framework for big data science
On Fishing for Significance and Statistician’s Degree of Freedom in the Era of Big Molecular Data
Experimental Discovery, Data Models, and Mechanisms in Biology: An Example from Mendel’s Work
Avoid common mistakes on your manuscript.
Introduction
The fields of molecular biology and genetics were transformed upon completion in 2001 of the Human Genome Project (Lander et al. 2001 ). This provided for the first time near-complete information on the genetic makeup of human beings, and marked the advent of what has become known as the ‘post-genomics’ era, defined by the availability of large-scale data sets derived from ‘genome-scale’ approaches. In turn, this has led to a shift in biological methodology, from carefully constructed hypothesis-driven research, to unbiased data-driven approaches, sometimes called ‘-omics’ studies. These studies have attracted philosophical interest in recent years: see e.g. Burian ( 2007 ); O’Malley et al. ( 2010 ); Ratti ( 2015 ); for more general philosophical discussions of large-scale data-driven approaches in contemporary post-genomics biology, see e.g. Leonelli ( 2016 ); Richardson and Stevens ( 2015 ).
Recall that -omics studies fall into three main categories: ‘genomics’, ‘transcriptomics’, and ‘proteomics’. The salient features of these three categories as as follows (we make no claim that these features exhaust any of the three categories; they are, however, the features which are relevant to the present article). Genomics is the study of the complete set of genes (composed of DNA) inside a cell. Cellular processes lead to genetic information being transcribed (copied) into molecules known as RNA. ‘Messenger RNA’ (mRNA) carries information corresponding to the genetic sequence of a gene. Transcriptomics is the study of the complete set of RNA transcripts that are produced by the genome. Finally, the information encoded in mRNA is used by cellular machinery called ribosomes to construct proteins; proteomics is the systematic study of these proteins within a cell. Proteins are the ultimate workhorses of the cell; proteomics studies aim to characterise cellular functions mediated by protein networks, in which nodes represent proteins and edges represent physical/functional interactions between them. For further background on genomics, transcriptomics, and proteomics, see Hasin et al. ( 2017 ).
Large-scale -omics studies are often described as being ‘hypothesis-free’. To take one example from genomics: advances in genome-editing techniques mean that it is now possible to generate ‘loss-of-function’ mutants in the laboratory. Such mutations are inactivating in the sense that they lead to the loss in the function of a gene within a cell. In the last few years, CRISPR-Cas9 technology has emerged, which makes it possible to create targetted loss-of-function mutants for any of the nearly 20,000 genes in the human genome (Doudna and Charpentier 2014 ). This allows researchers to ‘screen’ for a gene the loss of which leads to the phenotype of interest, thereby identifying the function of that gene. The methodological idea behind such screening approaches is that one does not require any background hypothesis as to which gene could be involved in a particular biological process, or associated with a particular phenotype: hence the widespread declaration that such approaches are ‘hypothesis-free’ (Shalem et al. 2015 ). As Burian writes, “Genomics, proteomics, and related “omics” disciplines represent a break with the ideal of hypothesis-driven science” (Burian 2007 , p. 289).
With Ratti ( 2015 ); Franklin ( 2005 ), and others, we find the terminology of ‘hypothesis-free’ to be misleading—for, in fact, such large-scale studies exhibit a Janus-faced dependence on mechanistic hypotheses of a quite standard sort. Ratti characterises such studies, and their connections with more orthodox mechanistic hypothesis-driven science, as involving three steps:
1. The generation of a preliminary set of hypotheses from an established set of premises; 2. The prioritization of some hypotheses and discarding of others by means of other premises and new evidence; 3. The search for more stringent evidence for prioritized hypotheses. (Ratti 2015 , p. 201)
In step (1), scientific hypothesising plays a role, insofar as it is used to delimit the domain of inquiry of the study. For example, a loss-of-function screen to identify the receptor for a pathogen would hypothesise that there exists a non-redundant mechanism for the interaction of the pathogen with the cells, and that the loss of this cellular factor/mechanism would lead to diminution of interaction of the pathogen with the cell surface. For the purpose of the test, such hypotheses are regarded as indubitable: they delimit the range of acceptable empirical enquiry. But there is also a forward-looking dependence of these approaches on scientific hypothesising: the results of such studies can be used to generate more specific mechanistic hypotheses, certain of which are prioritised in step (2) (based on certain additional assumptions—e.g., that there is a single cellular factor/mechanism responsible for pathogen-cell interaction in the above example), and which can then be validated in downstream analysis in step (3). For example, identification of candidate viral receptors using genome-wide loss-of-function screens can be used to generate specific hypotheses regarding the identity of the associated receptor, which can then be subject to empirical test.
Although broadly speaking we concur with Ratti on these matters (in addition to concurring with other philosophers who have written on this topic, e.g. Franklin ( 2005 ); Burian ( 2007 )), and find his work to deliver significant advances in our conceptual understanding of such large-scale studies, his citing of ‘genome-wide association studies’ (GWAS) as a means of illustrating the above points (see Ratti 2015 , p. 201) invites further consideration. GWAS aims to identify causal associations between genetic variations and diseases/traits; however, it encounters serious difficulties in identifying concrete hypotheses to prioritise, as per Ratti’s (2). Different solutions to this issue (and the related issue of GWAS ‘missing heritability’) manifest in different approaches to this prioritisation: something which deserves to be made explicit in the context of Ratti’s framework. Specifically, while Ratti focuses implicitly on a ‘core gene’ approach to GWAS (cf. Boyle et al. ( 2017 )), according to which a small number of ‘single nucleotide polymorphisms’ (this terminology will be explained in the body of this paper) are primarily responsible for the trait in question (note that this does not imply that only a small number of genes are associated with the relevant phenotype—rather, it assumes that there are some genes which are more central for the manifestation of the phenotype than the majority), there are other approaches to GWAS which do not presuppose this core gene model; as explained in Wray et al. ( 2018 ) (albeit without direct reference to Ratti’s work), such approaches would lead to the prioritisation of different hypotheses in Ratti’s (2). Footnote 1
The first goal of the present paper is to expand on these matters in full detail, and to revise Ratti’s framework in order to incorporate the above points: in so doing, we gain a clearer understanding of how GWAS approaches relate to more traditional, mechanistic, hypothesis-driven science. But there is also a second goal of this paper: to explore for the first time (to our knowledge) in the philosophical literature what it would take for the above-mentioned alternative approaches (often relying on network models)—particularly those which appeal to the field of (phospho)proteomics—to succeed. Although we make no claim that such (phospho)proteomics approaches are per se superior to other strategies for hypothesis prioritisation, they are nevertheless in our view worthy of philosophical attention unto themselves, for they constitute (we contend) a novel form of exploratory experimentation (cf. Burian ( 2007 ); Franklin ( 2005 ); Steinle ( 1997 )) featuring both iterativity (cf. Elliott ( 2012 ); O’Malley et al. ( 2010 )) and appeal to deep learning (cf. Bechtel ( 2019 ); Ratti ( 2020 )).
Bringing all this together, the plan for the paper is as follows. In Sect. " GWAS studies and prioritisation ", we recall the details of GWAS, and witness how different approaches to the so-called missing heritability and coherence problems lead to the prioritisation of different hypotheses in Ratti’s (2). In Sect. " Proteomics and iterative methodology ", we turn our attention to network approaches—specifically to those informed by (phospho)proteomics—and study these through the lens of the literature on exploratory experimentation, before returning to our considerations of GWAS and addressing the question of how such network-based approaches inform the question of hypothesis prioritisation in that context. We close with some discussion of future work to be done in the philosophy both of GWAS, and of big-data biology at large.
GWAS studies and prioritisation
Background on gwas.
Many applications of the framework presented in the introduction—perform genome-wide screens based on a general hypothesis (for example, ‘a gene/process is responsible for a disease’), and on the basis of the results obtained construct a more refined hypothesis for further testing—have been highly successful in biomedical research. However, there are cases in which the application of the approach has not been so straightforward. This can best be illustrated using the example of a field of genomics that studies common diseases such as inflammatory bowel disease (IBD), coronary artery disease, insomnia, and depression. These are often diseases complex in nature, and are thought to be controlled not by a single mutation, but rather to be influenced by multiple loci in the genome and even through the effect of the environment.
In the past decades, researchers have developed a method to characterise the genotype-phenotype associations in these diseases: the method is called ‘genome-wide association studies’ (GWAS). To understand this method, it is important to understand single nucleotide polymorphisms (SNPs). SNPs are variations in a single DNA building block, called a ‘nucleotide’, and they constitute the most common type of genetic variation among individuals. There are around 4-5 million SNPs in a person’s genome. Most SNPs have no effect on human health, but there are some cases in which these variations lead to increased chances of disease. GWAS was based originally upon a ‘common disease, common variant’ hypothesis, which states that common diseases can be attributed to common genetic variants (present in more than 1–5% of the population). By scanning the genomes of many different people, GWAS sought to identify the relationships between common genetic variations and common traits. GWAS studies remain very popular in the field of human genetics, and have been successful in identifying a number of novel variant-trait associations (for example, in diseases such as those mentioned above). For a clear introduction to GWAS from the biology literature, see Tam et al. ( 2019 ); for existing philosophical works on GWAS, with further details on such studies complimentary to those presented in this paper, see e.g. Bourrat ( 2020 ); Bourrat and Lu ( 2017 ).
GWAS’ discontents
GWAS is, however, not without its critics. A clear conclusion from multiple GWAS studies is that even statistically highly significant hits identified from such studies are able to account only for a small fraction of the heritability of the trait/disease in question. (Recall that ‘heritability’ is the measure of proportion of the phenotypic variance in a population that can be attributed to genetic differences—see Downes and Matthews ( 2020 ) and references therein for further details.) Moreover, GWAS studies often implicate large numbers of genes. To put this into perspective, three GWAS studies performed for height in 2008 identified 27, 12 and 20 associated genomic regions, which accounted merely for 3.7, 2.0, and 2.9% of the population variation in height, respectively ( Lettre et al. ( 2008 ); Weedon et al. ( 2008 ); Gudbjartsson et al. ( 2008 )). This was in sharp contrast with estimates from previous genetic epidemiology studies, based upon twin studies, Footnote 2 that estimated the heritability of height to be around 80% (Yang et al. ( 2010 )). In the early days of GWAS, this apparent discrepancy from GWAS came to be known as the missing heritability problem . For recent philosophical discussion of this problem, see Bourrat ( 2020 ); Bourrat and Lu ( 2017 ); Bourrat et al. ( 2017 ); Bourrat ( 2019 ); Downes and Matthews ( 2020 ); Matthews and Turkheimer ( 2019 ).
Geneticists have since proposed a number of solutions to the missing heritibility problem. The three most commonly-discussed such solutions are classified by Gibson ( 2012 ) as follows:
Complex dieseases are polygenic and many loci with small effects account for the phenotype variance.
Common diseases are caused by rare genetic variants each of which have large effect sizes.
Most common diseases are a result of interactions between many factors such as gene-gene interaction effects and effects from environmental factors.
(We take the proposals for solving the missing heritability problem presented in Bourrat ( 2020 ); Bourrat and Lu ( 2017 ); Bourrat et al. ( 2017 ); Bourrat ( 2019 ), which invoke factors from the epigenome, to fall into category (3); we discuss further these proposals in Sect. GWAS reprise .) From multiple GWAS studies on common diseases there is now overwhelming evidence that common diseases are polygenic, as large numbers of genes are often implicated for a given disease. However, using this framework, it is estimated that it would take 90,000–100,000 SNPs to explain 80% of the population variation in height. In light of this, Goldstein ( 2009 ) raised the concern with GWAS studies that “[i]n pointing at ‘everything’, the danger is that GWAS could point at ‘nothing”’.
It is understandable that one would find unpalatable its not being the case that a single gene or process can be associated with a particular disease. But the situation here is not as straightforward as the above remarks might suggest. Indeed, Boyle et al. ( 2017 ) propose the following refinement of this idea:
Intuitively, one might expect disease-causing variants to cluster into key pathways that drive disease etiology. But for complex traits, association signals tend to be spread across most of the genome—including near many genes without an obvious connection to disease. We propose that gene regulatory networks are sufficiently interconnected such that all genes expressed in disease-relevant cells are liable to affect the functions of core disease-related genes and that most heritability can be explained by effects on genes outside core pathways. We refer to this hypothesis as an ‘omnigenic’ model.
Boyle et al. ( 2017 ) propose that within the large number of implicated genes in GWAS, there are a few ‘core’ genes that play a direct role in disease biology; the large number of other genes identified are ‘peripheral’ and have no direct relevance to the specific disease but play a role in general regulatory cellular networks. By introducing their ‘omnigenic’ model, Boyle et al. ( 2017 ) acknowledge the empirical evidence that GWAS on complex diseases does in fact implicate large number of genes; they thereby seem to draw a distinction between complex diseases and classical Mendelian disorders, in which small number of highly deleterious variants drive the disease. However, their suggestion of the existence of a small number of ‘core’ genes backtracks on this and paints complex diseases in the same brushstrokes as classical Mendelian disorders. A number of authors have welcomed the suggestion that genes implicated for complex diseases play a role in regulatory networks but have found the dicotomy between core and peripheral genes to be an ill-motivated attempt to fit complex disease into what we intuitively think should be the framework of a disease (‘a small number of genes should be responsible for a given disease’). For example, Wray et al. ( 2018 ) write:
It seems to us to be a strong assumption that only a few genes have a core role in a common disease. Given the extent of biological robustness, we cannot exclude an etiology of many core genes, which in turn may become indistinguishable from a model of no core genes.
We concur with this verdict. One possible reconstruction of the reasons underlying the endorsement by Boyle et al. ( 2017 ) of ‘core’ versus ’peripheral’ genes could be in order to solve the missing heritability problem. These authors advocate for using experimental methods that are able to identify rare variants that have high effect sizes (solution (2) of the missing heritability problem as presented above), as this is where they suspect the ‘core’ genes can be identified. However, there is at present no evidence that the ‘core gene’ hypothesis need invariably be true for complex diseases (cf. Wray et al. ( 2018 )), so one might be inclined to reject the original hypothesis that all diseases must fit the mould of ‘small number of genes cause complex diseases’. In so doing, one would thereby need to embrace the claim that at least some complex diseases are polygenic and that putative ‘core’ genes are, in fact, no more important than putative ‘peripheral’ genes in this context.
This, however, still leaves us with the original issue that Boyle et al. ( 2017 ) were trying to address: how is it that genes which look disconnected are, in fact, together implicated in a given disease? In addressing this question, we again concur with Wray et al. ( 2018 ), who write:
To assume that a limited number of core genes are key to our understanding of common disease may underestimate the true biological complexity, which is better represented by systems genetics and network approaches.
That is to say, understanding gene functions and the interplay between the different genes is key to answering why many genes are involved in complex diseases. This is not a straightforward task and a full characterisation of the roles that genes play in biological systems remains a distant prospect.
One approach to addressing this issue is to identify relationships between genes in a cell by way of a systems biology approach, underlying premises of which are that cells are complex systems and that genetic units in cells rarely operate in isolation. Hence, on this view, understanding how genes relate to one another in a given context is key to establishing the true role of variants identified from GWAS hits. There are a number of approaches described in the field of systems biology to identify gene-gene relationships. One widely-implemented approach is to construct ‘regulatory networks’ relating these genes. A regulatory network is a set of genes, or parts of genes, that interact with each other to control a specific cell function. With recent advances in high-throughput transcriptomics, it is now possible to generate complex regulatory networks of how genes interact with each other in biological processes and define the roles of genes in a context-dependent manner based on mRNA expression in a cell. As the majority of GWAS hits often lie in non-coding regions of the genome, which are often involved in regulating gene expressions, networks based on mRNA expression are powerful means to interpret of the functional role of variants identified by GWAS.
Another approach to the functional validation of GWAS hits—currently substantially less common—proceeds by constructing networks generated from expression of proteins/phosphoproteins in a cell (more details of these approaches will be provided in the following section). Such approaches would in principle depict completely the underlying state of the cell. Combined with gene expression data, protein expression networks and signalling networks from proteomics would make transparent the functional role of the variants identified in GWAS studies in a given context—that is, they would provide a mechanistic account of disease pathogenesis without recourse to a neo-Mendelian ‘core gene’ model. Genes which prima facie appear disconnected and irrelevant to disease biology may be revealed by these approaches to be relevant after all. To illustrate, consider a complex disease such as IBD: it is thought that both (i) a disturbed interaction between the gut and the intestinal microbiota, and (ii) an over-reaction of the immune system, are required for this disease phenotype to manifest. Thus, it is likely that a number of genetic pathways will be important—pathways which need not prima facie be connected, but which may ultimately be discovered to be related in some deeper way. These proteomics-informed network approaches would thereby afford one resolution to what has been dubbed by Reimers et al. ( 2019 ) and Craver et al. ( 2020 ) the ‘coherence problem’ of GWAS: to explain how it is that all genes implicated in these studies are related to one another mechanistically. Footnote 3 Clearly, these approaches could be brought to bear in order to vindicate responses (1) or (3) to the missing heritability problem, presented above. Footnote 4
To close this subsection, it is worth reflecting on how the ‘core gene’ hypothesis might intersect with network-based approaches. If a core gene exists, then a network analysis should (at least in principle) be able to identify it; in this sense, a ‘core gene’ hypothesis can be compatible with a network approach. As already mentioned above, however, there is no evidence that such core genes invariably exist: a network analysis could (in principle) identify many ‘central hubs’, rather than just one—an outcome not obviously compatible with the ‘core gene’ hypothesis. (For more on this latter possibility, cf. the very recent work of Barrio-Hernandez et al. ( 2021 ), discussed further below.)
Ratti’s framework for large-scale studies
Suppose that one follows (our reconstruction of) Boyle et al. ( 2017 ), in embracing option (2) presented above as a solution to the GWAS missing heritability problem. One will thereby, in Ratti’s second step in his three-step programme characterising these data-driven approaches to biology, prioritise hypotheses according to which a few rare genes are responsible for the disease in question. This, indeed, is what Ratti ( 2015 ) suggests in §2.2 of his article. However, one might question whether this prioritisation is warranted, in light of the lack of direct empirical evidence for this neo-Mendelian hypothesis (as already discussed). Wray et al. ( 2018 ), for example, write that
... [t]o bias experimental design towards a hypothesis based upon a critical assumption that only a few genes play key roles in complex disease would be putting all eggs in one basket.
If one concurs with Wray et al. ( 2018 ) on this matter (as, indeed, we do), then one may prioritise different hypotheses in the second step of Ratti’s programme—in particular, one may prioritise specific hypotheses associated with ‘polygenic’ models which would constitute approach (1) and/or approach (3) to the missing heritability problem.
This latter point should be expanded. Even if one does embrace a ‘polygenic’ approach to the missing heritability problem (i.e., approach (1) and/or approach (3)), and applies e.g. networks (whether transcriptomics-based, or (phospho)proteomics-informed, or otherwise—nothing hinges on this for our purposes here) in order to model the genetic factors responsible for disease pathogenesis, ultimately one must prioritise specific hypotheses for laboratory test. For example, Schwartzentruber et al. ( 2021 ) implement in parallel a range of network models within the framework of a polygenic approach in order to prioritise genes such as TSPAN14 and ADAM10 in studies on Alzheimer’s disease (we discuss further the methodology of Schwartzentruber et al. ( 2021 ) in §3.3 ). Note, however, that these specific hypotheses might be selected for a range of reasons—e.g., our prior knowledge of the entities involved, or ease of testability, or even financial considerations—and that making such prioritisations emphatically does not imply that one is making implicit appeal to a ‘core gene’ model. This point is corroborated further by the fact that the above two genes are not the most statistically significant hits in the studies undertaken by Schwartzentruber et al. ( 2021 ), as one might expect from those working within the ‘core gene’ framework.
Returning to Ratti’s framework: we take our noting this plurality of options vis-à-vis hypothesis prioritisation to constitute a friendly modification to this framework appropriate to contexts such as that of GWAS. But of course, if one were to leave things here, questions would remain—for it would remain unclear which polygenic model of disease pathogenesis is to be preferred, and how such models are generated. Given this, it is now incumbent upon us to consider in more detail how such approaches set about achieving these tasks in practice: due both to their potential to offer underlying mechanistic models of the cell, as well as due to the novel iterative methodology for hypothesis generation involved, we focus largely in the remainder upon (phospho)proteomics-based approaches.
Proteomics and iterative methodology
Proteomics promises to afford the ultimate fundamental mechanistic account of cellular processes; data from proteomics would, therefore, illuminate the underlying relationships between the variants identified in GWAS studies. In this section, we explore in greater detail how such proteomics approaches proceed; they constitute a novel form of ‘exploratory experimentation’ (in the terminology of Burian ( 2007 ); Steinle ( 1997 )) worthy unto themselves of exposure in the philosophical literature. Footnote 5 In proteomics, further complications for hypothesis generation and testing arise, for data is sparse, and experiments often prohibitively expensive to perform. Given these constraints, how is progress to be made? It is to this question which we now turn; the structure of the section is as follows. In Sect. Proteomics: a data-deprived field , we present relevant background regarding proteomics. Then, in Sect. Methodological iteration , we argue that the development of this field can be understood on a model of a novel form of iterative methodology (cf. Chang 2004 ; O’Malley et al. 2010 ). We return to the relevance of these approaches for GWAS in Sect. GWAS reprise .
Proteomics: a data-deprived field
The ultimate aim of -omics studies is to understand the cell qua biological system. Transcriptomics is now sufficiently well-advanced to accommodate large-scale systematic studies to the point of being used to validate variants identified from GWAS. Footnote 6 By contrast, proteomics—the study of proteins in a cell—remains significantly under-studied. Technologies allowing for the systematic study of proteins are not as advanced as those for studying genes and transcripts; this is mainly because no method currently exists for directly amplifying proteins (i.e., increasing the amount of a desired protein in a controlled laboratory context): a methodology which has been key for genomics and transcriptomics. Proteins are very diverse in the cell: a single gene/transcript gives rise to multiple proteins. Proteins themselves can be modified in the cell after being created, thus further increasing the complexity of proteomics studies. Unlike genomics and transcriptomics, in which it is now common to perform systematic genome-wide or transcriptome-wide approaches, studies of proteins are therefore usually taken piecemeal.
Proteomics research tends to focus on families of proteins that are involved in a particular known biological process. Among the important families of proteins are kinases and phosphatases, which are molecules that are responsible for signal transmission in the cell. These proteins are able to modify other proteins by adding or removing a phosphate group (respectively). This modification changes the shape (‘conformation’) of the protein, rendering it active or inactive, Footnote 7 depending on the context. By examining the phopsphorylation state of the proteins inside a cell, it is possible to infer the signalling state of that cell. The field of phosphoproteomics aims to characterise all phospho-modified proteins within a cell. This is thought to be one of the most powerful and fundamental ways of inferring the signalling process within a cell; the approach could add a substantial new layer to our understanding of both basic and disease biology. That said, a recent estimate suggests that current approaches have identified kinases for less than 5% of the phosphoproteome. What is even more staggering is that almost 90% of the phosphorylation modifications that have been identified have been attributed to only 20% of kinases. The other 80% of the kinases are completely dark: their functions remain unknown. For many such kinases, we do not even know where in the cell they are located. (See Needham et al. ( 2019 ) for a review of the current state of play in phosphoproteomics.)
In such a field, systematic studies to quantify the entire phosphoproteome in a cell and an ability to assign a kinase to every phosphorylated component would be the ultimate aim. But phosphoproteomics studies themselves are currently extremely expensive, and there are technological limitations in mapping the global phosphoproteome—not least sparsity of data, which often comes as a result of limitations in the technical setup of laboratory measurements and experiments. For example: the same sample measured in the same machine at two different instances will give readings for different phosphoproteins. Some statistical methods can be used to overcome these limitations, but these require making assumptions regarding the underlying biology, which defeats the point of an unbiased study.
In spite of these difficulties, it has been shown that if one combines multiple large-scale phosphoprotemics data sets (each admittedly incomplete), it is possible to predict kinase-kinase regulatory relationships in a cell using data-driven phosphoprotein signalling networks obtained via supervised machine learning approaches (a recent study from Invergo et al. 2020 showcases one such approach; we will use this as a running example in the ensuing). Footnote 8 First, a training set of data is used to teach a machine a classification algorithm. Once the classification algorithm is learnt, the machine is set to the task of applying it to unlabelled data: in our case, the goal is to identify further, as-yet unknown, regulatory protein relationships or non-relationships. (On machine learning and network analysis of biological systems, see also Bechtel ( 2019 ) and Ratti ( 2020 ).)
Before assessing such phosphoproteomics machine learning algorithms as that of Invergo et al. ( 2020 ), there are two further complications with the current state of play in proteomics which need to be mentioned. First: it is much easier to curate positive lists of interactions than negative lists. (This is essentially a case of its being easier to confirm existentially quantified statements than universally quantifies statements: for how can we ever truly ascertain that any two given proteins never interact?) Thus, at present, negative lists obtained from laboratory experiments are underpopulated. Invergo et al. ( 2020 ) attempt to circumvent this issue in the following way: they assume that regulatory relationships are rare, so that if one were to randomly sample protein associations, one could create reliably large artificial negative sets; indeed, they do generate artificial negative sets in exactly this way. (Clearly, this means that these approaches again cannot be understood as being ‘hypothesis-free’: cf. Sect. Introduction .)
The second problem with the current state of play in proteomics is this: when a given interaction occurs is a function of multifarious factors, most notably cell context. This context-dependence means that an entry in a negative set in one context might, in fact, be an entry in a positive set in another. To illustrate: in the case of regulatory relationships between two kinases, it is known that such relationships can be prone to dysregulation in diseases such as cancer. Hence, a well-annotated positive set relationship can very well be dysregulated in a cancer context, so that this relationship no longer exists, effectively putting it into a negative set. The problem is that many data-driven approaches rely on data that are generated in simple reductionist systems such as cancer cell lines—so that the results obtained might not carry across to the target physiological context. (Cancer cell lines can grow infinitely, and thus are ideal for experiments.) The approach taken by Invergo et al. ( 2020 ) utilises data from breast cancer cell lines; hence, the relationships they predict could be specific to a dysregulated system. In response to this second problem, we suggest replying on behalf of Invergo et al. ( 2020 ) that most regulatory relationships fundamental to the functioning of the cell should hold true in most contexts. At present, however, given the data-deprived nature of proteomics, there is little direct evidence for this hypothesis. (Again, the appeal to any such hypothesis would mean that such proteomics approaches cannot be ‘hypothesis-free’.)
Thus, the fact that Invergo et al. ( 2020 ) utilise data from breast cancer cell lines raises the possibility that their machine learning algorithms might be trained on data unsuited to other contexts, leading to concerns regarding error propagation. This general concern regarding the context-specificity (or lack thereof) of input data sets is, however, recognised by authors in the field—for example, Barrio-Hernandez et al. ( 2021 ) note that “improvements in mapping coverage and computational or experimental approaches to derive tissue or cell type specific networks could have a large impact on future effectiveness of network expansion” (Barrio-Hernandez et al. 2021 , p. 14).
Methodological iteration
In spite of these problems, Invergo et al. ( 2020 ) argue that the results obtained from their approach afford a useful means of bootstrapping further progress in phosphoproteomics. As they put it:
Although we do not suggest that these predictions can replace established methods for confirming regulatory relationships, they can nevertheless be used to reduce the vast space of possible relationships under consideration in order to form credible hypotheses and to prioritize experiments, particularly for understudied kinases. (Invergo et al. 2020 , p. 393)
One way to take this point is the following. Ideally, in order to construct positive and negative sets, one would test in the laboratory each individual protein association. Practically, however, this would be an unrealistic undertaking, as we have already seen. What can be done instead is this:
Generate a global phosphoproteomics data set, albeit one that is incomplete and sparse (e.g., that presented in Wilkes et al. ( 2015 )), based upon laboratory experiments.
Train, using this data set and input background hypotheses of the kind discussed above, a machine learning algorithm (such as that presented in Invergo et al. ( 2020 )) to identify candidate interactions in the unknown space of protein-protein interactions. Footnote 9
Use these results to guide further laboratory experimentation, leading to the development of more complete data sets.
Train one’s machine learning algorithms on these new data sets, to improve performance; in turn, repeat further the above process.
Clearly, a process of reflective equilibrium is at play here (cf. Daniels ( 2016 )). As is well-known, Chang ( 2004 ) has proposed an iterative conception of scientific methodology, according to which the accrual of scientific hypotheses is not a linear matter; rather, initial data may lead to the construction of a theoretical edifice which leads one to develop new experiments to revise one’s data; at which point, the process iterates. This fits well with the above-described procedures deployed in phosphoproteomics; it also accords with previous registration of the role of iterative procedures in large-scale biological studies—see e.g. O’Malley et al. ( 2010 ) and Elliott ( 2012 ).
Let us delve into this a little deeper. As Chang notes,
There are two modes of progress enabled by iteration: enrichment , in which the initially affirmed system is not negated but refined, resulting in the enhancement of some of its epistemic virtues; and self-correction , in which the initially affirmed system is actually altered in its content as a result of inquiry based on itself. (Chang 2004 , p. 228)
Certainly and uncontroversially, enrichment occurs in the above four-step process in phosophoproteomics: the new data yield a refinement of our previous hypotheses in the field. In addition, however, it is plausible to understand the above iterative methodology as involving self-correction: for example, in might be that the machine learning algorithm of Invergo et al. ( 2020 ) identifies a false positive, yet nevertheless makes sufficiently focused novel predictions with respect to other candidate interactions in order to drive new experimentation, leading to a new data set on which the algorithm can be trained, such that, ultimately, the refined algorithm does not make a false positive prediction for that particular interaction. This is entirely possible in the above iterative programme; thus, we maintain that both modes of Changian iterative methodology are at play in this approach.
There is another distinction which is also relevant here: that drawn by Elliott ( 2012 ) between ‘epistemic iteration’—“a process by which scientific knowledge claims are progressively altered and refined via self-correction or enrichment”—and ‘methodological iteration’—“a process by which scientists move repetitively back and forth between different modes of research practice” (Elliott 2012 , p. 378). It should be transparent from our above discussion that epistemic iteration is involved in these proteomics approaches. Equally, though, it should be clear that methodological iteration is involved, for the approach alternates between machine learning and more traditional laboratory experimentation. That machine learning can play a role in an iterative methodology does not seem to have been noted previously in the philosophical literature—for example, it is not identified by Elliott ( 2012 ) as a potential element of a methodologically iterative approach; on the other hand, although the role of machine learning in network modelling and large-scale studies is acknowledged by Bechtel ( 2019 ) and Ratti ( 2020 ) (the latter of whom also discusses—albeit without explicitly using this terminology—the role of machine learning in epistemic iteration: see (Ratti 2020 , p. 89)), there is no mention of its role in an iterative methodology such as that described above.
GWAS reprise
Given the foregoing, we hope it is reasonable to state that the approaches to proteomics of e.g. Invergo et al. ( 2020 ) constitute novels forms of exploratory experimentation, worthy of study in their own right. Let us, however, return now to the matter of polygenic approaches to GWAS hits. In principle, the results of the methodologies of e.g. Invergo et al. ( 2020 ) could further vindicate these approaches, by providing mechanistic models of which genes interact in a disease context, and when and why they do so. In turn, they have the capacity to allow biologists to prioritise specific hypotheses in Ratti’s step (2), without falling back upon assumptions that only few genes are directly involved in complex disease biology.
Note that that there is a complex interplay between this iterative methodology and the ‘eliminative induction’ of stages (1) and (2) Ratti’s analysis (see Sect. Introduction ; for earlier sources on eliminative induction, see Earman ( 1992 ); Kitcher ( 1993 ); Norton ( 1995 )). We take this to consist in the following. First, a methodology such as that of Invergo et al. ( 2020 ) is used to generate a particular network-based model for the factors which are taken to underlie a particular phenotype. This model is used to prioritise ( à la eliminative induction) particular hypotheses, as per stage (2) of Ratti’s framework; these are then subject to specific test, as per stage (3) of Ratti’s framework. The data obtained from such more traditional experimentation is then used to construct more sophisticated network models within the framework of Invergo et al. ( 2020 ); these in turn lead to the (eliminative inductive) prioritisation of further specific hypotheses amenable to specific test. As already discussed above, this is a clear example of the ‘methodological iteration’ of Elliott ( 2012 ).
It bears stressing that (phospho)proteomics network-based approaches may, ultimately, constitute only one piece of the solution to the broader puzzle that is GWAS hypothesis prioritisation. In very recent work, Schwartzentruber et al. ( 2021 ) have brought to bear upon this problem consideration of, inter alia , epigenomic factors alongside network-based analyses. There are two salient points to be made on this work. First: although Bourrat et al. ( 2017 ) are correct that epigenomic studies and background may have a role to play in addressing the missing heritability problem (cf. Bourrat ( 2019 , 2020 ); Bourrat and Lu ( 2017 )), a view in contemporary large-scale biological studies—evident in papers such as Schwartzentruber et al. ( 2021 )—is that these considerations can be supplemented with yet other resources, such as network-based studies; we concur with this verdict. Second: in order to construct these networks, Schwartzentruber et al. ( 2021 ) rely on established protein-protein interaction databases such as STRING, IntAct and BioGRID (Schwartzentruber et al. 2021 , p. 397). While effective in their own right, networks developed from such databases have the disadvantage that they represent signalling in an ‘average’ cell, and are therefore unsuitable for studying dynamic context- and cell-type-specific signalling responses (cf. Sharma and Petsalaki ( 2019 )). In this regard, it would (at least in principle) be preferable to utilise regulatory and context-specific networks developed using methods described in work such as that of Invergo et al. ( 2020 ) in future approaches to GWAS hypothesis prioritisation. That being said, in practice this may not yet be fruitful, as at present contemporary large-scale biology is only at the early stages of the iterative processes discussed above; moreover, the training data sets used by such methods remain at this stage not completely context-specific (recall that Invergo et al. ( 2020 ) utilise a breast cancer training set)—meaning that the potential of such work to yield detailed, context-specific network-based models is yet to be realised in full.
With all of the above in hand, we close this subsection by considering more precisely the question of how the machine learning algorithms of Invergo et al. ( 2020 ) bear upon the missing heritability problem. Having developed regulatory protein-protein interaction networks on the basis of such algorithms, one can take (following here for the sake of concreteness the lead of Barrio-Hernandez et al. ( 2021 )) the connection with hypothesis prioritisation in GWAS (and, in turn, the missing heritability problem) to proceed via the following steps (also summarised visually in Fig. 1 ):
Select a protein-protein interaction network. Usually, this is a pre-existing curated network, such as those defined in the STRING database (discussed above). However, instead of such curated networks, use in their place networks developed on the machine learning models of e.g. Invergo et al. ( 2020 ).
Within those networks, identify the nodes (i.e., proteins) which correspond to hits from a particular GWAS (i.e., the proteins associated with the genes identified in the GWAS). Footnote 10
Use network propagation methods (see e.g. Cowen et al. ( 2017 ) for a review of such methods), potentially alongside other factors (as discussed in e.g. Schwartzentruber et al. ( 2021 )) in order to identify known modules (i.e., separated substructures within a network) associated with the disease in question.
Target elements of those modules, regardless of whether or not they were hits in the original GWAS. (This latter approach—of targeting beyond the original GWAS hits—is novel to the very recent work of Barrio-Hernandez et al. ( 2021 ).)
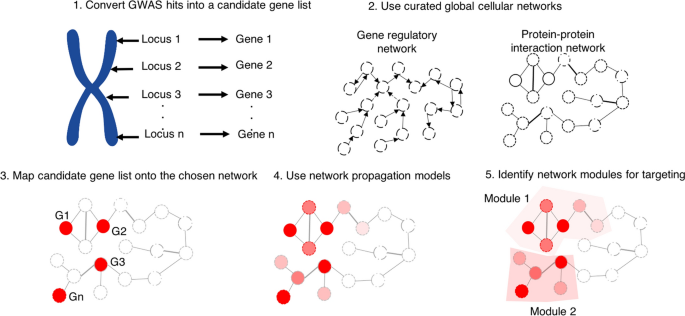
The application of networks to GWAS hit prioritisation. In (1), GWAS hits are converted to candidate gene lists. In (2), one selects a cellular network: this could be a gene regulatory network, or a protein-protein interaction network (e.g. from STRING), or a protein-protein regulatory network (possibly constructed via the machine learning methodologies of Invergo et al. ( 2020 )). In (3), genes associated with the GWAS loci are mapped to the chosen network. In (4), network propagation methods (e.g. diffusion techniques) are applied in order identify potential disease-related genes not picked up by the GWAS. In (5), the results of these network analyses are used to identify significant genetic modules to be targeted experimentally in investigations into disease pathogenesis. Note, following Wray et al. ( 2018 ) and Barrio-Hernandez et al. ( 2021 ), that this particular means of bridging the gap between cellular networks and investigations into the results of GWAS hits does not presuppose a ‘core gene’ hypothesis
On (2) and (3): Boyle et al. ( 2017 ) may or may not be correct that many genes are implicated (either in the original screen, or after the network analysis has been undertaken)—recall from Sect. GWAS’ discontents their ‘omnigenic’ model. However, on the basis of the work of Barrio-Hernandez et al. ( 2021 ) one might argue that this is not the most important question—rather, the important question is this: which gene modules provide insights into the disease mechanism? One can ask this question without subscribing to a ‘core gene’ model; thus, we take the work of Barrio-Hernandez et al. ( 2021 ) to be consistent with the above-discussed points raised by Wray et al. ( 2018 ).
This paper has had two goals. The first has been to propose revisions to the framework of Ratti ( 2015 ) for the study of the role of hypothesis-driven research in large-scale contemporary biological studies, in light of studies such as GWAS and its associated missing heritability problem. In this regard, we have seen that different hypotheses may be prioritised, depending upon whether one adopts a ‘core’ gene model (as Ratti ( 2015 ) assumes, and as is also advocated in Boyle et al. ( 2017 )), or whether one adopts a polygenic model (as endorsed by Wray et al. ( 2018 ); cf. Barrio-Hernandez et al. ( 2021 )). The second goal of this paper has been to consider how these hypotheses would be developed on polygenic approaches via (phospho)proteomics—which itself constitutes a novel form of exploratory experiment, featuring as it does both iterativity and deep learning—and to consider what it would take for these network-based proteomics approaches to succeed. A broader upshot of this paper has been the exposure for the first time to the philosophical literature of proteomics: given its potential to provide mechanistic models associated with disease phenotypes, the significance of this field cannot be overstated.
The issues discussed in this paper raise important questions regarding how researchers prioritise not just first-order hypotheses as per Ratti’s (2), but also the background assumptions which allow one to make such adjudications to begin with. To be concrete: in the case of GWAS, should one prioritise the assumption that rare variants of large effect in a small number of genes drive complex diseases, or rather invest in developing systems-based approaches and in improving under-studied fields, such as (phospho)proteomics, which may or may not ultimately shed light on the question of why complex diseases have thus far manifested empirically as polygenic? These choices lead to different first-order prioritisations in Ratti’s second step, and thereby have great potential to steer the course of large-scale studies in future years. Given limited resources in the field, it is, in our view, worth pausing to reflect on whether said resources are appropriately allocated between these options, and to strive to avoid any status quo bias in favour of currently-popular assumptions. Footnote 11
In fairness to Ratti, in other articles, e.g. López-Rubio and Ratti ( 2021 ), he does not make assumptions tantamount to a ‘core gene’ hypothesis; in this sense, our criticism falls most squarely on assumptions made in Ratti ( 2015 ).
Twin studies are powerful approaches to studying the genetics of complex traits. In simple terms, twin studies compare the phenotypic similarity of identical (monozygotic) twins to non-identical (dizygotic) twins. As monozygotic twins are genetically identical and non-identical twins are on average ‘half identical’, observing greater similarity of identical over non-identical twins can be used as an evidence to estimate the contribution of genetic variation to trait manifestation. For further discussion of twin studies in the philosophical literature, see e.g. Matthews and Turkheimer ( 2019 ); Downes and Matthews ( 2020 ).
There are many further questions to be addressed here in connection with the literature of mechanisms and mechanistic explanations. For example, are these network approaches best understood as revealing specific mechanisms, or rather as revealing mechanism schema (to use the terminology of (Craver and Darden 2013 , ch.3))? Although interesting and worthy of pursuit, for simplicity we set such questions aside in this paper, and simply speak of certain contemporary biology approaches as revealing ‘underlying mechanisms’. In this regard, we follow the lead of Ratti ( 2015 ).
To be completely clear: we do not claim that these (phospho)proteomics-based network approaches are superior to regulatory network approaches, given the current state of technology in the field. On the contrary—as we explain in Sect. Proteomics and iterative methodology —the former of these fields is very much nascent, and has yet to yield significant predictive or explanatory fruit. Nevertheless—again as we explain in Srct. Proteomics and iterative methodology —in our view these approaches are worthy of exposure in the philosophical literature in their own right, for (a) they offer one of the most promising means (in principle, if not yet in practice) of providing a mechanistic account of disease pathogenesis, and (b) the particular way in which hypotheses are developed and prioritised on these approaches is conceptually rich.
Recall: “Experiments count as exploratory when the concepts or categories in terms of which results should be understood are not obvious, the experimental methods and instruments for answering the questions are uncertain, or it is necessary first to establish relevant factual correlations in order to characterize the phenomena of a domain and the regularities that require (perhaps causal) explanation” (Burian 2013 ). Cf. e.g. Franklin ( 2005 ); Steinle ( 1997 ). All of the -omics approaches discussed in this paper were identified in Burian ( 2007 ) as cases of exploratory experimentation; the details of contemporary proteomics approaches have, however, not been presented in the philosophical literature up to this point (at least to our knowledge).
In this paper, we do not go into the details of specific transcriptomics studies. One interesting approach worthy of mention, however, is ‘single-cell RNA sequencing’ (SC-RNA), which allows biologists to assay the full transcriptome of hundreds of cells in an unbiased manner (see e.g. Hwang et al. ( 2018 ) for a recent review). The advantage of SC-RNA over older methods lies in its ability to identify the transcriptomes from heterocellular and poorly-classified tissue populations and disease-associated cell states.
As the addition or removal of phosphate groups regulates the activity of a protein, such relationships between a kinase and its target (also called a ‘substrate’) are referred to as ‘regulatory relationships’. Kinases themselves can also be phosphorylated by other kinases, so there exist also kinase-kinase regulatory relationships in a cell.
Supervised machine learning involves training a machine on a given data set (for example, a collection of cat photos versus dog photos), before assigning the machine the task of classifying entries in some new data set. By contrast, in unsupervised learning, the machine is instructed to find its own patterns in a given data set. For some recent philosophical considerations regarding machine learning, see Sullivan ( 2019 ).
One can also test the results of the machine binary classification algorithm on other data sets: this Invergo et al. ( 2020 ) did with reference to the data presented in Hijazi et al. ( 2020 ). The design of the algorithmic system and algorithm used by Invergo et al. ( 2020 ) is described with admirable clarity at (Invergo et al. 2020 , pp. e5ff.), to which the reader is referred for further details.
Note that identification of candidate genes from the loci which constitute GWAS hits is non-trivial. The recently-described ‘locus-to-gene’ (L2G) approach is a machine learning tool which can be used to prioritise likely causal genes at each locus given genetic and functional genomics features (see Mountjoy et al. ( 2020 )).
Cf. Samuelson and Zeckhauser ( 1988 ). For related discussion of funding decisions in the context of -omics studies, see Burian ( 2007 ).
Barrio-Hernandez I, Schwartzentruber J, Shrivastava A, del Toro N, Zhang Q, Bradley G, Hermjakob H, Orchard S, Dunham I, Anderson CA, Porras P, Beltrao P (2021) ‘Network expansion of genetic associations defines a pleiotropy map of human cell biology’, bioRxiv . https://www.biorxiv.org/content/early/2021/07/19/2021.07.19.452924
Bechtel W (2019) Hierarchy and levels: analysing networks to study mechanisms in molecular biology. Philos Transact R Soc B 375(20190320):20190320
Google Scholar
Bourrat P (2019) Evolutionary transitions in heritability and individuality. Theory Biosci 138:305–323
Article Google Scholar
Bourrat P (2020) Causation and single nucleotide polymorphism heritability. Philos Sci 87:1073–1083
Bourrat P, Lu Q (2017) Dissolving the Missing Heritability Problem. Philosophy of Science 84:1055–1067
Bourrat P, Lu Q, Jablonka E (2017) Why the missing heritability might not be in the DNA. BioEssays 39:1700067
Boyle E, Li Y, Pritchard J (2017) An expanded view of complex traits: from polygenic to omnigenic. Cell 169:1177–1186
Burian R (2013) Exploratory experimentation. In: Dubitzky W, Wolkenhauer O, Cho K-H, Yokota H (eds) Encyclopedia of systems biology. Springer, Berlin
Burian RM (2007) On MicroRNA and the need for exploratory experimentation in post-genomic molecular biology. Hist Philos Life Sci. 29(3):285–311. http://www.jstor.org/stable/23334263
Chang H (2004) Inventing temperature: measurement and scientific progress. Oxford University Press, Oxford
Book Google Scholar
Cowen L, Ideker T, Raphael BJ, Sharan R (2017) Network propagation: a universal amplifier of genetic associations. Nat Rev Genet 18(9):551–562. https://doi.org/10.1038/nrg.2017.38
Craver CF, Darden L (2013) In search of mechanisms. University of Chicago Press, Chicago
Craver CF, Dozmorov M, Reimers M, Kendler KS (2020) Gloomy prospects and roller coasters: finding coherence in genome-wide association studies. Philos Sci 87(5):1084–1095
Daniels N (2016) Reflective equilibrium. The Stanford Encyclopedia of Philosophy
Doudna JA, Charpentier E (2014) The new frontier of genome engineering with CRISPR-Cas9. Science 346(6213):1258096
Downes SM, Matthews L (2020) Heritability. In: Zalta EN (ed) The Stanford encyclopedia of philosophy. Stanford University, Metaphysics Research Lab
Earman J (1992) Bayes or bust? a critical examination of BayesianConfirmation Theory,. MIT Press, Cambridge
Elliott KC (2012) Epistemic and methodological iteration in scientific research. Stud Hist Philos Sci Part A 43(2):376–382
Franklin L (2005) Exploratory experiments. Philos Sci. 72(5):888–899. https://www.jstor.org/stable/10.1086/508117
Gibson G (2012) Rare and common variants: twenty arguments. Nat Rev Genet 13(2):135–145
Goldstein D (2009) Common genetic variation and human traits. N Engl J Med 360:1696–1698
Gudbjartsson DF, Walters GB, Thorleifsson G, Stefansson H, Halldorsson BV, Zusmanovich P, Sulem P, Thorlacius S, Gylfason A, Steinberg S, Helgadottir A, Ingason A, Steinthorsdottir V, Olafsdottir EJ, Olafsdottir GH, Jonsson T, Borch-Johnsen K, Hansen T, Andersen G, Jorgensen T, Pedersen O, Aben KK, Witjes JA, Swinkels DW, Heijer Md, Franke B, Verbeek ALM, Becker DM, Yanek LR, Becker LC, Tryggvadottir L, Rafnar T, Gulcher J, Kiemeney LA, Kong A, Thorsteinsdottir U, Stefansson K (2008) Many sequence variants affecting diversity of adult human height. Nat Genet 40(5):609–615. https://doi.org/10.1038/ng.122
Hasin Y, Seldin M, Lusis A (2017) Multi-omics approaches to disease. Genome Biol 18(1):83
Hijazi M, Smith R, Rajeeve V, Bessant C, Cutillas PR (2020) Reconstructing kinase network topologies from phosphoproteomics data reveals cancer-associated rewiring. Nat Biotechnol 38(4):493–502
Hwang B, Lee JH, Bang D (2018) Single-cell RNA sequencing technologies and bioinformatics pipelines. Exp Mol Med 50(8):96. https://doi.org/10.1038/s12276-018-0071-8
Invergo BM, Petursson B, Akhtar N, Bradley D, Giudice G, Hijazi M, Cutillas P, Petsalaki E, Beltrao P (2020) Prediction of signed protein kinase regulatory circuits. Cell Syst 10(5):384-396.e9
Kitcher PS (1993) The advancement of science. Oxford University Press, Oxford
Lander ES, Linton LM, Birren B, Nusbaum C, Zody MC, Baldwin J, Devon K, Dewar K, Doyle M, FitzHugh W, Funke R, Gage D, Harris K, Heaford A, Howland J, Kann L, Lehoczky J, LeVine R, McEwan P, McKernan K, Meldrim J, Mesirov JP, Miranda C, Morris W, Naylor J, Raymond C, Rosetti M, Santos R, Sheridan A, Sougnez C, Stange-Thomann Y, Stojanovic N, Subramanian A, Wyman D, Rogers J, Sulston J, Ainscough R, Beck S, Bentley D, Burton J, Clee C, Carter N, Coulson A, Deadman R, Deloukas P, Dunham A, Dunham I, Durbin R, French L, Grafham D, Gregory S, Hubbard T, Humphray S, Hunt A, Jones M, Lloyd C, McMurray A, Matthews L, Mercer S, Milne S, Mullikin JC, Mungall A, Plumb R, Ross M, Shownkeen R, Sims S, Waterston RH, Wilson RK, Hillier LW, McPherson JD, Marra MA, Mardis ER, Fulton LA, Chinwalla AT, Pepin KH, Gish WR, Chissoe SL, Wendl MC, Delehaunty KD, Miner TL, Delehaunty A, Kramer JB, Cook LL, Fulton RS, Johnson DL, Minx PJ, Clifton SW, Hawkins T, Branscomb E, Predki P, Richardson P, Wenning S, Slezak T, Doggett N, Cheng JF, Olsen A, Lucas S, Elkin C, Uberbacher E, Frazier M, Gibbs RA, Muzny DM, Scherer SE, Bouck JB, Sodergren EJ, Worley KC, Rives CM, Gorrell JH, Metzker ML, Naylor SL, Kucherlapati RS, Nelson DL, Weinstock GM, Sakaki Y, Fujiyama A, Hattori M, Yada T, Toyoda A, Itoh T, Kawagoe C, Watanabe H, Totoki Y, Taylor T, Weissenbach J, Heilig R, Saurin W, Artiguenave F, Brottier P, Bruls T, Pelletier E, Robert C, Wincker P, Smith DR, Doucette-Stamm L, Rubenfield M, Weinstock K, Lee HM, Dubois J, Rosenthal A, Platzer M, Nyakatura G, Taudien S, Rump A, Yang H, Yu J, Wang J, Huang G, Gu J, Hood L, Rowen L, Madan A, Qin S, Davis RW, Federspiel NA, Abola AP, Proctor MJ, Myers RM, Schmutz J, Dickson M, Grimwood J, Cox DR, Olson MV, Kaul R, Raymond C, Shimizu N, Kawasaki K, Minoshima S, Evans GA, Athanasiou M, Schultz R, Roe BA, Chen F, Pan H, Ramser J, Lehrach H, Reinhardt R, McCombie WR, de la Bastide M, Dedhia N, Blöcker H, Hornischer K, Nordsiek G, Agarwala R, Aravind L, Bailey JA, Bateman A, Batzoglou S, Birney E, Bork P, Brown DG, Burge CB, Cerutti L, Chen HC, Church D, Clamp M, Copley RR, Doerks T, Eddy SR, Eichler EE, Furey TS, Galagan J, Gilbert JG, Harmon C, Hayashizaki Y, Haussler D, Hermjakob H, Hokamp K, Jang W, Johnson LS, Jones TA, Kasif S, Kaspryzk A, Kennedy S, Kent WJ, Kitts, P, Koonin, E V, Korf I, Kulp, D, Lancet D, Lowe T M, McLysaght A, Mikkelsen T, Moran J V, Mulder N, Pollara V J, Ponting C P, Schuler G, Schultz J, Slater G, Smit A F, Stupka E, Szustakowki J, Thierry-Mieg D, Thierry-Mieg J, Wagner L, Wallis J, Wheeler R, Williams A, Wolf Y I, Wolfe, K H, Yang S P, Yeh R F, Collins F, Guyer M S, Peterson J, Felsenfeld A, Wetterstrand K A, Patrinos A, Morgan M J, de Jong P, Catanese J J, Osoegawa K, Shizuya H, Choi S, Chen Y J, Szustakowki J, and International Human Genome Sequencing Consortium (2001) Initial sequencing and analysis of the human genome. Nature 409(6822):860–921
Leonelli S (2016) Data-centric biology: a philosophical study. University of Chicago Press, Chicago
Lettre G, Jackson A. U., Gieger C., Schumacher F. R., Berndt S. I., Sanna S., Eyheramendy S., Voight B. F., Butler J. L., Guiducci C., Illig T., Hackett R., Heid I. M., Jacobs K. B., Lyssenko V., Uda M., Boehnke M., Chanock S. J., Groop L. C., Hu F. B., Isomaa B., Kraft P., Peltonen L., Salomaa V., Schlessinger D., Hunter D. J., Hayes R. B., Abecasis G. R., Wichmann H.-E., Mohlke K. L., Hirschhorn J. N., Initiative T. D. G., FUSION, KORA, The Prostate, LC, Trial OCS, Study TNH, SardiNIA (2008) Identification of ten loci associated with height highlights new biological pathways in human growth. Nat Genet 40(5):584–591. https://doi.org/10.1038/ng.125
López-Rubio E, Ratti E (2021) Data science and molecular biology: prediction and mechanistic explanation. Synthese 198(4):3131–3156. https://doi.org/10.1007/s11229-019-02271-0
Matthews LJ, Turkheimer E (2019) Across the great divide: pluralism and the hunt for missing heritability. Synthese. https://doi.org/10.1007/s11229-019-02205-w
Mountjoy E, Schmidt EM, Carmona M, Peat G, Miranda A, Fumis L, Hayhurst J, Buniello A, Schwartzentruber J, Karim MA, Wright D, Hercules A, Papa E, Fauman E, Barrett JC, Todd JA, Ochoa D, Dunham I, Ghoussaini M (2020) Open targets genetics: an open approach to systematically prioritize causal variants and genes at all published human GWAS trait-associated loci, bioRxiv . https://www.biorxiv.org/content/early/2020/09/21/2020.09.16.299271
Needham E, Parker B, Burykin T, James D, Humphreys S (2019) Illuminating the dark phosphoproteome, Sci Signal. Vol. 12
Norton J (1995) Eliminative induction as a method of discovery: how Einstein discovered general relativity. In: Leplin J (ed) The creation of ideas in physics. Kluwer, Alphen aan den Rijn, pp 29–69
Chapter Google Scholar
O‘Malley M, Elliott K, Burian R (2010) From genetic to genomic regulation: iterativity in microRNA research. Stud Hist Philos Sci Part C Stud Hist Philos Biol Biomed Sci 41(4):407–417
Ratti E (2015) Big Data biology: between eliminative inferences and exploratory experiments. Philos Sci 82:198–218
Ratti E (2020) What kind of novelties can machine learning possibly generate? The case of genomics. Stud Hist Philos Sci Part A. 83:86–96. https://www.sciencedirect.com/science/article/pii/S0039368119302924
Reimers M, Craver C, Dozmorov M, Bacanu S-A, Kendler K (2019) The coherence problem: finding meaning in GWAS complexity. Behav Genet 49:187–195
Richardson S, Stevens H (2015) Postgenomics: perspectives on biology after the genome. Duke University Press, Durham
Samuelson W, Zeckhauser R (1988) Status quo bias in decision making. J Risk Uncertain 1(1):7–59. https://doi.org/10.1007/BF00055564
Schwartzentruber J, Cooper S, Liu JZ, Barrio-Hernandez I, Bello E, Kumasaka N, Young AMH, Franklin RJM, Johnson T, Estrada K, Gaffney DJ, Beltrao P, Bassett A (2021) Genome-wide meta-analysis, fine-mapping and integrative prioritization implicate new Alzheimer’s disease risk genes. Nat Genet 53(3):392–402. https://doi.org/10.1038/s41588-020-00776-w
Shalem O, Sanjana NE, Zhang F (2015) High-throughput functional genomics using CRISPR-Cas9. Nat Rev Genet 16(5):299–311
Sharma S, Petsalaki E (2019) Large-scale datasets uncovering cell signalling networks in cancer: context matters. Curr Opin Genet Dev. 54:118–124 Cancer Genomics. https://www.sciencedirect.com/science/article/pii/S0959437X18301278
Steinle F (1997) Entering new fields: exploratory uses of experimentation. Philos Sci. 64:S65–S74. http://www.jstor.org/stable/188390
Sullivan E (2019) Understanding from machine learning models. British J Philos Sci
Tam V, Patel N, Turcotte M, Bossé Y, Paré G, Meyre D (2019) Benefits and limitations of genome-wide association studies. Nat Rev Genet 20(8):467–484. https://doi.org/10.1038/s41576-019-0127-1
Weedon MN, Lango H, Lindgren CM, Wallace C, Evans, DM, Mangino M, Freathy RM, Perry J. RB, Stevens S, Hall AS, Samani NJ, Shields B, Prokopenko I, Farrall M, Dominiczak A, Johnson T, Bergmann S, Beckmann, JS, Vollenweider, P, Waterworth DM, Mooser V, Palmer CNA Morris AD Ouwehand WH, Zhao JH, Li S, Loos R JF, Barroso I, Deloukas P, Sandhu MS, Wheeler E, Soranzo N, Inouye M, Wareham NJ, Caulfield M, Munroe PB, Hattersley AT, McCarthy MI, Frayling TM, Initiative, DG, Consortium TWTCC, Consortium, CG (2008) Genome-wide association analysis identifies 20 loci that influence adult height. Nat Genet 40(5):575–583. https://doi.org/10.1038/ng.121
Wilkes EH, Terfve C, Gribben JG, Saez-Rodriguez J, Cutillas PR (2015) Empirical inference of circuitry and plasticity in a kinase signaling network. Proc Natl Acad Sci U S A 112(25):7719–7724
Wray N, Wijmenga C, Sullivan P, Yang J, Visscher P (2018) Common disease is more complex than implied by the core gene omnigenic model. Cell 173:1573–1580
Yang J, Benyamin B, McEvoy BP, Gordon S, Henders AK, Nyholt DR, Madden PA, Heath AC, Martin NG, Montgomery GW, Goddard ME, Visscher PM (2010) Common SNPs explain a large proportion of the heritability for human height. Nat Genet 42(7):565–569. https://doi.org/10.1038/ng.608
Download references
Acknowledgements
We are grateful to Simon Davis, Katie de Lange, and the anonymous reviewers (one of whom turned out to be Pierrick Bourrat) for helpful discussions and feedback. S.S. is supported by a Sir Henry Wellcome Postdoctoral Fellowship at the University of Oxford.
Author information
Authors and affiliations.
Faculty of Philosophy, University of Oxford, Oxford, UK
Weatherall Institute for Molecular Medicine, University of Oxford, Oxford, UK
Sumana Sharma
You can also search for this author in PubMed Google Scholar
Corresponding author
Correspondence to James Read .
Ethics declarations
Conflict of interest, additional information, publisher's note.
Springer Nature remains neutral with regard to jurisdictional claims in published maps and institutional affiliations.
Rights and permissions
Open Access This article is licensed under a Creative Commons Attribution 4.0 International License, which permits use, sharing, adaptation, distribution and reproduction in any medium or format, as long as you give appropriate credit to the original author(s) and the source, provide a link to the Creative Commons licence, and indicate if changes were made. The images or other third party material in this article are included in the article's Creative Commons licence, unless indicated otherwise in a credit line to the material. If material is not included in the article's Creative Commons licence and your intended use is not permitted by statutory regulation or exceeds the permitted use, you will need to obtain permission directly from the copyright holder. To view a copy of this licence, visit http://creativecommons.org/licenses/by/4.0/ .
Reprints and permissions
About this article
Read, J., Sharma, S. Hypothesis-driven science in large-scale studies: the case of GWAS. Biol Philos 36 , 46 (2021). https://doi.org/10.1007/s10539-021-09823-0
Download citation
Received : 24 May 2021
Accepted : 08 September 2021
Published : 19 September 2021
DOI : https://doi.org/10.1007/s10539-021-09823-0
Share this article
Anyone you share the following link with will be able to read this content:
Sorry, a shareable link is not currently available for this article.
Provided by the Springer Nature SharedIt content-sharing initiative
- Systems biology
- Hypothesis-driven science
- Machine learning
- Find a journal
- Publish with us
- Track your research
Course is available
Hypothesis-driven approach: Problem solving in the context of global health
Your browser is not fully supported.
You are running an outdated browser version, which is not fully supported by OpenWHO. You might not be able to use crucial functionality such as the submission of quizzes . Please update your browser to the latest version before you continue (we recommend Mozilla Firefox or Google Chrome ).
Click here to hide this warning permanently.
- Discussions
- Certificates
- Collab Space
- Course Details
- Announcements
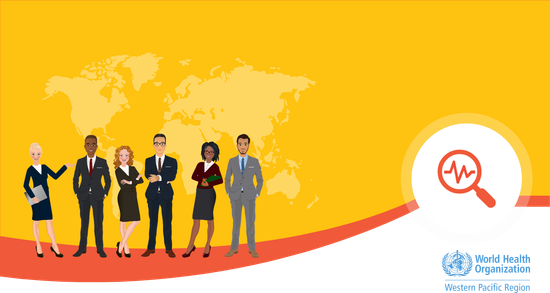
In this course, you will learn about the hypothesis-driven approach to problem-solving. This approach originated from academic research and later adopted in management consulting. The course consists of 4 modules that take you through a step-by-step process of solving problems in an effective and timely manner.
Course information
This course is also available in the following languages:
The hypothesis-driven approach is a problem-solving method that is necessary at WHO because the environment around us is changing rapidly. WHO needs a new way of problem-solving to process large amounts of information from different fields and deliver quick, tailored recommendations to meet the needs of Member States. The hypothesis-driven approach produces solutions quickly with continuous refinement throughout the research process.
What you'll learn
- Define the most important questions to address.
- Break down the question into components and develop an issue tree.
- Develop and validate the hypothesis.
- Synthesize findings and support recommendations by presenting evidence in a structured manner.
Who this course is for
- This course is for everyone. Whether your position is in administrative, operations, or technical area of work, you’re sure to run into problems to solve. Problem-solving is a key skill to continue developing and refining—the hypothesis-driven approach will surely be a great addition in your toolbox!
Course contents
Introduction: hypothesis-driven approach to problem solving:, module 1: identify the question:, module 2: develop & validate hypothesis:, module 3: synthesize findings & make recommendations:, enroll me for this course, certificate requirements.
- Gain a Record of Achievement by earning at least 80% of the maximum number of points from all graded assignments.
- Gain a Confirmation of Participation by completing at least 80% of the course material.
- Gain an Open Badge by completing the course.
Have a language expert improve your writing
Run a free plagiarism check in 10 minutes, generate accurate citations for free.
- Knowledge Base
Methodology
- How to Write a Strong Hypothesis | Steps & Examples
How to Write a Strong Hypothesis | Steps & Examples
Published on May 6, 2022 by Shona McCombes . Revised on November 20, 2023.
A hypothesis is a statement that can be tested by scientific research. If you want to test a relationship between two or more variables, you need to write hypotheses before you start your experiment or data collection .

Example: Hypothesis
Daily apple consumption leads to fewer doctor’s visits.
Table of contents
What is a hypothesis, developing a hypothesis (with example), hypothesis examples, other interesting articles, frequently asked questions about writing hypotheses.
A hypothesis states your predictions about what your research will find. It is a tentative answer to your research question that has not yet been tested. For some research projects, you might have to write several hypotheses that address different aspects of your research question.
A hypothesis is not just a guess – it should be based on existing theories and knowledge. It also has to be testable, which means you can support or refute it through scientific research methods (such as experiments, observations and statistical analysis of data).
Variables in hypotheses
Hypotheses propose a relationship between two or more types of variables .
- An independent variable is something the researcher changes or controls.
- A dependent variable is something the researcher observes and measures.
If there are any control variables , extraneous variables , or confounding variables , be sure to jot those down as you go to minimize the chances that research bias will affect your results.
In this example, the independent variable is exposure to the sun – the assumed cause . The dependent variable is the level of happiness – the assumed effect .
Receive feedback on language, structure, and formatting
Professional editors proofread and edit your paper by focusing on:
- Academic style
- Vague sentences
- Style consistency
See an example
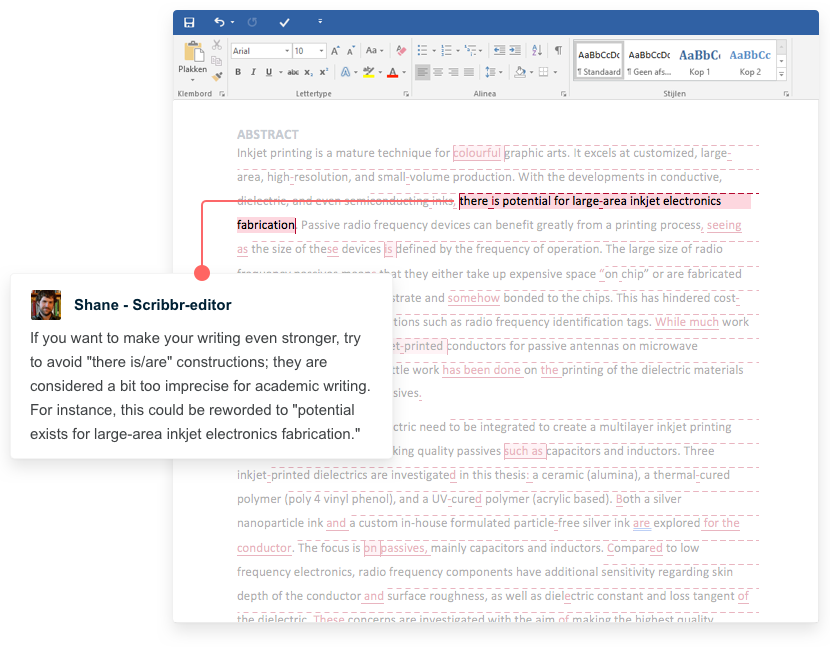
Step 1. Ask a question
Writing a hypothesis begins with a research question that you want to answer. The question should be focused, specific, and researchable within the constraints of your project.
Step 2. Do some preliminary research
Your initial answer to the question should be based on what is already known about the topic. Look for theories and previous studies to help you form educated assumptions about what your research will find.
At this stage, you might construct a conceptual framework to ensure that you’re embarking on a relevant topic . This can also help you identify which variables you will study and what you think the relationships are between them. Sometimes, you’ll have to operationalize more complex constructs.
Step 3. Formulate your hypothesis
Now you should have some idea of what you expect to find. Write your initial answer to the question in a clear, concise sentence.
4. Refine your hypothesis
You need to make sure your hypothesis is specific and testable. There are various ways of phrasing a hypothesis, but all the terms you use should have clear definitions, and the hypothesis should contain:
- The relevant variables
- The specific group being studied
- The predicted outcome of the experiment or analysis
5. Phrase your hypothesis in three ways
To identify the variables, you can write a simple prediction in if…then form. The first part of the sentence states the independent variable and the second part states the dependent variable.
In academic research, hypotheses are more commonly phrased in terms of correlations or effects, where you directly state the predicted relationship between variables.
If you are comparing two groups, the hypothesis can state what difference you expect to find between them.
6. Write a null hypothesis
If your research involves statistical hypothesis testing , you will also have to write a null hypothesis . The null hypothesis is the default position that there is no association between the variables. The null hypothesis is written as H 0 , while the alternative hypothesis is H 1 or H a .
- H 0 : The number of lectures attended by first-year students has no effect on their final exam scores.
- H 1 : The number of lectures attended by first-year students has a positive effect on their final exam scores.
Research question | Hypothesis | Null hypothesis |
---|---|---|
What are the health benefits of eating an apple a day? | Increasing apple consumption in over-60s will result in decreasing frequency of doctor’s visits. | Increasing apple consumption in over-60s will have no effect on frequency of doctor’s visits. |
Which airlines have the most delays? | Low-cost airlines are more likely to have delays than premium airlines. | Low-cost and premium airlines are equally likely to have delays. |
Can flexible work arrangements improve job satisfaction? | Employees who have flexible working hours will report greater job satisfaction than employees who work fixed hours. | There is no relationship between working hour flexibility and job satisfaction. |
How effective is high school sex education at reducing teen pregnancies? | Teenagers who received sex education lessons throughout high school will have lower rates of unplanned pregnancy teenagers who did not receive any sex education. | High school sex education has no effect on teen pregnancy rates. |
What effect does daily use of social media have on the attention span of under-16s? | There is a negative between time spent on social media and attention span in under-16s. | There is no relationship between social media use and attention span in under-16s. |
If you want to know more about the research process , methodology , research bias , or statistics , make sure to check out some of our other articles with explanations and examples.
- Sampling methods
- Simple random sampling
- Stratified sampling
- Cluster sampling
- Likert scales
- Reproducibility
Statistics
- Null hypothesis
- Statistical power
- Probability distribution
- Effect size
- Poisson distribution
Research bias
- Optimism bias
- Cognitive bias
- Implicit bias
- Hawthorne effect
- Anchoring bias
- Explicit bias
A hypothesis is not just a guess — it should be based on existing theories and knowledge. It also has to be testable, which means you can support or refute it through scientific research methods (such as experiments, observations and statistical analysis of data).
Null and alternative hypotheses are used in statistical hypothesis testing . The null hypothesis of a test always predicts no effect or no relationship between variables, while the alternative hypothesis states your research prediction of an effect or relationship.
Hypothesis testing is a formal procedure for investigating our ideas about the world using statistics. It is used by scientists to test specific predictions, called hypotheses , by calculating how likely it is that a pattern or relationship between variables could have arisen by chance.
Cite this Scribbr article
If you want to cite this source, you can copy and paste the citation or click the “Cite this Scribbr article” button to automatically add the citation to our free Citation Generator.
McCombes, S. (2023, November 20). How to Write a Strong Hypothesis | Steps & Examples. Scribbr. Retrieved August 29, 2024, from https://www.scribbr.com/methodology/hypothesis/
Is this article helpful?
Shona McCombes
Other students also liked, construct validity | definition, types, & examples, what is a conceptual framework | tips & examples, operationalization | a guide with examples, pros & cons, get unlimited documents corrected.
✔ Free APA citation check included ✔ Unlimited document corrections ✔ Specialized in correcting academic texts
Type of Research projects Part 2: Hypothesis-driven versus hypothesis-generating research (1 August 2018)
An official website of the United States government
Here’s how you know
Official websites use .gov A .gov website belongs to an official government organization in the United States.
Secure .gov websites use HTTPS A lock ( Lock Locked padlock ) or https:// means you’ve safely connected to the .gov website. Share sensitive information only on official, secure websites.
Guidance on building better digital services in government
You have reached a collection of archived material
The content available is no longer being updated and as a result you may encounter hyperlinks which no longer function. This content may contain text and references which are no longer applicable as a result of changes in law, regulation, and/or administration.
Using a Hypothesis-Driven Approach in Analyzing (and Making Sense) of Your Website Traffic Data
At the Digital Analytics Program (DAP), some of the most frequently asked questions we get are “how can I get access to the DAP data?” and “what do I do with all this data?” We all know that data is knowledge, and knowledge is power, but once we have access to it and realize that it is, indeed, oceans of data , how do we not “drown” in it, and, perhaps more importantly, how do we make sense of it?
What Questions Are You Trying to Answer?
In research and data analysis, a hypothesis-driven approach is one of the main methods for using data to test and, ultimately, prove (or disprove) assertions. To do that, researchers collect a sufficient amount of data on the subject and then approach it with a specific hypothesis in mind. On the flipside, there’s also exploratory data analysis, where data analysts “dive” into data in search of patterns (or lack there of). Often times, exploratory data analysis creates a foundation for hypotheses, or feeds our assertions that then get used as part of hypothesis-driven testing.
So what does this all mean? It means that when you are attempting to perform an analysis using multidimensional website traffic data, you, too, should approach it with a goal in mind, or, at minimum, specific questions you want to be answered. Hence, when people ask us, “what do I do with all this data?” our response is “what questions are you trying to answer?”
Example: Real-Time Gov-Wide Visitors on #TaxDay
Let’s use this in a concrete example. On April 14, 2015, in a conversation with my team regarding the gov-wide website traffic on #TaxDay, I casually made an assertion that the number of real-time visitors on April 15, 2015, may reach 300,000. The assertion was based on the fact that in almost three years of the DAP’s life, we’ve seen several big spikes (up to 220K real-time visitors), which had happened before IRS.gov became part of the DAP. So, I figured, now that DAP monitors IRS.gov traffic, in addition to other large governement websites, perhaps we’ll see a big spike in real-time visitor traffic on #TaxDay because lots of people will be online filing their tax returns and/or extensions on the last day. My hypothesis was not based on any previously done exploratory analysis.
To see if we’d reach that new record of real-time traffic in DAP on #TaxDay, our DigitalGov team performed live blogging and hourly monitoring throughout the day yesterday to report on the real-time users on the .gov websites as part of the public dashboard . And…. the hypothesis did not hold true (and was rejected by reality). The highest number of real-time visitors gov-wide we got on #TaxDay for 2015 was just shy of 200K.
What Happens After the Hypothesis is Tested
So now that the results of my hypothesis are known, the big question is “why?”
Well, the data tells us that for the last three months, the “ Where’s My Refund ?” page has been consistently in the top performing pages with the highest number of real-time users and a total 115M+ pageviews since February 1, 2015. IRS-related pages overall have been dominating the top 20 active pages consistently in the last three months, suggesting that people were filing their taxes online during the last few months and, then, naturally, spent most of their time wondering when they get their refund. We did see a spike in the extension applications [PDF] downloads yesterday, which makes sense, but the number of visitors filling out the application was not high enough to bring us anywhere even close to 300K real-time visitors.
Interestingly, the uptick in overall traffic to .gov websites on #TaxDay was modest compared to previous events driven by weather, space shuttle launches, and asteroid fly-bys. Hmmm…. that may be a good topic for a different blog post.
In Conclusion
The DAP yields a lot of data and may be overwhelming but is a goldmine for exploratory and hypothesis-driven analyses. With the right questions in mind, this data can help the government to better understand its visitors and what brings them to agency websites, and ultimately, continuously create better web content and digital services for online visitors.
Join a Community
- Federal Evidence and Evaluation
- Web Analytics and Optimization
- Web Managers
Related Topics
2015-04-16-using-a-hypothesis-driven-approach-in-analyzing-and-making-sense-of-your-website-traffic-data.md
news/2015/04/2015-04-16-using-a-hypothesis-driven-approach-in-analyzing-and-making-sense-of-your-website-traffic-data.md
Link Shortcode
{{< link "news/2015/04/2015-04-16-using-a-hypothesis-driven-approach-in-analyzing-and-making-sense-of-your-website-traffic-data.md
" >}}
Join 18,000 others in government and subscribe to our newsletter — a round-up of the best digital news in government and across our field.
Digital.gov
An official website of the U.S. General Services Administration

An official website of the United States government
The .gov means it’s official. Federal government websites often end in .gov or .mil. Before sharing sensitive information, make sure you’re on a federal government site.
The site is secure. The https:// ensures that you are connecting to the official website and that any information you provide is encrypted and transmitted securely.
- Publications
- Account settings
Preview improvements coming to the PMC website in October 2024. Learn More or Try it out now .
- Advanced Search
- Journal List
- v.53(4); 2010 Aug

Research questions, hypotheses and objectives
Patricia farrugia.
* Michael G. DeGroote School of Medicine, the
Bradley A. Petrisor
† Division of Orthopaedic Surgery and the
Forough Farrokhyar
‡ Departments of Surgery and
§ Clinical Epidemiology and Biostatistics, McMaster University, Hamilton, Ont
Mohit Bhandari
There is an increasing familiarity with the principles of evidence-based medicine in the surgical community. As surgeons become more aware of the hierarchy of evidence, grades of recommendations and the principles of critical appraisal, they develop an increasing familiarity with research design. Surgeons and clinicians are looking more and more to the literature and clinical trials to guide their practice; as such, it is becoming a responsibility of the clinical research community to attempt to answer questions that are not only well thought out but also clinically relevant. The development of the research question, including a supportive hypothesis and objectives, is a necessary key step in producing clinically relevant results to be used in evidence-based practice. A well-defined and specific research question is more likely to help guide us in making decisions about study design and population and subsequently what data will be collected and analyzed. 1
Objectives of this article
In this article, we discuss important considerations in the development of a research question and hypothesis and in defining objectives for research. By the end of this article, the reader will be able to appreciate the significance of constructing a good research question and developing hypotheses and research objectives for the successful design of a research study. The following article is divided into 3 sections: research question, research hypothesis and research objectives.
Research question
Interest in a particular topic usually begins the research process, but it is the familiarity with the subject that helps define an appropriate research question for a study. 1 Questions then arise out of a perceived knowledge deficit within a subject area or field of study. 2 Indeed, Haynes suggests that it is important to know “where the boundary between current knowledge and ignorance lies.” 1 The challenge in developing an appropriate research question is in determining which clinical uncertainties could or should be studied and also rationalizing the need for their investigation.
Increasing one’s knowledge about the subject of interest can be accomplished in many ways. Appropriate methods include systematically searching the literature, in-depth interviews and focus groups with patients (and proxies) and interviews with experts in the field. In addition, awareness of current trends and technological advances can assist with the development of research questions. 2 It is imperative to understand what has been studied about a topic to date in order to further the knowledge that has been previously gathered on a topic. Indeed, some granting institutions (e.g., Canadian Institute for Health Research) encourage applicants to conduct a systematic review of the available evidence if a recent review does not already exist and preferably a pilot or feasibility study before applying for a grant for a full trial.
In-depth knowledge about a subject may generate a number of questions. It then becomes necessary to ask whether these questions can be answered through one study or if more than one study needed. 1 Additional research questions can be developed, but several basic principles should be taken into consideration. 1 All questions, primary and secondary, should be developed at the beginning and planning stages of a study. Any additional questions should never compromise the primary question because it is the primary research question that forms the basis of the hypothesis and study objectives. It must be kept in mind that within the scope of one study, the presence of a number of research questions will affect and potentially increase the complexity of both the study design and subsequent statistical analyses, not to mention the actual feasibility of answering every question. 1 A sensible strategy is to establish a single primary research question around which to focus the study plan. 3 In a study, the primary research question should be clearly stated at the end of the introduction of the grant proposal, and it usually specifies the population to be studied, the intervention to be implemented and other circumstantial factors. 4
Hulley and colleagues 2 have suggested the use of the FINER criteria in the development of a good research question ( Box 1 ). The FINER criteria highlight useful points that may increase the chances of developing a successful research project. A good research question should specify the population of interest, be of interest to the scientific community and potentially to the public, have clinical relevance and further current knowledge in the field (and of course be compliant with the standards of ethical boards and national research standards).
FINER criteria for a good research question
Feasible | ||
Interesting | ||
Novel | ||
Ethical | ||
Relevant |
Adapted with permission from Wolters Kluwer Health. 2
Whereas the FINER criteria outline the important aspects of the question in general, a useful format to use in the development of a specific research question is the PICO format — consider the population (P) of interest, the intervention (I) being studied, the comparison (C) group (or to what is the intervention being compared) and the outcome of interest (O). 3 , 5 , 6 Often timing (T) is added to PICO ( Box 2 ) — that is, “Over what time frame will the study take place?” 1 The PICOT approach helps generate a question that aids in constructing the framework of the study and subsequently in protocol development by alluding to the inclusion and exclusion criteria and identifying the groups of patients to be included. Knowing the specific population of interest, intervention (and comparator) and outcome of interest may also help the researcher identify an appropriate outcome measurement tool. 7 The more defined the population of interest, and thus the more stringent the inclusion and exclusion criteria, the greater the effect on the interpretation and subsequent applicability and generalizability of the research findings. 1 , 2 A restricted study population (and exclusion criteria) may limit bias and increase the internal validity of the study; however, this approach will limit external validity of the study and, thus, the generalizability of the findings to the practical clinical setting. Conversely, a broadly defined study population and inclusion criteria may be representative of practical clinical practice but may increase bias and reduce the internal validity of the study.
PICOT criteria 1
Population (patients) | ||
Intervention (for intervention studies only) | ||
Comparison group | ||
Outcome of interest | ||
Time |
A poorly devised research question may affect the choice of study design, potentially lead to futile situations and, thus, hamper the chance of determining anything of clinical significance, which will then affect the potential for publication. Without devoting appropriate resources to developing the research question, the quality of the study and subsequent results may be compromised. During the initial stages of any research study, it is therefore imperative to formulate a research question that is both clinically relevant and answerable.
Research hypothesis
The primary research question should be driven by the hypothesis rather than the data. 1 , 2 That is, the research question and hypothesis should be developed before the start of the study. This sounds intuitive; however, if we take, for example, a database of information, it is potentially possible to perform multiple statistical comparisons of groups within the database to find a statistically significant association. This could then lead one to work backward from the data and develop the “question.” This is counterintuitive to the process because the question is asked specifically to then find the answer, thus collecting data along the way (i.e., in a prospective manner). Multiple statistical testing of associations from data previously collected could potentially lead to spuriously positive findings of association through chance alone. 2 Therefore, a good hypothesis must be based on a good research question at the start of a trial and, indeed, drive data collection for the study.
The research or clinical hypothesis is developed from the research question and then the main elements of the study — sampling strategy, intervention (if applicable), comparison and outcome variables — are summarized in a form that establishes the basis for testing, statistical and ultimately clinical significance. 3 For example, in a research study comparing computer-assisted acetabular component insertion versus freehand acetabular component placement in patients in need of total hip arthroplasty, the experimental group would be computer-assisted insertion and the control/conventional group would be free-hand placement. The investigative team would first state a research hypothesis. This could be expressed as a single outcome (e.g., computer-assisted acetabular component placement leads to improved functional outcome) or potentially as a complex/composite outcome; that is, more than one outcome (e.g., computer-assisted acetabular component placement leads to both improved radiographic cup placement and improved functional outcome).
However, when formally testing statistical significance, the hypothesis should be stated as a “null” hypothesis. 2 The purpose of hypothesis testing is to make an inference about the population of interest on the basis of a random sample taken from that population. The null hypothesis for the preceding research hypothesis then would be that there is no difference in mean functional outcome between the computer-assisted insertion and free-hand placement techniques. After forming the null hypothesis, the researchers would form an alternate hypothesis stating the nature of the difference, if it should appear. The alternate hypothesis would be that there is a difference in mean functional outcome between these techniques. At the end of the study, the null hypothesis is then tested statistically. If the findings of the study are not statistically significant (i.e., there is no difference in functional outcome between the groups in a statistical sense), we cannot reject the null hypothesis, whereas if the findings were significant, we can reject the null hypothesis and accept the alternate hypothesis (i.e., there is a difference in mean functional outcome between the study groups), errors in testing notwithstanding. In other words, hypothesis testing confirms or refutes the statement that the observed findings did not occur by chance alone but rather occurred because there was a true difference in outcomes between these surgical procedures. The concept of statistical hypothesis testing is complex, and the details are beyond the scope of this article.
Another important concept inherent in hypothesis testing is whether the hypotheses will be 1-sided or 2-sided. A 2-sided hypothesis states that there is a difference between the experimental group and the control group, but it does not specify in advance the expected direction of the difference. For example, we asked whether there is there an improvement in outcomes with computer-assisted surgery or whether the outcomes worse with computer-assisted surgery. We presented a 2-sided test in the above example because we did not specify the direction of the difference. A 1-sided hypothesis states a specific direction (e.g., there is an improvement in outcomes with computer-assisted surgery). A 2-sided hypothesis should be used unless there is a good justification for using a 1-sided hypothesis. As Bland and Atlman 8 stated, “One-sided hypothesis testing should never be used as a device to make a conventionally nonsignificant difference significant.”
The research hypothesis should be stated at the beginning of the study to guide the objectives for research. Whereas the investigators may state the hypothesis as being 1-sided (there is an improvement with treatment), the study and investigators must adhere to the concept of clinical equipoise. According to this principle, a clinical (or surgical) trial is ethical only if the expert community is uncertain about the relative therapeutic merits of the experimental and control groups being evaluated. 9 It means there must exist an honest and professional disagreement among expert clinicians about the preferred treatment. 9
Designing a research hypothesis is supported by a good research question and will influence the type of research design for the study. Acting on the principles of appropriate hypothesis development, the study can then confidently proceed to the development of the research objective.
Research objective
The primary objective should be coupled with the hypothesis of the study. Study objectives define the specific aims of the study and should be clearly stated in the introduction of the research protocol. 7 From our previous example and using the investigative hypothesis that there is a difference in functional outcomes between computer-assisted acetabular component placement and free-hand placement, the primary objective can be stated as follows: this study will compare the functional outcomes of computer-assisted acetabular component insertion versus free-hand placement in patients undergoing total hip arthroplasty. Note that the study objective is an active statement about how the study is going to answer the specific research question. Objectives can (and often do) state exactly which outcome measures are going to be used within their statements. They are important because they not only help guide the development of the protocol and design of study but also play a role in sample size calculations and determining the power of the study. 7 These concepts will be discussed in other articles in this series.
From the surgeon’s point of view, it is important for the study objectives to be focused on outcomes that are important to patients and clinically relevant. For example, the most methodologically sound randomized controlled trial comparing 2 techniques of distal radial fixation would have little or no clinical impact if the primary objective was to determine the effect of treatment A as compared to treatment B on intraoperative fluoroscopy time. However, if the objective was to determine the effect of treatment A as compared to treatment B on patient functional outcome at 1 year, this would have a much more significant impact on clinical decision-making. Second, more meaningful surgeon–patient discussions could ensue, incorporating patient values and preferences with the results from this study. 6 , 7 It is the precise objective and what the investigator is trying to measure that is of clinical relevance in the practical setting.
The following is an example from the literature about the relation between the research question, hypothesis and study objectives:
Study: Warden SJ, Metcalf BR, Kiss ZS, et al. Low-intensity pulsed ultrasound for chronic patellar tendinopathy: a randomized, double-blind, placebo-controlled trial. Rheumatology 2008;47:467–71.
Research question: How does low-intensity pulsed ultrasound (LIPUS) compare with a placebo device in managing the symptoms of skeletally mature patients with patellar tendinopathy?
Research hypothesis: Pain levels are reduced in patients who receive daily active-LIPUS (treatment) for 12 weeks compared with individuals who receive inactive-LIPUS (placebo).
Objective: To investigate the clinical efficacy of LIPUS in the management of patellar tendinopathy symptoms.
The development of the research question is the most important aspect of a research project. A research project can fail if the objectives and hypothesis are poorly focused and underdeveloped. Useful tips for surgical researchers are provided in Box 3 . Designing and developing an appropriate and relevant research question, hypothesis and objectives can be a difficult task. The critical appraisal of the research question used in a study is vital to the application of the findings to clinical practice. Focusing resources, time and dedication to these 3 very important tasks will help to guide a successful research project, influence interpretation of the results and affect future publication efforts.
Tips for developing research questions, hypotheses and objectives for research studies
- Perform a systematic literature review (if one has not been done) to increase knowledge and familiarity with the topic and to assist with research development.
- Learn about current trends and technological advances on the topic.
- Seek careful input from experts, mentors, colleagues and collaborators to refine your research question as this will aid in developing the research question and guide the research study.
- Use the FINER criteria in the development of the research question.
- Ensure that the research question follows PICOT format.
- Develop a research hypothesis from the research question.
- Develop clear and well-defined primary and secondary (if needed) objectives.
- Ensure that the research question and objectives are answerable, feasible and clinically relevant.
FINER = feasible, interesting, novel, ethical, relevant; PICOT = population (patients), intervention (for intervention studies only), comparison group, outcome of interest, time.
Competing interests: No funding was received in preparation of this paper. Dr. Bhandari was funded, in part, by a Canada Research Chair, McMaster University.
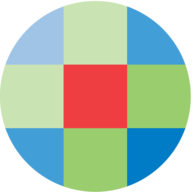
- Subscribe to journal Subscribe
- Get new issue alerts Get alerts
Secondary Logo
Journal logo.
Colleague's E-mail is Invalid
Your message has been successfully sent to your colleague.
Save my selection
A Comparison of Hypothesis-Driven and Data-Driven Research
A case study in multimodal data science in gut-brain axis research.
Dreisbach, Caitlin PhD, RN; Maki, Katherine PhD, APRN
Author Affiliations: Data Science Institute, Columbia University, New York, NY (Dr Dreisbach); and Translational Biobehavioral and Health Disparities Branch, National Institutes of Health Clinical Center (Dr Maki), Bethesda, MD.
This research was supported by intramural research funds from the National Institutes of Health Clinical Center (K.M.).
The authors have disclosed that they have no significant relationships with, or financial interest in, any commercial companies pertaining to this article.
The content is solely the responsibility of the authors and does not necessarily represent the official views of the National Institutes of Health.
Corresponding author: Caitlin Dreisbach, PhD, RN, 61 Claremont Ave, New York, NY 10027 ( [email protected] ).
Data science, bioinformatics, and machine learning are the advent and progression of the fourth paradigm of exploratory science. The need for human-supported algorithms to capture patterns in big data is at the center of personalized healthcare and directly related to translational research. This paper argues that hypothesis-driven and data-driven research work together to inform the research process. At the core of these approaches are theoretical underpinnings that drive progress in the field. Here, we present several exemplars of research on the gut-brain axis that outline the innate values and challenges of these approaches. As nurses are trained to integrate multiple body systems to inform holistic human health promotion and disease prevention, nurses and nurse scientists serve an important role as mediators between this advancing technology and the patients. At the center of person-knowing, nurses need to be aware of the data revolution and use their unique skills to supplement the data science cycle from data to knowledge to insight.
Full Text Access for Subscribers:
Individual subscribers.

Institutional Users
Not a subscriber.
You can read the full text of this article if you:
- + Favorites
- View in Gallery
Readers Of this Article Also Read
Development and effectiveness of a clinical decision support system for..., automated fall and pressure injury risk assessment systems: nurses'..., a review of dashboards for data analytics in nursing.
- Study Protocol
- Open access
- Published: 26 August 2024
Learning effect of online versus onsite education in health and medical scholarship – protocol for a cluster randomized trial
- Rie Raffing 1 ,
- Lars Konge 2 &
- Hanne Tønnesen 1
BMC Medical Education volume 24 , Article number: 927 ( 2024 ) Cite this article
123 Accesses
Metrics details
The disruption of health and medical education by the COVID-19 pandemic made educators question the effect of online setting on students’ learning, motivation, self-efficacy and preference. In light of the health care staff shortage online scalable education seemed relevant. Reviews on the effect of online medical education called for high quality RCTs, which are increasingly relevant with rapid technological development and widespread adaption of online learning in universities. The objective of this trial is to compare standardized and feasible outcomes of an online and an onsite setting of a research course regarding the efficacy for PhD students within health and medical sciences: Primarily on learning of research methodology and secondly on preference, motivation, self-efficacy on short term and academic achievements on long term. Based on the authors experience with conducting courses during the pandemic, the hypothesis is that student preferred onsite setting is different to online setting.
Cluster randomized trial with two parallel groups. Two PhD research training courses at the University of Copenhagen are randomized to online (Zoom) or onsite (The Parker Institute, Denmark) setting. Enrolled students are invited to participate in the study. Primary outcome is short term learning. Secondary outcomes are short term preference, motivation, self-efficacy, and long-term academic achievements. Standardized, reproducible and feasible outcomes will be measured by tailor made multiple choice questionnaires, evaluation survey, frequently used Intrinsic Motivation Inventory, Single Item Self-Efficacy Question, and Google Scholar publication data. Sample size is calculated to 20 clusters and courses are randomized by a computer random number generator. Statistical analyses will be performed blinded by an external statistical expert.
Primary outcome and secondary significant outcomes will be compared and contrasted with relevant literature. Limitations include geographical setting; bias include lack of blinding and strengths are robust assessment methods in a well-established conceptual framework. Generalizability to PhD education in other disciplines is high. Results of this study will both have implications for students and educators involved in research training courses in health and medical education and for the patients who ultimately benefits from this training.
Trial registration
Retrospectively registered at ClinicalTrials.gov: NCT05736627. SPIRIT guidelines are followed.
Peer Review reports
Medical education was utterly disrupted for two years by the COVID-19 pandemic. In the midst of rearranging courses and adapting to online platforms we, with lecturers and course managers around the globe, wondered what the conversion to online setting did to students’ learning, motivation and self-efficacy [ 1 , 2 , 3 ]. What the long-term consequences would be [ 4 ] and if scalable online medical education should play a greater role in the future [ 5 ] seemed relevant and appealing questions in a time when health care professionals are in demand. Our experience of performing research training during the pandemic was that although PhD students were grateful for courses being available, they found it difficult to concentrate related to the long screen hours. We sensed that most students preferred an onsite setting and perceived online courses a temporary and inferior necessity. The question is if this impacted their learning?
Since the common use of the internet in medical education, systematic reviews have sought to answer if there is a difference in learning effect when taught online compared to onsite. Although authors conclude that online learning may be equivalent to onsite in effect, they agree that studies are heterogeneous and small [ 6 , 7 ], with low quality of the evidence [ 8 , 9 ]. They therefore call for more robust and adequately powered high-quality RCTs to confirm their findings and suggest that students’ preferences in online learning should be investigated [ 7 , 8 , 9 ].
This uncovers two knowledge gaps: I) High-quality RCTs on online versus onsite learning in health and medical education and II) Studies on students’ preferences in online learning.
Recently solid RCTs have been performed on the topic of web-based theoretical learning of research methods among health professionals [ 10 , 11 ]. However, these studies are on asynchronous courses among medical or master students with short term outcomes.
This uncovers three additional knowledge gaps: III) Studies on synchronous online learning IV) among PhD students of health and medical education V) with long term measurement of outcomes.
The rapid technological development including artificial intelligence (AI) and widespread adaption as well as application of online learning forced by the pandemic, has made online learning well-established. It represents high resolution live synchronic settings which is available on a variety of platforms with integrated AI and options for interaction with and among students, chat and break out rooms, and exterior digital tools for teachers [ 12 , 13 , 14 ]. Thus, investigating online learning today may be quite different than before the pandemic. On one hand, it could seem plausible that this technological development would make a difference in favour of online learning which could not be found in previous reviews of the evidence. On the other hand, the personal face-to-face interaction during onsite learning may still be more beneficial for the learning process and combined with our experience of students finding it difficult to concentrate when online during the pandemic we hypothesize that outcomes of the onsite setting are different from the online setting.
To support a robust study, we design it as a cluster randomized trial. Moreover, we use the well-established and widely used Kirkpatrick’s conceptual framework for evaluating learning as a lens to assess our outcomes [ 15 ]. Thus, to fill the above-mentioned knowledge gaps, the objective of this trial is to compare a synchronous online and an in-person onsite setting of a research course regarding the efficacy for PhD students within the health and medical sciences:
Primarily on theoretical learning of research methodology and
Secondly on
◦ Preference, motivation, self-efficacy on short term
◦ Academic achievements on long term
Trial design
This study protocol covers synchronous online and in-person onsite setting of research courses testing the efficacy for PhD students. It is a two parallel arms cluster randomized trial (Fig. 1 ).
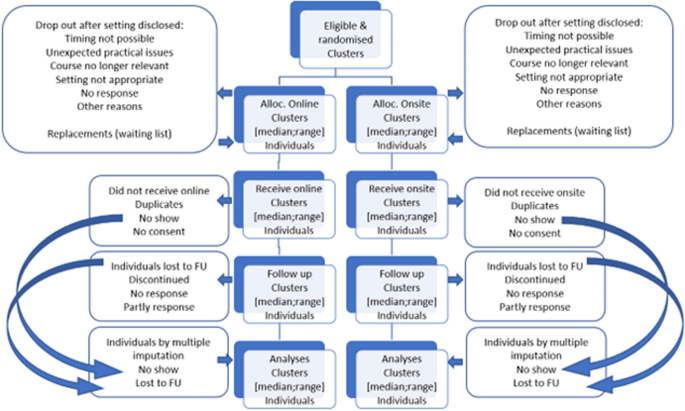
Consort flow diagram
The study measures baseline and post intervention. Baseline variables and knowledge scores are obtained at the first day of the course, post intervention measurement is obtained the last day of the course (short term) and monthly for 24 months (long term).
Randomization is stratified giving 1:1 allocation ratio of the courses. As the number of participants within each course might differ, the allocation ratio of participants in the study will not fully be equal and 1:1 balanced.
Study setting
The study site is The Parker Institute at Bispebjerg and Frederiksberg Hospital, University of Copenhagen, Denmark. From here the courses are organized and run online and onsite. The course programs and time schedules, the learning objective, the course management, the lecturers, and the delivery are identical in the two settings. The teachers use the same introductory presentations followed by training in break out groups, feed-back and discussions. For the online group, the setting is organized as meetings in the online collaboration tool Zoom® [ 16 ] using the basic available technicalities such as screen sharing, chat function for comments, and breakout rooms and other basics digital tools if preferred. The online version of the course is synchronous with live education and interaction. For the onsite group, the setting is the physical classroom at the learning facilities at the Parker Institute. Coffee and tea as well as simple sandwiches and bottles of water, which facilitate sociality, are available at the onsite setting. The participants in the online setting must get their food and drink by themselves, but online sociality is made possible by not closing down the online room during the breaks. The research methodology courses included in the study are “Practical Course in Systematic Review Technique in Clinical Research”, (see course programme in appendix 1) and “Getting started: Writing your first manuscript for publication” [ 17 ] (see course programme in appendix 2). The two courses both have 12 seats and last either three or three and a half days resulting in 2.2 and 2.6 ECTS credits, respectively. They are offered by the PhD School of the Faculty of Health and Medical Sciences, University of Copenhagen. Both courses are available and covered by the annual tuition fee for all PhD students enrolled at a Danish university.
Eligibility criteria
Inclusion criteria for participants: All PhD students enrolled on the PhD courses participate after informed consent: “Practical Course in Systematic Review Technique in Clinical Research” and “Getting started: Writing your first manuscript for publication” at the PhD School of the Faculty of Health and Medical Sciences, University of Copenhagen, Denmark.
Exclusion criteria for participants: Declining to participate and withdrawal of informed consent.
Informed consent
The PhD students at the PhD School at the Faculty of Health Sciences, University of Copenhagen participate after informed consent, taken by the daily project leader, allowing evaluation data from the course to be used after pseudo-anonymization in the project. They are informed in a welcome letter approximately three weeks prior to the course and again in the introduction the first course day. They register their consent on the first course day (Appendix 3). Declining to participate in the project does not influence their participation in the course.
Interventions
Online course settings will be compared to onsite course settings. We test if the onsite setting is different to online. Online learning is increasing but onsite learning is still the preferred educational setting in a medical context. In this case onsite learning represents “usual care”. The online course setting is meetings in Zoom using the technicalities available such as chat and breakout rooms. The onsite setting is the learning facilities, at the Parker Institute, Bispebjerg and Frederiksberg Hospital, The Capital Region, University of Copenhagen, Denmark.
The course settings are not expected to harm the participants, but should a request be made to discontinue the course or change setting this will be met, and the participant taken out of the study. Course participants are allowed to take part in relevant concomitant courses or other interventions during the trial.
Strategies to improve adherence to interventions
Course participants are motivated to complete the course irrespectively of the setting because it bears ECTS-points for their PhD education and adds to the mandatory number of ECTS-points. Thus, we expect adherence to be the same in both groups. However, we monitor their presence in the course and allocate time during class for testing the short-term outcomes ( motivation, self-efficacy, preference and learning). We encourage and, if necessary, repeatedly remind them to register with Google Scholar for our testing of the long-term outcome (academic achievement).
Outcomes are related to the Kirkpatrick model for evaluating learning (Fig. 2 ) which divides outcomes into four different levels; Reaction which includes for example motivation, self-efficacy and preferences, Learning which includes knowledge acquisition, Behaviour for practical application of skills when back at the job (not included in our outcomes), and Results for impact for end-users which includes for example academic achievements in the form of scientific articles [ 18 , 19 , 20 ].
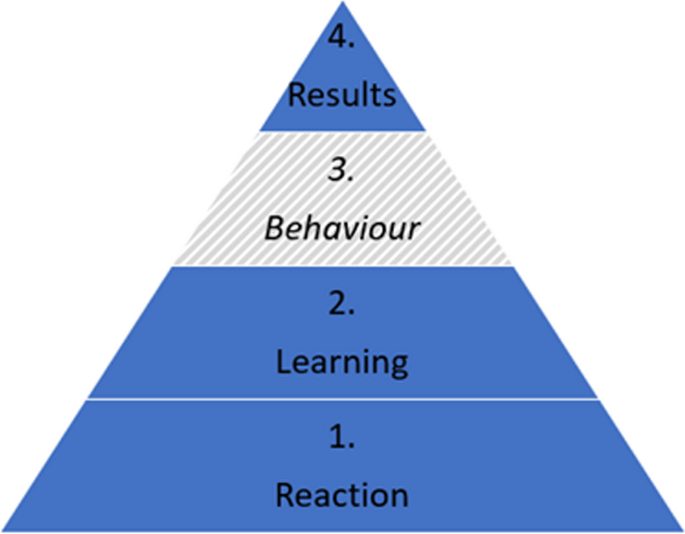
The Kirkpatrick model
Primary outcome
The primary outcome is short term learning (Kirkpatrick level 2).
Learning is assessed by a Multiple-Choice Questionnaire (MCQ) developed prior to the RCT specifically for this setting (Appendix 4). First the lecturers of the two courses were contacted and asked to provide five multiple choice questions presented as a stem with three answer options; one correct answer and two distractors. The questions should be related to core elements of their teaching under the heading of research training. The questions were set up to test the cognition of the students at the levels of "Knows" or "Knows how" according to Miller's Pyramid of Competence and not their behaviour [ 21 ]. Six of the course lecturers responded and out of this material all the questions which covered curriculum of both courses were selected. It was tested on 10 PhD students and within the lecturer group, revised after an item analysis and English language revised. The MCQ ended up containing 25 questions. The MCQ is filled in at baseline and repeated at the end of the course. The primary outcomes based on the MCQ is estimated as the score of learning calculated as number of correct answers out of 25 after the course. A decrease of points of the MCQ in the intervention groups denotes a deterioration of learning. In the MCQ the minimum score is 0 and 25 is maximum, where 19 indicates passing the course.
Furthermore, as secondary outcome, this outcome measurement will be categorized as binary outcome to determine passed/failed of the course defined by 75% (19/25) correct answers.
The learning score will be computed on group and individual level and compared regarding continued outcomes by the Mann–Whitney test comparing the learning score of the online and onsite groups. Regarding the binomial outcome of learning (passed/failed) data will be analysed by the Fisher’s exact test on an intention-to-treat basis between the online and onsite. The results will be presented as median and range and as mean and standard deviations, for possible future use in meta-analyses.
Secondary outcomes
Motivation assessment post course: Motivation level is measured by the Intrinsic Motivation Inventory (IMI) Scale [ 22 ] (Appendix 5). The IMI items were randomized by random.org on the 4th of August 2022. It contains 12 items to be assessed by the students on a 7-point Likert scale where 1 is “Not at all true”, 4 is “Somewhat true” and 7 is “Very true”. The motivation score will be computed on group and individual level and will then be tested by the Mann–Whitney of the online and onsite group.
Self-efficacy assessment post course: Self-efficacy level is measured by a single-item measure developed and validated by Williams and Smith [ 23 ] (Appendix 6). It is assessed by the students on a scale from 1–10 where 1 is “Strongly disagree” and 10 is “Strongly agree”. The self-efficacy score will be computed on group and individual level and tested by a Mann–Whitney test to compare the self-efficacy score of the online and onsite group.
Preference assessment post course: Preference is measured as part of the general course satisfaction evaluation with the question “If you had the option to choose, which form would you prefer this course to have?” with the options “onsite form” and “online form”.
Academic achievement assessment is based on 24 monthly measurements post course of number of publications, number of citations, h-index, i10-index. This data is collected through the Google Scholar Profiles [ 24 ] of the students as this database covers most scientific journals. Associations between onsite/online and long-term academic will be examined with Kaplan Meyer and log rank test with a significance level of 0.05.
Participant timeline
Enrolment for the course at the Faculty of Health Sciences, University of Copenhagen, Denmark, becomes available when it is published in the course catalogue. In the course description the course location is “To be announced”. Approximately 3–4 weeks before the course begins, the participant list is finalized, and students receive a welcome letter containing course details, including their allocation to either the online or onsite setting. On the first day of the course, oral information is provided, and participants provide informed consent, baseline variables, and base line knowledge scores.
The last day of scheduled activities the following scores are collected, knowledge, motivation, self-efficacy, setting preference, and academic achievement. To track students' long term academic achievements, follow-ups are conducted monthly for a period of 24 months, with assessments occurring within one week of the last course day (Table 1 ).
Sample size
The power calculation is based on the main outcome, theoretical learning on short term. For the sample size determination, we considered 12 available seats for participants in each course. To achieve statistical power, we aimed for 8 clusters in both online and onsite arms (in total 16 clusters) to detect an increase in learning outcome of 20% (learning outcome increase of 5 points). We considered an intraclass correlation coefficient of 0.02, a standard deviation of 10, a power of 80%, and a two-sided alpha level of 5%. The Allocation Ratio was set at 1, implying an equal number of subjects in both online and onsite group.
Considering a dropout up to 2 students per course, equivalent to 17%, we determined that a total of 112 participants would be needed. This calculation factored in 10 clusters of 12 participants per study arm, which we deemed sufficient to assess any changes in learning outcome.
The sample size was estimated using the function n4means from the R package CRTSize [ 25 ].
Recruitment
Participants are PhD students enrolled in 10 courses of “Practical Course in Systematic Review Technique in Clinical Research” and 10 courses of “Getting started: Writing your first manuscript for publication” at the PhD School of the Faculty of Health Sciences, University of Copenhagen, Denmark.
Assignment of interventions: allocation
Randomization will be performed on course-level. The courses are randomized by a computer random number generator [ 26 ]. To get a balanced randomization per year, 2 sets with 2 unique random integers in each, taken from the 1–4 range is requested.
The setting is not included in the course catalogue of the PhD School and thus allocation to online or onsite is concealed until 3–4 weeks before course commencement when a welcome letter with course information including allocation to online or onsite setting is distributed to the students. The lecturers are also informed of the course setting at this time point. If students withdraw from the course after being informed of the setting, a letter is sent to them enquiring of the reason for withdrawal and reason is recorded (Appendix 7).
The allocation sequence is generated by a computer random number generator (random.org). The participants and the lecturers sign up for the course without knowing the course setting (online or onsite) until 3–4 weeks before the course.
Assignment of interventions: blinding
Due to the nature of the study, it is not possible to blind trial participants or lecturers. The outcomes are reported by the participants directly in an online form, thus being blinded for the outcome assessor, but not for the individual participant. The data collection for the long-term follow-up regarding academic achievements is conducted without blinding. However, the external researcher analysing the data will be blinded.
Data collection and management
Data will be collected by the project leader (Table 1 ). Baseline variables and post course knowledge, motivation, and self-efficacy are self-reported through questionnaires in SurveyXact® [ 27 ]. Academic achievements are collected through Google Scholar profiles of the participants.
Given that we are using participant assessments and evaluations for research purposes, all data collection – except for monthly follow-up of academic achievements after the course – takes place either in the immediate beginning or ending of the course and therefore we expect participant retention to be high.
Data will be downloaded from SurveyXact and stored in a locked and logged drive on a computer belonging to the Capital Region of Denmark. Only the project leader has access to the data.
This project conduct is following the Danish Data Protection Agency guidelines of the European GDPR throughout the trial. Following the end of the trial, data will be stored at the Danish National Data Archive which fulfil Danish and European guidelines for data protection and management.
Statistical methods
Data is anonymized and blinded before the analyses. Analyses are performed by a researcher not otherwise involved in the inclusion or randomization, data collection or handling. All statistical tests will be testing the null hypotheses assuming the two arms of the trial being equal based on corresponding estimates. Analysis of primary outcome on short-term learning will be started once all data has been collected for all individuals in the last included course. Analyses of long-term academic achievement will be started at end of follow-up.
Baseline characteristics including both course- and individual level information will be presented. Table 2 presents the available data on baseline.
We will use multivariate analysis for identification of the most important predictors (motivation, self-efficacy, sex, educational background, and knowledge) for best effect on short and long term. The results will be presented as risk ratio (RR) with 95% confidence interval (CI). The results will be considered significant if CI does not include the value one.
All data processing and analyses were conducted using R statistical software version 4.1.0, 2021–05-18 (R Foundation for Statistical Computing, Vienna, Austria).
If possible, all analysis will be performed for “Practical Course in Systematic Review Technique in Clinical Research” and for “Getting started: Writing your first manuscript for publication” separately.
Primary analyses will be handled with the intention-to-treat approach. The analyses will include all individuals with valid data regardless of they did attend the complete course. Missing data will be handled with multiple imputation [ 28 ] .
Upon reasonable request, public assess will be granted to protocol, datasets analysed during the current study, and statistical code Table 3 .
Oversight, monitoring, and adverse events
This project is coordinated in collaboration between the WHO CC (DEN-62) at the Parker Institute, CAMES, and the PhD School at the Faculty of Health and Medical Sciences, University of Copenhagen. The project leader runs the day-to-day support of the trial. The steering committee of the trial includes principal investigators from WHO CC (DEN-62) and CAMES and the project leader and meets approximately three times a year.
Data monitoring is done on a daily basis by the project leader and controlled by an external independent researcher.
An adverse event is “a harmful and negative outcome that happens when a patient has been provided with medical care” [ 29 ]. Since this trial does not involve patients in medical care, we do not expect adverse events. If participants decline taking part in the course after receiving the information of the course setting, information on reason for declining is sought obtained. If the reason is the setting this can be considered an unintended effect. Information of unintended effects of the online setting (the intervention) will be recorded. Participants are encouraged to contact the project leader with any response to the course in general both during and after the course.
The trial description has been sent to the Scientific Ethical Committee of the Capital Region of Denmark (VEK) (21041907), which assessed it as not necessary to notify and that it could proceed without permission from VEK according to the Danish law and regulation of scientific research. The trial is registered with the Danish Data Protection Agency (Privacy) (P-2022–158). Important protocol modification will be communicated to relevant parties as well as VEK, the Joint Regional Information Security and Clinicaltrials.gov within an as short timeframe as possible.
Dissemination plans
The results (positive, negative, or inconclusive) will be disseminated in educational, scientific, and clinical fora, in international scientific peer-reviewed journals, and clinicaltrials.gov will be updated upon completion of the trial. After scientific publication, the results will be disseminated to the public by the press, social media including the website of the hospital and other organizations – as well as internationally via WHO CC (DEN-62) at the Parker Institute and WHO Europe.
All authors will fulfil the ICMJE recommendations for authorship, and RR will be first author of the articles as a part of her PhD dissertation. Contributors who do not fulfil these recommendations will be offered acknowledgement in the article.
This cluster randomized trial investigates if an onsite setting of a research course for PhD students within the health and medical sciences is different from an online setting. The outcomes measured are learning of research methodology (primary), preference, motivation, and self-efficacy (secondary) on short term and academic achievements (secondary) on long term.
The results of this study will be discussed as follows:
Discussion of primary outcome
Primary outcome will be compared and contrasted with similar studies including recent RCTs and mixed-method studies on online and onsite research methodology courses within health and medical education [ 10 , 11 , 30 ] and for inspiration outside the field [ 31 , 32 ]: Tokalic finds similar outcomes for online and onsite, Martinic finds that the web-based educational intervention improves knowledge, Cheung concludes that the evidence is insufficient to say that the two modes have different learning outcomes, Kofoed finds online setting to have negative impact on learning and Rahimi-Ardabili presents positive self-reported student knowledge. These conflicting results will be discussed in the context of the result on the learning outcome of this study. The literature may change if more relevant studies are published.
Discussion of secondary outcomes
Secondary significant outcomes are compared and contrasted with similar studies.
Limitations, generalizability, bias and strengths
It is a limitation to this study, that an onsite curriculum for a full day is delivered identically online, as this may favour the onsite course due to screen fatigue [ 33 ]. At the same time, it is also a strength that the time schedules are similar in both settings. The offer of coffee, tea, water, and a plain sandwich in the onsite course may better facilitate the possibility for socializing. Another limitation is that the study is performed in Denmark within a specific educational culture, with institutional policies and resources which might affect the outcome and limit generalization to other geographical settings. However, international students are welcome in the class.
In educational interventions it is generally difficult to blind participants and this inherent limitation also applies to this trial [ 11 ]. Thus, the participants are not blinded to their assigned intervention, and neither are the lecturers in the courses. However, the external statistical expert will be blinded when doing the analyses.
We chose to compare in-person onsite setting with a synchronous online setting. Therefore, the online setting cannot be expected to generalize to asynchronous online setting. Asynchronous delivery has in some cases showed positive results and it might be because students could go back and forth through the modules in the interface without time limit [ 11 ].
We will report on all the outcomes defined prior to conducting the study to avoid selective reporting bias.
It is a strength of the study that it seeks to report outcomes within the 1, 2 and 4 levels of the Kirkpatrick conceptual framework, and not solely on level 1. It is also a strength that the study is cluster randomized which will reduce “infections” between the two settings and has an adequate power calculated sample size and looks for a relevant educational difference of 20% between the online and onsite setting.
Perspectives with implications for practice
The results of this study may have implications for the students for which educational setting they choose. Learning and preference results has implications for lecturers, course managers and curriculum developers which setting they should plan for the health and medical education. It may also be of inspiration for teaching and training in other disciplines. From a societal perspective it also has implications because we will know the effect and preferences of online learning in case of a future lock down.
Future research could investigate academic achievements in online and onsite research training on the long run (Kirkpatrick 4); the effect of blended learning versus online or onsite (Kirkpatrick 2); lecturers’ preferences for online and onsite setting within health and medical education (Kirkpatrick 1) and resource use in synchronous and asynchronous online learning (Kirkpatrick 5).
Trial status
This trial collected pilot data from August to September 2021 and opened for inclusion in January 2022. Completion of recruitment is expected in April 2024 and long-term follow-up in April 2026. Protocol version number 1 03.06.2022 with amendments 30.11.2023.
Availability of data and materials
The project leader will have access to the final trial dataset which will be available upon reasonable request. Exception to this is the qualitative raw data that might contain information leading to personal identification.
Abbreviations
Artificial Intelligence
Copenhagen academy for medical education and simulation
Confidence interval
Coronavirus disease
European credit transfer and accumulation system
International committee of medical journal editors
Intrinsic motivation inventory
Multiple choice questionnaire
Doctor of medicine
Masters of sciences
Randomized controlled trial
Scientific ethical committee of the Capital Region of Denmark
WHO Collaborating centre for evidence-based clinical health promotion
Samara M, Algdah A, Nassar Y, Zahra SA, Halim M, Barsom RMM. How did online learning impact the academic. J Technol Sci Educ. 2023;13(3):869–85.
Article Google Scholar
Nejadghaderi SA, Khoshgoftar Z, Fazlollahi A, Nasiri MJ. Medical education during the coronavirus disease 2019 pandemic: an umbrella review. Front Med (Lausanne). 2024;11:1358084. https://doi.org/10.3389/fmed.2024.1358084 .
Madi M, Hamzeh H, Abujaber S, Nawasreh ZH. Have we failed them? Online learning self-efficacy of physiotherapy students during COVID-19 pandemic. Physiother Res Int. 2023;5:e1992. https://doi.org/10.1002/pri.1992 .
Torda A. How COVID-19 has pushed us into a medical education revolution. Intern Med J. 2020;50(9):1150–3.
Alhat S. Virtual Classroom: A Future of Education Post-COVID-19. Shanlax Int J Educ. 2020;8(4):101–4.
Cook DA, Levinson AJ, Garside S, Dupras DM, Erwin PJ, Montori VM. Internet-based learning in the health professions: A meta-analysis. JAMA. 2008;300(10):1181–96. https://doi.org/10.1001/jama.300.10.1181 .
Pei L, Wu H. Does online learning work better than offline learning in undergraduate medical education? A systematic review and meta-analysis. Med Educ Online. 2019;24(1):1666538. https://doi.org/10.1080/10872981.2019.1666538 .
Richmond H, Copsey B, Hall AM, Davies D, Lamb SE. A systematic review and meta-analysis of online versus alternative methods for training licensed health care professionals to deliver clinical interventions. BMC Med Educ. 2017;17(1):227. https://doi.org/10.1186/s12909-017-1047-4 .
George PP, Zhabenko O, Kyaw BM, Antoniou P, Posadzki P, Saxena N, Semwal M, Tudor Car L, Zary N, Lockwood C, Car J. Online Digital Education for Postregistration Training of Medical Doctors: Systematic Review by the Digital Health Education Collaboration. J Med Internet Res. 2019;21(2):e13269. https://doi.org/10.2196/13269 .
Tokalić R, Poklepović Peričić T, Marušić A. Similar Outcomes of Web-Based and Face-to-Face Training of the GRADE Approach for the Certainty of Evidence: Randomized Controlled Trial. J Med Internet Res. 2023;25:e43928. https://doi.org/10.2196/43928 .
Krnic Martinic M, Čivljak M, Marušić A, Sapunar D, Poklepović Peričić T, Buljan I, et al. Web-Based Educational Intervention to Improve Knowledge of Systematic Reviews Among Health Science Professionals: Randomized Controlled Trial. J Med Internet Res. 2022;24(8): e37000.
https://www.mentimeter.com/ . Accessed 4 Dec 2023.
https://www.sendsteps.com/en/ . Accessed 4 Dec 2023.
https://da.padlet.com/ . Accessed 4 Dec 2023.
Zackoff MW, Real FJ, Abramson EL, Li STT, Klein MD, Gusic ME. Enhancing Educational Scholarship Through Conceptual Frameworks: A Challenge and Roadmap for Medical Educators. Acad Pediatr. 2019;19(2):135–41. https://doi.org/10.1016/j.acap.2018.08.003 .
https://zoom.us/ . Accessed 20 Aug 2024.
Raffing R, Larsen S, Konge L, Tønnesen H. From Targeted Needs Assessment to Course Ready for Implementation-A Model for Curriculum Development and the Course Results. Int J Environ Res Public Health. 2023;20(3):2529. https://doi.org/10.3390/ijerph20032529 .
https://www.kirkpatrickpartners.com/the-kirkpatrick-model/ . Accessed 12 Dec 2023.
Smidt A, Balandin S, Sigafoos J, Reed VA. The Kirkpatrick model: A useful tool for evaluating training outcomes. J Intellect Dev Disabil. 2009;34(3):266–74.
Campbell K, Taylor V, Douglas S. Effectiveness of online cancer education for nurses and allied health professionals; a systematic review using kirkpatrick evaluation framework. J Cancer Educ. 2019;34(2):339–56.
Miller GE. The assessment of clinical skills/competence/performance. Acad Med. 1990;65(9 Suppl):S63–7.
Ryan RM, Deci EL. Self-Determination Theory and the Facilitation of Intrinsic Motivation, Social Development, and Well-Being. Am Psychol. 2000;55(1):68–78. https://doi.org/10.1037//0003-066X.55.1.68 .
Williams GM, Smith AP. Using single-item measures to examine the relationships between work, personality, and well-being in the workplace. Psychology. 2016;07(06):753–67.
https://scholar.google.com/intl/en/scholar/citations.html . Accessed 4 Dec 2023.
Rotondi MA. CRTSize: sample size estimation functions for cluster randomized trials. R package version 1.0. 2015. Available from: https://cran.r-project.org/package=CRTSize .
Random.org. Available from: https://www.random.org/
https://rambollxact.dk/surveyxact . Accessed 4 Dec 2023.
Sterne JAC, White IR, Carlin JB, Spratt M, Royston P, Kenward MG, et al. Multiple imputation for missing data in epidemiological and clinical research: Potential and pitfalls. BMJ (Online). 2009;339:157–60.
Google Scholar
Skelly C, Cassagnol M, Munakomi S. Adverse Events. StatPearls Treasure Island: StatPearls Publishing. 2023. Available from: https://www.ncbi.nlm.nih.gov/books/NBK558963/ .
Rahimi-Ardabili H, Spooner C, Harris MF, Magin P, Tam CWM, Liaw ST, et al. Online training in evidence-based medicine and research methods for GP registrars: a mixed-methods evaluation of engagement and impact. BMC Med Educ. 2021;21(1):1–14. Available from: https://www.ncbi.nlm.nih.gov/pmc/articles/PMC8439372/pdf/12909_2021_Article_2916.pdf .
Cheung YYH, Lam KF, Zhang H, Kwan CW, Wat KP, Zhang Z, et al. A randomized controlled experiment for comparing face-to-face and online teaching during COVID-19 pandemic. Front Educ. 2023;8. https://doi.org/10.3389/feduc.2023.1160430 .
Kofoed M, Gebhart L, Gilmore D, Moschitto R. Zooming to Class?: Experimental Evidence on College Students' Online Learning During Covid-19. SSRN Electron J. 2021;IZA Discussion Paper No. 14356.
Mutlu Aİ, Yüksel M. Listening effort, fatigue, and streamed voice quality during online university courses. Logop Phoniatr Vocol :1–8. Available from: https://doi.org/10.1080/14015439.2024.2317789
Download references
Acknowledgements
We thank the students who make their evaluations available for this trial and MSc (Public Health) Mie Sylow Liljendahl for statistical support.
Open access funding provided by Copenhagen University The Parker Institute, which hosts the WHO CC (DEN-62), receives a core grant from the Oak Foundation (OCAY-18–774-OFIL). The Oak Foundation had no role in the design of the study or in the collection, analysis, and interpretation of the data or in writing the manuscript.
Author information
Authors and affiliations.
WHO Collaborating Centre (DEN-62), Clinical Health Promotion Centre, The Parker Institute, Bispebjerg & Frederiksberg Hospital, University of Copenhagen, Copenhagen, 2400, Denmark
Rie Raffing & Hanne Tønnesen
Copenhagen Academy for Medical Education and Simulation (CAMES), Centre for HR and Education, The Capital Region of Denmark, Copenhagen, 2100, Denmark
You can also search for this author in PubMed Google Scholar
Contributions
RR, LK and HT have made substantial contributions to the conception and design of the work; RR to the acquisition of data, and RR, LK and HT to the interpretation of data; RR has drafted the work and RR, LK, and HT have substantively revised it AND approved the submitted version AND agreed to be personally accountable for their own contributions as well as ensuring that any questions which relates to the accuracy or integrity of the work are adequately investigated, resolved and documented.
Corresponding author
Correspondence to Rie Raffing .
Ethics declarations
Ethics approval and consent to participate.
The Danish National Committee on Health Research Ethics has assessed the study Journal-nr.:21041907 (Date: 21–09-2021) without objections or comments. The study has been approved by The Danish Data Protection Agency Journal-nr.: P-2022–158 (Date: 04.05.2022).
All PhD students participate after informed consent. They can withdraw from the study at any time without explanations or consequences for their education. They will be offered information of the results at study completion. There are no risks for the course participants as the measurements in the course follow routine procedure and they are not affected by the follow up in Google Scholar. However, the 15 min of filling in the forms may be considered inconvenient.
The project will follow the GDPR and the Joint Regional Information Security Policy. Names and ID numbers are stored on a secure and logged server at the Capital Region Denmark to avoid risk of data leak. All outcomes are part of the routine evaluation at the courses, except the follow up for academic achievement by publications and related indexes. However, the publications are publicly available per se.
Competing interests
The authors declare no competing interests
Additional information
Publisher’s note.
Springer Nature remains neutral with regard to jurisdictional claims in published maps and institutional affiliations.
Supplementary Information
Supplementary material 1., supplementary material 2., supplementary material 3., supplementary material 4., supplementary material 5., supplementary material 6., supplementary material 7., rights and permissions.
Open Access This article is licensed under a Creative Commons Attribution 4.0 International License, which permits use, sharing, adaptation, distribution and reproduction in any medium or format, as long as you give appropriate credit to the original author(s) and the source, provide a link to the Creative Commons licence, and indicate if changes were made. The images or other third party material in this article are included in the article's Creative Commons licence, unless indicated otherwise in a credit line to the material. If material is not included in the article's Creative Commons licence and your intended use is not permitted by statutory regulation or exceeds the permitted use, you will need to obtain permission directly from the copyright holder. To view a copy of this licence, visit http://creativecommons.org/licenses/by/4.0/ . The Creative Commons Public Domain Dedication waiver ( http://creativecommons.org/publicdomain/zero/1.0/ ) applies to the data made available in this article, unless otherwise stated in a credit line to the data.
Reprints and permissions
About this article
Cite this article.
Raffing, R., Konge, L. & Tønnesen, H. Learning effect of online versus onsite education in health and medical scholarship – protocol for a cluster randomized trial. BMC Med Educ 24 , 927 (2024). https://doi.org/10.1186/s12909-024-05915-z
Download citation
Received : 25 March 2024
Accepted : 14 August 2024
Published : 26 August 2024
DOI : https://doi.org/10.1186/s12909-024-05915-z
Share this article
Anyone you share the following link with will be able to read this content:
Sorry, a shareable link is not currently available for this article.
Provided by the Springer Nature SharedIt content-sharing initiative
- Self-efficacy
- Achievements
- Health and Medical education
BMC Medical Education
ISSN: 1472-6920
- General enquiries: [email protected]

IMAGES
VIDEO
COMMENTS
In a hypothesis-driven research, specifications of methodology help the grant reviewers to differentiate good science from bad science, and thus, hypothesis-driven research is the most funded research. "Hypotheses aren't simply useful tools in some potentially outmoded vision of science; they are the whole point."
Some research is not hypothesis-driven. Terms used to describe non-hypothesis-driven research are 'descriptive research,' in which information is collected without a particular question in mind, and 'discovery science,' where large volumes of experimental data are analyzed with the goal of finding new patterns or correlations.
Traditionalists argue that in purely data-driven methods, one may not know where to look for those interesting findings if no hypotheses were formed beforehand. Big data advocates, on the other hand, argue that with no prior beliefs, one is not constrained by established ways of thinking or doing, opening the possibilities of breakthrough ...
This statement is based on background research and current knowledge.8,9 The research hypothesis makes a specific prediction about a new phenomenon10 or a formal statement on the expected relationship between an independent variable and a dependent variable.3,11 It provides a tentative answer to the research question to be tested or explored.4
Secondary research is based on already published literature and does not directly involve human or animal subjects. Review articles are generated by secondary research. ... may not be hypothesis-driven, but form fertile ground to generate future hypotheses for evaluation. Fig. 1 summarizes which type of studies are hypothesis-driven and which ...
The traditional scientific method: Hypothesis-driven deduction. Research is the undisputed core activity defining science. Without research, the advancement of scientific knowledge would come to a screeching halt. While it is evident that researchers look for new information or insights, the term "research" is somewhat puzzling.
Hypothesis generation is an early and critical step in any hypothesis-driven clinical research project. Because it is not yet a well-understood cognitive process, the need to improve the process goes unrecognized. Without an impactful hypothesis, the significance of any research project can be questionable, regardless of the rigor or diligence applied in other steps of the study, e.g., study ...
Practicing Hypothesis-Driven Development is thinking about the development of new ideas, products and services - even organizational change - as a series of experiments to determine whether an expected outcome will be achieved. The process is iterated upon until a desirable outcome is obtained or the idea is determined to be not viable.
A research hypothesis (also called a scientific hypothesis) is a statement about the expected outcome of a study (for example, a dissertation or thesis). To constitute a quality hypothesis, the statement needs to have three attributes - specificity, clarity and testability. Let's take a look at these more closely.
This is a clear case of hypothesis-driven research, but it has strong impact for application: if the movement is driven by the matrix, the actomyosin system will provide molecular targets for pharmaceutical inhibition of cell proliferation (Forer et al. 2007 ), which is of high relevance for the search of novel anticancer compounds.
In a return to hypothesis-driven research, systems biologists are attempting to use the same 'omics' methods to generate data for use in quantitative biological models. Hypotheses are needed ...
The research hypothesis summarizes the elements of the study: the sample, the sample size, the design, the predictor and the outcome variables. The primary necessity of stating the. hypothesis is ...
This paper has had two goals. The first has been to propose revisions to the framework of Ratti ( 2015) for the study of the role of hypothesis-driven research in large-scale contemporary biological studies, in light of studies such as GWAS and its associated missing heritability problem.
In a hypothesis-driven research, specifications of methodology help the grant reviewers to differentiate good science from bad science, and thus, hypothesis-driven research is the most funded research. "Hypotheses aren't simply useful tools in some potentially outmoded vision of science; they are the whole point."
In this course, you will learn about the hypothesis-driven approach to problem-solving. This approach originated from academic research and later adopted in management consulting. The course consists of 4 modules that take you through a step-by-step process of solving problems in an effective and timely manner.
Such concerns actually reflect deeper and more widespread debates about the centrality of hypothesis-driven research that have challenged the scientific community for centuries. Most contemporary scientific disciplines share a commitment to a hypothesis-driven methodology (see Peters R 1991, Weinberg 2010, Keating and Cambrosio 2012, Fudge 2014).
5. Phrase your hypothesis in three ways. To identify the variables, you can write a simple prediction in if…then form. The first part of the sentence states the independent variable and the second part states the dependent variable. If a first-year student starts attending more lectures, then their exam scores will improve.
In hypothesis-driven research, we basically come up with a hypothesis that might explain a certain phenomenon. The hypothesis is usually based on doing prior research (published research or work in your own laboratory) and requires that you read, analyze and come up with a new idea. Or your supervisor may have done this for you.
In research and data analysis, a hypothesis-driven approach is one of the main methods for using data to test and, ultimately, prove (or disprove) assertions. To do that, researchers collect a sufficient amount of data on the subject and then approach it with a specific hypothesis in mind. ... 2015-04-16-using-a-hypothesis-driven-approach-in ...
It represented a shift away from the traditional paradigm of hypothesis-driven research but kept the individual investigator-initiated focus that NIH has historically and traditionally supported. It brought together individuals from multiple and historically disparate communities, institutions, and organizations in order to focus their ...
New Phytologist is an international journal owned by the New Phytologist Foundation publishing original research in plant science and its applications. This article is a Commentary on Böttner et al. (2023), 239: 1475-1489. ... Hypothesis-driven approach to build multiple lines of evidence based on previous observations. Testing a hypothesis ...
Research hypothesis. The primary research question should be driven by the hypothesis rather than the data. 1, 2 That is, the research question and hypothesis should be developed before the start of the study. This sounds intuitive; however, if we take, for example, a database of information, it is potentially possible to perform multiple ...
paper argues that hypothesis-driven and data-driven research work together to inform the research process. At the core of these approaches are theoretical underpinnings that drive progress in the field. Here, we present several exemplars of research on the gut-brain axis that outline the innate values and challenges of these approaches. As nurses are trained to integrate multiple body systems ...
In order to achieve the objectives of this study, this paper is organized as follows. First, the research gaps and divergent and conflicting perspectives of the existing literature are analyzed. Second, the relationship between IT innovation and innovation resistance is explained based on the psychological state of organizational members.
Based on the authors experience with conducting courses during the pandemic, the hypothesis is that student preferred onsite setting is different to online setting. Cluster randomized trial with two parallel groups. ... Recently solid RCTs have been performed on the topic of web-based theoretical learning of research methods among health ...
Section on data and research design introduces our dataset, ... The ideology-driven hypothesis. In our second hypothesis we argued that the distance on the environmental issue in predicting voting behaviour will have a more pronounced impact for leftist voters. In this respect, the interaction between the party-voter distance on the ...