- Introduction
- Conclusions
- Article Information
RCT indicates randomized clinical trial; RR, risk ratio. Diamonds indicate heterogeneity; different marker sizes, weights.
MD indicates mean difference; RCT, randomized clinical trial; RR, risk ratio; and USD, US dollars. Diamonds indicate heterogeneity; different marker sizes, weights.
eTable 1. Search Strategy and Result
eTable 2. Reasons for Excluding Full-Text Screening Studies
eTable 3. Inclusion and Exclusion Criteria and Care Bundle or Suggestions for Enrolled Studies
eTable 4. Trial Sequential Analysis With Only Enrolled Randomized Clinical Trials
eTable 5. Sensitivity Analysis
eTable 6. Summary of Certainty of Evidence Assessment
eFigure 1. PRISMA Flow Diagram
eFigure 2. Version 2 of the Cochrane Risk-of-Bias Tool for Randomized Trials (RoB 2.0) Assessment of Included Studies and Summary
eFigure 3. Risk of Bias in Nonrandomized Studies of Interventions (ROBINS-I) Assessment of Included Studies and Summary
eFigure 4. Forest Plot Illustrating the Association of Acute Kidney Injury (AKI) Electronic Alerts (e-Alerts) With Dialysis and Kidney Recovery after AKI
eFigure 5. Forest Plot Illustrating the Association of Acute Kidney Injury (AKI) Electronic Alerts (e-Alerts) With ACEI/ARB Prescription and Fluid Prescription After AKI
eFigure 6. Subgroup Analysis for AKI Progression
eFigure 7. Subgroup Analysis for Mortality
eFigure 8. Subgroup Analysis for Dialysis
eFigure 9. Subgroup Analysis for Kidney Recovery
eFigure 10. Subgroup Analysis for Nephrologist Consultation
eFigure 11. Subgroup Analysis for NSAID Exposure After AKI
eFigure 12. Subgroup Analysis for Hospital Length of Stay
eFigure 13. Subgroup Analysis for Medical Costs
eFigure 14. Subgroup Analysis for AKI Documentation
eFigure 15. Subgroup Analysis for Fluid prescription
eFigure 16. Subgroup Analysis for ACEI/ARB exposure
eFigure 17. Subgroup Analysis for Mortality With Different Follow-Up Period
eFigure 18. Trial Sequential Analysis for Mortality
eFigure 19. Trial Sequential Analysis for AKI Progression
eFigure 20. Trial Sequential Analysis for Dialysis
eFigure 21. Trial Sequential Analysis for Kidney Recovery
eFigure 22. Trial Sequential Analysis for Nephrologist Consultation
eFigure 23. Trial Sequential Analysis for NSAID Exposure After AKI
eFigure 24. Trial Sequential Analysis for AKI documentation
eFigure 25. Funnel Plots
eAppendix 1. Supplemental Method for Trial Sequential Analysis
eAppendix 2. Quality of Included Studies
eAppendix 3. Certainty of Evidence Assessment for AKI Progression
Data Sharing Statement

See More About
Sign up for emails based on your interests, select your interests.
Customize your JAMA Network experience by selecting one or more topics from the list below.
- Academic Medicine
- Acid Base, Electrolytes, Fluids
- Allergy and Clinical Immunology
- American Indian or Alaska Natives
- Anesthesiology
- Anticoagulation
- Art and Images in Psychiatry
- Artificial Intelligence
- Assisted Reproduction
- Bleeding and Transfusion
- Caring for the Critically Ill Patient
- Challenges in Clinical Electrocardiography
- Climate and Health
- Climate Change
- Clinical Challenge
- Clinical Decision Support
- Clinical Implications of Basic Neuroscience
- Clinical Pharmacy and Pharmacology
- Complementary and Alternative Medicine
- Consensus Statements
- Coronavirus (COVID-19)
- Critical Care Medicine
- Cultural Competency
- Dental Medicine
- Dermatology
- Diabetes and Endocrinology
- Diagnostic Test Interpretation
- Drug Development
- Electronic Health Records
- Emergency Medicine
- End of Life, Hospice, Palliative Care
- Environmental Health
- Equity, Diversity, and Inclusion
- Facial Plastic Surgery
- Gastroenterology and Hepatology
- Genetics and Genomics
- Genomics and Precision Health
- Global Health
- Guide to Statistics and Methods
- Hair Disorders
- Health Care Delivery Models
- Health Care Economics, Insurance, Payment
- Health Care Quality
- Health Care Reform
- Health Care Safety
- Health Care Workforce
- Health Disparities
- Health Inequities
- Health Policy
- Health Systems Science
- History of Medicine
- Hypertension
- Images in Neurology
- Implementation Science
- Infectious Diseases
- Innovations in Health Care Delivery
- JAMA Infographic
- Law and Medicine
- Leading Change
- Less is More
- LGBTQIA Medicine
- Lifestyle Behaviors
- Medical Coding
- Medical Devices and Equipment
- Medical Education
- Medical Education and Training
- Medical Journals and Publishing
- Mobile Health and Telemedicine
- Narrative Medicine
- Neuroscience and Psychiatry
- Notable Notes
- Nutrition, Obesity, Exercise
- Obstetrics and Gynecology
- Occupational Health
- Ophthalmology
- Orthopedics
- Otolaryngology
- Pain Medicine
- Palliative Care
- Pathology and Laboratory Medicine
- Patient Care
- Patient Information
- Performance Improvement
- Performance Measures
- Perioperative Care and Consultation
- Pharmacoeconomics
- Pharmacoepidemiology
- Pharmacogenetics
- Pharmacy and Clinical Pharmacology
- Physical Medicine and Rehabilitation
- Physical Therapy
- Physician Leadership
- Population Health
- Primary Care
- Professional Well-being
- Professionalism
- Psychiatry and Behavioral Health
- Public Health
- Pulmonary Medicine
- Regulatory Agencies
- Reproductive Health
- Research, Methods, Statistics
- Resuscitation
- Rheumatology
- Risk Management
- Scientific Discovery and the Future of Medicine
- Shared Decision Making and Communication
- Sleep Medicine
- Sports Medicine
- Stem Cell Transplantation
- Substance Use and Addiction Medicine
- Surgical Innovation
- Surgical Pearls
- Teachable Moment
- Technology and Finance
- The Art of JAMA
- The Arts and Medicine
- The Rational Clinical Examination
- Tobacco and e-Cigarettes
- Translational Medicine
- Trauma and Injury
- Treatment Adherence
- Ultrasonography
- Users' Guide to the Medical Literature
- Vaccination
- Venous Thromboembolism
- Veterans Health
- Women's Health
- Workflow and Process
- Wound Care, Infection, Healing
Get the latest research based on your areas of interest.
Others also liked.
- Download PDF
- X Facebook More LinkedIn
Chen J , Lee T , Chan M, et al. Electronic Alert Systems for Patients With Acute Kidney Injury : A Systematic Review and Meta-Analysis . JAMA Netw Open. 2024;7(8):e2430401. doi:10.1001/jamanetworkopen.2024.30401
Manage citations:
© 2024
- Permissions
Electronic Alert Systems for Patients With Acute Kidney Injury : A Systematic Review and Meta-Analysis
- 1 Kidney Research Center, Department of Nephrology, Chang Gung Memorial Hospital, Linkou Branch, Taoyuan, Taiwan
- 2 College of Medicine, Chang Gung University, Taoyuan, Taiwan
- 3 Department of Nephrology, Chansn Hospital, Taoyuan City, Taiwan
- 4 Department of Internal Medicine, National Taiwan University Hospital, Taipei, Taiwan
- 5 National Taiwan University Study Group on Acute Renal Failure, Taipei, Taiwan
- 6 Institute of Epidemiology and Preventive Medicine, College of Public Health, National Taiwan University
Question Are electronic alerts (e-alerts) for acute kidney injury (AKI) in the electronic health record associated with patient outcomes or clinical practice patterns?
Findings In this systematic review and meta-analysis of 13 unique studies with 41 837 unique patients, AKI e-alerts were not associated with a lower risk for mortality but were associated with a lower risk for AKI progression compared with standard care. There were associations between e-alerts and clinical practice patterns, including increased nephrologist consultations, dialysis, and AKI documentation and decreased post-AKI exposure to nonsteroidal anti-inflammatory drugs.
Meaning These findings suggest that AKI e-alerts are associated with changes to clinical practice patterns and lower risk for AKI progression, although more research is needed to support this conclusion.
Importance The acute kidney injury (AKI) electronic alert (e-alert) system was hypothesized to improve the outcomes of AKI. However, its association with different patient outcomes and clinical practice patterns remains systematically unexplored.
Objective To assess the association of AKI e-alerts with patient outcomes (mortality, AKI progression, dialysis, and kidney recovery) and clinical practice patterns.
Data Sources A search of Embase and PubMed on March 18, 2024, and a search of the Cochrane Library on March 20, 2024, to identify all relevant studies. There were no limitations on language or article types.
Study Selection Studies evaluating the specified outcomes in adult patients with AKI comparing AKI e-alerts with standard care or no e-alerts were included. Studies were excluded if they were duplicate cohorts, had insufficient outcome data, or had no control group.
Data Extraction and Synthesis Two investigators independently extracted data and assessed bias. The systematic review and meta-analysis followed the PRISMA guidelines. Random-effects model meta-analysis, with predefined subgroup analysis and trial sequential analyses, were conducted.
Main Outcomes and Measures Primary outcomes included mortality, AKI progression, dialysis, and kidney recovery. Secondary outcomes were nephrologist consultations, post-AKI exposure to nonsteroidal anti-inflammatory drugs (NSAID), post-AKI angiotensin-converting enzyme inhibitor and/or angiotensin receptor blocker (ACEI/ARB) prescription, hospital length of stay, costs, and AKI documentation.
Results Thirteen unique studies with 41 837 unique patients were included (mean age range, 60.5-79.0 years]; 29.3%-48.5% female). The risk ratios (RRs) for the AKI e-alerts group compared with standard care were 0.96 for mortality (95% CI, 0.89-1.03), 0.91 for AKI stage progression (95% CI, 0.84-0.99), 1.16 for dialysis (95% CI, 1.05-1.28), and 1.13 for kidney recovery (95% CI, 0.86-1.49). The AKI e-alerts group had RRs of 1.45 (95% CI, 1.04-2.02) for nephrologist consultation, 0.75 (95% CI, 0.59-0.95) for post-AKI NSAID exposure. The pooled RR for post-AKI ACEI/ARB exposure in the AKI e-alerts group compared with the control group was 0.91 (95% CI, 0.78-1.06) and 1.28 (95% CI, 1.04-1.58) for AKI documentation. Use of AKI e-alerts was not associated with lower hospital length of stay (mean difference, −0.09 [95% CI, −0.47 to 0.30] days) or lower cost (mean difference, US $655.26 [95% CI, −$656.98 to $1967.5]) but was associated with greater AKI documentation (RR, 1.28 [95% CI, 1.04-1.58]). Trial sequential analysis confirmed true-positive results of AKI e-alerts on increased nephrologist consultations and reduced post-AKI NSAID exposure and its lack of association with mortality.
Conclusions and Relevance In this systematic review and meta-analysis, AKI e-alerts were not associated with a lower risk for mortality but were associated with changes in clinical practices. They were associated with lower risk for AKI progression. Further research is needed to confirm these results and integrate early AKI markers or prediction models to improve outcomes.
Acute kidney injury (AKI) is a common complication in hospitalized patients, leading to increased comorbidities, health care costs, and both short- and long-term mortality. 1 - 3 The introduction of electronic health record systems has enabled early detection of AKI through electronic alerts (e-alerts), considered potential interventions to reduce AKI-related complications and improve outcomes. Consequently, the AKI e-alert system was initially launched in the US and the United Kingdom, later expanding globally. 4 - 7
A 2012 study by Colpaert et al 8 using RIFLE (risk, injury, failure, loss of kidney function, and end-stage kidney disease) criteria showed that AKI e-alerts could enhance short-term renal outcomes and timely interventions. The 27th Acute Disease Quality Initiative consensus also highlighted that “AKI alerts driven by concrete criteria improve early detection and prompt AKI management.” 9 Nevertheless, a 2017 published meta-analysis 6 and subsequent randomized clinical trials (RCTs) and non-RCTs, including Electronic Alerting for Acute Kidney Injury Amelioration (ELAIA)–1 10 and ELAIA-2, 11 questioned their impact on mortality. Despite assumptions about their efficacy in improving AKI outcomes and care, it remains uncertain whether AKI e-alerts, alone or with care bundles, are associated with lower mortality, AKI severity, or the need for kidney replacement or whether they impact clinical practices.
Given the lack of systematic analysis for several associated outcomes, an updated meta-analysis including recently published studies 10 - 12 is warranted. In the present study, we performed a systematic review and meta-analysis, incorporating subgroup analysis and trial sequential analysis using evidence-based medicine methods to assess the association between AKI e-alerts and patient survival, kidney outcomes, clinical practice patterns, and associated outcomes such as medical costs and hospital length of stay (LOS).
This systematic review and meta-analysis was performed in accordance with the Preferred Reporting Items for Systematic Reviews and Meta-Analyses ( PRISMA ) statement and checklist. We registered the protocol in PROSPERO ( CRD42024527189 ). Two investigators (J.-J.C. and T.-H.L.) systematically and independently conducted a review of published data on outcomes in patients with AKI e-alerts. A search of PubMed and Embase was performed on March 18, 2024, and the Cochrane Library was searched on March 20, 2024, to identify all relevant studies. Detailed search strategies, including search terms specific to each source, are provided in eTable 1 in Supplement 1 . There were no limitations on language or article types.
After removing duplicates, titles and abstracts were screened by 2 reviewers (J.-J.C. and T.-H.L.) for relevance. Full texts of potentially relevant articles were then reviewed for eligibility. Inclusion criteria required studies to involve adults, compare AKI e-alert groups with non–e-alert groups, and report on any of the primary or secondary outcomes. For eligibility disagreements, a third reviewer (C.-H.C.) was consulted for consensus. Exclusions were made for duplicate cohorts, insufficient outcome data, or absence of a control group.
The 2 investigators (J.-J.C. and T.-H.L.) independently extracted data (author[s], publication year, design, location, AKI care bundle presence, sample size, AKI criteria, mean age, proportion of population that was female) and outcomes from each study. For binary outcomes, participant and event numbers were noted; for continuous outcomes, mean and SD were extracted or calculated from median (IQR). Discrepancies were resolved through discussion with a third investigator (P.-C.F.).
This systematic review and meta-analysis evaluated the differences between AKI e-alerts vs standard care or no e-alerts for patient outcomes or clinical practice patterns. Primary outcomes included mortality and dialysis after AKI (prioritizing 28-day or 30-day, then 60-day, 90-day, and in-hospital mortality and dialysis), AKI stage progression, and kidney recovery after AKI. Secondary outcomes were nephrologist consultations, post-AKI exposure to nonsteroidal anti-inflammatory drugs (NSAIDs), post-AKI angiotensin-converting enzyme inhibitor and/or angiotensin receptor blocker (ACEI/ARB) prescription, AKI documentation, post-AKI intravenous fluid prescription, hospital LOS, and medical costs.
In the R meta package, the metabin and metacont functions were used for binary and continuous outcomes, respectively. 13 We applied a random-effects model using the inverse variance method. Between-study variance was estimated using the restricted maximum-likelihood estimator method, while the DerSimonian and Laird method estimated the 95% CI of the effect. We assessed the overall effect using pooled risk ratios (RRs) for binary outcomes and mean differences for continuous outcomes. Heterogeneity was evaluated with the I 2 statistic. Small study bias was examined using funnel plots and the Egger test via the metabias function. 14 Analyses were conducted in R, version 4.2.2 (R Program for Statistical Computing [October 31, 2022]), with 2-sided P < .05 considered statistically significant.
In our analysis, we differentiated studies as RCTs vs non-RCTs. We hypothesized that AKI e-alerts, combined with care recommendations or bundles, might be associated with patient outcomes. To explore this, we performed a subgroup analysis, dividing studies into those using e-alerts with AKI care bundles or recommendations and those using e-alerts alone. For studies reporting mortality outcomes over different time periods, we additionally conducted a subgroup analysis based on the specific time period.
To determine whether the primary outcome conclusions of our meta-analysis were premature, we performed trial sequential analysis (TSA) using TSA software, version 0.9.5.10 beta. 15 A more detailed description is found in eAppendix 1 in Supplement 1 .
Considering that the traditional DerSimonian and Laird method might underestimate between-study heterogeneity and the relatively small number of enrolled studies, we performed sensitivity analyses for binary outcomes using the Hartung-Knapp method and beta-binomial bayesian meta-analysis. The beta-binomial bayesian meta-analysis was conducted using R software and the JAGS (Just Another Gibbs Sampler) program, version 4.3.2 (GNU General Public License). Additionally, we conducted further TSA including only RCTs for both primary and secondary outcomes that showed associations in the conventional meta-analysis.
We assessed the risk of bias using RoB 2.0 (a revised tool to assess risk of bias in randomized trials) 16 and ROBINS-I tool (Risk of Bias in Nonrandomized Studies of Interventions) 17 for included RCTs and non-RCTs, respectively. Two independent reviewers (J.-J.C. and T.-H.L.) assessed the bias according to each domain, and the disagreements between the reviewers were resolved by discussion with another author (P.-C.F.). The quality of evidence was evaluated based on the guidelines of the GRADE (Grades of Recommendation, Assessment, Development, and Evaluation) Working Group. 18 , 19
A flowchart of the literature search is provided in eFigure 1 in Supplement 1 ). The electronic database search identified 189 potentially eligible studies from PubMed, 98 from Embase, and 42 from the Cochrane Library. After removing duplicate articles, the remaining 259 articles were screened. After screening the titles and abstracts, the full texts of 34 studies were reviewed to assess their eligibility. After excluding studies for various reasons (eTable 2 in Supplement 1 ), 13 unique studies including 41 837 unique patients 7 , 8 , 10 - 12 , 20 - 27 were included for analysis.
Table 1 summarizes the characteristics of the included studies. Patients’ mean ages varied from 60.5 to 79.0 years, with female representation between 29.3% and 48.5% and male representation between 51.5% and 70.7%. Among the 13 studies, 6 were RCTs, 10 - 12 , 23 , 26 , 27 4 were prospective cohort studies, 7 , 8 , 22 , 25 and 3 were retrospective cohort studies. 20 , 21 , 24 All but 1 study 8 adhered to Kidney Disease: Improving Global Outcomes (KDIGO) criteria for AKI, with the exception using RIFLE criteria. Additionally, 8 studies 7 , 11 , 12 , 20 - 23 , 25 provided AKI management recommendations or care bundles alongside AKI e-alerts. Further details on inclusion and exclusion criteria and AKI care recommendations are available in eTable 3 in Supplement 1 .
The RoB 2.0 and ROBINS-I assessments indicated varied risk of bias across the studies, with 8 of 13 studies presenting low to moderate risk. For RCTs, overall quality was ranked as low risk for 4 studies (66.7%), 10 - 12 , 26 of some concern for 1 study (16.7%), 27 and of high concern for 1 study (16.7%) 23 (eFigure 2 in Supplement 1 ). For non-RCTs, the overall quality was low risk for 1 study (14.3%), 20 of moderate concern for 3 studies (42.9%), 7 , 8 , 25 and of high concern for 3 studies (42.9%) 21 , 22 , 24 (eFigure 3 in Supplement 1 ) (see details in eAppendix 2 in Supplement 1 ).
In 12 studies with 38 644 participants, 7 , 8 , 10 - 12 , 20 - 23 , 25 - 27 the pooled mortality rate in the e-alert group was 15.7% (3041 of 19 409) and the pooled mortality rate in the control group was 16.7% (3220 of 19 235). Use of AKI e-alerts was not associated with a significant difference in mortality compared with the no use of AKI e-alerts, with substantial heterogeneity (RR, 0.96 [95% CI, 0.89-1.03]; I 2 = 47% [95% CI, 0%-73%]) ( Figure 1 A). There was no significant subgroup difference of pooled effects between RCTs and non-RCTs.
In 5 studies, 10 - 12 , 20 , 25 use of AKI e-alerts was associated with AKI stage progression (RR, 0.91 [95% CI, 0.84-0.99]), with substantial heterogeneity ( I 2 = 70% [95% CI, 23%-88%]). There was a significant subgroup difference of pooled effects between RCTs and non-RCTs ( Figure 1 B). All 5 studies reported AKI progression based on KDIGO criteria. Four studies defined AKI progression as advancing to a higher stage, except for Li et al. 12 Three studies 10 - 12 defined the period as in hospital, while 1 study 20 defined it within 7 days.
Use of AKI e-alerts was associated with dialysis (RR, 1.16 [95% CI, 1.05-1.28]), with substantial heterogeneity ( I 2 = 50% [95% CI, 1%-75%]) and without significant subgroup differences (eFigure 4 in Supplement 1 ). Use of AKI e-alerts was associated with kidney recovery (RR, 1.13 [95% CI, 0.86-1.49]), with high heterogeneity ( I 2 = 98% [95% CI, 97%-99%]) and without significant subgroup difference (eFigure 4 in Supplement 1 ).
Use of AKI e-alerts was associated with nephrologist consultation (RR, 1.45 [95% CI, 1.04-2.02]), with substantial heterogeneity ( I 2 = 95% [95% CI, 93%-97%]) and without significant subgroup difference ( Figure 2 A). Use of AKI e-alerts was associated with lower post-AKI NSAID exposure (RR, 0.75 [95% CI, 0.59-0.95]), with substantial heterogeneity ( I 2 = 69% [95% CI, 11%-89%]) ( Figure 2 B). The pooled RR for post-AKI ACEI/ARB exposure in the AKI e-alerts group compared with the control group was 0.91 (95% CI, 0.78-1.06), with substantial heterogeneity ( I 2 = 72% [95% CI, 19%-90%]) (eFigure 5 in Supplement 1 ). The pooled RR for post-AKI intravenous fluid prescription in the AKI e-alert group compared with the control group was 1.47 (95% CI, 0.86-2.54), with substantial heterogeneity ( I 2 = 97% [95% CI, 95%-98%]) and subgroup difference (eFigure 5 in Supplement 1 ).,
Use of AKI e-alerts was not associated with lower hospital LOS compared with the control group, with a mean difference of −0.09 (95% CI, −0.47 to 0.30) days and substantial heterogeneity ( I 2 = 62% [95% CI, 21%-81%]), without significant subgroup difference ( Figure 3 A). Use of AKI e-alerts was not associated with lower cost compared with the control group, with a mean difference of US $655.26 (95% CI, −$656.98 to $1967.5) and low heterogeneity ( I 2 = 45% [95% CI, 0%-84%]) ( Figure 3 B). Use of AKI e-alerts was associated with greater AKI documentation (RR, 1.28 [95% CI, 1.04-1.58]), with substantial heterogeneity ( I 2 = 94% [95% CI, 90%-96%]) ( Figure 3 C).
Subgroup analysis was performed by dividing enrolled studies into e-alerts in combination with an AKI care bundle or recommendation and those studies without. For AKI progression, studies with AKI e-alerts combined with AKI care bundle or recommendation had a lower RR compared with the non–e-alert groups (RR, 0.85 [95% CI, 0.77-0.93]; P = .03 for subgroup difference test) (eFigure 6 in Supplement 1 ). For the other 3 primary outcomes (mortality, dialysis, and kidney recovery) (eFigures 7-9 in Supplement 1 ) and most secondary outcomes (eFigures 10-15 in Supplement 1 ), there was no significant subgroup heterogeneity detected. Regarding post-AKI ACEI/ARB exposure, AKI e-alerts combined with an AKI care bundle were associated with lower RR (0.78 [95% CI, 0.70-0.88]; P = .002 for subgroup difference test) (eFigure 16 in Supplement 1 ). For studies reporting mortality outcomes over different time periods, there was no significant subgroup difference (eFigure 17 in Supplement 1 ).
A trial sequential analysis on mortality indicated that e-alerts were unlikely to be associated with a 10% risk reduction (eFigure 18 in Supplement 1 ). For AKI stage progression, TSA indicated a premature conclusion (eFigure 19 in Supplement 1 ). For dialysis, TSA show a true-positive finding with sufficient sample size (eFigure 20 in Supplement 1 ) and an uncertain result regarding kidney recovery (eFigure 21 in Supplement 1 ). Trial sequential analysis also supported the results from conventional analysis regarding nephrologist consultations, AKI documentation, and reduced post-AKI NSAID exposure (eFigures 22-24 in Supplement 1 ).
Including only RCTs in the TSA, e-alerts showed a true-positive finding for dialysis, NSAID exposure, and consultation (eTable 4 in Supplement 1 ). Other outcomes were premature, inconclusive, or ineffective. Sensitivity analysis using the Hartung-Knapp method still showed a significantly increased the RR for dialysis. The beta-binomial bayesian meta-analysis also showed a significantly lower RR for NSAID exposure after AKI (eTable 5 in Supplement 1 ).
The funnel plot for all primary and secondary outcomes are provided (eFigure 25 in Supplement 1 ). There was no significant asymmetry observed in the funnel plots. The Egger tests were performed for outcomes with more than 10 studies and found no publication bias for mortality (Egger P = .13), dialysis (Egger P = .63), or nephrologist consultation (Egger P = .26).
The overall certainty of evidence (CoE) varied from moderate to very low. We summarized the results of CoE assessment in eTable 6 in Supplement 1 . The detailed reasons for downgrading are provided in eTable 6 in Supplement 1 and eAppendix 3 in Supplement 1 . We also summarized the results and CoE assessment ( Table 2 ).
This systematic review and meta-analysis highlights 4 key findings. First, AKI e-alerts may be unlikely to be associated with a 10% reduction of risk for mortality in patients with AKI, a finding supported by TSA. Second, AKI e-alerts might be associated with lower RR of AKI progression, but more research is needed to support this conclusion. Third, AKI e-alerts were linked to increased dialysis events. Fourth, AKI e-alerts seem to be associated with different clinical practices (eg, more nephrologist consultations and AKI documentation and less post-AKI NSAID exposure).
Our analysis suggests AKI e-alerts may not be associated with lower RR of mortality but with lower RR of AKI progression, and the pooled effect was associated with heterogeneity. Most studies found AKI e-alerts have a neutral effect on mortality, with exceptions in 2 studies. 10 , 25 Tome et al 25 observed lower mortality with AKI e-alerts plus care recommendations in early-stage AKI, but not in stage 3. Conversely, the ELAIA-1 study 10 noted increased mortality in nonteaching hospitals. The increased primary composite outcome from AKI e-alerts group in nonteaching hospitals was driven by increased mortality, but the dialysis or AKI progression rates were similar across different hospitals. 10 In that study, 10 the authors considered the harm from e-alerts in nonteaching hospitals to be a true effect and postulated several possible mechanisms, including unnecessary intravenous fluid prescription and/or fluid overload, alarm fatigue, and the pressure on clinicians to take potentially harmful actions (which could be prevented by systems in teaching hospitals).
The apparent neutrality, yet underlying heterogeneity, of AKI e-alerts’ association with mortality may result from several factors. First, evaluated AKI e-alert systems use creatinine-based diagnoses, with unreported variations in testing frequency and timing affecting AKI detection. Creatinine level, a delayed and less predictive AKI marker than urine output in patients with critical illness, could affect outcomes. Bianchi et al 28 noted oliguria over 12 hours as a crucial outcome marker, independent of creatinine levels. Second, the AKI e-alert system’s success may depend more on alarm detection and management. The analysis by Shi et al 29 showed physician response to and detection rate of e-alerts might result in different 14-day mortality rates. Third, reducing post-AKI NSAID exposure might explain the lower RR for AKI progression. As previously mentioned, the current limitations of AKI e-alerts may arise from the delayed nature of serum creatinine levels in AKI diagnosis and the heterogeneous effects of AKI e-alerts (which might be raised from different AKI care bundles, different hospitals, or physicians’ responses). Therefore, we suggest that an e-alert system should be integrated with earlier risk stratification methods, such as the renal angina index, 30 , 31 artificial intelligence − based continuous AKI prediction, 32 and care bundle implementation within a clinical decision support system to enhance early diagnosis and management, potentially improving outcomes.
Our analysis suggests that AKI e-alerts may increase post-AKI dialysis events, with more nephrologist consultations and reduced NSAID exposure. The higher dialysis rates in the e-alert group might result from the lack of standardized kidney replacement therapy initiation protocols and increased nephrologist involvement. Despite a lack of significant subgroup differences between RCTs and non-RCTs, 2 non-RCTs 20 , 21 noted higher dialysis rates with e-alerts. Atia et al 20 attributed this to more nephrologist consultations and earlier dialysis initiation. Our analysis found that the e-alert had lower RRs for post-AKI ACEI/ARB prescription. Only Wilson et al 11 reported the effect of e-alerts on post-AKI proton-pump inhibitor prescriptions. In that study, the e-alert group had a higher RR for proton-pump inhibitor therapy discontinuation (RR, 1.26 [95% CI, 1.10-1.45]).
Additionally, AKI e-alerts might reduce AKI stage progression, though with notable subgroup heterogeneity. Studies with AKI e-alerts plus care recommendations showed reduced AKI progression risk (eFigure 6 in Supplement 1 ). However, this finding, according to trial sequential analysis (eFigure 15 in Supplement 1 ), is premature, given the small number of studies reporting this outcome and the reliance on non-RCTs.
Our study has several strengths. It is an updated systematic review and meta-analysis on AKI e-alerts using contemporary evidence-based methods, includes trial sequential analysis, and evaluates the CoE across all outcomes using the GRADE framework. However, limitations exist. First, the scarcity of RCTs led us to combine findings from RCTs, prospective trials, and retrospective studies. Second, few studies examined the impact on hospital LOS, cost, AKI stage progression, post-AKI kidney recovery and ACEI/ARB prescription, limiting our ability to make conclusive statements on these aspects. Third, the evidence lacks exploration of urine output–based AKI e-alerts and early biomarkers for risk stratification, which could enhance early detection and intervention. Fourth, none of the enrolled studies reported major adverse kidney events at 28 and 90 days, which are crucial outcomes after AKI. This leaves the impact of AKI e-alerts and increased dialysis events on long-term outcomes uncertain. Further investigation in these areas is needed.
The current meta-analysis suggests that the implementation of AKI e-alerts might not be associated with a lower risk for mortality but may be associated with different practice patterns (including higher RRs for nephrologist consultations, dialysis, and AKI documentation and lower RRs for post-AKI NSAID exposure). Implementation was associated with a lower RR for AKI progression, but this result was heterogeneous and possibly premature. We recommend that each hospital establish its own AKI e-alert system and individualized AKI management protocol tailored to its specific needs. Additionally, future studies should focus on combining e-alert systems with AKI prediction or early biomarker risk stratification, along with clinical decision support systems or care bundles, which might be beneficial.
Accepted for Publication: July 2, 2024.
Published: August 27, 2024. doi:10.1001/jamanetworkopen.2024.30401
Open Access: This is an open access article distributed under the terms of the CC-BY License . © 2024 Chen JJ et al. JAMA Network Open .
Corresponding Author: Chih-Hsiang Chang, MD, PhD, Department of Nephrology, Linkou Chang Gung Memorial Hospital, No.5 Fusing St, Taoyuan 333, Taiwan ( [email protected] ).
Author Contributions: Drs Chen and Chang had full access to all of the data in the study and take responsibility for the integrity of the data and the accuracy of the data analysis.
Concept and design: Chen, T.-H. Lee, Tsai, C.-C Lee, Chang.
Acquisition, analysis, or interpretation of data: Chen, T.-H. Lee, Chan, Fan, Wu, Tu, Chang.
Drafting of the manuscript: Chen, T.-H. Lee, Chang.
Critical review of the manuscript for important intellectual content: Chan, Tsai, Fan, C.-C. Lee, Wu, Tu, Chang.
Statistical analysis: Chen, Chan, Tsai, Tu, Chang.
Obtained funding: Chang.
Administrative, technical, or material support: T.-H. Lee, Fan, Tu.
Supervision: C.-C. Lee, Wu, Chang.
Conflict of Interest Disclosures: None reported.
Funding/Support: This study was supported by grants MOHW110-TDU-B-212-124005, MOHW111-TDU-B-212-134005, and MOHW112-TDU-B-212-144005 from the Taiwanese Ministry of Health and Welfare and grant CMRPG5M0181 from Linkou Chang Gung Memorial Hospital (Dr Chang).
Role of the Funder/Sponsor: The funders had no role in the design and conduct of the study; collection, management, analysis, and interpretation of the data; preparation, review, or approval of the manuscript; and decision to submit the manuscript for publication.
Data Sharing Statement: See Supplement 2 .
- Register for email alerts with links to free full-text articles
- Access PDFs of free articles
- Manage your interests
- Save searches and receive search alerts
- Correspondence
- Open access
- Published: 26 August 2024
Comparison of proton therapy and photon therapy for early-stage non-small cell lung cancer: a meta-analysis
- Junyi He 1 , 3 na1 ,
- Yingxin Liu 2 , 3 na1 ,
- Xiaojing Zhang 2 , 3 ,
- Butuo Li 2 , 3 ,
- Linlin Yang 2 , 3 ,
- Haohua Wang 1 , 3 ,
- Shijiang Wang 3 , 1 ,
- Jinming Yu 1 , 3 &
- Linlin Wang 1 , 3
Biomarker Research volume 12 , Article number: 90 ( 2024 ) Cite this article
107 Accesses
1 Altmetric
Metrics details
The use of proton therapy (PT) in early-stage non-small cell lung cancer (ES-NSCLC) remains controversial, with insufficient evidence to determine its superiority over photon therapy (XRT). We conducted a systematic review of PT trials in ES-NSCLC, analyzing dosimetry, efficacy, and safety across to inform clinical decision-making. Our study showed that PT reduced lung and heart dosimetric parameters compared to XRT, with significant differences in lung V5, lung V10 and mean heart dose (MHD). In terms of efficacy, there were no significant differences in 1-year OS, 3-year OS and 3-year PFS between PT and XRT. For toxicity, no significant difference was observed in treatment-related adverse events (TRAEs) and radiation pneumonitis (RP). Single-arm analysis of PT found that V5, V10, V20 of lung and heart V5 were 13.4%, 11.3%, 7.9% and 0.7%, respectively. The mean lung dose and MHD were 4.15 Gy and 0.17 Gy, respectively. The single-arm pooled 1-, 2-, 3- and 5-year OS rates for PT were 95.3%, 82.5%, 81.3% and 69.3%, respectively. PFS rate and local control rate at 3 years were 68.1% and 91.2%, respectively. The rates of TRAEs of grade ≥ 3 and grade ≥ 2 were 2.8% and 19.8%, respectively. The grade ≥ 2 RP occurred at a rate of 8.7%. In conclusion, PT had acceptable efficacy and safety, and was better at protecting organs at risk than XRT in ES-NSCLC. However, the survival and safety benefit of PT was not significant compared to XRT.
To the editor: Radiotherapy is now the standard treatment for patients with unresectable early-stage non-small cell lung cancer (ES-NSCLC) [ 1 ]. Stereotactic body radiotherapy offers excellent survival outcomes, but is limited by the dose to organs at risk (OARs) [ 2 ]. Proton beam, with its Bragg peak, stops precisely at edge of targets, resulting in lower dosimetry of OARs and better dose deposition in tumors. However, some studies have suggested that proton therapy (PT) does not provide a survival benefit over photon therapy (XRT) [ 3 , 4 ]. Currently, the use of PT in the treatment of ES-NSCLC remains controversial due to insufficient evidence, including the lack of large randomized controlled trials, to definitively establish its superiority over XRT. Therefore, we conducted a systematic review of PT trials in ES-NSCLC, analyzing dosimetry, efficacy, and safety across to inform clinical decisions (Figure S1 )0.19 studies were finally included in the meta-analysis, of which 5 were comparative studies [ 3 , 4 , 5 , 6 ] (Table S1 ), and 14 were single-arm studies (Table S2 ).
In terms of dosimetry, PT showed reduced lung and heart dosimetric parameters versus XRT (Fig. 1 ). For lung, significant reductions were observed in lung V5 with 6.2% (95% confidence interval (CI): 5.0, 7.5) and lung V10 with 2.6% (95% CI: 1.5, 3.6) when compared PT to XRT. No significant difference was found in other parameters of lung, including V20, V40 and mean lung dose (MLD). For the heart, PT was significantly associated with a lower mean heart dose (MHD), with a reduction of 1.4 Gy (95% CI: 0.6, 2.2).
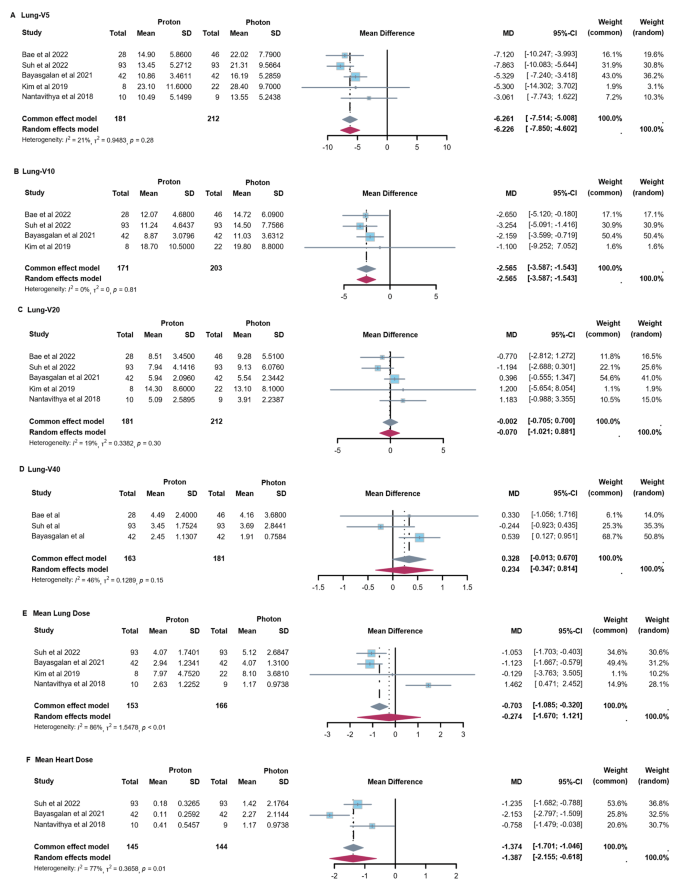
Forest Plots of Dose-Volume Parameters for OARs with Proton vs. Photon Therapy. ( A ) Lung-V5 of PT versus XRT. ( B ) Lung-V10 of PT versus XRT. ( C ) Lung-V20 of PT versus XRT. ( D ) Lung-V40 of PT versus XRT. ( E ) Mean lung dose of PT versus XRT ( F ) Mean heart dose of PT versus XRT
When comparing survival data between PT and XRT, no significant difference was found (Fig. 2 A-C). For progression-free survival (PFS), there was a 14.1% (95% CI: -25.0%, 53.2%) increase in 3-year PFS with PT, from 45.5 to 59.6%. For overall survival (OS), there was 1.6% (95% CI: -4.6%, 7.9%) increase in 1-year OS with PT, from 89.7 to 91.3%. While for 3-year OS rate, there was 3.6% (95% CI: -28.6%, 21.5%) decrease with PT, from 59.7 to 56.1%. Regarding treatment-related adverse events (TRAEs), no significant differences were found between PT and XRT in the rates of grade ≥ 2 (OR = 0.58, 95% CI: 0.32, 1.07) and grade ≥ 3 (OR = 0.69, 95% CI: 0.26, 1.79) TRAEs. Similarly, no significant differences were found in the incidence of grade ≥ 2 radiation pneumonitis (RP) (OR = 0.61, 95% CI: 0.29, 1.29) and grade ≥ 3 RP (OR = 0.88, 95% CI: 0.12, 6.51) (Fig. 2 D-G).
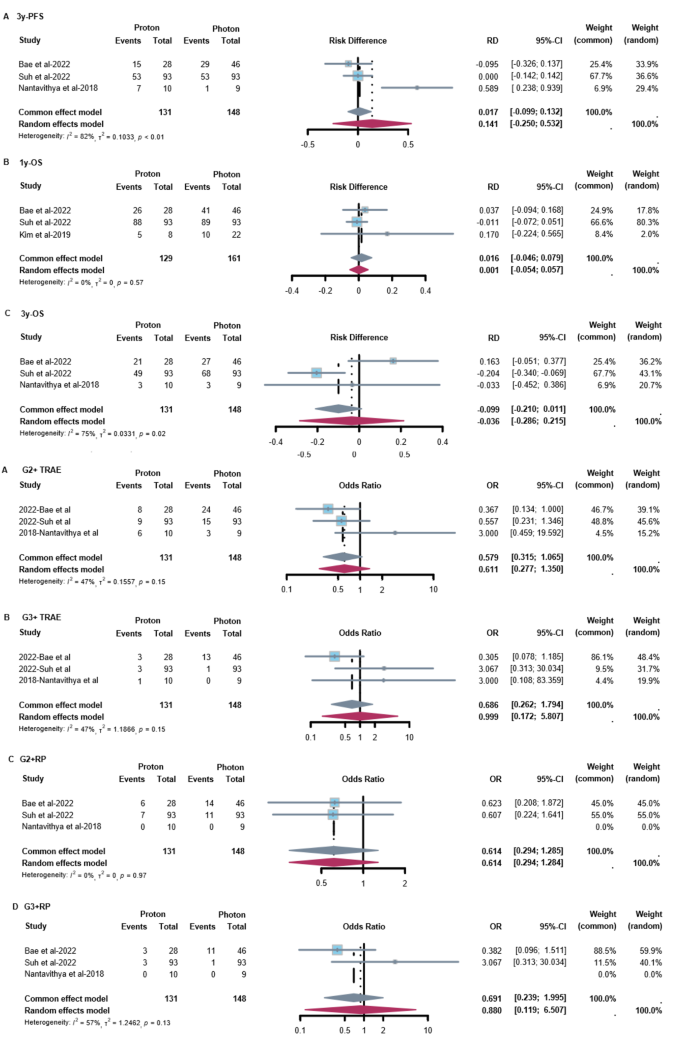
Forest Plots of efficacy and safety with Proton vs. Photon Therapy. ( A ) 3-year PFS of PT versus XRT. ( B ) 1-year OS of PT versus XRT. ( C ) 3-year OS of PT versus XRT. ( D ) G2 + TRAE of PT versus XRT. ( E ) G3 + TRAE of PT versus XRT. ( F ) G2 + RP of PT versus XRT. ( G ) G3 + RP of PT versus XRT
In single-arm analysis of PT, we also assessed dosimetry, efficacy and safety (Figure S2 - S4 ). In terms of dosimetry, pooled V5, V10 and V20 of lung were 13.38% (95%CI: 11.79%, 14.96%), 11.29% (95%CI: 9.00%, 13.59%) and 7.94% (95%CI: 6.32%, 9.56%), respectively. Besides, the pooled MLD was 4.15 Gy (95%CI: 3.04, 5.26). For heart, V5 was 0.69% (95%CI: 0.01%, 1.37%) and the MHD was 0.17 Gy (95%CI: 0.09, 0.24). For esophagus and spinal cord, the maximum dose was 14.26 Gy (95%CI: 1.57, 26.94) and 1.45 Gy (95%CI: 0.58, 2.31), respectively. For survival outcomes of PT, the pooled analysis showed 1-, 2-, 3- and 5-year OS rates were 95.3% (95%CI: 91.8%, 98.8%), 82.5% (95%CI: 77.0%, 87.9%), 81.3% (95%CI: 76.4%, 86.2%) and 69.3% (95%CI: 50.4%, 88.3%), respectively. The 3-year PFS and local control rates were 68.1% (95%CI: 62.1%, 74.0%) and 91.2% (95%CI: 86.9%, 95.5%), respectively. In terms of toxicity, the rates of grade ≥ 3 and grade ≥ 2 TRAEs were 2.8% (95% CI: 1.5%, 4.5%) and 19.8% (95% CI: 15.6%, 25.1%), respectively. The incidences of grade ≥ 2 RP, grade ≥ 2 dermatitis and grade ≥ 2 chest wall pain were 8.7% (95% CI: 5.6%, 11.7%), 7.1% (95% CI: 3.3%, 15.4%) and 3.4% (95% CI: 0%, 6.9%), respectively.
Our meta-analyses showed that PT reduced cardiopulmonary dose but did not differ significantly from XRT in terms of survival outcomes and adverse events. Liao et al. noted a learning curve for PT, suggesting that technological advancements and increased experience improve trial results [ 7 ]. Other studies have shown that PT has a more pronounced immunomodulatory effect and causes less lymphopenia than XRT [ 8 , 9 , 10 ]. It is reported that XRT combined with immunotherapy can achieve better outcomes for patients [ 11 , 12 ], suggesting the potential to explore the further benefits of combining PT with immunotherapy.
Data availability
No datasets were generated or analysed during the current study.
Abbreviations
Confidence interval.
Early-stage non-small cell lung cancer
Mean heart dose
Mean lung dose
Organs at risk
Overall survival
Progression-free survival
- Proton therapy
Radiation pneumonitis
Treatment-related adverse events
Photon therapy
Baumann P, Nyman J, Hoyer M, Wennberg B, Gagliardi G, Lax I, et al. Outcome in a prospective phase II trial of medically inoperable stage I non–small-cell lung Cancer patients treated with stereotactic body Radiotherapy. J Clin Oncol. 2009;27:3290–6.
Article PubMed Google Scholar
Palma D, Daly M, Urbanic J, Giuliani M. Stereotactic Radiation for Ultra-central Lung tumors: good idea, or Ultra-risky? Int J Radiation Oncology*Biology*Physics. 2019;103:788–91.
Article Google Scholar
Bae BK, Yang K, Noh JM, Pyo H, Ahn YC. Clinical outcomes following Proton and Photon Stereotactic Body Radiation Therapy for Early-Stage Lung Cancer. Cancers. 2022;14:4152.
Article PubMed PubMed Central Google Scholar
Suh Y-G, Noh JM, Lee DY, Kim TH, Bayasgalan U, Pyo H, et al. Proton Beam Therapy versus Photon Radiotherapy for Stage I Non-small Cell Lung Cancer. Cancers. 2022;14:3627.
Article CAS PubMed PubMed Central Google Scholar
Bayasgalan U, Moon SH, Kim TH, Kim TY, Lee SH, Suh Y-G. Dosimetric comparisons between Proton Beam Therapy and Modern Photon Radiation techniques for stage I non-small cell Lung Cancer according to Tumor Location. Cancers. 2021;13:6356.
Kim H, Pyo H, Noh JM, Lee W, Park B, Park HY, et al. Preliminary result of definitive radiotherapy in patients with non-small cell lung cancer who have underlying idiopathic pulmonary fibrosis: comparison between X-ray and proton therapy. Radiat Oncol. 2019;14:19.
Liao Z, Lee JJ, Komaki R, Gomez DR, O’Reilly MS, Fossella FV, et al. Bayesian adaptive randomization trial of Passive Scattering Proton Therapy and Intensity-Modulated Photon Radiotherapy for locally Advanced non–small-cell Lung Cancer. J Clin Oncol. 2018;36:1813–22.
Lee HJ Jr, Zeng J, Rengan R. Proton Beam therapy and immunotherapy: an emerging partnership for immune activation in non-small cell lung cancer. Transl Lung Cancer Res. 2018;7:180–8.
Zhou Z, Guan B, Xia H, Zheng R, Xu B. Particle radiotherapy in the era of radioimmunotherapy. Cancer Lett. 2023;567:216268.
Article CAS PubMed Google Scholar
Hu M, Jiang L, Cui X, Zhang J, Yu J. Proton Beam therapy for cancer in the era of precision medicine. J Hematol Oncol. 2018;11:1–16.
Chang JY, Lin SH, Dong W, Liao Z, Gandhi SJ, Gay CM, et al. Stereotactic ablative radiotherapy with or without immunotherapy for early-stage or isolated lung parenchymal recurrent node-negative non-small-cell lung cancer: an open-label, randomised, phase 2 trial. Lancet. 2023;402:871–81.
Spigel DR, Faivre-Finn C, Gray JE, Vicente D, Planchard D, Paz-Ares L, et al. Five-year survival outcomes from the PACIFIC Trial: Durvalumab after Chemoradiotherapy in Stage III non–small-cell Lung Cancer. JCO. 2022;40:1301–11.
Article CAS Google Scholar
Download references
Acknowledgements
Not applicable.
This study was funded by National Natural Science Foundation of China (Grant number 82172865 and 8203000516).
Author information
Junyi He and Yingxin Liu these authors contributed to the work equally and should be regarded as co-first authors.
Authors and Affiliations
Cheeloo College of Medicine, Shandong University Cancer Center, Shandong University, Jinan, Shandong, China
Junyi He, Haohua Wang, Shijiang Wang, Jinming Yu & Linlin Wang
Shandong First Medical University, Shandong Academy of Medical Sciences, Jinan, Shandong, China
Yingxin Liu, Xiaojing Zhang, Butuo Li & Linlin Yang
Department of Radiation Oncology, Shandong Cancer Hospital and Institute, Shandong First Medical University, Shandong Academy of Medical Sciences, Jiyan Road 440, Jinan, 250117, Shandong, China
Junyi He, Yingxin Liu, Xiaojing Zhang, Butuo Li, Linlin Yang, Haohua Wang, Shijiang Wang, Jinming Yu & Linlin Wang
You can also search for this author in PubMed Google Scholar
Contributions
JH, JY, and LW conceptualized and developed the methodology. YL, XZ, and BL carried out the literature search and screening. JH, HW, and SW curated and collected the data. JH, YL, and LY handled the data analysis and interpretation. JH and YL wrote the original draft, while JH, YL and LW took care of the reviewing and editing process. All authors contributed to the article and approved the submitted version.
Corresponding author
Correspondence to Linlin Wang .
Ethics declarations
Ethics approval and consent to participate, consent for publication, competing interests.
The authors declare no competing interests.
Additional information
Publisher’s note.
Springer Nature remains neutral with regard to jurisdictional claims in published maps and institutional affiliations.
Electronic supplementary material
Below is the link to the electronic supplementary material.
Supplementary Material 1
Supplementary material 2, supplementary material 3, supplementary material 4, supplementary material 5, supplementary material 6, supplementary material 7, supplementary material 8, rights and permissions.
Open Access This article is licensed under a Creative Commons Attribution 4.0 International License, which permits use, sharing, adaptation, distribution and reproduction in any medium or format, as long as you give appropriate credit to the original author(s) and the source, provide a link to the Creative Commons licence, and indicate if changes were made. The images or other third party material in this article are included in the article’s Creative Commons licence, unless indicated otherwise in a credit line to the material. If material is not included in the article’s Creative Commons licence and your intended use is not permitted by statutory regulation or exceeds the permitted use, you will need to obtain permission directly from the copyright holder. To view a copy of this licence, visit http://creativecommons.org/licenses/by/4.0/ . The Creative Commons Public Domain Dedication waiver ( http://creativecommons.org/publicdomain/zero/1.0/ ) applies to the data made available in this article, unless otherwise stated in a credit line to the data.
Reprints and permissions
About this article
Cite this article.
He, J., Liu, Y., Zhang, X. et al. Comparison of proton therapy and photon therapy for early-stage non-small cell lung cancer: a meta-analysis. Biomark Res 12 , 90 (2024). https://doi.org/10.1186/s40364-024-00642-5
Download citation
Received : 05 July 2024
Accepted : 16 August 2024
Published : 26 August 2024
DOI : https://doi.org/10.1186/s40364-024-00642-5
Share this article
Anyone you share the following link with will be able to read this content:
Sorry, a shareable link is not currently available for this article.
Provided by the Springer Nature SharedIt content-sharing initiative
- Lung cancer
- Early stage
- Meta-analysis
Biomarker Research
ISSN: 2050-7771
- General enquiries: [email protected]
- Systematic Review
- Open access
- Published: 20 August 2024
Influence of aerobic exercise on depression in young people: a meta-analysis
- Weicheng Li 1 ,
- Yongfeng Liu 1 ,
- Jiaxin Deng 1 &
- Tong Wang 1
BMC Psychiatry volume 24 , Article number: 571 ( 2024 ) Cite this article
276 Accesses
3 Altmetric
Metrics details
To investigate the influence of aerobic exercise on depression among the young people.
Data sources
PubMed, Web of science, Embase, Cochrane, EBSCO were searched from inception to November 2023.
Study selection
RCT studies, assessing the use of aerobic exercise in young people aged 6–35 years and then determining the development of depression in young people (aged 6–35 years), were selected, and mean ± SD values adjusted for the presence of baseline depression were extracted.
Data extraction and synthesis
Study quality was assessed using the Review manager 5.4.1 and Cochrane 5.1 item on risk of bias and precision of observational studies. Two reviewers conducted all review stages independently. Selected data were pooled using random-effects meta-analysis.
Main outcomes and measures
The included studies evaluated the relationship between aerobic exercise and depression at various time points among young individuals, and provided corresponding mean ± SD values. Depression diagnosis in the selected studies was conducted using the Depression Correlation Scale.
After screening 2296 articles, 163 articles were selected for full-text review, and 8 of those were further reviewed. Ultimately, 12 studies, involving 658 individuals, were included in the meta-analysis. The results of the meta-analysis indicated that aerobic exercise could effectively improve depression in young people (d = -1.33, 95%CI: -1.78 ~ -0.87, P < 0.05). Subgroup analysis demonstrated that aerobic exercise was beneficial for both depression (d = -2.68, 95%CI: -3.87 ~ -1.48, P < 0.05) and non-depression (d = -0.85, 95%CI: -1.20 ~ -0.51, P < 0.05) conditions, as well as for low intensity (d = -0.93, 95%CI: -1.29 ~ 0.58, P < 0.05) and moderate intensity (d = -2.08,95%CI:-2.88 ~ -1.27, P < 0.05) exercises. Additionally, aerobic exercise was found to significantly alleviate depression in young people when performed for 40 min or less (d = -2.00,95%CI: -2.96 ~ -1.04, P < 0.05), whereas durations exceeding 40 min showed a lesser effect (d = -0.85,95%CI:-1.47 ~ -0.24, P < 0.05). Furthermore, the duration-based analysis revealed that aerobic exercise improved depression levels in young people regardless of duration, whether it was for less than 6 weeks (d = -1.27,95%CI: 2.12 ~ 0.14, P < 0.05), 6 to 11 weeks (d = 1.55, 95% CI: 2.16 ~ 0.94, P < 0.05), or more than 12 weeks (d = 1.00, 95% CI: -1.76 ~ -0.24, P < 0.05). Overall, these findings suggest that aerobic exercise is a promising intervention for alleviating depression in young individuals.
Conclusions
Both short (less than 40 min) and long (more than 40 min) periods of aerobic exercise were effective in improving depressive symptoms. However, the optimal duration of aerobic exercise may vary depending on the specific population and individual factors studied, such as age, health status, and exercise tolerance. Therefore, this study concluded that the most effective exercise regimen, 40 min of moderate-intensity aerobic exercise three times a week for 6 to 11 weeks, showed more significant improvement in depression indicators in individuals with depressive symptoms.
Peer Review reports
Introduction
Depression stands as the prevailing mental health disorder and a pronounced public concern in contemporary society. Distinguished from ordinary mood fluctuations, depressive symptoms manifest as recurrent episodes characterized by diminished concentration, pronounced feelings of guilt, profound despair regarding the future, and, in severe cases, tendencies toward self-harm or suicidal ideation. The severity of depressive symptoms is categorized into low, moderate, and high, with research indicating a lifetime prevalence of depression ranging from 17 to 30% [ 1 ]. The current trend indicates a noticeable increase in the prevalence of mental disorders among progressively younger individuals, accounting for approximately 13% of the global burden of disease within the age group of 10–19. Statistical data indicates that 1.1% of adolescents aged 10–14 and 2.8% of those aged 15–19 report experiencing symptoms of depression [ 2 ]. Hence, investigating the prevalence of depression in young individuals is of paramount importance. For the purposes of this study, we define “young people” as individuals who are no older than 35 years of age. This definition is consistent with various international organizations and studies, which recognize that the youth category can extend to 35 years in some cases. For example, the United Nations acknowledges that while youth is usually defined as individuals aged 15–24, it can be extended to 35 in different circumstances. For instance, the African Union’s African Youth Charter defines youth as individuals aged 15–35 years [ 3 ].
While medical advancements have led to a growing diversity in the treatment of depression, the predominant methods still revolve around pharmacological and psychotherapeutic interventions. Pharmacological treatments, in particular, find frequent application in clinical settings. Pigott, H.E [ 4 ] has suggested that, through pharmacological intervention, only 50% of patients exhibit marked improvement in depressive symptoms, often accompanied by corresponding side effects. Therefore, there is a clinical imperative to actively seek novel approaches for treating depression. Many studies have shown that physical exercise is a non-pharmacological treatment for depression. It can be treated alone or as an adjunct to medication and psychotherapy [ 5 ]. Aerobic exercise, as the primary form of exercise therapy, offers several advantages, including high adherence, straightforward exercise planning, minimal adverse effects, and broad suitability for diverse populations. Numerous studies have confirmed the positive impact of aerobic exercise interventions on various physiological manifestations associated with different conditions. These benefits encompass aspects such as including emotional regulation, cognitive health, and overall quality of life [ 6 , 7 , 8 ].
Therefore, choosing aerobic exercise to improve depressive symptoms in young individuals may yield significant benefits. However, the quantitative relationship between aerobic exercise and depression indicators in adolescents remains unclear. Current research primarily focuses on investigating various exercise interventions such as resistance training, Tai Chi, and yoga, on depression indicators in young people, without strong specificity towards particular exercises. Current research often compares the efficacy of various exercise modalities, such as aerobic, resistance, and combined exercises, in managing depression. However, these studies frequently overlook the potential benefits of focusing exclusively on a single exercise modality. Therefore, our study aims to provide detailed insights into the optimal aerobic exercise period, frequency, duration, and intensity. This can help young patients better determine the best aerobic training regimen when choosing aerobic exercise. we aim to synthesize the effect sizes of different aerobic exercises and their impact on adolescent depression indicators. Through subgroup analysis, this study seeks to identify subtle relationships between various forms of aerobic exercise and depression indicators, thus providing a theoretical basis for developing personalized aerobic exercise prescriptions for young individuals.
Study design
This systematic review and meta-analysis was registered with PROSPERO (registration number:CRD42023494476). Inclusion criteria, defined by Population, Intervention, Comparator, Outcome, and Study design (PICOS) [ 9 ] were:
Participants: We included young adults (aged 6–35) diagnosed with depressive symptoms.
Intervention: Aerobic exercise interventions were the focus, including activities such as running, cycling, or swimming.
Comparison: The comparison group consisted of individuals receiving no exercise or usual care for depression management.
Outcome: The primary outcome measured was improvement in depressive symptoms, assessed using standardized depression scales such as the BDI,CGI,BDI- ii, CMAS-R, das -21, BRUMS, SCL-90.
Study Design: RCTs.
Search strategy
This systematic review and meta-analysis adheres to the Preferred Reporting Items for Systematic Reviews and Meta-Analyses (PRISMA 2020) guidelines [ 10 ]. Through systematic exploration of the PubMed, Web of Science, Embase, Cochrane, and EBSCO databases, the search period spans from the inception of database records to November 2023. Employing a comprehensive search strategy, the approach integrates subject terms and free-text terms into three distinct groups. The first group centers on outcome measures, utilizing “Depression” as the subject term and encompassing associated free-text terms such as (Depressive Symptoms, Depressive Symptom, Symptom, Depressive, Emotional Depression, Depression, Emotional). The second group addresses the study population, employing “Adolescent” as the free-text term and incorporating related terms like (Teenagers, Youths, Adolescence). The third group pertains to intervention methods, utilizing “Exercise” as the subject term and incorporating pertinent free-text terms such as (Aerobic exercise, Exercise Training, Physical Activity). Terms within each group are logically connected using the logical operator OR, while the distinct groups are interconnected with the logical operator AND. Upon completion of the literature retrieval process, a dual-blind methodology will be implemented by two independent reviewers for the purposes of inclusion and exclusion. The retrieved literature will be consolidated within the EndnoteX9 reference management software, which will subsequently facilitate an initial duplicate check through its inherent system. Further scrutiny will ensue through a detailed examination of titles and author years. Subsequent to this, a preliminary screening of identified articles will be executed based on a meticulous review of titles and abstracts. The selected articles following this preliminary screening will be downloaded, and in instances where disparities arise between the two reviewers, a third reviewer will be engaged to deliberate on the inclusion status of the divergent articles. The search Strategy is shown in Table 1 below.
Inclusion criteria
Two reviewers (D,JX and W.T) independently assessed all titles and abstracts along with full texts of potentially relevant articles. Studies were included if they met the following criteria: (1) Include literature that involves study subjects aged 6–35 years [ 11 ]; (2) Incorporate literature specifically focused on aerobic exercise as the modality of physical activity; (3) Include literature with outcome measures based on relevant depression assessment scales; (4) Include literature exclusively derived from randomized controlled trials.
Exclusion criteria
Two reviewers (D,JX and W,T) independently assessed all titles and abstracts along with full texts of potentially relevant articles. Studies were excluded if they did not meet the following criteria: (1) Exclude non-English literature; (2) Exclude conference papers.(3) Exclude review articles and meta-analyses; (4) Exclude animal experiments; (5) Exclude literature involving participants aged 35 years and above; (6) Exclude literature for which the full text cannot be obtained; (7) Exclude literature where outcome measures are not expressed as mean ± standard deviation; (8) Exclude literature wherein the exercise intervention does not encompass aerobic exercise.
Data extraction
A structured form in Excel 2019 was used to extract the following data. Relevant information was extracted independently by two authors(D,JX and W,T) and imported into EndnoteX9 software:(1) Extract fundamental bibliographic details from the literature, encompassing authorship and publication year; (2) Retrieve demographic information concerning study subjects, comprising age, gender distribution, and sample sizes for both experimental and control groups; (3) Collect details pertaining to the exercise intervention, including the modality of intervention, duration, intensity, frequency, and the overall duration of the exercise regimen; (4) Capture information related to outcome measures, specifically focusing on pertinent depression assessment scales.
Quality assessment
The quality assessment of the included literature follows the standards outlined in the Cochrane 5.1 Handbook. This comprehensive approach includes evaluating randomization methods, allocation concealment, blinding procedures, completeness of outcome data, selective reporting of study results, and identification of potential biases. Additionally, the data extraction process and quality assessment adhere to the Cochrane Risk of Bias Assessment version 2 (August 2019 version). Literature that meets specific criteria and demonstrates low risk is categorized accordingly. High risk is assigned to studies that fail to meet these criteria, while instances where criteria are not explicitly addressed are categorized as unclear risk, with specific reasons provided.
The assessment of literature quality is independently conducted by two reviewers (D, JX and W, T), with any discrepancies resolved through consultation with a third party (L, YF).
Statistical analysis
The synthesis of effect sizes, assessment of publication bias, sensitivity analyses, and subgroup analyses are performed using Review Manager 5.4.1. Given that the outcome measures in this study are continuous variables, mean ± standard deviation is employed as the effect scale. Heterogeneity across studies is quantified through I 2 and Q tests; when I 2 < 50% and P > 0.1, denoting negligible heterogeneity, a fixed-effect model is applied, while in cases of significant heterogeneity, a random-effects model is employed. Publication bias is scrutinized using a funnel plot, and the robustness of results is ascertained through sensitivity analysis.
Standardization of depression scales
In this meta-analysis, we included studies that used various scales to measure depressive symptoms, including the Beck Depression Inventory (BDI), Clinical Global Impression (CGI), Beck Depression Inventory-II (BDI-II), Children’s Manifest Anxiety Scale-Revised (CMAS-R), Depression Anxiety Stress Scales-21 (DASS-21), Brunel Mood Scale (BRUMS), and Symptom Checklist-90 (SCL-90). To standardize the results across these different tools, we calculated the standardized mean difference (SMD) for each study.
The SMD is calculated by subtracting the mean score of the control group from the mean score of the intervention group and dividing the result by the pooled standard deviation of both groups. This approach allows us to combine and compare the effect sizes from different studies, despite the use of different scales. Additionally, we used a random-effects model to account for the variability among studies.
Search result
A total of 2296 articles were identified, with 281 from the Web of Science database, 138 from PubMed, 325 from Embase, 495 from Cochrane, and 1057 from EBSCO. After importing these articles into the Endnote software and eliminating duplicates, 1780 unique articles remained. During the title review, 270 articles related to reviews, animal experiments, and conferences were excluded, resulting in 1510 remaining articles. Subsequent abstract screening led to the exclusion of 1347 articles that did not meet criteria related to outcome indicators, intervention measures, and study subjects, leaving a total of 107 articles. Full-text reading further narrowed down the selection by excluding articles with inconsistent data indicators, non-English articles, and those without full text, resulting in a final selection of 8 articles for the Meta-analysis. The detailed screening process is depicted in Fig. 1 .
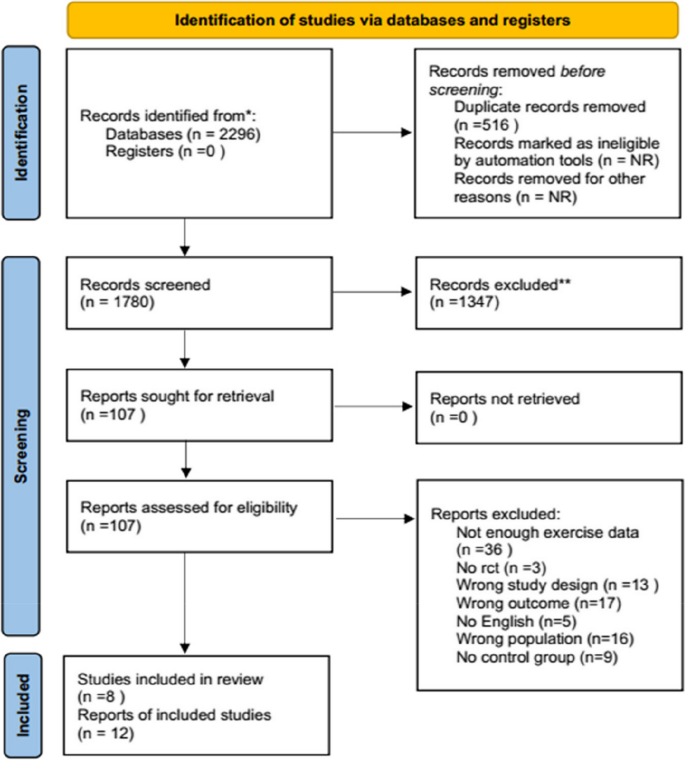
PRISMA flow diagram of the study selection process
Basic features of included studies
This study systematically incorporated a total of 8 articles, encompassing 12 randomized controlled trials (RCTs). Each study adhered to an RCT design, with sample sizes ranging from 26 to 55 participants, contributing to a cumulative total of 658 subjects. Among these, the experimental group comprised 329 individuals, mirroring the control group. The included articles comprehensively reported participant health status, exercise modalities, intensity, duration, frequency, and depression assessment scales. 1 study focused on individuals with existing depressive conditions, while 11 targeted non-depressed participants. Common exercise modalities included cycling, treadmill walking, with varying exercise intensities from low to high. Exercise durations spanned from 27.5 to 60 min, cycles ranged from 3 to 22 weeks, and frequency generally ranged from 2 to 4 sessions per week. Table 2 provides a succinct summary of the fundamental characteristics of the incorporated literature.
Literature quality assessment
All incorporated articles meticulously delineated the methods employed for group allocation, yet failed to specify whether the execution of the allocation strategy was conducted covertly. Given that participants were required to endorse informed consent documents before engaging in the experiment and that the implementation of the motor intervention necessitated relevant medical personnel to furnish protective measures or oversight, the implementation of blinding for both participants and researchers proved to be relatively challenging, thereby categorizing all 12 studies as high-risk endeavors. Despite this, each study exhibited minimal or negligible participant attrition during the intervention phase, signifying a low-risk profile concerning data stability. Notably, there was an absence of selective reporting or other biases across all scrutinized literature, as depicted in the quality assessment results outlined in the accompanying Fig. 2 .
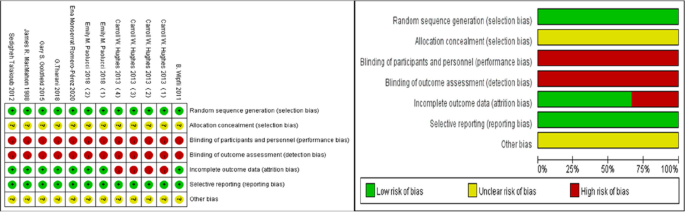
Cochrane risk of bias assessment chart
Bias assessment
The outcome indicators from the included studies demonstrate a symmetrical distribution of data points on either side of the funnel plot, indicating the absence of publication bias, as illustrated in Fig. 3 below.
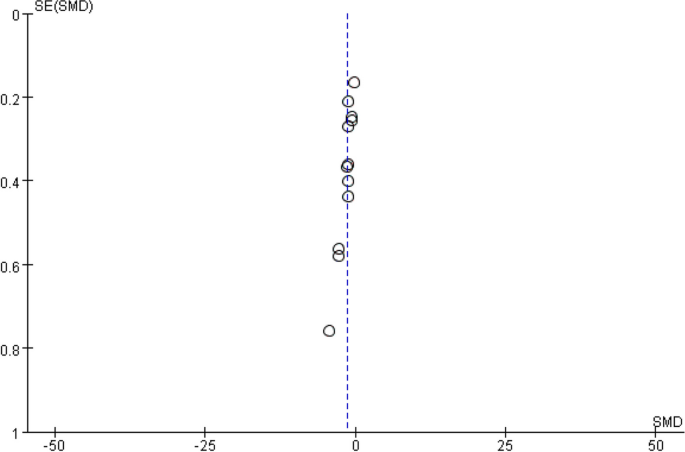
Publication bias funnel plot
Sensitivity analysis
Performing a sensitivity analysis on the included studies, systematically excluding each literature one by one, revealed no significant changes in the outcome indicators. This indicates that the results of this meta-analysis are stable and reliable.
Effect size test
The included articles underwent a heterogeneity test, and given that the I 2 value exceeded 50% in this study, a random-effects model was employed to merge the effect sizes. Furthermore, due to variations in the assessment tools for depression across studies, the Standardized Mean Difference (SMD) was adopted as the effect size metric. Among the 12 studies, a collective data set from 659 participants was analyzed for depression indicators. The results indicate that aerobic exercise has a significant positive impact on reducing depression levels in young individuals (d = -1.33, 95% CI: -1.78 to -0.87, P < 0.05), as illustrated in Fig. 4 .

Forest plot for heterogeneity test
Subgroup analysis
Study participants.
Due to substantial heterogeneity indicated by I 2 > 50%, a subgroup analysis was conducted to pinpoint the source of this heterogeneity. Initially, the study participants underwent a subgroup analysis, being divided into non-depressed and depressed groups. The non-depressed group comprised 554 individuals providing depression level data, while the depressed group involved 104 individuals. The findings revealed that aerobic exercise demonstrated a significant positive impact on improving depression levels in both the depressed group (d = -2.68, 95% CI: -3.87 to -1.48, P < 0.05) and the non-depressed group (d = -0.85, 95% CI: -1.20 to -0.51, P < 0.05). Detailed results are presented in Fig. 5 below.

Forest plot for subgroup analysis of study participants
Exercise intensity
Employing exercise intensity as a subgroup for analysis, it was segmented into low, moderate, and high intensity. A dataset of 159 individuals contributed to the low-intensity group, 246 individuals to the moderate-intensity group, and 253 individuals to the high-intensity group. The findings revealed that both low-intensity (d = -0.93, 95% CI: -1.29 to 0.58, P < 0.05) aerobic exercise and moderate-intensity (d = -2.08, 95% CI: -2.88 to -1.27, P < 0.05) aerobic exercise exhibited efficacy in ameliorating depression levels in the depressed group (d = -2.68, 95% CI: -3.87 to -1.48, P < 0.05). However, high-intensity aerobic exercise did not yield a statistically significant improvement in depression levels among young individuals ( P > 0.05). Detailed results are depicted in Fig. 6 below.
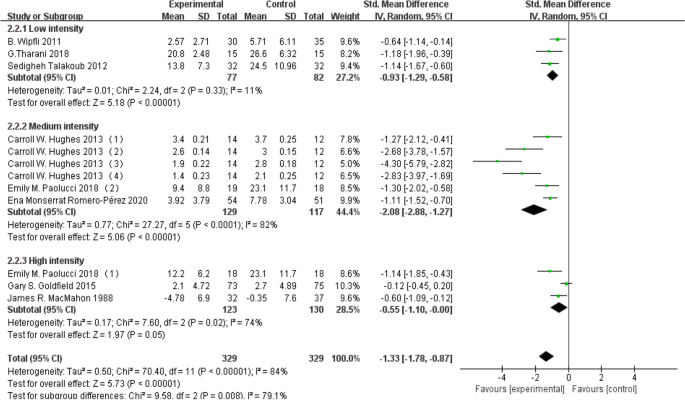
Subgroup analysis based on exercise intensity
Exercise duration
Segmenting exercise duration into two categories—40 min or less and over 40 min—subgroup analysis was conducted. A dataset of 209 individuals contributed to the 40 min or less group, while 347 individuals provided data for the over 40 min group. The results revealed that both aerobic exercises lasting 40 min or less (d = -2.00, 95% CI: -2.96 to -1.04, P < 0.05) and those exceeding 40 min (d = -0.85, 95% CI: -1.47 to -0.24, P < 0.05) were effective in ameliorating depression levels in young individuals. Detailed results are depicted in Fig. 7 below.
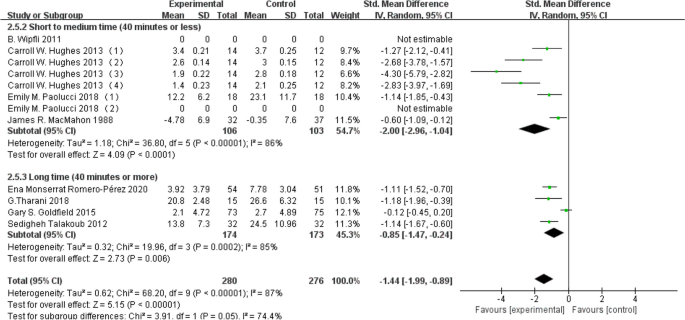
Subgroup analysis based on exercise duration
Exercise period
Subdividing exercise period into three categories—less than 6 weeks, 6–11 weeks, and 12 weeks or more conducted a subgroup analysis. A dataset of 105 individuals contributed to the less than 6 weeks group, 405 individuals to the 6–11 weeks group, and 348 individuals to the 12 weeks or more group. The results indicated that aerobic exercises lasting less than 6 weeks (d = -1.27, 95% CI: -2.12 to -0.14, P < 0.05), 6–11 weeks (d = -1.55, 95% CI: -2.16 to -0.94, P < 0.05), and 12 weeks or more (d = -1.00, 95% CI: -1.76 to -0.24, P < 0.05) were all effective in improving depression levels in young individuals. Detailed results are depicted in Fig. 8 below.

Forest plot for subgroup analysis based on exercise period
Exercise frequency
Due to the consistent exercise frequency of approximately three times per week, no subgroup analysis was conducted.
Impact analysis of aerobic exercise on depression indicators among young people
This study concludes that aerobic exercise can improve depression in young adults. The research highlights the crucial role of the hippocampus in depression studies, as it is a key brain structure related to emotions. Individuals with depression often exhibit a smaller hippocampal volume [ 20 ]. Hence, emphasizing the significance of investigating the relationship between hippocampal volume and depression. X, Y. M [ 21 ] conducted an aerobic exercise intervention study targeting individuals with severe depression. The research findings indicate that continuous aerobic exercise over six weeks led to improvements in participants’ depressive conditions. Furthermore, engaging in moderate-intensity aerobic exercise for 12 months (3 times per week) resulted in an approximate 2% increase in hippocampal volume among patients. The proposed mechanism involves aerobic exercise promoting blood circulation, facilitating the delivery of sufficient oxygen and nutrients to brain regions. This, in turn, contributes to the augmentation of neurogenesis and synaptic connectivity. Through the formation of new neural cells, neural connections, and the reinforcement of synapses, aerobic exercise maintains or increases hippocampal volume, thereby ameliorating the depressive conditions in individuals with severe depression.
Other studies have suggested a strong correlation between depression and brain-derived neurotrophic factor (BDNF), with a marked reduction in BDNF levels observed in the brains of individuals with depression [ 22 ]. Luo, Lu [ 23 ]contends that post-aerobic exercise, there is an elevation in BDNF levels in individuals with severe depression, thereby ameliorating depressive conditions among participants. Exercise induces muscle contractions, energy metabolism, and an elevation in body temperature, activating the production and release of BDNF. The released BDNF stimulates neurogenesis, enhances synaptic formation and stability, ultimately resulting in an augmentation of hippocampal volume and an improvement in depressive conditions among participants. Moreover, the enduring emotional stress and pressure associated with depression have deleterious effects on brain structure. Bender, T [ 24 ] suggests that aerobic exercise can elevate β-endorphin levels, thereby improving depressive conditions among participants. β-endorphin is a hormone and neurotransmitter associated with regulating depression [ 25 ]. Aerobic exercise triggers a physiological stress response by activating the hypothalamic–pituitary–adrenal axis (HPA axis). Subsequently, this activation prompts the release of adrenaline and cortisol. The release of cortisol, in turn, stimulates hypothalamic neurons to produce β-endorphin, ultimately contributing to the improvement of depressive conditions among participants.
Impact analysis of aerobic exercise on depression indicators in both depressed and non-depressed populations
This study found that aerobic exercise improves depressive symptoms in both depressed and non-depressed individuals, with greater benefits observed in those diagnosed with major depressive disorder (MDD). Hughes, C. W [ 13 ] 和Goldfield, Gary S [ 26 ] conducted separate aerobic exercise interventions with young individuals to assess the impact on depression indicators. However, their conclusions appear contradictory. Hughes observed improvements in depression indicators among young individuals engaging in aerobic exercise, while Goldfield found no such improvement in the same population. Upon comparison, it was noted that Hughes focused on participants diagnosed with major depressive disorder, whereas Goldfield, targeted individuals with obesity. It is suggested that individuals with major depressive disorder may derive more significant benefits from aerobic exercise in alleviating depressive conditions.
Clinical studies indicate that individuals with severe depression typically exhibit dysfunction in the HPA axis. This dysfunction is characterized by abnormal secretion and release of glucocorticoids (GC), corticotropin-releasing hormone (CRH), and adrenocorticotropin hormone(ACTH). These irregularities lead to a weakening of hippocampal neurons, disrupting the hippocampus’s normal regulatory role over the HPA axis. Consequently, there is an excessive activation of the HPA axis, giving rise to depressive emotions [ 27 ]. Pietrelli, Adriana [ 28 ] suggests that aerobic exercise can regulate the functioning of the HPA axis, improving depressive symptoms in individuals with severe depression. Aerobic exercise induces a physiological stress response, activating the HPA axis and leading to the release of CRH. This prompts the pituitary gland to release ACTH, triggering the synthesis and release of cortisol. Elevated cortisol levels then initiate a negative feedback loop on the hypothalamus and pituitary, inhibiting further release of CRH and ACTH, thus maintaining hormonal balance. This process contributes to the alleviation of depressive symptoms in individuals with severe depression.
Impact analysis of aerobic exercises on depression indicators in varying intensities among young people
This study found that both low and moderate-intensity aerobic exercise effectively alleviate depression symptoms in young individuals. However, high-intensity aerobic exercise did not show significant improvement. Low-intensity aerobic exercise, exceeding the minimum threshold, induces a stress response, while moderate-intensity exercise produces a more pronounced effect. Conversely, high-intensity aerobic exercise, surpassing the body’s critical threshold, leads to adverse reactions such as fatigue. Helgadóttir, Björg [ 29 ] contends that mild to moderate depression patients can reduce depressive symptoms through a 12-week intervention of low-intensity aerobic exercise. The relaxed and enjoyable nature of low-intensity aerobic exercise can alleviate both physical and mental stress, reducing psychological tension and positively impacting the alleviation of depressive symptoms. Balchin, R [ 30 ] contends that intervention involving moderate-intensity aerobic exercise holds the potential to ameliorate depressive symptoms in the targeted demographic. By actively participating in moderate-intensity aerobic activities, individuals may experience notable improvements in cardiovascular health, heightened metabolic efficiency, an increased sense of pleasure, thereby fostering a positive impact on the overall depressive condition. Contrastingly, Legrand [ 31 ] contends that high-intensity aerobic exercise holds the potential to improve depressive symptoms among individuals in fitness or sports environments. Engaging in vigorous aerobic activities leads to an elevation in maximal oxygen consumption, enhanced cardiovascular function, and the cultivation of increased self-confidence, thereby contributing to the amelioration of depressive conditions.
Physiologically, Helgadóttir, B [ 29 ] contends that low-intensity aerobic exercise improves depressive symptoms in the studied population by promoting the release of the BDNF. This, as mentioned earlier, enhances neuronal survival and connectivity, subsequently leading to an increased hippocampal volume and an improvement in depressive conditions. Song, Dan [ 32 ] contends that moderate-intensity aerobic exercise can alleviate depressive symptoms in elderly individuals with mild cognitive impairment. This is achieved by inducing the release of dopamine hormones during exercise, enhancing feelings of pleasure and subsequently improving depressive conditions. Aerobic exercise stimulates neurons, leading to dopamine production, which is released into the synaptic cleft. Binding to receptors, it initiates intracellular signal transduction, ultimately influencing emotion-related brain regions and contributing to the amelioration of depressive symptoms [ 33 ]. High-intensity aerobic exercise places the body under excessive physiological stress, intensifying depressive pressure. It is often challenging to sustain for an extended period and proves more difficult to incorporate into daily life.
Impact analysis of aerobic exercise on depression indicators in different exercise durations among young people
The research findings indicate that both aerobic exercises lasting under 40 min and those exceeding 40 min have a positive impact on alleviating depression in young adults. Notably, aerobic exercises under 40 min demonstrate a more significant improvement. Gordon, B. A [ 34 ] suggests in their study involving adolescents with Chronic Fatigue Syndrome (CFS) that aerobic exercise lasting 20–40 min can improve depressive symptoms. Bouaziz, Walid [ 35 ] suggests in their study focusing on sedentary elderly individuals that 30 min of aerobic exercise can ameliorate depressive emotions in this population. Similarly, Hughes, C. W [ 13 ] suggests that engaging in aerobic exercise for 30–40 min significantly improved depressive symptoms in adolescents with severe depression. Fatigue and depression often occur together, with physical and mental fatigue intensifying depressive symptoms. Extended aerobic exercise consumes significant energy and nutrients, leading to psychological fatigue. Additionally, fatigue affects the regulation of mood-related factors like dopamine, brain norepinephrine, and cortisol hormones [ 36 ].
The three studies involving elderly and diseased populations suggest that these groups, with lower tolerance for exercise intensity, are prone to fatigue, leading to the onset of depressive symptoms. In a state of fatigue, dopamine levels decline, reducing feelings of pleasure and increasing norepinephrine secretion, thereby elevating heart rate and exacerbating depression. Similarly, fatigue stimulates the adrenal cortex to produce more cortisol hormones, and elevated cortisol levels worsen depressive symptoms. Therefore, shorter durations of aerobic exercise are more easily accepted, reducing the risk of exercise-induced fatigue and discomfort. This approach facilitates adherence to the exercise regimen, contributing to the establishment of a consistent exercise habit and ultimately improving depressive conditions. Contrarily, Kwok [ 37 ] suggests that intervention through 90 min of aerobic exercise can improve depressive symptoms in adult Parkinson’s patients. Prolonged aerobic exercise continuously enhances cardiovascular capacity, boosts the body’s fatigue resistance, and further stimulates neurotransmitter release and hormonal level changes, thereby more effectively ameliorating depressive conditions.
Impact analysis of aerobic exercise on depression indicators in different exercise periods among young people
The study concludes that aerobic exercise interventions lasting less than 6 weeks, 6–11 weeks, and over 12 weeks all contribute to improvements in depression among young adults. Notably, the most significant improvement is observed in the 6–11 week exercise duration. Gordon [ 34 ] suggests that adolescents can experience improved depressive symptoms after a 4-week aerobic exercise intervention, Short-term exercise rapidly induces mood enhancement, facilitated by the release of endogenous substances such as endorphins [ 38 ]. This results in a quick sense of pleasure and relaxation, accompanied by a rapid enhancement of cardiovascular capacity. It promotes increased delivery of oxygen and nutrients to muscle tissues, supporting neuronal generation and release, ultimately improving depressive symptoms. Tharani, G [ 16 ] suggests that an 8-week aerobic exercise intervention improves depressive symptoms in young women with dysmenorrhea. After 6–11 weeks of aerobic exercise, participants exhibit increased cardiovascular adaptability, enhanced physical adaptation, and heightened fat oxidation capacity, positively influencing stable energy levels and mood improvement [ 39 ]. During the 6–11 weeks aerobic exercise period, observable changes in muscle and joint adaptability can enhance the efficiency of exercise, reduce the risk of injury, and improve exercise sustainability and psychological well-being. Greenwood, Sharlene A [ 40 ] suggests that patients with chronic kidney disease experience improved depressive emotions after a 12-week aerobic exercise intervention. As the exercise progresses, observable adaptations in participants’ physiological functions occur gradually. This not only provides short-term feelings of pleasure but also generates longer-term stable psychological effects. Additionally, it effectively enhances the basal metabolic rate and muscle adaptability, resulting in improved endurance and more effective exercise performance. This, in turn, boosts self-confidence and self-esteem, ultimately contributing to the amelioration of depression.
Contrastingly, an early study suggests that an optimal exercise duration should exceed 20 weeks [ 41 ]. Depression involves intricate neurochemical, hormonal, and physiological processes that necessitate a longer duration for transmission and triggering. Short to medium exercise periods may not induce substantial physiological adjustments, and the frequency and intensity of exercise during these periods might not sustain continuous adaptive changes in the body. Consequently, improvement in depressive conditions may be limited.
Limitations
In our study, there are some potential limitations that warrant consideration. Firstly, the stratification of our sample age range lacks granularity, potentially not adequately capturing the differences in various developmental stages among young individuals. Secondly, in terms of measuring aerobic exercise, our study comparatively focuses on a rather singular type of exercise and does not employ diverse methods to assess aerobic activity. This limitation may impact our comprehensive understanding of the effects of depression indicators across different age groups and types of aerobic exercises. Future research endeavors could aim for finer age stratification and employ a more comprehensive approach to measuring aerobic exercise, thereby enhancing our understanding of this field. Additionally, we observed a degree of heterogeneity in the included studies. Although meta-regression analyses can help identify potential sources of heterogeneity, the results of meta-regression analyses may be limited by these unreported factors because many original studies do not report in detail all the factors that could influence the size of the effect (such as the specific health status of the participants, the specific implementation details of the intervention, etc.).
Overall, both short (less than 40 min) and long (more than 40 min) periods of aerobic exercise were effective in improving depressive symptoms. However, the optimal duration of aerobic exercise may vary depending on the specific population and individual factors studied, such as age, health status, and exercise tolerance. Therefore, this study concluded that the most effective exercise regimen, 40 min of moderate-intensity aerobic exercise three times a week for 6 to 11 weeks, showed more significant improvement in depression indicators in individuals with depressive symptoms.
Availability of data and materials
The datasets used and/or analyzed during the current study available from the corresponding author on reasonable request.
Abbreviations
Brain-derived neurotrophic factor
Hypothalamic–pituitary–adrenal
Major depressive disorder
Corticotropin-releasing hormone
Adrenocorticotropic hormone
Beck Depression Inventory
Clinical Global Impression
Beck Depression Inventory-II
Children’s Manifest Anxiety Scale-Revised
Depression Anxiety Stress Scales-21
Brunel Mood Scale
Symptom Checklist-90
Global, regional, and national incidence, prevalence, and years lived with disability for 310 diseases and injuries, 1990–2015: a systematic analysis for the Global Burden of Disease Study 2015. Lancet. 2016;388(10053):1545–602.
Mental health of adolescents. World Health Organization. https://www.who.int/zh/news-room/fact-sheets/detail/adolescent-mental-health . Accessed 14 Feb 2024.
African Union Commission. African Youth Charter. Addis Ababa, Ethiopia: African Union; 2006.
Pigott HE. The STAR*D Trial: It Is Time to Reexamine the Clinical Beliefs That Guide the Treatment of Major Depression. Can J Psychiatry. 2015;60(1):9–13.
Article PubMed PubMed Central Google Scholar
Knöchel C, Oertel-Knöchel V, O'Dwyer L, et al. Cognitive and behavioural effects of physical exercise in psychiatric patients. Prog Neurobiol. 2012;96(1):46–68. https://doi.org/10.1016/j.pneurobio.2011.11.007 .
Brunet, Jennifer and Sitara Sharma. “A scoping review of studies exploring physical activity and cognition among persons with cancer.” J Cancer Surviv. 2023:1–19.
Montero-Odasso M, Zou G, Speechley M, et al. Effects of Exercise Alone or Combined With Cognitive Training and Vitamin D Supplementation to Improve Cognition in Adults With Mild Cognitive Impairment: A Randomized Clinical Trial. JAMA Netw Open. 2023;6(7):e2324465. https://doi.org/10.1001/jamanetworkopen.2023.24465 .
Russell C. "We Can Do This!": The Role of Physical Activity in What Comes Next for Dementia. Int J Environ Res Public Health. 2023;20(15):6503. https://doi.org/10.3390/ijerph20156503 .
Moher D, Liberati A, Tetzlaff J, Altman DG. Preferred reporting items for systematic reviews and meta-analyses: the PRISMA statement. PLoS Med. 2009;6(7):e1000097.
Hasani H, Mardi S, Shakerian S, Taherzadeh-Ghahfarokhi N, Mardi P. The novel coronavirus disease (COVID-19): A PRISMA systematic review and meta-analysis of clinical and paraclinical characteristics. Biomed Res Int. 2020;2020:3149020.
Volkov D. Russian Youth: Their Attitude to the Ruling Elite and Political Processes. IAI PAPERS. 2022;22:14. Accessed 14 Mar 2024.
Wipfli B, Landers D, Nagoshi C, Ringenbach S. An examination of serotonin and psychological variables in the relationship between exercise and mental health. Scand J Med Sci Sports. 2011;21(3):474–81.
Article CAS PubMed Google Scholar
Hughes CW, Barnes S, Barnes C, DeFina LE, Nakonezny P, Emslie GJ. Depressed Adolescents Treated with Exercise (DATE): A pilot randomized controlled trial to test feasibility and establish preliminary effect sizes. Ment Health Phys Act. 2013;6(2):119–31.
Article Google Scholar
Paolucci EM, Loukov D, Bowdish DME, Heisz JJ. Exercise reduces depression and inflammation but intensity matters. Biol Psychol. 2018;133:79–84.
Article PubMed Google Scholar
Romero-Pérez EM, González-Bernal JJ, Soto-Cámara R, et al. Influence of a Physical Exercise Program in the Anxiety and Depression in Children with Obesity. Int J Environ Res Public Health. 2020;17(13):465 5. https://doi.org/10.3390/ijerph17134655 .
Tharani G, Dharshini E, Rajalaxmi V, Kamatchi K, Vaishnavi G. To compare the effects of stretching exercise versus aerobic dance in primary dysmenorrhea among collegiates. Drug Invent Today. 2018;10(Special Issue 1):2844–8.
Google Scholar
Goldfield GS, Kenny GP, Alberga AS, Prud’homme D, Hadjiyannakis S, Gougeon R, Phillips P, Tulloch H, Malcolm J, Doucette S, et al. Effects of aerobic training, resistance training, or both on psychological health in adolescents with obesity: the HEARTY randomized controlled trial. J Consult Clin Psychol. 2015;83(6):1123–35.
MacMahon JR, Gross RT. Physical and psychological effects of aerobic exercise in delinquent adolescent males. Am J Dis Child (1960). 1988;142(12):1361–6.
CAS Google Scholar
Talakoub S, Gorbani S, Hasanpour M, Zolaktaf V, Amini M. Impact of exercise on affective responses in female adolescents with type I diabetes. Iran J Nurs Midwifery Res. 2012;17(6):434–9.
PubMed PubMed Central Google Scholar
Schmaal L, Veltman DJ, van Erp TGM, Sämann PG, Frodl T, Jahanshad N, Loehrer E, Tiemeier H, Hofman A, Niessen WJ, et al. Subcortical brain alterations in major depressive disorder: findings from the ENIGMA Major Depressive Disorder working group. Mol Psychiatry. 2016;21(6):806–12.
Xie Y, Wu Z, Sun L, Zhou L, Wang G, Xiao L, Wang H. The Effects and Mechanisms of Exercise on the Treatment of Depression. Front Psychiatry. 2021;12:705559. https://doi.org/10.3389/fpsyt.2021.705559 .
Khan H, Amin S, Patel S. Targeting BDNF modulation by plant glycosides as a novel therapeutic strategy in the treatment of depression. Life Sci. 2018;196:18–27.
Luo L, Li C, Deng Y, Wang Y, Meng P, Wang Q. High-intensity interval training on neuroplasticity, balance between brain-derived neurotrophic factor and precursor brain-derived neurotrophic factor in poststroke depression rats. J Stroke Cerebrovasc Dis. 2019;28(3):672–82.
Bender T, Nagy G, Barna I, Tefner I, Kádas E, Géher P. The effect of physical therapy on beta-endorphin levels. Eur J Appl Physiol. 2007;100(4):371–82.
Fichna J, Janecka A, Costentin J, Do Rego JC. The endomorphin system and its evolving neurophysiological role. Pharmacol Rev. 2007;59(1):88–123. https://doi.org/10.1124/pr.59.1.3 .
Goldfield GS, Kenny GP, Prud’homme D, Holcik M, Alberga AS, Fahnestock M, Cameron JD, Doucette S, Hadjiyannakis S, Tulloch H, et al. Effects of aerobic training, resistance training, or both on brain-derived neurotrophic factor in adolescents with obesity: The hearty randomized controlled trial. Physiol Behav. 2018;191:138–45.
Pariante CM, Miller AH. Glucocorticoid receptors in major depression: relevance to pathophysiology and treatment. Biol Psychiatry. 2001;49(5):391–404. https://doi.org/10.1016/s0006-3223(00)01088-x .
Pietrelli A, Di Nardo M, Masucci A, Brusco A, Basso N, Matkovic L. Lifelong Aerobic Exercise Reduces the Stress Response in Rats. Neuroscience. 2018;376:94-107. https://doi.org/10.1016/j.neuroscience.2018.02.019 .
Helgadóttir B, Forsell Y, Hallgren M, Möller J, Ekblom Ö. Long-term effects of exercise at different intensity levels on depression: A randomized controlled trial. Prev Med. 2017;105:37–46.
Balchin R, Linde J, Blackhurst D, Rauch HL, Schönbächler G. Sweating away depression? The impact of intensive exercise on depression. J Affect Disord. 2016;200:218–21.
Legrand F, Heuze JP. Antidepressant effects associated with different exercise conditions in participants with depression: a pilot study. J Sport Exerc Psychol. 2007;29(3):348–64. https://doi.org/10.1123/jsep.29.3.348 .
Song D, Yu DSF. Effects of a moderate-intensity aerobic exercise programme on the cognitive function and quality of life of community-dwelling elderly people with mild cognitive impairment: A randomised controlled trial. Int J Nurs Stud. 2019;93:97–105.
Matas-Navarro P, Carratalá-Ros C, Olivares-García R, Martínez-Verdú A, Salamone JD, Correa M. Sex and age differences in mice models of effort-based decision-making and anergia in depression: the role of dopamine, and cerebral-dopamine-neurotrophic-factor. Psychopharmacology. 2023;240(11):2285–302.
Article CAS PubMed PubMed Central Google Scholar
Gordon BA, Knapman LM, Lubitz L. Graduated exercise training and progressive resistance training in adolescents with chronic fatigue syndrome: a randomized controlled pilot study. Clin Rehabil. 2010;24(12):1072–9.
Bouaziz W, Schmitt E, Vogel T, et al. Effects of a short-term Interval Aerobic Training Programme with active Recovery bouts (IATP-R) on cognitive and mental health, functional performance and quality of life: A randomised controlled trial in sedentary seniors. Int J Clin Pract. 2019;73(1):e13219. https://doi.org/10.1111/ijcp.13219 .
Fang Y, Li Y, Liao X, Deng J, Wang Q, Liang J, Yan B. Corydalis yanhusuo polysaccharides ameliorate chronic stress-induced depression in mice through gut microbiota-derived short-chain fatty acid activation of 5-hydroxytryptamine signaling. J Med Food. 2023;26(12):890–901.
Kwok JYY, Kwan JCY, Auyeung M, Mok VCT, Lau CKY, Choi KC, Chan HYL. Effects of Mindfulness Yoga vs Stretching and Resistance Training Exercises on Anxiety and Depression for People With Parkinson Disease: A Randomized Clinical Trial. JAMA Neurol. 2019;76(7):755–63.
Dinas PC, Koutedakis Y, Flouris AD. Effects of exercise and physical activity on depression. Ir J Med Sci. 2011;180(2):319–25.
Vetrovsky T, Fortova T, Conesa-Ros E, et al. Increased Cardiopulmonary Fitness Is Associated with a Greater Reduction in Depression among People Who Underwent Bariatric Surgery.Int J Environ Res Public Health . 2021;18(5):2508. https://doi.org/10.3390/ijerph18052508 .
Greenwood SA, Lindup H, Taylor K, Koufaki P, Rush RW, Macdougall IC, Mercer THJN, dialysis, transplantation : official publication of the European Dialysis, Association TA-ER: Evaluation of a pragmatic exercise rehabilitation programme in chronic kidney disease. 2012;27(Suppl 3):iii126–134.
North TC, McCullagh P, Tran ZV. Effect of exercise on depression. Exerc Sport Sci Rev. 1990;18:379–415.
Download references
Acknowledgements
The authors would like to thank Liu Yongfeng, Wang Tong, Deng Jiaxin , Qin Wenjing their assistance with this study.
National Social Science Foundation 22XTY013.
Author information
Authors and affiliations.
School of Sports Training, Chengdu Sport University, Chengdu, 610041, Sichuan, China
Weicheng Li, Yongfeng Liu, Jiaxin Deng & Tong Wang
You can also search for this author in PubMed Google Scholar
Contributions
Conceptualization: [Li weicheng], [Liu yongfeng], Methodology: [Li weicheng], [Liu yongfeng], Data Collection: [Li weicheng], [wang tong], [Deng jiaxin], Data Analysis: [Li weicheng], [Deng jiaxin], [wang tong], Writing—Original Draft Preparation: [Li weicheng], Writing—Review & Editing: [Li weicheng], [Liu yongfeng], Funding support: [Liu yongfeng].
Corresponding author
Correspondence to Yongfeng Liu .
Ethics declarations
Ethics approval and consent to participate.
Not applicable.
Consent for publication
Competing interests.
The authors declare no competing interests.
Additional information
Publisher’s note.
Springer Nature remains neutral with regard to jurisdictional claims in published maps and institutional affiliations.
Supplementary Information
Supplementary material 1, rights and permissions.
Open Access This article is licensed under a Creative Commons Attribution-NonCommercial-NoDerivatives 4.0 International License, which permits any non-commercial use, sharing, distribution and reproduction in any medium or format, as long as you give appropriate credit to the original author(s) and the source, provide a link to the Creative Commons licence, and indicate if you modified the licensed material. You do not have permission under this licence to share adapted material derived from this article or parts of it. The images or other third party material in this article are included in the article’s Creative Commons licence, unless indicated otherwise in a credit line to the material. If material is not included in the article’s Creative Commons licence and your intended use is not permitted by statutory regulation or exceeds the permitted use, you will need to obtain permission directly from the copyright holder. To view a copy of this licence, visit http://creativecommons.org/licenses/by-nc-nd/4.0/ .
Reprints and permissions
About this article
Cite this article.
Li, W., Liu, Y., Deng, J. et al. Influence of aerobic exercise on depression in young people: a meta-analysis. BMC Psychiatry 24 , 571 (2024). https://doi.org/10.1186/s12888-024-06013-6
Download citation
Received : 26 December 2023
Accepted : 09 August 2024
Published : 20 August 2024
DOI : https://doi.org/10.1186/s12888-024-06013-6
Share this article
Anyone you share the following link with will be able to read this content:
Sorry, a shareable link is not currently available for this article.
Provided by the Springer Nature SharedIt content-sharing initiative
- Aerobic Exercise
- Young people
BMC Psychiatry
ISSN: 1471-244X
- General enquiries: [email protected]
- - Google Chrome
Intended for healthcare professionals
- My email alerts
- BMA member login
- Username * Password * Forgot your log in details? Need to activate BMA Member Log In Log in via OpenAthens Log in via your institution

Search form
- Advanced search
- Search responses
- Search blogs
- Comparative oral...
Comparative oral monotherapy of psilocybin, lysergic acid diethylamide, 3,4-methylenedioxymethamphetamine, ayahuasca, and escitalopram for depressive symptoms: systematic review and Bayesian network meta-analysis
- Related content
- Peer review
- Tien-Wei Hsu , doctoral researcher 1 2 3 ,
- Chia-Kuang Tsai , associate professor 4 ,
- Yu-Chen Kao , associate professor 5 6 ,
- Trevor Thompson , professor 7 ,
- Andre F Carvalho , professor 8 ,
- Fu-Chi Yang , professor 4 ,
- Ping-Tao Tseng , assistant professor 9 10 11 12 ,
- Chih-Wei Hsu , assistant professor 13 ,
- Chia-Ling Yu , clinical pharmacist 14 ,
- Yu-Kang Tu , professor 15 16 ,
- 1 Department of Psychiatry, E-DA Dachang Hospital, I-Shou University, Kaohsiung, Taiwan
- 2 Department of Psychiatry, E-DA Hospital, I-Shou University, Kaohsiung, Taiwan
- 3 Graduate Institute of Clinical Medicine, College of Medicine, Kaohsiung Medical University, Kaohsiung, Taiwan
- 4 Department of Neurology, Tri-Service General Hospital, National Defense Medical Centre, Taipei, Taiwan
- 5 Department of Psychiatry, National Defense Medical Centre, Taipei, Taiwan
- 6 Department of Psychiatry, Beitou Branch, Tri-Service General Hospital, Taipei, Taiwan
- 7 Centre for Chronic Illness and Ageing, University of Greenwich, London, UK
- 8 IMPACT (Innovation in Mental and Physical Health and Clinical Treatment) Strategic Research Centre, School of Medicine, Barwon Health, Deakin University, Geelong, VIC, Australia
- 9 Institute of Biomedical Sciences, National Sun Yat-sen University, Kaohsiung, Taiwan
- 10 Department of Psychology, College of Medical and Health Science, Asia University, Taichung, Taiwan
- 11 Prospect Clinic for Otorhinolaryngology and Neurology, Kaohsiung, Taiwan
- 12 Institute of Precision Medicine, National Sun Yat-sen University, Kaohsiung, Taiwan
- 13 Department of Psychiatry, Kaohsiung Chang Gung Memorial Hospital and Chang Gung University College of Medicine, Kaohsiung, Taiwan
- 14 Department of Pharmacy, Chang Gung Memorial Hospital Linkou, Taoyuan, Taiwan
- 15 Institute of Health Data Analytics and Statistics, College of Public Health, National Taiwan University, Taipei, Taiwan
- 16 Department of Dentistry, National Taiwan University Hospital, Taipei, Taiwan
- Correspondence to: C-S Liang lcsyfw{at}gmail.com
- Accepted 20 June 2024
Objective To evaluate the comparative effectiveness and acceptability of oral monotherapy using psychedelics and escitalopram in patients with depressive symptoms, considering the potential for overestimated effectiveness due to unsuccessful blinding.
Design Systematic review and Bayesian network meta-analysis.
Data sources Medline, Cochrane Central Register of Controlled Trials, Embase, PsycINFO, ClinicalTrial.gov, and World Health Organization’s International Clinical Trials Registry Platform from database inception to 12 October 2023.
Eligibility criteria for selecting studies Randomised controlled trials on psychedelics or escitalopram in adults with depressive symptoms. Eligible randomised controlled trials of psychedelics (3,4-methylenedioxymethamphetamine (known as MDMA), lysergic acid diethylamide (known as LSD), psilocybin, or ayahuasca) required oral monotherapy with no concomitant use of antidepressants.
Data extraction and synthesis The primary outcome was change in depression, measured by the 17-item Hamilton depression rating scale. The secondary outcomes were all cause discontinuation and severe adverse events. Severe adverse events were those resulting in any of a list of negative health outcomes including, death, admission to hospital, significant or persistent incapacity, congenital birth defect or abnormality, and suicide attempt. Data were pooled using a random effects model within a Bayesian framework. To avoid estimation bias, placebo responses were distinguished between psychedelic and antidepressant trials.
Results Placebo response in psychedelic trials was lower than that in antidepression trials of escitalopram (mean difference −3.90 (95% credible interval −7.10 to −0.96)). Although most psychedelics were better than placebo in psychedelic trials, only high dose psilocybin was better than placebo in antidepression trials of escitalopram (mean difference 6.45 (3.19 to 9.41)). However, the effect size (standardised mean difference) of high dose psilocybin decreased from large (0.88) to small (0.31) when the reference arm changed from placebo response in the psychedelic trials to antidepressant trials. The relative effect of high dose psilocybin was larger than escitalopram at 10 mg (4.66 (95% credible interval 1.36 to 7.74)) and 20 mg (4.69 (1.64 to 7.54)). None of the interventions was associated with higher all cause discontinuation or severe adverse events than the placebo.
Conclusions Of the available psychedelic treatments for depressive symptoms, patients treated with high dose psilocybin showed better responses than those treated with placebo in the antidepressant trials, but the effect size was small.
Systematic review registration PROSPERO, CRD42023469014.
Introduction
Common psychedelics belong to two classes: classic psychedelics, such as psilocybin, lysergic acid diethylamide (known as LSD), and ayahuasca; and entactogens, such as 3,4-methylenedioxymethamphetamine (MDMA). 1 Several randomised controlled trials have shown efficacy of psychedelics for people with clinical depression. 2 3 The proposed mechanism of its fast and persistent antidepressant effects is to promote structural and functional neuroplasticity through the activation of intracellular 5-HT 2A receptors in the cortical neurons. 4 Additionally, the increased neuroplasticity was associated with psychedelic’s high affinity directly binding to brain derived neurotrophic factor receptor TrkB, indicating a dissociation between the hallucinogenic and plasticity promoting effects of psychedelics. 5 A meta-analysis published in 2023 reported that the standardised mean difference of psychedelics for depression reduction ranged from 1.37 to 3.12, 2 which are considered large effect sizes. 6 Notably, the standardised mean difference of antidepressant trials is approximately 0.3 (a small effect size). 7 8
Although modern randomised controlled trials involving psychedelics usually use a double blinded design, the subjective effects of these substances can compromise blinding. 9 Unsuccessful blinding may lead to differing placebo effects between the active and control groups, potentially introducing bias into the estimation of relative treatment effects. 10 Concerns have arisen regarding the overestimated effect sizes of psychedelics due to the issues of blinding and response expectancy. 9 Psychedelic treatment is usually administered with psychological support or psychotherapy, and thereby the isolated pharmacological effects of psychedelics remain to be determined. 2 Surprisingly, on 1 July 2023, Australia approved psilocybin for the treatment of depression 11 ; the first country to classify psychedelics as a medicine at a national level.
To date, only one double blind, head-to-head randomised controlled trial has directly compared a psychedelic drug (psilocybin) with an antidepressant drug (escitalopram) for patients with major depressive disorder. 12 This randomised controlled trial reported that psilocybin showed a better efficacy than escitalopram on the 17 item Hamilton depression rating scale (HAMD-17).
We aimed to assess the comparative effectiveness and acceptability of oral monotherapy with psychedelics and escitalopram in patients experiencing depressive symptoms. Given that unsuccessful blinding can potentially lead to a reduced placebo response in psychedelic trials, we distinguished between the placebo responses in psychedelic and antidepressant trials. We also investigated the differences in patient responses between people who received extremely low dose psychedelics as a placebo and those who received a placebo in the form of a fake pill, such as niacin, in psilocybin trials. 13 14 Our study allowed for a relative effect assessment of psychedelics compared with placebo responses observed in antidepressant trials.
The study protocol was registered with PROSPERO (CRD42023469014). We followed the Preferred Reporting Items for Systematic Reviews and Meta-Analyses (PRISMA) extension statement for reporting systematic reviews incorporating network meta-analysis (NMA) (appendix 1). 15
Data sources and searches
A comprehensive search of the Medline, Cochrane Central Register of Controlled Trials (CENTRAL), Embase, PsycINFO, ClinicalTrial.gov, and World Health Organization’s International Clinical Trials Registry Platform databases were performed without language restrictions from database inception to 12 October 2023. We also searched the grey literature and reviewed reference lists of the included studies and related systematic reviews. 2 3
Study selection
Eligible studies were randomised controlled trials with parallel group or crossover designs. We included: (i) adults (≥18 years) with clinically diagnosed depression (eg, major depressive disorder, bipolar disorder, or other psychiatric disorders with comorbid clinical depression) or life threatening diagnoses and terminal illness with depressive symptoms; and (ii) adults with assessment of treatment response (preapplication/postapplication) using standard, validated, and internationally recognised instruments, such as HAMD-17. The outcome of interest was the change in depressive symptoms at the end of treatment compared with the controls, and we only extracted data from the first phase of crossover randomised controlled trials to avoid carry-over effects. Eligible psychedelic randomised controlled trials (including psilocybin, lysergic acid diethylamide, MDMA, and ayahuasca without dosage limit) required oral monotherapy without the concomitant use of antidepressants. For escitalopram, we included only fixed dose randomised controlled trials that compared at least two arms with different doses of oral form escitalopram (maximum dose of 20 mg/day) with placebo because psychedelic therapies usually use a fixed dose study design. We also included randomised controlled trials that evaluated psychedelic monotherapy compared with escitalopram monotherapy. We excluded follow-up studies and studies with healthy volunteers. We also excluded conference abstracts, editorials, reviews, meta-analyses, case reports, and case series, as well as publications reporting duplicate data. We did not consider ketamine because this drug is usually administered parenterally and is not a classic psychedelic. 16 Screening and selection of the studies were performed independently by two authors. Discrepancies in study inclusion were resolved by deliberation among the reviewer pairs or with input from a third author. Appendix 2 shows the complete search strategies, and appendix 3 presents the reasons for exclusion.
Definition of outcomes, data extraction, and risk of bias assessment
The primary outcome was change in depressive symptoms from baseline (continuous outcome), as measured by a validated rating scale, such as HAMD-17. When multiple measurement tools were used, they were selected in the following order: the HAMD-17, Montgomery-Åsberg depression rating scale, and Beck depression inventory (second edition). To improve interpretability, all extracted depression scores were converted to corresponding HAMD-17 scores using a validated method. 17 We used a conservative correlation coefficient of 0.5 or other statistics (eg, t statistics) to calculate the standard deviation of change from baseline when unreported. 18 The secondary outcomes were all cause discontinuation and severe adverse events (categorical outcomes). Severe adverse events were classified as those resulting in any of a list of negative health outcomes including, death, admission to hospital, significant or persistent incapacity, congenital birth defect or abnormality, and suicide attempt. Outcome data were extracted from original intention-to-treat or last observation carrying forward analysis, as well as from estimates of mixed-effect models for repeated measures.
Two authors independently extracted and reviewed the data, each being reviewed by another author. WebPlot Digitizer ( https://automeris.io/WebPlotDigitizer/ ) was used to extract numerical data from the figures. Two authors independently used the Cochrane randomised trial risk of bias tool (version 2.0) to assess the risk of bias in the included trials, and discrepancies were resolved by consensus. 19
Data synthesis
To estimate the relative effect between two interventions, we computed mean difference on the basis of change values (with 95% credible interval) for continuous outcomes (change in depressive symptoms) and odds ratios for categorical outcomes (all cause discontinuation and severe adverse event). To assess the clinical significance of the relative effect, we evaluated whether the mean difference exceeded the minimal important difference, which is estimated to be 3 points for HAMD-17. 20 We defined high, low, and extremely low doses of the included psychedelics as follows: (i) psilocybin: high dose (≥20 mg), extremely low dose (1-3 mg), low dose (other range); and (ii) MDMA: high dose (≥100 mg), extremely low dose (≤40 mg), low dose (other range). Escitalopram was divided into escitalopram 10 mg and escitalopram ≥20 mg. In previous clinical trials, a dose of 1 mg of psilocybin or a dose range of 1-3 mg/70 kg were used as an active control because these doses were believed not to produce significant psychedelic effects. 21 22 A dose of 5 mg/70 kg can produce noticeable psychedelic effects. 22 In many two arm psilocybin trials, the psilocybin dose in the active group typically falls within the range of 20-30 mg. 12 21 23 24 In a three arm trial, 25 mg was defined as high dose, and 10 mg was considered a moderate dose. 21 Another clinical trial also defined 0.215 mg/kg of psilocybin as a moderate dose for the active group. 25 Therefore, we used 20 mg and 3 mg as the boundaries for grouping psilocybin doses; when the dosage was calculated per kilogram in the study, we converted it to per 70 kg. For MDMA, in two trials with three arms, 125 mg was defined as high dose, and 30-40 mg was defined as active control. 26 27 Thus, we used 100 mg and 40 mg as the boundaries for grouping MDMA doses.
We conducted random effects network meta-analysis and meta-analysis within a Bayesian framework. 28 29 Previous meta-analyses considered all control groups as a common comparator; however, concerns have been raised regarding the overestimated effect sizes of psychedelics because of unsuccessful blinding and poor placebo response. 9 Therefore, we treated the three treatments as distinct interventions: the placebo response observed in psychedelic trials, the placebo response observed in antidepressant escitalopram trials, and extremely low dose psychedelics (ie, psilocybin and MDMA). We calculated the relative effects of all interventions compared with these three groups, indicating the following three conditions: (1) the treatment response of placebo response in the psychedelic trials is assumed to be lower than that of placebo response in antidepressant trials because of unsuccessful blinding. 9 As such, the relative effects compared with placebo response in the psychedelic trials represented potential overestimated effect sizes. (2) the placebo response in antidepressant trials is assumed to be the placebo response in antidepressant trials with adequate blinding, therefore, the relative effects compared with placebo response in antidepressant trials represents effect sizes in trials with adequate blinding. (3) Psychedelic drugs are usually administered with psychotherapy 13 or psychological support, 14 the relative effects of psychedelics compared with extremely low dose psychedelics might eliminate the concomitant effects from psychotherapeutic support, approximating so-called pure pharmacological effects.
In network meta-analysis, the validity of indirect comparison relies on transitivity assumption. 30 We assessed the transitivity assumption by comparing the distribution of potential effect modifies across treatment comparisons. In addition, we assessed whether the efficacy of escitalopram is similar in placebo controlled randomised controlled trials (escitalopram v placebo response in antidepressant trials) and in the head-to-head randomised controlled trial (psilocybin v escitalopram) using network meta-analysis. 12 Furthermore, we assessed the efficacy of the different placebo responses (placebo response in the psychedelic trials v placebo response in antidepressant trials) as additional proof of transitivity. If the placebo response in antidepressant trials was better than that in the psychedelic trials, the transitivity assumption did not hold when grouping placebo response in antidepressant trials and placebo response in the psychedelic trials together. Finally, for the primary outcome (change in depressive symptoms), network meta-regression analyses were conducted to evaluate the impact of potential effect modifiers, including proportion of men and women in the study, mean age, baseline depression severity, disorder type, and follow-up assessment period. We assumed a common effect on all treatment comparisons for each of the effect modifiers. In other words, all interactions between the treatment comparisons and the effect modifier were constrained to be identical.
We also conducted the following sensitivity analyses: analysing studies of patients with major depressive disorder; excluding studies with a high risk of bias; adjusting for baseline depression severity; and using correlation coefficient of zero (most conservative) to calculate the standard deviation of change from baseline when unreported.
Publication bias was assessed by visual inspection of a comparison adjusted funnel plots. The first funnel plot used placebo response in the psychedelic trials as the comparator. The second funnel plot used placebo response in antidepressant trials as the comparator. The third funnel plot used both placebo response in the psychedelic trials and placebo response in antidepressant trials as comparators simultaneously. Additionally, we conducted the Egger test, Begg test, and Thompson test to examine the asymmetry of the third funnel plot. A previous meta-analysis reported that the standardised mean difference of psychedelics for depression reduction ranged from 1.37 to 3.12. 2 Therefore, we also transformed the effect size of mean difference to standardised mean difference (Hedges’ g) for the primary outcome. The global inconsistency of the network meta-analysis was examined by fitting an unrelated main effects model. Local inconsistency of the network meta-analysis was examined using node splitting methods. 31 Four Markov chains were implemented. 50 000 iterations occurred per chain and the first 20 000 iterations for each chain were discarded as a warm-up. Convergence was assessed by visual inspection of the trace plots of the key parameters for each analysis. The prior settings and convergence results are shown in appendix 4. All statistical analyses were done using R version 4.3.1. The network meta-analysis and pairwise meta-analysis within a Bayesian framework were fitted using the Bayesian statistical software called Stan within the R packages multinma 28 and brms, 29 respectively. The frequentist random effects network meta-analysis, funnel plots, and tests for funnel plot asymmetry were conducted using the R package netmeta. Reasons for protocol changes are in appendix 5.
Assessment certainty of evidence for the primary outcome
The certainty of evidence produced by the network meta-analysis was evaluated using GRADE (Grading of recommendations, assessment, development and evaluation). 32 33 We used a minimally contextualised framework with the value of 3 (minimal important difference) as our decision threshold. The certainty of evidence refers to our certainty that the intervention had, relative to minimal intervention, any clinically minimal important difference. The optimal information size was calculated using a validated method. 32 33 34
Patient and public involvement
Both patients and the public are interested in research on novel depression treatments and their efficacy compared with existing antidepressants. However, due to a scarcity of available funding for recruitment and researcher training, patients and members of the public were not directly involved in the planning or writing of this manuscript. We did speak to patients about the study, and we asked a member of the public to read our manuscript after submission.
Characteristics of included study
After searching the database and excluding duplicated records, we identified 3104 unique potential studies. We then screened the titles and abstracts of these studies for eligibility and excluded 3062 of them, in which 42 studies remained. Twenty six studies were excluded after an assessment of the full text for various reasons (appendix 3). We identified three additional studies through a manual search resulting in total 19 eligible studies (efigure 1). Details of the characteristics of the included studies are shown in etable 1. Protocols of psychological support or psychotherapy with psychedelic treatment are shown in etable 2. Overall, 811 people (mean age of 42.49 years, 54.2% (440/811) were women) were included in psychedelic trials (15 trials), and 1968 participants (mean age of 39.35 years, 62.5% (1230/1968) were women) were included in escitalopram trials (five trials).
Risk of bias of the included studies
No psychedelic study (0/15) had a high overall risk of bias (efigure 2A and efigure 3A). The percentages of studies with high, some concerns, or low risk of bias in the 15 psychedelic trials were as follows: 0% (k=15), 33% (k=5), and 67% (k=10) for randomisation; 0% (k=0), 33% (k=5), and 67% (k=10) for deviations from intended interventions; 0% (k=0), 13% (k=2), and 87% (k=13) for missing outcome data; 0% (k=0), 33% (k=5), and 67% (k=10) for measurements of outcomes; 0% (k=0), 67% (k=1), and 93% (k=14) for selection of reported results. No non-psychedelic studies (0/5) were rated as high risk of bias (efigure 2B and efigure 3B). The percentages of studies with high, some concerns, and low risk of bias in the five non-psychedelic trials were as follows: 0% (k=0), 80% (k=4), and 20% (k=1) for randomisation; 0% (k=0), 100% (k=5), and 0% (k=0) for deviations from intended interventions; 0% (k=0), 80% (k=4), and 20% (k=1) for missing outcome data; 0% (k=0), 80% (k=4), and 20% (k=1) for measurements of outcomes; 0% (k=0), 20% (k=1), and 80% (k=4) for selection of reported results.
Network meta-analysis
In the network structure, all interventions were connected, with two main structures ( fig 1 ). All psychedelics were compared with placebo response in the psychedelic trials, and escitalopram was compared with placebo response in antidepressant trials. A head-to-head comparison of high dose psilocybin and 20 mg escitalopram connected the two main structures. 12

Network structure. LSD=lysergic acid diethylamide; MDMA=3,4-methylenedioxymethamphetamine
- Download figure
- Open in new tab
- Download powerpoint
In the main network meta-analysis, all interventions, except for extremely low dose and low dose MDMA, were associated with a larger mean difference exceeding the minimal important difference of 3 points on the HAMD-17 than with placebo response in the psychedelic trials ( fig 2 ). Notably, placebo response in antidepressant trials (3.79 (95% credibile interval 0.77 to 6.80)) and extremely low dose psilocybin (3.96 (0.61 to 7.17)) were better than placebo response in the psychedelic trials, with mean differences exceeding 3 and 95% credibile intervals that did not cross zero. Additionally, in comparison with placebo response in antidepressant trials ( fig 2 ), the relative effects of high dose psilocybin (6.52 (3.19 to 9.57)), escitalopram 10 mg (1.86 (0.21 to 3.50)), and escitalopram 20 mg (1.82 (0.16 to 3.43)) did not cross zero. Only high dose psilocybin resulted in a mean difference that was greater than 3. The standardised mean difference of high dose psilocybin decreased from large (0.88) to small (0.31) when the reference arm was changed from placebo response in the psychedelic trials to placebo response in antidepressant trials.

Forest plots of network meta-analytical estimates v different reference arms by observed placebo response. The dotted line represents the minimal important difference of 3 whereas the red line indicates 0. LSD=lysergic acid diethylamide; MDMA=3,4-methylenedioxymethamphetamine
When compared with extremely low dose psilocybin ( fig 2 ), only the relative effects of high dose psilocybin (6.35 (95% credibile interval 3.41 to 9.21)) and placebo response in the psychedelic trials (−3.96 (−7.17 to −0.61)) showed a larger mean difference exceeding 3, without crossing zero. All relative effects between interventions are showed in efigure 4. Importantly, the mean differences of high dose psilocybin compared with escitalopram 10 mg (4.66 (1.36 to 7.74); standardised mean difference 0.22), escitalopram 20 mg (4.69 (1.64 to 7.54); 0.24), high dose MDMA (4.98 (1.23 to 8.67); 0.32), and low dose psilocybin (4.36 (1.20 to 7.51); 0.32) all exceeded 3 and did not cross zero (efigure 4).
Transitivity assumption
The assessment of transitivity assumption is showed in efigure 5 and efigure 6. We compared the efficacy of escitalopram in the placebo controlled antidepressant trials 8 with that in the head-to-head trial (psilocybin v escitalopram) 12 using network meta-analysis and pairwise meta-analysis. The results of the network meta-analysis showed that the relative effects between these two study designs (0.64 (95% credibile interval −4.41 to 5.40), efigure 6A; 1.94 (−2.66 to 6.14), efigure 6B) included zero, and the mean differences did not exceed 3. Placebo response in antidepressant trials was better than placebo response in the psychedelic trials with a small effect size (3.79 (0.77 to 6.80), standardised mean difference 0.2), and the mean difference exceed 3 ( fig 2 ).
Sensitivity analyses
When including only patients with major depressive disorder, the relative effects of escitalopram 20 mg, escitalopram 10 mg, ayahuasca, and high dose psilocybin were better than placebo response in antidepressant trials, while placebo response in the psychedelic trials was worse than placebo response in antidepressant trials ( fig 3 ). However, only the mean differences for high dose psilocybin (6.82 (95% credibile interval 3.84 to 9.67)), ayahuasca (5.38 (0.02 to 10.61)), and placebo response in the psychedelic trials (−4.00 (−6.87 to −1.13)) exceeded 3. When compared with extremely low dose psilocybin (excluding the effects from concomitant psychotherapeutic support), only the 95% credibile intervals of the relative effects of high dose psilocybin (4.36 (0.54 to 8.27); standardised mean difference 0.30) and placebo response in the psychedelic trials (−6.46 (−10.41 to −2.32), standardised mean difference −0.46) exceeded 3 and did not cross zero ( fig 3 ). All of the relative effects between interventions are showed in efigure 7. Notably, the relative effects of high dose psilocybin compared with escitalopram 10 mg (4.96 (1.97 to 7.82)), escitalopram 20 mg (4.97 (2.19 to 7.64)), and low dose psilocybin (3.82 (0.61 to 7.04)) all exceeded 3 and did not cross zero (efigure 7).

Forest plots of network meta-analytical estimates when considering a population with major depressive disorder
The other three sensitivity analyses showed similar findings with the main analyses: exclusion of studies with high risk of bias (efigure 8); adjustment of baseline depression severity (efigure 9); and use of most conservative correlation coefficient of zero (efigure 10).
All cause discontinuation and severe adverse event
When referencing placebo in psychedelic trials, no interventions were associated with higher risks of all cause discontinuation rate nor severe adverse event rate (efigure 11).
Network meta-regression and publication bias
In network meta-regression analyses, the 95% credibile intervals of the relative effects of the baseline depressive severity, mean age, and percentage of women, crossed zero (etable 3). The results of the statistical tests (Egger, Begg, and Thompson-Sharp tests) for funnel plot asymmetry and visual inspection of funnel plots did not show publication bias (efigure 12). The results of GRADE assessment are provided in the efigure 13. Most of the certainty of evidence for treatment comparisons was moderate or low.
Consistency assumptions
The back calculation methods for all the models (appendix 6) did not show any inconsistencies. The node splitting methods also did not show any inconsistencies (appendix 7).
Principal findings
This network meta-analysis investigated the comparative effectiveness between psychedelics and escitalopram for depressive symptoms. Firstly, we found that the placebo response observed in antidepressant trials was associated with greater effectiveness than that observed in psychedelic trials. Secondly, when compared with placebo responses in antidepressant trials, only escitalopram and high dose psilocybin were associated with greater effectiveness, and only high dose psilocybin exceeded minimal important difference of 3. Notably, the effect size of high dose psilocybin decreased from large to small. Thirdly, among the included psychedelics, only high dose psilocybin was more likely to be better than escitalopram 10 mg or 20 mg, exceeding the minimally important difference of 3. Fourthly, in patients with major depressive disorder, escitalopram, ayahuasca, and high dose psilocybin were associated with greater effectiveness than placebo responses in antidepressant trials; however, only high dose psilocybin was better than extremely low dose psilocybin, exceeding minimal important difference of 3. Taken together, our study findings suggest that among psychedelic treatments, high dose psilocybin is more likely to reach the minimal important difference for depressive symptoms in studies with adequate blinding design, while the effect size of psilocybin was similar to that of current antidepressant drugs, showing a mean standardised mean difference of 0.3. 7
Comparison with other studies
In a randomised controlled trial, treatment response was defined as the response observed in the active arm; placebo response was defined as the response observed in the control (placebo) arm. 10 Treatment response consists of non-specific effects, placebo effect, and true treatment effect; placebo response consisted of non-specific effects and placebo effect. Therefore, when the placebo effect is not the same for the active and control arms within an randomised controlled trial, the estimation of the true treatment effect is biased. For example, in a psychedelic trial, unsuccessful blinding may occur due to the profound subjective effects of psychedelics. This unblinding may lead to high placebo effect in the active arm and low placebo effect in the control arms, and the true treatment effect is overestimated. 10 Without addressing unequal placebo effects within studies, the estimation of meta-analysis and network meta-analysis are biased. 10 However, in most psychedelic trials, blinding was either reported as unsuccessful or not assessed at all. For example, two trials of lysergic acid diethylamide reported unsuccessful blinding, 35 36 whereas the trial of ayahuasca only reported that five of 10 participants misclassified the placebo as ayahuasca. 37 In trials of MDMA, participants' accuracy in guessing which treatment arm they were in ranged from approximately 60-90%. 26 27 38 39 40 In the case of most psilocybin trials, blinding was not assessed, with the exception of the study by Ross and colleagues in 2016. 13 In that study, participants were asked to guess whether the psilocybin or an active control was received, and the correct guessing rate was 97%. In our study, we established several network meta-analysis models addressing this issue, and we found that placebo response in the psychedelic trials was associated with less effectiveness than that in antidepressant trials. Therefore, the effect sizes of psychedelics compared with placebo response observed in psychedelic trials may be overestimated. All of the psychedelics’ 95% credibile intervals of the relative effects crossed zero when compared with the placebo response in antidepressant trials, except for high dose psilocybin.
The comparisons between psychedelics and escitalopram showed that high dose psilocybin was more likely to be better than escitalopram. Psilocybin was usually administered with psychotherapy or psychological support. 13 14 Therefore, the greater effectiveness of psilocybin may be from not only pharmacological effects but also psychotherapeutic support. However, we also found that high doses of psilocybin was associated greater effectiveness than extremely low doses of psilocybin. This effect also indicates that the effectiveness of psilocybin cannot be attributed only to concomitant psychotherapy or psychological support.
In patients with major depressive disorder, ayahuasca, low dose psilocybin, high dose psilocybin, escitalopram 10 mg, and escitalopram 20 mg were associated with greater effectiveness than the placebo response in antidepressant trials . However, when compared with extremely low dose psilocybin, only high dose psilocybin was associated with better effectiveness; the standardised mean difference decreased from 0.38 (compared with placebo response in antidepressant trials) to 0.30 (compared with extremely low dose psilocybin). As such, the effectiveness of psilocybin should be considered with concomitant psychotherapeutic support in people with major depressive disorder. The effect size of high dose psilocybin was similar with antidepressant trials of patients with major depressive disorder showing a mean standardised mean difference of 0.3. 7 8
Strengths and limitations of this study
This study has several strengths. We conducted separate analyses for placebo response in antidepressant trials, placebo response in psychedelic trials, and an extremely low active dose of psychedelics, thereby mitigating the effect of placebo response variations across different studies. This approach allowed us to assess the efficacy of psychedelics more impartially and make relatively unbiased comparisons than if these groups were not separated. This study supported the transitivity assumption of the efficacy of escitalopram in placebo controlled antidepressant trials with that in psilocybin versus escitalopram head-to-head trial, thereby bridging the escitalopram trials and psychedelic trials. We also performed various sensitivity analyses to ensure the validation of our statistical results.
Nevertheless, our study has several limitations. Firstly, we extracted only the acute effects of the interventions. A comparison of the long term effects of psychedelics and escitalopram remains unclear. Secondly, participants in the randomised controlled trials on MDMA were predominantly diagnosed with post-traumatic stress disorder, whereas participants in the randomised controlled trials on escitalopram were patients with major depressive disorder. However, depressive symptoms in post-traumatic stress disorder could be relatively treatment resistant, requiring high doses of psychotropic drugs. 41 Moreover, our study focused not only on major depressive disorder but also on the generalisability of psychedelic treatment for depressive symptoms. Thirdly, although all available studies were included, the sample size of the psychedelic randomised controlled trials was small (k=15). Fourthly, when using extremely low dose psychedelics as a reference group, the relative effect may also eliminate some pharmacological effects because our study found that extremely low dose psychedelics could not be considered a placebo. Fifthly, in network meta-analysis, direct evidence for one treatment comparison may serve as indirect evidence for other treatment comparisons, 42 and biases in the direct evidence might affect estimates of other treatment comparisons. Because the absolute effect of escitalopram in the head-to-head trial (high dose psilocybin v escitalopram 20 mg) 12 was lower than those of placebo controlled trials, the relative effects of high dose psilocybin might be slightly overestimated when compared with other treatments in the current study. We addressed this issue by use of a Bayesian network meta-analysis, distinguishing between placebo response in psychedelic trials and placebo response in antidepressant trials. Specifically, we only considered that the 95% credibile interval of the relative effect between two comparisons did not cross zero. Indeed, the relative effect of escitalopram 20 mg between these two study designs included zero. Finally, our network meta-analysis may not have sufficient statistical power to detect potential publication bias due to the scarcity of trials and participants.
Implications and conclusions
Serotonergic psychedelics, especially high dose psilocybin, appeared to have the potential to treat depressive symptoms. However, study designs may have overestimated the efficacy of psychedelics. Our analysis suggested that the standardised mean difference of high dose psilocybin was similar to that of current antidepressant drugs, showing a small effect size. Improved blinding methods and standardised psychotherapies can help researchers to better estimate the efficacy of psychedelics for depressive symptoms and other psychiatric conditions.
What is already known on this topic
Psychedelic treatment resulted in significant efficacy in treating depressive symptoms and alleviating distress related to life threatening diagnoses and terminal illness
Meta-analyses have reported standardised mean difference of psychedelics for depression reduction ranging from 1.37 to 3.12, while antidepressant trials were approximately 0.3
No network meta-analysis has examined comparative efficacy between psychedelics and antidepressants for depressive symptoms, and effect sizes of psychedelics might be overestimated because of unsuccessful blinding and response expectancies
What this study adds
To avoid estimation bias, placebo responses in psychedelic and antidepressant trials were separated; placebo response in psychedelic trials was lower than that in antidepressant trials
Among all psychedelics studied, only high dose psilocybin was associated with greater effectiveness than placebo response in antidepressant trials (standardised mean difference 0.31)
Among all psychedelics, only high dose psilocybin was associated with greater effectiveness than escitalopram
Ethics statements
Ethical approval.
Not required because this study is an analysis of aggregated identified clinical trial data.
Data availability statement
The data that support the findings of this study are available from the corresponding author (C-SL) upon reasonable request.
Contributors: T-WH and C-KT contributed equally to this work and are joint first authors. Y-KT and C-SL contributed equally to this work and are joint last/corresponding authors. C-SL, T-WH, and Y-KT conceived and designed the study. T-WH, C-KT, C-WH, and P-TT selected the articles, extracted the data, and assess the risk of bias. C-LY did the systemic search. T-WH and C-SL wrote the first draught of the manuscript. TT, AFC, Y-CK, F-CY, and Y-KT interpreted the data and contributed to the writing of the final version of the manuscript. C-KT and T-WH have accessed and verified the data. C-SL and Y-KT were responsible for the decision to submit the manuscript. All authors confirmed that they had full access to all the data in the study and accept responsibility to submit for publication. The corresponding author attests that all listed authors meet authorship criteria and that no others meeting the criteria have been omitted.
Funding: The study was supported by grant from the National Science and Technology Council (NSTC 112-2314-B-016−036-MY2 and NSTC 112-2314-B-002−210-MY3). The funding source had no role in any process of our study.
Competing interests: All authors have completed the ICMJE uniform disclosure form at www.icmje.org/disclosure-of-interest/ and declare: support from National Science and Technology Council for the submitted work; no financial relationships with any organisations that might have an interest in the submitted work in the previous three years; no other relationships or activities that could appear to have influenced the submitted work.
Transparency: The lead author (C-SL) affirms that the manuscript is an honest, accurate, and transparent account of the study being reported; that no important aspects of the study have been omitted; and that any discrepancies from the study as planned
Dissemination to participants and related patient and public communities: Dissemination of the work to the public and clinical community through social media and lectures is planned.
Provenance and peer review: Not commissioned; externally peer reviewed.
This is an Open Access article distributed in accordance with the terms of the Creative Commons Attribution (CC BY 4.0) license, which permits others to distribute, remix, adapt and build upon this work, for commercial use, provided the original work is properly cited. See: http://creativecommons.org/licenses/by/4.0/ .
- Tupper KW ,
- Cleare AJ ,
- Muttoni S ,
- Ardissino M ,
- Vargas MV ,
- Dunlap LE ,
- Moliner R ,
- Brunello CA ,
- Sawilowsky SS
- Cipriani A ,
- Furukawa TA ,
- Salanti G ,
- Muthukumaraswamy SD ,
- Forsyth A ,
- Nikolakopoulou A ,
- Chaimani A ,
- Carhart-Harris R ,
- Giribaldi B ,
- Raison CL ,
- Sanacora G ,
- Woolley J ,
- Caldwell DM ,
- Marcantoni WS ,
- Akoumba BS ,
- Thorlund K ,
- Walter SD ,
- Johnston BC ,
- Higgins JPT ,
- Chandler J ,
- Sterne JAC ,
- Savović J ,
- Hengartner MP ,
- Goodwin GM ,
- Aaronson ST ,
- Alvarez O ,
- Griffiths RR ,
- Johnson MW ,
- Carducci MA ,
- Barrett FS ,
- von Rotz R ,
- Schindowski EM ,
- Jungwirth J ,
- Mithoefer MC ,
- Mithoefer AT ,
- Feduccia AA ,
- Ot’alora G M ,
- Grigsby J ,
- Poulter B ,
- ↵ Phillippo DM. multinma: Bayesian network meta-analysis of individual and aggregate data. 2020.
- Bürkner P-C
- Del Giovane C ,
- Welton NJ ,
- Brignardello-Petersen R ,
- Alexander PE ,
- GRADE Working Group
- Izcovich A ,
- Mustafa RA ,
- Brignardello-Petersen R
- Guyatt GH ,
- Holstein D ,
- Dolder PC ,
- Palhano-Fontes F ,
- Barreto D ,
- Mitchell JM ,
- Bogenschutz M ,
- Lilienstein A ,
- Wagner MT ,
- Wolfson PE ,
- Andries J ,
30 Years of Experimental Education Research in the Post-Soviet Space: A Meta-Analysis of Interventions
Description.
This is supplementary material to the article "30 Years of Experimental Education Research in the Post-Soviet Space: A Meta-Analysis of Interventions". This meta-analysis systematically evaluates the potential of available research in post-Soviet countries as a basis for an evidence-based approach to improving student achievement. The study was conducted on a selection of 41 publications describing educational interventions aimed at improving student achievement. The supplementary material provided here consists of three files that we would like to share with you: Supplementary_file_1 - xlsx database with coded characteristics of all studies and effect sizes included in the analysis; Supplementary_file_2 - a short version of the database in xlsx format with variables used in the multi-level analysis to calculate the pooled effect size and to examine moderators; Supplementary_file_3 - docx file with all R codes used for the analysis in the article.
- Open access
- Published: 22 August 2024
Incidence and factors influencing delayed onset of lactation: a systematic review and meta-analysis
- Yijuan Peng 1 , 2 na1 ,
- Ke Zhuang 1 , 2 na1 &
- Yan Huang 1 , 2
International Breastfeeding Journal volume 19 , Article number: 59 ( 2024 ) Cite this article
238 Accesses
1 Altmetric
Metrics details
Breastfeeding has many benefits for mothers and infants. Lactogenesis II is one of the key steps in the implementation of breastfeeding. If lactogenesis II occurs more than 72 h after delivery, it is termed delayed onset of lactation (DOL). DOL is associated with decreased milk production, shortened breastfeeding time, and pathological neonatal weight loss. A comprehensive summary of the incidence and factors influencing DOL is needed to provide a basis for improving breastfeeding practices and health outcomes.
Studies on the incidence and factors influencing DOL were retrieved from 13 Chinese and English databases (PubMed, Embase, Web of Science, Cochrane Library, CINAHL, etc.) from database inception to August 2023. Two researchers independently conducted the study screening, data extraction and quality evaluation. Stata 16.0 SE software was used for data analysis, and sensitivity analysis and publication bias tests were also performed. The qualitative description method was used to analyse studies that could not be combined quantitatively.
A total of 35 studies involving 19,176 parturients, including 4,922 who had DOL, were included. The mean Newcastle‒Ottawa scale score of the included studies was ≥ 6, indicating that the quality was relatively high. Finally, the incidence of DOL was 30%, and 13 factors influencing DOL with robust results and no publication bias were obtained: prepregnancy body mass index (overweight or obesity), gestational diabetes, gestational hypertension, thyroid disease during pregnancy, serum albumin levels (< 35 g/L), parity, (unscheduled) caesarean section, caesarean section history, daily sleep duration, gestational age, birth weight (< 2.5 kg), breastfeeding guidance and daily breastfeeding frequency. However, there were still six influencing factors with undetermined associations: age, gestational weight gain, birth weight (≥ 4 kg), anxiety, time of first breastfeeding session (maternal separation) and breast massage or treatment.
Conclusions
The incidence of DOL is high. Clinicians should pay attention to parturients at high risk of DOL and formulate targeted prevention strategies according to the influencing factors to reduce the occurrence of DOL and promote better maternal and infant outcomes.
Trial registration
PROSPERO (ID: CRD42023458786), September 10, 2023.
Lactation involves four stages: secretory differentiation, secretory activation, reaching volume, and maintenance of established lactation [ 1 , 2 ]. Among these stages, secretory activation (lactogenesis II) is triggered by a decrease in progesterone levels after delivery of the placenta and involves changes in prolactin and cortisol (glucocorticoid) secretion and the closure of paracellular pathways [ 3 ], indicating that a large amount of milk is being secreted by the mother [ 4 ]. Delayed onset of lactation (DOL) is defined as the occurrence of lactogenesis II 72 h after birth [ 4 ]. The most commonly used evaluation method for the onset of lactation (OL) is maternal perception of milk coming in [ 1 ]. Importantly, studies have shown that the time of OL is negatively correlated with the amount of milk produced on the 14th day postpartum [ 5 ]. DOL independently increases the risk of the cessation of any or exclusive breastfeeding at 4 weeks postpartum by 62% [ 6 ], thereby shortening the duration of breastfeeding [ 7 , 8 ] and reducing the rate of exclusive breastfeeding [ 7 , 9 ]. Moreover, DOL can increase the risk of pathological neonatal weight loss (more than 10% of the birth weight) by 7.1-fold [ 10 , 11 ]. Consequently, actively taking effective intervention measures to prevent DOL has an important impact on improving maternal and child health outcomes and breastfeeding practices.
Liu et al. [ 12 ] and Miao et al. [ 13 ] published systematic reviews on the prevalence and factors influencing DOL in Chinese women in 2021 and 2023, respectively. The results revealed that the prevalence of DOL was 24% [ 12 ] and 31% [ 13 ], respectively, and an increasing trend of DOL, which should attract the attention of clinical workers. In addition, the existing systematic reviews may not be sufficiently comprehensive in literature retrieval and statistical analysis strategies, and their reporting of results may also be inadequate [ 12 , 13 , 14 ], potentially affecting the comprehensiveness and consistency of the findings. Therefore, this study focused on the global perspective and prospective research to determine the incidence of DOL and analyse the factors influencing DOL quantitatively through meta-analysis and to summarize the influencing factors that cannot be quantitatively analysed via qualitative description, to provide evidence supporting the development of effective evaluation and intervention measures for preventing DOL.
This review was conducted according to the Preferred Reporting Items for Systematic Reviews and Meta-Analyses (PRISMA) 2020 statement [ 15 ] and was registered in the International Prospective Register of Systematic Reviews (PROSPERO) (ID: CRD42023458786).
Inclusion and exclusion criteria
Studies were considered eligible if they met all of the following criteria: (1) included women who chose to breastfeed after delivery; (2) studied the incidence or factors influencing DOL; (3) included the occurrence of DOL as the outcome and used the time of obvious breast tenderness or the sensation of milk coming in more than 72 h after delivery as the diagnostic criteria; and (4) used a prospective observational design.
Studies that met one of the following exclusion criteria were excluded: (1) studies with incomplete or erroneous data on variables; (2) studies for which data could not be directly or indirectly extracted; (3) studies for which the original text could not be obtained or the type of article was a review, conference paper, correspondence, comments, or study protocol; (4) studies published in different articles including the same participants: (a) for multiple studies of the same research object, the study with the most abundant research content or the most detailed description of the data was included; (b) for multiple studies with overlapping samples, the study with the longest study period was included; otherwise, the most recent study was included; 5) studies not published in Chinese or English; 6) studies with a sample size < 60; 7) nonhuman studies; or 8) studies for which the literature quality was low (quality assessment score ≤ 5).
Systematic search and strategy
Three researchers (YJP, KZ, and YH) jointly developed the search strategy and comprehensively searched the following databases for all relevant Chinese and English studies from database inception to August 2023: (1) Chinese databases: China National Knowledge Infrastructure (CNKI), Wanfang Database, China Science and Technology Journal Database (Weipu Database), and Chinese Biomedical Database (CBM); (2) English databases: PubMed, Ovid-Embase, Web of Science (via Web of Science Core Collection), Cochrane Library (the Cochrane central register of controlled trials, CENTRAL), CINAHL Plus (via EBSCOhost), APA Psycinfo (via EBSCOhost), Scopus, OpenGrey, and ProQuest Dissertations and Theses database (see full search strategy in Supplementary Material 1). To prevent the omission of relevant research, we reviewed the references of the included studies and relevant reviews. After the search was completed, duplicates were automatically removed by Endnote X9, followed by manual screening.
Study selection process and data extraction
Two researchers (YJP and KZ) independently screened the studies and extracted the data, and a third senior researcher (YH) independently reviewed and discussed the differences. Preliminary screening was performed by reading the title and abstract, followed by rescreening by reading the full text. If the title and abstract were not sufficient to make a decision, a decision was done by reading the full text. After the screening process was completed using Endnote X9, data were extracted from the final included studies, including the title, first author, year, study design, population characteristics, number of cases of DOL, sample size, study period, country, incidence, follow-up method and endpoint. In addition, all the influencing factors mentioned in the original study were extracted, and the influencing factors mentioned in two or more studies with the same definition were then identified. Finally, the exposure and outcome data of these influencing factors were extracted. For exposure variables, the number of cases for categorical variables and the mean ± standard deviation or median [interquartile range] for continuous variables were extracted.
Quality assessment
Prospective observational studies were included, so the Newcastle‒Ottawa Scale (NOS) [ 16 ] was used to evaluate the quality of the included studies. The NOS includes three columns and eight items. The three columns specifically include the selection of the research population, comparability between groups, and the measurement of results or exposure factors, and the total score ranges from 0 to 9 points. Because the data extracted were the number of cases, the influence of confounding factors could not be controlled for, which might lead to the deterioration of ‘comparability between groups’. The diagnostic criteria for DOL were based on maternal self-reported breast distension, which would lead to an insufficient evaluation; therefore, two points were deducted for all included studies. On this basis, studies with a score ≥ 6 points were considered high-quality studies. Two researchers (YJP and KZ) independently evaluated the quality of the included studies according to the evaluation criteria of the NOS [ 17 ]. When the opinions of the two researchers were inconsistent, the study was assigned to a third senior researcher (YH) for independent evaluation and discussion.
Statistical analysis
The extracted data and quality evaluation results were collated into Microsoft Excel 2021, and the data that could be used for quantitative analysis were entered into Stata 16.0 SE software for statistical analysis. In this study, a combined analysis or qualitative description of the influencing factors was performed only for variables included in at least two or more original studies. Because these were prospective observational studies, the risk ratio (RR) was used to combine the effect values for categorical variables, and the weighted mean difference (WMD) was used to combine the effect values for continuous variables. Cochran’s Q test and the I 2 statistic were used to quantitatively analyse the heterogeneity between studies: (1) if I 2 < 50% and p > 0.05, the heterogeneity between studies was considered low, then a fixed effect model was used; (2) if I 2 ≥ 50% or p ≤ 0.05, the heterogeneity between studies was considered high, then a random effect model was used for more conservative statistical analysis. Subgroup analysis was performed to explore the source of heterogeneity.
When a certain influencing factor was included in three or more original studies, sensitivity analysis was carried out by eliminating the studies one by one and merging the remaining studies to test whether the results of the meta-analysis were robust, and we explored the reasons for nonrobust results. If not, the meta-analysis was abandoned, and a qualitative description was carried out instead. When the number of original studies including a certain influencing factor was ≥ 10, a funnel plot was drawn, and Egger’s test was performed to explore whether publication bias existed. If so, the clipping method was used to correct the asymmetry of the funnel plot and the combined effect caused by publication bias. p < 0.05 was considered statistically significant.
Search results and selection
We retrieved 13,112 studies from Chinese and English databases conducted before August 2023, and 9,489 studies were obtained by removing duplicate studies through Endnote and manual methods. After title and abstract screening, 189 studies were included. After rescreening by reading the full text, 32 studies that reported both the incidence and factors influencing DOL and 3 studies that reported only the incidence were ultimately included. No new studies were found after reviewing the references of the included studies and related reviews. The study screening process is shown in Fig. 1 .

PRISMA flowchart for the identification of studies
Characteristics and quality evaluation of the included studies
A total of 35 included studies were conducted from 1999 to 2023 in 8 countries, including China ( n = 23), the United States of America (USA, n = 6), Canada ( n = 1), Peru ( n = 1), India ( n = 1), Australia ( n = 1), Brazil ( n = 1), and Ghana ( n = 1). A total of 19,176 women were included in these studies, 4,922 of whom had DOL. The methods of follow-up involved medical records, questionnaires, or interviews. The mean NOS score of all the studies was ≥ 6 points, indicating that these studies had good methodological quality. The general characteristics and NOS scores of the included studies, sorted alphabetically by author name, are summarized in Table 1 .
Meta-analysis and systematic review results
The combined incidence of DOL was obtained via meta-analysis. The meta-analysis results of factors influencing DOL are summarized in Table 2 according to the order of reporting. Combined with the qualitative description of the influencing factors, all the influencing factors involved could be divided into three categories: maternal-related factors, infant-related factors, and breastfeeding-related factors.
DOL incidence
A random-effects model was used to assess the incidence of DOL in 35 studies, and the result was 30% (95% CI 26, 34) (Fig. 2 ). Subgroup analysis by country category, combined with at least two or more studies, revealed an incidence of DOL of 30% in China (95% CI 26, 35) and 34% in the USA (95% CI 24, 43). The incidence of DOL in the USA was slightly higher than that in China (Fig. 3 ).
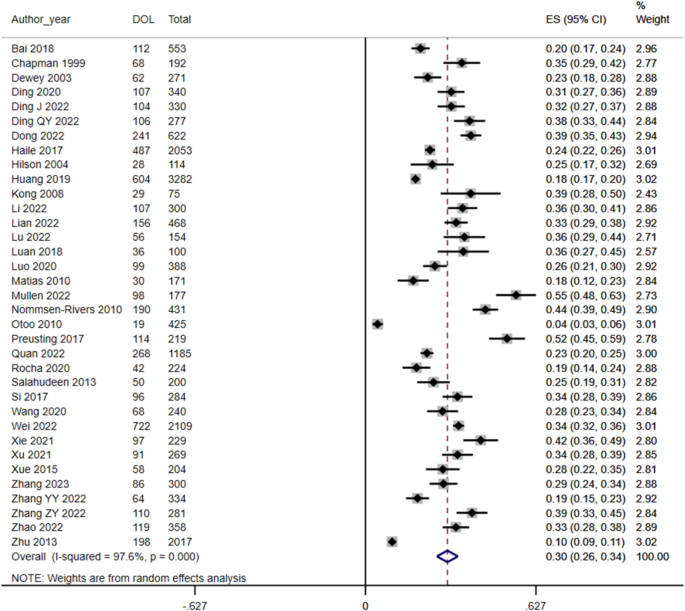
Forest plot of DOL incidence
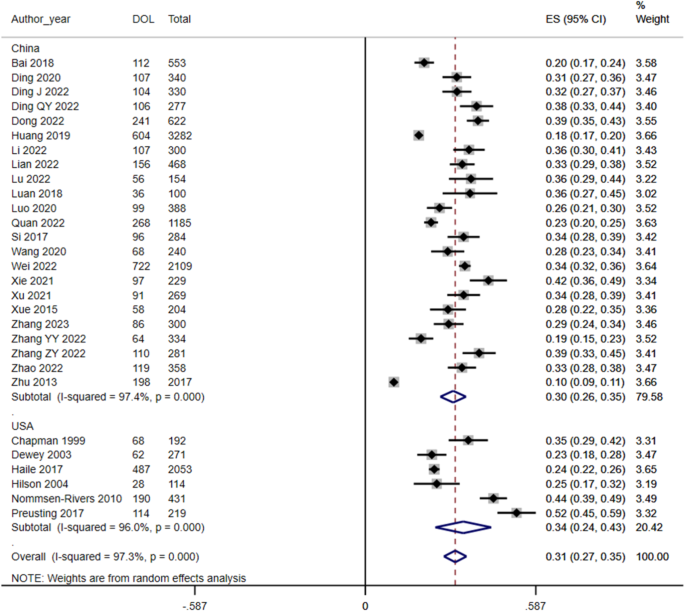
Forest plot of the subgroup analysis of DOL incidence
Maternal-related influencing factors
There was a statistically significant difference in age [ 5 , 26 , 28 , 33 ] between the DOL and non-DOL groups (WMD =-0.30; 95% CI -0.573, -0.40), but the sensitivity analysis result was not robust. The combined results of 9 studies [ 24 , 31 , 36 , 41 , 42 , 43 , 45 , 46 , 48 ] and 5 studies [ 4 , 20 , 30 , 40 , 49 ], respectively, could not determine the association between a maternal age ≥ 35 years (RR = 1.40; 95% CI 0.96, 2.04) and ≥ 30 years (RR = 1.33; 95% CI 0.98, 1.80) and DOL.
The pooled results of 8 studies [ 20 , 21 , 23 , 24 , 37 , 40 , 45 , 48 ] and 3 studies [ 27 , 29 , 46 ], respectively, revealed that a prepregnancy BMI ≥ 25.0 kg/m 2 (RR = 1.47; 95% CI 1.17, 1.84) and a prepregnancy BMI ≥ 24.0 kg/m 2 (RR = 1.41; 95% CI: 1.14, 1.74) were risk factors for DOL. However, the correlation between prepregnancy BMI [ 26 , 30 , 33 ] (WMD = 1.26; 95% CI -1.22, 3.75) and DOL was uncertain. Excessive gestational weight gain (GWG) [ 24 , 37 , 40 , 41 , 45 , 46 , 48 ] (RR = 1.38; 95% CI 1.07, 1.77) was a risk factor for DOL, but the sensitivity analysis result was not robust.
The combined results of 14 [ 20 , 21 , 24 , 26 , 28 , 29 , 30 , 33 , 36 , 37 , 38 , 41 , 45 , 48 ], 13 [ 5 , 20 , 21 , 27 , 28 , 29 , 30 , 36 , 37 , 38 , 41 , 42 , 45 ], 6 [ 21 , 29 , 35 , 38 , 41 , 47 ] and 2 [ 5 , 29 ] studies, respectively, revealed that gestational diabetes mellitus (GDM) (RR = 1.32; 95% CI 1.18, 1.49), hypertensive disorders of pregnancy (HDP) (RR = 1.66; 95% CI 1.30, 2.12), thyroid disease during pregnancy (RR = 1.18; 95% CI 1.05, 1.32), and a serum albumin level < 35 g/L (RR = 1.57; 95% CI 1.12, 2.20) were risk factors for DOL. The descriptive analysis could not determine whether anaemia [ 21 , 29 , 38 ] was associated with DOL, and there might be no association between ovarian cysts during pregnancy [ 21 , 29 ] and DOL.
The pooled results of 23 studies [ 5 , 19 , 20 , 21 , 22 , 23 , 25 , 26 , 27 , 28 , 29 , 31 , 33 , 35 , 36 , 38 , 39 , 40 , 41 , 43 , 44 , 46 , 48 ] revealed that primiparity (RR = 1.40; 95% CI 1.25, 1.56) was a risk factor for DOL. The combined results of 20 [ 4 , 5 , 24 , 26 , 28 , 30 , 31 , 32 , 33 , 35 , 36 , 37 , 38 , 40 , 41 , 44 , 45 , 46 , 48 , 49 ] and 2 [ 21 , 29 ] studies, respectively, revealed that caesarean delivery (RR = 1.33; 95% CI 1.17, 1.52) and unscheduled caesarean delivery (RR = 1.24; 95% CI 1.02, 1.51) were risk factors for DOL. A history of caesarean delivery [ 21 , 29 ] (RR = 0.75; 95% CI 0.60, 0.93) was a protective factor against DOL. The correlations between the duration of labour [ 23 , 45 ] (WMD = 1.97; 95% CI -2.21, 6.16), vaginal delivery, and the duration of the second stage of labour > 1 h [ 4 , 19 ] (RR = 1.41; 95% CI 0.73, 2.72) and DOL were undefined.
Daily sleep duration [ 5 , 28 ] (WMD =-0.24; 95% CI -0.45, -0.02) was a protective factor against DOL. The correlation between an Edinburgh Postnatal Depression Scale (EPDS) score ≥ 9 points [ 35 , 48 ] (RR = 1.24; 95% CI 0.80, 1.93) and DOL was unknown. Moreover, descriptive analysis revealed that the relationship between depression [ 4 , 31 , 45 ] and DOL remained unknown, but there might be a correlation between anxiety [ 22 , 31 , 45 ] and DOL.
The results of the meta-analysis revealed that the relationships between the following variables and DOL could not be determined: education level (≥ high school [ 22 , 32 ], ≥junior college [ 20 , 41 , 44 ], ≥junior undergraduate [ 4 , 21 , 23 , 24 , 37 , 46 , 48 ], and > 9 years [ 29 , 49 ]), occupational status [ 21 , 23 , 29 , 44 , 48 ], mean monthly household income per person (≤ 5000 RMB [ 29 , 31 , 44 ], and ≥ 10000 RMB [ 21 , 26 , 41 ]), nationality (Hispanic [ 4 , 24 , 35 ], White [ 33 , 35 ], and Han Chinese [ 20 , 22 , 26 , 44 , 45 ]), prenatal smoking status [ 4 , 24 , 26 , 35 ], prenatal alcohol consumption status [ 21 , 26 , 27 , 35 , 37 ], assisted reproductive technology (ART) use [ 21 , 41 ], planned pregnancy [ 22 , 43 ], insulin treatment [ 21 , 31 ], and fluid infusion [ 5 , 28 ]. The descriptive results revealed that height [ 4 , 26 ], intraoperative or delivery blood loss [ 4 , 21 , 29 ], and drug-induced labour [ 4 , 35 , 37 ] might not be related to DOL, whereas the relationships between stressful life events [ 22 , 45 , 49 ] during pregnancy, exercise during pregnancy [ 20 , 48 ], and anaesthesia or painkiller use [ 4 , 21 , 24 , 27 , 29 , 35 , 37 ] and DOL remained unclear.
Infant-related influencing factors
The pooled results of 7 [ 29 , 30 , 31 , 40 , 41 , 42 , 49 ] and 2 [ 5 , 28 ] studies, respectively, revealed that a gestational age < 37 weeks (RR = 1.29; 95% CI 1.06, 1.57) and a young gestational age at birth (WMD =-0.47; 95% CI -0.89, -0.06) were risk factors for DOL. Nevertheless, whether gestational age ≥ 39 weeks [ 4 , 32 ] (RR = 1.11; 95% CI 0.86, 1.43) and gestational age (full-term) [ 26 , 45 , 46 ] (WMD =-0.04; 95% CI -0.26, 0.19) were associated with DOL could not be determined.
A birth weight < 2.5 kg [ 29 , 31 ] (RR = 1.34; 95% CI 1.07, 1.67) was a risk factor for DOL. A birth weight ≥ 4 kg [ 22 , 26 , 48 ] (RR = 1.29; 95% CI 1.07, 1.56) was also a risk factor, but the sensitivity analysis result was not robust. However, the correlations between neonatal birth weight [ 30 , 33 , 45 ] (WMD =-0.36; 95% CI -0.86, 0.14) and preterm birth weight [ 5 , 28 ] (WMD =-17.09; 95% CI -102.28, 68.09) and DOL were unclear.
The meta-analysis results could not determine whether neonatal sex [ 4 , 21 , 22 , 26 , 29 , 32 , 46 , 48 , 49 ] and the 1-min Apgar score (< 7 points [ 21 , 28 , 38 ] and < 8 points [ 4 , 32 , 35 ]) were associated with DOL. The descriptive results revealed that the relationships between maternal separation [ 21 , 36 , 43 ] and skin-to-skin contact [ 4 , 35 , 36 , 37 ] and DOL were unknown.
Breastfeeding-related influencing factors
The combined results of 7 studies [ 21 , 22 , 29 , 36 , 37 , 46 , 48 ] revealed that receiving breastfeeding guidance (RR = 0.72; 95% CI 0.64, 0.81) was a protective factor against DOL. The correlation between ≥ 3 breastfeeding information sources [ 22 , 43 ] (RR = 0.50; 95% CI 0.15, 1.65) and DOL was unknown. Descriptive analysis revealed that breast massage or treatment [ 22 , 36 ] might be associated with DOL.
The descriptive analysis results revealed that there might be a relationship between a first breastfeeding session after maternal separation [ 5 , 28 , 42 ] and DOL, but it was not clear whether the first breastfeeding session of general mothers [ 22 , 29 , 32 , 33 , 35 , 37 , 45 ] was related to DOL. The combined results of 5 studies [ 5 , 28 , 42 , 45 , 46 ] revealed that breastfeeding frequency (WMD =-0.63; 95% CI -1.10, -0.16) was a protective factor against DOL. Similarly, a breastfeeding frequency ≤ 2 times on the first day [ 22 , 44 ] (RR = 1.92; 95% CI 1.36, 2.72) and the second day after surgery [ 22 , 44 ] (RR = 1.71; 95% CI 1.34, 2.20) was a risk factor for DOL. The correlation between a breastfeeding frequency < 8 times [ 4 , 32 ] from 0 to 24 h after birth (RR = 1.00; 95% CI 0.78, 1.28) and DOL was unknown.
The meta-analysis results revealed that the relationships between a history of breastfeeding [ 5 , 22 , 28 ], previous insufficient lactation [ 21 , 29 ], prenatal breast enlargement [ 4 , 19 ], flat or sunken nipples [ 4 , 21 , 32 , 35 ], and a bra cup size ≥ D [ 4 , 46 ] and DOL could not be confirmed. The descriptive results revealed that the relationships between formula milk use (within 24 h [ 4 , 32 , 46 ] or 48 h [ 4 , 32 , 35 , 48 ]), LATCH score [ 26 , 35 , 46 ] and nipple pain during lactation [ 4 , 22 , 35 ] and DOL remained unknown.
Sensitivity and publishing bias analysis
The sensitivity analysis results for age (continuous variable), GWG and neonatal birth weight ≥ 4 kg were not robust, whereas the results for the remaining variables were robust. An analysis of the full texts of the studies including these three variables and the sensitivity analysis results revealed that when studies with relatively small samples (< 400 participants) were excluded one by one, the sensitivity analysis results were robust; however, when studies with relatively large samples (> 1,000 participants) were excluded, the sensitivity analysis results became not robust (see Supplementary Material 2).
The results of Egger’s test indicated that there was no publication bias for GDM ( p = 0.129), HDP ( p = 0.136), primipara ( p = 0.125), and caesarean delivery ( p = 0.675) (see Table 2 ). The funnel plots for these four variables also exhibited a basically symmetrical distribution, further suggesting the absence of significant publication bias (see Supplementary Material 3, 4, 5, and 6).
The total incidence of DOL was 30% among the 35 included studies. Subgroup analysis revealed that the incidence of DOL was 30% in China and 34% in the USA. Both China and the USA have made many efforts to support breastfeeding and have introduced policies related to breastfeeding, which focus on the positive role of baby-friendly hospitals and policy support, as well as the ‘Ten Steps to Successful Breastfeeding’ framework, breastfeeding clinics and human milk donation programs [ 51 , 52 ]. Additionally, the American Academy of Pediatrics policy mentions relevant content regarding OL [ 52 ]; however, attention to the important impact of OL on breastfeeding is lacking, and China’s policy does not consider OL [ 51 ], which may be the reason for the high DOL incidence. Moreover, Patel et al.’s systematic review [ 53 ] revealed the effectiveness of dedicated certified lactation consultants or counsellors in promoting breastfeeding, which suggests that they may also have a positive effect on OL support, but this remains to be verified.
The analysis of potential factors influencing DOL revealed statistically significant correlations between DOL and 15 factors: maternal age, prepregnancy BMI (overweight or obesity), GWG, GDM, HDP, thyroid disease during pregnancy, serum albumin levels (< 35 g/L), parity, (unscheduled) caesarean section, caesarean section history, daily sleep duration, gestational age, birth weight (< 2.5 kg or ≥ 4 kg), breastfeeding guidance and daily breastfeeding frequency. However, the sensitivity analysis results for age, GWG and birth weight ≥ 4 kg were not robust. Through descriptive analysis, three factors were found to be likely related to DOL: anxiety, time of first breastfeeding session (maternal separation), and breast massage or treatment.
Combined with the meta-analysis and sensitivity analysis results, although there was a correlation between age and DOL, the result was not robust. A relationship between a maternal age ≥ 35 years or ≥ 30 years and DOL was not found. This suggests that the relationship between maternal age and DOL is still controversial, and more research is needed.
Although the WHO [ 54 ] and China [ 55 ] have slightly different BMI classification criteria, when the prepregnancy BMI reaches the overweight or obese range, the risk for DOL increases. Studies have shown that women who are overweight or obese before pregnancy have a lower response to prolactin stimulated by sucking [ 56 ]. Animal experiments have shown that obesity may impair lactation performance by inducing prolactin resistance [ 57 ]. Obesity is an important risk factor for insulin resistance and impaired insulin secretion; insulin is now thought to play a direct role in lactation, including secretory differentiation, secretory activation and mature milk production [ 58 ]. The results of this study also revealed that a high prepregnancy BMI was a risk factor for DOL after the standard of overweight or obesity was reached. High GWG may increase the risk of DOL. Although the sensitivity analysis results were not robust, considering the adverse effects of overweight or obesity on DOL, these findings still suggest that GWG has a potentially dangerous effect on DOL, which still requires exploration and verification in further research.
Our analysis revealed that GDM, HDP and thyroid disease during pregnancy were risk factors for DOL. De Bortoli et al.’s systematic review [ 59 ] also supports that GDM is a risk factor for DOL. The possible mechanism is that insulin resistance and/or insulin secretion disorders in β cells lead to GDM, of which insulin resistance is the main cause [ 60 ], and insulin resistance affects lactation [ 61 ]. The ratio of insulin to glucose and adiponectin may also be related to the start time of lactation [ 62 ]. Combined with the relationship between obesity and insulin resistance, GDM, obesity and insulin resistance may be associated with DOL in some way [ 63 ]. HDP can affect the initiation and duration of breastfeeding [ 64 ], and the treatment of HDP may also affect lactation; for example, diuretics may reduce milk production [ 65 ]. HDP may also lead to placental dysfunction and decreased prolactin secretion, thereby affecting lactation [ 66 ]. Endothelial dysfunction caused by preeclampsia may lead to hypoalbuminaemia in women [ 67 ], and lower serum albumin levels indicate poor nutritional status, which may be the cause of DOL [ 29 ]. Consistently, this study also revealed that low serum albumin levels were a risk factor for DOL. Animal experiments have shown that hypothyroidism may hinder the ability of the breast to achieve normal milk synthesis and excretion, leading to lactation disorders in pregnant women with thyroid dysfunction [ 68 ]. Similarly, hyperthyroidism can also induce impaired release of oxytocin, resulting in milk deposition, apoptosis of glandular cells that secrete milk, and lactation effects [ 69 ].
This study revealed that primiparity and (unscheduled) caesarean section were risk factors for DOL, whereas a history of caesarean section was a protective factor against DOL. Compared with multiparas, primiparas may experience longer delivery times, resulting in higher cortisol levels [ 70 , 71 ], and multiparas may have more prolactin receptors than primiparas [ 72 ]. Multiparas may also have better breastfeeding skills than primiparas [ 73 ]; hence, primiparas are more likely to experience DOL than multiparas. Similarly, women with a history of caesarean section could have certain breastfeeding experiences; their fear of childbirth in late pregnancy is relatively low [ 74 ], and the pressure of childbirth seems to be related to DOL [ 71 ]. These factors may be why a history of caesarean section is a protective factor against DOL. Compared with vaginal delivery, (unscheduled) caesarean section may lead to lower levels of oxytocin and prolactin secretion [ 75 , 76 ], thus increasing the risk of DOL.
Sleep and emotional state may affect the occurrence of DOL. Prolactin secretion has circadian rhythm changes, and sleep deprivation may lead to decreased levels of prolactin secretion [ 77 ]. Anxiety and depression are associated with lower oxytocin during feeding [ 78 ], and mothers with depression may have insufficient confidence in their ability to breastfeed [ 79 ]. In addition, studies have shown that poor sleep quality is associated with depression and anxiety [ 80 , 81 ]. However, combined with the results of the meta-analysis and descriptive analysis of this study, the relationship between depression and DOL needs further exploration.
This study revealed that young gestational age and low birth weight were risk factors for DOL. The shortening of pregnancy may lead to insufficient prenatal breast preparation, and the immature sucking skills of premature infants can lead to insufficient milk discharge [ 82 ]. A low birth weight may mean that an infant’s motor development is deficient, which may also affect the infant’s sucking skills, subsequently increasing the risk of DOL [ 73 , 83 ]. Colostrum is produced before OL (paracellular pathway closure) [ 84 ]. Unlike mature milk formed after OL, colostrum is rich in immune factors and cytokines, and the concentration of these substances is inversely proportional to the duration of gestation [ 84 ]. Newborns have immature immune systems, especially premature infants whose immune substance transport through the placenta is interrupted prematurely, and colostrum can address this lack of development by providing many bioactive substances [ 85 ]. In addition, preterm birth may trigger delayed closure of the paracellular pathway to prolong the supply of protective substances in colostrum [ 84 ], although this can lead to DOL. These findings also suggest that medical staff should pay attention to the special therapeutic effect of colostrum on premature infants. For example, oropharyngeal colostrum administration, as proposed by Rodriguez et al. [ 84 ] in 2009, has been shown to have a positive effect on the outcomes of preterm infants [ 86 ]. Therefore, in clinical practice, it is necessary to help mothers start breastfeeding early and express colostrum, especially for mothers with premature or low-birth-weight infants, to facilitate the use of colostrum for neonatal immune protection.
The WHO recommends that breastfeeding counselling be provided to all pregnant women and women with babies to help enhance their skills, abilities and confidence in breastfeeding [ 87 ]. McFadden et al.’s systematic review [ 88 ] also revealed that breastfeeding counselling has a positive effect on breastfeeding. Consistent with the results of our study, breastfeeding counselling had a positive protective effect on OL. This study revealed that the frequency of breastfeeding was a protective factor against DOL and should not be less than 2 times/day. Sucking stimulation can trigger the pituitary to release oxytocin, which may be beneficial for uterine involution [ 89 ], and frequent breastfeeding and effective milk emptying have positive effects on milk secretion [ 90 , 91 ]. These findings suggest that in the case of maternal separation, due to the lack of infant sucking, it is necessary to start hand expressing or using a breast pump as soon as possible to mechanically stimulate the areola to promote the release of oxytocin [ 91 ], thereby reducing the risk of DOL; moreover, informing mothers of the potential benefits of frequent sucking on uterine involution is recommended to improve their compliance. However, whether the time of the first breastfeeding session of general parturients is related to DOL still needs further exploration. This study revealed that breast massage or treatment might be a protective factor against DOL, and the protective effect may be achieved by simulating sucking and dredging the mammary duct [ 36 ].
Strengths and limitations
In the original studies included, different researchers might use different criteria for the same influencing factor. Therefore, this study combined quantitative and qualitative analyses to comprehensively summarize the available studies on the incidence and factors influencing DOL. However, this study inevitably has several limitations: (1) Since most of the original studies reported only statistically significant multivariate analysis results and the multivariate analysis methods used were inconsistent, we chose to extract the exposure and outcome data corresponding to the influencing factors after weighing the effects of bias and confounding on the results; regrettably, few studies reported only statistically significant univariate analysis results, but no publication bias was found by funnel plots or Egger’s tests. (2) There were three factors for which the sensitivity analysis results were not robust. A review of the original studies included revealed that some studies had relatively small sample sizes, which might have resulted in insufficient statistical power. When large-sample studies are eliminated, the results might be affected by the combination of small-sample studies; moreover, sensitivity analysis is not applicable to factors that sourced from only two original studies, so robustness cannot be evaluated. Accordingly, larger samples and higher-quality studies are needed to improve the accuracy and robustness of the results. (3) At present, there are no objective and unified diagnostic criteria for DOL, and the most commonly used method is still the subjective perception of maternal breast distension; however, this method may have a large bias. Therefore, it is still necessary to research milk biomarkers to develop an objective and standard evaluation method for use in clinical practice. (4) The selection of the qualitative description method for some influencing factors was due to the high degree of heterogeneity among the studies, so the results of the qualitative description only have implications, and exact conclusions cannot be drawn. (5) As Chinese researchers, considering the accessibility of the Chinese language, we searched Chinese databases, which may have resulted in the inclusion of many Chinese studies. The studies included were mainly from China and the USA, owing to differences in culture and policy, the results concerning the incidence and factors influencing DOL may vary greatly across countries and even within individual countries. Nevertheless, the results may play a role in the implementation of DOL incidence and influencing factor research by researchers from other countries.
This study revealed that the incidence of DOL was 30%, and the factors influencing DOL may include prepregnancy BMI (overweight or obesity), GDM, HDP, thyroid disease during pregnancy, serum albumin levels (< 35 g/L), parity, (unscheduled) caesarean section, caesarean section history, daily sleep duration, gestational age, birth weight (< 2.5 kg), breastfeeding guidance and daily breastfeeding frequency; however, the relationships between age, GWG, birth weight (≥ 4 kg), anxiety, time of first breastfeeding session (maternal separation) and breast massage or treatment and DOL remain unknown. Considering the adverse effects of DOL, policymakers should pay more attention to OL, a critical period of breastfeeding, and formulate corresponding supportive policies. Researchers are advised to explore and verify objective diagnostic criteria for DOL and the influencing factors for which the associations with DOL remain unknown. In addition, establishing breastfeeding support teams in hospitals is recommended, and clinicians should conduct targeted assessments, risk stratification management, health education and interventions for mothers according to the influencing factors to reduce the occurrence of DOL in the case of rational medical resource use.
Data availability
The datasets used and/or analysed during the current study are available from the corresponding author on reasonable request.
Abbreviations
Assisted reproductive technology
Body mass index
- Delayed onset of lactation
Edinburgh Postnatal Depression Scale
Gestational diabetes mellitus
Gestational weight gain
Hypertensive disorders of pregnancy
Maternal intensive care unit
Maternal separation
Newcastle‒Ottawa Scale
Onset of lactation
United States of America
World Health Organization
Weighted mean difference
Medina Poeliniz C, Engstrom JL, Hoban R, Patel AL, Meier P. Measures of secretory activation for research and practice: an integrative review. Breastfeed Med. 2020;15(4):191–212.
Article PubMed Google Scholar
Meier PP, Patel AL, Hoban R, Engstrom JL. Which breast pump for which mother: an evidence-based approach to individualizing breast pump technology. J Perinatol. 2016;36(7):493–9.
Article CAS PubMed PubMed Central Google Scholar
McManaman JL, Neville MC. Mammary physiology and milk secretion. Adv Drug Deliv Rev. 2003;55(5):629–41.
Article CAS PubMed Google Scholar
Nommsen-Rivers LA, Chantry CJ, Peerson JM, Cohen RJ, Dewey KG. Delayed onset of lactogenesis among first-time mothers is related to maternal obesity and factors associated with ineffective breastfeeding. Am J Clin Nutr. 2010;92(3):574–84.
Luan D. [The research on milk volume and influencing factors in mothers with preterm infants hospitalized in NlCU] [Thesis]: Binzhou Medical University; 2018.
Brownell E, Howard CR, Lawrence RA, Dozier AM. Delayed onset lactogenesis II predicts the cessation of any or exclusive breastfeeding. J Pediatr. 2012;161(4):608–14.
Article PubMed PubMed Central Google Scholar
Huang L, Xu S, Chen X, Li Q, Lin L, Zhang Y, et al. Delayed lactogenesis is associated with suboptimal breastfeeding practices: a prospective cohort study. J Nutr. 2020;150(4):894–900.
Chapman DJ, Pérez-Escamilla R. Does delayed perception of the onset of lactation shorten breastfeeding duration? J Hum Lactation. 1999;15(2):107–11.
Article CAS Google Scholar
Hruschka DJ, Sellen DW, Stein AD, Martorell R. Delayed onset of lactation and risk of ending full breast-feeding early in rural Guatemala. J Nutr. 2003;133(8):2592–9.
Michel MP, Gremmo-Féger G, Oger E, Sizun J. [Pilot study of early breastfeeding difficulties of term newborns: incidence and risk factors]. Archives de Pédiatrie. 2007;14(5):454–60.
Dewey KG, Nommsen-Rivers LA, Heinig MJ, Cohen RJ. Risk factors for suboptimal infant breastfeeding behavior, delayed onset of lactation, and excess neonatal weight loss. Pediatrics. 2003;112(3 Pt 1):607–19.
Liu J, Liu M, Jiang P, Chen H, Hu S, Fu J. [Incidence and influencing factors of delayed onset of lactogenesis II in Chinese parturient women: a systematic review]. Chin J Mod Nurs. 2021;27(10):1300–5.
Google Scholar
Miao Y, Zhao S, Liu W, Jiang H, Li Y, Wang A, et al. Prevalence and risk factors of delayed onset lactogenesis II in China: a systematic review and meta-analysis. J Maternal-Fetal Neonatal Med. 2023;36(1):2214833.
Article Google Scholar
Hu S, Liu J, Jiang P, Sun Z, Zhu Q, Fu J. [A systematic review of the incidence and influencing factors of delayed onset of lactogenesis II]. Chin Gen Pract. 2021;24(24):3110–5.
Page MJ, McKenzie JE, Bossuyt PM, Boutron I, Hoffmann TC, Mulrow CD, et al. The PRISMA 2020 statement: an updated guideline for reporting systematic reviews. BMJ. 2021;372:n71.
Stang A. Critical evaluation of the Newcastle-Ottawa scale for the assessment of the quality of nonrandomized studies in meta-analyses. Eur J Epidemiol. 2010;25(9):603–5.
Zeng X, Liu H, Chen X, Leng W. [Meta-analysis series (IV): quality assessment tools for observational studies]. Chin J Evidence-Based Cardiovasc Med. 2012;4(04):297–9.
Bai T, Yang Y, Fu X, Zhou Y, Wei X, Zhang F. [Analysis of the impact of postpartum fatigue on the onset of lactation]. Chin J Nurs. 2018;53(4):438–42.
Chapman DJ, Perez-Escamilla R. Identification of risk factors for delayed onset of lactation. J Am Diet Assoc. 1999;99(4):450–512.
Ding P, Zhao M, Zhang F, Wang L, Yao J, Qiu J, et al. [Nutrients intake in the third trimester and associated factors of delayed onset of lactogenesis II in maternal women]. Chin Gen Pract. 2020;23(5):534–9.
Ding J, Lian W, Ma X. [Construction and validation of risk prediction model for delayed onset of lactogenesis stage II following cesarean section]. Chin J Perinat Med. 2022;25(9):661–9.
Ding Q. [Analysis of factors influencing delayed onset of lactogenesis and construction of a predictive model in advanced cesarean section mothers] [Thesis]: Guangxi University of Chinese Medicine; 2022.
Dong F, Li L, Zhu K, Zhang S, Guan Y, Han J, et al. [Analysis of current status and influencing factors of lactation initiation delay in women with vaginal delivery]. Chin J Practical Nurs. 2022;38(19):1496–502.
Haile ZT, Chavan BB, Teweldeberhan A, Chertok IR. Association between gestational weight gain and delayed onset of lactation: the moderating effects of race/ethnicity. Breastfeed Med. 2017;12:79–85.
Hilson JA, Rasmussen KM, Kjolhede CL. High prepregnant body mass index is associated with poor lactation outcomes among white, rural women independent of psychosocial and demographic correlates. J Hum Lactation. 2004;20(1):18–29.
Huang L, Chen X, Zhang Y, Sun G, Zhong C, Wang W, et al. Gestational weight gain is associated with delayed onset of lactogenesis in the TMCHC study: a prospective cohort study. Clin Nutr. 2019;38(5):2436–41.
Kong M, Bajorek B. Medications in pregnancy: impact on time to lactogenesis after parturition. J Pharm Pract Res. 2008;38(3):205–8.
Li J, Yu X, Wang Y, Liu W, Kong H. [The influencing factors of delayed onset of lactogenesis II in preterm parturient women separated from their infants]. Chin J Nurs Educ. 2022;19(4):368–73.
Lian W, Ding J, Xiong T, Liuding J, Nie L. Determinants of delayed onset of lactogenesis II among women who delivered via cesarean section at a tertiary hospital in China: a prospective cohort study. Int Breastfeed J. 2022;17:81.
Lu Y, Yin Y, Jiang H. [Influence of pregnancy stress and coping style on delayed onset of lactogenesis in maternal separation]. Chin Nurs Res. 2022;36(23):4148–53.
Luo F, Bao N, Li S. [Establishment and validation of a predictive model for the risk of delayed lactation initiation in pregnant women with gestational diabetes mellitus]. J Clin Pathological Res. 2020;40(6):1394–400.
Matias SL, Nommsen-Rivers LA, Creed-Kanashiro H, Dewey KG. Risk factors for early lactation problems among Peruvian primiparous mothers. Matern Child Nutr. 2010;6(2):120–33.
PubMed Google Scholar
Mullen AJ, O’Connor DL, Hanley AJ, Piedimonte G, Wallace M, Ley SH. Associations of metabolic and obstetric risk parameters with timing of lactogenesis II. Nutrients. 2022;14(4):876.
Otoo GE, Marquis GS, Sellen DW, Chapman DJ, Pérez-Escamilla R. HIV-negative status is associated with very early onset of lactation among Ghanaian women. J Hum Lactation. 2010;26(2):107–17.
Preusting I, Brumley J, Odibo L, Spatz DL, Louis JM. Obesity as a predictor of delayed lactogenesis II. J Hum Lactation. 2017;33(4):684–91.
Quan Y, Liu S, Zhou H. [First lactation time and delayed lactation of parturient in Zhumadian area]. South China J Prev Med. 2022;48(9):1037–40.
Rocha BO, Machado MP, Bastos LL, Barbosa Silva L, Santos AP, Santos LC, et al. Risk factors for delayed onset of lactogenesis II among primiparous mothers from a Brazilian baby-friendly hospital. J Hum Lactation. 2020;36(1):146–56.
Salahudeen MS, Koshy AM, Sen S. A study of the factors affecting time to onset of lactogenesis-II after parturition. J Pharm Res. 2013;6(1):68–72.
CAS Google Scholar
Si M. [Correlation between gestational diabetes mellitus (GDM) and delayed onset of lactogenesis (DOL) and risk factors of DOL among GDM puerperae] [Thesis]: Nanjing Medical University; 2017.
Wang S, Guo N, Jiang H. [Correlations of pre-pregnancy body mass index and gestational weight gain with delayed onset of lactogenesis]. Mod Clin Nurs. 2020;19(9):1–6.
Wei Z, Zhao Z, Meng T, Ye Q, Wan Y, Liu Y. [Occurrence and related factors of delayed lactation initiation of parturients in Tongzhou District, Beijing]. South China J Prev Med. 2022;48(7):817–21.
Xie X, Zhao M. [Current status of delayed onset of lactogenesis II of high-risk pregnant women in maternal intensive care unit and its influence factors: a 229-case study]. J Nursing(China). 2021;28(7):49–53.
Xu H. [A study on the correlation between delayed onset of lactogenesis and breastfeeding self-efficacy and analysis of the factors influencing them] [Thesis]: Soochow University; 2021.
Xue Y, Xu Q, Liu L, Liu Z, Huang X, Ma J. [Dietary intake and factors influencing delayed onset of lactation among postpartum women in Guangzhou]. South China J Prev Med. 2015;41(3):218–23.
Zhang L, Li Z, Wang F, Du Q. [Analysis of influencing factors of postpartum delayed onset of lactation in primiparas in family delivery room]. J Med Theory Pract. 2023;36(14):2483–6.
Zhang Y, Zhou H, Wang J, Zhang J, Cai Q. [Effects of pre-pregnancy body mass index, gestational weight gain and early feeding behavior on lactogenesis stage II: a prospective study]. Chin J Perinat Med. 2022;25(7):504–12.
Zhang Z, Liu J, Hu S, Jiang P, Liu M, Zhou Y. [Correlation between pregnancy women with hypothyroidism and delayed lactation initiation]. J Mod Med Health. 2022;38(15):2536–9.
Zhao J. [Effect of physical activity of pregnant women in the third trimester on the delayed onset of lactogenesis and exclusive breastfeeding] [Thesis]: Anhui Medical University; 2022.
Zhu P, Hao J, Jiang X, Huang K, Tao F. New insight into onset of lactation: mediating the negative effect of multiple perinatal biopsychosocial stress on breastfeeding duration. Breastfeed Med. 2013;8(2):151–8.
Institute of Medicine (US) and National Research Council (US) Committee to Reexamine IOM Pregnancy Weight Guidelines. Rasmussen KM, Yaktine AL, editors. Weight gain during pregnancy: reexamining the guidelines. Washington (DC): National Academies Press (US); 2009. Summary. https://www.ncbi.nlm.nih.gov/books/NBK32799/ . Accessed 25 Feb 2024.
[Circular on issuing the breastfeeding promotion action plan(. 2021-2025)]. Gazette of the National Health Commission of the People’s Republic of China. 2021(11):12 – 5.
Meek JY, Noble L. Policy statement: breastfeeding and the use of human milk. Pediatrics. 2022;150(1).
Patel S, Patel S. The effectiveness of lactation consultants and lactation counselors on breastfeeding outcomes. J Hum Lactation. 2016;32(3):530–41.
Obesity: preventing and managing the global epidemic. Report of a WHO consultation. World Health Organization Technical Report Series. 2000;894:i-xii, 1-253.
Zhou B. [Predictive values of body mass index and waist circumference to risk factors of related diseases in Chinese adult population]. Chin J Epidemiol. 2002;23(1):5–10.
Buonfiglio DC, Ramos-Lobo AM, Freitas VM, Zampieri TT, Nagaishi VS, Magalhães M, et al. Obesity impairs lactation performance in mice by inducing prolactin resistance. Sci Rep. 2016;6:22421.
Rasmussen KM, Kjolhede CL. Prepregnant overweight and obesity diminish the prolactin response to suckling in the first week postpartum. Pediatrics. 2004;113(5):e465–71.
Nommsen-Rivers LA. Does insulin explain the relation between maternal obesity and poor lactation outcomes? An overview of the literature. Adv Nutr. 2016;7(2):407–14.
De Bortoli J, Amir LH. Is onset of lactation delayed in women with diabetes in pregnancy? A systematic review. Diabet Med. 2016;33(1):17–24.
Powe CE, Allard C, Battista MC, Doyon M, Bouchard L, Ecker JL, et al. Heterogeneous contribution of insulin sensitivity and secretion defects to gestational diabetes mellitus. Diabetes Care. 2016;39(6):1052–5.
Lemay DG, Ballard OA, Hughes MA, Morrow AL, Horseman ND, Nommsen-Rivers LA. RNA sequencing of the human milk fat layer transcriptome reveals distinct gene expression profiles at three stages of lactation. PLoS ONE. 2013;8(7):e67531.
Nommsen-Rivers LA, Dolan LM, Huang B. Timing of stage II lactogenesis is predicted by antenatal metabolic health in a cohort of primiparas. Breastfeed Med. 2012;7(1):43–9.
Ramanjaneya M, Butler AE, Alkasem M, Bashir M, Jerobin J, Godwin A, et al. Association of complement-related proteins in subjects with and without second trimester gestational diabetes. Front Endocrinol. 2021;12:641361.
Leeners B, Rath W, Kuse S, Neumaier-Wagner P. Breast-feeding in women with hypertensive disorders in pregnancy. J Perinat Med. 2005;33(6):553–60.
Vest AR, Cho LS. Hypertension in pregnancy. Curr Atheroscler Rep. 2014;16(3):395.
Garrido-Gomez T, Quiñonero A, Dominguez F, Rubert L, Perales A, Hajjar KA, et al. Preeclampsia: a defect in decidualization is associated with deficiency of annexin A2. Am J Obstet Gynecol. 2020;222(4):376. e1-e17.
Saitou T, Watanabe K, Kinoshita H, Iwasaki A, Owaki Y, Matsushita H, et al. Hypoalbuminemia is related to endothelial dysfunction resulting from oxidative stress in parturients with preeclampsia. Nagoya J Med Sci. 2021;83(4):741–8.
CAS PubMed PubMed Central Google Scholar
Campo Verde Arboccó F, Sasso CV, Nasif DL, Hapon MB, Jahn GA. Effect of hypothyroidism on the expression of nuclear receptors and their co-regulators in mammary gland during lactation in the rat. Mol Cell Endocrinol. 2015;412:26–35.
Varas SM, Muñoz EM, Hapon MB, Aguilera Merlo CI, Giménez MS, Jahn GA. Hyperthyroidism and production of precocious involution in the mammary glands of lactating rats. Reproduction. 2002;124(5):691–702.
Chen DC, Nommsen-Rivers L, Dewey KG, Lönnerdal B. Stress during labor and delivery and early lactation performance. Am J Clin Nutr. 1998;68(2):335–44.
Dimitraki M, Tsikouras P, Manav B, Gioka T, Koutlaki N, Zervoudis S, et al. Evaluation of the effect of natural and emotional stress of labor on lactation and breast-feeding. Arch Gynecol Obstet. 2016;293(2):317–28.
Zuppa AA, Tornesello A, Papacci P, Tortorolo G, Segni G, Lafuenti G, et al. Relationship between maternal parity, basal prolactin levels and neonatal breast milk intake. Biol Neonate. 1988;53(3):144–7.
Doucet S, Soussignan R, Sagot P, Schaal B. An overlooked aspect of the human breast: areolar glands in relation with breastfeeding pattern, neonatal weight gain, and the dynamics of lactation. Early Hum Dev. 2012;88(2):119–28.
Zhang T, Liu M, Min F, Wei W, Liu Y, Tong J, et al. Fear of childbirth and its determinants in pregnant women in the third trimester: a cross-sectional study. BMC Psychiatry. 2023;23:574.
Nissen E, Uvnäs-Moberg K, Svensson K, Stock S, Widström AM, Winberg J. Different patterns of oxytocin, prolactin but not cortisol release during breastfeeding in women delivered by caesarean section or by the vaginal route. Early Hum Dev. 1996;45(1–2):103–18.
Wang Y, Tao F, Zhu P, Jiang X, Yao Y, Xu Y. [The level of serum prolactin, self-estimated milk yield and growth factor level in colostrum of pregnant women adopting different delivery modes]. Maternal Child Health Care China. 2010;25(10):1411–4.
Fu M, Zhang L, Ahmed A, Plaut K, Haas DM, Szucs K, et al. Does circadian disruption play a role in the metabolic-hormonal link to delayed lactogenesis II? Front Nutr. 2015;2:4.
Stuebe AM, Grewen K, Meltzer-Brody S. Association between maternal mood and oxytocin response to breastfeeding. J Women’s Health. 2013;22(4):352–61.
Zubaran C, Foresti K. The correlation between breastfeeding self-efficacy and maternal postpartum depression in southern Brazil. Sex Reproductive Healthc. 2013;4(1):9–15.
Khadka R, Hong SA, Chang YS. Prevalence and determinants of poor sleep quality and depression among postpartum women: a community-based study in Ramechhap district, Nepal. Int Health. 2020;12(2):125–31.
Okun ML, Mancuso RA, Hobel CJ, Schetter CD, Coussons-Read M. Poor sleep quality increases symptoms of depression and anxiety in postpartum women. J Behav Med. 2018;41(5):703–10.
Krebs NF, Belfort MB, Meier PP, Mennella JA, O’Connor DL, Taylor SN, et al. Infant factors that impact the ecology of human milk secretion and composition-a report from Breastmilk Ecology: Genesis of Infant Nutrition (BEGIN) Working Group 3. Am J Clin Nutr. 2023;117:S43–60.
Canals J, Fernández-Ballart J, Esparó G. Evolution of neonatal behavior assessment scale scores in the first month of life. Infant Behav Dev. 2003;26(2):227–37.
Rodriguez NA, Meier PP, Groer MW, Zeller JM. Oropharyngeal administration of colostrum to extremely low birth weight infants: theoretical perspectives. J Perinatol. 2009;29(1):1–7.
Garofoli F, Civardi E, Pisoni C, Angelini M, Ghirardello S. Anti-inflammatory and anti-allergic properties of colostrum from mothers of full-term and preterm babies: the importance of maternal lactation in the first days. Nutrients. 2023;15(19):4249.
Fu ZY, Huang C, Lei L, Chen LC, Wei LJ, Zhou J, et al. The effect of oropharyngeal colostrum administration on the clinical outcomes of premature infants: a meta-analysis. Int J Nurs Stud. 2023;144:104527.
Guideline: counselling of women to improve breastfeeding practices. Geneva: World Health Organization. 2018. Evidence and Recommendations. https://www.ncbi.nlm.nih.gov/books/NBK539310/ . Accessed 25 Feb 2024.
McFadden A, Siebelt L, Marshall JL, Gavine A, Girard LC, Symon A, et al. Counselling interventions to enable women to initiate and continue breastfeeding: a systematic review and meta-analysis. Int Breastfeed J. 2019;14:42.
Neville MC. Anatomy and physiology of lactation. Pediatr Clin North Am. 2001;48(1):13–34.
Weaver SR, Hernandez LL. Autocrine-paracrine regulation of the mammary gland. J Dairy Sci. 2016;99(1):842–53.
Truchet S, Honvo-Houéto E. Physiology of milk secretion. Best Pract Res Clin Endocrinol Metab. 2017;31(4):367–84.
Download references
Acknowledgements
Not applicable.
Author information
Yijuan Peng and Ke Zhuang contributed equally to this study and should be considered co-first authors.
Authors and Affiliations
Department of Nursing, West China Second University Hospital, Sichuan University/West China School of Nursing, Sichuan University, No. 20 Third Section, Renmin South Road, Chengdu, Sichuan Province, 610041, China
Yijuan Peng, Ke Zhuang & Yan Huang
Key Laboratory of Birth Defects and Related Diseases of Women and Children (Sichuan University), Ministry of Education, Chengdu, Sichuan, China
You can also search for this author in PubMed Google Scholar
Contributions
YJP, KZ, and YH conceptualized and designed this review. YJP, KZ, and YH conducted the literature search, literature screening, data extraction, quality evaluation, and statistical analysis. YJP and KZ wrote the manuscript. YH reviewed and modified the manuscript. All the authors read and approved the final manuscript.
Corresponding author
Correspondence to Yan Huang .
Ethics declarations
Ethics approval and consent to participate, consent for publication, competing interests.
The authors declare no competing interests.
Additional information
Publisher’s note.
Springer Nature remains neutral with regard to jurisdictional claims in published maps and institutional affiliations.
Electronic supplementary material
Below is the link to the electronic supplementary material.
Supplementary Material 1
Supplementary material 2, supplementary material 3, supplementary material 4, supplementary material 5, supplementary material 6, rights and permissions.
Open Access This article is licensed under a Creative Commons Attribution-NonCommercial-NoDerivatives 4.0 International License, which permits any non-commercial use, sharing, distribution and reproduction in any medium or format, as long as you give appropriate credit to the original author(s) and the source, provide a link to the Creative Commons licence, and indicate if you modified the licensed material. You do not have permission under this licence to share adapted material derived from this article or parts of it. The images or other third party material in this article are included in the article’s Creative Commons licence, unless indicated otherwise in a credit line to the material. If material is not included in the article’s Creative Commons licence and your intended use is not permitted by statutory regulation or exceeds the permitted use, you will need to obtain permission directly from the copyright holder. To view a copy of this licence, visit http://creativecommons.org/licenses/by-nc-nd/4.0/ .
Reprints and permissions
About this article
Cite this article.
Peng, Y., Zhuang, K. & Huang, Y. Incidence and factors influencing delayed onset of lactation: a systematic review and meta-analysis. Int Breastfeed J 19 , 59 (2024). https://doi.org/10.1186/s13006-024-00666-5
Download citation
Received : 28 February 2024
Accepted : 16 August 2024
Published : 22 August 2024
DOI : https://doi.org/10.1186/s13006-024-00666-5
Share this article
Anyone you share the following link with will be able to read this content:
Sorry, a shareable link is not currently available for this article.
Provided by the Springer Nature SharedIt content-sharing initiative
- Influencing factor
- Meta-analysis
- Systematic review
International Breastfeeding Journal
ISSN: 1746-4358
- General enquiries: [email protected]
- Open access
- Published: 26 August 2024
The association between ibuprofen administration in children and the risk of developing or exacerbating asthma: a systematic review and meta-analysis
- Luke Baxter 1 ,
- Maria M. Cobo 1 , 2 ,
- Aomesh Bhatt 1 ,
- Rebeccah Slater 1 ,
- Olutoba Sanni 3 &
- Nutan Shinde 4
BMC Pulmonary Medicine volume 24 , Article number: 412 ( 2024 ) Cite this article
26 Accesses
Metrics details
Ibuprofen is one of the most commonly used analgesic and antipyretic drugs in children. However, its potential causal role in childhood asthma pathogenesis remains uncertain. In this systematic review, we assessed the association between ibuprofen administration in children and the risk of developing or exacerbating asthma.
We searched MEDLINE, Embase, Cochrane Library, CINAHL, Web of Science, and Scopus from inception to May 2022, with no language limits; searched relevant reviews; and performed citation searching. We included studies of any design that were primary empirical peer-reviewed publications, where ibuprofen use in children 0–18 years was reported. Screening was performed in duplicate by blinded review. In total, 24 studies met our criteria. Data were extracted according to PRISMA guidelines, and the risk of bias was assessed using RoB2 and NOS tools. Quantitative data were pooled using fixed effect models, and qualitative data were pooled using narrative synthesis. Primary outcomes were asthma or asthma-like symptoms. The results were grouped according to population (general, asthmatic, and ibuprofen-hypersensitive), comparator type (active and non-active) and follow-up duration (short- and long-term).
Comparing ibuprofen with active comparators, there was no evidence of a higher risk associated with ibuprofen over both the short and long term in either the general or asthmatic population. Comparing ibuprofen use with no active alternative over a short-term follow-up, ibuprofen may provide protection against asthma-like symptoms in the general population when used to ease symptoms of fever or bronchiolitis. In contrast, it may cause asthma exacerbation for those with pre-existing asthma. However, in both populations, there were no clear long-term follow-up effects.
Conclusions
Ibuprofen use in children had no elevated risk relative to active comparators. However, use in children with asthma may lead to asthma exacerbation. The results are driven by a very small number of influential studies, and research in several key clinical contexts is limited to single studies. Both clinical trials and observational studies are needed to understand the potential role of ibuprofen in childhood asthma pathogenesis.
Peer Review reports
Asthma is a noncommunicable disease affecting approximately 235 million people worldwide and is characterised by inflammation and narrowing of the small airways in the lungs, leading to any combination of cough, wheeze, shortness of breath, and chest tightness [ 1 ]. The prevalence of asthma has increased in many countries in recent decades, especially among children, making asthma a serious global public health problem [ 2 , 3 ]. The reason for increasing asthma prevalence in children is uncertain, but there is likely a complex interaction of multiple risk factors, including environmental (e.g., increased air pollution, changes to housing conditions) and lifestyle factors (e.g., decreased physical activity, changes in diet, increased childhood obesity) [ 4 ].
Increased early-life use of pharmacological agents, such as analgesics and antipyretics, could be causal factors in childhood asthma pathogenesis. Due to fears of a causal relationship between aspirin use and Reye’s syndrome [ 5 ] and the risk of aspirin-induced asthma [ 6 ], aspirin use in children has dramatically decreased in recent decades. Consequently, drugs such as ibuprofen and paracetamol have become increasingly popular for treating fever and pain in children. In the United Kingdom, the National Health Service describes both paracetamol and ibuprofen as safe for treating pain and high temperature in babies and children [ 7 ]. However, caution is advised for ibuprofen use in children with asthma [ 8 ], while no such warning is supplied for paracetamol [ 9 ], suggesting that ibuprofen may be linked to asthma development or exacerbation in those with pre-existing asthma.
Ibuprofen is a non-steroidal anti-inflammatory drug (NSAID) that is frequently prescribed or administered over-the-counter (OTC) to treat fever and pain. Links between childhood ibuprofen use and asthma development or exacerbation are being investigated [ 10 , 11 , 12 , 13 , 14 , 15 , 16 ]. Ibuprofen’s inhibition of the cyclooxygenase system can lead to activation of the lipoxygenase system, resulting in bronchospasm [ 6 , 17 ], which could precipitate asthma. Additionally, empirical evidence exists demonstrating ibuprofen-induced asthma exacerbation in children with asthma and self-reported aspirin allergy [ 18 ].
Despite these points, two recent systematic reviews did not identify a risk difference between ibuprofen and paracetamol in asthma development or exacerbation in children [ 14 , 16 ]. However, one of these reviews limited the scope to randomised controlled trials (RCTs) [ 14 ], and the other to a relatively narrow age range of less than 2 years [ 16 ], restricting the generalisability of the findings.
We conducted a systematic review to assess the association between ibuprofen administration in children and the risk of developing or exacerbating asthma. The aim was to expand on previous reviews by looking across the entire age range of childhood from 0 to 18 years, including both interventional and observational studies, and assessing the association separately for clinically distinct paediatric subpopulations: general, asthmatic, and ibuprofen-hypersensitive.
Protocol development
We registered our review on PROSPERO on 8 July 2022 (CRD42022344838). The protocol was written according to PRISMA-P guidelines [ 19 , 20 ] and made publicly available on OSF prior to registration with PROSPERO. Further methodological details can be found in our online protocol ( https://doi.org/10.17605/OSF.IO/Z37KW ).
Eligibility criteria
A full list of eligibility criteria is provided in Supplementary Methods S1.1 (Supplementary Tables 1–2). The numeric results from studies included in our review were grouped by population for synthesis: (i) general population of children (i.e., studies not limiting eligibility to specific clinical subpopulations; however, some study-specific exclusion will always occur, for example, children with severe asthma, ibuprofen hypersensitivity, or other contraindications for safety reasons; children with conditions that could interfere with ibuprofen administration or absorption, such as inability to swallow or frequent vomiting; children receiving treatments that could interfere with the outcome assessment, such as leukotriene receptor antagonist and other anti-asthmatic treatments); (ii) children with asthma; and (iii) children with ibuprofen hypersensitivity.
Search strategy
We searched six bibliographic databases (MEDLINE, Embase, Cochrane Library, CINAHL, Web Of Science, Scopus) to identify records on 21-May-2022, and our searches were independently peer-reviewed using the PRESS Checklist [ 21 , 22 ] by an outreach librarian at the Bodleian Health Care Libraries, University of Oxford ( https://doi.org/10.17605/OSF.IO/R3AV6 ). All search strategies are provided in full in Supplementary Methods S1.2. Additional information sources included relevant reviews that were identified during screening [ 10 , 11 , 12 , 13 , 14 , 15 , 16 ] and backwards citation searching using the citationchaser tool [ 23 ]. EPPI-Reviewer [ 24 ] was used for de-duplication, and screening was performed independently in duplicate, with disagreements settled by discussion between both reviewers.
Data extraction and bias assessment
Data extraction and bias assessment were performed by one reviewer and then verified by a second reviewer, with disagreements settled by discussion. Our primary outcomes of interest were asthma, asthma-like symptoms, or asthma exacerbation [ 2 ]. For risk of bias assessment, the Cochrane risk of bias tool (RoB2) was used for RCTs [ 25 ], and the Newcastle-Ottawa Scale (NOS) [ 26 ] was used for observational studies. The results from these assessments were used to decide which studies to include in primary syntheses (Supplementary Figs. 1–2). Our approach to assessing meta-biases (outcome reporting and publication biases) is detailed in Supplementary Methods S1.3.
Data synthesis
A narrative synthesis was performed when outcomes were too heterogeneous to synthesise quantitatively. Otherwise, meta-analysis was performed using the R package meta [ 27 ]. Given the sparsity of the data for quantitative synthesis, we report the common effect model results as primary results. For completion, we report additional analysis outputs, e.g., both odds and risk ratios; both common and random effects model effect sizes; I 2 , tau 2 , and chi 2 for heterogeneity. Due to the sparsity of the results, subgroup analyses were not performed.
For meta-analysis of dichotomous data, ORs were pooled using Peto’s method [ 28 ] due to zero events in some arms. Where multiple outcomes from a study were available, the primary analysis was performed by selecting the outcomes with the expected lowest risk of bias. To test the robustness of the primary analysis, sensitivity analyses were performed using alternative combinations of studies’ numeric results.
Study selection characteristics
Of the 820 records screened, 18 relevant studies were identified, with a further 6 from relevant reviews (Supplementary Fig. 3). The study characteristics for all 24 studies are summarised in Table 1 . Relevant numeric results were grouped by population: (i) general population of children (Table 2 ), (ii) children with asthma (Table 3 ), and (iii) children with ibuprofen hypersensitivity (Table 4 ). For the general population and children with asthma, data synthesis was performed for (i) ibuprofen versus an active comparator (Fig. 1 ) and (ii) ibuprofen versus baseline (i.e., children not taking an alternative antipyretic or analgesic). To increase homogeneity, the results were also grouped based on the duration of follow-up, in line with a recent similar systematic review [ 16 ]: short duration of ≤ 28 days or long duration of > 28 days.
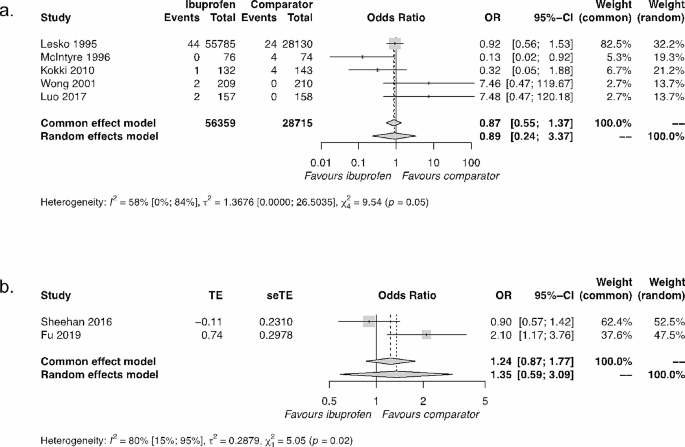
Synthesis of results of ibuprofen versus active comparators. The active comparator for Kokki 2010 was ketoprofen; for all other studies, the active comparator was paracetamol. ( a ) General population of children over a short duration. ( b ) Children with asthma over a long duration. Abbreviations: OR = odds ratio; 95% CI = 95% confidence interval
General population
In total, 13 numeric results from 9 studies relevant to assessing ibuprofen use in a general population of children were identified [ 29 , 30 , 31 , 32 , 33 , 34 , 35 , 36 , 37 ] (Table 2 ).
Ibuprofen versus active comparator
There were six results from six interventional studies (all RCTs) and two results from one observational cohort study that compared ibuprofen use with an active comparator in the general population. The main active comparator was paracetamol, with one study [ 29 ] using ketoprofen (Table 2 ). The durations of study for the interventional RCT were all short (≤ 28 days). Two of these results were from publications based on the same dataset, the Boston University Fever Study [ 30 , 31 ], of which the original publication was selected for primary analysis.
The synthesis of five results comparing ibuprofen with active comparators (four paracetamol, one ketoprofen) resulted in a common effect OR = 0.87; 95% CI=[0.55, 1.37], demonstrating a lack of significant difference between ibuprofen and active comparators (Fig. 1 a). Our sensitivity analyses were in agreement with this primary result (Supplementary Fig. 4).
A single observational study [ 36 ] assessed ibuprofen relative to paracetamol over both short and long durations (Table 2 ) in a general population of children. Over a short duration (14 days), no significant difference in wheezing was identified, but over a long duration (1 year), they observed a significant advantage to ibuprofen over paracetamol, with a reduction in health care practitioner visits for wheezing illness consistent with bronchiolitis or asthma.
Taken together, these interventional and observational results suggest that there is no difference between ibuprofen and active comparators in the general population over a short duration (≤ 28 days). This finding is driven largely by a single study, the Boston University Fever Study [ 31 ], conducted almost 30 years ago on a large sample ( n = 83,915) of children aged 6 months to 12 years. Over longer follow-up durations of one year, there is evidence from only a single cohort study [ 36 ] to suggest that there may be a reduction in wheezing when ibuprofen is prescribed, rather than paracetamol, for a first episode of bronchiolitis in children aged 0–12 months.
Ibuprofen versus baseline
Five numeric results from three studies relevant to assessing ibuprofen relative to baseline (children not taking an alternative antipyretic or analgesic) in the general population were identified (Table 2 ). All outcomes were from observational studies. Due to the sparsity and substantive heterogeneity of the results, quantitative synthesis was not possible.
Two studies looked at general populations over short durations (≤ 28 days) [ 33 , 36 ]. Both studies suggest that ibuprofen might decrease wheezing when taken for either acute febrile illness or bronchiolitis (Table 2 ).
Two studies looked at general populations of children over long durations [ 35 , 36 ] and produced conflicting results. One study [ 36 ] compared those prescribed ibuprofen for a first episode of bronchiolitis to those not prescribed ibuprofen (or another drug) and followed up participants over a 1-year duration, observing a positive impact of ibuprofen prescription. The second study [ 35 ] compared children administered ibuprofen to those not administered ibuprofen during the first postnatal year and followed-up participants at a 3–5 year duration, observing a negative impact of ibuprofen on asthma development, and at a 7–10 year duration, observing no difference between cohorts (Table 2 ).
Taken together, ibuprofen use in the general population of children during acute febrile illness or bronchiolitis might decrease wheezing when assessed in the short-term (≤ 28 days), with both observational studies reporting strong significant effects (Table 2 ). Over longer durations, the two observational studies identified in this review have substantive heterogeneity in design, analysis, and outcome, preventing meaningful synthesis. Additionally, their numeric findings are inconsistent (Table 2 ).
Asthmatic population
Five numeric results from four studies relevant to assessing ibuprofen in asthmatic paediatric populations were identified [ 38 , 39 , 40 , 41 ] (Table 3 ).
Three results across three studies compared ibuprofen with an active comparator (paracetamol in all cases) in asthmatic populations (Table 3 ). One interventional study assessed outcomes over a short duration [ 39 ] and found no difference between treatments. While further analyses in this paper did suggest a favourable outcome for ibuprofen relative to paracetamol, the results from this second post-hoc Boston Fever Study report are at very high risk of bias in the selection of the reported result (Supplementary Fig. 2).
Two studies looked at the comparison between ibuprofen and paracetamol in asthmatic populations over long durations [ 38 , 41 ]. The RCT study [ 41 ] identified no difference between drugs (OR = 0.90 [ 0.57, 1.41]). In contrast, the observational cohort study [ 38 ] identified a significant disadvantage for ibuprofen relative to paracetamol in asthmatic populations (aOR = 2.10 [1.17, 3.76]). These conflicting results for ibuprofen relative to paracetamol in asthmatic populations over long durations are challenging to resolve due to the different experimental designs. However, there are also several similarities in their designs: use of the same active comparator, inclusion of asthmatic populations of children with similar age ranges (Sheehan: 1–4.9 years; Fu: 1–5 years) over similar follow-up durations (Sheehan: 46 weeks; Fu: 52 weeks), and use of asthma exacerbation as the outcome. As an exploratory analysis, we synthesised these results, which resulted in a common effect OR = 1.24; 95% CI=[0.87, 1.77], suggesting an overall non-significant effect, which is consistent with the RCT study result alone (Fig. 1 b).
Taken together, these interventional and observational results suggest that there is no difference in asthma exacerbation between ibuprofen and paracetamol in asthmatic populations over short or long durations.
Only a single study looked at an asthmatic population over both short and long durations [ 40 ]. Over a short duration, this study found that ibuprofen increased asthma exacerbation. Over a long duration, they found no effect of ibuprofen on asthma exacerbation in the asthmatic population.
Ibuprofen hypersensitive population
Four drug provocation studies were identified that studied ibuprofen-hypersensitive children where ibuprofen was ingested and adverse events reported as part of hypersensitivity diagnosis [ 42 , 43 , 44 , 45 ]. A range of respiratory adverse effects were reported that included asthma, coughing, wheezing, dyspnoea, and respiratory distress (Table 4 ). Across the four studies, there was a total of 10 children with respiratory adverse events reported in a total of 80 children. Thus, in children with ibuprofen hypersensitivity, the average rate of respiratory adverse events following ibuprofen ingestion was 12.5%.
Unsynthesised papers
Seven studies were identified that reported the relationship between ibuprofen and asthma in children, which were not synthesised in this review [ 18 , 46 , 47 , 48 , 49 , 50 , 51 ]: five studies reported on single cases, and two group analysis studies had substantive differences in methodology and outcomes relative to other studies included in this review.
One crossover RCT [ 46 ] assessed the prevalence of ibuprofen-sensitive asthma in children with mild or moderate persistent asthma using bronchoprovocation challenge and found a prevalence of 2%. Another non-randomised controlled study [ 18 ] assessed the impact of short-term ibuprofen treatment on pulmonary function in children with mild to moderate stable asthma and self-reported aspirin allergy. Relative to a healthy control group, the asthmatic group exhibited a drop in FEV1 (forced expiratory volume in the first second) of 18.85% and an increase in FeNO (fractional exhaled nitric oxide) of 20.76 ppb. A summary of the results from these two studies is provided in Supplementary Table 3.
Four case reports of severe adverse events to ibuprofen were identified [ 47 , 48 , 50 , 51 ], and in all cases, the children had pre-existing asthma. Last, in a case series of fatal asthma in Finland, a single death due to ibuprofen ingestion was reported in a child with severe asthma and a known allergy to ibuprofen [ 49 ].
Here, we assessed the association between ibuprofen use and asthma in children aged 0–18 years. Both observational and interventional studies were reviewed in the general population as well as the asthmatic population. Studies that benchmarked ibuprofen against an active comparator almost exclusively used paracetamol, and in both populations of children, the combined evidence suggested no difference in asthma-related adverse events between ibuprofen and paracetamol (or ketoprofen) use. A single observational study suggested a potential benefit of ibuprofen over paracetamol prescription in response to bronchiolitis in the general paediatric population after a one-year follow-up. When ibuprofen use was assessed relative to no alternative drug administration, differences emerged between the general and asthmatic populations. In the short-term follow-up (1–14 days) to ibuprofen use, two observational studies reported favourable effects in the general population, while one observational and one interventional study observed unfavourable effects in the asthmatic population. Over a longer follow-up period (12 weeks to 10 years), no clear effect emerged for either population.
The majority of research on the association between ibuprofen use and asthma-related adverse events in children has been conducted in the general population, benchmarked relative to paracetamol, and participants followed-up over a short duration [ 29 , 30 , 31 , 32 , 34 , 36 , 37 ]. The aggregate result from five RCTs conducted in this context is driven primarily by the Boston University Fever Study [ 31 ], conducted almost 30 years ago on children aged 6 months to 12 years. While a single observational study [ 36 ] conducted five years ago corroborates this finding, research is sparse. Furthermore, only a single study comparing ibuprofen with paracetamol use with a short-term follow-up was conducted in children with asthma [ 39 ], and this study was a second post-hoc analysis publication of the same Boston University Fever Study dataset. Given the increased vulnerability of the asthmatic population to respiratory adverse events from ibuprofen use that was observed in our review, there is a clear lack of research comparing the short-term effects of ibuprofen relative to alternative analgesics and antipyretics such as paracetamol in children with asthma.
Two studies [ 38 , 41 ] assessing differences between ibuprofen and paracetamol use over longer follow-up periods in asthmatic populations report conflicting results. Due to several study similarities, we tentatively synthesised the two results, and no aggregate difference between ibuprofen and paracetamol was observed. However, in the RCT [ 41 ], the median dose of trial medication (ibuprofen or paracetamol) was 5.5 doses (IQR = 1–15) and matched between trial arms. In the retrospective cohort study [ 38 ], it could not be determined by the original investigators whether patients took the medication prescribed. Additionally, the observational study did not control for upper respiratory tract infections, a well-documented source of confounding by indication [ 35 , 52 ], which were not well-matched between the ibuprofen and paracetamol cohorts. For these reasons, the RCT finding alone or the synthesised outcome of no difference between drugs seems most justifiable.
Comparing the asthmatic and general populations for short-term asthma-relevant outcomes after ibuprofen use, no conflicts in results were observed. The two observational studies in the general population [ 33 , 36 ] both observed reductions in asthma-related outcomes, while one observational [ 40 ] and one interventional [ 18 ] study in the asthmatic population both observed increases in asthma-related outcomes. These findings highlight the importance of avoiding naïve pooling of results from studies in these different paediatric populations.
It is noteworthy that all RCTs reviewed compared ibuprofen with an active comparator. Of the studies comparing ibuprofen with a baseline of no alternative drug, three were cohort studies [ 35 , 36 , 40 ], and one was cross-sectional [ 33 ]. One non-randomised interventional study [ 18 ] compared an asthmatic sample with a healthy control sample. This highlights one of the limitations of the RCT design approach in assessing adverse events in the youngest children [ 53 , 54 ]. As a recent RCT feasibility study found [ 55 ], almost three quarters of parents surveyed described the use of a placebo comparator treatment as unacceptable for treating their child’s fever or pain. This ethical unacceptability of using a placebo arm in clinical trials for treating pain and fever in young children [ 55 , 56 ] introduces an ambiguity into these active comparator RCT studies, as a lack of difference among active comparators does not exclude the possibility that both ibuprofen and active comparator use may be associated with parallel increases in asthma exacerbations [ 41 , 56 ]. It has been argued that, given that ibuprofen and paracetamol have different mechanisms of action, it is unlikely that their use could be associated with similar increases in the rate of asthma-related complications that are known to be determined by disparate mechanisms of disease [ 41 , 56 ]. However, this speculation requires careful examination and empirical support. Observational studies with comparator groups in which an active treatment was not prescribed or taken can be used as a baseline control to assess the impact of ibuprofen alone, acknowledging the challenges of inferring causality in observational studies. It is these advantages and disadvantages of both RCTs and observational designs that require a review of the association between ibuprofen use and asthma-related outcomes in children to consider and attempt to synthesise all study design types. This feature of our review adds substantially to two recent systematic reviews in this area [ 14 , 56 ] that either limited the study designs to RCTs [ 14 ] or limited the population to those under 2 years [ 56 ].
We identified four drug provocation trials in which ibuprofen hypersensitivity was confirmed in children by controlled administration of ibuprofen [ 42 , 43 , 44 , 45 ] and respiratory adverse events were recorded. The average percentage of children with confirmed ibuprofen hypersensitivity who displayed respiratory adverse events was 12.5%. Relative to other adverse events, such as angio-oedema and urticaria (which were by far the most common adverse events), asthma and asthma-like respiratory events were less commonly reported. While adverse respiratory reactions to ibuprofen ingestion in those with ibuprofen hypersensitivity can be quite severe, as reported in a handful of case reports [ 47 , 48 , 50 , 51 ], fatalities appear to be very rare. In this review, only a single case of ibuprofen-induced asthma fatality was identified [ 49 ].
The number of studies in this review that were relevant to important clinical populations and contexts was unfortunately sparse. Only a single publication was identified for each of the following three contexts: the general population where ibuprofen is compared with an active comparator with a follow-up duration longer than 1 month [ 36 ]; the asthmatic population where ibuprofen is compared with an active comparator with a short-term follow-up [ 39 ]; and the asthmatic population where ibuprofen is compared with a baseline of no active comparator with a follow-up duration longer than 1 month [ 40 ]. These limitations hinder the generalisability of findings to several important clinical contexts and are an ongoing issue to be addressed.
Here, we found that research is most lacking for populations of children with pre-existing asthma, who are the population at most risk for potential respiratory adverse events following ibuprofen use. Our review highlights the importance of assessing both interventional and observational studies and analysing the general population and asthmatic population separately. Continued investigation into the role of early-life ibuprofen use and its short-term and long-term impact on childhood asthma is needed.
Data availability
All data (data collection form, risk of bias assessment forms, and data used for all analyses) are publicly available on the project’s OSF site: https://doi.org/10.17605/OSF.IO/ZBDS7 . All code used for the meta-analysis is publicly available on Zenodo: https://doi.org/10.5281/zenodo.11258287 .
WHO. Asthma [Internet]. 2023 [cited 2023 Apr 4]. https://www.who.int/news-room/fact-sheets/detail/asthma
Reddel HK, Bacharier LB, Bateman ED, Brightling CE, Brusselle GG, Buhl R et al. Global Initiative for Asthma Strategy 2021: executive summary and rationale for key changes. European Respiratory Journal [Internet]. 2022 Jan 1 [cited 2022 Apr 27];59(1). https://erj.ersjournals.com/content/59/1/2102730
Vos T, Murray CJL, GBD 2016 Disease and Injury Incidence and Prevalence Collaborators. Global, regional, and national incidence, prevalence, and years lived with disability for 328 diseases and injuries for 195 countries, 1990–2016: a systematic analysis for the global burden of Disease Study 2016. Lancet. 2017;390(10100):1211–59.
Article Google Scholar
Beasley R, Semprini A, Mitchell EA. Risk factors for asthma: is prevention possible? Lancet. 2015;386(9998):1075–85.
Article PubMed Google Scholar
Schrör K. Aspirin and Reye syndrome: a review of the evidence. Paediatr Drugs. 2007;9(3):195–204.
Szczeklik A, Stevenson DD. Aspirin-induced asthma: advances in pathogenesis and management. J Allergy Clin Immunol. 1999;104(1):5–13.
Article PubMed CAS Google Scholar
NHS. nhs.uk. 2020 [cited 2023 Apr 4]. Medicines for babies and children. https://www.nhs.uk/conditions/baby/health/medicines-for-babies-and-children/
NHS. nhs.uk. 2022 [cited 2023 Apr 4]. Who can and cannot take ibuprofen for children. https://www.nhs.uk/medicines/ibuprofen-for-children/who-can-and-cannot-take-ibuprofen-for-children/
NHS. nhs.uk. 2022 [cited 2023 Apr 4]. Who can and cannot take paracetamol for children. https://www.nhs.uk/medicines/paracetamol-for-children/who-can-and-cannot-take-paracetamol-for-children/
Kanabar D, Dale S, Rawat M. A review of ibuprofen and acetaminophen use in febrile children and the occurrence of asthma-related symptoms. Clin Ther. 2007;29(12):2716–23.
Kanabar DJ. A clinical and safety review of Paracetamol and Ibuprofen in children. Inflammopharmacology. 2017;25(1):1–9.
Article PubMed PubMed Central CAS Google Scholar
Kauffman RE, Lieh-Lai M. Ibuprofen and increased morbidity in children with asthma: fact or fiction? Paediatr Drugs. 2004;6(5):267–72.
Pierce CA, Voss B. Efficacy and safety of ibuprofen and acetaminophen in children and adults: a meta-analysis and qualitative review. Ann Pharmacother. 2010;44(3):489–506.
Sherbash M, Furuya-Kanamori L, Nader JD, Thalib L. Risk of wheezing and asthma exacerbation in children treated with Paracetamol versus Ibuprofen: a systematic review and meta-analysis of randomised controlled trials. BMC Pulm Med. 2020;20(1):72.
Southey ER, Soares-Weiser K, Kleijnen J. Systematic review and meta-analysis of the clinical safety and tolerability of ibuprofen compared with paracetamol in paediatric pain and fever. Current medical research and opinion [Internet]. 2009 Sep [cited 2022 May 19];25(9). https://pubmed.ncbi.nlm.nih.gov/19606950/
Tan E, Braithwaite I, McKinlay CJD, Dalziel SR. Comparison of Acetaminophen (paracetamol) with Ibuprofen for Treatment of Fever or Pain in children younger than 2 years: a systematic review and Meta-analysis. JAMA Netw Open. 2020;3(10):e2022398.
Article PubMed PubMed Central Google Scholar
Szczeklik A. The cyclooxygenase theory of aspirin-induced asthma. Eur Respir J. 1990;3(5):588–93.
Su YM, Huang CS, Wan KS. Short-term ibuprofen treatment and pulmonary function in children with asthma. Indian Pediatr. 2015;52(8):691–3.
Moher D, Shamseer L, Clarke M, Ghersi D, Liberati A, Petticrew M, et al. Preferred reporting items for systematic review and meta-analysis protocols (PRISMA-P) 2015 statement. Syst Reviews. 2015;4(1):1.
Shamseer L, Moher D, Clarke M, Ghersi D, Liberati A, Petticrew M, et al. Preferred reporting items for systematic review and meta-analysis protocols (PRISMA-P) 2015: elaboration and explanation. BMJ. 2015;349:g7647.
McGowan J, Sampson M, Salzwedel DM, Cogo E, Foerster V, Lefebvre C. PRESS peer review of electronic search strategies: 2015 Guideline Statement. J Clin Epidemiol. 2016;75:40–6.
McGowan J, Sampson M, Salzwedel DM, Cogo E, Lefebvre C, PRESS Peer Review of Electronic Search Strategies. : 2015 Guideline Explanation and Elaboration (PRESS E&E). CADTH Methods and Guidelines [Internet]. 2016 [cited 2022 Mar 3]; https://www.cadth.ca/press-peer-review-electronic-search-strategies-2015-guideline-explanation-and-elaboration
Haddaway NR, Grainger MJ, Gray CT. citationchaser: An R package and Shiny app for forward and backward citations chasing in academic searching [Internet]. Zenodo; 2021 [cited 2023 Jan 17]. https://zenodo.org/record/4543513
Thomas J, Graziosi S, Brunton J, Ghouze Z, O’Driscoll P, Bond M et al. EPPI-Reviewer: advanced software for systematic reviews, maps and evidence synthesis [Internet]. EPPI-Centre, UCL Social Research Institute, University College London; 2022. http://eppi.ioe.ac.uk/cms/Default.aspx?tabid=2914
Sterne JAC, Savović J, Page MJ, Elbers RG, Blencowe NS, Boutron I, et al. RoB 2: a revised tool for assessing risk of bias in randomised trials. BMJ. 2019;366:l4898.
Wells G, Shea B, O’Connell D, Peterson J, Welch V, Losos M et al. The Newcastle–Ottawa Scale (NOS) for assessing the quality of nonrandomised studies in meta-analyses [Internet]. 2008 [cited 2022 May 23]. http://www.ohri.ca/programs/clinical_epidemiology/oxford.asp
Balduzzi S, Rücker G, Schwarzer G. How to perform a meta-analysis with R: a practical tutorial. Evid Based Ment Health. 2019;22(4):153–60.
Yusuf S, Peto R, Lewis J, Collins R, Sleight P. Beta blockade during and after myocardial infarction: an overview of the randomized trials. Prog Cardiovasc Dis. 1985;27(5):335–71.
Kokki H, Kokki M. Ketoprofen versus Paracetamol (acetaminophen) or ibuprofen in the management of fever: results of two randomized, double-blind, double-dummy, parallel-group, repeated-dose, multicentre, phase III studies in children. Clin Drug Investig. 2010;30(6):375–86.
Lesko SM, Mitchell AA. The safety of acetaminophen and ibuprofen among children younger than two years old. Pediatrics. 1999;104(4):e39.
Lesko SM, Mitchell AA. An assessment of the safety of pediatric ibuprofen. A practitioner-based randomized clinical trial. JAMA. 1995;273(12):929–33.
Luo S, Ran M, Luo Q, Shu M, Guo Q, Zhu Y, et al. Alternating Acetaminophen and Ibuprofen versus monotherapies in improvements of distress and reducing refractory fever in Febrile children: a Randomized Controlled Trial. Paediatr Drugs. 2017;19(5):479–86.
Matok I, Elizur A, Perlman A, Ganor S, Levine H, Kozer E. Association of Acetaminophen and Ibuprofen Use with Wheezing in Children with Acute Febrile illness. Ann Pharmacother. 2017;51(3):239–44.
McIntyre J, Hull D. Comparing efficacy and tolerability of Ibuprofen and Paracetamol in fever. Arch Dis Child. 1996;74(2):164–7.
Sordillo JE, Scirica CV, Rifas-Shiman SL, Gillman MW, Bunyavanich S, Camargo CA, et al. Prenatal and infant exposure to acetaminophen and ibuprofen and the risk for wheeze and asthma in children. J Allergy Clin Immunol. 2015;135(2):441–8.
Walsh P, Rothenberg SJ. Wheezing after the use of acetaminophen and or ibuprofen for first episode of bronchiolitis or respiratory tract infection. PLoS ONE. 2018;13(9):e0203770.
Wong A, Sibbald A, Ferrero F, Plager M, Santolaya ME, Escobar AM, et al. Antipyretic effects of dipyrone versus ibuprofen versus acetaminophen in children: results of a multinational, randomized, modified double-blind study. Clin Pediatr (Phila). 2001;40(6):313–24.
Fu LS, Lin CC, Wei CY, Lin CH, Huang YC. Risk of acute exacerbation between acetaminophen and ibuprofen in children with asthma. PeerJ. 2019;7:e6760.
Lesko SM, Louik C, Vezina RM, Mitchell AA. Asthma morbidity after the short-term use of ibuprofen in children. Pediatrics. 2002;109(2):E20.
Lo PC, Tsai YT, Lin SK, Lai JN. Risk of asthma exacerbation associated with nonsteroidal anti-inflammatory drugs in childhood asthma: a nationwide population-based cohort study in Taiwan. Med (Baltim). 2016;95(41):e5109.
Article CAS Google Scholar
Sheehan WJ, Mauger DT, Paul IM, Moy JN, Boehmer SJ, Szefler SJ, et al. Acetaminophen versus Ibuprofen in Young children with mild persistent asthma. N Engl J Med. 2016;375(7):619–30.
Corzo JL, Zambonino MA, Muñoz C, Mayorga C, Requena G, Urda A, et al. Tolerance to COX-2 inhibitors in children with hypersensitivity to nonsteroidal anti-inflammatory drugs. Br J Dermatol. 2014;170(3):725–9.
Ertoy Karagol HI, Yilmaz O, Topal E, Ceylan A, Bakirtas A. Nonsteroidal anti-inflammatory drugs-exacerbated respiratory disease in adolescents. Int Forum Allergy Rhinol. 2015;5(5):392–8.
Guvenir H, Dibek Misirlioglu E, Vezir E, Toyran M, Ginis T, Civelek E et al. Nonsteroidal anti-inflammatory drug hypersensitivity among children. Allergy Asthma Proc. 2015;36(5):386–93.
Yilmaz Topal O, Kulhas Celik I, Turgay Yagmur I, Toyran M, Civelek E, Karaatmaca B, et al. Results of NSAID provocation tests and difficulties in the classification of children with nonsteroidal anti-inflammatory drug hypersensitivity. Ann Allergy Asthma Immunol. 2020;125(2):202–7.
Debley JS, Carter ER, Gibson RL, Rosenfeld M, Redding GJ. The prevalence of ibuprofen-sensitive asthma in children: a randomized controlled bronchoprovocation challenge study. J Pediatr. 2005;147(2):233–8.
Goraya JS, Virdi VS. To the editor: exacerbation of asthma by ibuprofen in a very young child. Pediatr Pulmonol. 2001;32(3):262.
King G, Byrne A, Fleming P. A case of severe NSAID exacerbated respiratory disease (NERD) following a dental procedure in a child. Eur Arch Paediatr Dent. 2016;17(4):277–81.
Malmström K, Kaila M, Kajosaari M, Syvänen P, Juntunen-Backman K. Fatal asthma in Finnish children and adolescents 1976–1998: validity of death certificates and a clinical description. Pediatr Pulmonol. 2007;42(3):210–5.
Menendez R, Venzor J, Ortiz G. Failure of zafirlukast to prevent ibuprofen-induced anaphylaxis. Ann Allergy Asthma Immunol. 1998;80(3):225–6.
Palmer GM. A teenager with severe asthma exacerbation following ibuprofen. Anaesth Intensive Care. 2005;33(2):261–5.
Schnabel E, Heinrich J. Respiratory tract infections and not Paracetamol medication during infancy are associated with asthma development in childhood. J Allergy Clin Immunol. 2010;126(5):1071–3.
CRD. Chapter 4: systematic reviews of adverse effects. Systematic reviews: CRD’s guidance for undertaking reviews in health care. York Publishing Services; 2009.
Reeves B, Deeks J, Higgins J, Shea B, Tugwell P, Wells G. Chapter 24: Including non-randomized studies on intervention effects. In: Higgins J, Thomas J, Chandler J, Cumpston M, Li T, Page M, editors. Cochrane Handbook for Systematic Reviews of Interventions [Internet]. 6.3. Cochrane; 2022 [cited 2023 Apr 7]. https://training.cochrane.org/handbook/current/chapter-24
Riley J, Braithwaite I, Shirtcliffe P, Caswell-Smith R, Hunt A, Bowden V, et al. Randomized controlled trial of asthma risk with Paracetamol use in infancy–a feasibility study. Clin Exp Allergy. 2015;45(2):448–56.
Tan E, Braithwaite I, McKinlay C, Riley J, Hoare K, Okesene-Gafa K, et al. Randomised controlled trial of Paracetamol or Ibuprofen, as required for fever and pain in the first year of life, for prevention of asthma at age 6 years: Paracetamol or Ibuprofen in the primary prevention of asthma in Tamariki (PIPPA Tamariki) protocol. BMJ Open. 2020;10(12):e038296.
Download references
Acknowledgements
We thank Imran Lodhi, Fiona Murray-Zmijewski, Frederic Esclassan, and Bill Laughey for reviewing and advising on improvements for this systematic review. We thank Carolyn Smith, the Outreach Librarian at University of Oxford’s Bodleian Libraries, who performed the search strategy PRESS Peer Review.
This work was funded by Reckitt. Employees of Reckitt were involved in the design and conduct of the study; collection, management, analysis, and interpretation of the data; preparation, review, or approval of a manuscript; and decision regarding where to submit the manuscript for publication.
Author information
Authors and affiliations.
Department of Paediatrics, University of Oxford, Oxford, UK
Luke Baxter, Maria M. Cobo, Aomesh Bhatt & Rebeccah Slater
Colegio de Ciencias Biologicas y Ambientales, Universidad San Francisco de Quito USFQ, Quito, Ecuador
Maria M. Cobo
Reckitt, Dansom Lane, Hull, HU8 7DS, UK
Olutoba Sanni
Reckitt (Global Headquarters), Turner House, 103-105 Bath Road, Slough, Berkshire, SL1 3UH, UK
Nutan Shinde
You can also search for this author in PubMed Google Scholar
Contributions
LB: conceptualization, methodology, data curation, formal analysis, investigation, visualization, writing – original draft, writing – review & editing. MC: methodology, data curation, investigation, validation, writing – review & editing. AB: conceptualization, methodology, data curation, investigation, writing – review & editing. RS: conceptualization, methodology, data curation, investigation, writing – review & editing. OS: conceptualization, methodology, project administration, writing – review & editing. NS: conceptualization, methodology, project administration, writing – review & editing.
Corresponding author
Correspondence to Luke Baxter .
Ethics declarations
Ethics approval and consent to participate.
Not Applicable.
Consent for publication
Competing interests.
OS and NS are current employees of Reckitt and may hold equity interest in Reckitt. LB and RS were compensated by Reckitt for activities related to execution of the study. MC and AB declare no competing interests. No other disclosures were reported.
Additional information
Publisher’s note.
Springer Nature remains neutral with regard to jurisdictional claims in published maps and institutional affiliations.
Electronic supplementary material
Below is the link to the electronic supplementary material.
Supplementary Material 1
Rights and permissions.
Open Access This article is licensed under a Creative Commons Attribution 4.0 International License, which permits use, sharing, adaptation, distribution and reproduction in any medium or format, as long as you give appropriate credit to the original author(s) and the source, provide a link to the Creative Commons licence, and indicate if changes were made. The images or other third party material in this article are included in the article’s Creative Commons licence, unless indicated otherwise in a credit line to the material. If material is not included in the article’s Creative Commons licence and your intended use is not permitted by statutory regulation or exceeds the permitted use, you will need to obtain permission directly from the copyright holder. To view a copy of this licence, visit http://creativecommons.org/licenses/by/4.0/ .
Reprints and permissions
About this article
Cite this article.
Baxter, L., Cobo, M.M., Bhatt, A. et al. The association between ibuprofen administration in children and the risk of developing or exacerbating asthma: a systematic review and meta-analysis. BMC Pulm Med 24 , 412 (2024). https://doi.org/10.1186/s12890-024-03179-3
Download citation
Received : 24 October 2023
Accepted : 22 July 2024
Published : 26 August 2024
DOI : https://doi.org/10.1186/s12890-024-03179-3
Share this article
Anyone you share the following link with will be able to read this content:
Sorry, a shareable link is not currently available for this article.
Provided by the Springer Nature SharedIt content-sharing initiative
- Hypersensitivity
- Bronchospasm
- Bronchoconstriction
BMC Pulmonary Medicine
ISSN: 1471-2466
- Submission enquiries: [email protected]
- General enquiries: [email protected]

- Biological and Environmental Research
- BER Highlights
- Biological and Environmental Research Advisory Committee (BERAC)
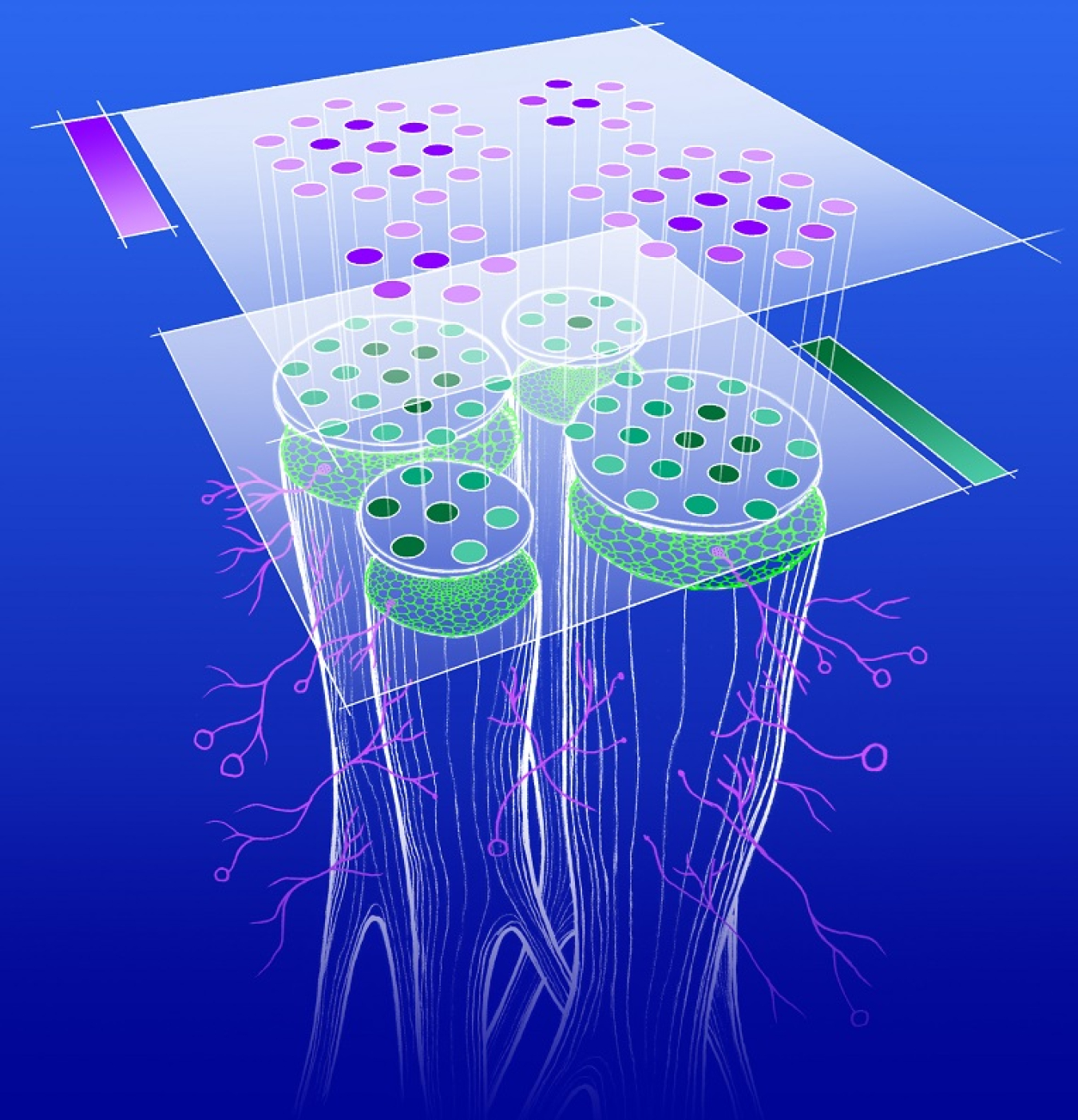
The Science
In most plants, fungi are found in close association (symbiosis) with their roots. These fungi, called mycorrhizae, help plants obtain water and other nutrients. Meanwhile, plants provide the fungi with carbon nutrients generated through photosynthesis. This symbiosis occurs in microscopic structures called arbuscules that help transfer nutrients between the plants and the fungi. To better understand gene expression—how genes tell cells what to do—in plant/mycorrhizae symbioses, researchers analyzed roots of a model plant colonized by fungi. They used a combination of advanced techniques to measure gene activity in tens of thousands of individual cells and to visualize gene expression in two-dimensional sections of roots. The microscopic resolution of the analysis allowed the researchers to generate a spatial map of gene expression in both the root and the fungal cells.
Symbioses between arbuscular mycorrhizae and plants occur in most ecosystems. These symbioses are important for agriculture, as the fungi provide critical nutrients to the plants. However, this interaction is restricted to a few root cells, making it difficult to study. This study’s spatial and single-cell examination of plant-fungal interactions sheds new light on this process. Understanding both sides of this symbiosis at the molecular level may enable researchers to make targeted improvements to the way plants and mycorrhizae interact. This could be applied to bioenergy crops to increase their productivity and their ability to store carbon .
The symbiotic interaction of plants with arbuscular mycorrhizal (AM) fungi is ancient and widespread. Plants provide AM fungi with carbon in exchange for nutrients and water, making this interaction a prime target for crop improvement. However, plant–fungal interactions are restricted to a small subset of root cells, precluding the application of most conventional functional genomic techniques to study the molecular bases of these interactions.
Researchers at the Joint Genome Institute (JGI), a Department of Energy (DOE) user facility, and the DOE Joint Bioenergy Institute used single-nucleus and spatial RNA sequencing to explore both Medicago truncatula and Rhizophagus irregularis transcriptomes in AM symbiosis at cellular and spatial resolution. Integrated, spatially registered single-cell maps revealed infected and uninfected plant root cell types. The researchers observed that cortex cells exhibit distinct transcriptome profiles during different stages of colonization by AM fungi. This indicates dynamic interplay between both organisms during establishment of the cellular interface enabling successful symbiosis. This study provides insight into a symbiotic relationship of major agricultural and environmental importance and demonstrates a paradigm combining single-cell and spatial transcriptomics for the analysis of complex organismal interactions.
Benjamin Cole Joint Genome Institute [email protected]
This study was performed at the Department of Energy’s Joint BioEnergy Institute and Joint Genome Institute and was supported by the DOE Office of Science, Biological and Environmental Research Program. This study was also supported by a Laboratory Directed Research and Development award at Lawrence Berkeley National Laboratory and a DOE Early Career Research Program. Two of the researchers were funded by the Novo Nordisk Foundation.
Publications
Serrano, K., et al. , Spatial co-transcriptomics reveals discrete stages of the arbuscular mycorrhizal symbiosis . Nature Plants 10 , 673–688 (2024). [DOI: 10.1038/s41477-024-01666-3]
Related Links
JGI Press Release: An Inside Look at How Plants and Mycorrhizal Fungi Cooperate
JGI Genome Insider Podcast: Better Crops With a Pointillist Approach to Plant Genomics
Cookies on GOV.UK
We use some essential cookies to make this website work.
We’d like to set additional cookies to understand how you use GOV.UK, remember your settings and improve government services.
We also use cookies set by other sites to help us deliver content from their services.
You have accepted additional cookies. You can change your cookie settings at any time.
You have rejected additional cookies. You can change your cookie settings at any time.
Generative AI in education: user research and technical report
Reports on the insights from teachers, leaders and pupils on the potential uses of generative artificial intelligence in education.
Applies to England
Use cases for generative ai in education: user research report.
Ref: ISBN 978-1-83870-564-0, RR1423
PDF , 1.66 MB , 121 pages
Use cases for generative AI in education: technical report
Ref: ISBN 978-1-83870-565-7, RR1442
PDF , 2.33 MB , 124 pages
The reporting from this project contains 2 documents:
- a narrative report on the process, user research, and findings of the generative AI hackathons project
- a detailed technical report describing the process of optimising a generative AI ‘proof of concept’ tool based on the findings of the hackathon
Updates to this page
Sign up for emails or print this page, related content, is this page useful.
- Yes this page is useful
- No this page is not useful
Help us improve GOV.UK
Don’t include personal or financial information like your National Insurance number or credit card details.
To help us improve GOV.UK, we’d like to know more about your visit today. Please fill in this survey (opens in a new tab) .
Meta's use of AI has far from peaked. But the technology may already be getting the company in trouble.
- Meta CEO Mark Zuckerberg has promised that AI will revolutionize the company's ad services.
- But Meta's use of AI for moderating ads may already be putting the company in hot water.
- A bipartisan group of lawmakers accused Meta of allowing ads that promote the sale of illicit drugs.

During July's earnings call, Meta CEO Mark Zuckerberg laid out a vision for his company's valuable ad services once they are further bolstered by artificial intelligence.
"In the coming years," he said, "AI will be able to generate creative for advertisers as well and will also be able to personalize it as people see it."
But as the trillion-dollar company hopes to revolutionize its ad tech , Meta's usage of AI may already have put the company in the hot seat.
On Thursday, a bipartisan group of lawmakers, led by Republican Rep. Tim Walberg of Michigan and Democratic Rep. Kathy Castor of Florida, sent a letter to Zuckerberg demanding the CEO answer questions about Meta's advertising services.
The letter comes in light of a March Wall Street Journal report that revealed how federal prosecutors are probing the company for its role in the illicit sale of drugs on its platforms.
"Meta appears to have continued to shirk its social responsibility and defy its own community guidelines," the letter said. "Protecting users online, especially children and teenagers, is one of our top priorities. We are continuously concerned that Meta is not up to the task and this dereliction of duty needs to be addressed."
Zuckerberg already faced senators who grilled the CEO about safety measures for children who use Meta's social media sites. During the senate hearing, Zuckerberg stood up and apologized to families who felt that social media use harmed their kids.
In July, the Tech Transparency Project , a nonprofit watchdog group, reported that Meta continued to make money from hundreds of ads that promoted the sale of illegal or recreational drugs, including cocaine and opioids, which Meta prohibits in its policy regarding ads.
"Many of the ads made no secret of their intentions, showing photos of prescription drug bottles, piles of pills and powders, or bricks of cocaine, and encouraging users to place orders," the watchdog group wrote.
"Our systems are designed to proactively detect and enforce against violating content, and we reject hundreds of thousands of ads for violating our drug policies," a Meta spokesperson told Business Insider, reiterating a statement shared with the Journal. "We continue to invest resources and further improve our enforcement on this kind of content. Our hearts go out to those suffering from the tragic consequences of this epidemic — it requires all of us to work together to stop it."
Related stories
The spokesperson did not address how Meta uses AI to moderate ads.
Ads poke holes in Meta's AI system
The exact processes for how Meta approves and moderates ads are not public information.
What is known is that the company, in part, relies on artificial intelligence to screen content, as reported by the Journal . The outlet reported that using photos to display the drugs may allow the ads to slip past Meta's moderation system.
Here's what Meta has revealed about its "ad review system":
"Our ad review system relies primarily on automated technology to apply the Advertising Standards to the millions of ads that are run across Meta technologies. However, we do use human reviewers to improve and train our automated systems, and in some cases, to manually review ads."
The company also said it's continuously working to automate the review process further to rely less on humans.
But the revelation of ads promoting drugs on Meta's platforms shows how policy-violating content can still slip through its automated system, even while Zuckerberg paints a picture of a sophisticated ad service that promises improved targeting and creates content for advertisers with generative AI.
Meta's bumpy AI rollout
Meta has experienced a bumpy rollout of its AI-powered services outside ad tech.
Less than a year after Meta introduced celebrity AI assistants , the company discontinued the product and focused on allowing users to create their own AI bots.
Meta also continues to work out kinks for Meta AI, the company's chatbot and AI assistant, which has been shown to hallucinate answers or, with BI's Rob Price, act like a user and hand out his phone number to strangers.
The technical and ethical issues that pervade AI products — not just Meta's — concern many top US companies.
A survey by Arize AI, which conducts research around AI technology, showed that 56% of Fortune 500 companies view AI as a "risk factor," The Financial Times reported.
Sifting by industry, 86% of technology groups, including Salesforce, said that AI presents a business risk, according to the report.
Those concerns, however, conflict with tech companies' evident push to implement AI into every corner of their products, even as the path toward profitability also remains murky .
"There are significant risks involved in developing and deploying AI," Meta said in a 2023 annual report, "and there can be no assurance that the usage of AI will enhance our products or services or be beneficial to our business, including our efficiency or profitability."
Watch: US Bank has used AI for fraud detection as well as brand campaigns, but CMO Michael Lacorazza says the potential of the technology is yet to be fully understood
- Main content
InfoQ Software Architects' Newsletter
A monthly overview of things you need to know as an architect or aspiring architect.
View an example
We protect your privacy.
QCon London (April 7-9, 2025): Get actionable advice for your engineering challenges. Adopt the right emerging trends. Register
Facilitating the Spread of Knowledge and Innovation in Professional Software Development
- English edition
- Chinese edition
- Japanese edition
- French edition
Back to login
Login with:
Don't have an infoq account, helpful links.
- About InfoQ
- InfoQ Editors
Write for InfoQ
- About C4Media
Choose your language
Get clarity from senior software practitioners on today's critical dev priorities. Register Now.
Level up your software skills by uncovering the emerging trends you should focus on. Register now.
Discover emerging trends, insights, and real-world best practices in software development & tech leadership. Join now.
Your monthly guide to all the topics, technologies and techniques that every professional needs to know about. Subscribe for free.
InfoQ Homepage News Advancing System Reliability: Meta's AI-Driven Approach to Root Cause Analysis
Advancing System Reliability: Meta's AI-Driven Approach to Root Cause Analysis
Aug 22, 2024 2 min read
Claudio Masolo
Meta recently shared how they are enhancing their system reliability through advanced investigation tools, including the AI-assisted Hawkeye , which aids in debugging machine learning workflows. By integrating Artificial Intelligence, Meta has developed a new investigation system that combines heuristic-based retrieval with large language model (LLM) ranking to assist in root cause analysis. This system has shown promising results, achieving 42% accuracy in identifying root causes at the start of an investigation related to Meta's web monorepo.
HawkEye is a toolkit developed by Meta as part of the Prediction Robustness program. It was created to drive innovative tools and services and ensure the quality of Meta products relying on machine learning (ML) model predictions. HawkEye is developed to enhance the monitoring, observability and debuggability of Meta ML products. It includes everything from mining root causes with UX workflows for guided explorations.
Investigating issues within large systems like Meta's can be complex, especially when dealing with monolithic repositories that involve multiple teams and numerous changes. To build context and isolate the root cause, traditional investigations require significant time and effort. To streamline this process, Meta's new system reduces the search space for potential causes using heuristics, such as code ownership and runtime code graphs. After narrowing down to a few hundred relevant changes, an LLM-based ranking system identifies the most likely root causes, ultimately focusing on the top five changes.
The ranking system, which uses a fine-tuned Llama model , employs a structured prompt technique to handle context window limitations, allowing it to rank changes effectively. Back testing has shown that in 42% of cases, the actual root cause is among the top five ranked suggestions.

Training the LLM involved fine-tuning a Llama 2 (7B) model using Meta's historical investigation data, which helped the model learn to follow root cause analysis (RCA) instructions. This training process used a specially curated dataset of 5,000 instruction-tuning examples with details of 2-20 changes from Meta retriever, including the known root cause and information about the investigation at its start, e.g., its title and impact. This curated dataset allows the model to rank potential code changes based on their relevance to an investigation with a good confidence level.
Meta's AI-assisted investigation tools aim to reduce the time and effort needed for root cause analysis, but they also present challenges, such as the risk of incorrect suggestions. To address this, Meta ensures that the system's results are explainable and reproducible, with confidence measurements used to avoid low-confidence recommendations.
Other AI-assisted investigation tools available on the market are:
- BigPanda Root Cause Analysis: An AI-powered tool that quickly identifies the root cause of issues in IT systems by analyzing data and providing recommendations. It features automatic issue identification in real time, reducing investigation and resolution time.
- ZDX AI-Powered Root Cause Analysis: A tool that leverages AI and machine learning to analyze data and provide recommendations for remediation, enabling fast identification of issues in networks and applications.
- IBM Watson AIOps : An AI-powered tool that analyzes data to identify the root cause of issues in IT systems, providing recommendations for remediation and automatic issue identification in real time.
- Skylar Automated Root Cause Analysis: A tool that automates log analysis using machine learning, processing millions or billions of log messages from applications to identify the root cause of issues quickly.
Looking ahead, Meta plans to expand the capabilities of its AI systems, potentially allowing them to autonomously execute workflows and even detect potential incidents before they occur, further enhancing system reliability.
About the Author
Rate this article, this content is in the devops topic, related topics:.
- Artificial Intelligence
- Site Reliability Engineering
Related Editorial
Related sponsored content, how to end the confusion in cloud transformations: insights from mckinsey & company, related sponsor.
/filters:no_upscale()/sponsorship/topic/c3ea523a-b725-40e0-9341-fcdbf57bf0f1/McKLogoRSB-1720613430518.png)
Building digital futures. Together.
Related Content
The infoq newsletter.
A round-up of last week’s content on InfoQ sent out every Tuesday. Join a community of over 250,000 senior developers. View an example
Study Design 101: Meta-Analysis
- Case Report
- Case Control Study
- Cohort Study
- Randomized Controlled Trial
- Practice Guideline
- Systematic Review
Meta-Analysis
- Helpful Formulas
- Finding Specific Study Types
A subset of systematic reviews; a method for systematically combining pertinent qualitative and quantitative study data from several selected studies to develop a single conclusion that has greater statistical power. This conclusion is statistically stronger than the analysis of any single study, due to increased numbers of subjects, greater diversity among subjects, or accumulated effects and results.
Meta-analysis would be used for the following purposes:
- To establish statistical significance with studies that have conflicting results
- To develop a more correct estimate of effect magnitude
- To provide a more complex analysis of harms, safety data, and benefits
- To examine subgroups with individual numbers that are not statistically significant
If the individual studies utilized randomized controlled trials (RCT), combining several selected RCT results would be the highest-level of evidence on the evidence hierarchy, followed by systematic reviews, which analyze all available studies on a topic.
- Greater statistical power
- Confirmatory data analysis
- Greater ability to extrapolate to general population affected
- Considered an evidence-based resource
Disadvantages
- Difficult and time consuming to identify appropriate studies
- Not all studies provide adequate data for inclusion and analysis
- Requires advanced statistical techniques
- Heterogeneity of study populations

Design pitfalls to look out for
The studies pooled for review should be similar in type (i.e. all randomized controlled trials).
Are the studies being reviewed all the same type of study or are they a mixture of different types?
The analysis should include published and unpublished results to avoid publication bias.
Does the meta-analysis include any appropriate relevant studies that may have had negative outcomes?
Fictitious Example
Do individuals who wear sunscreen have fewer cases of melanoma than those who do not wear sunscreen? A MEDLINE search was conducted using the terms melanoma, sunscreening agents, and zinc oxide, resulting in 8 randomized controlled studies, each with between 100 and 120 subjects. All of the studies showed a positive effect between wearing sunscreen and reducing the likelihood of melanoma. The subjects from all eight studies (total: 860 subjects) were pooled and statistically analyzed to determine the effect of the relationship between wearing sunscreen and melanoma. This meta-analysis showed a 50% reduction in melanoma diagnosis among sunscreen-wearers.
Real-life Examples
Goyal, A., Elminawy, M., Kerezoudis, P., Lu, V., Yolcu, Y., Alvi, M., & Bydon, M. (2019). Impact of obesity on outcomes following lumbar spine surgery: A systematic review and meta-analysis. Clinical Neurology and Neurosurgery, 177 , 27-36. https://doi.org/10.1016/j.clineuro.2018.12.012
This meta-analysis was interested in determining whether obesity affects the outcome of spinal surgery. Some previous studies have shown higher perioperative morbidity in patients with obesity while other studies have not shown this effect. This study looked at surgical outcomes including "blood loss, operative time, length of stay, complication and reoperation rates and functional outcomes" between patients with and without obesity. A meta-analysis of 32 studies (23,415 patients) was conducted. There were no significant differences for patients undergoing minimally invasive surgery, but patients with obesity who had open surgery had experienced higher blood loss and longer operative times (not clinically meaningful) as well as higher complication and reoperation rates. Further research is needed to explore this issue in patients with morbid obesity.
Nakamura, A., van Der Waerden, J., Melchior, M., Bolze, C., El-Khoury, F., & Pryor, L. (2019). Physical activity during pregnancy and postpartum depression: Systematic review and meta-analysis. Journal of Affective Disorders, 246 , 29-41. https://doi.org/10.1016/j.jad.2018.12.009
This meta-analysis explored whether physical activity during pregnancy prevents postpartum depression. Seventeen studies were included (93,676 women) and analysis showed a "significant reduction in postpartum depression scores in women who were physically active during their pregnancies when compared with inactive women." Possible limitations or moderators of this effect include intensity and frequency of physical activity, type of physical activity, and timepoint in pregnancy (e.g. trimester).
Related Terms
A document often written by a panel that provides a comprehensive review of all relevant studies on a particular clinical or health-related topic/question.
Publication Bias
A phenomenon in which studies with positive results have a better chance of being published, are published earlier, and are published in journals with higher impact factors. Therefore, conclusions based exclusively on published studies can be misleading.
Now test yourself!
1. A Meta-Analysis pools together the sample populations from different studies, such as Randomized Controlled Trials, into one statistical analysis and treats them as one large sample population with one conclusion.
a) True b) False
2. One potential design pitfall of Meta-Analyses that is important to pay attention to is:
a) Whether it is evidence-based. b) If the authors combined studies with conflicting results. c) If the authors appropriately combined studies so they did not compare apples and oranges. d) If the authors used only quantitative data.
Evidence Pyramid - Navigation
- Meta- Analysis
- Case Reports
- << Previous: Systematic Review
- Next: Helpful Formulas >>

- Last Updated: Sep 25, 2023 10:59 AM
- URL: https://guides.himmelfarb.gwu.edu/studydesign101

- Himmelfarb Intranet
- Privacy Notice
- Terms of Use
- GW is committed to digital accessibility. If you experience a barrier that affects your ability to access content on this page, let us know via the Accessibility Feedback Form .
- Himmelfarb Health Sciences Library
- 2300 Eye St., NW, Washington, DC 20037
- Phone: (202) 994-2962
- [email protected]
- https://himmelfarb.gwu.edu
How to conduct a meta-analysis in eight steps: a practical guide
- Open access
- Published: 30 November 2021
- Volume 72 , pages 1–19, ( 2022 )
Cite this article
You have full access to this open access article
- Christopher Hansen 1 ,
- Holger Steinmetz 2 &
- Jörn Block 3 , 4 , 5
170k Accesses
57 Citations
158 Altmetric
Explore all metrics
Avoid common mistakes on your manuscript.
1 Introduction
“Scientists have known for centuries that a single study will not resolve a major issue. Indeed, a small sample study will not even resolve a minor issue. Thus, the foundation of science is the cumulation of knowledge from the results of many studies.” (Hunter et al. 1982 , p. 10)
Meta-analysis is a central method for knowledge accumulation in many scientific fields (Aguinis et al. 2011c ; Kepes et al. 2013 ). Similar to a narrative review, it serves as a synopsis of a research question or field. However, going beyond a narrative summary of key findings, a meta-analysis adds value in providing a quantitative assessment of the relationship between two target variables or the effectiveness of an intervention (Gurevitch et al. 2018 ). Also, it can be used to test competing theoretical assumptions against each other or to identify important moderators where the results of different primary studies differ from each other (Aguinis et al. 2011b ; Bergh et al. 2016 ). Rooted in the synthesis of the effectiveness of medical and psychological interventions in the 1970s (Glass 2015 ; Gurevitch et al. 2018 ), meta-analysis is nowadays also an established method in management research and related fields.
The increasing importance of meta-analysis in management research has resulted in the publication of guidelines in recent years that discuss the merits and best practices in various fields, such as general management (Bergh et al. 2016 ; Combs et al. 2019 ; Gonzalez-Mulé and Aguinis 2018 ), international business (Steel et al. 2021 ), economics and finance (Geyer-Klingeberg et al. 2020 ; Havranek et al. 2020 ), marketing (Eisend 2017 ; Grewal et al. 2018 ), and organizational studies (DeSimone et al. 2020 ; Rudolph et al. 2020 ). These articles discuss existing and trending methods and propose solutions for often experienced problems. This editorial briefly summarizes the insights of these papers; provides a workflow of the essential steps in conducting a meta-analysis; suggests state-of-the art methodological procedures; and points to other articles for in-depth investigation. Thus, this article has two goals: (1) based on the findings of previous editorials and methodological articles, it defines methodological recommendations for meta-analyses submitted to Management Review Quarterly (MRQ); and (2) it serves as a practical guide for researchers who have little experience with meta-analysis as a method but plan to conduct one in the future.
2 Eight steps in conducting a meta-analysis
2.1 step 1: defining the research question.
The first step in conducting a meta-analysis, as with any other empirical study, is the definition of the research question. Most importantly, the research question determines the realm of constructs to be considered or the type of interventions whose effects shall be analyzed. When defining the research question, two hurdles might develop. First, when defining an adequate study scope, researchers must consider that the number of publications has grown exponentially in many fields of research in recent decades (Fortunato et al. 2018 ). On the one hand, a larger number of studies increases the potentially relevant literature basis and enables researchers to conduct meta-analyses. Conversely, scanning a large amount of studies that could be potentially relevant for the meta-analysis results in a perhaps unmanageable workload. Thus, Steel et al. ( 2021 ) highlight the importance of balancing manageability and relevance when defining the research question. Second, similar to the number of primary studies also the number of meta-analyses in management research has grown strongly in recent years (Geyer-Klingeberg et al. 2020 ; Rauch 2020 ; Schwab 2015 ). Therefore, it is likely that one or several meta-analyses for many topics of high scholarly interest already exist. However, this should not deter researchers from investigating their research questions. One possibility is to consider moderators or mediators of a relationship that have previously been ignored. For example, a meta-analysis about startup performance could investigate the impact of different ways to measure the performance construct (e.g., growth vs. profitability vs. survival time) or certain characteristics of the founders as moderators. Another possibility is to replicate previous meta-analyses and test whether their findings can be confirmed with an updated sample of primary studies or newly developed methods. Frequent replications and updates of meta-analyses are important contributions to cumulative science and are increasingly called for by the research community (Anderson & Kichkha 2017 ; Steel et al. 2021 ). Consistent with its focus on replication studies (Block and Kuckertz 2018 ), MRQ therefore also invites authors to submit replication meta-analyses.
2.2 Step 2: literature search
2.2.1 search strategies.
Similar to conducting a literature review, the search process of a meta-analysis should be systematic, reproducible, and transparent, resulting in a sample that includes all relevant studies (Fisch and Block 2018 ; Gusenbauer and Haddaway 2020 ). There are several identification strategies for relevant primary studies when compiling meta-analytical datasets (Harari et al. 2020 ). First, previous meta-analyses on the same or a related topic may provide lists of included studies that offer a good starting point to identify and become familiar with the relevant literature. This practice is also applicable to topic-related literature reviews, which often summarize the central findings of the reviewed articles in systematic tables. Both article types likely include the most prominent studies of a research field. The most common and important search strategy, however, is a keyword search in electronic databases (Harari et al. 2020 ). This strategy will probably yield the largest number of relevant studies, particularly so-called ‘grey literature’, which may not be considered by literature reviews. Gusenbauer and Haddaway ( 2020 ) provide a detailed overview of 34 scientific databases, of which 18 are multidisciplinary or have a focus on management sciences, along with their suitability for literature synthesis. To prevent biased results due to the scope or journal coverage of one database, researchers should use at least two different databases (DeSimone et al. 2020 ; Martín-Martín et al. 2021 ; Mongeon & Paul-Hus 2016 ). However, a database search can easily lead to an overload of potentially relevant studies. For example, key term searches in Google Scholar for “entrepreneurial intention” and “firm diversification” resulted in more than 660,000 and 810,000 hits, respectively. Footnote 1 Therefore, a precise research question and precise search terms using Boolean operators are advisable (Gusenbauer and Haddaway 2020 ). Addressing the challenge of identifying relevant articles in the growing number of database publications, (semi)automated approaches using text mining and machine learning (Bosco et al. 2017 ; O’Mara-Eves et al. 2015 ; Ouzzani et al. 2016 ; Thomas et al. 2017 ) can also be promising and time-saving search tools in the future. Also, some electronic databases offer the possibility to track forward citations of influential studies and thereby identify further relevant articles. Finally, collecting unpublished or undetected studies through conferences, personal contact with (leading) scholars, or listservs can be strategies to increase the study sample size (Grewal et al. 2018 ; Harari et al. 2020 ; Pigott and Polanin 2020 ).
2.2.2 Study inclusion criteria and sample composition
Next, researchers must decide which studies to include in the meta-analysis. Some guidelines for literature reviews recommend limiting the sample to studies published in renowned academic journals to ensure the quality of findings (e.g., Kraus et al. 2020 ). For meta-analysis, however, Steel et al. ( 2021 ) advocate for the inclusion of all available studies, including grey literature, to prevent selection biases based on availability, cost, familiarity, and language (Rothstein et al. 2005 ), or the “Matthew effect”, which denotes the phenomenon that highly cited articles are found faster than less cited articles (Merton 1968 ). Harrison et al. ( 2017 ) find that the effects of published studies in management are inflated on average by 30% compared to unpublished studies. This so-called publication bias or “file drawer problem” (Rosenthal 1979 ) results from the preference of academia to publish more statistically significant and less statistically insignificant study results. Owen and Li ( 2020 ) showed that publication bias is particularly severe when variables of interest are used as key variables rather than control variables. To consider the true effect size of a target variable or relationship, the inclusion of all types of research outputs is therefore recommended (Polanin et al. 2016 ). Different test procedures to identify publication bias are discussed subsequently in Step 7.
In addition to the decision of whether to include certain study types (i.e., published vs. unpublished studies), there can be other reasons to exclude studies that are identified in the search process. These reasons can be manifold and are primarily related to the specific research question and methodological peculiarities. For example, studies identified by keyword search might not qualify thematically after all, may use unsuitable variable measurements, or may not report usable effect sizes. Furthermore, there might be multiple studies by the same authors using similar datasets. If they do not differ sufficiently in terms of their sample characteristics or variables used, only one of these studies should be included to prevent bias from duplicates (Wood 2008 ; see this article for a detection heuristic).
In general, the screening process should be conducted stepwise, beginning with a removal of duplicate citations from different databases, followed by abstract screening to exclude clearly unsuitable studies and a final full-text screening of the remaining articles (Pigott and Polanin 2020 ). A graphical tool to systematically document the sample selection process is the PRISMA flow diagram (Moher et al. 2009 ). Page et al. ( 2021 ) recently presented an updated version of the PRISMA statement, including an extended item checklist and flow diagram to report the study process and findings.
2.3 Step 3: choice of the effect size measure
2.3.1 types of effect sizes.
The two most common meta-analytical effect size measures in management studies are (z-transformed) correlation coefficients and standardized mean differences (Aguinis et al. 2011a ; Geyskens et al. 2009 ). However, meta-analyses in management science and related fields may not be limited to those two effect size measures but rather depend on the subfield of investigation (Borenstein 2009 ; Stanley and Doucouliagos 2012 ). In economics and finance, researchers are more interested in the examination of elasticities and marginal effects extracted from regression models than in pure bivariate correlations (Stanley and Doucouliagos 2012 ). Regression coefficients can also be converted to partial correlation coefficients based on their t-statistics to make regression results comparable across studies (Stanley and Doucouliagos 2012 ). Although some meta-analyses in management research have combined bivariate and partial correlations in their study samples, Aloe ( 2015 ) and Combs et al. ( 2019 ) advise researchers not to use this practice. Most importantly, they argue that the effect size strength of partial correlations depends on the other variables included in the regression model and is therefore incomparable to bivariate correlations (Schmidt and Hunter 2015 ), resulting in a possible bias of the meta-analytic results (Roth et al. 2018 ). We endorse this opinion. If at all, we recommend separate analyses for each measure. In addition to these measures, survival rates, risk ratios or odds ratios, which are common measures in medical research (Borenstein 2009 ), can be suitable effect sizes for specific management research questions, such as understanding the determinants of the survival of startup companies. To summarize, the choice of a suitable effect size is often taken away from the researcher because it is typically dependent on the investigated research question as well as the conventions of the specific research field (Cheung and Vijayakumar 2016 ).
2.3.2 Conversion of effect sizes to a common measure
After having defined the primary effect size measure for the meta-analysis, it might become necessary in the later coding process to convert study findings that are reported in effect sizes that are different from the chosen primary effect size. For example, a study might report only descriptive statistics for two study groups but no correlation coefficient, which is used as the primary effect size measure in the meta-analysis. Different effect size measures can be harmonized using conversion formulae, which are provided by standard method books such as Borenstein et al. ( 2009 ) or Lipsey and Wilson ( 2001 ). There also exist online effect size calculators for meta-analysis. Footnote 2
2.4 Step 4: choice of the analytical method used
Choosing which meta-analytical method to use is directly connected to the research question of the meta-analysis. Research questions in meta-analyses can address a relationship between constructs or an effect of an intervention in a general manner, or they can focus on moderating or mediating effects. There are four meta-analytical methods that are primarily used in contemporary management research (Combs et al. 2019 ; Geyer-Klingeberg et al. 2020 ), which allow the investigation of these different types of research questions: traditional univariate meta-analysis, meta-regression, meta-analytic structural equation modeling, and qualitative meta-analysis (Hoon 2013 ). While the first three are quantitative, the latter summarizes qualitative findings. Table 1 summarizes the key characteristics of the three quantitative methods.
2.4.1 Univariate meta-analysis
In its traditional form, a meta-analysis reports a weighted mean effect size for the relationship or intervention of investigation and provides information on the magnitude of variance among primary studies (Aguinis et al. 2011c ; Borenstein et al. 2009 ). Accordingly, it serves as a quantitative synthesis of a research field (Borenstein et al. 2009 ; Geyskens et al. 2009 ). Prominent traditional approaches have been developed, for example, by Hedges and Olkin ( 1985 ) or Hunter and Schmidt ( 1990 , 2004 ). However, going beyond its simple summary function, the traditional approach has limitations in explaining the observed variance among findings (Gonzalez-Mulé and Aguinis 2018 ). To identify moderators (or boundary conditions) of the relationship of interest, meta-analysts can create subgroups and investigate differences between those groups (Borenstein and Higgins 2013 ; Hunter and Schmidt 2004 ). Potential moderators can be study characteristics (e.g., whether a study is published vs. unpublished), sample characteristics (e.g., study country, industry focus, or type of survey/experiment participants), or measurement artifacts (e.g., different types of variable measurements). The univariate approach is thus suitable to identify the overall direction of a relationship and can serve as a good starting point for additional analyses. However, due to its limitations in examining boundary conditions and developing theory, the univariate approach on its own is currently oftentimes viewed as not sufficient (Rauch 2020 ; Shaw and Ertug 2017 ).
2.4.2 Meta-regression analysis
Meta-regression analysis (Hedges and Olkin 1985 ; Lipsey and Wilson 2001 ; Stanley and Jarrell 1989 ) aims to investigate the heterogeneity among observed effect sizes by testing multiple potential moderators simultaneously. In meta-regression, the coded effect size is used as the dependent variable and is regressed on a list of moderator variables. These moderator variables can be categorical variables as described previously in the traditional univariate approach or (semi)continuous variables such as country scores that are merged with the meta-analytical data. Thus, meta-regression analysis overcomes the disadvantages of the traditional approach, which only allows us to investigate moderators singularly using dichotomized subgroups (Combs et al. 2019 ; Gonzalez-Mulé and Aguinis 2018 ). These possibilities allow a more fine-grained analysis of research questions that are related to moderating effects. However, Schmidt ( 2017 ) critically notes that the number of effect sizes in the meta-analytical sample must be sufficiently large to produce reliable results when investigating multiple moderators simultaneously in a meta-regression. For further reading, Tipton et al. ( 2019 ) outline the technical, conceptual, and practical developments of meta-regression over the last decades. Gonzalez-Mulé and Aguinis ( 2018 ) provide an overview of methodological choices and develop evidence-based best practices for future meta-analyses in management using meta-regression.
2.4.3 Meta-analytic structural equation modeling (MASEM)
MASEM is a combination of meta-analysis and structural equation modeling and allows to simultaneously investigate the relationships among several constructs in a path model. Researchers can use MASEM to test several competing theoretical models against each other or to identify mediation mechanisms in a chain of relationships (Bergh et al. 2016 ). This method is typically performed in two steps (Cheung and Chan 2005 ): In Step 1, a pooled correlation matrix is derived, which includes the meta-analytical mean effect sizes for all variable combinations; Step 2 then uses this matrix to fit the path model. While MASEM was based primarily on traditional univariate meta-analysis to derive the pooled correlation matrix in its early years (Viswesvaran and Ones 1995 ), more advanced methods, such as the GLS approach (Becker 1992 , 1995 ) or the TSSEM approach (Cheung and Chan 2005 ), have been subsequently developed. Cheung ( 2015a ) and Jak ( 2015 ) provide an overview of these approaches in their books with exemplary code. For datasets with more complex data structures, Wilson et al. ( 2016 ) also developed a multilevel approach that is related to the TSSEM approach in the second step. Bergh et al. ( 2016 ) discuss nine decision points and develop best practices for MASEM studies.
2.4.4 Qualitative meta-analysis
While the approaches explained above focus on quantitative outcomes of empirical studies, qualitative meta-analysis aims to synthesize qualitative findings from case studies (Hoon 2013 ; Rauch et al. 2014 ). The distinctive feature of qualitative case studies is their potential to provide in-depth information about specific contextual factors or to shed light on reasons for certain phenomena that cannot usually be investigated by quantitative studies (Rauch 2020 ; Rauch et al. 2014 ). In a qualitative meta-analysis, the identified case studies are systematically coded in a meta-synthesis protocol, which is then used to identify influential variables or patterns and to derive a meta-causal network (Hoon 2013 ). Thus, the insights of contextualized and typically nongeneralizable single studies are aggregated to a larger, more generalizable picture (Habersang et al. 2019 ). Although still the exception, this method can thus provide important contributions for academics in terms of theory development (Combs et al., 2019 ; Hoon 2013 ) and for practitioners in terms of evidence-based management or entrepreneurship (Rauch et al. 2014 ). Levitt ( 2018 ) provides a guide and discusses conceptual issues for conducting qualitative meta-analysis in psychology, which is also useful for management researchers.
2.5 Step 5: choice of software
Software solutions to perform meta-analyses range from built-in functions or additional packages of statistical software to software purely focused on meta-analyses and from commercial to open-source solutions. However, in addition to personal preferences, the choice of the most suitable software depends on the complexity of the methods used and the dataset itself (Cheung and Vijayakumar 2016 ). Meta-analysts therefore must carefully check if their preferred software is capable of performing the intended analysis.
Among commercial software providers, Stata (from version 16 on) offers built-in functions to perform various meta-analytical analyses or to produce various plots (Palmer and Sterne 2016 ). For SPSS and SAS, there exist several macros for meta-analyses provided by scholars, such as David B. Wilson or Andy P. Field and Raphael Gillet (Field and Gillett 2010 ). Footnote 3 Footnote 4 For researchers using the open-source software R (R Core Team 2021 ), Polanin et al. ( 2017 ) provide an overview of 63 meta-analysis packages and their functionalities. For new users, they recommend the package metafor (Viechtbauer 2010 ), which includes most necessary functions and for which the author Wolfgang Viechtbauer provides tutorials on his project website. Footnote 5 Footnote 6 In addition to packages and macros for statistical software, templates for Microsoft Excel have also been developed to conduct simple meta-analyses, such as Meta-Essentials by Suurmond et al. ( 2017 ). Footnote 7 Finally, programs purely dedicated to meta-analysis also exist, such as Comprehensive Meta-Analysis (Borenstein et al. 2013 ) or RevMan by The Cochrane Collaboration ( 2020 ).
2.6 Step 6: coding of effect sizes
2.6.1 coding sheet.
The first step in the coding process is the design of the coding sheet. A universal template does not exist because the design of the coding sheet depends on the methods used, the respective software, and the complexity of the research design. For univariate meta-analysis or meta-regression, data are typically coded in wide format. In its simplest form, when investigating a correlational relationship between two variables using the univariate approach, the coding sheet would contain a column for the study name or identifier, the effect size coded from the primary study, and the study sample size. However, such simple relationships are unlikely in management research because the included studies are typically not identical but differ in several respects. With more complex data structures or moderator variables being investigated, additional columns are added to the coding sheet to reflect the data characteristics. These variables can be coded as dummy, factor, or (semi)continuous variables and later used to perform a subgroup analysis or meta regression. For MASEM, the required data input format can deviate depending on the method used (e.g., TSSEM requires a list of correlation matrices as data input). For qualitative meta-analysis, the coding scheme typically summarizes the key qualitative findings and important contextual and conceptual information (see Hoon ( 2013 ) for a coding scheme for qualitative meta-analysis). Figure 1 shows an exemplary coding scheme for a quantitative meta-analysis on the correlational relationship between top-management team diversity and profitability. In addition to effect and sample sizes, information about the study country, firm type, and variable operationalizations are coded. The list could be extended by further study and sample characteristics.

Exemplary coding sheet for a meta-analysis on the relationship (correlation) between top-management team diversity and profitability
2.6.2 Inclusion of moderator or control variables
It is generally important to consider the intended research model and relevant nontarget variables before coding a meta-analytic dataset. For example, study characteristics can be important moderators or function as control variables in a meta-regression model. Similarly, control variables may be relevant in a MASEM approach to reduce confounding bias. Coding additional variables or constructs subsequently can be arduous if the sample of primary studies is large. However, the decision to include respective moderator or control variables, as in any empirical analysis, should always be based on strong (theoretical) rationales about how these variables can impact the investigated effect (Bernerth and Aguinis 2016 ; Bernerth et al. 2018 ; Thompson and Higgins 2002 ). While substantive moderators refer to theoretical constructs that act as buffers or enhancers of a supposed causal process, methodological moderators are features of the respective research designs that denote the methodological context of the observations and are important to control for systematic statistical particularities (Rudolph et al. 2020 ). Havranek et al. ( 2020 ) provide a list of recommended variables to code as potential moderators. While researchers may have clear expectations about the effects for some of these moderators, the concerns for other moderators may be tentative, and moderator analysis may be approached in a rather exploratory fashion. Thus, we argue that researchers should make full use of the meta-analytical design to obtain insights about potential context dependence that a primary study cannot achieve.
2.6.3 Treatment of multiple effect sizes in a study
A long-debated issue in conducting meta-analyses is whether to use only one or all available effect sizes for the same construct within a single primary study. For meta-analyses in management research, this question is fundamental because many empirical studies, particularly those relying on company databases, use multiple variables for the same construct to perform sensitivity analyses, resulting in multiple relevant effect sizes. In this case, researchers can either (randomly) select a single value, calculate a study average, or use the complete set of effect sizes (Bijmolt and Pieters 2001 ; López-López et al. 2018 ). Multiple effect sizes from the same study enrich the meta-analytic dataset and allow us to investigate the heterogeneity of the relationship of interest, such as different variable operationalizations (López-López et al. 2018 ; Moeyaert et al. 2017 ). However, including more than one effect size from the same study violates the independency assumption of observations (Cheung 2019 ; López-López et al. 2018 ), which can lead to biased results and erroneous conclusions (Gooty et al. 2021 ). We follow the recommendation of current best practice guides to take advantage of using all available effect size observations but to carefully consider interdependencies using appropriate methods such as multilevel models, panel regression models, or robust variance estimation (Cheung 2019 ; Geyer-Klingeberg et al. 2020 ; Gooty et al. 2021 ; López-López et al. 2018 ; Moeyaert et al. 2017 ).
2.7 Step 7: analysis
2.7.1 outlier analysis and tests for publication bias.
Before conducting the primary analysis, some preliminary sensitivity analyses might be necessary, which should ensure the robustness of the meta-analytical findings (Rudolph et al. 2020 ). First, influential outlier observations could potentially bias the observed results, particularly if the number of total effect sizes is small. Several statistical methods can be used to identify outliers in meta-analytical datasets (Aguinis et al. 2013 ; Viechtbauer and Cheung 2010 ). However, there is a debate about whether to keep or omit these observations. Anyhow, relevant studies should be closely inspected to infer an explanation about their deviating results. As in any other primary study, outliers can be a valid representation, albeit representing a different population, measure, construct, design or procedure. Thus, inferences about outliers can provide the basis to infer potential moderators (Aguinis et al. 2013 ; Steel et al. 2021 ). On the other hand, outliers can indicate invalid research, for instance, when unrealistically strong correlations are due to construct overlap (i.e., lack of a clear demarcation between independent and dependent variables), invalid measures, or simply typing errors when coding effect sizes. An advisable step is therefore to compare the results both with and without outliers and base the decision on whether to exclude outlier observations with careful consideration (Geyskens et al. 2009 ; Grewal et al. 2018 ; Kepes et al. 2013 ). However, instead of simply focusing on the size of the outlier, its leverage should be considered. Thus, Viechtbauer and Cheung ( 2010 ) propose considering a combination of standardized deviation and a study’s leverage.
Second, as mentioned in the context of a literature search, potential publication bias may be an issue. Publication bias can be examined in multiple ways (Rothstein et al. 2005 ). First, the funnel plot is a simple graphical tool that can provide an overview of the effect size distribution and help to detect publication bias (Stanley and Doucouliagos 2010 ). A funnel plot can also support in identifying potential outliers. As mentioned above, a graphical display of deviation (e.g., studentized residuals) and leverage (Cook’s distance) can help detect the presence of outliers and evaluate their influence (Viechtbauer and Cheung 2010 ). Moreover, several statistical procedures can be used to test for publication bias (Harrison et al. 2017 ; Kepes et al. 2012 ), including subgroup comparisons between published and unpublished studies, Begg and Mazumdar’s ( 1994 ) rank correlation test, cumulative meta-analysis (Borenstein et al. 2009 ), the trim and fill method (Duval and Tweedie 2000a , b ), Egger et al.’s ( 1997 ) regression test, failsafe N (Rosenthal 1979 ), or selection models (Hedges and Vevea 2005 ; Vevea and Woods 2005 ). In examining potential publication bias, Kepes et al. ( 2012 ) and Harrison et al. ( 2017 ) both recommend not relying only on a single test but rather using multiple conceptionally different test procedures (i.e., the so-called “triangulation approach”).
2.7.2 Model choice
After controlling and correcting for the potential presence of impactful outliers or publication bias, the next step in meta-analysis is the primary analysis, where meta-analysts must decide between two different types of models that are based on different assumptions: fixed-effects and random-effects (Borenstein et al. 2010 ). Fixed-effects models assume that all observations share a common mean effect size, which means that differences are only due to sampling error, while random-effects models assume heterogeneity and allow for a variation of the true effect sizes across studies (Borenstein et al. 2010 ; Cheung and Vijayakumar 2016 ; Hunter and Schmidt 2004 ). Both models are explained in detail in standard textbooks (e.g., Borenstein et al. 2009 ; Hunter and Schmidt 2004 ; Lipsey and Wilson 2001 ).
In general, the presence of heterogeneity is likely in management meta-analyses because most studies do not have identical empirical settings, which can yield different effect size strengths or directions for the same investigated phenomenon. For example, the identified studies have been conducted in different countries with different institutional settings, or the type of study participants varies (e.g., students vs. employees, blue-collar vs. white-collar workers, or manufacturing vs. service firms). Thus, the vast majority of meta-analyses in management research and related fields use random-effects models (Aguinis et al. 2011a ). In a meta-regression, the random-effects model turns into a so-called mixed-effects model because moderator variables are added as fixed effects to explain the impact of observed study characteristics on effect size variations (Raudenbush 2009 ).
2.8 Step 8: reporting results
2.8.1 reporting in the article.
The final step in performing a meta-analysis is reporting its results. Most importantly, all steps and methodological decisions should be comprehensible to the reader. DeSimone et al. ( 2020 ) provide an extensive checklist for journal reviewers of meta-analytical studies. This checklist can also be used by authors when performing their analyses and reporting their results to ensure that all important aspects have been addressed. Alternative checklists are provided, for example, by Appelbaum et al. ( 2018 ) or Page et al. ( 2021 ). Similarly, Levitt et al. ( 2018 ) provide a detailed guide for qualitative meta-analysis reporting standards.
For quantitative meta-analyses, tables reporting results should include all important information and test statistics, including mean effect sizes; standard errors and confidence intervals; the number of observations and study samples included; and heterogeneity measures. If the meta-analytic sample is rather small, a forest plot provides a good overview of the different findings and their accuracy. However, this figure will be less feasible for meta-analyses with several hundred effect sizes included. Also, results displayed in the tables and figures must be explained verbally in the results and discussion sections. Most importantly, authors must answer the primary research question, i.e., whether there is a positive, negative, or no relationship between the variables of interest, or whether the examined intervention has a certain effect. These results should be interpreted with regard to their magnitude (or significance), both economically and statistically. However, when discussing meta-analytical results, authors must describe the complexity of the results, including the identified heterogeneity and important moderators, future research directions, and theoretical relevance (DeSimone et al. 2019 ). In particular, the discussion of identified heterogeneity and underlying moderator effects is critical; not including this information can lead to false conclusions among readers, who interpret the reported mean effect size as universal for all included primary studies and ignore the variability of findings when citing the meta-analytic results in their research (Aytug et al. 2012 ; DeSimone et al. 2019 ).
2.8.2 Open-science practices
Another increasingly important topic is the public provision of meta-analytical datasets and statistical codes via open-source repositories. Open-science practices allow for results validation and for the use of coded data in subsequent meta-analyses ( Polanin et al. 2020 ), contributing to the development of cumulative science. Steel et al. ( 2021 ) refer to open science meta-analyses as a step towards “living systematic reviews” (Elliott et al. 2017 ) with continuous updates in real time. MRQ supports this development and encourages authors to make their datasets publicly available. Moreau and Gamble ( 2020 ), for example, provide various templates and video tutorials to conduct open science meta-analyses. There exist several open science repositories, such as the Open Science Foundation (OSF; for a tutorial, see Soderberg 2018 ), to preregister and make documents publicly available. Furthermore, several initiatives in the social sciences have been established to develop dynamic meta-analyses, such as metaBUS (Bosco et al. 2015 , 2017 ), MetaLab (Bergmann et al. 2018 ), or PsychOpen CAMA (Burgard et al. 2021 ).
3 Conclusion
This editorial provides a comprehensive overview of the essential steps in conducting and reporting a meta-analysis with references to more in-depth methodological articles. It also serves as a guide for meta-analyses submitted to MRQ and other management journals. MRQ welcomes all types of meta-analyses from all subfields and disciplines of management research.
Gusenbauer and Haddaway ( 2020 ), however, point out that Google Scholar is not appropriate as a primary search engine due to a lack of reproducibility of search results.
One effect size calculator by David B. Wilson is accessible via: https://www.campbellcollaboration.org/escalc/html/EffectSizeCalculator-Home.php .
The macros of David B. Wilson can be downloaded from: http://mason.gmu.edu/~dwilsonb/ .
The macros of Field and Gillet ( 2010 ) can be downloaded from: https://www.discoveringstatistics.com/repository/fieldgillett/how_to_do_a_meta_analysis.html .
The tutorials can be found via: https://www.metafor-project.org/doku.php .
Metafor does currently not provide functions to conduct MASEM. For MASEM, users can, for instance, use the package metaSEM (Cheung 2015b ).
The workbooks can be downloaded from: https://www.erim.eur.nl/research-support/meta-essentials/ .
Aguinis H, Dalton DR, Bosco FA, Pierce CA, Dalton CM (2011a) Meta-analytic choices and judgment calls: Implications for theory building and testing, obtained effect sizes, and scholarly impact. J Manag 37(1):5–38
Google Scholar
Aguinis H, Gottfredson RK, Joo H (2013) Best-practice recommendations for defining, identifying, and handling outliers. Organ Res Methods 16(2):270–301
Article Google Scholar
Aguinis H, Gottfredson RK, Wright TA (2011b) Best-practice recommendations for estimating interaction effects using meta-analysis. J Organ Behav 32(8):1033–1043
Aguinis H, Pierce CA, Bosco FA, Dalton DR, Dalton CM (2011c) Debunking myths and urban legends about meta-analysis. Organ Res Methods 14(2):306–331
Aloe AM (2015) Inaccuracy of regression results in replacing bivariate correlations. Res Synth Methods 6(1):21–27
Anderson RG, Kichkha A (2017) Replication, meta-analysis, and research synthesis in economics. Am Econ Rev 107(5):56–59
Appelbaum M, Cooper H, Kline RB, Mayo-Wilson E, Nezu AM, Rao SM (2018) Journal article reporting standards for quantitative research in psychology: the APA publications and communications BOARD task force report. Am Psychol 73(1):3–25
Aytug ZG, Rothstein HR, Zhou W, Kern MC (2012) Revealed or concealed? Transparency of procedures, decisions, and judgment calls in meta-analyses. Organ Res Methods 15(1):103–133
Begg CB, Mazumdar M (1994) Operating characteristics of a rank correlation test for publication bias. Biometrics 50(4):1088–1101. https://doi.org/10.2307/2533446
Bergh DD, Aguinis H, Heavey C, Ketchen DJ, Boyd BK, Su P, Lau CLL, Joo H (2016) Using meta-analytic structural equation modeling to advance strategic management research: Guidelines and an empirical illustration via the strategic leadership-performance relationship. Strateg Manag J 37(3):477–497
Becker BJ (1992) Using results from replicated studies to estimate linear models. J Educ Stat 17(4):341–362
Becker BJ (1995) Corrections to “Using results from replicated studies to estimate linear models.” J Edu Behav Stat 20(1):100–102
Bergmann C, Tsuji S, Piccinini PE, Lewis ML, Braginsky M, Frank MC, Cristia A (2018) Promoting replicability in developmental research through meta-analyses: Insights from language acquisition research. Child Dev 89(6):1996–2009
Bernerth JB, Aguinis H (2016) A critical review and best-practice recommendations for control variable usage. Pers Psychol 69(1):229–283
Bernerth JB, Cole MS, Taylor EC, Walker HJ (2018) Control variables in leadership research: A qualitative and quantitative review. J Manag 44(1):131–160
Bijmolt TH, Pieters RG (2001) Meta-analysis in marketing when studies contain multiple measurements. Mark Lett 12(2):157–169
Block J, Kuckertz A (2018) Seven principles of effective replication studies: Strengthening the evidence base of management research. Manag Rev Quart 68:355–359
Borenstein M (2009) Effect sizes for continuous data. In: Cooper H, Hedges LV, Valentine JC (eds) The handbook of research synthesis and meta-analysis. Russell Sage Foundation, pp 221–235
Borenstein M, Hedges LV, Higgins JPT, Rothstein HR (2009) Introduction to meta-analysis. John Wiley, Chichester
Book Google Scholar
Borenstein M, Hedges LV, Higgins JPT, Rothstein HR (2010) A basic introduction to fixed-effect and random-effects models for meta-analysis. Res Synth Methods 1(2):97–111
Borenstein M, Hedges L, Higgins J, Rothstein H (2013) Comprehensive meta-analysis (version 3). Biostat, Englewood, NJ
Borenstein M, Higgins JP (2013) Meta-analysis and subgroups. Prev Sci 14(2):134–143
Bosco FA, Steel P, Oswald FL, Uggerslev K, Field JG (2015) Cloud-based meta-analysis to bridge science and practice: Welcome to metaBUS. Person Assess Decis 1(1):3–17
Bosco FA, Uggerslev KL, Steel P (2017) MetaBUS as a vehicle for facilitating meta-analysis. Hum Resour Manag Rev 27(1):237–254
Burgard T, Bošnjak M, Studtrucker R (2021) Community-augmented meta-analyses (CAMAs) in psychology: potentials and current systems. Zeitschrift Für Psychologie 229(1):15–23
Cheung MWL (2015a) Meta-analysis: A structural equation modeling approach. John Wiley & Sons, Chichester
Cheung MWL (2015b) metaSEM: An R package for meta-analysis using structural equation modeling. Front Psychol 5:1521
Cheung MWL (2019) A guide to conducting a meta-analysis with non-independent effect sizes. Neuropsychol Rev 29(4):387–396
Cheung MWL, Chan W (2005) Meta-analytic structural equation modeling: a two-stage approach. Psychol Methods 10(1):40–64
Cheung MWL, Vijayakumar R (2016) A guide to conducting a meta-analysis. Neuropsychol Rev 26(2):121–128
Combs JG, Crook TR, Rauch A (2019) Meta-analytic research in management: contemporary approaches unresolved controversies and rising standards. J Manag Stud 56(1):1–18. https://doi.org/10.1111/joms.12427
DeSimone JA, Köhler T, Schoen JL (2019) If it were only that easy: the use of meta-analytic research by organizational scholars. Organ Res Methods 22(4):867–891. https://doi.org/10.1177/1094428118756743
DeSimone JA, Brannick MT, O’Boyle EH, Ryu JW (2020) Recommendations for reviewing meta-analyses in organizational research. Organ Res Methods 56:455–463
Duval S, Tweedie R (2000a) Trim and fill: a simple funnel-plot–based method of testing and adjusting for publication bias in meta-analysis. Biometrics 56(2):455–463
Duval S, Tweedie R (2000b) A nonparametric “trim and fill” method of accounting for publication bias in meta-analysis. J Am Stat Assoc 95(449):89–98
Egger M, Smith GD, Schneider M, Minder C (1997) Bias in meta-analysis detected by a simple, graphical test. BMJ 315(7109):629–634
Eisend M (2017) Meta-Analysis in advertising research. J Advert 46(1):21–35
Elliott JH, Synnot A, Turner T, Simmons M, Akl EA, McDonald S, Salanti G, Meerpohl J, MacLehose H, Hilton J, Tovey D, Shemilt I, Thomas J (2017) Living systematic review: 1. Introduction—the why, what, when, and how. J Clin Epidemiol 91:2330. https://doi.org/10.1016/j.jclinepi.2017.08.010
Field AP, Gillett R (2010) How to do a meta-analysis. Br J Math Stat Psychol 63(3):665–694
Fisch C, Block J (2018) Six tips for your (systematic) literature review in business and management research. Manag Rev Quart 68:103–106
Fortunato S, Bergstrom CT, Börner K, Evans JA, Helbing D, Milojević S, Petersen AM, Radicchi F, Sinatra R, Uzzi B, Vespignani A (2018) Science of science. Science 359(6379). https://doi.org/10.1126/science.aao0185
Geyer-Klingeberg J, Hang M, Rathgeber A (2020) Meta-analysis in finance research: Opportunities, challenges, and contemporary applications. Int Rev Finan Anal 71:101524
Geyskens I, Krishnan R, Steenkamp JBE, Cunha PV (2009) A review and evaluation of meta-analysis practices in management research. J Manag 35(2):393–419
Glass GV (2015) Meta-analysis at middle age: a personal history. Res Synth Methods 6(3):221–231
Gonzalez-Mulé E, Aguinis H (2018) Advancing theory by assessing boundary conditions with metaregression: a critical review and best-practice recommendations. J Manag 44(6):2246–2273
Gooty J, Banks GC, Loignon AC, Tonidandel S, Williams CE (2021) Meta-analyses as a multi-level model. Organ Res Methods 24(2):389–411. https://doi.org/10.1177/1094428119857471
Grewal D, Puccinelli N, Monroe KB (2018) Meta-analysis: integrating accumulated knowledge. J Acad Mark Sci 46(1):9–30
Gurevitch J, Koricheva J, Nakagawa S, Stewart G (2018) Meta-analysis and the science of research synthesis. Nature 555(7695):175–182
Gusenbauer M, Haddaway NR (2020) Which academic search systems are suitable for systematic reviews or meta-analyses? Evaluating retrieval qualities of Google Scholar, PubMed, and 26 other resources. Res Synth Methods 11(2):181–217
Habersang S, Küberling-Jost J, Reihlen M, Seckler C (2019) A process perspective on organizational failure: a qualitative meta-analysis. J Manage Stud 56(1):19–56
Harari MB, Parola HR, Hartwell CJ, Riegelman A (2020) Literature searches in systematic reviews and meta-analyses: A review, evaluation, and recommendations. J Vocat Behav 118:103377
Harrison JS, Banks GC, Pollack JM, O’Boyle EH, Short J (2017) Publication bias in strategic management research. J Manag 43(2):400–425
Havránek T, Stanley TD, Doucouliagos H, Bom P, Geyer-Klingeberg J, Iwasaki I, Reed WR, Rost K, Van Aert RCM (2020) Reporting guidelines for meta-analysis in economics. J Econ Surveys 34(3):469–475
Hedges LV, Olkin I (1985) Statistical methods for meta-analysis. Academic Press, Orlando
Hedges LV, Vevea JL (2005) Selection methods approaches. In: Rothstein HR, Sutton A, Borenstein M (eds) Publication bias in meta-analysis: prevention, assessment, and adjustments. Wiley, Chichester, pp 145–174
Hoon C (2013) Meta-synthesis of qualitative case studies: an approach to theory building. Organ Res Methods 16(4):522–556
Hunter JE, Schmidt FL (1990) Methods of meta-analysis: correcting error and bias in research findings. Sage, Newbury Park
Hunter JE, Schmidt FL (2004) Methods of meta-analysis: correcting error and bias in research findings, 2nd edn. Sage, Thousand Oaks
Hunter JE, Schmidt FL, Jackson GB (1982) Meta-analysis: cumulating research findings across studies. Sage Publications, Beverly Hills
Jak S (2015) Meta-analytic structural equation modelling. Springer, New York, NY
Kepes S, Banks GC, McDaniel M, Whetzel DL (2012) Publication bias in the organizational sciences. Organ Res Methods 15(4):624–662
Kepes S, McDaniel MA, Brannick MT, Banks GC (2013) Meta-analytic reviews in the organizational sciences: Two meta-analytic schools on the way to MARS (the Meta-Analytic Reporting Standards). J Bus Psychol 28(2):123–143
Kraus S, Breier M, Dasí-Rodríguez S (2020) The art of crafting a systematic literature review in entrepreneurship research. Int Entrepreneur Manag J 16(3):1023–1042
Levitt HM (2018) How to conduct a qualitative meta-analysis: tailoring methods to enhance methodological integrity. Psychother Res 28(3):367–378
Levitt HM, Bamberg M, Creswell JW, Frost DM, Josselson R, Suárez-Orozco C (2018) Journal article reporting standards for qualitative primary, qualitative meta-analytic, and mixed methods research in psychology: the APA publications and communications board task force report. Am Psychol 73(1):26
Lipsey MW, Wilson DB (2001) Practical meta-analysis. Sage Publications, Inc.
López-López JA, Page MJ, Lipsey MW, Higgins JP (2018) Dealing with effect size multiplicity in systematic reviews and meta-analyses. Res Synth Methods 9(3):336–351
Martín-Martín A, Thelwall M, Orduna-Malea E, López-Cózar ED (2021) Google Scholar, Microsoft Academic, Scopus, Dimensions, Web of Science, and OpenCitations’ COCI: a multidisciplinary comparison of coverage via citations. Scientometrics 126(1):871–906
Merton RK (1968) The Matthew effect in science: the reward and communication systems of science are considered. Science 159(3810):56–63
Moeyaert M, Ugille M, Natasha Beretvas S, Ferron J, Bunuan R, Van den Noortgate W (2017) Methods for dealing with multiple outcomes in meta-analysis: a comparison between averaging effect sizes, robust variance estimation and multilevel meta-analysis. Int J Soc Res Methodol 20(6):559–572
Moher D, Liberati A, Tetzlaff J, Altman DG, Prisma Group (2009) Preferred reporting items for systematic reviews and meta-analyses: the PRISMA statement. PLoS medicine. 6(7):e1000097
Mongeon P, Paul-Hus A (2016) The journal coverage of Web of Science and Scopus: a comparative analysis. Scientometrics 106(1):213–228
Moreau D, Gamble B (2020) Conducting a meta-analysis in the age of open science: Tools, tips, and practical recommendations. Psychol Methods. https://doi.org/10.1037/met0000351
O’Mara-Eves A, Thomas J, McNaught J, Miwa M, Ananiadou S (2015) Using text mining for study identification in systematic reviews: a systematic review of current approaches. Syst Rev 4(1):1–22
Ouzzani M, Hammady H, Fedorowicz Z, Elmagarmid A (2016) Rayyan—a web and mobile app for systematic reviews. Syst Rev 5(1):1–10
Owen E, Li Q (2021) The conditional nature of publication bias: a meta-regression analysis. Polit Sci Res Methods 9(4):867–877
Page MJ, McKenzie JE, Bossuyt PM, Boutron I, Hoffmann TC, Mulrow CD, Shamseer L, Tetzlaff JM, Akl EA, Brennan SE, Chou R, Glanville J, Grimshaw JM, Hróbjartsson A, Lalu MM, Li T, Loder EW, Mayo-Wilson E,McDonald S,McGuinness LA, Stewart LA, Thomas J, Tricco AC, Welch VA, Whiting P, Moher D (2021) The PRISMA 2020 statement: an updated guideline for reporting systematic reviews. BMJ 372. https://doi.org/10.1136/bmj.n71
Palmer TM, Sterne JAC (eds) (2016) Meta-analysis in stata: an updated collection from the stata journal, 2nd edn. Stata Press, College Station, TX
Pigott TD, Polanin JR (2020) Methodological guidance paper: High-quality meta-analysis in a systematic review. Rev Educ Res 90(1):24–46
Polanin JR, Tanner-Smith EE, Hennessy EA (2016) Estimating the difference between published and unpublished effect sizes: a meta-review. Rev Educ Res 86(1):207–236
Polanin JR, Hennessy EA, Tanner-Smith EE (2017) A review of meta-analysis packages in R. J Edu Behav Stat 42(2):206–242
Polanin JR, Hennessy EA, Tsuji S (2020) Transparency and reproducibility of meta-analyses in psychology: a meta-review. Perspect Psychol Sci 15(4):1026–1041. https://doi.org/10.1177/17456916209064
R Core Team (2021). R: A language and environment for statistical computing . R Foundation for Statistical Computing, Vienna, Austria. URL https://www.R-project.org/ .
Rauch A (2020) Opportunities and threats in reviewing entrepreneurship theory and practice. Entrep Theory Pract 44(5):847–860
Rauch A, van Doorn R, Hulsink W (2014) A qualitative approach to evidence–based entrepreneurship: theoretical considerations and an example involving business clusters. Entrep Theory Pract 38(2):333–368
Raudenbush SW (2009) Analyzing effect sizes: Random-effects models. In: Cooper H, Hedges LV, Valentine JC (eds) The handbook of research synthesis and meta-analysis, 2nd edn. Russell Sage Foundation, New York, NY, pp 295–315
Rosenthal R (1979) The file drawer problem and tolerance for null results. Psychol Bull 86(3):638
Rothstein HR, Sutton AJ, Borenstein M (2005) Publication bias in meta-analysis: prevention, assessment and adjustments. Wiley, Chichester
Roth PL, Le H, Oh I-S, Van Iddekinge CH, Bobko P (2018) Using beta coefficients to impute missing correlations in meta-analysis research: Reasons for caution. J Appl Psychol 103(6):644–658. https://doi.org/10.1037/apl0000293
Rudolph CW, Chang CK, Rauvola RS, Zacher H (2020) Meta-analysis in vocational behavior: a systematic review and recommendations for best practices. J Vocat Behav 118:103397
Schmidt FL (2017) Statistical and measurement pitfalls in the use of meta-regression in meta-analysis. Career Dev Int 22(5):469–476
Schmidt FL, Hunter JE (2015) Methods of meta-analysis: correcting error and bias in research findings. Sage, Thousand Oaks
Schwab A (2015) Why all researchers should report effect sizes and their confidence intervals: Paving the way for meta–analysis and evidence–based management practices. Entrepreneurship Theory Pract 39(4):719–725. https://doi.org/10.1111/etap.12158
Shaw JD, Ertug G (2017) The suitability of simulations and meta-analyses for submissions to Academy of Management Journal. Acad Manag J 60(6):2045–2049
Soderberg CK (2018) Using OSF to share data: A step-by-step guide. Adv Methods Pract Psychol Sci 1(1):115–120
Stanley TD, Doucouliagos H (2010) Picture this: a simple graph that reveals much ado about research. J Econ Surveys 24(1):170–191
Stanley TD, Doucouliagos H (2012) Meta-regression analysis in economics and business. Routledge, London
Stanley TD, Jarrell SB (1989) Meta-regression analysis: a quantitative method of literature surveys. J Econ Surveys 3:54–67
Steel P, Beugelsdijk S, Aguinis H (2021) The anatomy of an award-winning meta-analysis: Recommendations for authors, reviewers, and readers of meta-analytic reviews. J Int Bus Stud 52(1):23–44
Suurmond R, van Rhee H, Hak T (2017) Introduction, comparison, and validation of Meta-Essentials: a free and simple tool for meta-analysis. Res Synth Methods 8(4):537–553
The Cochrane Collaboration (2020). Review Manager (RevMan) [Computer program] (Version 5.4).
Thomas J, Noel-Storr A, Marshall I, Wallace B, McDonald S, Mavergames C, Glasziou P, Shemilt I, Synnot A, Turner T, Elliot J (2017) Living systematic reviews: 2. Combining human and machine effort. J Clin Epidemiol 91:31–37
Thompson SG, Higgins JP (2002) How should meta-regression analyses be undertaken and interpreted? Stat Med 21(11):1559–1573
Tipton E, Pustejovsky JE, Ahmadi H (2019) A history of meta-regression: technical, conceptual, and practical developments between 1974 and 2018. Res Synth Methods 10(2):161–179
Vevea JL, Woods CM (2005) Publication bias in research synthesis: Sensitivity analysis using a priori weight functions. Psychol Methods 10(4):428–443
Viechtbauer W (2010) Conducting meta-analyses in R with the metafor package. J Stat Softw 36(3):1–48
Viechtbauer W, Cheung MWL (2010) Outlier and influence diagnostics for meta-analysis. Res Synth Methods 1(2):112–125
Viswesvaran C, Ones DS (1995) Theory testing: combining psychometric meta-analysis and structural equations modeling. Pers Psychol 48(4):865–885
Wilson SJ, Polanin JR, Lipsey MW (2016) Fitting meta-analytic structural equation models with complex datasets. Res Synth Methods 7(2):121–139. https://doi.org/10.1002/jrsm.1199
Wood JA (2008) Methodology for dealing with duplicate study effects in a meta-analysis. Organ Res Methods 11(1):79–95
Download references
Open Access funding enabled and organized by Projekt DEAL. No funding was received to assist with the preparation of this manuscript.
Author information
Authors and affiliations.
University of Luxembourg, Luxembourg, Luxembourg
Christopher Hansen
Leibniz Institute for Psychology (ZPID), Trier, Germany
Holger Steinmetz
Trier University, Trier, Germany
Erasmus University Rotterdam, Rotterdam, The Netherlands
Wittener Institut Für Familienunternehmen, Universität Witten/Herdecke, Witten, Germany
You can also search for this author in PubMed Google Scholar
Corresponding author
Correspondence to Jörn Block .
Ethics declarations
Conflict of interest.
The authors have no relevant financial or non-financial interests to disclose.
Additional information
Publisher's note.
Springer Nature remains neutral with regard to jurisdictional claims in published maps and institutional affiliations.
See Table 1 .
Rights and permissions
Open Access This article is licensed under a Creative Commons Attribution 4.0 International License, which permits use, sharing, adaptation, distribution and reproduction in any medium or format, as long as you give appropriate credit to the original author(s) and the source, provide a link to the Creative Commons licence, and indicate if changes were made. The images or other third party material in this article are included in the article's Creative Commons licence, unless indicated otherwise in a credit line to the material. If material is not included in the article's Creative Commons licence and your intended use is not permitted by statutory regulation or exceeds the permitted use, you will need to obtain permission directly from the copyright holder. To view a copy of this licence, visit http://creativecommons.org/licenses/by/4.0/ .
Reprints and permissions
About this article
Hansen, C., Steinmetz, H. & Block, J. How to conduct a meta-analysis in eight steps: a practical guide. Manag Rev Q 72 , 1–19 (2022). https://doi.org/10.1007/s11301-021-00247-4
Download citation
Published : 30 November 2021
Issue Date : February 2022
DOI : https://doi.org/10.1007/s11301-021-00247-4
Share this article
Anyone you share the following link with will be able to read this content:
Sorry, a shareable link is not currently available for this article.
Provided by the Springer Nature SharedIt content-sharing initiative
- Find a journal
- Publish with us
- Track your research

Meta-analysis
Reviewed by Psychology Today Staff
Meta-analysis is an objective examination of published data from many studies of the same research topic identified through a literature search. Through the use of rigorous statistical methods, it can reveal patterns hidden in individual studies and can yield conclusions that have a high degree of reliability. It is a method of analysis that is especially useful for gaining an understanding of complex phenomena when independent studies have produced conflicting findings.
Meta-analysis provides much of the underpinning for evidence-based medicine. It is particularly helpful in identifying risk factors for a disorder, diagnostic criteria, and the effects of treatments on specific populations of people, as well as quantifying the size of the effects. Meta-analysis is well-suited to understanding the complexities of human behavior.
- How Does It Differ From Other Studies?
- When Is It Used?
- What Are Some Important Things Revealed by Meta-analysis?
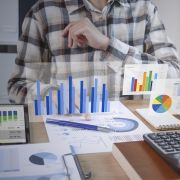
There are well-established scientific criteria for selecting studies for meta-analysis. Usually, meta-analysis is conducted on the gold standard of scientific research—randomized, controlled, double-blind trials. In addition, published guidelines not only describe standards for the inclusion of studies to be analyzed but also rank the quality of different types of studies. For example, cohort studies are likely to provide more reliable information than case reports.
Through statistical methods applied to the original data collected in the included studies, meta-analysis can account for and overcome many differences in the way the studies were conducted, such as the populations studied, how interventions were administered, and what outcomes were assessed and how. Meta-analyses, and the questions they are attempting to answer, are typically specified and registered with a scientific organization, and, with the protocols and methods openly described and reviewed independently by outside investigators, the research process is highly transparent.
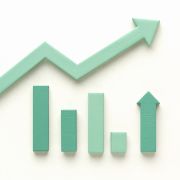
Meta-analysis is often used to validate observed phenomena, determine the conditions under which effects occur, and get enough clarity in clinical decision-making to indicate a course of therapeutic action when individual studies have produced disparate findings. In reviewing the aggregate results of well-controlled studies meeting criteria for inclusion, meta-analysis can also reveal which research questions, test conditions, and research methods yield the most reliable results, not only providing findings of immediate clinical utility but furthering science.
The technique can be used to answer social and behavioral questions large and small. For example, to clarify whether or not having more options makes it harder for people to settle on any one item, a meta-analysis of over 53 conflicting studies on the phenomenon was conducted. The meta-analysis revealed that choice overload exists—but only under certain conditions. You will have difficulty selecting a TV show to watch from the massive array of possibilities, for example, if the shows differ from each other in multiple ways or if you don’t have any strong preferences when you finally get to sit down in front of the TV.
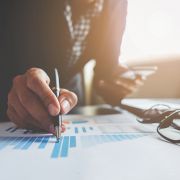
A meta-analysis conducted in 2000, for example, answered the question of whether physically attractive people have “better” personalities . Among other traits, they prove to be more extroverted and have more social skills than others. Another meta-analysis, in 2014, showed strong ties between physical attractiveness as rated by others and having good mental and physical health. The effects on such personality factors as extraversion are too small to reliably show up in individual studies but real enough to be detected in the aggregate number of study participants. Together, the studies validate hypotheses put forth by evolutionary psychologists that physical attractiveness is important in mate selection because it is a reliable cue of health and, likely, fertility.
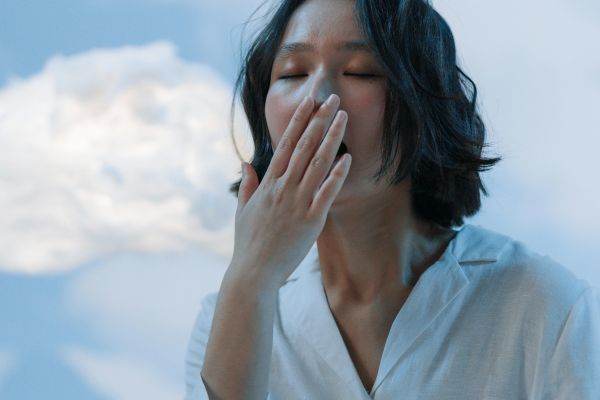
The replication crisis, publication bias, and careerism undermine scientific rigor and ethical responsibility in counseling to provide effective and safe care for clients.
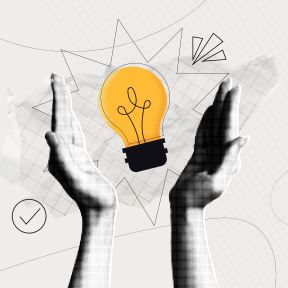
The human brain has two halves. A new study highlights when differences between them start.
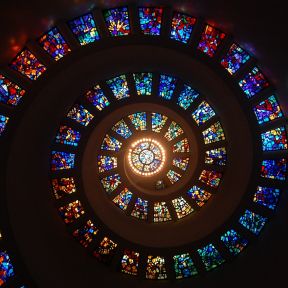
When considered across a lifetime, no within-person association exists between religiosity and psychological well-being.
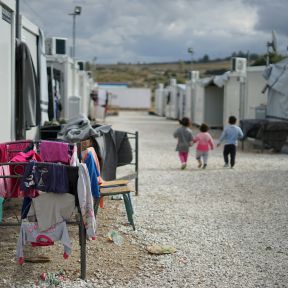
What are the prevalence rates of psychosis for displaced refugees?
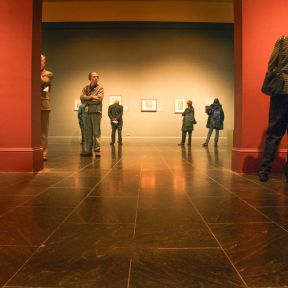
A recent review provides compelling evidence that arts engagement significantly reduces cognitive decline and enhances the quality of life among healthy older adults.
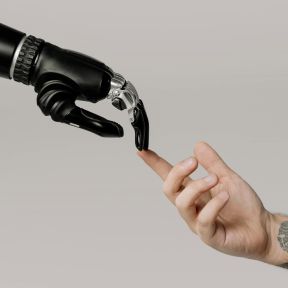
Personal Perspective: Mental healthcare AI is evolving beyond administrative roles. By automating routine tasks, therapists can spend sessions focusing on human interactions.
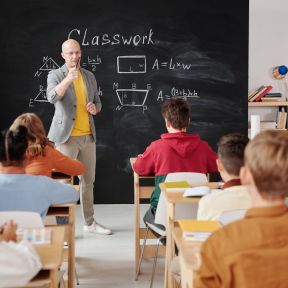
Investing in building a positive classroom climate holds benefits for students and teachers alike.
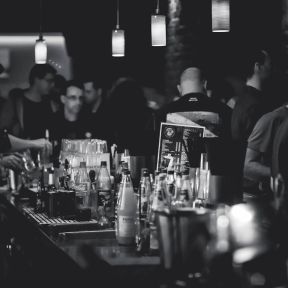
Mistakenly blaming cancer-causing chemicals and radiation for most cancers lets us avoid the simple lifestyle changes that could protect us from cancer far more.
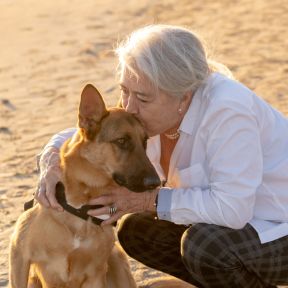
According to astronomer Carl Sagan, "Extraordinary claims require extraordinary evidence." Does the claim that pet owners live longer pass the extraordinary evidence requirement?
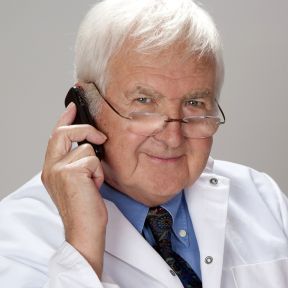
People, including leading politicians, are working later in life than ever before. Luckily, social science suggests that aging does not get in the way of job performance.
- Find a Therapist
- Find a Treatment Center
- Find a Psychiatrist
- Find a Support Group
- Find Online Therapy
- United States
- Brooklyn, NY
- Chicago, IL
- Houston, TX
- Los Angeles, CA
- New York, NY
- Portland, OR
- San Diego, CA
- San Francisco, CA
- Seattle, WA
- Washington, DC
- Asperger's
- Bipolar Disorder
- Chronic Pain
- Eating Disorders
- Passive Aggression
- Personality
- Goal Setting
- Positive Psychology
- Stopping Smoking
- Low Sexual Desire
- Relationships
- Child Development
- Self Tests NEW
- Therapy Center
- Diagnosis Dictionary
- Types of Therapy
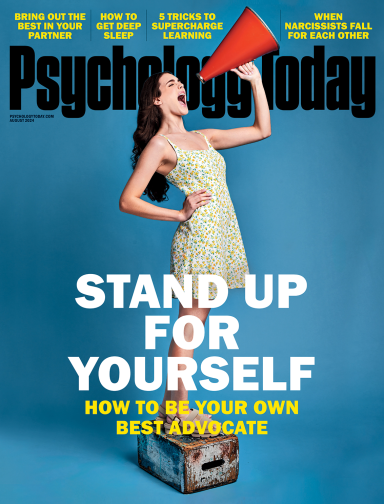
Sticking up for yourself is no easy task. But there are concrete skills you can use to hone your assertiveness and advocate for yourself.
- Emotional Intelligence
- Gaslighting
- Affective Forecasting
- Neuroscience

An official website of the United States government
The .gov means it’s official. Federal government websites often end in .gov or .mil. Before sharing sensitive information, make sure you’re on a federal government site.
The site is secure. The https:// ensures that you are connecting to the official website and that any information you provide is encrypted and transmitted securely.
- Publications
- Account settings
Preview improvements coming to the PMC website in October 2024. Learn More or Try it out now .
- Advanced Search
- Journal List
- Wiley Open Access Collection

A brief introduction of meta‐analyses in clinical practice and research
Xiao‐meng wang.
1 Department of Epidemiology, School of Public Health, Southern Medical University, Guangzhou Guangdong, China
Xi‐Ru Zhang
Zhi‐hao li, wen‐fang zhong, associated data.
Data sharing is not applicable to this article because no datasets were generated or analyzed during the current study.
With the explosive growth of medical information, it is almost impossible for healthcare providers to review and evaluate all relevant evidence to make the best clinical decisions. Meta‐analyses, which summarize all existing evidence and quantitatively synthesize individual studies, have become the best available evidence for informing clinical practice. This article introduces the common methods, steps, principles, strengths and limitations of meta‐analyses and aims to help healthcare providers and researchers obtain a basic understanding of meta‐analyses in clinical practice and research.
This article introduces the common methods, principles, steps, strengths and limitations of meta‐analyses and aims to help clinicians and researchers obtain a basic understanding of meta‐analyses in clinical practice and research.

1. INTRODUCTION
With the explosive growth of medical information, it has become almost impossible for healthcare providers to review and evaluate all related evidence to inform their decision making. 1 , 2 Furthermore, the inconsistent and often even conflicting conclusions of different studies can confuse these individuals. Systematic reviews were developed to resolve such situations, which comprehensively and systematically summarize all relevant empirical evidence. 3 Many systematic reviews contain meta‐analysis, which use statistical methods to combine the results of individual studies. 4 Through meta‐analyses, researchers can objectively and quantitatively synthesize results from different studies and increase the statistical strength and precision for estimating effects. 5 In the late 1970s, meta‐analysis began to appear regularly in the medical literature. 6 Subsequently, a plethora of meta‐analyses have emerged and the growth is exponential over time. 7 When conducted properly, a meta‐analysis of medical studies is considered as decisive evidence because it occupies a top level in the hierarchy of evidence. 8
An understanding of the principles, performance, advantages and weaknesses of meta‐analyses is important. Therefore, we aim to provide a basic understanding of meta‐analyses for clinicians and researchers in the present article by introducing the common methods, principles, steps, strengths and limitations of meta‐analyses.
2. COMMON META‐ANALYSIS METHODS
There are many types of meta‐analysis methods (Table 1 ). In this article, we mainly introduce five meta‐analysis methods commonly used in clinical practice.
Meta‐analysis methods
Methods | Definitions |
---|---|
Aggregate data meta‐analysis | Extracting summary results of studies available in published accounts |
Individual participant data meta‐analysis | Collecting individual participant‐level data from original studies |
Cumulative meta‐analysis | Adding studies to a meta‐analysis based on a predetermined order |
Network meta‐analysis | Combining direct and indirect evidence to compare the effectiveness between different interventions |
Meta‐analysis of diagnostic test accuracy | Identifying and synthesizing evidence on the accuracy of tests |
Prospective meta‐analysis | Conducting meta‐analysis for studies that specify research selection criteria, hypotheses and analysis, but for which the results are not yet known |
Sequential meta‐analysis | Combining the methodology of cumulative meta‐analysis with the technique of formal sequential testing, which can sequentially evaluate the available evidence at consecutive interim steps during the data collection |
Meta‐analysis of the adverse events | Following the basic meta‐analysis principles to analyze the incidences of adverse events of studies |
2.1. Aggregated data meta‐analysis
Although more information can be obtained based on individual participant‐level data from original studies, it is usually impossible to obtain these data from all included studies in meta‐analysis because such data may have been corrupted, or the main investigator may no longer be contacted or refuse to release the data. Therefore, by extracting summary results of studies available in published accounts, an aggregate data meta‐analysis (AD‐MA) is the most commonly used of all the quantitative approaches. 9 A study has found that > 95% of published meta‐analyses were AD‐MA. 10 In addition, AD‐MA is the mainstay of systematic reviews conducted by the US Preventive Services Task Force, the Cochrane Collaboration and many professional societies. 9 Moreover, AD‐MA can be completed relatively quickly at a low cost, and the data are relatively easy to obtain. 11 , 12 However, AD‐MA has very limited control over the data. A challenge with AD‐MA is that the association between an individual participant‐level covariate and the effect of the interventions at the study level may not reflect the individual‐level effect modification of that covariate. 13 It is also difficult to extract sufficient compatible data to undertake meaningful subgroup analyses in AD‐MA. 14 Furthermore, AD‐MA is prone to ecological bias, as well as to confounding from variables not included in the model, and may have limited power. 15
2.2. Individual participant data meta‐analysis
An individual participant data meta‐analysis (IPD‐MA) is considered the “gold standard” for meta‐analysis; this type of analysis collects individual participant‐level data from original studies. 15 Compared with AD‐MA, IPD‐MA has many advantages, including improved data quality, a greater variety of analytical types that can be performed and the ability to obtain more reliable results. 16 , 17
It is crucial to maintain clusters of participants within studies in the statistical implementation of an IPD‐MA. Clusters can be retained during the analysis using a one‐step or two‐step approach. 18 In the one‐step approach, the individual participant data from all studies are modeled simultaneously, at the same time as accounting for the clustering of participants within studies. 19 This approach requires a model specific to the type of data being synthesized and an appropriate account of the meta‐analysis assumptions (e.g. fixed or random effects across studies). Cheng et al . 20 proposed using a one‐step IPD‐MA to handle binary rare events and found that this method was superior to traditional methods of inverse variance, the Mantel–Haenszel method and the Yusuf‐Peto method. In the two‐step approach, the individual participant data from each study are analyzed independently for each separate study to produce aggregate data for each study (e.g. a mean treatment effect estimate and its standard error) using a statistical method appropriate for the type of data being analyzed (e.g. a linear regression model might be fitted for continuous responses, or a Cox regression might be applied for time‐to‐event data). The aggregate data are then combined to obtain an summary effect in the second step using a suitable model, such as weighting studies by the inverse of the variance. 21 For example, using a two‐step IPD‐MA, Grams et al . 22 found that apolipoprotein‐L1 kidney‐risk variants were not associated with incident cardiovascular disease or death independent of kidney measures.
Compared to the two‐step approach, the one‐step IPD‐MA is recommended for small meta‐analyses 23 and, conveniently, must only specify one model; however, this requires careful distinction of within‐study and between‐study variability. 24 The two‐step IPD‐MA is more laborious, although it allows the use of traditional, well‐known meta‐analysis techniques in the second step, such as those used by the Cochrane Collaboration (e.g. the Mantel–Haenszel method).
2.3. Cumulative meta‐analysis
Meta‐analyses are traditionally used retrospectively to review existing evidence. However, current evidence often undergoes several updates as new studies become available. Thus, updated data must be continuously obtained to simplify and digest the ever‐expanding literature. Therefore, cumulative meta‐analysis was developed, which adds studies to a meta‐analysis based on a predetermined order and then tracks the magnitude of the mean effect and its variance. 25 A cumulative meta‐analysis can be performed multiple times; not only can it obtain summary results and provide a comparison of the dynamic results, but also it can assess the impact of newly added studies on the overall conclusions. 26 For example, initial observational studies and systematic reviews and meta‐analyses suggested that frozen embryo transfer was better for mothers and babies; however, recent primary studies have begun to challenge these conclusions. 27 Maheshwari et al . 27 therefore conducted a cumulative meta‐analysis to investigate whether these conclusions have remained consistent over time and found that the decreased risks of harmful outcomes associated with pregnancies conceived from frozen embryos have been consistent in terms of direction and magnitude of effect over several years, with an increasing precision around the point estimates. Furthermore, continuously updated cumulative meta‐analyses may avoid unnecessary large‐scale randomized controlled trials (RCTs) and prevent wasted research efforts. 28
2.4. Network meta‐analysis
Although RCTs can directly compare the effectiveness of interventions, most of them compare the effectiveness of an intervention with a placebo, and there is almost no direct comparison between different interventions. 29 , 30 Network meta‐analyses comprise a relatively recent development that combines direct and indirect evidence to compare the effectiveness between different interventions. 31 Evidence obtained from RCTs is considered as direct evidence, whereas evidence obtained through one or more common comparators is considered as indirect evidence. For example, when comparing interventions A and C, direct evidence refers to the estimate of the relative effects between A and C. When no RCTs have directly compared interventions A and C, these interventions can be compared indirectly if both have been compared with B (placebo or some standard treatments) in other studies (forming an A–B–C “loop” of evidence). 32 , 33
A valid network meta‐analysis can correctly combine the relative effects of more than two studies and obtain a consistent estimate of the relative effectiveness of all interventions in one analysis. 34 This meta‐analysis may lead to a greater accuracy of estimating intervention effectiveness and the ability to compare all available interventions to calculate the rank of different interventions. 34 , 35 For example, phosphodiesterase type 5 inhibitors (PDE5‐Is) are the first‐line therapy for erectile dysfunction, although there are limited available studies on the comparative effects of different types of PDE5‐Is. 36 Using a network meta‐analysis, Yuan et al . 36 calculated the absolute effects and the relative rank of different PDE5‐Is to provide an overview of the effectiveness and safety of all PDE5‐Is.
Notably, a network meta‐analysis should satisfy the transitivity assumption, in which there are no systematic differences between the available comparisons other than the interventions being compared 37 ; in other words, the participants could be randomized to any of the interventions in a hypothetical RCT consisting of all the interventions included in the network meta‐analysis.
2.5. Meta‐analysis of diagnostic test accuracy
Sensitivity and specificity are commonly used to assess diagnostic accuracy. However, diagnostic tests in clinical practice are rarely 100% specific or sensitive. 38 It is difficult to obtain accurate estimates of sensitivity and specificity in small diagnostic accuracy studies. 39 , 40 Even in a large sample size study, the number of cases may still be small as a result of the low prevalence. By identifying and synthesizing evidence on the accuracy of tests, the meta‐analysis of diagnostic test accuracy (DTA) provides insight into the ability of medical tests to detect the target diseases 41 ; it also can provide estimates of test performance, allow comparisons of the accuracy of different tests and facilitate the identification of sources of variability. 42 For example, the FilmArray® (Biomerieux, Marcy‐l'Étoile, France) meningitis/encephalitis (ME) panel can detect the most common pathogens in central nervous system infections, although reports of false positives and false negatives are confusing. 43 Based on meta‐analysis of DTA, Tansarli et al . 43 calculated that the sensitivity and specificity of the ME panel were both > 90%, indicating that the ME panel has high diagnostic accuracy.
3. HOW TO PERFORM A META‐ANALYSIS
3.1. frame a question.
Researchers must formulate an appropriate research question at the beginning. A well‐formulated question will guide many aspects of the review process, including determining eligibility criteria, searching for studies, collecting data from included studies, structuring the syntheses and presenting results. 44 There are some tools that may facilitate the construction of research questions, including PICO, as used in clinical practice 45 ; PEO and SPICE, as used for qualitative research questions 46 , 47 ; and SPIDER, as used for mixed‐methods research. 48
3.2. Form the search strategy
It is crucial for researchers to formulate a search strategy in advance that includes inclusion and exclusion criteria, as well as a standardized data extraction form. The definition of inclusion and exclusion criteria depends on established question elements, such as publication dates, research design, population and results. A reasonable inclusion and exclusion criteria will reduce the risk of bias, increase transparency and make the review systematic. Broad criteria may increase the heterogeneity between studies, and narrow criteria may make it difficult to find studies; therefore, a compromise should be found. 49
3.3. Search of the literature databases
To minimize bias and reduce hampered interpretation of outcomes, the search strategy should be as comprehensive as possible, employing multiple databases, such as PubMed, Embase, Cochrane Central Registry of Controlled Trials, Scopus, Web of Science and Google Scholar. 50 , 51 Removing language restrictions and actively searching for non‐English bibliographic databases may also help researchers to perform a comprehensive meta‐analysis. 52
3.4. Select the articles
The selection or rejection of the included articles should be guided by the criteria. 53 Two independent reviewers may screen the included articles, and any disagreements should be resolved by consensus through discussion. First, the titles and abstracts of all relevant searched papers should be read, and inclusion or exclusion criteria applied to determine whether these papers meet. Then, the full texts of the included articles should be reviewed once more to perform the rejection again. Finally, the reference lists of these articles should be searched to widen the research as much as possible. 54
3.5. Data extraction
A pre‐formed standardized data extraction form should be used to extract data of included studies. All data should be carefully converted using uniform standards. Simultaneous extraction by multiple researchers might also make the extracted data more accurate.
3.6. Assess quality of articles
Checklists and scales are often used to assess the quality of articles. For example, the Cochrane Collaboration's tool 55 is usually used to assess the quality of RCTs, whereas the Newcastle Ottawa Scale 56 is one of the most common method to assess the quality of non‐randomized trials. In addition, Quality Assessment of Diagnostic Accuracy Studies 2 57 is often used to evaluate the quality of diagnostic accuracy studies.
3.7. Test for heterogeneity
Several methods have been proposed to detect and quantify heterogeneity, such as Cochran's Q and I 2 values. Cochran's Q test is used to determine whether there is heterogeneity in primary studies or whether the variation observed is due to chance, 58 but it may be underpowered because of the inclusion of a small number of studies or low event rates. 59 Therefore, p < 0.10 (not 0.05) indicates the presence of heterogeneity given the low statistical strength and insensitivity of Cochran's Q test. 60 Another common method for testing heterogeneity is the I 2 value, which describes the percentage of variation across studies that is attributable to heterogeneity rather than chance; this value does not depend on the number of studies. 61 I 2 values of 25%, 50% and 75% are considered to indicate low, moderate and high heterogeneity, respectively. 60
3.8. Estimate the summary effect
Fixed effects and random effects models are commonly used to estimate the summary effect in a meta‐analysis. 62 Fixed effects models, which consider the variability of the results as “random variation”, simply weight individual studies by their precision (inverse of the variance). Conversely, random effects models assume a different underlying effect for each study and consider this an additional source of variation that is randomly distributed. A substantial difference in the summary effect calculated by fixed effects models and random effects models will be observed only if the studies are markedly heterogeneous (heterogeneity p < 0.10) and the random effects model typically provides wider confidence intervals than the fixed effect model. 63 , 64
3.9. Evaluate sources of heterogeneity
Several methods have been proposed to explore the possible reasons for heterogeneity. According to factors such as ethnicity, the number of studies or clinical features, subgroup analyses can be performed that divide the total data into several groups to assess the impact of a potential source of heterogeneity. Sensitivity analysis is a common approach for examining the sources of heterogeneity on a case‐by‐case basis. 65 In sensitivity analysis, one or more studies are excluded at a time and the impact of removing each or several studies is evaluated on the summary results and the between‐study heterogeneity. Sequential and combinatorial algorithms are usually implemented to evaluate the change in between‐study heterogeneity as one or more studies are excluded from the calculations. 66 Moreover, a meta‐regression model can explain heterogeneity based on study‐level covariates. 67
3.10. Assess publication bias
A funnel plot is a scatterplot that is commonly used to assess publication bias. In a funnel plot, the x ‐axis indicates the study effect and the y ‐axis indicates the study precision, such as the standard error or sample size. 68 , 69 If there is no publication bias, the plot will have a symmetrical inverted funnel; conversely, asymmetry indicates the possibility of publication bias.
3.11. Present results
A forest plot is a valid and useful tool for summarizing the results of a meta‐analysis. In a forest plot, the results from each individual study are shown as a blob or square; the confidence interval, usually representing 95% confidence, is shown as a horizontal line that passes through the square; and the summary effect is shown as a diamond. 70
4. PRINCIPLES OF META‐ANALYSIS PERFORMANCE
There are four most important principles of meta‐analysis performance that should be emphasized. First, the search scope of meta‐analysis should be expanded as much as possible to contain all relevant research, and it is important to remove language restrictions and actively search for non‐English bibliographic databases. Second, any meta‐analysis should include studies selected based on strict criteria established in advance. Third, appropriate tools must be selected to evaluate the quality of evidence according to different types of primary studies. Fourth, the most suitable statistical model should be chosen for the meta‐analysis and a weighted mean estimate of the effect size should be calculated. Finally, the possible causes of heterogeneity should be identified and publication bias in the meta‐analysis must be assessed.
5. STRENGTHS OF META‐ANALYSIS
Meta‐analyses have several strengths. First, a major advantage is their ability to improve the precision of effect estimates with considerably increased statistical power, which is particularly important when the power of the primary study is limited as a result of the small sample size. Second, a meta‐analysis has more power to detect small but clinically significant effects and to examine the effectiveness of interventions in demographic or clinical subgroups of participants, which can help researchers identify beneficial (or harmful) effects in specific groups of patients. 71 , 72 Third, meta‐analyses can be used to analyze rare outcomes and outcomes that individual studies were not designed to test (e.g. adverse events). Fourth, meta‐analyses can be used to examine heterogeneity in study results and explore possible sources in case this heterogeneity would lead to bias from “mixing apples and oranges”. 73 Furthermore, meta‐analyses can compare the effectiveness of various interventions, supplement the existing evidence, and then offer a rational and helpful way of addressing a series of practical difficulties that plague healthcare providers and researchers. Lastly, meta‐analyses may resolve disputes caused by apparently conflicting studies, determine whether new studies are necessary for further investigation and generate new hypotheses for future studies. 7 , 74
6. LIMITATIONS OF META‐ANALYSIS
6.1. missing related research.
The primary limitation of a meta‐analysis is missing related research. Even in the ideal case in which all relevant studies are available, a faulty search strategy can miss some of these studies. Small differences in search strategies can produce large differences in the set of studies found. 75 When searching databases, relevant research can be missed as a result of the omission of keywords. The search engine (e.g. PubMed, Google) may also affect the type and number of studies that are found. 76 Moreover, it may be impossible to identify all relevant evidence if the search scope is limited to one or two databases. 51 , 77 Finally, language restrictions and the failure to search non‐English bibliographic databases may also lead to an incomplete meta‐analysis. 52 Comprehensive search strategies for different databases and languages might help solve this issue.
6.2. Publication bias
Publication bias means that positive findings are more likely to be published and then identified through literature searches rather than ambiguous or negative findings. 78 This is an important and key source of bias that is recognized as a potential threat to the validity of results. 79 The real research effect may be exaggerated or even falsely positive if only published articles are included. 80 For example, based on studies registered with the US Food and Drug Administration, Turner et al . 81 reviewed 74 trials of 12 antidepressants to assess publication bias and its influence on apparent efficacy. It was found that antidepressant studies with favorable outcomes were 16 times more likely to be published than those with unfavorable outcomes, and the apparent efficacy of antidepressants increased between 11% and 69% when the non‐published studies were not included in the analysis. 81 Moreover, failing to identify and include non‐English language studies may also increase publication bias. 82 Therefore, all relevant studies should be identified to reduce the impact of publication bias on meta‐analysis.
6.3. Selection bias
Because many of the studies identified are not directly related to the subject of the meta‐analysis, it is crucial for researchers to select which studies to include based on defined criteria. Failing to evaluate, select or reject relevant studies based on stricter criteria regarding the study quality may also increase the possibility of selection bias. Missing or inappropriate quality assessment tools may lead to the inclusion of low‐quality studies. If a meta‐analysis includes low‐quality studies, its results will be biased and incorrect, which is also called “garbage in, garbage out”. 83 Strictly defined criteria for included studies and scoring by at least two researchers might help reduce the possibility of selection bias. 84 , 85
6.4. Unavailability of information
The best‐case scenario for meta‐analyses is the availability of individual participant data. However, most individual research reports only contain summary results, such as the mean, standard deviation, proportions, relative risk and odds ratio. In addition to the possibility of reporting errors, the lack of information can severely limit the types of analyses and conclusions that can be achieved in a meta‐analysis. For example, the unavailability of information from individual studies may preclude the comparison of effects in predetermined subgroups of participants. Therefore, if feasible, the researchers could contact the author of the primary study for individual participant data.
6.5. Heterogeneity
Although the studies included in a meta‐analysis have the same research hypothesis, there is still the potential for several areas of heterogeneity. 86 Heterogeneity may exist in various parts of the studies’ design and conduct, including participant selection, interventions/exposures or outcomes studied, data collection, data analyses and selective reporting of results. 87 Although the difference of the results can be overcome by assessing the heterogeneity of the studies and performing subgroup analyses, 88 the results of the meta‐analysis may become meaningless and even may obscure the real effect if the selected studies are too heterogeneous to be comparable. For example, Nicolucci et al . 89 conducted a review of 150 published randomized trials on the treatment of lung cancer. Their review showed serious methodological drawbacks and concluded that heterogeneity made the meta‐analysis of existing trials unlikely to be constructive. 89 Therefore, combining the data in meta‐analysis for studies with large heterogeneity is not recommended.
6.6. Misleading funnel plot
Funnel plots are appealing because they are a simple technique used to investigate the possibility of publication bias. However, their objective is to detect a complex effect, which can be misleading. For example, the lack of symmetry in a funnel plot can also be caused by heterogeneity. 90 Another problem with funnel plots is the difficulty of interpreting them when few studies are included. Readers may also be misled by the choice of axes or the outcome measure. 91 Therefore, in the absence of a consensus on how the plot should be constructed, asymmetrical funnel plots should be interpreted cautiously. 91
6.7. Inevitable subjectivity
Researchers must make numerous judgments when performing meta‐analyses, 92 which inevitably introduces considerable subjectivity into the meta‐analysis review process. For example, there is often a certain amount of subjectivity when deciding how similar studies should be before it is appropriate to combine them. To minimize subjectivity, at least two researchers should jointly conduct a meta‐analysis and reach a consensus.
The explosion of medical information and differences between individual studies make it almost impossible for healthcare providers to make the best clinical decisions. Meta‐analyses, which summarize all eligible evidence and quantitatively synthesize individual results on a specific clinical question, have become the best available evidence for informing clinical practice and are increasingly important in medical research. This article has described the basic concept, common methods, principles, steps, strengths and limitations of meta‐analyses to help clinicians and investigators better understand meta‐analyses and make clinical decisions based on the best evidence.
AUTHOR CONTRIBUTIONS
CM designed and directed the study. XMW and XRZ had primary responsibility for drafting the manuscript. CM, ZHL, WFZ and PY provided insightful discussions and suggestions. All authors critically reviewed the manuscript for important intellectual content.
CONFLICT OF INTEREST STATEMENT
The authors declare that they have no conflicts of interest.
ACKNOWLEDGEMENTS
This work was supported by the Project Supported by Guangdong Province Universities and Colleges Pearl River Scholar Funded Scheme (2019 to CM) and the Construction of High‐level University of Guangdong (G820332010, G618339167 and G618339164 to CM). The funders played no role in the study design or implementation; manuscript preparation, review or approval; or the decision to submit the manuscript for publication.
Wang X‐M, Zhang X‐R, Li Z‐H, Zhong W‐F, Yang P, Mao C. A brief introduction of meta‐analyses in clinical practice and research . J Gene Med . 2021; 23 :e3312. 10.1002/jgm.3312 [ PMC free article ] [ PubMed ] [ CrossRef ] [ Google Scholar ]
Xiao‐Meng Wang and Xi‐Ru Zhang contributed equally to this work.
DATA AVAILABILITY STATEMENT
Log in using your username and password
- Search More Search for this keyword Advanced search
- Latest content
- Current issue
- Write for Us
- BMJ Journals
You are here
- Volume 16, Issue 1
What is meta-analysis?
- Article Text
- Article info
- Citation Tools
- Rapid Responses
- Article metrics

- Allison Shorten 1 ,
- Brett Shorten 2
- 1 School of Nursing , Yale University , New Haven, Connecticut , USA
- 2 Informed Health Choices Trust, Wollongong, New South Wales, Australia
- Correspondence to : Dr Allison Shorten Yale University School of Nursing, 100 Church Street South, PO Box 9740, New Haven, CT 06536, USA; allison.shorten{at}yale.edu
https://doi.org/10.1136/eb-2012-101118
Statistics from Altmetric.com
Request permissions.
If you wish to reuse any or all of this article please use the link below which will take you to the Copyright Clearance Center’s RightsLink service. You will be able to get a quick price and instant permission to reuse the content in many different ways.
When clinicians begin their search for the best available evidence to inform decision-making, they are usually directed to the top of the ‘evidence pyramid’ to find out whether a systematic review and meta-analysis have been conducted. The Cochrane Library 1 is fast filling with systematic reviews and meta-analyses that aim to answer important clinical questions and provide the most reliable evidence to inform practice and research. So what is meta-analysis and how can it contribute to practice?
The Five-step process
There is debate about the best practice for meta-analysis, however there are five common steps.
Step 1: the research question
A clinical research question is identified and a hypothesis proposed. The likely clinical significance is explained and the study design and analytical plan are justified.
Step 2: systematic review
A systematic review (SR) is specifically designed to address the research question and conducted to identify all studies considered to be both relevant and of sufficiently good quality to warrant inclusion. Often, only studies published in established journals are identified, but identification of ‘unpublished’ data is important to avoid ‘publication bias’ or exclusion of studies with negative findings. 4 Some meta-analyses only consider randomised control trials (RCTs) in the quest for highest quality evidence. Other types of ‘experimental’ and ‘quasi-experimental’ studies may be included if they satisfy the defined inclusion/exclusion criteria.
Step 3: data extraction
Once studies are selected for inclusion in the meta-analysis, summary data or outcomes are extracted from each study. In addition, sample sizes and measures of data variability for both intervention and control groups are required. Depending on the study and the research question, outcome measures could include numerical measures or categorical measures. For example, differences in scores on a questionnaire or differences in a measurement level such as blood pressure would be reported as a numerical mean. However, differences in the likelihood of being in one category versus another (eg, vaginal birth versus cesarean birth) are usually reported in terms of risk measures such as OR or relative risk (RR).
Step 4: standardisation and weighting studies
Having assembled all the necessary data, the fourth step is to calculate appropriate summary measures from each study for further analysis. These measures are usually called Effect Sizes and represent the difference in average scores between intervention and control groups. For example, the difference in change in blood pressure between study participants who used drug X compared with participants who used a placebo. Since units of measurement typically vary across included studies, they usually need to be ‘standardised’ in order to produce comparable estimates of this effect. When different outcome measures are used, such as when researchers use different tests, standardisation is imperative. Standardisation is achieved by taking, for each study, the mean score for the intervention group, subtracting the mean for the control group and dividing this result by the appropriate measure of variability in that data set.
The results of some studies need to carry more weight than others. Larger studies (as measured by sample sizes) are thought to produce more precise effect size estimates than smaller studies. Second, studies with less data variability, for example, smaller SD or narrower CIs are often regarded as ‘better quality’ in study design. A weighting statistic that seeks to incorporate both these factors, known as inverse variance , is commonly used.
Step 5: final estimates of effect
The final stage is to select and apply an appropriate model to compare Effect Sizes across different studies. The most common models used are Fixed Effects and Random Effects models. Fixed Effects models are based on the ‘assumption that every study is evaluating a common treatment effect’. 5 This means that the assumption is that all studies would estimate the same Effect Size were it not for different levels of sample variability across different studies. In contrast, the Random Effects model ‘assumes that the true treatment effects in the individual studies may be different from each other’. 5 and attempts to allow for this additional source of interstudy variation in Effect Sizes . Whether this latter source of variability is likely to be important is often assessed within the meta-analysis by testing for ‘heterogeneity’.
Forest plot
The final estimates from a meta-analysis are often graphically reported in the form of a ‘Forest Plot’.
In the hypothetical Forest Plot shown in figure 1 , for each study, a horizontal line indicates the standardised Effect Size estimate (the rectangular box in the centre of each line) and 95% CI for the risk ratio used. For each of the studies, drug X reduced the risk of death (the risk ratio is less than 1.0). However, the first study was larger than the other two (the size of the boxes represents the relative weights calculated by the meta-analysis). Perhaps, because of this, the estimates for the two smaller studies were not statistically significant (the lines emanating from their boxes include the value of 1). When all the three studies were combined in the meta-analysis, as represented by the diamond, we get a more precise estimate of the effect of the drug, where the diamond represents both the combined risk ratio estimate and the limits of the 95% CI.
- Download figure
- Open in new tab
- Download powerpoint
Hypothetical Forest Plot
Relevance to practice and research
Many Evidence Based Nursing commentaries feature recently published systematic review and meta-analysis because they not only bring new insight or strength to recommendations about the most effective healthcare practices but they also identify where future research should be directed to bridge the gaps or limitations in current evidence. The strength of conclusions from meta-analysis largely depends on the quality of the data available for synthesis. This reflects the quality of individual studies and the systematic review. Meta-analysis does not magically resolve the problem of underpowered or poorly designed studies and clinicians can be frustrated to find that even when a meta-analysis has been conducted, all that the researchers can conclude is that the evidence is weak, there is uncertainty about the effects of treatment and that higher quality research is needed to better inform practice. This is still an important finding and can inform our practice and challenge us to fill the evidence gaps with better quality research in the future.
- ↵ The Cochrane Library . http://www.thecochranelibrary.com/view/0/index.html (accessed 23 Oct 2012).
- Davey Smith G
- Davey Smoth G
- Higgins JPT ,
Competing interests None.
Read the full text or download the PDF:
- En español – ExME
- Em português – EME
Systematic reviews vs meta-analysis: what’s the difference?
Posted on 24th July 2023 by Verónica Tanco Tellechea
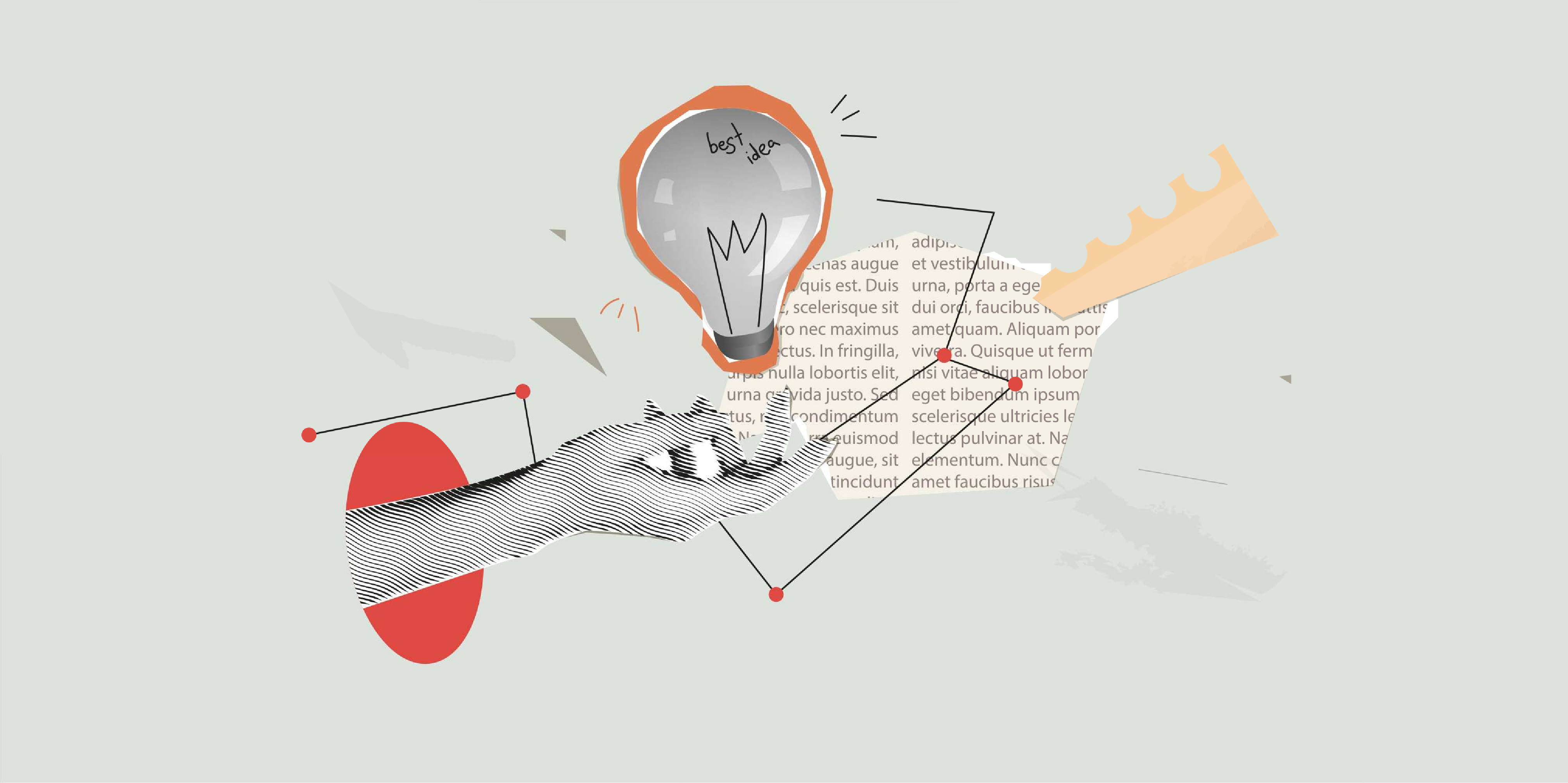
You may hear the terms ‘systematic review’ and ‘meta-analysis being used interchangeably’. Although they are related, they are distinctly different. Learn more in this blog for beginners.
What is a systematic review?
According to Cochrane (1), a systematic review attempts to identify, appraise and synthesize all the empirical evidence to answer a specific research question. Thus, a systematic review is where you might find the most relevant, adequate, and current information regarding a specific topic. In the levels of evidence pyramid , systematic reviews are only surpassed by meta-analyses.
To conduct a systematic review, you will need, among other things:
- A specific research question, usually in the form of a PICO question.
- Pre-specified eligibility criteria, to decide which articles will be included or discarded from the review.
- To follow a systematic method that will minimize bias.
You can find protocols that will guide you from both Cochrane and the Equator Network , among other places, and if you are a beginner to the topic then have a read of an overview about systematic reviews.
What is a meta-analysis?
A meta-analysis is a quantitative, epidemiological study design used to systematically assess the results of previous research (2) . Usually, they are based on randomized controlled trials, though not always. This means that a meta-analysis is a mathematical tool that allows researchers to mathematically combine outcomes from multiple studies.
When can a meta-analysis be implemented?
There is always the possibility of conducting a meta-analysis, yet, for it to throw the best possible results it should be performed when the studies included in the systematic review are of good quality, similar designs, and have similar outcome measures.
Why are meta-analyses important?
Outcomes from a meta-analysis may provide more precise information regarding the estimate of the effect of what is being studied because it merges outcomes from multiple studies. In a meta-analysis, data from various trials are combined and generate an average result (1), which is portrayed in a forest plot diagram. Moreover, meta-analysis also include a funnel plot diagram to visually detect publication bias.
Conclusions
A systematic review is an article that synthesizes available evidence on a certain topic utilizing a specific research question, pre-specified eligibility criteria for including articles, and a systematic method for its production. Whereas a meta-analysis is a quantitative, epidemiological study design used to assess the results of articles included in a systematic-review.
DEFINITION | Synthesis of empirical evidence regarding a specific research question | Statistical tool used with quantitative outcomes of various studies regarding a specific topic |
RESULTS | Synthesizes relevant and current information regarding a specific research question (qualitative). | Merges multiple outcomes from different researches and provides an average result (quantitative). |
Remember: All meta-analyses involve a systematic review, but not all systematic reviews involve a meta-analysis.
If you would like some further reading on this topic, we suggest the following:
The systematic review – a S4BE blog article
Meta-analysis: what, why, and how – a S4BE blog article
The difference between a systematic review and a meta-analysis – a blog article via Covidence
Systematic review vs meta-analysis: what’s the difference? A 5-minute video from Research Masterminds:
- About Cochrane reviews [Internet]. Cochranelibrary.com. [cited 2023 Apr 30]. Available from: https://www.cochranelibrary.com/about/about-cochrane-reviews
- Haidich AB. Meta-analysis in medical research. Hippokratia. 2010;14(Suppl 1):29–37.
Verónica Tanco Tellechea
Leave a reply cancel reply.
Your email address will not be published. Required fields are marked *
Save my name, email, and website in this browser for the next time I comment.
Subscribe to our newsletter
You will receive our monthly newsletter and free access to Trip Premium.
Related Articles
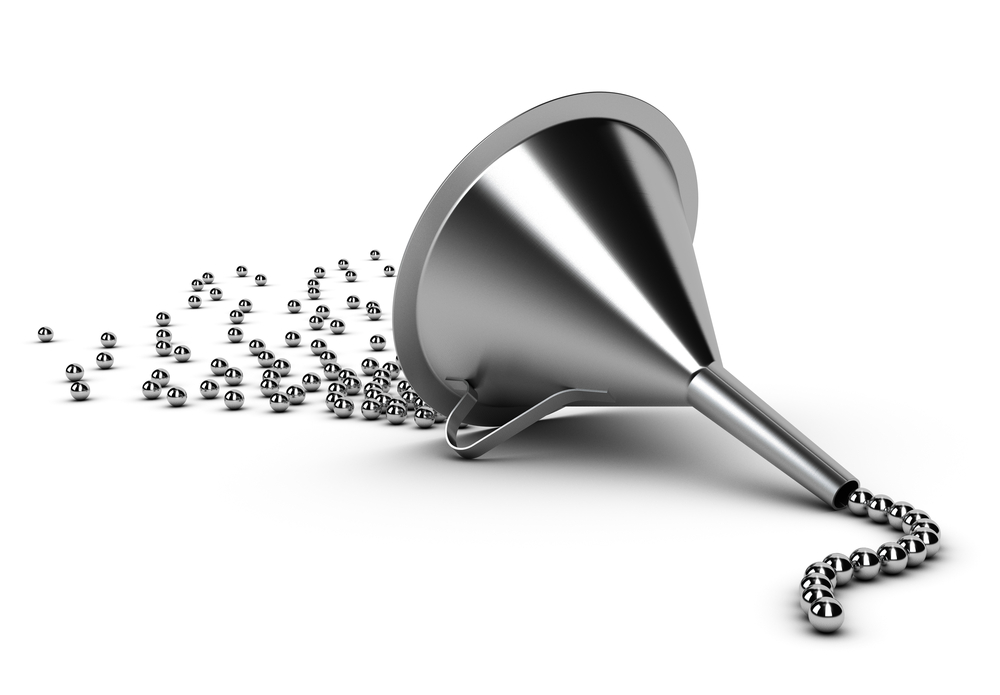
How to read a funnel plot
This blog introduces you to funnel plots, guiding you through how to read them and what may cause them to look asymmetrical.
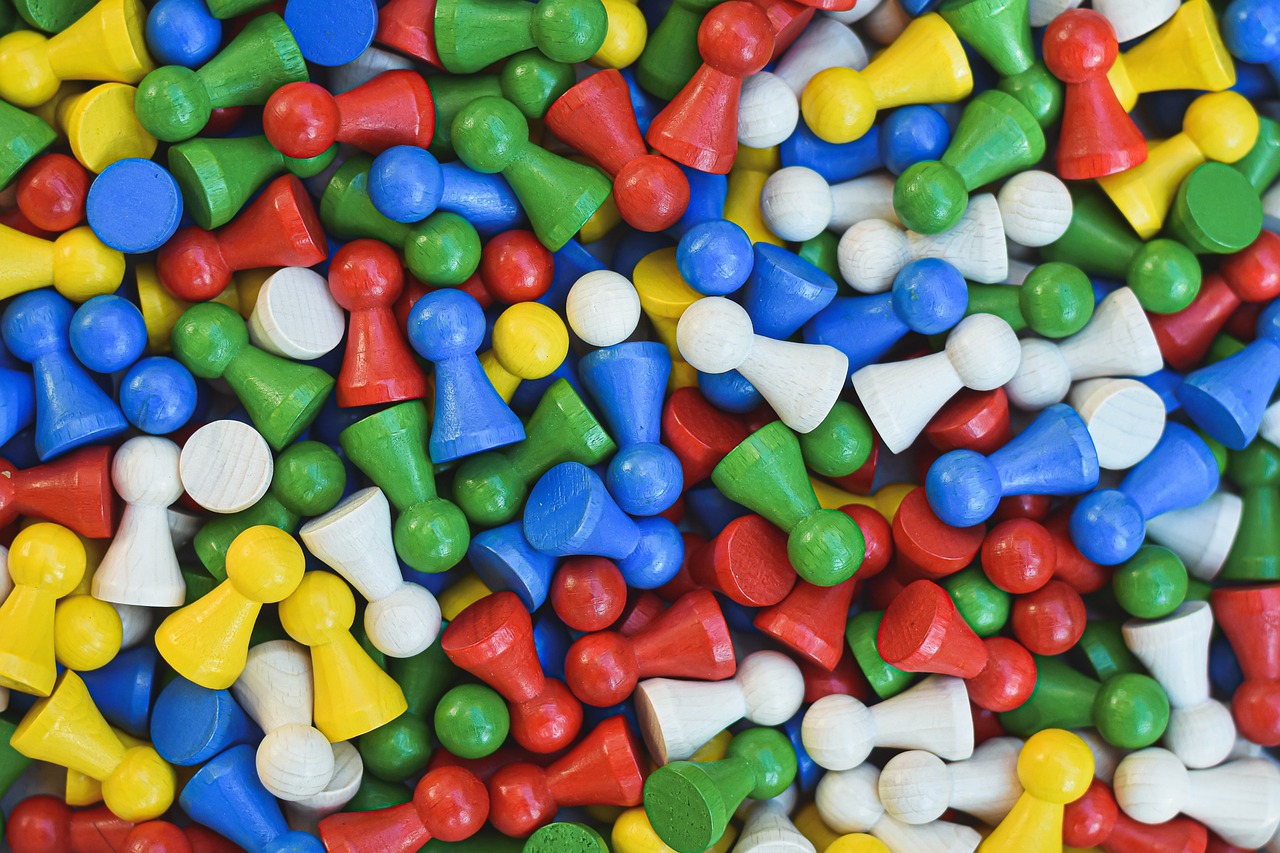
Heterogeneity in meta-analysis
When you bring studies together in a meta-analysis, one of the things you need to consider is the variability in your studies – this is called heterogeneity. This blog presents the three types of heterogeneity, considers the different types of outcome data, and delves a little more into dealing with the variations.
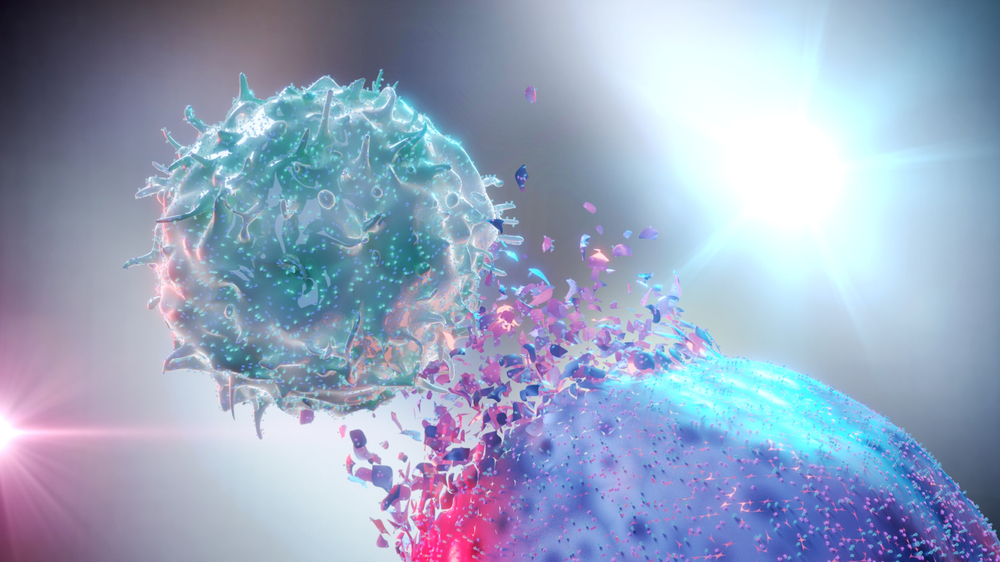
Natural killer cells in glioblastoma therapy
As seen in a previous blog from Davide, modern neuroscience often interfaces with other medical specialities. In this blog, he provides a summary of new evidence about the potential of a therapeutic strategy born at the crossroad between neurology, immunology and oncology.
- How it works
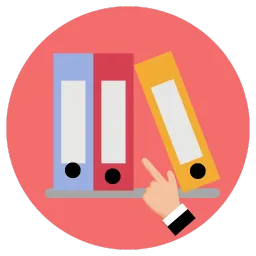
Meta-Analysis – Guide with Definition, Steps & Examples
Published by Owen Ingram at April 26th, 2023 , Revised On April 26, 2023
“A meta-analysis is a formal, epidemiological, quantitative study design that uses statistical methods to generalise the findings of the selected independent studies. “
Meta-analysis and systematic review are the two most authentic strategies in research. When researchers start looking for the best available evidence concerning their research work, they are advised to begin from the top of the evidence pyramid. The evidence available in the form of meta-analysis or systematic reviews addressing important questions is significant in academics because it informs decision-making.
What is Meta-Analysis
Meta-analysis estimates the absolute effect of individual independent research studies by systematically synthesising or merging the results. Meta-analysis isn’t only about achieving a wider population by combining several smaller studies. It involves systematic methods to evaluate the inconsistencies in participants, variability (also known as heterogeneity), and findings to check how sensitive their findings are to the selected systematic review protocol.
When Should you Conduct a Meta-Analysis?
Meta-analysis has become a widely-used research method in medical sciences and other fields of work for several reasons. The technique involves summarising the results of independent systematic review studies.
The Cochrane Handbook explains that “an important step in a systematic review is the thoughtful consideration of whether it is appropriate to combine the numerical results of all, or perhaps some, of the studies. Such a meta-analysis yields an overall statistic (together with its confidence interval) that summarizes the effectiveness of an experimental intervention compared with a comparator intervention” (section 10.2).
A researcher or a practitioner should choose meta-analysis when the following outcomes are desirable.
For generating new hypotheses or ending controversies resulting from different research studies. Quantifying and evaluating the variable results and identifying the extent of conflict in literature through meta-analysis is possible.
To find research gaps left unfilled and address questions not posed by individual studies. Primary research studies involve specific types of participants and interventions. A review of these studies with variable characteristics and methodologies can allow the researcher to gauge the consistency of findings across a wider range of participants and interventions. With the help of meta-analysis, the reasons for differences in the effect can also be explored.
To provide convincing evidence. Estimating the effects with a larger sample size and interventions can provide convincing evidence. Many academic studies are based on a very small dataset, so the estimated intervention effects in isolation are not fully reliable.
Elements of a Meta-Analysis
Deeks et al. (2019), Haidilch (2010), and Grant & Booth (2009) explored the characteristics, strengths, and weaknesses of conducting the meta-analysis. They are briefly explained below.
Characteristics:
- A systematic review must be completed before conducting the meta-analysis because it provides a summary of the findings of the individual studies synthesised.
- You can only conduct a meta-analysis by synthesising studies in a systematic review.
- The studies selected for statistical analysis for the purpose of meta-analysis should be similar in terms of comparison, intervention, and population.
Strengths:
- A meta-analysis takes place after the systematic review. The end product is a comprehensive quantitative analysis that is complicated but reliable.
- It gives more value and weightage to existing studies that do not hold practical value on their own.
- Policy-makers and academicians cannot base their decisions on individual research studies. Meta-analysis provides them with a complex and solid analysis of evidence to make informed decisions.
Criticisms:
- The meta-analysis uses studies exploring similar topics. Finding similar studies for the meta-analysis can be challenging.
- When and if biases in the individual studies or those related to reporting and specific research methodologies are involved, the meta-analysis results could be misleading.
Steps of Conducting the Meta-Analysis
The process of conducting the meta-analysis has remained a topic of debate among researchers and scientists. However, the following 5-step process is widely accepted.
Step 1: Research Question
The first step in conducting clinical research involves identifying a research question and proposing a hypothesis . The potential clinical significance of the research question is then explained, and the study design and analytical plan are justified.
Step 2: Systematic Review
The purpose of a systematic review (SR) is to address a research question by identifying all relevant studies that meet the required quality standards for inclusion. While established journals typically serve as the primary source for identified studies, it is important to also consider unpublished data to avoid publication bias or the exclusion of studies with negative results.
While some meta-analyses may limit their focus to randomized controlled trials (RCTs) for the sake of obtaining the highest quality evidence, other experimental and quasi-experimental studies may be included if they meet the specific inclusion/exclusion criteria established for the review.
Step 3: Data Extraction
After selecting studies for the meta-analysis, researchers extract summary data or outcomes, as well as sample sizes and measures of data variability for both intervention and control groups. The choice of outcome measures depends on the research question and the type of study, and may include numerical or categorical measures.
For instance, numerical means may be used to report differences in scores on a questionnaire or changes in a measurement, such as blood pressure. In contrast, risk measures like odds ratios (OR) or relative risks (RR) are typically used to report differences in the probability of belonging to one category or another, such as vaginal birth versus cesarean birth.
Step 4: Standardisation and Weighting Studies
After gathering all the required data, the fourth step involves computing suitable summary measures from each study for further examination. These measures are typically referred to as Effect Sizes and indicate the difference in average scores between the control and intervention groups. For instance, it could be the variation in blood pressure changes between study participants who used drug X and those who used a placebo.
Since the units of measurement often differ across the included studies, standardization is necessary to create comparable effect size estimates. Standardization is accomplished by determining, for each study, the average score for the intervention group, subtracting the average score for the control group, and dividing the result by the relevant measure of variability in that dataset.
In some cases, the results of certain studies must carry more significance than others. Larger studies, as measured by their sample sizes, are deemed to produce more precise estimates of effect size than smaller studies. Additionally, studies with less variability in data, such as smaller standard deviation or narrower confidence intervals, are typically regarded as higher quality in study design. A weighting statistic that aims to incorporate both of these factors, known as inverse variance, is commonly employed.
Step 5: Absolute Effect Estimation
The ultimate step in conducting a meta-analysis is to choose and utilize an appropriate model for comparing Effect Sizes among diverse studies. Two popular models for this purpose are the Fixed Effects and Random Effects models. The Fixed Effects model relies on the premise that each study is evaluating a common treatment effect, implying that all studies would have estimated the same Effect Size if sample variability were equal across all studies.
Conversely, the Random Effects model posits that the true treatment effects in individual studies may vary from each other, and endeavors to consider this additional source of interstudy variation in Effect Sizes. The existence and magnitude of this latter variability is usually evaluated within the meta-analysis through a test for ‘heterogeneity.’
Forest Plot
The results of a meta-analysis are often visually presented using a “Forest Plot”. This type of plot displays, for each study, included in the analysis, a horizontal line that indicates the standardized Effect Size estimate and 95% confidence interval for the risk ratio used. Figure A provides an example of a hypothetical Forest Plot in which drug X reduces the risk of death in all three studies.
However, the first study was larger than the other two, and as a result, the estimates for the smaller studies were not statistically significant. This is indicated by the lines emanating from their boxes, including the value of 1. The size of the boxes represents the relative weights assigned to each study by the meta-analysis. The combined estimate of the drug’s effect, represented by the diamond, provides a more precise estimate of the drug’s effect, with the diamond indicating both the combined risk ratio estimate and the 95% confidence interval limits.
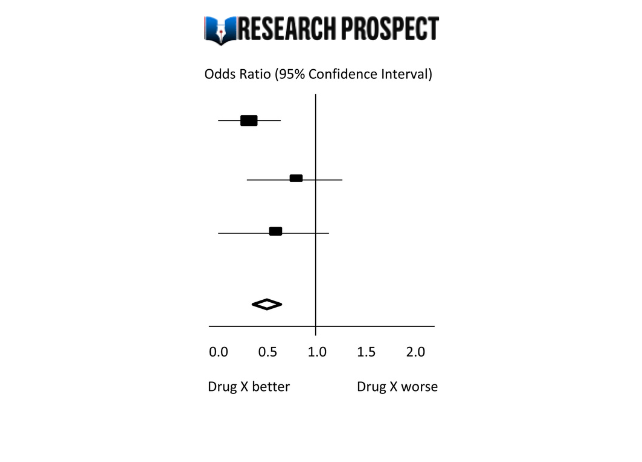
Figure-A: Hypothetical Forest Plot
Relevance to Practice and Research
Evidence Based Nursing commentaries often include recently published systematic reviews and meta-analyses, as they can provide new insights and strengthen recommendations for effective healthcare practices. Additionally, they can identify gaps or limitations in current evidence and guide future research directions.
The quality of the data available for synthesis is a critical factor in the strength of conclusions drawn from meta-analyses, and this is influenced by the quality of individual studies and the systematic review itself. However, meta-analysis cannot overcome issues related to underpowered or poorly designed studies.
Therefore, clinicians may still encounter situations where the evidence is weak or uncertain, and where higher-quality research is required to improve clinical decision-making. While such findings can be frustrating, they remain important for informing practice and highlighting the need for further research to fill gaps in the evidence base.
Methods and Assumptions in Meta-Analysis
Ensuring the credibility of findings is imperative in all types of research, including meta-analyses. To validate the outcomes of a meta-analysis, the researcher must confirm that the research techniques used were accurate in measuring the intended variables. Typically, researchers establish the validity of a meta-analysis by testing the outcomes for homogeneity or the degree of similarity between the results of the combined studies.
Homogeneity is preferred in meta-analyses as it allows the data to be combined without needing adjustments to suit the study’s requirements. To determine homogeneity, researchers assess heterogeneity, the opposite of homogeneity. Two widely used statistical methods for evaluating heterogeneity in research results are Cochran’s-Q and I-Square, also known as I-2 Index.
Difference Between Meta-Analysis and Systematic Reviews
Meta-analysis and systematic reviews are both research methods used to synthesise evidence from multiple studies on a particular topic. However, there are some key differences between the two.
Systematic reviews involve a comprehensive and structured approach to identifying, selecting, and critically appraising all available evidence relevant to a specific research question. This process involves searching multiple databases, screening the identified studies for relevance and quality, and summarizing the findings in a narrative report.
Meta-analysis, on the other hand, involves using statistical methods to combine and analyze the data from multiple studies, with the aim of producing a quantitative summary of the overall effect size. Meta-analysis requires the studies to be similar enough in terms of their design, methodology, and outcome measures to allow for meaningful comparison and analysis.
Therefore, systematic reviews are broader in scope and summarize the findings of all studies on a topic, while meta-analyses are more focused on producing a quantitative estimate of the effect size of an intervention across multiple studies that meet certain criteria. In some cases, a systematic review may be conducted without a meta-analysis if the studies are too diverse or the quality of the data is not sufficient to allow for statistical pooling.
Software Packages For Meta-Analysis
Meta-analysis can be done through software packages, including free and paid options. One of the most commonly used software packages for meta-analysis is RevMan by the Cochrane Collaboration.
Assessing the Quality of Meta-Analysis
Assessing the quality of a meta-analysis involves evaluating the methods used to conduct the analysis and the quality of the studies included. Here are some key factors to consider:
- Study selection: The studies included in the meta-analysis should be relevant to the research question and meet predetermined criteria for quality.
- Search strategy: The search strategy should be comprehensive and transparent, including databases and search terms used to identify relevant studies.
- Study quality assessment: The quality of included studies should be assessed using appropriate tools, and this assessment should be reported in the meta-analysis.
- Data extraction: The data extraction process should be systematic and clearly reported, including any discrepancies that arose.
- Analysis methods: The meta-analysis should use appropriate statistical methods to combine the results of the included studies, and these methods should be transparently reported.
- Publication bias: The potential for publication bias should be assessed and reported in the meta-analysis, including any efforts to identify and include unpublished studies.
- Interpretation of results: The results should be interpreted in the context of the study limitations and the overall quality of the evidence.
- Sensitivity analysis: Sensitivity analysis should be conducted to evaluate the impact of study quality, inclusion criteria, and other factors on the overall results.
Overall, a high-quality meta-analysis should be transparent in its methods and clearly report the included studies’ limitations and the evidence’s overall quality.
Hire an Expert Writer
Orders completed by our expert writers are
- Formally drafted in an academic style
- Free Amendments and 100% Plagiarism Free – or your money back!
- 100% Confidential and Timely Delivery!
- Free anti-plagiarism report
- Appreciated by thousands of clients. Check client reviews
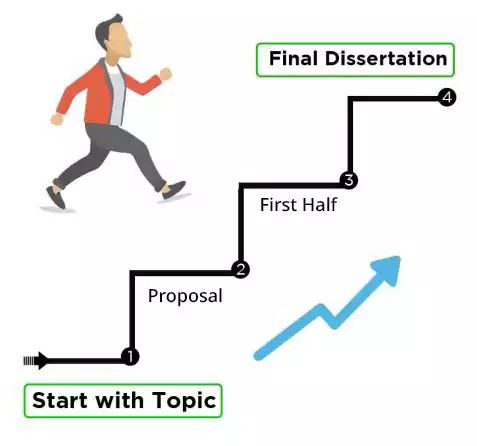
Examples of Meta-Analysis
- STANLEY T.D. et JARRELL S.B. (1989), « Meta-regression analysis : a quantitative method of literature surveys », Journal of Economics Surveys, vol. 3, n°2, pp. 161-170.
- DATTA D.K., PINCHES G.E. et NARAYANAN V.K. (1992), « Factors influencing wealth creation from mergers and acquisitions : a meta-analysis », Strategic Management Journal, Vol. 13, pp. 67-84.
- GLASS G. (1983), « Synthesising empirical research : Meta-analysis » in S.A. Ward and L.J. Reed (Eds), Knowledge structure and use : Implications for synthesis and interpretation, Philadelphia : Temple University Press.
- WOLF F.M. (1986), Meta-analysis : Quantitative methods for research synthesis, Sage University Paper n°59.
- HUNTER J.E., SCHMIDT F.L. et JACKSON G.B. (1982), « Meta-analysis : cumulating research findings across studies », Beverly Hills, CA : Sage.
Frequently Asked Questions
What is a meta-analysis in research.
Meta-analysis is a statistical method used to combine results from multiple studies on a specific topic. By pooling data from various sources, meta-analysis can provide a more precise estimate of the effect size of a treatment or intervention and identify areas for future research.
Why is meta-analysis important?
Meta-analysis is important because it combines and summarizes results from multiple studies to provide a more precise and reliable estimate of the effect of a treatment or intervention. This helps clinicians and policymakers make evidence-based decisions and identify areas for further research.
What is an example of a meta-analysis?
A meta-analysis of studies evaluating physical exercise’s effect on depression in adults is an example. Researchers gathered data from 49 studies involving a total of 2669 participants. The studies used different types of exercise and measures of depression, which made it difficult to compare the results.
Through meta-analysis, the researchers calculated an overall effect size and determined that exercise was associated with a statistically significant reduction in depression symptoms. The study also identified that moderate-intensity aerobic exercise, performed three to five times per week, was the most effective. The meta-analysis provided a more comprehensive understanding of the impact of exercise on depression than any single study could provide.
What is the definition of meta-analysis in clinical research?
Meta-analysis in clinical research is a statistical technique that combines data from multiple independent studies on a particular topic to generate a summary or “meta” estimate of the effect of a particular intervention or exposure.
This type of analysis allows researchers to synthesise the results of multiple studies, potentially increasing the statistical power and providing more precise estimates of treatment effects. Meta-analyses are commonly used in clinical research to evaluate the effectiveness and safety of medical interventions and to inform clinical practice guidelines.
Is meta-analysis qualitative or quantitative?
Meta-analysis is a quantitative method used to combine and analyze data from multiple studies. It involves the statistical synthesis of results from individual studies to obtain a pooled estimate of the effect size of a particular intervention or treatment. Therefore, meta-analysis is considered a quantitative approach to research synthesis.
You May Also Like
Discourse analysis is an essential aspect of studying a language. It is used in various disciplines of social science and humanities such as linguistic, sociolinguistics, and psycholinguistic.
In historical research, a researcher collects and analyse the data, and explain the events that occurred in the past to test the truthfulness of observations.
A hypothesis is a research question that has to be proved correct or incorrect through hypothesis testing – a scientific approach to test a hypothesis.
USEFUL LINKS
LEARNING RESOURCES
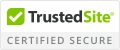
COMPANY DETAILS
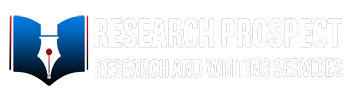
- How It Works
Doing a Meta-Analysis: A Practical, Step-by-Step Guide
Saul McLeod, PhD
Editor-in-Chief for Simply Psychology
BSc (Hons) Psychology, MRes, PhD, University of Manchester
Saul McLeod, PhD., is a qualified psychology teacher with over 18 years of experience in further and higher education. He has been published in peer-reviewed journals, including the Journal of Clinical Psychology.
Learn about our Editorial Process
Olivia Guy-Evans, MSc
Associate Editor for Simply Psychology
BSc (Hons) Psychology, MSc Psychology of Education
Olivia Guy-Evans is a writer and associate editor for Simply Psychology. She has previously worked in healthcare and educational sectors.
On This Page:
What is a Meta-Analysis?
Meta-analysis is a statistical procedure used to combine and synthesize findings from multiple independent studies to estimate the average effect size for a particular research question.
Meta-analysis goes beyond traditional narrative reviews by using statistical methods to integrate the results of several studies, leading to a more objective appraisal of the evidence.
This method addresses limitations like small sample sizes in individual studies, providing a more precise estimate of a treatment effect or relationship strength.
Meta-analyses are particularly valuable when individual study results are inconclusive or contradictory, as seen in the example of vitamin D supplementation and the prevention of fractures.
For instance, a meta-analysis published in JAMA in 2017 by Zhao et al. examined 81 randomized controlled trials involving 53,537 participants.
The results of this meta-analysis suggested that vitamin D supplementation was not associated with a lower risk of fractures among community-dwelling adults. This finding contradicted some earlier beliefs and individual study results that had suggested a protective effect.
What’s the difference between a meta-analysis, systematic review, and literature review?
Literature reviews can be conducted without defined procedures for gathering information. Systematic reviews use strict protocols to minimize bias when gathering and evaluating studies, making them more transparent and reproducible.
While a systematic review thoroughly maps out a field of research, it cannot provide unbiased information on the magnitude of an effect. Meta-analysis statistically combines effect sizes of similar studies, going a step further than a systematic review by weighting each study by its precision.
What is Effect Size?
Statistical significance is a poor metric in meta-analysis because it only indicates whether an effect is likely to have occurred by chance. It does not provide information about the magnitude or practical importance of the effect.
While a statistically significant result may indicate an effect different from zero, this effect might be too small to hold practical value. Effect size, on the other hand, offers a standardized measure of the magnitude of the effect, allowing for a more meaningful interpretation of the findings
Meta-analysis goes beyond simply synthesizing effect sizes; it uses these statistics to provide a weighted average effect size from studies addressing similar research questions. The larger the effect size the stronger the relationship between two variables.
If effect sizes are consistent, the analysis demonstrates that the findings are robust across the included studies. When there is variation in effect sizes, researchers should focus on understanding the reasons for this dispersion rather than just reporting a summary effect.
Meta-regression is one method for exploring this variation by examining the relationship between effect sizes and study characteristics.
T here are three primary families of effect sizes used in most meta-analyses:
- Mean difference effect sizes : Used to show the magnitude of the difference between means of groups or conditions, commonly used when comparing a treatment and control group.
- Correlation effect sizes : Represent the degree of association between two continuous measures, indicating the strength and direction of their relationship.
- Odds ratio effect sizes : Used with binary outcomes to compare the odds of an event occurring between two groups, like whether a patient recovers from an illness or not.
The most appropriate effect size family is determined by the nature of the research question and dependent variable. All common effect sizes are able to be transformed from one version to another.
Real-Life Example
Brewin, C. R., Andrews, B., & Valentine, J. D. (2000). Meta-analysis of risk factors for posttraumatic stress disorder in trauma-exposed adults. Journal of Consulting and Clinical Psychology , 68 (5), 748.
This meta-analysis of 77 articles examined risk factors for posttraumatic stress disorder (PTSD) in trauma-exposed adults, with sample sizes ranging from 1,149 to over 11,000. Several factors consistently predicted PTSD with small effect sizes (r = 0.10 to 0.19), including female gender, lower education, lower intelligence, previous trauma, childhood adversity, and psychiatric history. Factors occurring during or after trauma showed somewhat stronger effects (r = 0.23 to 0.40), including trauma severity, lack of social support, and additional life stress. Most risk factors did not predict PTSD uniformly across populations and study types, with only psychiatric history, childhood abuse, and family psychiatric history showing homogeneous effects. Notable differences emerged between military and civilian samples, and methodological factors influenced some risk factor effects. The authors concluded that identifying a universal set of pretrauma predictors is premature and called for more research to understand how vulnerability to PTSD varies across populations and contexts.
How to Conduct a Meta-Analysis
Researchers should develop a comprehensive research protocol that outlines the objectives and hypotheses of their meta-analysis.
This document should provide specific details about every stage of the research process, including the methodology for identifying, selecting, and analyzing relevant studies.
For example, the protocol should specify search strategies for relevant studies, including whether the search will encompass unpublished works.
The protocol should be created before beginning the research process to ensure transparency and reproducibility.
Research Protocol
- To estimate the overall effect of growth mindset interventions on the academic achievement of students in primary and secondary school.
- To investigate if the effect of growth mindset interventions on academic achievement differs for students of different ages (e.g., elementary school students vs. high school students).
- To examine if the duration of the growth mindset intervention impacts its effectiveness.
- Growth mindset interventions will have a small, but statistically significant, positive effect on student academic achievement.
- Growth mindset interventions will be more effective for younger students than for older students.
- Longer growth mindset interventions will be more effective than shorter interventions.
Eligibility Criteria
- Published studies in English-language journals.
- Studies must include a quantitative measure of academic achievement (e.g., GPA, course grades, exam scores, or standardized test scores).
- Studies must involve a growth mindset intervention as the primary focus (including control vs treatment group comparison).
- Studies that combine growth mindset training with other interventions (e.g., study skills training, other types of psychological interventions) should be excluded.
Search Strategy
The researchers will search the following databases:
Keywords Combined with Boolean Operators:
- (“growth mindset” OR “implicit theories of intelligence” OR “mindset theory”) AND (“intervention” OR “training” OR “program”) ” OR “educational outcomes”) * OR “pupil ” OR “learner*”)**
Additional Search Strategies:
- Citation Chaining: Examining the reference lists of included studies can uncover additional relevant articles.
- Contacting Experts: Reaching out to researchers in the field of growth mindset can reveal unpublished studies or ongoing research.
Coding of Studies
The researchers will code each study for the following information:
- Sample size
- Age of participants
- Duration of intervention
- Type of academic outcome measured
- Study design (e.g., randomized controlled trial, quasi-experiment)
Statistical Analysis
- The researchers will calculate an effect size (e.g., standardized mean difference) for each study.
- The researchers will use a random-effects model to account for variation in effect sizes across studies.
- The researchers will use meta-regression to test the hypotheses about moderators of the effect of growth mindset interventions.
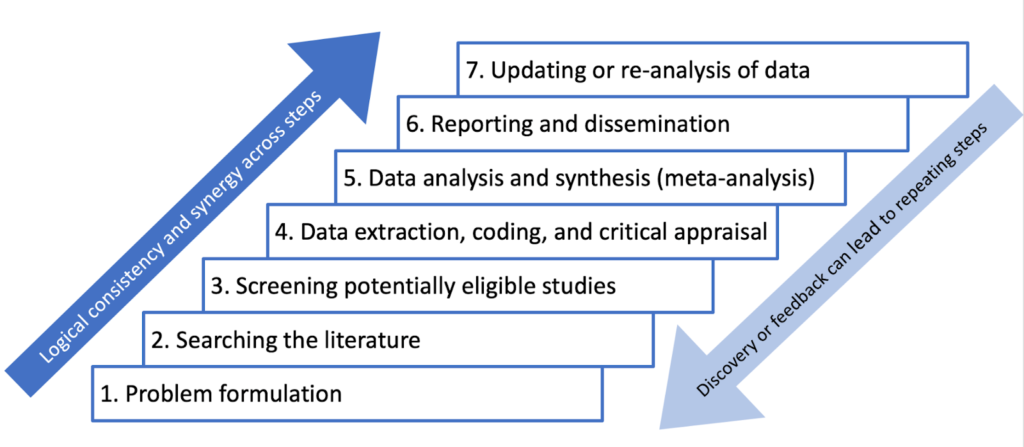
PRISMA (Preferred Reporting Items for Systematic Reviews and Meta-Analyses) is a reporting guideline designed to improve the transparency and completeness of systematic review reporting.
PRISMA was created to tackle the issue of inadequate reporting often found in systematic reviews
- Checklist : PRISMA features a 27-item checklist covering all aspects of a meta-analysis, from the rationale and objectives to the synthesis of findings and discussion of limitations. Each checklist item is accompanied by detailed reporting recommendations in an Explanation and Elaboration document .
- Flow Diagram : PRISMA also includes a flow diagram to visually represent the study selection process, offering a clear, standardized way to illustrate how researchers arrived at the final set of included studies
Step 1: Defining a Research Question
A well-defined research question is a fundamental starting point for any research synthesis. The research question should guide decisions about which studies to include in the meta-analysis, and which statistical model is most appropriate.
For example:
- How do dysfunctional attitudes and negative automatic thinking directly and indirectly impact depression?
- Do growth mindset interventions generally improve students’ academic achievement?
- What is the association between child-parent attachment and prosociality in children?
- What is the relation of various risk factors to Post Traumatic Stress Disorder (PTSD)?
Step 2: Search Strategy
Present the full search strategies for all databases, registers and websites, including any filters and limits used. PRISMA 2020 Checklist
A search strategy is a comprehensive and reproducible plan for identifying all relevant research studies that address a specific research question.
This systematic approach to searching helps minimize bias.
It’s important to be transparent about the search strategy and document all decisions for auditability. The goal is to identify all potentially relevant studies for consideration.
PRISMA (Preferred Reporting Items for Systematic reviews and Meta-Analyses) provide appropriate guidance for reporting quantitative literature searches.
Information Sources
The primary goal is to find all published and unpublished studies that meet the predefined criteria of the research question. This includes considering various sources beyond typical databases
Information sources for a meta-analysis can include a wide range of resources like scholarly databases, unpublished literature, conference papers, books, and even expert consultations.
Specify all databases, registers, websites, organisations, reference lists and other sources searched or consulted to identify studies. Specify the date when each source was last searched or consulted. PRISMA 2020 Checklist
An exhaustive, systematic search strategy is developed with the assistance of an expert librarian.
- Databases: Searches should include seven key databases: CINAHL, Medline, APA PsycArticles, Psychology and Behavioral Sciences Collection, APA PsycInfo, SocINDEX with Full Text, and Web of Science: Core Collections.
- Grey Literature : In addition to databases, forensic or ‘expansive’ searches can be conducted. This includes: grey literature database searches (e.g. OpenGrey , WorldCat , Ethos ), conference proceedings, unpublished reports, theses , clinical trial databases , searches by names of authors of relevant publications. Independent research bodies may also be good sources of material, e.g. Centre for Research in Ethnic Relations , Joseph Rowntree Foundation , Carers UK .
- Citation Searching : Reference lists often lead to highly cited and influential papers in the field, providing valuable context and background information for the review.
- Contacting Experts: Reaching out to researchers or experts in the field can provide access to unpublished data or ongoing research not yet publicly available.
It is important to note that this may not be an exhaustive list of all potential databases.
Search String Construction
It is recommended to consult topic experts on the review team and advisory board in order to create as complete a list of search terms as possible for each concept.
To retrieve the most relevant results, a search string is used. This string is made up of:
- Keywords: Search terms should be relevant to the research questions, key variables, participants, and research design. Searches should include indexed terms, titles, and abstracts. Additionally, each database has specific indexed terms, so a targeted search strategy must be created for each database.
- Synonyms: These are words or phrases with similar meanings to the keywords, as authors may use different terms to describe the same concepts. Including synonyms helps cover variations in terminology and increases the chances of finding all relevant studies. For example, a drug intervention may be referred to by its generic name or by one of its several proprietary names.
- Truncation symbols : These broaden the search by capturing variations of a keyword. They function by locating every word that begins with a specific root. For example, if a user was researching interventions for smoking, they might use a truncation symbol to search for “smok*” to retrieve records with the words “smoke,” “smoker,” “smoking,” or “smokes.” This can save time and effort by eliminating the need to input every variation of a word into a database.
- Boolean operators: The use of Boolean operators (AND/OR/NEAR/NOT) helps to combine these terms effectively, ensuring that the search strategy is both sensitive and specific. For instance, using “AND” narrows the search to include only results containing both terms, while “OR” expands it to include results containing either term.
When conducting these searches, it is important to combine browsing of texts (publications) with periods of more focused systematic searching. This iterative process allows the search to evolve as the review progresses.
It is important to note that this information may not be entirely comprehensive and up-to-date.
Studies were identified by searching PubMed, PsycINFO, and the Cochrane Library. We conducted searches for studies published between the first available year and April 1, 2009, using the search term mindfulness combined with the terms meditation, program, therapy, or intervention and anxi , depress , mood, or stress. Additionally, an extensive manual review was conducted of reference lists of relevant studies and review articles extracted from the database searches. Articles determined to be related to the topic of mindfulness were selected for further examination.
Specify the inclusion and exclusion criteria for the review. PRISMA 2020 Checklist
Before beginning the literature search, researchers should establish clear eligibility criteria for study inclusion
To maintain transparency and minimize bias, eligibility criteria for study inclusion should be established a priori. Ideally, researchers should aim to include only high-quality randomized controlled trials that adhere to the intention-to-treat principle.
The selection of studies should not be arbitrary, and the rationale behind inclusion and exclusion criteria should be clearly articulated in the research protocol.
When specifying the inclusion and exclusion criteria, consider the following aspects:
- Intervention Characteristics: Researchers might decide that, in order to be included in the review, an intervention must have specific characteristics. They might require the intervention to last for a certain length of time, or they might determine that only interventions with a specific theoretical basis are appropriate for their review.
- Population Characteristics: A meta-analysis might focus on the effects of an intervention for a specific population. For instance, researchers might choose to focus on studies that included only nurses or physicians.
- Outcome Measures: Researchers might choose to include only studies that used outcome measures that met a specific standard.
- Age of Participants: If a meta-analysis is examining the effects of a treatment or intervention for children, the authors of the review will likely choose to exclude any studies that did not include children in the target age range.
- Diagnostic Status of Participants: Researchers conducting a meta-analysis of treatments for anxiety will likely exclude any studies where the participants were not diagnosed with an anxiety disorder.
- Study Design: Researchers might determine that only studies that used a particular research design, such as a randomized controlled trial, will be included in the review.
- Control Group: In a meta-analysis of an intervention, researchers might choose to include only studies that included certain types of control groups, such as a waiting list control or another type of intervention.
- Publication status : Decide whether only published studies will be included or if unpublished works, such as dissertations or conference proceedings, will also be considered.
Studies were selected if (a) they included a mindfulness-based intervention, (b) they included a clinical sample (i.e., participants had a diagnosable psychological or physical/medical disorder), (c) they included adult samples (18 – 65 years of age), (d) the mindfulness program was not coupled with treatment using acceptance and commitment therapy or dialectical behavior therapy, (e) they included a measure of anxiety and/or mood symptoms at both pre and postintervention, and (f) they provided sufficient data to perform effect size analyses (i.e., means and standard deviations, t or F values, change scores, frequencies, or probability levels). Studies were excluded if the sample overlapped either partially or completely with the sample of another study meeting inclusion criteria for the meta-analysis. In these cases, we selected for inclusion the study with the larger sample size or more complete data for measures of anxiety and depression symptoms. For studies that provided insufficient data but were otherwise appropriate for the analyses, authors were contacted for supplementary data.
Iterative Process
The iterative nature of developing a search strategy stems from the need to refine and adapt the search process based on the information encountered at each stage.
A single attempt rarely yields the perfect final strategy. Instead, it is an evolving process involving a series of test searches, analysis of results, and discussions among the review team.
Here’s how the iterative process unfolds:
- Initial Strategy Formulation: Based on the research question, the team develops a preliminary search strategy, including identifying relevant keywords, synonyms, databases, and search limits.
- Test Searches and Refinement: The initial search strategy is then tested on chosen databases. The results are reviewed for relevance, and the search strategy is refined accordingly. This might involve adding or modifying keywords, adjusting Boolean operators, or reconsidering the databases used.
- Discussions and Iteration: The search results and proposed refinements are discussed within the review team. The team collaboratively decides on the best modifications to improve the search’s comprehensiveness and relevance.
- Repeating the Cycle: This cycle of test searches, analysis, discussions, and refinements is repeated until the team is satisfied with the strategy’s ability to capture all relevant studies while minimizing irrelevant results.
By constantly refining the search strategy based on the results and feedback, researchers can be more confident that they have identified all relevant studies.
This iterative process ensures that the applied search strategy is sensitive enough to capture all relevant studies while maintaining a manageable scope.
Throughout this process, meticulous documentation of the search strategy, including any modifications, is crucial for transparency and future replication of the meta-analysis.
Step 3: Search the Literature
Conduct a systematic search of the literature using clearly defined search terms and databases.
Applying the search strategy involves entering the constructed search strings into the respective databases’ search interfaces. These search strings, crafted using Boolean operators, truncation symbols, wildcards, and database-specific syntax, aim to retrieve all potentially relevant studies addressing the research question.
The researcher, during this stage, interacts with the database’s features to refine the search and manage the retrieved results.
This might involve employing search filters provided by the database to focus on specific study designs, publication types, or other relevant parameters.
Applying the search strategy is not merely a mechanical process of inputting terms; it demands a thorough understanding of database functionalities and a discerning eye to adjust the search based on the nature of retrieved results.
Step 4: Screening & Selecting Research Articles
Once the literature search is complete, the next step is to screen and select the studies that will be included in the meta-analysis.
This involves carefully reviewing each study to determine its relevance to the research question and its methodological quality.
The goal is to identify studies that are both relevant to the research question and of sufficient quality to contribute to a meaningful synthesis.
Studies meeting the eligibility criteria are usually saved into electronic databases, such as Endnote or Mendeley , and include title, authors, date and publication journal along with an abstract (if available).
Selection Process
Specify the methods used to decide whether a study met the inclusion criteria of the review, including how many reviewers screened each record and each report retrieved, whether they worked independently, and if applicable, details of automation tools used in the process. PRISMA 2020 Checklist
The selection process in a meta-analysis involves multiple reviewers to ensure rigor and reliability.
Two reviewers should independently screen titles and abstracts, removing duplicates and irrelevant studies based on predefined inclusion and exclusion criteria.
- Initial screening of titles and abstracts: After applying a strategy to search the literature,, the next step involves screening the titles and abstracts of the identified articles against the predefined inclusion and exclusion criteria. During this initial screening, reviewers aim to identify potentially relevant studies while excluding those clearly outside the scope of the review. It is crucial to prioritize over-inclusion at this stage, meaning that reviewers should err on the side of keeping studies even if there is uncertainty about their relevance. This cautious approach helps minimize the risk of inadvertently excluding potentially valuable studies.
- Retrieving and assessing full texts: For studies which a definitive decision cannot be made based on the title and abstract alone, reviewers need to obtain the full text of the articles for a comprehensive assessment against the predefined inclusion and exclusion criteria. This stage involves meticulously reviewing the full text of each potentially relevant study to determine its eligibility definitively.
- Resolution of Disagreements : In cases of disagreement between reviewers regarding a study’s eligibility, a predefined strategy involving consensus-building discussions or arbitration by a third reviewer should be in place to reach a final decision. This collaborative approach ensures a fair and impartial selection process, further strengthening the review’s reliability.
PRISMA Flowchart
The PRISMA flowchart is a visual representation of the study selection process within a systematic review.
The flowchart illustrates the step-by-step process of screening, filtering, and selecting studies based on predefined inclusion and exclusion criteria.
The flowchart visually depicts the following stages:
- Identification: The initial number of titles and abstracts identified through database searches.
- Screening: The screening process, based on titles and abstracts.
- Eligibility: Full-text copies of the remaining records are retrieved and assessed for eligibility.
- Inclusion: Applying the predefined inclusion criteria resulted in the inclusion of publications that met all the criteria for the review.
- Exclusion: The flowchart details the reasons for excluding the remaining records.
This systematic and transparent approach, as visualized in the PRISMA flowchart, ensures a robust and unbiased selection process, enhancing the reliability of the systematic review’s findings.
The flowchart serves as a visual record of the decisions made during the study selection process, allowing readers to assess the rigor and comprehensiveness of the review.
- How to fill a PRISMA flow diagram
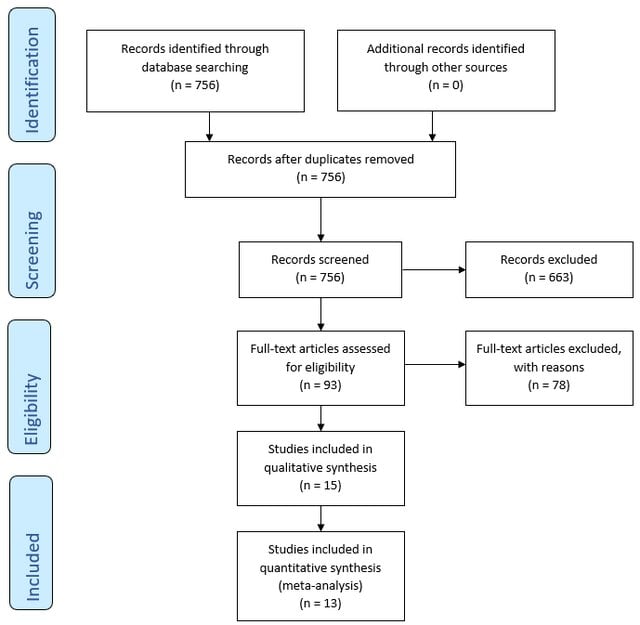
Step 5: Evaluating the Quality of Studies
Data collection process.
Specify the methods used to collect data from reports, including how many reviewers collected data from each report, whether they worked independently, any processes for obtaining or confirming data from study investigators, and if applicable, details of automation tools used in the process. PRISMA 2020 Checklist
Data extraction focuses on information relevant to the research question, such as risk or recovery factors related to a particular phenomenon.
Extract data relevant to the research question, such as effect sizes, sample sizes, means, standard deviations, and other statistical measures.
It can be useful to focus on the authors’ interpretations of findings rather than individual participant quotes, as the latter lacks the full context of the original data.
The coding of studies in a meta-analysis involves carefully and systematically extracting data from each included study in a standardized and reliable manner. This step is essential for ensuring the accuracy and validity of the meta-analysis’s findings.
This information is then used to calculate effect sizes, examine potential moderators, and draw overall conclusions.
Coding procedures typically involve creating a standardized record form or coding protocol. This form guides the extraction of data from each study in a consistent and organized manner. Two independent observers can help to ensure accuracy and minimize errors during data extraction.
Beyond basic information like authors and publication year, code crucial study characteristics relevant to the research question.
For example, if the meta-analysis focuses on the effects of a specific therapy, relevant characteristics to code might include:
- Study characteristics : Publicatrion year, authors, country of origin, publication status ( Published : Peer-reviewed journal articles and book chapters Unpublished : Government reports, websites, theses/dissertations, conference presentations, unpublished manuscripts).
- Intervention : Type (e.g., CBT), duration of treatment, frequency (e.g., weekly sessions), delivery method (e.g., individual, group, online), intention-to-treat analysis (Yes/No)
- Outcome measures : Primary vs. secondary outcomes, time points of measurement (e.g., post-treatment, follow-up).
- Moderators : Participant characteristics that might moderate the effect size. (e.g., age, gender, diagnosis, socioeconomic status, education level, comorbidities).
- Study design : Design (RCT quasi-experiment, etc.), blinding, control group used (e.g., waitlist control, treatment as usual), study setting (clinical, community, online/remote, inpatient vs. outpatient), pre-registration (yes/no), allocation method (simple randomization, block randomization, etc.).
- Sample : Recruitment method (snowball, random, etc.), sample size (total and groups), sample location (treatment & control group), attrition rate, overlap with sample(s) from another study?
- Adherence to reporting guidelines : e.g., CONSORT, STROBE, PRISMA
- Funding source : Government, industry, non-profit, etc.
- Effect Size : Comprehensive meta-analysis program is used to compute d and/or r. Include up to 3 digits after the decimal point for effect size information and internal consistency information. Also record the page number and table number from which the information is coded. This information helps when checking reliability and accuracy to ensure we are coding from the same information.
Before applying the coding protocol to all studies, it’s crucial to pilot test it on a small subset of studies. This helps identify any ambiguities, inconsistencies, or areas for improvement in the coding protocol before full-scale coding begins.
It’s common to encounter missing data in primary research articles. Develop a clear strategy for handling missing data, which might involve contacting study authors, using imputation methods, or performing sensitivity analyses to assess the impact of missing data on the overall results.
Quality Appraisal Tools
Researchers use standardized tools to assess the quality and risk of bias in the quantitative studies included in the meta-analysis. Some commonly used tools include:
- Recommended by the Cochrane Collaboration for assessing randomized controlled trials (RCTs).
- Evaluates potential biases in selection, performance, detection, attrition, and reporting.
- Used for assessing the quality of non-randomized studies, including case-control and cohort studies.
- Evaluates selection, comparability, and outcome assessment.
- Assesses risk of bias in non-randomized studies of interventions.
- Evaluates confounding, selection bias, classification of interventions, deviations from intended interventions, missing data, measurement of outcomes, and selection of reported results.
- Specifically designed for diagnostic accuracy studies.
- Assesses risk of bias and applicability concerns in patient selection, index test, reference standard, and flow and timing.
By using these tools, researchers can ensure that the studies included in their meta-analysis are of high methodological quality and contribute reliable quantitative data to the overall analysis.
Step 6: Choice of Effect Size
The choice of effect size metric is typically determined by the research question and the nature of the dependent variable.
- Odds Ratio (OR) : For instance, if researchers are working in medical and health sciences where binary outcomes are common (e.g., yes/no, failed/success), effect sizes like relative risk and odds ratio are often used.
- Mean Difference : Studies focusing on experimental or between-group comparisons often employ mean differences. The raw mean difference, or unstandardized mean difference, is suitable when the scale of measurement is inherently meaningful and comparable across studies.
- Standardized Mean Difference (SMD) : If studies use different scales or measures, the standardized mean difference (e.g., Cohen’s d) is more appropriate. When analyzing observational studies, the correlation coefficient is commonly chosen as the effect size.
- Pearson correlation coefficient (r) : A statistical measure frequently employed in meta-analysis to examine the strength of the relationship between two continuous variables.
Conversion of efect sizes to a common measure
May be necessary to convert reported findings to the chosen primary effect size. The goal is to harmonize different effect size measures to a common metric for meaningful comparison and analysis.
This conversion allows researchers to include studies that report findings using various effect size metrics. For instance, r can be approximately converted to d, and vice versa, using specific equations. Similarly, r can be derived from an odds ratio using another formula.
Many equations relevant to converting effect sizes can be found in Rosenthal (1991).
Step 7: Assessing Heterogeneity
Heterogeneity refers to the variation in effect sizes across studies after accounting for within-study sampling errors.
Heterogeneity refers to how much the results (effect sizes) vary between different studies, where no variation would mean all studies showed the same improvement (no heterogeneity), while greater variation indicates more heterogeneity.
Assessing heterogeneity matters because it helps us understand if the study intervention works consistently across different contexts and guides how we combine and interpret the results of multiple studies.
While little heterogeneity allows us to be more confident in our overall conclusion, significant heterogeneity necessitates further investigation into its underlying causes.
How to assess heterogeneity
- Homogeneity Test : Meta-analyses typically include a homogeneity test to determine if the effect sizes are estimating the same population parameter. The test statistic, denoted as Q, is a weighted sum of squares that follows a chi-square distribution. A significant Q statistic suggests that the effect sizes are heterogeneous.
- I2 Statistic : The I2 statistic is a relative measure of heterogeneity that represents the ratio of between-study variance (τ2) to the total variance (between-study variance plus within-study variance). Higher I2 values indicate greater heterogeneity.
- Prediction Interval : Examining the width of a prediction interval can provide insights into the degree of heterogeneity. A wide prediction interval suggests substantial heterogeneity in the population effect size.
Step 8: Choosing the Meta-Analytic Model
Meta-analysts address heterogeneity by choosing between fixed-effects and random-effects analytical models.
Use a random-effects model if heterogeneity is high. Use a fixed-effect model if heterogeneity is low, or if all studies are functionally identical and you are not seeking to generalize to a range of scenarios.
Although a statistical test for homogeneity can help assess the variability in effect sizes across studies, it shouldn’t dictate the choice between fixed and random effects models.
The decision of which model to use is ultimately a conceptual one, driven by the researcher’s understanding of the research field and the goals of the meta-analysis.
If the number of studies is limited, a fixed-effects analysis is more appropriate, while more studies are required for a stable estimate of the between-study variance in a random-effects model.
It is important to note that using a random-effects model is generally a more conservative approach.
Fixed-effects models
- Assumes all studies are measuring the exact same thing
- Gives much more weight to larger studies
- Use when studies are very similar
Fixed-effects models assume that there is one true effect size underlying all studies. The goal is to estimate this common effect size with the greatest precision, which is achieved by minimizing the within-study (sampling).
Consequently, studies are weighted by the inverse of their variance.
This means that larger studies, which generally have smaller variances, are assigned greater weight in the analysis because they provide more precise estimates of the common effect size
- Simplicity: The fixed-effect model is straightforward to implement and interpret, making it computationally simpler.
- Precision: When the assumption of a common effect size is met, fixed-effect models provide more precise estimates with narrower confidence intervals compared to random-effects models.
- Suitable for Conditional Inferences: Fixed-effect models are appropriate when the goal is to make inferences specifically about the studies included in the meta-analysis, without generalizing to a broader population.
- Restrictive Assumptions: The fixed-effect model assumes all studies estimate the same population parameter, which is often unrealistic, particularly with studies drawn from diverse methodologies or populations.
- Limited Generalizability: Findings from fixed-effect models are conditional on the included studies, limiting their generalizability to other contexts or populations.
- Sensitivity to Heterogeneity: Fixed-effect models are sensitive to the presence of heterogeneity among studies, and may produce misleading results if substantial heterogeneity exists.
Random-effects models
- Assumes studies might be measuring slightly different things
- Gives more balanced weight to both large and small studies
- Use when studies might vary in methods or populations
Random-effects models assume that the true effect size can vary across studies. The goal here is to estimate the mean of these varying effect sizes, considering both within-study variance and between-study variance (heterogeneity).
This approach acknowledges that each study might estimate a slightly different effect size due to factors beyond sampling error, such as variations in study populations, interventions, or designs.
This balanced weighting prevents large studies from disproportionately influencing the overall effect size estimate, leading to a more representative average effect size that reflects the distribution of effects across a range of studies.
- Realistic Assumptions: Random-effects models acknowledge the presence of between-study variability by assuming true effects are randomly distributed, making it more suitable for real-world research scenarios.
- Generalizability: Random-effects models allow for broader inferences to be made about a population of studies, enhancing the generalizability of findings.
- Accommodation of Heterogeneity: Random-effects models explicitly model heterogeneity, providing a more accurate representation of the overall effect when studies have varying effect sizes.
- Complexity: Random-effects models are computationally more complex, requiring the estimation of additional parameters, such as between-study variance.
- Reduced Precision: Confidence intervals tend to be wider compared to fixed-effect models, particularly when between-study heterogeneity is substantial.
- Requirement for Sufficient Studies: Accurate estimation of between-study variance necessitates a sufficient number of studies, making random-effects models less reliable with smaller meta-analyses.
Step 9: Perform the Meta-Analysis
This step involves statistically combining effect sizes from chosen studies. Meta-analysis uses the weighted mean of effect sizes, typically giving larger weights to more precise studies (often those with larger sample sizes).
The main function of meta-analysis is to estimate effects in a population by combining the effect sizes from multiple articles.
It uses a weighted mean of the effect sizes, typically giving larger weights to more precise studies, often those with larger sample sizes.
This weighting scheme makes statistical sense because an effect size with good sampling accuracy (i.e., likely to be an accurate reflection of reality) is weighted highly.
On the other hand, effect sizes from studies with lower sampling accuracy are given less weight in the calculations.
the process:
- Calculate weights for each study
- Multiply each study’s effect by its weight
- Add up all these weighted effects
- Divide by the sum of all weights
Estimating effect size using fixed effects
The fixed-effects model in meta-analysis operates under the assumption that all included studies are estimating the same true effect size.
This model focuses solely on within-study variance when determining the weight of each study.
The weight is calculated as the inverse of the within-study variance, which typically results in larger studies receiving substantially more weight in the analysis.
This approach is based on the idea that larger studies provide more precise estimates of the true effect.
The weighted mean effect size (M) is calculated by summing the products of each study’s effect size (ESi) and its corresponding weight (wi) and dividing that sum by the total sum of the weights:
1. Calculate weights (wi) for each study:
The weight is often the inverse of the variance of the effect size. This means studies with larger sample sizes and less variability will have greater weight, as they provide more precise estimates of the effect size
This weighting scheme reflects the assumption in a fixed-effect model that all studies are estimating the same true effect size, and any observed differences in effect sizes are solely due to sampling error. Therefore, studies with less sampling error (i.e., smaller variances) are considered more reliable and are given more weight in the analysis.
Here’s the formula for calculating the weight in a fixed-effect meta-analysis:
Wi = 1 / VYi 1
- Wi represents the weight assigned to study i.
- VYi is the within-study variance for study i.
Practical steps:
- The weight for each study is calculated as: Weight = 1 / (within-study variance)
- For example: Let’s say a study reports a within-study variance of 0.04. The weight for this study would be: 1 / 0.04 = 25
- Calculate the weight for every study included in your meta-analysis using this method.
- These weights will be used in subsequent calculations, such as computing the weighted mean effect size.
- Note : In a fixed-effects model, we do not calculate or use τ² (tau squared), which represents between-study variance. This is only used in random-effects models.
2. Multiply each study’s effect by its weight:
After calculating the weight for each study, multiply the effect size by its corresponding weight. This step is crucial because it ensures that studies with more precise effect size estimates contribute proportionally more to the overall weighted mean effect size
- For each study, multiply its effect size by the weight we just calculated.
3. Add up all these weighted effects:
Sum up all the products from step 2.
4. Divide by the sum of all weights:
- Add up all the weights we calculated in step 1.
- Divide the sum from step 3 by this total weight.
Implications of the fixed-effects model
- Larger studies (with smaller within-study variance) receive substantially more weight.
- This model assumes that differences between study results are due only to sampling error.
- It’s most appropriate when studies are very similar in methods and sample characteristics.
Estimating effect size using random effects
Random effects meta-analysis is slightly more complicated because multiple sources of differences potentially affecting effect sizes must be accounted for.
The main difference in the random effects model is the inclusion of τ² (tau squared) in the weight calculation. This accounts for between-study heterogeneity, recognizing that studies might be measuring slightly different effects.
This process results in an overall effect size that takes into account both within-study and between-study variability, making it more appropriate when studies differ in methods or populations.
The model estimates the variance of the true effect sizes (τ²). This requires a reasonable number of studies, so random effects estimation might not be feasible with very few studies.
Estimation is typically done using statistical software, with restricted maximum likelihood (REML) being a common method.
1. Calculate weights for each study:
In a random-effects meta-analysis, the weight assigned to each study (W*i) is calculated as the inverse of that study’s variance, similar to a fixed-effect model. However, the variance in a random-effects model considers both the within-study variance (VYi) and the between-studies variance (T^2).
The inclusion of T^2 in the denominator of the weight formula reflects the random-effects model’s assumption that the true effect size can vary across studies.
This means that in addition to sampling error, there is another source of variability that needs to be accounted for when weighting the studies. The between-studies variance, T^2, represents this additional source of variability.
Here’s the formula for calculating the weight in a random-effects meta-analysis:
W*i = 1 / (VYi + T^2)
- W*i represents the weight assigned to study i.
- T^2 is the estimated between-studies variance.
First, we need to calculate something called τ² (tau squared). This represents the between-study variance.
The estimation of T^2 can be done using different methods, one common approach being the method of moments (DerSimonian and Laird method).
The formula for T^2 using the method of moments is: T^2 = (Q – df) / C
- Q is the homogeneity statistic.
- df is the degrees of freedom (number of studies -1).
- C is a constant calculated based on the study weights
- The weight for each study is then calculated as: Weight = 1 / (within-study variance + τ²). This is different from the fixed effects model because we’re adding τ² to account for between-study variability.
Add up all the weights we calculated in step 1. Divide the sum from step 3 by this total weight
Implications of the random-effects model
- Weights are more balanced between large and small studies compared to the fixed-effects model.
- It’s most appropriate when studies vary in methods, sample characteristics, or other factors that might influence the true effect size.
- The random-effects model typically produces wider confidence intervals, reflecting the additional uncertainty from between-study variability.
- Results are more generalizable to a broader population of studies beyond those included in the meta-analysis.
- This model is often more realistic for social and behavioral sciences, where true effects may vary across different contexts or populations.
Step 10: Sensitivity Analysis
Assess the robustness of your findings by repeating the analysis using different statistical methods, models (fixed-effects and random-effects), or inclusion criteria. This helps determine how sensitive your results are to the choices made during the process.
Sensitivity analysis strengthens a meta-analysis by revealing how robust the findings are to the various decisions and assumptions made during the process. It helps to determine if the conclusions drawn from the meta-analysis hold up when different methods, criteria, or data subsets are used.
This is especially important since opinions may differ on the best approach to conducting a meta-analysis, making the exploration of these variations crucial.
Here are some key ways sensitivity analysis contributes to a more robust meta-analysis:
- Assessing Impact of Different Statistical Methods : A sensitivity analysis can involve calculating the overall effect using different statistical methods, such as fixed and random effects models. This comparison helps determine if the chosen statistical model significantly influences the overall results. For instance, in the meta-analysis of β-blockers after myocardial infarction, both fixed and random effects models yielded almost identical overall estimates. This suggests that the meta-analysis findings are resilient to the statistical method employed.
- Evaluating the Influence of Trial Quality and Size : By analyzing the data with and without trials of questionable quality or varying sizes, researchers can assess the impact of these factors on the overall findings.
- Examining the Effect of Trials Stopped Early : Including trials that were stopped early due to interim analysis results can introduce bias. Sensitivity analysis helps determine if the inclusion or exclusion of such trials noticeably changes the overall effect. In the example of the β-blocker meta-analysis, excluding trials stopped early had a negligible impact on the overall estimate.
- Addressing Publication Bias : It’s essential to assess and account for publication bias, which occurs when studies with statistically significant results are more likely to be published than those with null or nonsignificant findings. This can be accomplished by employing techniques like funnel plots, statistical tests (e.g., Begg and Mazumdar’s rank correlation test, Egger’s test), and sensitivity analyses.
By systematically varying different aspects of the meta-analysis, researchers can assess the robustness of their findings and address potential concerns about the validity of their conclusions.
This process ensures a more reliable and trustworthy synthesis of the research evidence.
Common Mistakes
When conducting a meta-analysis, several common pitfalls can arise, potentially undermining the validity and reliability of the findings. Sources caution against these mistakes and offer guidance on conducting methodologically sound meta-analyses.
- Insufficient Number of Studies: If there are too few primary studies available, a meta-analysis might not be appropriate. While a meta-analysis can technically be conducted with only two studies, the research community might not view findings based on a limited number of studies as reliable evidence. A small number of studies could suggest that the research field is not mature enough for meaningful synthesis.
- Inappropriate Combination of Studies : Meta-analyses should not simply combine studies indiscriminately. Avoid the “apples and oranges” problem, where studies with different research objectives, designs, measures, or samples are inappropriately combined. Such practices can obscure important differences between studies and lead to misleading conclusions.
- Misinterpreting Heterogeneity : One common mistake is using the Q statistic or p-value from a test of heterogeneity as the sole indicator of heterogeneity. While these statistics can signal heterogeneity, they do not quantify the extent of variation in effect sizes.
- Over-Reliance on Published Studies : This dependence on published literature introduces the risk of publication bias, where studies with statistically significant or favorable results are more likely to be published. Failure to acknowledge and address publication bias can lead to overestimating the true effect size.
- Neglecting Study Quality : Including studies with poor methodological quality can bias the results of a meta-analysis leading to unreliable and inaccurate effect size estimates. The decision of which studies to include should be based on predefined eligibility criteria to ensure the quality and relevance of the synthesis.
- Fixation on Statistical Significance : Placing excessive emphasis on the statistical significance of an overall effect while neglecting its practical significance is a critical mistake in meta-analysis, as is the case in primary studies. Considers both statistical and clinical or substantive significance.
- Misinterpreting Significance Testing in Subgroup Analyses : When comparing effect sizes across subgroups, merely observing that an effect is statistically significant in one subgroup but not another is insufficient. Conduct formal tests of statistical significance for the difference in effects between subgroups or to calculate the difference in effects with confidence intervals.
- Ignoring Dependence : Neglecting dependence among effect sizes, particularly when multiple effect sizes are extracted from the same study, is a mistake. This oversight can inflate Type I error rates and lead to inaccurate estimations of average effect sizes and standard errors.
- Inadequate Reporting : Failing to transparently and comprehensively report the meta-analysis process is a crucial mistake. A meta-analysis should include a detailed written protocol outlining the research question, search strategy, inclusion criteria, and analytical methods.
Reading List
- Bar-Haim, Y., Lamy, D., Pergamin, L., Bakermans-Kranenburg, M. J., & Van Ijzendoorn, M. H. (2007). Threat-related attentional bias in anxious and nonanxious individuals: a meta-analytic study . Psychological bulletin , 133 (1), 1.
- Borenstein, M., Hedges, L. V., Higgins, J. P., & Rothstein, H. R. (2021). Introduction to meta-analysis . John Wiley & Sons.
- Crits-Christoph, P. (1992). A Meta-analysis . American Journal of Psychiatry , 149 , 151-158.
- Duval, S. J., & Tweedie, R. L. (2000). A nonparametric “trim and fill” method of accounting for publication bias in meta-analysis. Journal of the American Statistical Association, 95 (449), 89–98.
- Egger, M., Davey Smith, G., Schneider, M., & Minder, C. (1997). Bias in meta-analysis detected by a simple, graphical test . BMJ, 315 (7109), 629–634.
- Egger, M., Smith, G. D., & Phillips, A. N. (1997). Meta-analysis: principles and procedures . Bmj , 315 (7121), 1533-1537.
- Field, A. P., & Gillett, R. (2010). How to do a meta‐analysis . British Journal of Mathematical and Statistical Psychology , 63 (3), 665-694.
- Hedges, L. V., & Pigott, T. D. (2004). The power of statistical tests for moderators in meta-analysis . Psychological methods , 9 (4), 426.
- Hedges, L. V., & Olkin, I. (2014). Statistical methods for meta-analysis . Academic press.
- Hofmann, S. G., Sawyer, A. T., Witt, A. A., & Oh, D. (2010). The effect of mindfulness-based therapy on anxiety and depression: A meta-analytic review . Journal of consulting and clinical psychology , 78 (2), 169.
- Littell, J. H., Corcoran, J., & Pillai, V. (2008). Systematic reviews and meta-analysis . Oxford University Press.
- Lyubomirsky, S., King, L., & Diener, E. (2005). The benefits of frequent positive affect: Does happiness lead to success? . Psychological bulletin , 131 (6), 803.
- Macnamara, B. N., & Burgoyne, A. P. (2022). Do growth mindset interventions impact students’ academic achievement? A systematic review and meta-analysis with recommendations for best practices. Psychological Bulletin .
- Polanin, J. R., & Pigott, T. D. (2015). The use of meta‐analytic statistical significance testing . Research Synthesis Methods , 6 (1), 63-73.
- Rodgers, M. A., & Pustejovsky, J. E. (2021). Evaluating meta-analytic methods to detect selective reporting in the presence of dependent effect sizes . Psychological methods , 26 (2), 141.
- Rosenthal, R. (1991). Meta-analysis: a review. Psychosomatic medicine , 53 (3), 247-271.
- Tipton, E., Pustejovsky, J. E., & Ahmadi, H. (2019). A history of meta‐regression: Technical, conceptual, and practical developments between 1974 and 2018 . Research synthesis methods , 10 (2), 161-179.
- Zhao, J. G., Zeng, X. T., Wang, J., & Liu, L. (2017). Association between calcium or vitamin D supplementation and fracture incidence in community-dwelling older adults: a systematic review and meta-analysis. Jama , 318 (24), 2466-2482.


IMAGES
VIDEO
COMMENTS
The use of social media has grown tremendously, but a considerable number of individuals have stopped using it. This meta-analysis aims to examine the factors that contribute to discontinuing social media use by reviewing 88 studies with a cumulative sample size of 42,159, including 33 effect sizes. Our study reveals that various stressors, including messaging overload (CO), social overload ...
This federated meta-analysis used data from cohorts participating in the InterConnect project. This international research project aims to optimise the use of individual-participant data by enabling cross-cohort analyses without pooling data at a central location. The InterConnect registry was compiled using systematic searches of the ...
The Meta-analysis was performed using R 4.2.1 software (R Core Team, Citation 2023). The 'meta' package (version 5.2-0) was used to perform the meta-analysis (Schwarzer, Citation 2023). The mean difference (MD) was used as the effect size for continuous variables, and the relative risk (RR) was used as the effect size for dichotomous variables.
Key Points. Question Are electronic alerts (e-alerts) for acute kidney injury (AKI) in the electronic health record associated with patient outcomes or clinical practice patterns?. Findings In this systematic review and meta-analysis of 13 unique studies with 41 837 unique patients, AKI e-alerts were not associated with a lower risk for mortality but were associated with a lower risk for AKI ...
The use of proton therapy (PT) in early-stage non-small cell lung cancer (ES-NSCLC) remains controversial, with insufficient evidence to determine its superiority over photon therapy (XRT). We conducted a systematic review of PT trials in ES-NSCLC, analyzing dosimetry, efficacy, and safety across to inform clinical decision-making. Our study showed that PT reduced lung and heart dosimetric ...
To investigate the influence of aerobic exercise on depression among the young people. PubMed, Web of science, Embase, Cochrane, EBSCO were searched from inception to November 2023. RCT studies, assessing the use of aerobic exercise in young people aged 6-35 years and then determining the development of depression in young people (aged 6-35 years), were selected, and mean ± SD values ...
Objective To evaluate the comparative effectiveness and acceptability of oral monotherapy using psychedelics and escitalopram in patients with depressive symptoms, considering the potential for overestimated effectiveness due to unsuccessful blinding. Design Systematic review and Bayesian network meta-analysis. Data sources Medline, Cochrane Central Register of Controlled Trials, Embase ...
This is supplementary material to the article "30 Years of Experimental Education Research in the Post-Soviet Space: A Meta-Analysis of Interventions". This meta-analysis systematically evaluates the potential of available research in post-Soviet countries as a basis for an evidence-based approach to improving student achievement. The study was conducted on a selection of 41 publications ...
Stata 16.0 SE software was used for data analysis, and sensitivity analysis and publication bias tests were also performed. ... this study focused on the global perspective and prospective research to determine the incidence of DOL and analyse the factors influencing DOL quantitatively through meta-analysis and to summarize the influencing ...
The results are driven by a very small number of influential studies, and research in several key clinical contexts is limited to single studies. Both clinical trials and observational studies are needed to understand the potential role of ibuprofen in childhood asthma pathogenesis. ... For meta-analysis of dichotomous data, ORs were pooled ...
They used a combination of advanced techniques to measure gene activity in tens of thousands of individual cells and to visualize gene expression in two-dimensional sections of roots. The microscopic resolution of the analysis allowed the researchers to generate a spatial map of gene expression in both the root and the fungal cells. The Impact
US News is a recognized leader in college, grad school, hospital, mutual fund, and car rankings. Track elected officials, research health conditions, and find news you can use in politics ...
Track elected officials, research health conditions, and find news you can use in politics, business, health, and education. ... Meta forecast 2024 capital expenditure would come in between $37 ...
Research and statistics. Reports, analysis and official statistics. Policy papers and consultations. Consultations and strategy. Transparency. Data, Freedom of Information releases and corporate ...
Meta CEO Mark Zuckerberg has promised that AI will revolutionize the company's ad services. But Meta's use of AI for moderating ads may already be putting the company in hot water. A bipartisan ...
US News is a recognized leader in college, grad school, hospital, mutual fund, and car rankings. Track elected officials, research health conditions, and find news you can use in politics ...
This training process used a specially curated dataset of 5,000 instruction-tuning examples with details of 2-20 changes from Meta retriever, including the known root cause and information about ...
It is easy to confuse systematic reviews and meta-analyses. A systematic review is an objective, reproducible method to find answers to a certain research question, by collecting all available studies related to that question and reviewing and analyzing their results. A meta-analysis differs from a systematic review in that it uses statistical ...
Unlike clinical research, where consolidation efforts are facilitated by systematic review and meta-analysis, the basic sciences seldom use such rigorous quantitative methods. The goal of this study is to present a brief theoretical foundation, computational resources and workflow outline along with a working example for performing systematic ...
The graphical output of meta-analysis is a forest plot which provides information on individual studies and the pooled effect. Systematic reviews of literature can be undertaken for all types of questions, and all types of study designs. This article highlights the key features of systematic reviews, and is designed to help readers understand ...
Meta-analysis would be used for the following purposes: To establish statistical significance with studies that have conflicting results. To develop a more correct estimate of effect magnitude. To provide a more complex analysis of harms, safety data, and benefits. To examine subgroups with individual numbers that are not statistically significant.
2.1 Step 1: defining the research question. The first step in conducting a meta-analysis, as with any other empirical study, is the definition of the research question. Most importantly, the research question determines the realm of constructs to be considered or the type of interventions whose effects shall be analyzed.
The term meta-analysis has been used to denote the full range of quantitative methods for research reviews. Meta-analyses are studies of studies.[ 13 ] Meta-analysis provides a logical framework to a research review where similar measures from comparable studies are listed systematically and the available effect measures are combined wherever ...
Meta-analysis is an objective examination of published data from many studies of the same research topic identified through a literature search. Through the use of rigorous statistical methods, it ...
When conducted properly, a meta‐analysis of medical studies is considered as decisive evidence because it occupies a top level in the hierarchy of evidence. An understanding of the principles, performance, advantages and weaknesses of meta‐analyses is important. Therefore, we aim to provide a basic understanding of meta‐analyses for ...
What is meta-analysis? Meta-analysis is a research process used to systematically synthesise or merge the findings of single, independent studies, using statistical methods to calculate an overall or 'absolute' effect.2 Meta-analysis does not simply pool data from smaller studies to achieve a larger sample size. Analysts use well recognised, systematic methods to account for differences in ...
Meta-analysis is a research method for systematically combining and synthesizing findings from multiple quantitative studies in a research domain. ... Later, Samaha et al. used meta-analysis to study the role of culture in relationship marketing in the international context, which has not been studied in previous meta-analyses. Furthermore ...
A systematic review is an article that synthesizes available evidence on a certain topic utilizing a specific research question, pre-specified eligibility criteria for including articles, and a systematic method for its production. Whereas a meta-analysis is a quantitative, epidemiological study design used to assess the results of articles ...
Definition. "A meta-analysis is a formal, epidemiological, quantitative study design that uses statistical methods to generalise the findings of the selected independent studies. Meta-analysis and systematic review are the two most authentic strategies in research. When researchers start looking for the best available evidence concerning ...
Meta-analysis is a statistical procedure used to combine and synthesize findings from multiple independent studies to estimate the average effect size for a particular research question. Meta-analysis goes beyond traditional narrative reviews by using statistical methods to integrate the results of several studies, leading to a more objective ...