- Voxco Online
- Voxco Panel Management
- Voxco Panel Portal
- Voxco Audience
- Voxco Mobile Offline
- Voxco Dialer Cloud
- Voxco Dialer On-premise
- Voxco TCPA Connect
- Voxco Analytics
- Voxco Text & Sentiment Analysis

- 40+ question types
- Drag-and-drop interface
- Skip logic and branching
- Multi-lingual survey
- Text piping
- Question library
- CSS customization
- White-label surveys
- Customizable ‘Thank You’ page
- Customizable survey theme
- Reminder send-outs
- Survey rewards
- Social media
- Website surveys
- Correlation analysis
- Cross-tabulation analysis
- Trend analysis
- Real-time dashboard
- Customizable report
- Email address validation
- Recaptcha validation
- SSL security
Take a peek at our powerful survey features to design surveys that scale discoveries.
Download feature sheet.
- Hospitality
- Academic Research
- Customer Experience
- Employee Experience
- Product Experience
- Market Research
- Social Research
- Data Analysis
Explore Voxco
Need to map Voxco’s features & offerings? We can help!
Watch a Demo
Download Brochures
Get a Quote
- NPS Calculator
- CES Calculator
- A/B Testing Calculator
- Margin of Error Calculator
- Sample Size Calculator
- CX Strategy & Management Hub
- Market Research Hub
- Patient Experience Hub
- Employee Experience Hub
- NPS Knowledge Hub
- Market Research Guide
- Customer Experience Guide
- Survey Research Guides
- Survey Template Library
- Webinars and Events
- Feature Sheets
- Try a sample survey
- Professional Services

Get exclusive insights into research trends and best practices from top experts! Access Voxco’s ‘State of Research Report 2024 edition’ .
We’ve been avid users of the Voxco platform now for over 20 years. It gives us the flexibility to routinely enhance our survey toolkit and provides our clients with a more robust dataset and story to tell their clients.
VP Innovation & Strategic Partnerships, The Logit Group
- Client Stories
- Voxco Reviews
- Why Voxco Research?
- Careers at Voxco
- Vulnerabilities and Ethical Hacking
Explore Regional Offices
- Survey Software The world’s leading omnichannel survey software
- Online Survey Tools Create sophisticated surveys with ease.
- Mobile Offline Conduct efficient field surveys.
- Text Analysis
- Close The Loop
- Automated Translations
- NPS Dashboard
- CATI Manage high volume phone surveys efficiently
- Cloud/On-premise Dialer TCPA compliant Cloud on-premise dialer
- IVR Survey Software Boost productivity with automated call workflows.
- Analytics Analyze survey data with visual dashboards
- Panel Manager Nurture a loyal community of respondents.
- Survey Portal Best-in-class user friendly survey portal.
- Voxco Audience Conduct targeted sample research in hours.
- Predictive Analytics
- Customer 360
- Customer Loyalty
- Fraud & Risk Management
- AI/ML Enablement Services
- Credit Underwriting

Find the best survey software for you! (Along with a checklist to compare platforms)
Get Buyer’s Guide
- 100+ question types
- SMS surveys
- Financial Services
- Banking & Financial Services
- Retail Solution
- Risk Management
- Customer Lifecycle Solutions
- Net Promoter Score
- Customer Behaviour Analytics
- Customer Segmentation
- Data Unification
Explore Voxco
Watch a Demo
Download Brochures
- CX Strategy & Management Hub
- The Voxco Guide to Customer Experience
- Professional services
- Blogs & White papers
- Case Studies
Find the best customer experience platform
Uncover customer pain points, analyze feedback and run successful CX programs with the best CX platform for your team.
Get the Guide Now

VP Innovation & Strategic Partnerships, The Logit Group
- Why Voxco Intelligence?
- Our clients
- Client stories
- Featuresheets


Descriptive research questions: Definition, examples and designing methodology
- October 4, 2021
SHARE THE ARTICLE ON
Conducting thorough market research is all about framing the right questions that provide accurate answers to research questions. The two main categories of questions namely: Quantitative and Qualitative questions focus on differential aspects.
While quantitative research questions are based on numerical data that provides a substantial backing to the decision making process, qualitative research questions aim to derive insights based on textual responses. Both these questions are used based on their relevance and suitability to meet end objectives of the user.
One such useful quantitative question type are the descriptive research questions.

What is descriptive research?
Descriptive research questions aim to provide a description of the variable under consideration. It is one of the easiest and commonly used ways to quantify research variables.
Questions that begin with:
- How much: How much time does an average teenager spend on watching documentaries on OTT platforms?
Variable: time spent on watching documentaries
Group: Teenagers
- How often: How often do you take an international family trip in a year?
Variable: International trips
Group: Families
- How likely: How likely is it for a person to purchase life insurance within the age group of 20-26?
Variable: Likelihood of purchasing a life insurance
Group: People within the age group of 20-26
- What percentage: What percentage of high school students exercise on a daily basis?
Variable: Daily Exercise
Group: High School Students
- How many: How many smartphone users make use of curated apps to manage daily tasks?
Variable: Usage of curated apps
Group: Smartphone users
- What proportion: What proportion of students prefer online education to offline education?
Variable: Educational format
Group: Students
- How regularly: How regularly does a woman engage or purchase from a cosmetic brand outlet as against e-commerce websites?
Variable: Purchasing Behaviour of cosmetics
Group: Women

- What is: What is the ratio of passengers indulging in train travel to travelling by flight?
Variable: Travelling medium
Group: Passengers
- What are: What are the influencing factors that impact the choice of purchasing a house in the UK?
Variable: Influencing factors
Group: UK property investors/ New buyers
Among other such phrases are all classified as descriptive questions. By gathering sufficient responses to such questions, end users are able to make intelligent decisions based on hard figures that help in gathering stakeholder confidence.
For example: What percentage of college students make use of e-libraries for their academic needs. In this example the variable under observation is usage of e-libraries and the group that is evaluated are the college going students.

By providing percentages, averages, sum, proportions and other such figures, descriptive research questions provide a complete view of the target groups responses with respect to that variable. The above example has restricted the usage of variables to one, but many researchers alternatively choose to incorporate multiple variables under a single head.
Why are descriptive research questions important?
Descriptive research questions are a systematic methodology that helps in understanding the what, where, when and how. Important variables can be rigidly defined using descriptive research, unlike qualitative research where the subjectivity in responses makes it relatively difficult to get a grasp on the overall picture. The multiple methods available allow for in-person as well as online research to be carried out based on whatever the need of the end user is.
The data provided by descriptive research assists comprehensive understanding by providing an in-depth view of the variable that is being studied.
Steps to conduct Cluster Sampling
These are the following steps used to perform single-stage cluster sampling:
- Decide on a target population and desired sample size.
- Divide the target population into clusters based on a specific criteria.
- Select clusters using methods of random selection while keeping in mind the desired sample size.
- Collect data from the final sample group.
Further steps may be taken using two-stage or multistage sampling to achieve desired sample size if it cannot be achieved through one-stage sampling.

Types of descriptive research questions?
Descriptive research questions has divisions based on multiple business applications:
Market performance:
Descriptive research questions can be centred around organizational market performance in terms of sales figures, competitive appeal, updated practices, market share analytics, concept studies and other data collection processes that intend to gather market know-how. Target market analysis can also be done using descriptive question types wherein organizations can precisely define their niche audience.
Consumer behaviour:
Consumer perceptions and ideas about what suits them best can be understood using descriptive question types. These studies are used to design curated products that meet target market requirements. Anything from products, services, offers, incentives, promotions and marketing, pricing, packaging, feedback mechanism can be put into perspective and gauged to extract material results.

Internal trends:
While market performance looks at external variables, internal trends focus on departmental contributions, revenue generation, product specific demands, sales figures etc. This internal summary helps appraise performance within the organization and contrast it with external performance for benchmarking purposes.

How to frame descriptive research questions?
There is no rocket science behind framing the right question for your variable. It’s just a matter of figuring out what you want to assess and the numerical measure you’re looking for. The usage of descriptive questions in your study also comes with the condition of keeping the entire process concise and to the point.
To start off, figure out the variable that you wish to gauge and the target group that needs to be evaluated. This will determine the centre point of your research questions. Avoid providing vague descriptions and instead, try narrowing the details. Such a practice will direct the questioning to the exact audience you wish to examine without adding in unnecessary responses.
Choose the starting phrase that encompasses what you’re looking to measure. For example: If you’re looking to examine or separate a certain type of person from the entire target audience, phrases such as “what proportion” or “what percentage” can prove highly useful.
Questioning tips:
- Proceed from general to specific questions while making sure that you don’t lose focus of your target variable and audience.
- Avoid using ambiguous terminologies that are likely to confuse your respondents into misunderstanding questions as this can adversely affect the quality of your responses.
- Keep the questions simple and easy to understand in such a way that all targeted respondents are able to grasp the overall meaning equally.
- Avoid leading questions that skew the respondent into answering a certain way. Research is all about getting the information that you want in an authentic manner and such questions can sway the respondent into giving artificial responses.
Make sure that your answer choices are balanced. This is another bias that forces the respondent into altering their actual responses. Try to provide equal representation to all possible answers such that the probability of receiving each response is equally likely.
Lastly, look for variables of questions that you can club together without affecting the overall questioning process. However, it is often useful to bifurcate combined questions wherever you can, combining relevant questions together can provide useful information about existing relationships. This goes without saying that such clubbing must not act as a hindrance to the understanding of these variables as separate characteristics.
Explore Voxco Survey Software
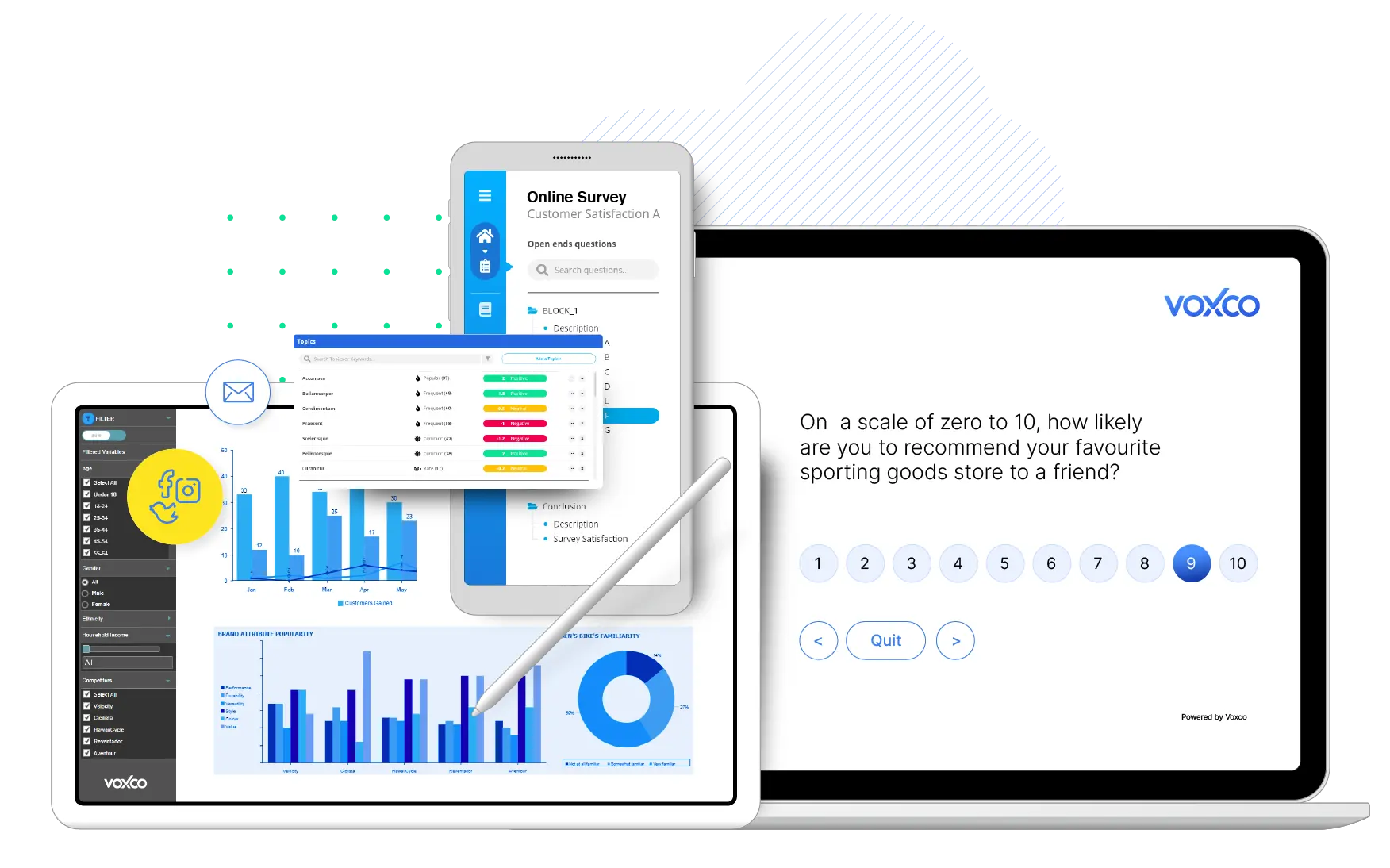
+ Omnichannel Survey Software
+ Online Survey Software
+ CATI Survey Software
+ IVR Survey Software
+ Market Research Tool
+ Customer Experience Tool
+ Product Experience Software
+ Enterprise Survey Software
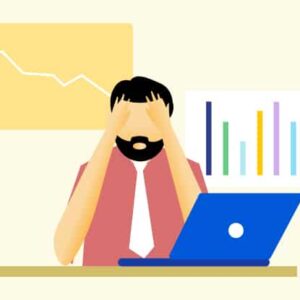
Factor analysis: Definition, sample questions
Unveiling Patterns with Factor Analysis: Definition and Sample Questions SHARE THE ARTICLE ON Table of Contents Factor analysis is a statistical technique that aids in
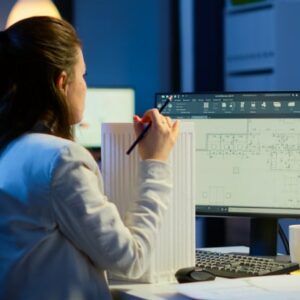
A/B Testing Experimental Design
A/B Testing Experimental Design SHARE THE ARTICLE ON Table of Contents What is an A/B Testing Experimental Design? Simply put, A/B testing is a way
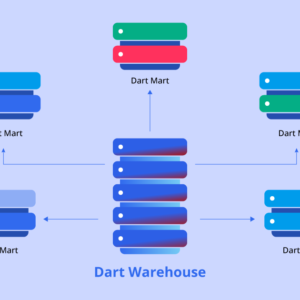
The Key Differences between a Data Mart and a Data Warehouse
The Key Differences between a Data Mart and a Data Warehouse SHARE THE ARTICLE ON Table of Contents Introduction Large organizations are constantly collecting and
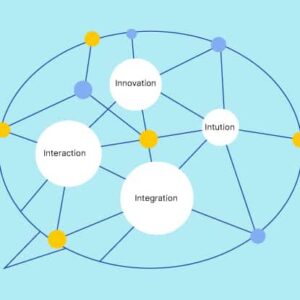
What is Digital Customer Experience?
What is Digital Customer Experience? SHARE THE ARTICLE ON Table of Contents With 4.75 billion people using the internet and 28% of adults staying online
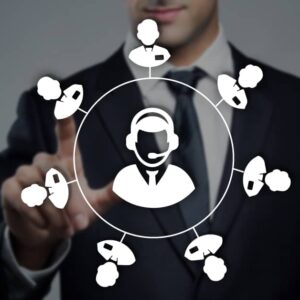
Call centers: How to build a successful one
Call centers: How to build a successful one SHARE THE ARTICLE ON Table of Contents Businesses around the world run on customer support and customer

What NPS Stands For ?
What does NPS® stand for? Free Download: Enhance NPS® Scores using our NPS® Survey Templates Download Now SHARE THE ARTICLE ON What is NPS® (Net
We use cookies in our website to give you the best browsing experience and to tailor advertising. By continuing to use our website, you give us consent to the use of cookies. Read More
Name | Domain | Purpose | Expiry | Type |
---|---|---|---|---|
hubspotutk | www.voxco.com | HubSpot functional cookie. | 1 year | HTTP |
lhc_dir_locale | amplifyreach.com | --- | 52 years | --- |
lhc_dirclass | amplifyreach.com | --- | 52 years | --- |
Name | Domain | Purpose | Expiry | Type |
---|---|---|---|---|
_fbp | www.voxco.com | Facebook Pixel advertising first-party cookie | 3 months | HTTP |
__hstc | www.voxco.com | Hubspot marketing platform cookie. | 1 year | HTTP |
__hssrc | www.voxco.com | Hubspot marketing platform cookie. | 52 years | HTTP |
__hssc | www.voxco.com | Hubspot marketing platform cookie. | Session | HTTP |
Name | Domain | Purpose | Expiry | Type |
---|---|---|---|---|
_gid | www.voxco.com | Google Universal Analytics short-time unique user tracking identifier. | 1 days | HTTP |
MUID | bing.com | Microsoft User Identifier tracking cookie used by Bing Ads. | 1 year | HTTP |
MR | bat.bing.com | Microsoft User Identifier tracking cookie used by Bing Ads. | 7 days | HTTP |
IDE | doubleclick.net | Google advertising cookie used for user tracking and ad targeting purposes. | 2 years | HTTP |
_vwo_uuid_v2 | www.voxco.com | Generic Visual Website Optimizer (VWO) user tracking cookie. | 1 year | HTTP |
_vis_opt_s | www.voxco.com | Generic Visual Website Optimizer (VWO) user tracking cookie that detects if the user is new or returning to a particular campaign. | 3 months | HTTP |
_vis_opt_test_cookie | www.voxco.com | A session (temporary) cookie used by Generic Visual Website Optimizer (VWO) to detect if the cookies are enabled on the browser of the user or not. | 52 years | HTTP |
_ga | www.voxco.com | Google Universal Analytics long-time unique user tracking identifier. | 2 years | HTTP |
_uetsid | www.voxco.com | Microsoft Bing Ads Universal Event Tracking (UET) tracking cookie. | 1 days | HTTP |
vuid | vimeo.com | Vimeo tracking cookie | 2 years | HTTP |
Name | Domain | Purpose | Expiry | Type |
---|---|---|---|---|
__cf_bm | hubspot.com | Generic CloudFlare functional cookie. | Session | HTTP |
Name | Domain | Purpose | Expiry | Type |
---|---|---|---|---|
_gcl_au | www.voxco.com | --- | 3 months | --- |
_gat_gtag_UA_3262734_1 | www.voxco.com | --- | Session | --- |
_clck | www.voxco.com | --- | 1 year | --- |
_ga_HNFQQ528PZ | www.voxco.com | --- | 2 years | --- |
_clsk | www.voxco.com | --- | 1 days | --- |
visitor_id18452 | pardot.com | --- | 10 years | --- |
visitor_id18452-hash | pardot.com | --- | 10 years | --- |
lpv18452 | pi.pardot.com | --- | Session | --- |
lhc_per | www.voxco.com | --- | 6 months | --- |
_uetvid | www.voxco.com | --- | 1 year | --- |
Looking for the best research tools?
Voxco offers the best online & offline survey research tools!

Quant Analysis 101: Descriptive Statistics
Everything You Need To Get Started (With Examples)
By: Derek Jansen (MBA) | Reviewers: Kerryn Warren (PhD) | October 2023
If you’re new to quantitative data analysis , one of the first terms you’re likely to hear being thrown around is descriptive statistics. In this post, we’ll unpack the basics of descriptive statistics, using straightforward language and loads of examples . So grab a cup of coffee and let’s crunch some numbers!
Overview: Descriptive Statistics
What are descriptive statistics.
- Descriptive vs inferential statistics
- Why the descriptives matter
- The “ Big 7 ” descriptive statistics
- Key takeaways
At the simplest level, descriptive statistics summarise and describe relatively basic but essential features of a quantitative dataset – for example, a set of survey responses. They provide a snapshot of the characteristics of your dataset and allow you to better understand, roughly, how the data are “shaped” (more on this later). For example, a descriptive statistic could include the proportion of males and females within a sample or the percentages of different age groups within a population.
Another common descriptive statistic is the humble average (which in statistics-talk is called the mean ). For example, if you undertook a survey and asked people to rate their satisfaction with a particular product on a scale of 1 to 10, you could then calculate the average rating. This is a very basic statistic, but as you can see, it gives you some idea of how this data point is shaped .
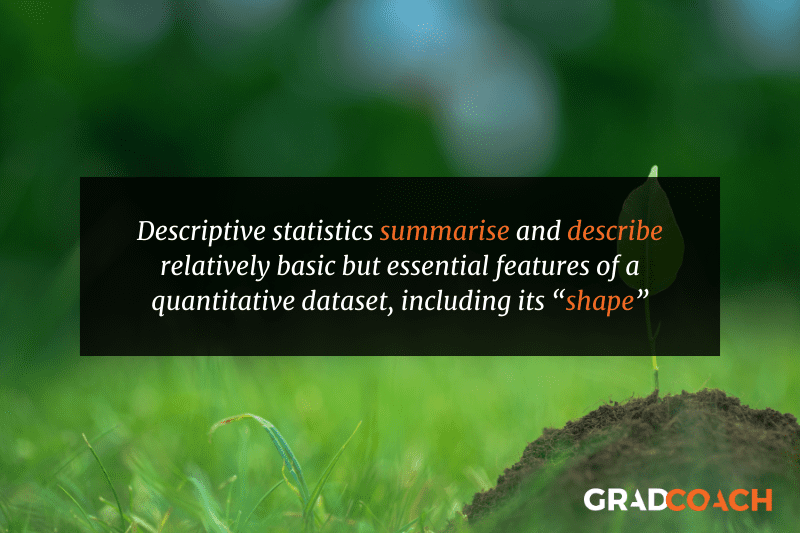
What about inferential statistics?
Now, you may have also heard the term inferential statistics being thrown around, and you’re probably wondering how that’s different from descriptive statistics. Simply put, descriptive statistics describe and summarise the sample itself , while inferential statistics use the data from a sample to make inferences or predictions about a population .
Put another way, descriptive statistics help you understand your dataset , while inferential statistics help you make broader statements about the population , based on what you observe within the sample. If you’re keen to learn more, we cover inferential stats in another post , or you can check out the explainer video below.
Why do descriptive statistics matter?
While descriptive statistics are relatively simple from a mathematical perspective, they play a very important role in any research project . All too often, students skim over the descriptives and run ahead to the seemingly more exciting inferential statistics, but this can be a costly mistake.
The reason for this is that descriptive statistics help you, as the researcher, comprehend the key characteristics of your sample without getting lost in vast amounts of raw data. In doing so, they provide a foundation for your quantitative analysis . Additionally, they enable you to quickly identify potential issues within your dataset – for example, suspicious outliers, missing responses and so on. Just as importantly, descriptive statistics inform the decision-making process when it comes to choosing which inferential statistics you’ll run, as each inferential test has specific requirements regarding the shape of the data.
Long story short, it’s essential that you take the time to dig into your descriptive statistics before looking at more “advanced” inferentials. It’s also worth noting that, depending on your research aims and questions, descriptive stats may be all that you need in any case . So, don’t discount the descriptives!
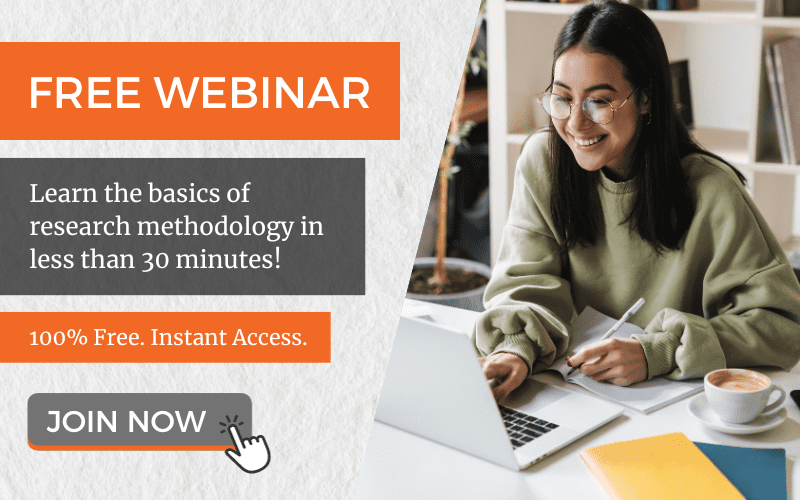
The “Big 7” descriptive statistics
With the what and why out of the way, let’s take a look at the most common descriptive statistics. Beyond the counts, proportions and percentages we mentioned earlier, we have what we call the “Big 7” descriptives. These can be divided into two categories – measures of central tendency and measures of dispersion.
Measures of central tendency
True to the name, measures of central tendency describe the centre or “middle section” of a dataset. In other words, they provide some indication of what a “typical” data point looks like within a given dataset. The three most common measures are:
The mean , which is the mathematical average of a set of numbers – in other words, the sum of all numbers divided by the count of all numbers.
The median , which is the middlemost number in a set of numbers, when those numbers are ordered from lowest to highest.
The mode , which is the most frequently occurring number in a set of numbers (in any order). Naturally, a dataset can have one mode, no mode (no number occurs more than once) or multiple modes.
To make this a little more tangible, let’s look at a sample dataset, along with the corresponding mean, median and mode. This dataset reflects the service ratings (on a scale of 1 – 10) from 15 customers.
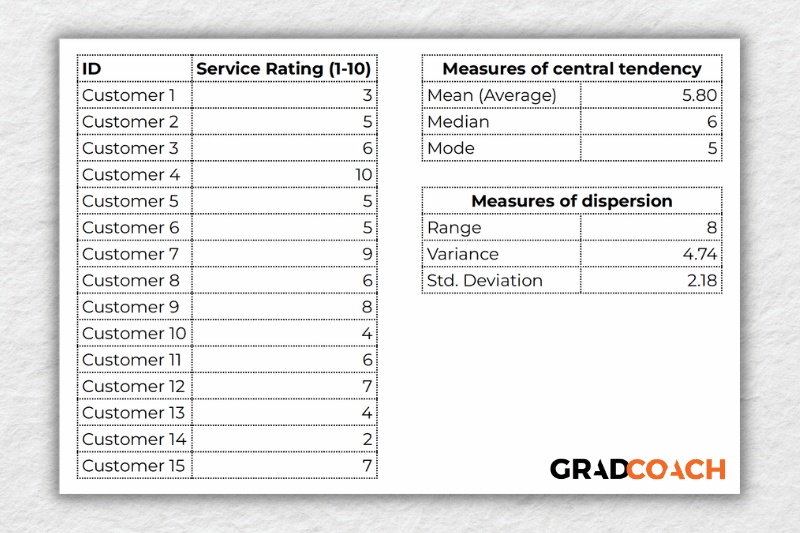
As you can see, the mean of 5.8 is the average rating across all 15 customers. Meanwhile, 6 is the median . In other words, if you were to list all the responses in order from low to high, Customer 8 would be in the middle (with their service rating being 6). Lastly, the number 5 is the most frequent rating (appearing 3 times), making it the mode.
Together, these three descriptive statistics give us a quick overview of how these customers feel about the service levels at this business. In other words, most customers feel rather lukewarm and there’s certainly room for improvement. From a more statistical perspective, this also means that the data tend to cluster around the 5-6 mark , since the mean and the median are fairly close to each other.
To take this a step further, let’s look at the frequency distribution of the responses . In other words, let’s count how many times each rating was received, and then plot these counts onto a bar chart.
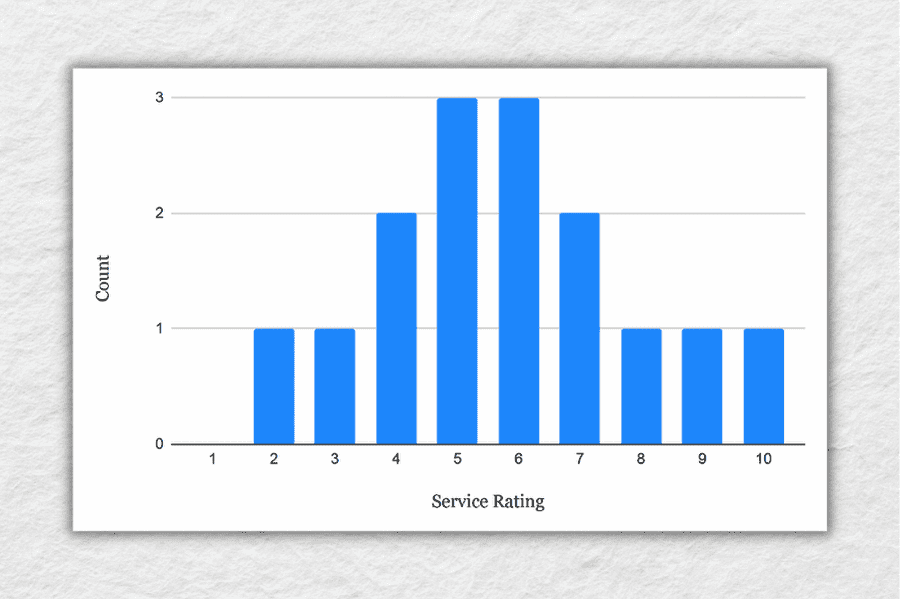
As you can see, the responses tend to cluster toward the centre of the chart , creating something of a bell-shaped curve. In statistical terms, this is called a normal distribution .
As you delve into quantitative data analysis, you’ll find that normal distributions are very common , but they’re certainly not the only type of distribution. In some cases, the data can lean toward the left or the right of the chart (i.e., toward the low end or high end). This lean is reflected by a measure called skewness , and it’s important to pay attention to this when you’re analysing your data, as this will have an impact on what types of inferential statistics you can use on your dataset.
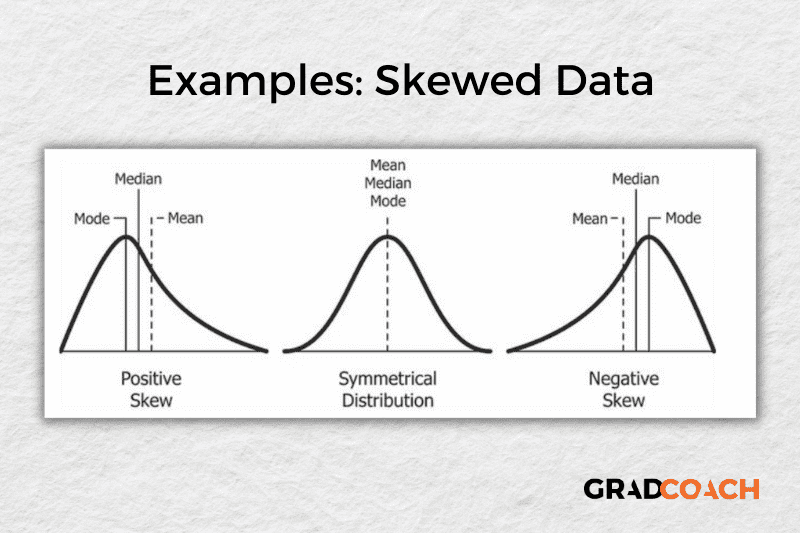
Measures of dispersion
While the measures of central tendency provide insight into how “centred” the dataset is, it’s also important to understand how dispersed that dataset is . In other words, to what extent the data cluster toward the centre – specifically, the mean. In some cases, the majority of the data points will sit very close to the centre, while in other cases, they’ll be scattered all over the place. Enter the measures of dispersion, of which there are three:
Range , which measures the difference between the largest and smallest number in the dataset. In other words, it indicates how spread out the dataset really is.
Variance , which measures how much each number in a dataset varies from the mean (average). More technically, it calculates the average of the squared differences between each number and the mean. A higher variance indicates that the data points are more spread out , while a lower variance suggests that the data points are closer to the mean.
Standard deviation , which is the square root of the variance . It serves the same purposes as the variance, but is a bit easier to interpret as it presents a figure that is in the same unit as the original data . You’ll typically present this statistic alongside the means when describing the data in your research.
Again, let’s look at our sample dataset to make this all a little more tangible.
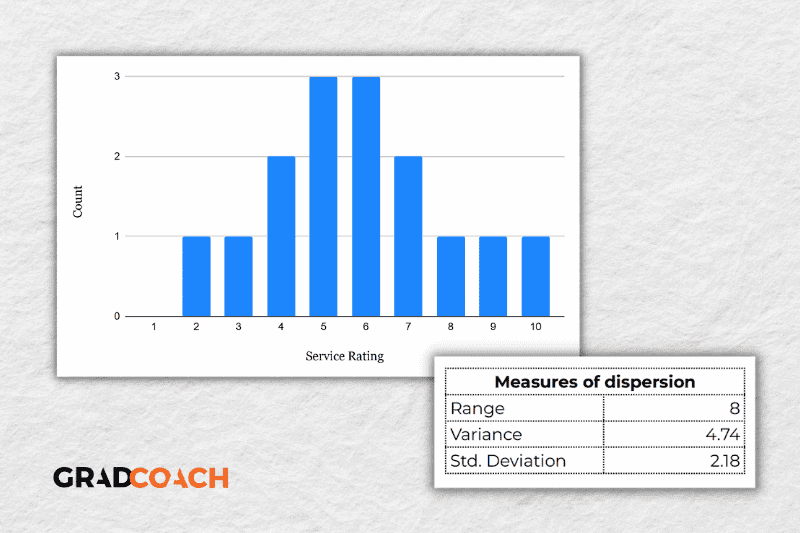
As you can see, the range of 8 reflects the difference between the highest rating (10) and the lowest rating (2). The standard deviation of 2.18 tells us that on average, results within the dataset are 2.18 away from the mean (of 5.8), reflecting a relatively dispersed set of data .
For the sake of comparison, let’s look at another much more tightly grouped (less dispersed) dataset.
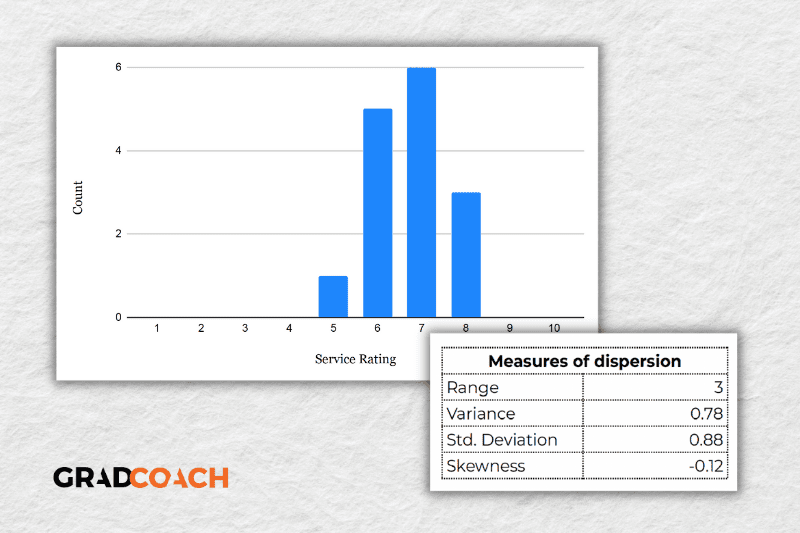
As you can see, all the ratings lay between 5 and 8 in this dataset, resulting in a much smaller range, variance and standard deviation . You might also notice that the data are clustered toward the right side of the graph – in other words, the data are skewed. If we calculate the skewness for this dataset, we get a result of -0.12, confirming this right lean.
In summary, range, variance and standard deviation all provide an indication of how dispersed the data are . These measures are important because they help you interpret the measures of central tendency within context . In other words, if your measures of dispersion are all fairly high numbers, you need to interpret your measures of central tendency with some caution , as the results are not particularly centred. Conversely, if the data are all tightly grouped around the mean (i.e., low dispersion), the mean becomes a much more “meaningful” statistic).
Key Takeaways
We’ve covered quite a bit of ground in this post. Here are the key takeaways:
- Descriptive statistics, although relatively simple, are a critically important part of any quantitative data analysis.
- Measures of central tendency include the mean (average), median and mode.
- Skewness indicates whether a dataset leans to one side or another
- Measures of dispersion include the range, variance and standard deviation
If you’d like hands-on help with your descriptive statistics (or any other aspect of your research project), check out our private coaching service , where we hold your hand through each step of the research journey.
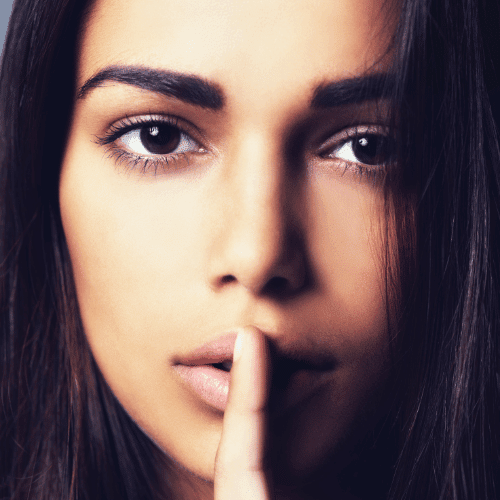
Psst… there’s more!
This post is an extract from our bestselling short course, Methodology Bootcamp . If you want to work smart, you don't want to miss this .
Good day. May I ask about where I would be able to find the statistics cheat sheet?
Right above you comment 🙂
Good job. you saved me
Brilliant and well explained. So much information explained clearly!
Submit a Comment Cancel reply
Your email address will not be published. Required fields are marked *
Save my name, email, and website in this browser for the next time I comment.
- Print Friendly
Root out friction in every digital experience, super-charge conversion rates, and optimize digital self-service
Uncover insights from any interaction, deliver AI-powered agent coaching, and reduce cost to serve
Increase revenue and loyalty with real-time insights and recommendations delivered to teams on the ground
Know how your people feel and empower managers to improve employee engagement, productivity, and retention
Take action in the moments that matter most along the employee journey and drive bottom line growth
Whatever they’re saying, wherever they’re saying it, know exactly what’s going on with your people
Get faster, richer insights with qual and quant tools that make powerful market research available to everyone
Run concept tests, pricing studies, prototyping + more with fast, powerful studies designed by UX research experts
Track your brand performance 24/7 and act quickly to respond to opportunities and challenges in your market
Explore the platform powering Experience Management
- Free Account
- Product Demos
- For Digital
- For Customer Care
- For Human Resources
- For Researchers
- Financial Services
- All Industries
Popular Use Cases
- Customer Experience
- Employee Experience
- Net Promoter Score
- Voice of Customer
- Customer Success Hub
- Product Documentation
- Training & Certification
- XM Institute
- Popular Resources
- Customer Stories
- Artificial Intelligence
Market Research
- Partnerships
- Marketplace
The annual gathering of the experience leaders at the world’s iconic brands building breakthrough business results, live in Salt Lake City.
- English/AU & NZ
- Español/Europa
- Español/América Latina
- Português Brasileiro
- REQUEST DEMO
- Experience Management
- Descriptive Statistics
Try Qualtrics for free
Descriptive statistics in research: a critical component of data analysis.
15 min read With any data, the object is to describe the population at large, but what does that mean and what processes, methods and measures are used to uncover insights from that data? In this short guide, we explore descriptive statistics and how it’s applied to research.
What do we mean by descriptive statistics?
With any kind of data, the main objective is to describe a population at large — and using descriptive statistics, researchers can quantify and describe the basic characteristics of a given data set.
For example, researchers can condense large data sets, which may contain thousands of individual data points or observations, into a series of statistics that provide useful information on the population of interest. We call this process “describing data”.
In the process of producing summaries of the sample, we use measures like mean, median, variance, graphs, charts, frequencies, histograms, box and whisker plots, and percentages. For datasets with just one variable, we use univariate descriptive statistics. For datasets with multiple variables, we use bivariate correlation and multivariate descriptive statistics.
Want to find out the definitions?
Univariate descriptive statistics: this is when you want to describe data with only one characteristic or attribute
Bivariate correlation: this is when you simultaneously analyze (compare) two variables to see if there is a relationship between them
Multivariate descriptive statistics: this is a subdivision of statistics encompassing the simultaneous observation and analysis of more than one outcome variable
Then, after describing and summarizing the data, as well as using simple graphical analyses, we can start to draw meaningful insights from it to help guide specific strategies. It’s also important to note that descriptive statistics can employ and use both quantitative and qualitative research .
Describing data is undoubtedly the most critical first step in research as it enables the subsequent organization, simplification and summarization of information — and every survey question and population has summary statistics. Let’s take a look at a few examples.
Examples of descriptive statistics
Consider for a moment a number used to summarize how well a striker is performing in football — goals scored per game. This number is simply the number of shots taken against how many of those shots hit the back of the net (reported to three significant digits). If a striker is scoring 0.333, that’s one goal for every three shots. If they’re scoring one in four, that’s 0.250.
A classic example is a student’s grade point average (GPA). This single number describes the general performance of a student across a range of course experiences and classes. It doesn’t tell us anything about the difficulty of the courses the student is taking, or what those courses are, but it does provide a summary that enables a degree of comparison with people or other units of data.
Ultimately, descriptive statistics make it incredibly easy for people to understand complex (or data intensive) quantitative or qualitative insights across large data sets.
Take your research to the next level with XM for Strategy & Research
Types of descriptive statistics
To quantitatively summarize the characteristics of raw, ungrouped data, we use the following types of descriptive statistics:
- Measures of Central Tendency ,
- Measures of Dispersion and
- Measures of Frequency Distribution.
Following the application of any of these approaches, the raw data then becomes ‘grouped’ data that’s logically organized and easy to understand. To visually represent the data, we then use graphs, charts, tables etc.
Let’s look at the different types of measurement and the statistical methods that belong to each:
Measures of Central Tendency are used to describe data by determining a single representative of central value. For example, the mean, median or mode.
Measures of Dispersion are used to determine how spread out a data distribution is with respect to the central value, e.g. the mean, median or mode. For example, while central tendency gives the person the average or central value, it doesn’t describe how the data is distributed within the set.
Measures of Frequency Distribution are used to describe the occurrence of data within the data set (count).
The methods of each measure are summarized in the table below:
Measures of Central Tendency | Measures of Dispersion | Measures of Frequency Distribution |
---|---|---|
Mean | Range | Count |
Median | Standard deviation | |
Mode | Quartile deviation | |
Variance | ||
Absolute deviation |
Mean: The most popular and well-known measure of central tendency. The mean is equal to the sum of all the values in the data set divided by the number of values in the data set.
Median: The median is the middle score for a set of data that has been arranged in order of magnitude. If you have an even number of data, e.g. 10 data points, take the two middle scores and average the result.
Mode: The mode is the most frequently occurring observation in the data set.
Range: The difference between the highest and lowest value.
Standard deviation: Standard deviation measures the dispersion of a data set relative to its mean and is calculated as the square root of the variance.
Quartile deviation : Quartile deviation measures the deviation in the middle of the data.
Variance: Variance measures the variability from the average of mean.
Absolute deviation: The absolute deviation of a dataset is the average distance between each data point and the mean.
Count: How often each value occurs.
Scope of descriptive statistics in research
Descriptive statistics (or analysis) is considered more vast than other quantitative and qualitative methods as it provides a much broader picture of an event, phenomenon or population.
But that’s not all: it can use any number of variables, and as it collects data and describes it as it is, it’s also far more representative of the world as it exists.
However, it’s also important to consider that descriptive analyses lay the foundation for further methods of study. By summarizing and condensing the data into easily understandable segments, researchers can further analyze the data to uncover new variables or hypotheses.
Mostly, this practice is all about the ease of data visualization. With data presented in a meaningful way, researchers have a simplified interpretation of the data set in question. That said, while descriptive statistics helps to summarize information, it only provides a general view of the variables in question.
It is, therefore, up to the researchers to probe further and use other methods of analysis to discover deeper insights.
Things you can do with descriptive statistics
Define subject characteristics
If a marketing team wanted to build out accurate buyer personas for specific products and industry verticals, they could use descriptive analyses on customer datasets (procured via a survey) to identify consistent traits and behaviors.
They could then ‘describe’ the data to build a clear picture and understanding of who their buyers are, including things like preferences, business challenges, income and so on.
Measure data trends
Let’s say you wanted to assess propensity to buy over several months or years for a specific target market and product. With descriptive statistics, you could quickly summarize the data and extract the precise data points you need to understand the trends in product purchase behavior.
Compare events, populations or phenomena
How do different demographics respond to certain variables? For example, you might want to run a customer study to see how buyers in different job functions respond to new product features or price changes. Are all groups as enthusiastic about the new features and likely to buy? Or do they have reservations? This kind of data will help inform your overall product strategy and potentially how you tier solutions.
Validate existing conditions
When you have a belief or hypothesis but need to prove it, you can use descriptive techniques to ascertain underlying patterns or assumptions.
Form new hypotheses
With the data presented and surmised in a way that everyone can understand (and infer connections from), you can delve deeper into specific data points to uncover deeper and more meaningful insights — or run more comprehensive research.
Guiding your survey design to improve the data collected
To use your surveys as an effective tool for customer engagement and understanding, every survey goal and item should answer one simple, yet highly important question:
What am I really asking?
It might seem trivial, but by having this question frame survey research, it becomes significantly easier for researchers to develop the right questions that uncover useful, meaningful and actionable insights.
Planning becomes easier, questions clearer and perspective far wider and yet nuanced.
Hypothesize – what’s the problem that you’re trying to solve? Far too often, organizations collect data without understanding what they’re asking, and why they’re asking it.
Finally, focus on the end result. What kind of data do you need to answer your question? Also, are you asking a quantitative or qualitative question? Here are a few things to consider:
- Clear questions are clear for everyone. It takes time to make a concept clear
- Ask about measurable, evident and noticeable activities or behaviors.
- Make rating scales easy. Avoid long lists, confusing scales or “don’t know” or “not applicable” options.
- Ensure your survey makes sense and flows well. Reduce the cognitive load on respondents by making it easy for them to complete the survey.
- Read your questions aloud to see how they sound.
- Pretest by asking a few uninvolved individuals to answer.
Furthermore…
As well as understanding what you’re really asking, there are several other considerations for your data:
Keep it random
How you select your sample is what makes your research replicable and meaningful. Having a truly random sample helps prevent bias, increasingly the quality of evidence you find.
Plan for and avoid sample error
Before starting your research project, have a clear plan for avoiding sample error. Use larger sample sizes, and apply random sampling to minimize the potential for bias.
Don’t over sample
Remember, you can sample 500 respondents selected randomly from a population and they will closely reflect the actual population 95% of the time.
Think about the mode
Match your survey methods to the sample you select. For example, how do your current customers prefer communicating? Do they have any shared characteristics or preferences? A mixed-method approach is critical if you want to drive action across different customer segments.
Use a survey tool that supports you with the whole process
Surveys created using a survey research software can support researchers in a number of ways:
- Employee satisfaction survey template
- Employee exit survey template
- Customer satisfaction (CSAT) survey template
- Ad testing survey template
- Brand awareness survey template
- Product pricing survey template
- Product research survey template
- Employee engagement survey template
- Customer service survey template
- NPS survey template
- Product package testing survey template
- Product features prioritization survey template
These considerations have been included in Qualtrics’ survey software , which summarizes and creates visualizations of data, making it easy to access insights, measure trends, and examine results without complexity or jumping between systems.
Uncover your next breakthrough idea with Stats iQ™
What makes Qualtrics so different from other survey providers is that it is built in consultation with trained research professionals and includes high-tech statistical software like Qualtrics Stats iQ .
With just a click, the software can run specific analyses or automate statistical testing and data visualization. Testing parameters are automatically chosen based on how your data is structured (e.g. categorical data will run a statistical test like Chi-squared), and the results are translated into plain language that anyone can understand and put into action.
Get more meaningful insights from your data
Stats iQ includes a variety of statistical analyses, including: describe, relate, regression, cluster, factor, TURF, and pivot tables — all in one place!
Confidently analyze complex data
Built-in artificial intelligence and advanced algorithms automatically choose and apply the right statistical analyses and return the insights in plain english so everyone can take action.
Integrate existing statistical workflows
For more experienced stats users, built-in R code templates allow you to run even more sophisticated analyses by adding R code snippets directly in your survey analysis.
Advanced statistical analysis methods available in Stats iQ
Regression analysis – Measures the degree of influence of independent variables on a dependent variable (the relationship between two or multiple variables).
Analysis of Variance (ANOVA) test – Commonly used with a regression study to find out what effect independent variables have on the dependent variable. It can compare multiple groups simultaneously to see if there is a relationship between them.
Conjoint analysis – Asks people to make trade-offs when making decisions, then analyses the results to give the most popular outcome. Helps you understand why people make the complex choices they do.
T-Test – Helps you compare whether two data groups have different mean values and allows the user to interpret whether differences are meaningful or merely coincidental.
Crosstab analysis – Used in quantitative market research to analyze categorical data – that is, variables that are different and mutually exclusive, and allows you to compare the relationship between two variables in contingency tables.
Go from insights to action
Now that you have a better understanding of descriptive statistics in research and how you can leverage statistical analysis methods correctly, now’s the time to utilize a tool that can take your research and subsequent analysis to the next level.
Try out a Qualtrics survey software demo so you can see how it can take you through descriptive research and further research projects from start to finish.
Related resources
Mixed methods research 17 min read, market intelligence 10 min read, marketing insights 11 min read, ethnographic research 11 min read, qualitative vs quantitative research 13 min read, qualitative research questions 11 min read, qualitative research design 12 min read, request demo.
Ready to learn more about Qualtrics?
Have a thesis expert improve your writing
Check your thesis for plagiarism in 10 minutes, generate your apa citations for free.
- Knowledge Base
Descriptive Statistics | Definitions, Types, Examples
Published on 4 November 2022 by Pritha Bhandari . Revised on 9 January 2023.
Descriptive statistics summarise and organise characteristics of a data set. A data set is a collection of responses or observations from a sample or entire population .
In quantitative research , after collecting data, the first step of statistical analysis is to describe characteristics of the responses, such as the average of one variable (e.g., age), or the relation between two variables (e.g., age and creativity).
The next step is inferential statistics , which help you decide whether your data confirms or refutes your hypothesis and whether it is generalisable to a larger population.
Table of contents
Types of descriptive statistics, frequency distribution, measures of central tendency, measures of variability, univariate descriptive statistics, bivariate descriptive statistics, frequently asked questions.
There are 3 main types of descriptive statistics:
- The distribution concerns the frequency of each value.
- The central tendency concerns the averages of the values.
- The variability or dispersion concerns how spread out the values are.
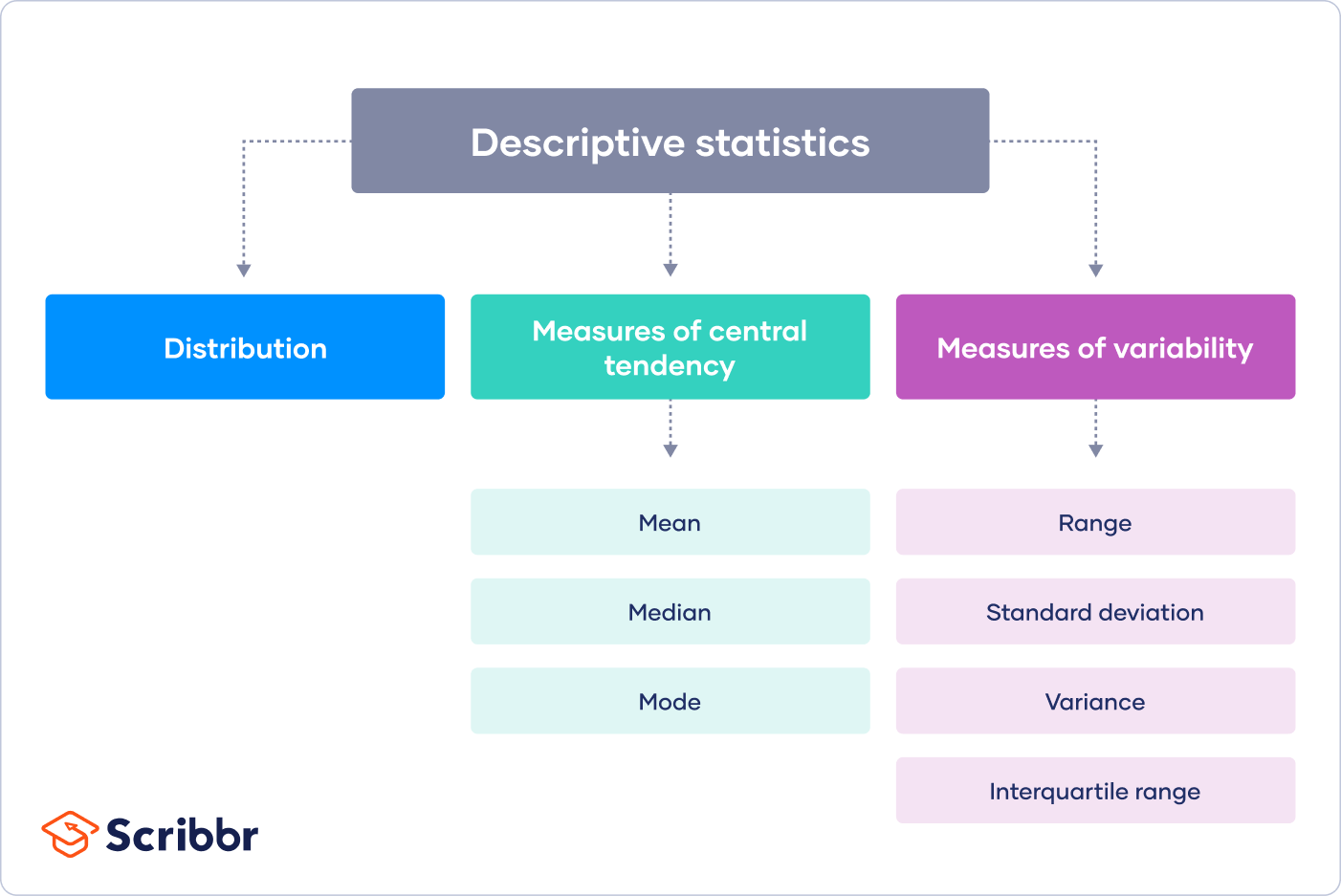
You can apply these to assess only one variable at a time, in univariate analysis, or to compare two or more, in bivariate and multivariate analysis.
- Go to a library
- Watch a movie at a theater
- Visit a national park
A data set is made up of a distribution of values, or scores. In tables or graphs, you can summarise the frequency of every possible value of a variable in numbers or percentages.
- Simple frequency distribution table
- Grouped frequency distribution table
Gender | Number |
---|---|
Male | 182 |
Female | 235 |
Other | 27 |
From this table, you can see that more women than men or people with another gender identity took part in the study. In a grouped frequency distribution, you can group numerical response values and add up the number of responses for each group. You can also convert each of these numbers to percentages.
Library visits in the past year | Percent |
---|---|
0–4 | 6% |
5–8 | 20% |
9–12 | 42% |
13–16 | 24% |
17+ | 8% |
Measures of central tendency estimate the center, or average, of a data set. The mean , median and mode are 3 ways of finding the average.
Here we will demonstrate how to calculate the mean, median, and mode using the first 6 responses of our survey.
The mean , or M , is the most commonly used method for finding the average.
To find the mean, simply add up all response values and divide the sum by the total number of responses. The total number of responses or observations is called N .
Data set | 15, 3, 12, 0, 24, 3 |
---|---|
Sum of all values | 15 + 3 + 12 + 0 + 24 + 3 = 57 |
Total number of responses | = 6 |
Mean | Divide the sum of values by to find : 57/6 = |
The median is the value that’s exactly in the middle of a data set.
To find the median, order each response value from the smallest to the biggest. Then, the median is the number in the middle. If there are two numbers in the middle, find their mean.
Ordered data set | 0, 3, 3, 12, 15, 24 |
---|---|
Middle numbers | 3, 12 |
Median | Find the mean of the two middle numbers: (3 + 12)/2 = |
The mode is the simply the most popular or most frequent response value. A data set can have no mode, one mode, or more than one mode.
To find the mode, order your data set from lowest to highest and find the response that occurs most frequently.
Ordered data set | 0, 3, 3, 12, 15, 24 |
---|---|
Mode | Find the most frequently occurring response: |
Measures of variability give you a sense of how spread out the response values are. The range, standard deviation and variance each reflect different aspects of spread.
The range gives you an idea of how far apart the most extreme response scores are. To find the range , simply subtract the lowest value from the highest value.
Standard deviation
The standard deviation ( s ) is the average amount of variability in your dataset. It tells you, on average, how far each score lies from the mean. The larger the standard deviation, the more variable the data set is.
There are six steps for finding the standard deviation:
- List each score and find their mean.
- Subtract the mean from each score to get the deviation from the mean.
- Square each of these deviations.
- Add up all of the squared deviations.
- Divide the sum of the squared deviations by N – 1.
- Find the square root of the number you found.
Raw data | Deviation from mean | Squared deviation |
---|---|---|
15 | 15 – 9.5 = 5.5 | 30.25 |
3 | 3 – 9.5 = -6.5 | 42.25 |
12 | 12 – 9.5 = 2.5 | 6.25 |
0 | 0 – 9.5 = -9.5 | 90.25 |
24 | 24 – 9.5 = 14.5 | 210.25 |
3 | 3 – 9.5 = -6.5 | 42.25 |
= 9.5 | Sum = 0 | Sum of squares = 421.5 |
Step 5: 421.5/5 = 84.3
Step 6: √84.3 = 9.18
The variance is the average of squared deviations from the mean. Variance reflects the degree of spread in the data set. The more spread the data, the larger the variance is in relation to the mean.
To find the variance, simply square the standard deviation. The symbol for variance is s 2 .
Univariate descriptive statistics focus on only one variable at a time. It’s important to examine data from each variable separately using multiple measures of distribution, central tendency and spread. Programs like SPSS and Excel can be used to easily calculate these.
Visits to the library | |
---|---|
6 | |
Mean | 9.5 |
Median | 7.5 |
Mode | 3 |
Standard deviation | 9.18 |
Variance | 84.3 |
Range | 24 |
If you were to only consider the mean as a measure of central tendency, your impression of the ‘middle’ of the data set can be skewed by outliers, unlike the median or mode.
Likewise, while the range is sensitive to extreme values, you should also consider the standard deviation and variance to get easily comparable measures of spread.
If you’ve collected data on more than one variable, you can use bivariate or multivariate descriptive statistics to explore whether there are relationships between them.
In bivariate analysis, you simultaneously study the frequency and variability of two variables to see if they vary together. You can also compare the central tendency of the two variables before performing further statistical tests .
Multivariate analysis is the same as bivariate analysis but with more than two variables.
Contingency table
In a contingency table, each cell represents the intersection of two variables. Usually, an independent variable (e.g., gender) appears along the vertical axis and a dependent one appears along the horizontal axis (e.g., activities). You read ‘across’ the table to see how the independent and dependent variables relate to each other.
Number of visits to the library in the past year | |||||
---|---|---|---|---|---|
Group | 0–4 | 5–8 | 9–12 | 13–16 | 17+ |
Children | 32 | 68 | 37 | 23 | 22 |
Adults | 36 | 48 | 43 | 83 | 25 |
Interpreting a contingency table is easier when the raw data is converted to percentages. Percentages make each row comparable to the other by making it seem as if each group had only 100 observations or participants. When creating a percentage-based contingency table, you add the N for each independent variable on the end.
Visits to the library in the past year (Percentages) | ||||||
---|---|---|---|---|---|---|
Group | 0–4 | 5–8 | 9–12 | 13–16 | 17+ | |
Children | 18% | 37% | 20% | 13% | 12% | 182 |
Adults | 15% | 20% | 18% | 35% | 11% | 235 |
From this table, it is more clear that similar proportions of children and adults go to the library over 17 times a year. Additionally, children most commonly went to the library between 5 and 8 times, while for adults, this number was between 13 and 16.
Scatter plots
A scatter plot is a chart that shows you the relationship between two or three variables. It’s a visual representation of the strength of a relationship.
In a scatter plot, you plot one variable along the x-axis and another one along the y-axis. Each data point is represented by a point in the chart.
From your scatter plot, you see that as the number of movies seen at movie theaters increases, the number of visits to the library decreases. Based on your visual assessment of a possible linear relationship, you perform further tests of correlation and regression.
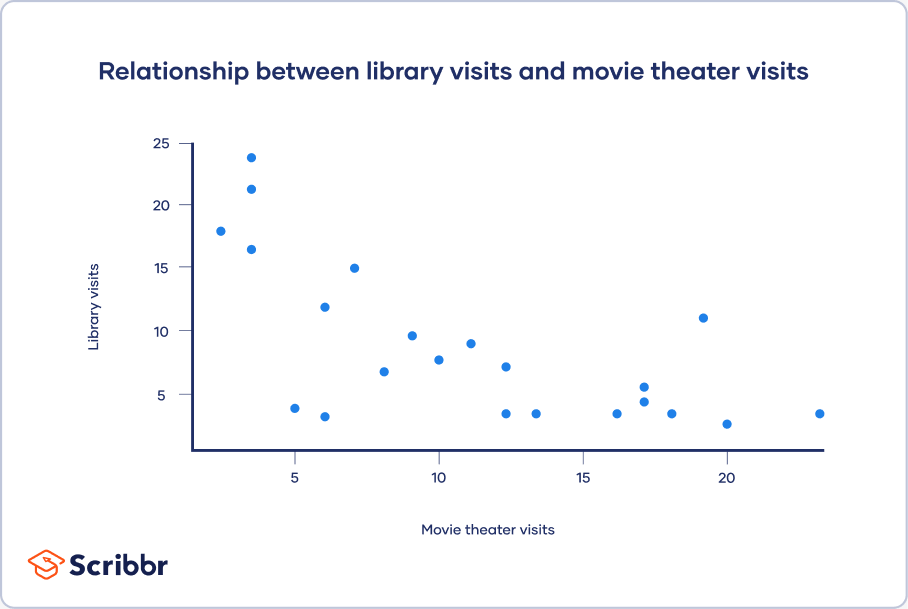
Descriptive statistics summarise the characteristics of a data set. Inferential statistics allow you to test a hypothesis or assess whether your data is generalisable to the broader population.
The 3 main types of descriptive statistics concern the frequency distribution, central tendency, and variability of a dataset.
- Distribution refers to the frequencies of different responses.
- Measures of central tendency give you the average for each response.
- Measures of variability show you the spread or dispersion of your dataset.
- Univariate statistics summarise only one variable at a time.
- Bivariate statistics compare two variables .
- Multivariate statistics compare more than two variables .
Cite this Scribbr article
If you want to cite this source, you can copy and paste the citation or click the ‘Cite this Scribbr article’ button to automatically add the citation to our free Reference Generator.
Bhandari, P. (2023, January 09). Descriptive Statistics | Definitions, Types, Examples. Scribbr. Retrieved 9 September 2024, from https://www.scribbr.co.uk/stats/descriptive-statistics-explained/
Is this article helpful?
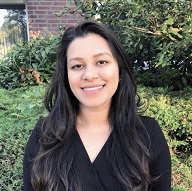
Pritha Bhandari
Other students also liked, data collection methods | step-by-step guide & examples, variability | calculating range, iqr, variance, standard deviation, normal distribution | examples, formulas, & uses.
Warning: The NCBI web site requires JavaScript to function. more...

An official website of the United States government
The .gov means it's official. Federal government websites often end in .gov or .mil. Before sharing sensitive information, make sure you're on a federal government site.
The site is secure. The https:// ensures that you are connecting to the official website and that any information you provide is encrypted and transmitted securely.
- Publications
- Account settings
- Browse Titles
NCBI Bookshelf. A service of the National Library of Medicine, National Institutes of Health.
StatPearls [Internet]. Treasure Island (FL): StatPearls Publishing; 2024 Jan-.

StatPearls [Internet].
Exploratory data analysis: frequencies, descriptive statistics, histograms, and boxplots.
Jacob Shreffler ; Martin R. Huecker .
Affiliations
Last Update: November 3, 2023 .
- Definition/Introduction
Researchers must utilize exploratory data techniques to present findings to a target audience and create appropriate graphs and figures. Researchers can determine if outliers exist, data are missing, and statistical assumptions will be upheld by understanding data. Additionally, it is essential to comprehend these data when describing them in conclusions of a paper, in a meeting with colleagues invested in the findings, or while reading others’ work.
- Issues of Concern
This comprehension begins with exploring these data through the outputs discussed in this article. Individuals who do not conduct research must still comprehend new studies, and knowledge of fundamentals in analyzing data and interpretation of histograms and boxplots facilitates the ability to appraise recent publications accurately. Without this familiarity, decisions could be implemented based on inaccurate delivery or interpretation of medical studies.
Frequencies and Descriptive Statistics
Effective presentation of study results, in presentation or manuscript form, typically starts with frequencies and descriptive statistics (ie, mean, medians, standard deviations). One can get a better sense of the variables by examining these data to determine whether a balanced and sufficient research design exists. Frequencies also inform on missing data and give a sense of outliers (will be discussed below).
Luckily, software programs are available to conduct exploratory data analysis. For this chapter, we will be examining the following research question.
RQ: Are there differences in drug life (length of effect) for Drug 23 based on the administration site?
A more precise hypothesis could be: Is drug 23 longer-lasting when administered via site A compared to site B?
To address this research question, exploratory data analysis is conducted. First, it is essential to start with the frequencies of the variables. To keep things simple, only variables of minutes (drug life effect) and administration site (A vs B) are included. See Image. Figure 1 for outputs for frequencies.
Figure 1 shows that the administration site appears to be a balanced design with 50 individuals in each group. The excerpt for minutes frequencies is the bottom portion of Figure 1 and shows how many cases fell into each time frame with the cumulative percent on the right-hand side. In examining Figure 1, one suspiciously low measurement (135) was observed, considering time variables. If a data point seems inaccurate, a researcher should find this case and confirm if this was an entry error. For the sake of this review, the authors state that this was an entry error and should have been entered 535 and not 135. Had the analysis occurred without checking this, the data analysis, results, and conclusions would have been invalid. When finding any entry errors and determining how groups are balanced, potential missing data is explored. If not responsibly evaluated, missing values can nullify results.
After replacing the incorrect 135 with 535, descriptive statistics, including the mean, median, mode, minimum/maximum scores, and standard deviation were examined. Output for the research example for the variable of minutes can be seen in Figure 2. Observe each variable to ensure that the mean seems reasonable and that the minimum and maximum are within an appropriate range based on medical competence or an available codebook. One assumption common in statistical analyses is a normal distribution. Image . Figure 2 shows that the mode differs from the mean and the median. We have visualization tools such as histograms to examine these scores for normality and outliers before making decisions.
Histograms are useful in assessing normality, as many statistical tests (eg, ANOVA and regression) assume the data have a normal distribution. When data deviate from a normal distribution, it is quantified using skewness and kurtosis. [1] Skewness occurs when one tail of the curve is longer. If the tail is lengthier on the left side of the curve (more cases on the higher values), this would be negatively skewed, whereas if the tail is longer on the right side, it would be positively skewed. Kurtosis is another facet of normality. Positive kurtosis occurs when the center has many values falling in the middle, whereas negative kurtosis occurs when there are very heavy tails. [2]
Additionally, histograms reveal outliers: data points either entered incorrectly or truly very different from the rest of the sample. When there are outliers, one must determine accuracy based on random chance or the error in the experiment and provide strong justification if the decision is to exclude them. [3] Outliers require attention to ensure the data analysis accurately reflects the majority of the data and is not influenced by extreme values; cleaning these outliers can result in better quality decision-making in clinical practice. [4] A common approach to determining if a variable is approximately normally distributed is converting values to z scores and determining if any scores are less than -3 or greater than 3. For a normal distribution, about 99% of scores should lie within three standard deviations of the mean. [5] Importantly, one should not automatically throw out any values outside of this range but consider it in corroboration with the other factors aforementioned. Outliers are relatively common, so when these are prevalent, one must assess the risks and benefits of exclusion. [6]
Image . Figure 3 provides examples of histograms. In Figure 3A, 2 possible outliers causing kurtosis are observed. If values within 3 standard deviations are used, the result in Figure 3B are observed. This histogram appears much closer to an approximately normal distribution with the kurtosis being treated. Remember, all evidence should be considered before eliminating outliers. When reporting outliers in scientific paper outputs, account for the number of outliers excluded and justify why they were excluded.
Boxplots can examine for outliers, assess the range of data, and show differences among groups. Boxplots provide a visual representation of ranges and medians, illustrating differences amongst groups, and are useful in various outlets, including evidence-based medicine. [7] Boxplots provide a picture of data distribution when there are numerous values, and all values cannot be displayed (ie, a scatterplot). [8] Figure 4 illustrates the differences between drug site administration and the length of drug life from the above example.
Image . Figure 4 shows differences with potential clinical impact. Had any outliers existed (data from the histogram were cleaned), they would appear outside the line endpoint. The red boxes represent the middle 50% of scores. The lines within each red box represent the median number of minutes within each administration site. The horizontal lines at the top and bottom of each line connected to the red box represent the 25th and 75th percentiles. In examining the difference boxplots, an overlap in minutes between 2 administration sites were observed: the approximate top 25 percent from site B had the same time noted as the bottom 25 percent at site A. Site B had a median minute amount under 525, whereas administration site A had a length greater than 550. If there were no differences in adverse reactions at site A, analysis of this figure provides evidence that healthcare providers should administer the drug via site A. Researchers could follow by testing a third administration site, site C. Image . Figure 5 shows what would happen if site C led to a longer drug life compared to site A.
Figure 5 displays the same site A data as Figure 4, but something looks different. The significant variance at site C makes site A’s variance appear smaller. In order words, patients who were administered the drug via site C had a larger range of scores. Thus, some patients experience a longer half-life when the drug is administered via site C than the median of site A; however, the broad range (lack of accuracy) and lower median should be the focus. The precision of minutes is much more compacted in site A. Therefore, the median is higher, and the range is more precise. One may conclude that this makes site A a more desirable site.
- Clinical Significance
Ultimately, by understanding basic exploratory data methods, medical researchers and consumers of research can make quality and data-informed decisions. These data-informed decisions will result in the ability to appraise the clinical significance of research outputs. By overlooking these fundamentals in statistics, critical errors in judgment can occur.
- Nursing, Allied Health, and Interprofessional Team Interventions
All interprofessional healthcare team members need to be at least familiar with, if not well-versed in, these statistical analyses so they can read and interpret study data and apply the data implications in their everyday practice. This approach allows all practitioners to remain abreast of the latest developments and provides valuable data for evidence-based medicine, ultimately leading to improved patient outcomes.
- Review Questions
- Access free multiple choice questions on this topic.
- Comment on this article.
Exploratory Data Analysis Figure 1 Contributed by Martin Huecker, MD and Jacob Shreffler, PhD
Exploratory Data Analysis Figure 2 Contributed by Martin Huecker, MD and Jacob Shreffler, PhD
Exploratory Data Analysis Figure 3 Contributed by Martin Huecker, MD and Jacob Shreffler, PhD
Exploratory Data Analysis Figure 4 Contributed by Martin Huecker, MD and Jacob Shreffler, PhD
Exploratory Data Analysis Figure 5 Contributed by Martin Huecker, MD and Jacob Shreffler, PhD
Disclosure: Jacob Shreffler declares no relevant financial relationships with ineligible companies.
Disclosure: Martin Huecker declares no relevant financial relationships with ineligible companies.
This book is distributed under the terms of the Creative Commons Attribution-NonCommercial-NoDerivatives 4.0 International (CC BY-NC-ND 4.0) ( http://creativecommons.org/licenses/by-nc-nd/4.0/ ), which permits others to distribute the work, provided that the article is not altered or used commercially. You are not required to obtain permission to distribute this article, provided that you credit the author and journal.
- Cite this Page Shreffler J, Huecker MR. Exploratory Data Analysis: Frequencies, Descriptive Statistics, Histograms, and Boxplots. [Updated 2023 Nov 3]. In: StatPearls [Internet]. Treasure Island (FL): StatPearls Publishing; 2024 Jan-.
In this Page
Bulk download.
- Bulk download StatPearls data from FTP
Related information
- PMC PubMed Central citations
- PubMed Links to PubMed
Similar articles in PubMed
- Contour boxplots: a method for characterizing uncertainty in feature sets from simulation ensembles. [IEEE Trans Vis Comput Graph. 2...] Contour boxplots: a method for characterizing uncertainty in feature sets from simulation ensembles. Whitaker RT, Mirzargar M, Kirby RM. IEEE Trans Vis Comput Graph. 2013 Dec; 19(12):2713-22.
- Review Univariate Outliers: A Conceptual Overview for the Nurse Researcher. [Can J Nurs Res. 2019] Review Univariate Outliers: A Conceptual Overview for the Nurse Researcher. Mowbray FI, Fox-Wasylyshyn SM, El-Masri MM. Can J Nurs Res. 2019 Mar; 51(1):31-37. Epub 2018 Jul 3.
- [Descriptive statistics]. [Rev Alerg Mex. 2016] [Descriptive statistics]. Rendón-Macías ME, Villasís-Keever MÁ, Miranda-Novales MG. Rev Alerg Mex. 2016 Oct-Dec; 63(4):397-407.
- An exploratory data analysis of electroencephalograms using the functional boxplots approach. [Front Neurosci. 2015] An exploratory data analysis of electroencephalograms using the functional boxplots approach. Ngo D, Sun Y, Genton MG, Wu J, Srinivasan R, Cramer SC, Ombao H. Front Neurosci. 2015; 9:282. Epub 2015 Aug 19.
- Review Graphics and statistics for cardiology: comparing categorical and continuous variables. [Heart. 2016] Review Graphics and statistics for cardiology: comparing categorical and continuous variables. Rice K, Lumley T. Heart. 2016 Mar; 102(5):349-55. Epub 2016 Jan 27.
Recent Activity
- Exploratory Data Analysis: Frequencies, Descriptive Statistics, Histograms, and ... Exploratory Data Analysis: Frequencies, Descriptive Statistics, Histograms, and Boxplots - StatPearls
Your browsing activity is empty.
Activity recording is turned off.
Turn recording back on
Connect with NLM
National Library of Medicine 8600 Rockville Pike Bethesda, MD 20894
Web Policies FOIA HHS Vulnerability Disclosure
Help Accessibility Careers

An official website of the United States government
The .gov means it’s official. Federal government websites often end in .gov or .mil. Before sharing sensitive information, make sure you’re on a federal government site.
The site is secure. The https:// ensures that you are connecting to the official website and that any information you provide is encrypted and transmitted securely.
- Publications
- Account settings
- My Bibliography
- Collections
- Citation manager
Save citation to file
Email citation, add to collections.
- Create a new collection
- Add to an existing collection
Add to My Bibliography
Your saved search, create a file for external citation management software, your rss feed.
- Search in PubMed
- Search in NLM Catalog
- Add to Search
Descriptive Statistics: Reporting the Answers to the 5 Basic Questions of Who, What, Why, When, Where, and a Sixth, So What?
Affiliation.
- 1 From the Department of Surgery and Perioperative Care, Dell Medical School at the University of Texas at Austin, Austin, Texas.
- PMID: 28891910
- DOI: 10.1213/ANE.0000000000002471
Descriptive statistics are specific methods basically used to calculate, describe, and summarize collected research data in a logical, meaningful, and efficient way. Descriptive statistics are reported numerically in the manuscript text and/or in its tables, or graphically in its figures. This basic statistical tutorial discusses a series of fundamental concepts about descriptive statistics and their reporting. The mean, median, and mode are 3 measures of the center or central tendency of a set of data. In addition to a measure of its central tendency (mean, median, or mode), another important characteristic of a research data set is its variability or dispersion (ie, spread). In simplest terms, variability is how much the individual recorded scores or observed values differ from one another. The range, standard deviation, and interquartile range are 3 measures of variability or dispersion. The standard deviation is typically reported for a mean, and the interquartile range for a median. Testing for statistical significance, along with calculating the observed treatment effect (or the strength of the association between an exposure and an outcome), and generating a corresponding confidence interval are 3 tools commonly used by researchers (and their collaborating biostatistician or epidemiologist) to validly make inferences and more generalized conclusions from their collected data and descriptive statistics. A number of journals, including Anesthesia & Analgesia, strongly encourage or require the reporting of pertinent confidence intervals. A confidence interval can be calculated for virtually any variable or outcome measure in an experimental, quasi-experimental, or observational research study design. Generally speaking, in a clinical trial, the confidence interval is the range of values within which the true treatment effect in the population likely resides. In an observational study, the confidence interval is the range of values within which the true strength of the association between the exposure and the outcome (eg, the risk ratio or odds ratio) in the population likely resides. There are many possible ways to graphically display or illustrate different types of data. While there is often latitude as to the choice of format, ultimately, the simplest and most comprehensible format is preferred. Common examples include a histogram, bar chart, line chart or line graph, pie chart, scatterplot, and box-and-whisker plot. Valid and reliable descriptive statistics can answer basic yet important questions about a research data set, namely: "Who, What, Why, When, Where, How, How Much?"
PubMed Disclaimer
Similar articles
- Fundamentals of Research Data and Variables: The Devil Is in the Details. Vetter TR. Vetter TR. Anesth Analg. 2017 Oct;125(4):1375-1380. doi: 10.1213/ANE.0000000000002370. Anesth Analg. 2017. PMID: 28787341 Review.
- Repeated Measures Designs and Analysis of Longitudinal Data: If at First You Do Not Succeed-Try, Try Again. Schober P, Vetter TR. Schober P, et al. Anesth Analg. 2018 Aug;127(2):569-575. doi: 10.1213/ANE.0000000000003511. Anesth Analg. 2018. PMID: 29905618 Free PMC article.
- Preparing for the first meeting with a statistician. De Muth JE. De Muth JE. Am J Health Syst Pharm. 2008 Dec 15;65(24):2358-66. doi: 10.2146/ajhp070007. Am J Health Syst Pharm. 2008. PMID: 19052282 Review.
- Summarizing and presenting numerical data. Pupovac V, Petrovecki M. Pupovac V, et al. Biochem Med (Zagreb). 2011;21(2):106-10. doi: 10.11613/bm.2011.018. Biochem Med (Zagreb). 2011. PMID: 22135849
- Introduction to biostatistics: Part 2, Descriptive statistics. Gaddis GM, Gaddis ML. Gaddis GM, et al. Ann Emerg Med. 1990 Mar;19(3):309-15. doi: 10.1016/s0196-0644(05)82052-9. Ann Emerg Med. 1990. PMID: 2310070
- Applying a "medical deserts" lens to cancer care services in the North-West region of Romania from 2009 to 2022 - a mixed-methods analysis. Brînzac MG, Ungureanu MI, Baba CO. Brînzac MG, et al. Arch Public Health. 2024 Sep 5;82(1):149. doi: 10.1186/s13690-024-01353-x. Arch Public Health. 2024. PMID: 39232788 Free PMC article.
- Canadian midwives' perspectives on the clinical impacts of point of care ultrasound in obstetrical care: A concurrent mixed-methods study. Johnston BK, Darling EK, Malott A, Thomas L, Murray-Davis B. Johnston BK, et al. Heliyon. 2024 Mar 5;10(6):e27512. doi: 10.1016/j.heliyon.2024.e27512. eCollection 2024 Mar 30. Heliyon. 2024. PMID: 38533003 Free PMC article.
- Validation and psychometric testing of the Chinese version of the prenatal body image questionnaire. Wang Q, Lin J, Zheng Q, Kang L, Zhang X, Zhang K, Lin R, Lin R. Wang Q, et al. BMC Pregnancy Childbirth. 2024 Feb 1;24(1):102. doi: 10.1186/s12884-024-06281-w. BMC Pregnancy Childbirth. 2024. PMID: 38302902 Free PMC article.
- Cracking the code: uncovering the factors that drive COVID-19 standard operating procedures compliance among school management in Malaysia. Ahmad NS, Karuppiah K, Praveena SM, Ali NF, Ramdas M, Mohammad Yusof NAD. Ahmad NS, et al. Sci Rep. 2024 Jan 4;14(1):556. doi: 10.1038/s41598-023-49968-4. Sci Rep. 2024. PMID: 38177620 Free PMC article.
- Comparison of Nonneurological Structures at Risk During Anterior-to-Psoas Versus Transpsoas Surgical Approaches Using Abdominal CT Imaging From L1 to S1. Razzouk J, Ramos O, Harianja G, Carter M, Mehta S, Wycliffe N, Danisa O, Cheng W. Razzouk J, et al. Int J Spine Surg. 2023 Dec 26;17(6):809-815. doi: 10.14444/8542. Int J Spine Surg. 2023. PMID: 37748918 Free PMC article.
- Search in MeSH
Related information
- Cited in Books
LinkOut - more resources
Full text sources.
- Ingenta plc
- Ovid Technologies, Inc.
- Wolters Kluwer
Other Literature Sources
- scite Smart Citations
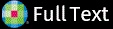
- Citation Manager
NCBI Literature Resources
MeSH PMC Bookshelf Disclaimer
The PubMed wordmark and PubMed logo are registered trademarks of the U.S. Department of Health and Human Services (HHS). Unauthorized use of these marks is strictly prohibited.
- Privacy Policy

Home » Descriptive Statistics – Types, Methods and Examples
Descriptive Statistics – Types, Methods and Examples
Table of Contents

Descriptive Statistics
Descriptive statistics is a branch of statistics that deals with the summarization and description of collected data. This type of statistics is used to simplify and present data in a manner that is easy to understand, often through visual or numerical methods. Descriptive statistics is primarily concerned with measures of central tendency, variability, and distribution, as well as graphical representations of data.
Here are the main components of descriptive statistics:
- Measures of Central Tendency : These provide a summary statistic that represents the center point or typical value of a dataset. The most common measures of central tendency are the mean (average), median (middle value), and mode (most frequent value).
- Measures of Dispersion or Variability : These provide a summary statistic that represents the spread of values in a dataset. Common measures of dispersion include the range (difference between the highest and lowest values), variance (average of the squared differences from the mean), standard deviation (square root of the variance), and interquartile range (difference between the upper and lower quartiles).
- Measures of Position : These are used to understand the distribution of values within a dataset. They include percentiles and quartiles.
- Graphical Representations : Data can be visually represented using various methods like bar graphs, histograms, pie charts, box plots, and scatter plots. These visuals provide a clear, intuitive way to understand the data.
- Measures of Association : These measures provide insight into the relationships between variables in the dataset, such as correlation and covariance.
Descriptive Statistics Types
Descriptive statistics can be classified into two types:
Measures of Central Tendency
These measures help describe the center point or average of a data set. There are three main types:
- Mean : The average value of the dataset, obtained by adding all the data points and dividing by the number of data points.
- Median : The middle value of the dataset, obtained by ordering all data points and picking out the one in the middle (or the average of the two middle numbers if the dataset has an even number of observations).
- Mode : The most frequently occurring value in the dataset.
Measures of Variability (or Dispersion)
These measures describe the spread or variability of the data points in the dataset. There are four main types:
- Range : The difference between the largest and smallest values in the dataset.
- Variance : The average of the squared differences from the mean.
- Standard Deviation : The square root of the variance, giving a measure of dispersion that is in the same units as the original dataset.
- Interquartile Range (IQR) : The range between the first quartile (25th percentile) and the third quartile (75th percentile), which provides a measure of variability that is resistant to outliers.
Descriptive Statistics Formulas
Sure, here are some of the most commonly used formulas in descriptive statistics:
Mean (μ or x̄) :
The average of all the numbers in the dataset. It is computed by summing all the observations and dividing by the number of observations.
Formula : μ = Σx/n or x̄ = Σx/n (where Σx is the sum of all observations and n is the number of observations)
The middle value in the dataset when the observations are arranged in ascending or descending order. If there is an even number of observations, the median is the average of the two middle numbers.
The most frequently occurring number in the dataset. There’s no formula for this as it’s determined by observation.
The difference between the highest (max) and lowest (min) values in the dataset.
Formula : Range = max – min
Variance (σ² or s²) :
The average of the squared differences from the mean. Variance is a measure of how spread out the numbers in the dataset are.
Population Variance formula : σ² = Σ(x – μ)² / N Sample Variance formula: s² = Σ(x – x̄)² / (n – 1)
(where x is each individual observation, μ is the population mean, x̄ is the sample mean, N is the size of the population, and n is the size of the sample)
Standard Deviation (σ or s) :
The square root of the variance. It measures the amount of variability or dispersion for a set of data. Population Standard Deviation formula: σ = √σ² Sample Standard Deviation formula: s = √s²
Interquartile Range (IQR) :
The range between the first quartile (Q1, 25th percentile) and the third quartile (Q3, 75th percentile). It measures statistical dispersion, or how far apart the data points are.
Formula : IQR = Q3 – Q1
Descriptive Statistics Methods
Here are some of the key methods used in descriptive statistics:
This method involves arranging data into a table format, making it easier to understand and interpret. Tables often show the frequency distribution of variables.
Graphical Representation
This method involves presenting data visually to help reveal patterns, trends, outliers, or relationships between variables. There are many types of graphs used, such as bar graphs, histograms, pie charts, line graphs, box plots, and scatter plots.
Calculation of Central Tendency Measures
This involves determining the mean, median, and mode of a dataset. These measures indicate where the center of the dataset lies.
Calculation of Dispersion Measures
This involves calculating the range, variance, standard deviation, and interquartile range. These measures indicate how spread out the data is.
Calculation of Position Measures
This involves determining percentiles and quartiles, which tell us about the position of particular data points within the overall data distribution.
Calculation of Association Measures
This involves calculating statistics like correlation and covariance to understand relationships between variables.
Summary Statistics
Often, a collection of several descriptive statistics is presented together in what’s known as a “summary statistics” table. This provides a comprehensive snapshot of the data at a glanc
Descriptive Statistics Examples
Descriptive Statistics Examples are as follows:
Example 1: Student Grades
Let’s say a teacher has the following set of grades for 7 students: 85, 90, 88, 92, 78, 88, and 94. The teacher could use descriptive statistics to summarize this data:
- Mean (average) : (85 + 90 + 88 + 92 + 78 + 88 + 94)/7 = 88
- Median (middle value) : First, rearrange the grades in ascending order (78, 85, 88, 88, 90, 92, 94). The median grade is 88.
- Mode (most frequent value) : The grade 88 appears twice, more frequently than any other grade, so it’s the mode.
- Range (difference between highest and lowest) : 94 (highest) – 78 (lowest) = 16
- Variance and Standard Deviation : These would be calculated using the appropriate formulas, providing a measure of the dispersion of the grades.
Example 2: Survey Data
A researcher conducts a survey on the number of hours of TV watched per day by people in a particular city. They collect data from 1,000 respondents and can use descriptive statistics to summarize this data:
- Mean : Calculate the average hours of TV watched by adding all the responses and dividing by the total number of respondents.
- Median : Sort the data and find the middle value.
- Mode : Identify the most frequently reported number of hours watched.
- Histogram : Create a histogram to visually display the frequency of responses. This could show, for example, that the majority of people watch 2-3 hours of TV per day.
- Standard Deviation : Calculate this to find out how much variation there is from the average.
Importance of Descriptive Statistics
Descriptive statistics are fundamental in the field of data analysis and interpretation, as they provide the first step in understanding a dataset. Here are a few reasons why descriptive statistics are important:
- Data Summarization : Descriptive statistics provide simple summaries about the measures and samples you have collected. With a large dataset, it’s often difficult to identify patterns or tendencies just by looking at the raw data. Descriptive statistics provide numerical and graphical summaries that can highlight important aspects of the data.
- Data Simplification : They simplify large amounts of data in a sensible way. Each descriptive statistic reduces lots of data into a simpler summary, making it easier to understand and interpret the dataset.
- Identification of Patterns and Trends : Descriptive statistics can help identify patterns and trends in the data, providing valuable insights. Measures like the mean and median can tell you about the central tendency of your data, while measures like the range and standard deviation tell you about the dispersion.
- Data Comparison : By summarizing data into measures such as the mean and standard deviation, it’s easier to compare different datasets or different groups within a dataset.
- Data Quality Assessment : Descriptive statistics can help identify errors or outliers in the data, which might indicate issues with data collection or entry.
- Foundation for Further Analysis : Descriptive statistics are typically the first step in data analysis. They help create a foundation for further statistical or inferential analysis. In fact, advanced statistical techniques often assume that one has first examined their data using descriptive methods.
When to use Descriptive Statistics
They can be used in a wide range of situations, including:
- Understanding a New Dataset : When you first encounter a new dataset, using descriptive statistics is a useful first step to understand the main characteristics of the data, such as the central tendency, dispersion, and distribution.
- Data Exploration in Research : In the initial stages of a research project, descriptive statistics can help to explore the data, identify trends and patterns, and generate hypotheses for further testing.
- Presenting Research Findings : Descriptive statistics can be used to present research findings in a clear and understandable way, often using visual aids like graphs or charts.
- Monitoring and Quality Control : In fields like business or manufacturing, descriptive statistics are often used to monitor processes, track performance over time, and identify any deviations from expected standards.
- Comparing Groups : Descriptive statistics can be used to compare different groups or categories within your data. For example, you might want to compare the average scores of two groups of students, or the variance in sales between different regions.
- Reporting Survey Results : If you conduct a survey, you would use descriptive statistics to summarize the responses, such as calculating the percentage of respondents who agree with a certain statement.
Applications of Descriptive Statistics
Descriptive statistics are widely used in a variety of fields to summarize, represent, and analyze data. Here are some applications:
- Business : Businesses use descriptive statistics to summarize and interpret data such as sales figures, customer feedback, or employee performance. For instance, they might calculate the mean sales for each month to understand trends, or use graphical representations like bar charts to present sales data.
- Healthcare : In healthcare, descriptive statistics are used to summarize patient data, such as age, weight, blood pressure, or cholesterol levels. They are also used to describe the incidence and prevalence of diseases in a population.
- Education : Educators use descriptive statistics to summarize student performance, like average test scores or grade distribution. This information can help identify areas where students are struggling and inform instructional decisions.
- Social Sciences : Social scientists use descriptive statistics to summarize data collected from surveys, experiments, and observational studies. This can involve describing demographic characteristics of participants, response frequencies to survey items, and more.
- Psychology : Psychologists use descriptive statistics to describe the characteristics of their study participants and the main findings of their research, such as the average score on a psychological test.
- Sports : Sports analysts use descriptive statistics to summarize athlete and team performance, such as batting averages in baseball or points per game in basketball.
- Government : Government agencies use descriptive statistics to summarize data about the population, such as census data on population size and demographics.
- Finance and Economics : In finance, descriptive statistics can be used to summarize past investment performance or economic data, such as changes in stock prices or GDP growth rates.
- Quality Control : In manufacturing, descriptive statistics can be used to summarize measures of product quality, such as the average dimensions of a product or the frequency of defects.
Limitations of Descriptive Statistics
While descriptive statistics are a crucial part of data analysis and provide valuable insights about a dataset, they do have certain limitations:
- Lack of Depth : Descriptive statistics provide a summary of your data, but they can oversimplify the data, resulting in a loss of detail and potentially significant nuances.
- Vulnerability to Outliers : Some descriptive measures, like the mean, are sensitive to outliers. A single extreme value can significantly skew your mean, making it less representative of your data.
- Inability to Make Predictions : Descriptive statistics describe what has been observed in a dataset. They don’t allow you to make predictions or generalizations about unobserved data or larger populations.
- No Insight into Correlations : While some descriptive statistics can hint at potential relationships between variables, they don’t provide detailed insights into the nature or strength of these relationships.
- No Causality or Hypothesis Testing : Descriptive statistics cannot be used to determine cause and effect relationships or to test hypotheses. For these purposes, inferential statistics are needed.
- Can Mislead : When used improperly, descriptive statistics can be used to present a misleading picture of the data. For instance, choosing to only report the mean without also reporting the standard deviation or range can hide a large amount of variability in the data.
About the author
Muhammad Hassan
Researcher, Academic Writer, Web developer
You may also like

Textual Analysis – Types, Examples and Guide

Grounded Theory – Methods, Examples and Guide

MANOVA (Multivariate Analysis of Variance) –...

Methodological Framework – Types, Examples and...

Phenomenology – Methods, Examples and Guide

Cluster Analysis – Types, Methods and Examples
Popular searches
- How to Get Participants For Your Study
- How to Do Segmentation?
- Conjoint Preference Share Simulator
- MaxDiff Analysis
- Likert Scales
- Reliability & Validity
Request consultation
Do you need support in running a pricing or product study? We can help you with agile consumer research and conjoint analysis.
Looking for an online survey platform?
Conjointly offers a great survey tool with multiple question types, randomisation blocks, and multilingual support. The Basic tier is always free.
Research Methods Knowledge Base
- Navigating the Knowledge Base
- Foundations
- Measurement
- Research Design
- Conclusion Validity
- Data Preparation
- Correlation
- Inferential Statistics
- Table of Contents
Fully-functional online survey tool with various question types, logic, randomisation, and reporting for unlimited number of surveys.
Completely free for academics and students .
Descriptive Statistics
Descriptive statistics are used to describe the basic features of the data in a study. They provide simple summaries about the sample and the measures. Together with simple graphics analysis, they form the basis of virtually every quantitative analysis of data.
Descriptive statistics are typically distinguished from inferential statistics . With descriptive statistics you are simply describing what is or what the data shows. With inferential statistics, you are trying to reach conclusions that extend beyond the immediate data alone. For instance, we use inferential statistics to try to infer from the sample data what the population might think. Or, we use inferential statistics to make judgments of the probability that an observed difference between groups is a dependable one or one that might have happened by chance in this study. Thus, we use inferential statistics to make inferences from our data to more general conditions; we use descriptive statistics simply to describe what’s going on in our data.
Descriptive Statistics are used to present quantitative descriptions in a manageable form. In a research study we may have lots of measures. Or we may measure a large number of people on any measure. Descriptive statistics help us to simplify large amounts of data in a sensible way. Each descriptive statistic reduces lots of data into a simpler summary. For instance, consider a simple number used to summarize how well a batter is performing in baseball, the batting average. This single number is simply the number of hits divided by the number of times at bat (reported to three significant digits). A batter who is hitting .333 is getting a hit one time in every three at bats. One batting .250 is hitting one time in four. The single number describes a large number of discrete events. Or, consider the scourge of many students, the Grade Point Average (GPA). This single number describes the general performance of a student across a potentially wide range of course experiences.
Every time you try to describe a large set of observations with a single indicator you run the risk of distorting the original data or losing important detail. The batting average doesn’t tell you whether the batter is hitting home runs or singles. It doesn’t tell whether she’s been in a slump or on a streak. The GPA doesn’t tell you whether the student was in difficult courses or easy ones, or whether they were courses in their major field or in other disciplines. Even given these limitations, descriptive statistics provide a powerful summary that may enable comparisons across people or other units.
Univariate Analysis
Univariate analysis involves the examination across cases of one variable at a time. There are three major characteristics of a single variable that we tend to look at:
- the distribution
- the central tendency
- the dispersion
In most situations, we would describe all three of these characteristics for each of the variables in our study.
The Distribution
The distribution is a summary of the frequency of individual values or ranges of values for a variable. The simplest distribution would list every value of a variable and the number of persons who had each value. For instance, a typical way to describe the distribution of college students is by year in college, listing the number or percent of students at each of the four years. Or, we describe gender by listing the number or percent of males and females. In these cases, the variable has few enough values that we can list each one and summarize how many sample cases had the value. But what do we do for a variable like income or GPA? With these variables there can be a large number of possible values, with relatively few people having each one. In this case, we group the raw scores into categories according to ranges of values. For instance, we might look at GPA according to the letter grade ranges. Or, we might group income into four or five ranges of income values.
Category | Percent |
---|---|
Under 35 years old | 9% |
36–45 | 21% |
46–55 | 45% |
56–65 | 19% |
66+ | 6% |
One of the most common ways to describe a single variable is with a frequency distribution . Depending on the particular variable, all of the data values may be represented, or you may group the values into categories first (e.g. with age, price, or temperature variables, it would usually not be sensible to determine the frequencies for each value. Rather, the value are grouped into ranges and the frequencies determined.). Frequency distributions can be depicted in two ways, as a table or as a graph. The table above shows an age frequency distribution with five categories of age ranges defined. The same frequency distribution can be depicted in a graph as shown in Figure 1. This type of graph is often referred to as a histogram or bar chart.
Distributions may also be displayed using percentages. For example, you could use percentages to describe the:
- percentage of people in different income levels
- percentage of people in different age ranges
- percentage of people in different ranges of standardized test scores
Central Tendency
The central tendency of a distribution is an estimate of the “center” of a distribution of values. There are three major types of estimates of central tendency:
The Mean or average is probably the most commonly used method of describing central tendency. To compute the mean all you do is add up all the values and divide by the number of values. For example, the mean or average quiz score is determined by summing all the scores and dividing by the number of students taking the exam. For example, consider the test score values:
The sum of these 8 values is 167 , so the mean is 167/8 = 20.875 .
The Median is the score found at the exact middle of the set of values. One way to compute the median is to list all scores in numerical order, and then locate the score in the center of the sample. For example, if there are 500 scores in the list, score #250 would be the median. If we order the 8 scores shown above, we would get:
There are 8 scores and score #4 and #5 represent the halfway point. Since both of these scores are 20 , the median is 20 . If the two middle scores had different values, you would have to interpolate to determine the median.
The Mode is the most frequently occurring value in the set of scores. To determine the mode, you might again order the scores as shown above, and then count each one. The most frequently occurring value is the mode. In our example, the value 15 occurs three times and is the model. In some distributions there is more than one modal value. For instance, in a bimodal distribution there are two values that occur most frequently.
Notice that for the same set of 8 scores we got three different values ( 20.875 , 20 , and 15 ) for the mean, median and mode respectively. If the distribution is truly normal (i.e. bell-shaped), the mean, median and mode are all equal to each other.
Dispersion refers to the spread of the values around the central tendency. There are two common measures of dispersion, the range and the standard deviation. The range is simply the highest value minus the lowest value. In our example distribution, the high value is 36 and the low is 15 , so the range is 36 - 15 = 21 .
The Standard Deviation is a more accurate and detailed estimate of dispersion because an outlier can greatly exaggerate the range (as was true in this example where the single outlier value of 36 stands apart from the rest of the values. The Standard Deviation shows the relation that set of scores has to the mean of the sample. Again lets take the set of scores:
to compute the standard deviation, we first find the distance between each value and the mean. We know from above that the mean is 20.875 . So, the differences from the mean are:
Notice that values that are below the mean have negative discrepancies and values above it have positive ones. Next, we square each discrepancy:
Now, we take these “squares” and sum them to get the Sum of Squares (SS) value. Here, the sum is 350.875 . Next, we divide this sum by the number of scores minus 1 . Here, the result is 350.875 / 7 = 50.125 . This value is known as the variance . To get the standard deviation, we take the square root of the variance (remember that we squared the deviations earlier). This would be SQRT(50.125) = 7.079901129253 .
Although this computation may seem convoluted, it’s actually quite simple. To see this, consider the formula for the standard deviation:
- X is each score,
- X̄ is the mean (or average),
- n is the number of values,
- Σ means we sum across the values.
In the top part of the ratio, the numerator, we see that each score has the mean subtracted from it, the difference is squared, and the squares are summed. In the bottom part, we take the number of scores minus 1 . The ratio is the variance and the square root is the standard deviation. In English, we can describe the standard deviation as:
the square root of the sum of the squared deviations from the mean divided by the number of scores minus one.
Although we can calculate these univariate statistics by hand, it gets quite tedious when you have more than a few values and variables. Every statistics program is capable of calculating them easily for you. For instance, I put the eight scores into SPSS and got the following table as a result:
Metric | Value |
---|---|
N | 8 |
Mean | 20.8750 |
Median | 20.0000 |
Mode | 15.00 |
Standard Deviation | 7.0799 |
Variance | 50.1250 |
Range | 21.00 |
which confirms the calculations I did by hand above.
The standard deviation allows us to reach some conclusions about specific scores in our distribution. Assuming that the distribution of scores is normal or bell-shaped (or close to it!), the following conclusions can be reached:
- approximately 68% of the scores in the sample fall within one standard deviation of the mean
- approximately 95% of the scores in the sample fall within two standard deviations of the mean
- approximately 99% of the scores in the sample fall within three standard deviations of the mean
For instance, since the mean in our example is 20.875 and the standard deviation is 7.0799 , we can from the above statement estimate that approximately 95% of the scores will fall in the range of 20.875-(2*7.0799) to 20.875+(2*7.0799) or between 6.7152 and 35.0348 . This kind of information is a critical stepping stone to enabling us to compare the performance of an individual on one variable with their performance on another, even when the variables are measured on entirely different scales.
Cookie Consent
Conjointly uses essential cookies to make our site work. We also use additional cookies in order to understand the usage of the site, gather audience analytics, and for remarketing purposes.
For more information on Conjointly's use of cookies, please read our Cookie Policy .
Which one are you?
I am new to conjointly, i am already using conjointly.
Reading list
Basics of machine learning, machine learning lifecycle, importance of stats and eda, understanding data, probability, exploring continuous variable, exploring categorical variables, missing values and outliers, central limit theorem, bivariate analysis introduction, continuous - continuous variables, continuous categorical, categorical categorical, multivariate analysis, different tasks in machine learning, build your first predictive model, evaluation metrics, preprocessing data, linear models, selecting the right model, feature selection techniques, decision tree, feature engineering, naive bayes, multiclass and multilabel, basics of ensemble techniques, advance ensemble techniques, hyperparameter tuning, support vector machine, advance dimensionality reduction, unsupervised machine learning methods, recommendation engines, improving ml models, working with large datasets, interpretability of machine learning models, automated machine learning, model deployment, deploying ml models, embedded devices, descriptive statistics: definitions, types, examples.
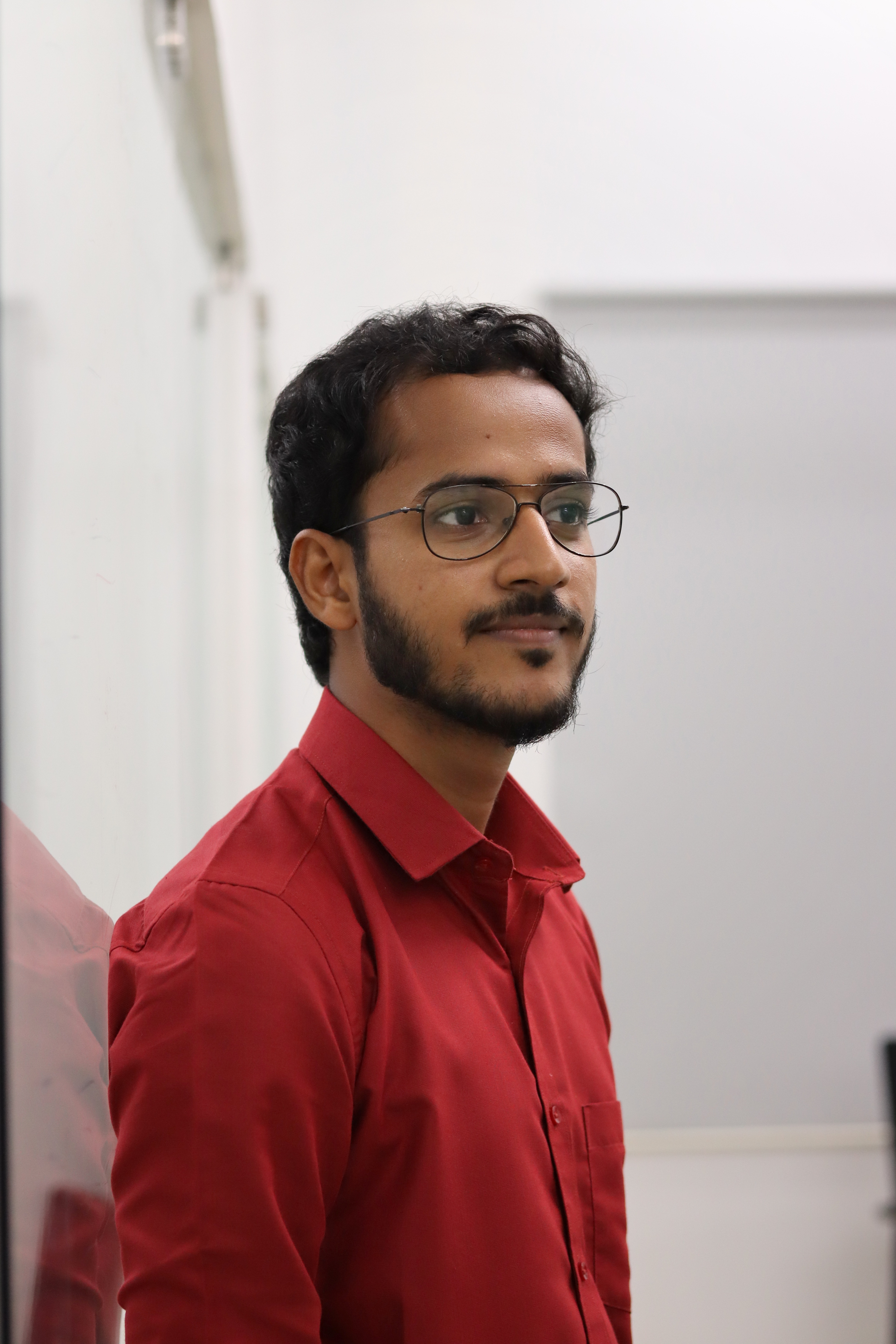
Introduction
The first step of any data-related process is the collection of data. Once we have collected the data, what do we do with it? Data can be sorted, analyzed, and used in various methods and formats, depending on the project’s needs. While analyzing a dataset, We use statistical methods to arrive at a conclusion. Data-driven decision-making also depends on how efficiently we use these methods. Two types of statistical methods are widely used in data analysis: descriptive and inferential. This article will focus more on descriptive statistics, its types, calculations, examples,percentages etc.
This article was published as a part of the Data Science Blogathon .
Table of contents
What is descriptive statistics, types of statistics, what is inferential statistics, types of descriptive statistics, descriptive statistics based on the central tendency of data, descriptive statistics based on the dispersion of data, descriptive statistics based on the shape of the data, univariate data vs. bivariate data in descriptive statistics, what are the 10 commonly used descriptive statistics, can descriptive statistics be used to make inferences or predictions, frequently asked questions.
Descriptive statistics serves as the initial step in understanding and summarizing data . It involves organizing, visualizing, and summarizing raw data to create a coherent picture. The primary goal of descriptive statistics is to provide a clear and concise overview of the data’s main features. This helps us identify patterns, trends, and characteristics within the data set without making broader inferences.
Key Aspects of Descriptive Statistics
- Measures of Central Tendency: Descriptive statistics include calculating the mean, median, and mode, which offer insights into the center of the data distribution.
- Measures of Dispersion: Variance, standard deviation, and range help us understand the spread or variability of the data.
- Visualizations: Creating graphs, histograms, bar charts, and pie charts visually represent the data’s distribution and characteristics
When you delve into the world of statistics, you’ll encounter two fundamental branches: descriptive statistics and inferential statistics. These two distinct approaches help us make sense of data and draw conclusions. Let’s look at the differences between these two branches to shed light on their roles in the realm of statistical analysis and their total number of branches.
Aspect | Descriptive Statistics | Inferential Statistics |
---|---|---|
Purpose | Summarize and describe data | Draw conclusions or predictions |
Data Sample | Analyzes the entire dataset | Analyzes a sample of the data |
Examples | Mean, Median, Range, Variance | Hypothesis testing, Regression |
Scope | Focuses on data characteristics | Makes inferences about populations |
Goal | Provides insights and simplifies data | Generalizes findings to a larger population |
Assumptions | No assumptions about populations | Requires assumptions about populations |
Common Use Cases | Data visualization, data exploration | Scientific research, hypothesis testing |
Inferential statistics takes data analysis to the next level by drawing conclusions about populations based on a sample. It involves making predictions, generalizations, and hypotheses about a larger group using a smaller subset of data. Inferential statistics bridges the gap between our data and the conclusions we want to reach. This is particularly useful when obtaining data from an entire population is impractical or impossible.
Key Aspects of Inferential Statistics
- Sampling Techniques: Inferential statistics relies on carefully selecting representative samples from a population to make valid inferences.
- Hypothesis Testing: This process involves setting up hypotheses about population characteristics and using sample data to determine if these hypotheses are statistically significant.
- Confidence Intervals: These provide a range of values within which we’re confident a population parameter lies based on sample data.
- Regression Analysis: Inferential statistics also encompass techniques like regression analysis to model relationships between variables and predict outcomes.
Now we will look at descriptive statistics in detail.
There are various dimensions in which this data can be described. The three main dimensions used for describing data are the central tendency, dispersion, and the shape of the data. Now, let’s look at them in detail, one by one.
The central tendency of data is the center of the distribution of data. It describes the location of data and concentrates on where the data is located. The three most widely used measures of the “center” of the data are Mean, Median, and Mode.
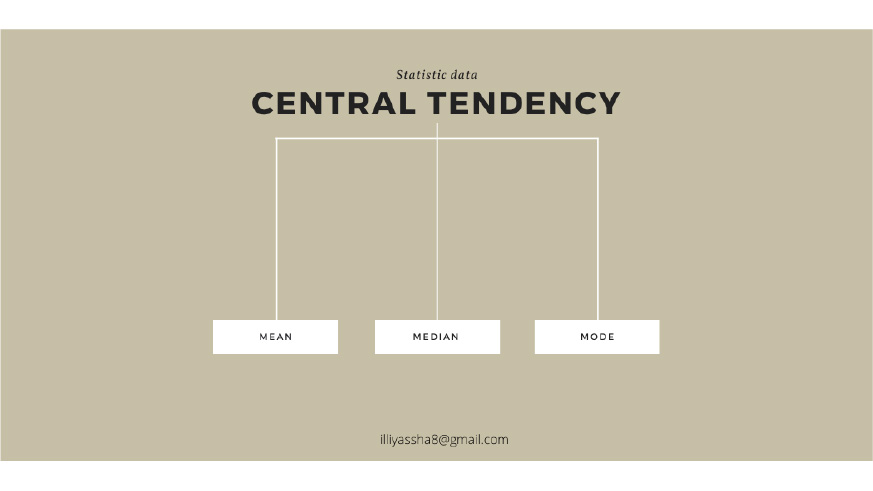
The “Mean” is the average of the data. The average can be identified by summing up all the numbers and then dividing them by the number of observations.
Mean = X 1 + X 2 + X 3 +… + X n / n
Data – 10,20,30,40,50 and Number of observations = 5 Mean = [ 10+20+30+40+50 ] / 5 Mean = 30
The central tendency of the data may be influenced by outliers. You may now ask, ‘ What are outliers? ‘ Well, outliers are extreme behaviors. An outlier is a data point that differs significantly from other observations. It can cause serious problems in analysis.
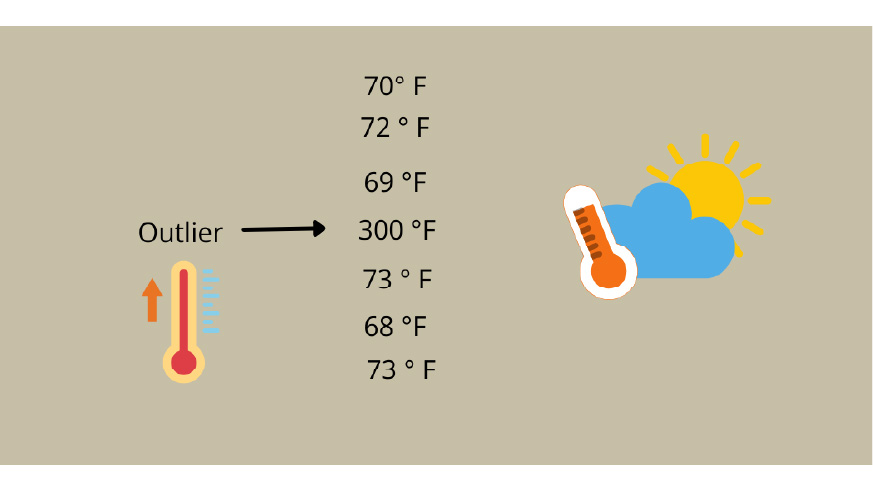
Data – 10,20,30,40,200 Mean = [ 10+20+30+40+200 ] / 5 Mean = 60
Solution for the outliers problem: Removing the outliers while taking averages will give us better results.
It is the 50th percentile of the data. In other words, it is exactly the center point of the data. The median can be identified by ordering the data, splitting it into two equal parts, and then finding the number in the middle. It is the best way to find the center of the data.
Note that, in this case, the central tendency of the data is not affected by outliers.
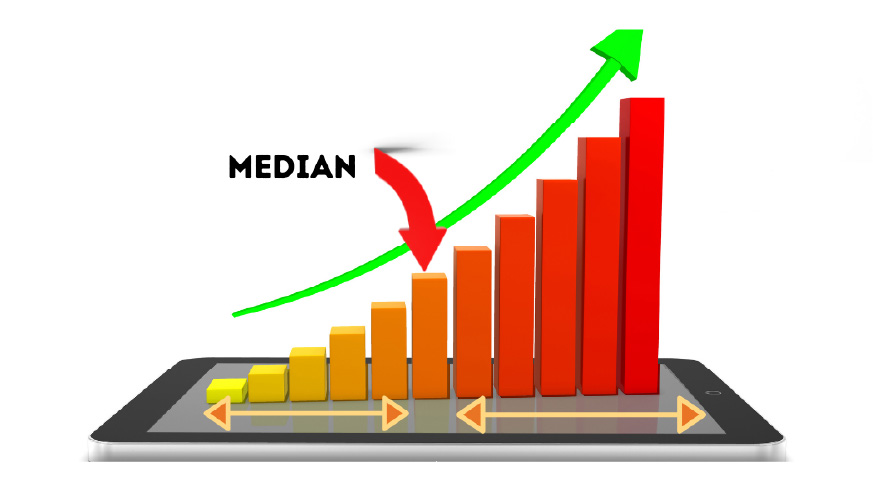
Odd number of Data – 10,20,30,40,50 Median is 30. Even the number of data – 10,20,30,40,50,60
Find the middle 2 data and take the mean of those two values. Here, 30 and 40 are middle values. Now, add them and divide the result by 2 30+40 / 2 =35 Median is 35
The mode of the data is the most frequently occurring data or elements in a dataset. If an element occurs the highest number of times, it is the mode of that data. If no number in the data is repeated, then that data has no mode. There can be more than one mode in a dataset if two values have the same frequency, which is also the highest frequency.
Outliers don’t influence the data in this case. The mode can be calculated for both quantitative and qualitative data.
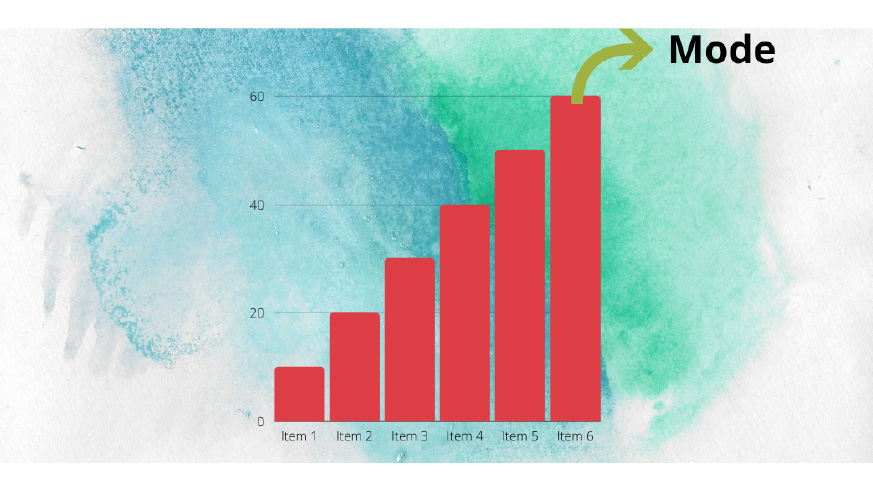
Data – 1,3,4,6,7,3,3,5,10, 3 Mode is 3, because 3 has the highest frequency (4 times)
The dispersion is the “spread of the data”. It measures how far the data is spread. In most of the dataset, the data values are closely located near the mean. The values are widely spread out of the mean on some other datasets. These dispersions of data can be measured by the Inter Quartile Range (IQR), range, standard deviation, and variance of the data.
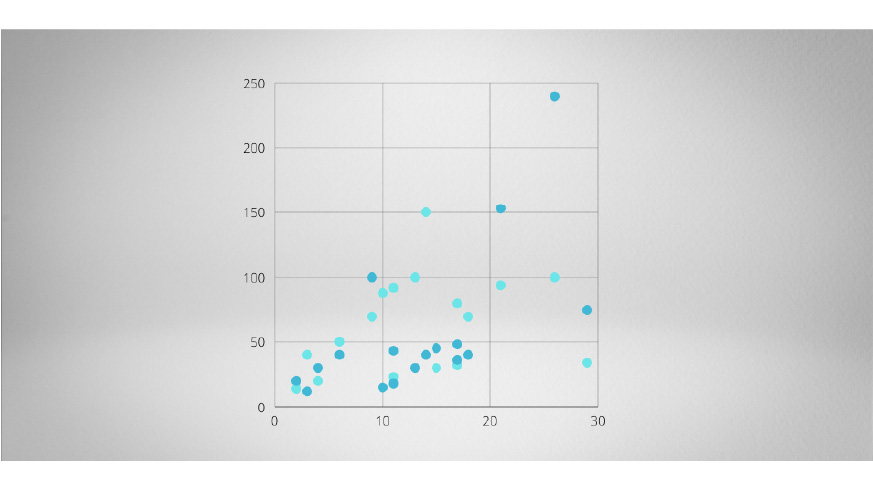
Let us see these measures in detail.
Inter Quartile Range (IQR)
Quartiles are special percentiles. 1st Quartile Q1 is the same as the 25th percentile. 2nd Quartile Q2 is the same as 50th percentile. 3rd Quratile Q3 is same as 75th percentile
Steps to find quartile and percentile
- The data should sorted and ordered from the smallest to the largest.
- For Quartiles, ordered data is divided into 4 equal parts.
- For Percentiles, ordered data is divided into 100 equal parts.
The Inter Quartile Range is the difference between the third quartile (Q3) and the first quartile (Q1)
IQR = Q3 – Q1
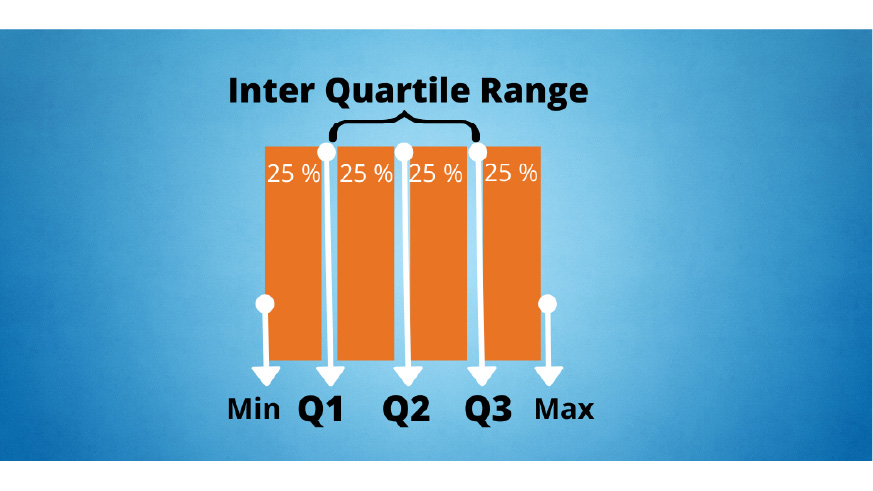
In this example, the Inter Quartile range is the spread of the middle half (50%) of the data.
The range is the difference between the largest and the smallest value in the data.
Standard Deviation
The most common measure of spread is the standard deviation. The Standard deviation measures how far the data deviates from the mean value. The standard deviation formula varies for population and and highest value of sample. Both formulas are similar but not the same.
Symbol used for Sample Standard Deviation – “s” (lowercase) Symbol used for Population Standard Deviation – “ σ” (sigma, lower case)
Steps to find the Standard Deviation
If x is a number, then the difference “x – mean” is its deviation. The deviations are used to calculate the standard deviation.
Sample Standard Deviation, s = Square root of sample variance Sample Standard Deviation, s = Square root of [Σ(x − x ¯ ) 2 / n-1] where x ¯ is average and n is no. of samples
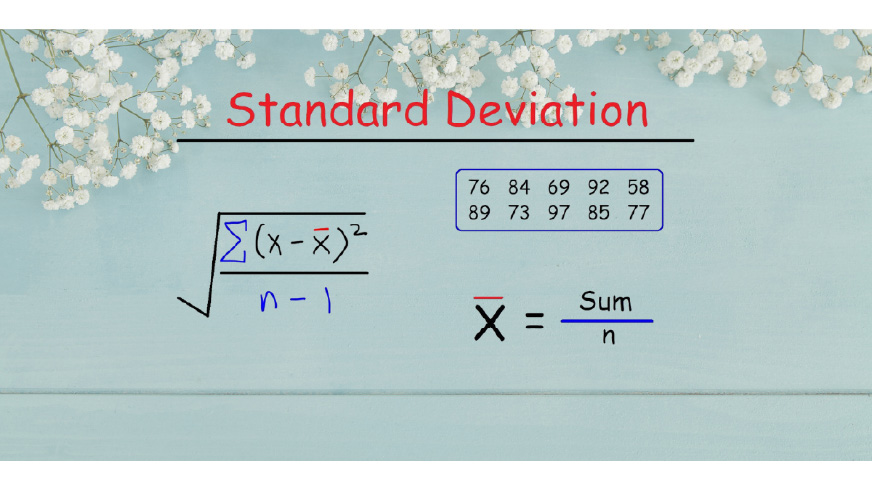
Population Standard Deviation, σ = Square root of population variance Population Standard Deviation, σ = Square root of [ Σ(x − μ) 2 / N ] where μ is Mean and N is no.of population.
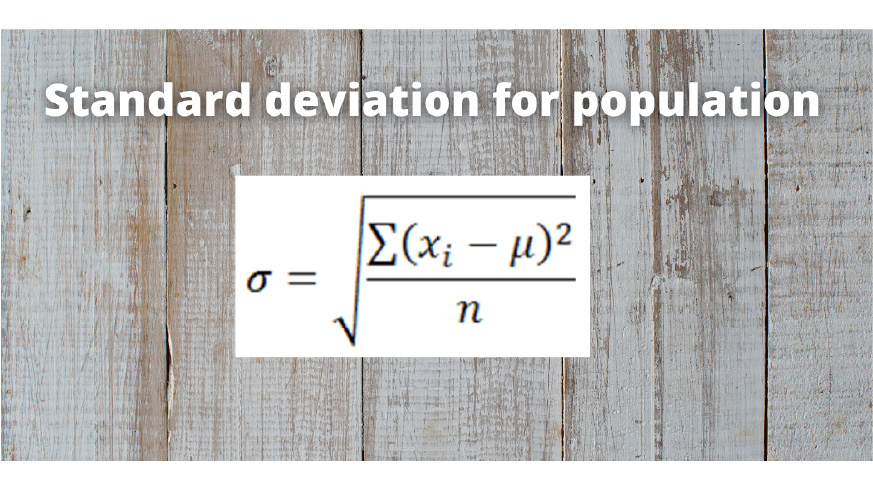
The standard deviation is always positive or zero. It will be large when the data values are spread out from the mean.
The variance is a measure of variability. It is the average squared deviation from the mean. The symbol σ 2 represents the population variance, and the symbol for s 2 represents sample variance.
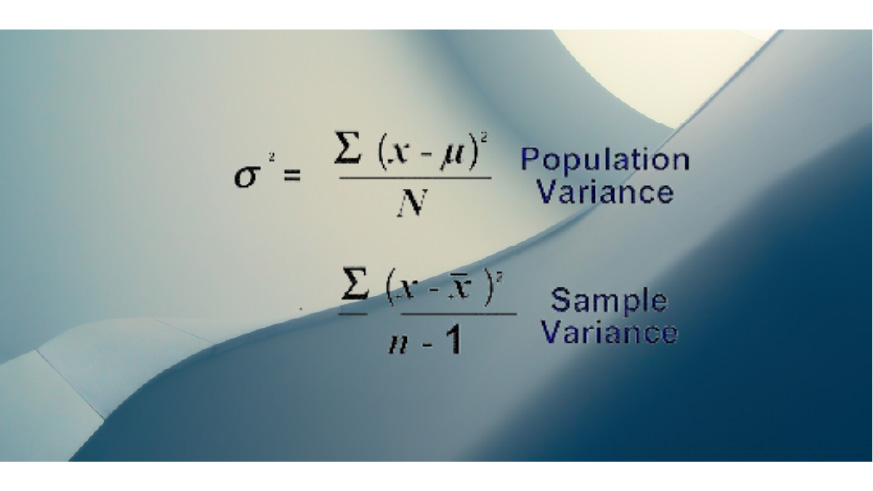
The shape of the data is important because deciding the probability of data is based on its shape. The shape describes the type of the graph.
.png)
The shape of the data can be measured by three methodologies: symmetric, skewness, kurtosis
In the symmetric shape of the graph, the data is distributed the same on both sides. In symmetric data, the mean and median are located close together. The curve formed by this symmetric graph is called a normal curve.
.png)
Skewness is the measure of the asymmetry of the distribution of data. The data is not symmetrical (i.e.) it is skewed towards one side. Skewness is classified into two types: positive skew and negative skew.
- Positively skewed : In a Positively skewed distribution, the data values are clustered around the left side of the distribution, and the right side is longer. The mean and median will be greater than the mode in the positive skew.
- Negatively skewed : In a Negatively skewed distribution, the data values are clustered around the right side of the distribution, and the left side is longer. The mean and median will be less than the mode.
.png)
Kurtosis is the measure of describing the distribution of data. This data is distributed in three different ways: platykurtic, mesokurtic, and leptokurtic.
.png)
- Platykurtic : The platykurtic shows a distribution with flat tails. Here, the data is distributed fairly. The flat tails indicated the small outliers in the distribution.
.png)
- Mesokurtic : In Mesokurtic, the data is widely distributed. It is normally distributed, and it also matches normal distribution.
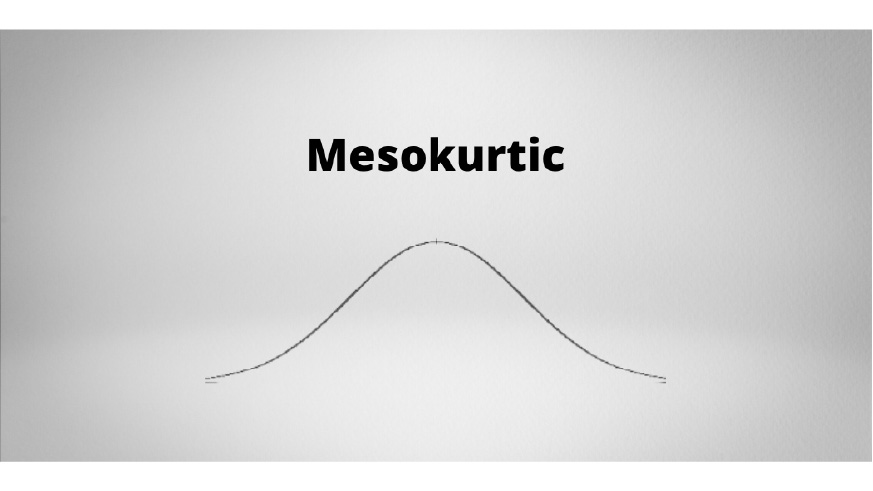
- Leptokurtic : In leptokurtic, the data is very closely distributed. The height of the peak is greater than the width of the peak.
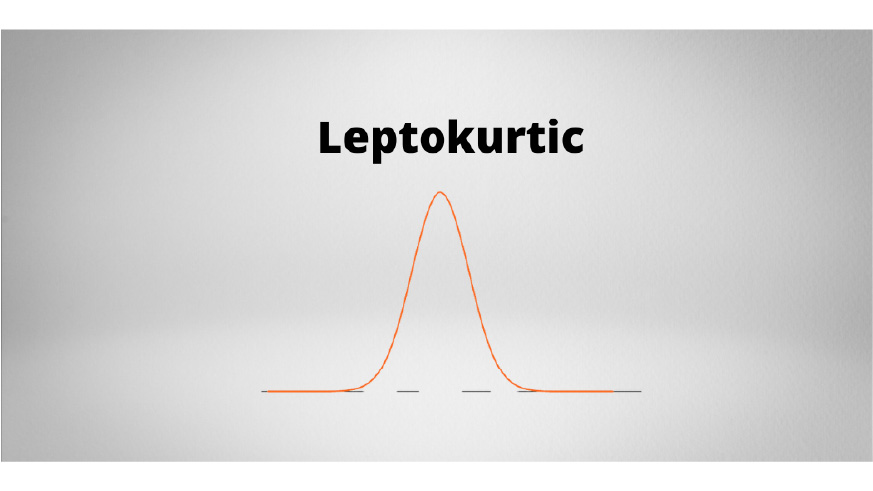
When it comes to delving into the world of data analysis, two key terms you’re likely to encounter are “ Univariate ” and “ Bivariate .” These terms are crucial in descriptive statistics, as they help us categorize and understand the data types we’re working with. Whether you’re deciphering the properties of individual data points or unraveling the intricate dance between two variables, the concepts of univariate and bivariate data provide the foundation for insightful data analysis.
the key difference between univariate and bivariate data lies in the focus of analysis. Univariate analysis centers on understanding the characteristics of a single variable, while bivariate analysis explores connections and interactions between two variables. Let’s break down the differences between univariate and bivariate data to better grasp their significance.
Univariate Data
Univariate data focuses on a single variable, essentially spotlighting one aspect of your data. In this scenario, you’re interested in studying the distribution, central tendency, and dispersion of a single set of values. For instance, if you’re analyzing the heights of a group of individuals, you’re dealing with univariate data. Here, the variable of interest is height, and you aim to uncover insights about that specific characteristic.
In univariate analysis, you’re often looking at measures like:
- Measures of Central Tendency: Mean, median, and mode provide insights into where the center of the data lies.
- Measures of Dispersion: Range, variance, and standard deviation help you understand how spread out the data is.
- Frequency Distribution: Creating histograms, bar charts, and pie charts allows you to visualize the data’s distribution.
Bivariate Data
Bivariate data, on the other hand, adds an extra layer of complexity to your analysis by involving two variables. Here, you’re not just interested in understanding individual characteristics; you’re also keen on uncovering relationships and patterns between two different variables. For example, if you’re examining the relationship between hours of study and exam scores, you’re working with bivariate data. The goal is to determine whether changes in one variable (study hours) have an impact on another (exam scores).
Bivariate analysis often involves techniques such as:
- Scatter Plots: These visualizations showcase the relationship between two variables, with each data point plotted on the graph.
- Correlation: Calculating correlation coefficients helps you quantify the strength and direction of the relationship between variables.
- Regression Analysis: This technique allows you to model the relationship between variables, predicting the outcome of one based on the other.
There are actually many useful descriptive statistics, but here are 5 of the most commonly used:
- Mean : This is the average of all the values in a data set. It’s a good indicator of the overall center of the data, but can be sensitive to outliers, especially in multivariate data with extreme values.
- Median : This is the ‘middle’ value when the data is ordered from least to greatest. It’s less affected by outliers than the mean, making it a robust measure for box plot analyses.
- Mode : This is the most frequent value in a data set. There can be one mode, or even multiple modes in some cases, especially when dealing with categorical variables.
- Standard Deviation : This tells you how spread out the data is from the mean. A larger standard deviation indicates a wider spread of data points. It’s crucial in understanding the dispersion in multivariate data.
- Range : This is the difference between the highest and lowest values in the data set. It’s a simple way to gauge how much variation there is but doesn’t tell you anything about the distribution within that range. It’s often represented in graphical representations like box plots.
- Categorical Variables : These are variables that represent distinct groups or categories. Analysis often involves graphical representations and contingency tables to understand the relationships between categories.
- Contingency Tables : These tables are used to display the frequency distribution of categorical variables. They help in analyzing the relationship between different categorical variables in multivariate data.
- Box Plot : A graphical representation that shows the distribution of a dataset through its quartiles. It highlights the median, quartiles, and extreme values, providing a clear picture of the data’s spread and potential outliers.
- Graphical Representation : This involves using visual tools like box plots, histograms, and scatter plots to summarize and analyze data, making it easier to identify patterns, trends, and extreme values in both univariate and multivariate datasets.
- Extreme Values : These are the data points that are significantly higher or lower than the majority of the data. They can heavily influence the mean and standard deviation and are often highlighted in box plots and other graphical representations
Descriptive statistics themselves are not used for predictions, but they can lay the groundwork for them. Here’s the key difference:
Descriptive statistics summarize the data you have. They use measures like mean, median, and standard deviation to give you a general idea of what the data looks like. This process often involves exploratory data analysis, where open exploration of the data can reveal patterns and insights. For instance, calculating mean scores is a common part of this analysis.
Inferential statistics use the data you have to draw conclusions about a larger population. This allows you to make predictions about things you haven’t observed yet. Here, you would identify the dependent variable and independent variable in your study, which are crucial for making these inferences.
Think of it like this: Descriptive statistics describe your apartment, while inferential statistics use the features of your apartment to guess about the entire apartment building.
So, while descriptive statistics can’t directly predict the future, they can help you understand the data and prepare it for inferential statistics, which can then be used for predictions. Summary statistics from your exploratory data analysis can provide the foundation for these predictive models.
In a world flooded with data, understanding, interpreting, and communicating information is paramount. Descriptive statistics doesn’t just crunch numbers; it crafts narratives, constructs visualizations, and empowers us to make informed decisions. Hope this article has given you a brief introduction to descriptive statistics. In this article, we have seen how the various measures of descriptive statistics, such as central tendency, dispersion, and shape of the data curve, help decipher the numbers. We have also bridged the gap between individual characteristics and the dance between variables by learning about univariate and bivariate data.
Also, this article will help you with the standard deviation of these statistics and statisticians. Not only Multivariate analysis measures of spread the sample size of the shape of the distribution of these statistics.
Ans. The methods used to summarize and describe the main features of a dataset are called descriptive statistics. Measures of central tendencies, measures of variability, etc., which give information about the typical values in a dataset, are all examples of descriptive statistics.
Ans. The 5 descriptive statistics include standard deviation, minimum and maximum variables, variance, kurtosis, and skewness.
Ans. The frequency distribution, central tendency, and variability of a dataset are the 3 main types of descriptive statistics.
Ans. Descriptive statistics are of 3 types: frequency distribution, central tendency, and variability.
The media shown in this article are not owned by Analytics Vidhya and are used at the Author’s discretion.
I am a Machine Learning professional with a strong background in Natural Language Processing (NLP). I am passionate about predictive modeling, data analysis, and deep learning, as they provide unique opportunities to uncover valuable insights from complex datasets. Recently, my focus has been on Language Models (LLMs), an exciting area within NLP. I have been actively involved in researching, developing, and refining LLMs to enhance their capabilities and applicability in real-world scenarios. Through my work, I strive to advance the field of NLP and contribute to the development of intelligent systems that can understand and generate human-like language. Sharing knowledge and collaborating with others is an essential part of my professional journey. I find great joy in exchanging ideas, insights, and expertise with fellow professionals and enthusiasts. By sharing my knowledge, I aim to contribute to the growth of the Machine Learning and NLP community, fostering an environment of continuous learning and innovation.
Free Courses

Generative AI - A Way of Life
Explore Generative AI for beginners: create text and images, use top AI tools, learn practical skills, and ethics.

Getting Started with Large Language Models
Master Large Language Models (LLMs) with this course, offering clear guidance in NLP and model training made simple.

Building LLM Applications using Prompt Engineering
This free course guides you on building LLM apps, mastering prompt engineering, and developing chatbots with enterprise data.

Building Your first RAG System using LlamaIndex
Build your first RAG model with LlamaIndex in this free course. Dive into Retrieval-Augmented Generation now!

Building Production Ready RAG systems using LlamaIndex
Learn Retrieval-Augmented Generation (RAG): learn how it works, the RAG framework, and use LlamaIndex for advanced systems.
Recommended Articles
Descriptive vs Inferential Statistics: WhatR...
Get Started with Statistics for Data Science
Data Types in Statistics for Data Science
A Guide To Complete Statistics For Data Science...
End to End Statistics for Data Science
15 Basic Statistics Concepts Every Data Science...
Various Uses of Python Statistics Module &...
An Introduction to Statistics For Data Science:...
Mathematics for Data Science
Top 40 Data Science Statistics Interview Questions
Lorem ipsum dolor sit amet, consectetur adipiscing elit,
Write for us
Write, captivate, and earn accolades and rewards for your work
- Reach a Global Audience
- Get Expert Feedback
- Build Your Brand & Audience
- Cash In on Your Knowledge
- Join a Thriving Community
- Level Up Your Data Science Game
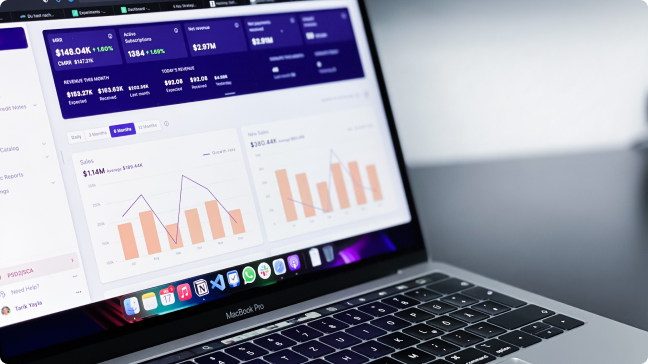
Sion Chakrabarti
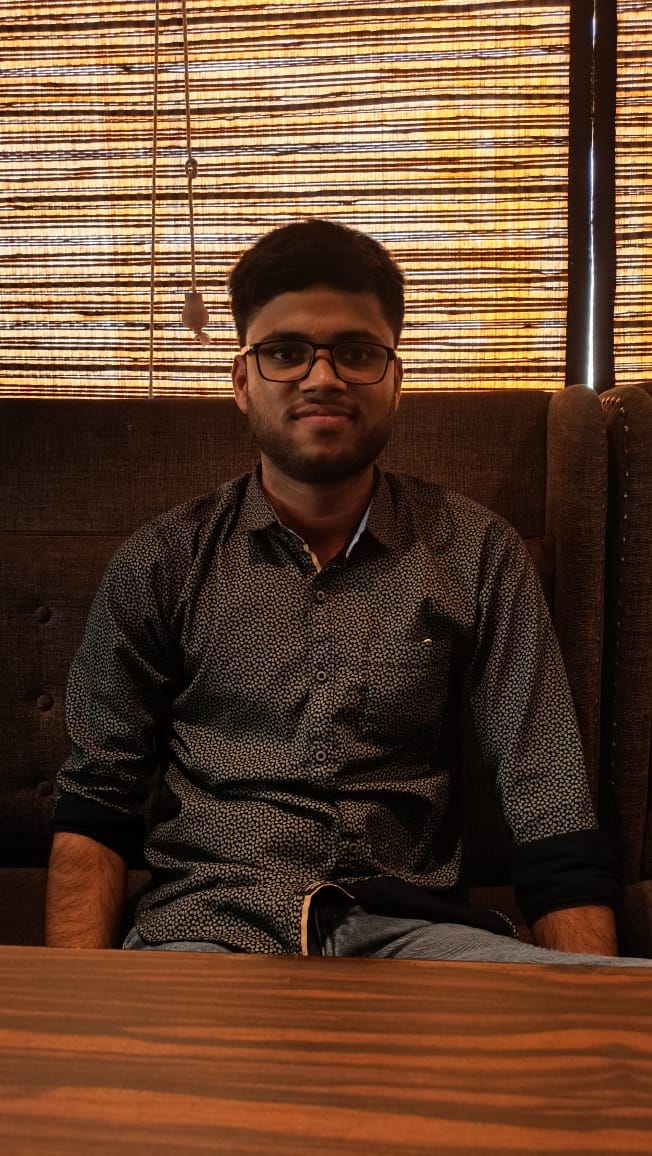
CHIRAG GOYAL
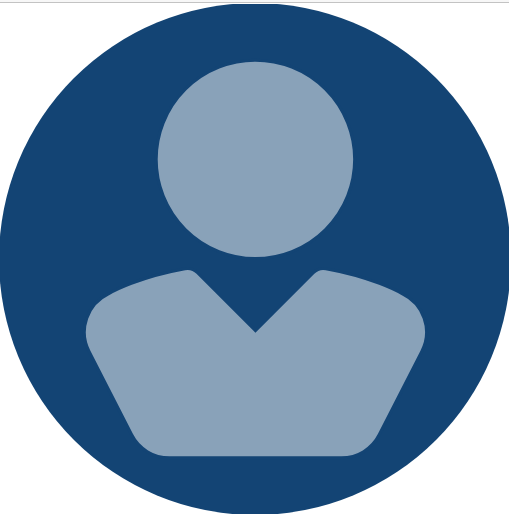
Barney Darlington
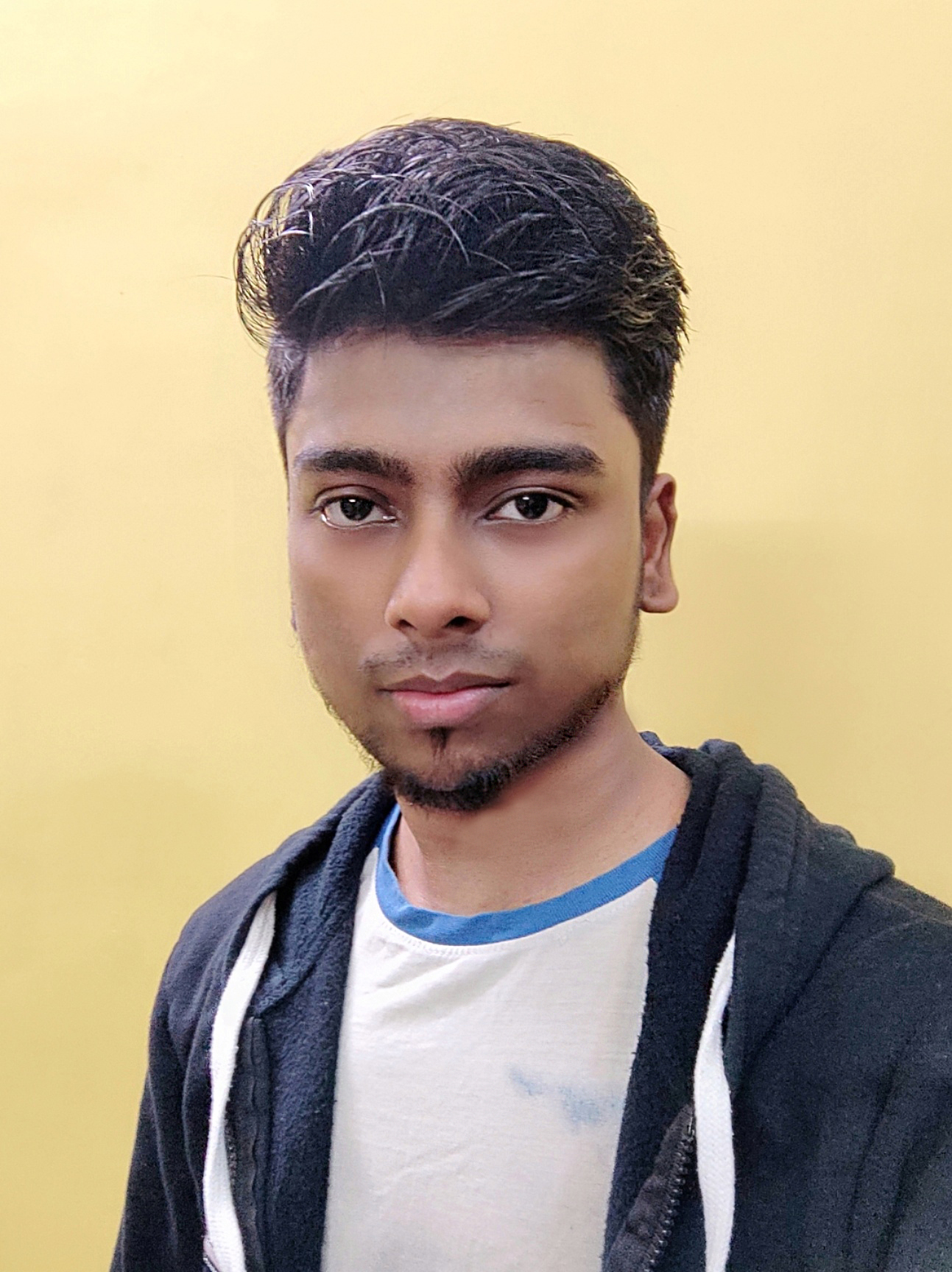
Suvojit Hore
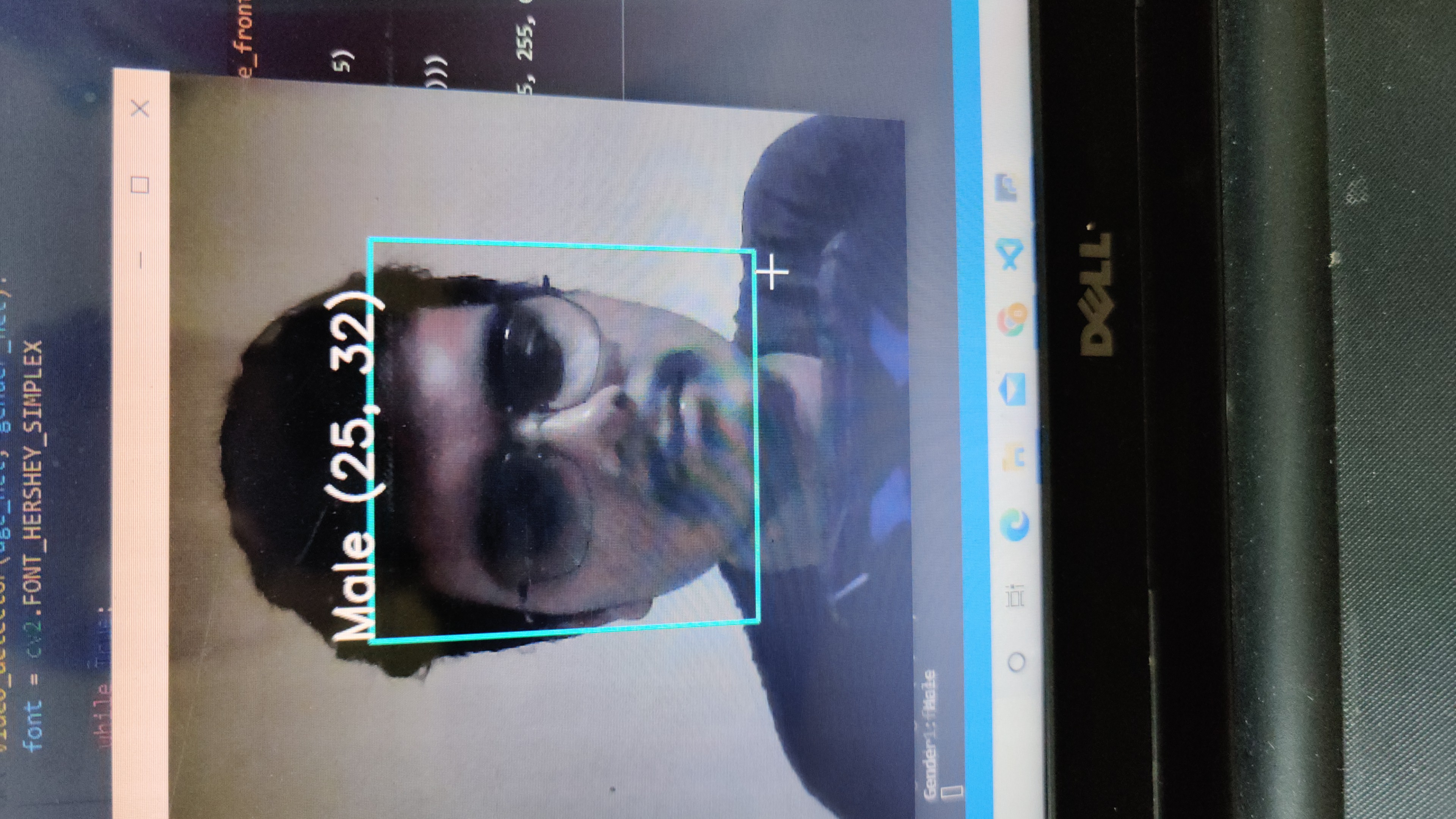
Arnab Mondal
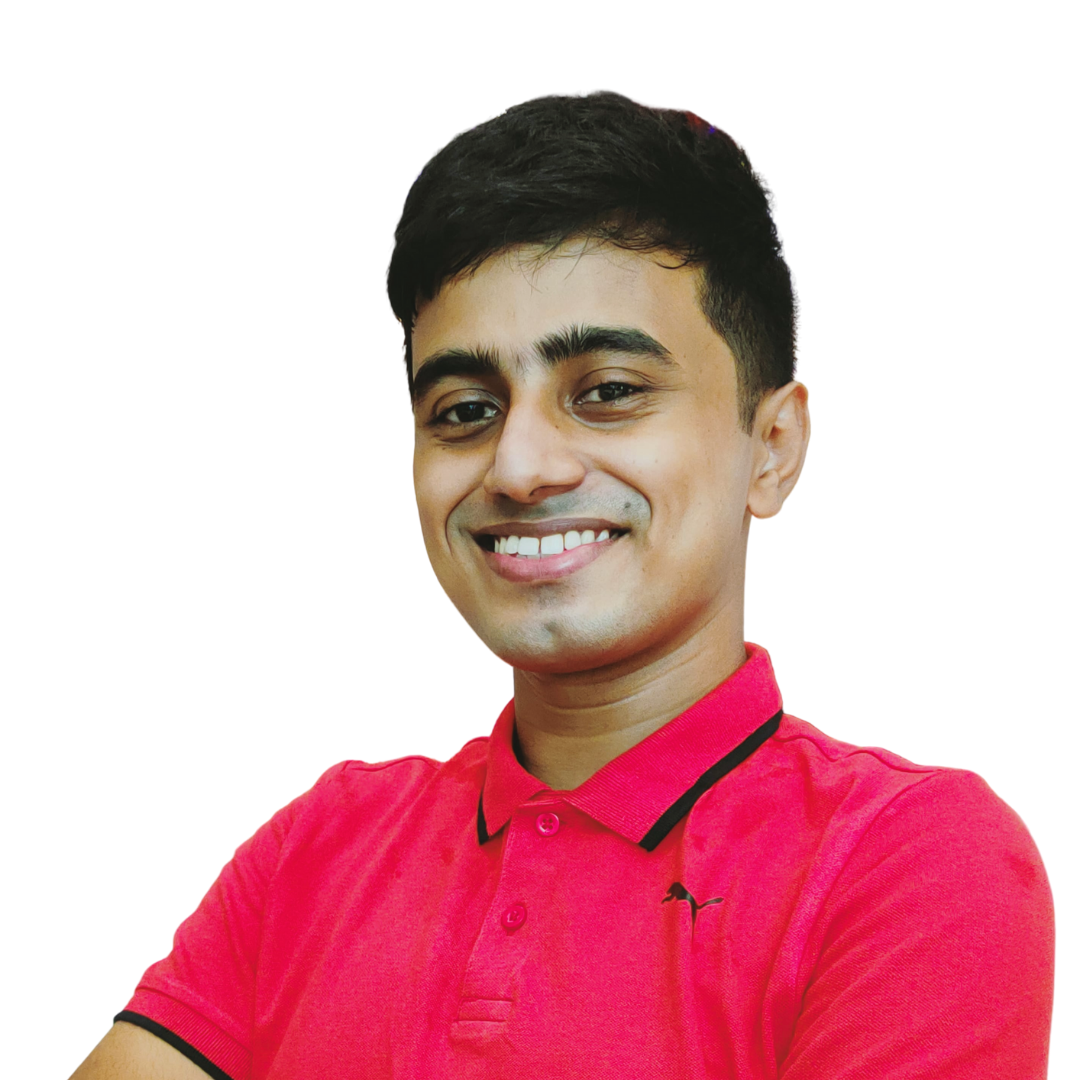
Prateek Majumder
Flagship Courses
Popular categories, generative ai tools and techniques, popular genai models, data science tools and techniques, genai pinnacle program, revolutionizing ai learning & development.
- 1:1 Mentorship with Generative AI experts
- Advanced Curriculum with 200+ Hours of Learning
- Master 26+ GenAI Tools and Libraries

Enroll with us today!
Continue your learning for free, enter email address to continue, enter otp sent to.
Resend OTP in 45s
Privacy Overview
When to use descriptive Statistics to answer RQs
Descriptive statistics are the appropriate analyses when the goal of the research is to present the participants’ responses to survey items in order to address the research questions. There are no hypotheses in descriptive statistics.
Descriptive statistics include: frequencies and percentages for categorical (ordinal and nominal) data; and averages (means, medians, and/or ranges) and standard deviations for continuous data. Frequency is the number of participants that fit into a certain category or group; it is beneficial to know the percent of the sample that coincides with that category/group. Percentages can be calculated to assess the percent of the sample that corresponds with the given frequency; typically presented without decimal places (according to APA 6 th ed. standards). Typically, the average that is calculated/presented is the mean. Means describe the average unit for a continuous item; and standard deviations describe the spread of those units in reference to the mean.
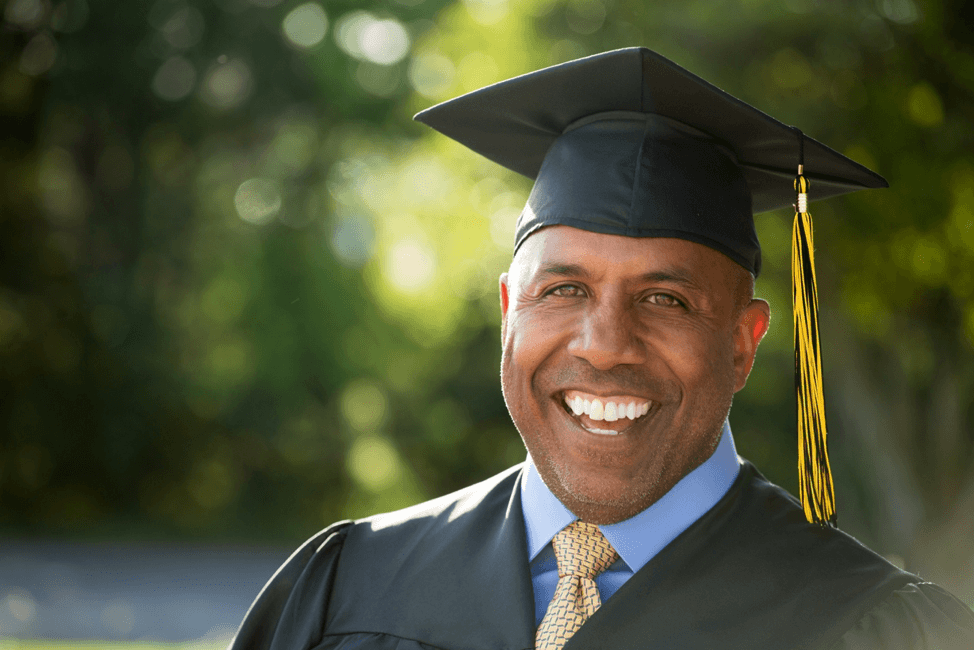
Discover How We Assist to Edit Your Dissertation Chapters
Aligning theoretical framework, gathering articles, synthesizing gaps, articulating a clear methodology and data plan, and writing about the theoretical and practical implications of your research are part of our comprehensive dissertation editing services.
- Bring dissertation editing expertise to chapters 1-5 in timely manner.
- Track all changes, then work with you to bring about scholarly writing.
- Ongoing support to address committee feedback, reducing revisions.
- You cannot (statistically) infer results with descriptive statistics. Inferential (parametric and non-parametric) statistics are conducted when the goal of the research is to draw conclusions about the statistical significance of the relationships and/or differences among variables of interest.
- Power analyses (sample size and effect size ) can be conducted when the analyses used to address the research questions are inferential; not for descriptive statistics and there is not a minimum sample size that is required to conduct descriptive statistics.
- What is the percentage of X, Y, and Z participants?
- How long have X, Y, and Z participants been in a certain group/category?
- What are, or describe, the factors of X?
- What is the average of variable Y?
- How much do X participants agree about a certain topic?
- What are, or describe, the similarities and/or differences on a certain topic by group/category?
Example: a study was conducted on a group of college students about specific courses offered, where the questions had “check all that apply” responses. The study’s research question asked “What courses offered to college students are most prevalent?” Descriptive statistics would be the appropriate analysis to address the research question. Frequencies and percentages could be conducted on the survey’s listed courses that students took/registered for. See the table below for details.
Frequencies and Percentages on the Survey’s Listed Courses
Purdue Online Writing Lab Purdue OWL® College of Liberal Arts
Writing with Descriptive Statistics
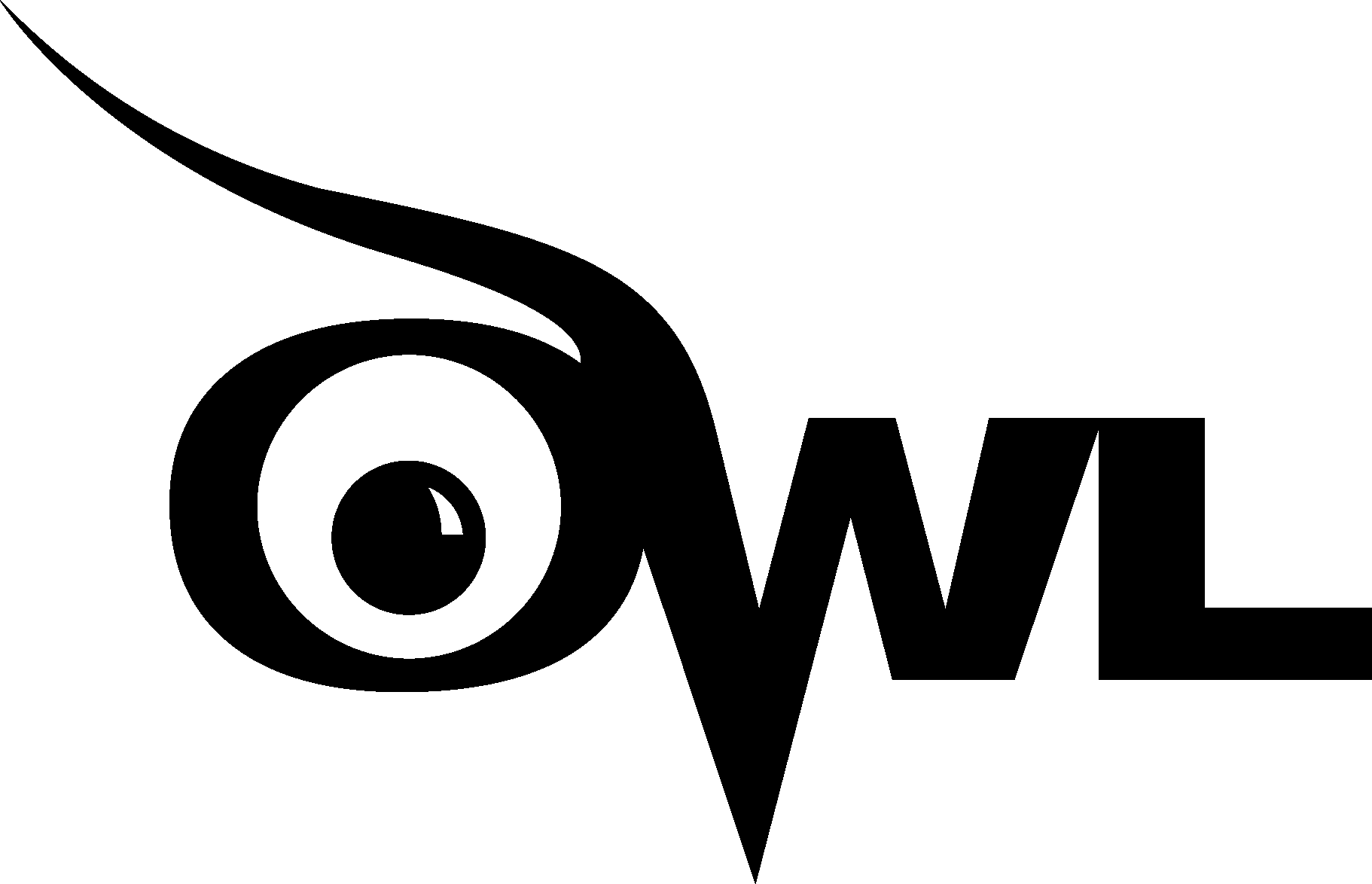
Welcome to the Purdue OWL
This page is brought to you by the OWL at Purdue University. When printing this page, you must include the entire legal notice.
Copyright ©1995-2018 by The Writing Lab & The OWL at Purdue and Purdue University. All rights reserved. This material may not be published, reproduced, broadcast, rewritten, or redistributed without permission. Use of this site constitutes acceptance of our terms and conditions of fair use.
Usually there is no good way to write a statistic. It rarely sounds good, and often interrupts the structure or flow of your writing. Oftentimes the best way to write descriptive statistics is to be direct. If you are citing several statistics about the same topic, it may be best to include them all in the same paragraph or section.
The mean of exam two is 77.7. The median is 75, and the mode is 79. Exam two had a standard deviation of 11.6.
Overall the company had another excellent year. We shipped 14.3 tons of fertilizer for the year, and averaged 1.7 tons of fertilizer during the summer months. This is an increase over last year, where we shipped only 13.1 tons of fertilizer, and averaged only 1.4 tons during the summer months. (Standard deviations were as followed: this summer .3 tons, last summer .4 tons).
Some fields prefer to put means and standard deviations in parentheses like this:
If you have lots of statistics to report, you should strongly consider presenting them in tables or some other visual form. You would then highlight statistics of interest in your text, but would not report all of the statistics. See the section on statistics and visuals for more details.
If you have a data set that you are using (such as all the scores from an exam) it would be unusual to include all of the scores in a paper or article. One of the reasons to use statistics is to condense large amounts of information into more manageable chunks; presenting your entire data set defeats this purpose.
At the bare minimum, if you are presenting statistics on a data set, it should include the mean and probably the standard deviation. This is the minimum information needed to get an idea of what the distribution of your data set might look like. How much additional information you include is entirely up to you. In general, don't include information if it is irrelevant to your argument or purpose. If you include statistics that many of your readers would not understand, consider adding the statistics in a footnote or appendix that explains it in more detail.

Want to create or adapt books like this? Learn more about how Pressbooks supports open publishing practices.
2.1 Descriptive Statistics and Frequency Distributions
Learning Objectives
By the end of this chapter, the student should be able to:
- Display and interpret categorical data
- Display and interpret quantitative data
- Recognize, describe, and calculate the measures of the center of quantitative data
- Recognize, describe, and calculate the measures of spread of quantitative data
- Recognize, describe, and calculate the measures of location of quantitative data
- Identify outliers in quantitative data
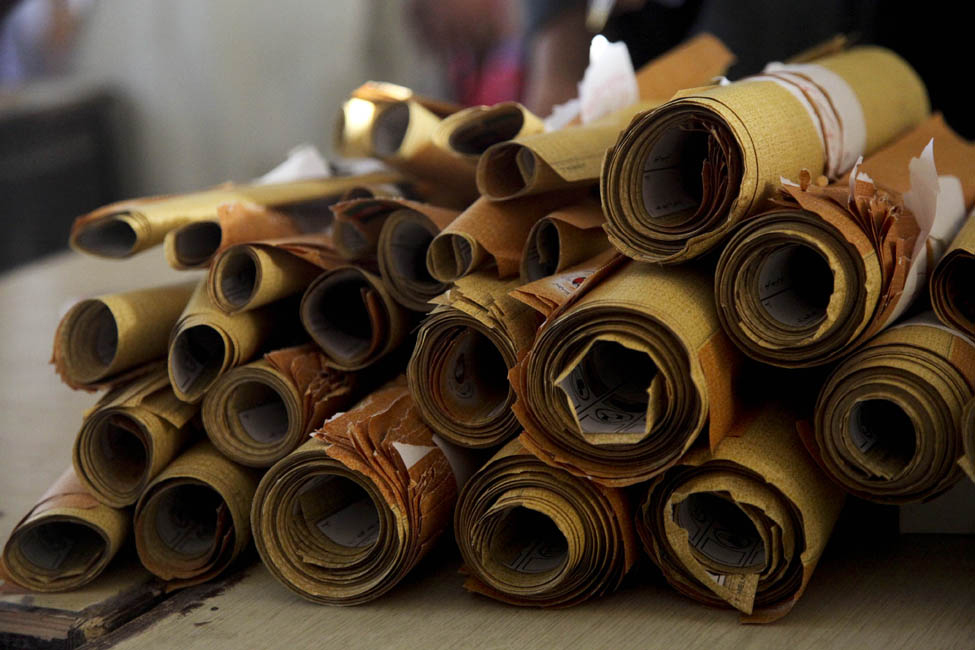
Descriptive Statistics
Once you collect data, what do you do with it? Data can be described and presented in many different formats. For example, suppose you are interested in buying a house in a particular area. If you have no clue about house prices, you might ask your real estate agent to give you a sample dataset of prices, but looking through all the prices can be overwhelming. A better way might be to look at numerical descriptions such as the average or median house price. Your agent might also provide you with a graph of the data.
In this chapter, you will study numerical and graphical ways to describe and display your data. This area of statistics is called descriptive statistics . We will look at both graphical and numerical descriptive methods. You will learn how to construct, calculate, and, most importantly, interpret these measurements and graphs.
Numerical descriptors consist of summary statistics (typically calculated from a sample) that represent important aspects such as the central tendency and variability of a distribution or the relative standing of a single observation with regard to the rest of the distribution.
Graphical descriptive methods consist of chart, tables, and graphs. These are tools that help you learn about the distribution , or shape, of a sample or a population. A graph can be a more effective way of presenting data than a mass of numbers because we can see where the data clusters and where there are only a few data values. Newspapers and the internet sources use graphs to show trends and to enable readers to compare facts and figures quickly. Statisticians often graph data first to get a picture of the data before more formal tools are applied.
The type of graph you choose first depends on the type of data with which you are working. Some of the types of graphs used to display categorical data are pie charts and bar charts. Some graphs that are used to summarize and organize quantitative data are the dot plot, the histogram, the stem-and-leaf plot, the frequency polygon, the box plot, and, in special cases, the time series plot. The emphasis here will be on histograms and box plots.
We will start by looking at a graphical method that can display any type of data, the frequency table.
Frequency Tables
Frequency tables are a great starting place for summarizing and organizing your data. Once you have a set of data, you may first want to organize it to see the frequency (how often each value occurs in the set).
Frequency tables can be used to show either quantitative or categorical data. Displaying categorical data in a frequency table is fairly straightforward since you already have clearly defined categories. For example, if you polled 20 kindergarteners on their favorite colors, you could construct the following simple frequency table:
Color | Frequency |
---|---|
Red | 2 |
Orange | 2 |
Yellow | 1 |
Green | 3 |
Blue | 4 |
Purple | 3 |
Pink | 4 |
Clear with sparkles | 1 |
Some quantitative data, especially discrete, may only a contain a limited number of values and little thought would be needed in creating the frequency table. Some data may have a natural grouping. For example, if you were organizing adults aged 20-69, it might make intuitive sense to group them as follows:
Consider the 30-39 grouping. Each group is typically called a class, or bin. In this case, 30 would be the lower class limit , while 39 is the upper class limit . The class width is defined as the difference between consecutive lower class limits. For the class 30–39, the class width is 40–30 = 10. The class midpoint is found by adding the lower limit and upper limit, then dividing by 2. For the class 30–39, the class midpoint would be calculated as follows:

Depending on the format and precision of the data reported, we may have to decide how best to bin, or group, our data. Grouping data may not always be a clean or intuitive process. A convenient starting point is a lower value carried out to one more decimal place than the value with the most decimal places. For example, if the value with the most decimal places is 6.1 and this is the smallest value, a convenient starting point is 6.05 (6.1 – 0.05 = 6.05), which is more precise. If the value with the most decimal places is 2.23 and the lowest value is 1.5, a convenient starting point is 1.495 (1.5 – 0.005 = 1.495). If the value with the most decimal places is 3.234 and the lowest value is 1.0, a convenient starting point is 0.9995 (1.0 – 0.0005 = 0.9995). If the data is entirely made up of integers and the smallest value is 2, then a convenient starting point is 1.5 (2 – 0.5 = 1.5). When the starting point and other boundaries are carried to one additional decimal place, no data value will fall on a boundary. The next two examples go into detail about how to construct a histogram using continuous data and how to create a histogram using discrete data.
The next question may concern how many bins to use. Generally anywhere from 5-20 bins, since too few does not display distribution well, but too many can have strange effects. A good place to start is the square root of your number of observations ( n ). Some other basic guidelines are that bins should not overlap or have gaps between them and should have the same width and cover the entire range of the data. The class limits and width should be “reasonable” numbers (e.g., whole numbers, or multiples of five or ten). In the end, it really just depends on the format of your data, but following these general guidelines should make sure your table is useful.
Relative Frequencies
A relative frequency is the ratio (fraction or proportion) of the number of times a value of the data occurs in the set of all outcomes to the total number of outcomes. To find the relative frequencies, divide each frequency by the total number of students in the sample—in this case, 20. Relative frequencies can be written as fractions, percents, or decimals. To find the relative frequency:

- f = frequency
- n = total number of data values (or the sum of the individual frequencies)
- RF = relative frequency

Cumulative relative frequency is the accumulation of the previous relative frequencies. To find the cumulative relative frequencies, add all the previous relative frequencies to the relative frequency for the current row, as shown in the figure below.
- The sum of all frequencies will add up to n , or your sample size.
- All relative frequencies should add up to one (pending rounding).
- The first entry of the cumulative relative frequency column will be the same as the first entry of the relative frequency column since there is nothing to accumulate.
- The last entry of the cumulative relative frequency column is one, indicating that 100% of the data has been accumulated.
The following table represents one way of grouping the heights, in inches, of a sample of 100 male semiprofessional soccer players.
Heights (inches) | Frequency | Relative frequency | Cumulative relative frequency |
---|---|---|---|
59.95–61.95 | 5 | 0.05 | |
61.95–63.95 | 3 | 0.05 + 0.03 = 0.08 | |
63.95–65.95 | 15 | 0.08 + 0.15 = 0.23 | |
65.95–67.95 | 40 | 0.23 + 0.40 = 0.63 | |
67.95–69.95 | 17 | 0.63 + 0.17 = 0.80 | |
69.95–71.95 | 12 | 0.80 + 0.12 = 0.92 | |
71.95–73.95 | 7 | 0.92 + 0.07 = 0.99 | |
73.95–75.95 | 1 | 0.99 + 0.01 = 1.00 | |
In this sample, there are five players whose heights fall within the interval 59.95–61.95 inches, three players whose heights fall within the interval 61.95–63.95 inches, 15 players whose heights fall within the interval 63.95–65.95 inches, 40 players whose height falls within the interval 65.95–67.95 inches, 17 players whose heights fall within the interval 67.95–69.95 inches, 12 players whose heights fall within the interval 69.95–71.95, seven players whose heights fall within the interval 71.95–73.95, and one player whose heights fall within the interval 73.95–75.95. All heights fall between the endpoints of an interval and not at the endpoints.
a. From the figure above, find the percentage of heights that are less than 65.95 inches.
b. Find the percentage of heights that fall between 61.95 and 65.95 inches.
c. Use the heights of the 100 male semiprofessional soccer players. Fill in the blanks, and check your answers.
e. Describe how you could gather this data (the heights) to make it characteristic of all male semiprofessional soccer players.
Remember, you count frequencies. To find the relative frequency, divide the frequency by the total number of data values. To find the cumulative relative frequency, add all of the previous relative frequencies to the relative frequency for the current row.
Twenty students were asked how many hours they worked per day. Their responses, in hours, are as follows: 5, 6, 3, 3, 2, 4, 7, 5, 2, 3, 5, 6, 5, 4, 4, 3, 5, 2, 5, and 3. Construct a bar graph that shows the registered voter population by district.
Construct an appropriate table including frequencies, relative frequencies, and cumulative relative frequencies.
Click here for more multimedia resources, including podcasts, videos, lecture notes, and worked examples.
Figure References
Figure 2.1: U.S. Marine Corps photo by Staff Sgt. William Greeson (2009). “US Navy 090821-M-0440G-043 Voting ballots organized and arranged for counting by Afghan presidential election workers at a local school in the Nawa District.” Public domain. https://commons.wikimedia.org/wiki/File:US_Navy_090821-M-0440G-043_Voting_ballots_organized_and_arranged_for_counting_by_Afghan_presidential_election_workers_at_a_local_school_in_the_Nawa_District.jpg
Methods of organizing, summarizing, and presenting data
Organizing, summarizing, or presenting data visually in graphs, figures, or charts
Numbers that summarize some aspect of a dataset, often calculated
The possible values a variable can take on and how often it does so
The number of times a value occurs in the data
The lower end of a bin or class in a frequency table or histogram
The upper end of a bin or class in a frequency table or histogram
The difference in consecutive lower class limits
Found by adding the lower limit and upper limit, then dividing by two
The percentage, proportion, or ratio of the frequency of a value of the data to the total number of outcomes
The sum of the relative frequencies for all values that are less than or equal to the given value
Significant Statistics Copyright © 2024 by John Morgan Russell, OpenStaxCollege, OpenIntro is licensed under a Creative Commons Attribution-ShareAlike 4.0 International License , except where otherwise noted.
Share This Book
MA121: Introduction to Statistics
Descriptive and Inferential Statistics
Read these sections and complete the questions at the end of each section. Here, we introduce descriptive statistics using examples and discuss the difference between descriptive and inferential statistics. We also talk about samples and populations, explain how you can identify biased samples, and define differential statistics.
Descriptive Statistics
- inferential and descriptive.
- population and sample.
- sampling and scaling.
- mean and median.
- allow random assignment to experimental conditions.
- use data from a sample to answer questions about a population.
- summarize and describe data.
- allow you to generalize beyond the data at hand.
- The mean age of people in Detroit.
- The number of people who watched the superbowl in the year 2002.
- A prediction of next month's unemployment rate.
- The median price of new homes sold in Miami.
- The height of the tallest woman in the world.
Have a language expert improve your writing
Run a free plagiarism check in 10 minutes, generate accurate citations for free.
- Knowledge Base
- Inferential Statistics | An Easy Introduction & Examples
Inferential Statistics | An Easy Introduction & Examples
Published on September 4, 2020 by Pritha Bhandari . Revised on June 22, 2023.
While descriptive statistics summarize the characteristics of a data set, inferential statistics help you come to conclusions and make predictions based on your data.
When you have collected data from a sample , you can use inferential statistics to understand the larger population from which the sample is taken.
Inferential statistics have two main uses:
- making estimates about populations (for example, the mean SAT score of all 11th graders in the US).
- testing hypotheses to draw conclusions about populations (for example, the relationship between SAT scores and family income).
Table of contents
Descriptive versus inferential statistics, estimating population parameters from sample statistics, hypothesis testing, other interesting articles, frequently asked questions about inferential statistics.
Descriptive statistics allow you to describe a data set, while inferential statistics allow you to make inferences based on a data set.
- Descriptive statistics
Using descriptive statistics, you can report characteristics of your data:
- The distribution concerns the frequency of each value.
- The central tendency concerns the averages of the values.
- The variability concerns how spread out the values are.
In descriptive statistics, there is no uncertainty – the statistics precisely describe the data that you collected. If you collect data from an entire population, you can directly compare these descriptive statistics to those from other populations.
Inferential statistics
Most of the time, you can only acquire data from samples, because it is too difficult or expensive to collect data from the whole population that you’re interested in.
While descriptive statistics can only summarize a sample’s characteristics, inferential statistics use your sample to make reasonable guesses about the larger population.
With inferential statistics, it’s important to use random and unbiased sampling methods . If your sample isn’t representative of your population, then you can’t make valid statistical inferences or generalize .
Sampling error in inferential statistics
Since the size of a sample is always smaller than the size of the population, some of the population isn’t captured by sample data. This creates sampling error , which is the difference between the true population values (called parameters) and the measured sample values (called statistics).
Sampling error arises any time you use a sample, even if your sample is random and unbiased. For this reason, there is always some uncertainty in inferential statistics. However, using probability sampling methods reduces this uncertainty.
Prevent plagiarism. Run a free check.
The characteristics of samples and populations are described by numbers called statistics and parameters :
- A statistic is a measure that describes the sample (e.g., sample mean ).
- A parameter is a measure that describes the whole population (e.g., population mean).
Sampling error is the difference between a parameter and a corresponding statistic. Since in most cases you don’t know the real population parameter, you can use inferential statistics to estimate these parameters in a way that takes sampling error into account.
There are two important types of estimates you can make about the population: point estimates and interval estimates .
- A point estimate is a single value estimate of a parameter. For instance, a sample mean is a point estimate of a population mean.
- An interval estimate gives you a range of values where the parameter is expected to lie. A confidence interval is the most common type of interval estimate.
Both types of estimates are important for gathering a clear idea of where a parameter is likely to lie.
Confidence intervals
A confidence interval uses the variability around a statistic to come up with an interval estimate for a parameter. Confidence intervals are useful for estimating parameters because they take sampling error into account.
While a point estimate gives you a precise value for the parameter you are interested in, a confidence interval tells you the uncertainty of the point estimate. They are best used in combination with each other.
Each confidence interval is associated with a confidence level. A confidence level tells you the probability (in percentage) of the interval containing the parameter estimate if you repeat the study again.
A 95% confidence interval means that if you repeat your study with a new sample in exactly the same way 100 times, you can expect your estimate to lie within the specified range of values 95 times.
Although you can say that your estimate will lie within the interval a certain percentage of the time, you cannot say for sure that the actual population parameter will. That’s because you can’t know the true value of the population parameter without collecting data from the full population.
However, with random sampling and a suitable sample size, you can reasonably expect your confidence interval to contain the parameter a certain percentage of the time.
Your point estimate of the population mean paid vacation days is the sample mean of 19 paid vacation days.
Hypothesis testing is a formal process of statistical analysis using inferential statistics. The goal of hypothesis testing is to compare populations or assess relationships between variables using samples.
Hypotheses , or predictions, are tested using statistical tests . Statistical tests also estimate sampling errors so that valid inferences can be made.
Statistical tests can be parametric or non-parametric. Parametric tests are considered more statistically powerful because they are more likely to detect an effect if one exists.
Parametric tests make assumptions that include the following:
- the population that the sample comes from follows a normal distribution of scores
- the sample size is large enough to represent the population
- the variances , a measure of variability , of each group being compared are similar
When your data violates any of these assumptions, non-parametric tests are more suitable. Non-parametric tests are called “distribution-free tests” because they don’t assume anything about the distribution of the population data.
Statistical tests come in three forms: tests of comparison, correlation or regression.
Comparison tests
Comparison tests assess whether there are differences in means, medians or rankings of scores of two or more groups.
To decide which test suits your aim, consider whether your data meets the conditions necessary for parametric tests, the number of samples, and the levels of measurement of your variables.
Means can only be found for interval or ratio data , while medians and rankings are more appropriate measures for ordinal data .
test | Yes | Means | 2 samples |
---|---|---|---|
Yes | Means | 3+ samples | |
Mood’s median | No | Medians | 2+ samples |
Wilcoxon signed-rank | No | Distributions | 2 samples |
Wilcoxon rank-sum (Mann-Whitney ) | No | Sums of rankings | 2 samples |
Kruskal-Wallis | No | Mean rankings | 3+ samples |
Correlation tests
Correlation tests determine the extent to which two variables are associated.
Although Pearson’s r is the most statistically powerful test, Spearman’s r is appropriate for interval and ratio variables when the data doesn’t follow a normal distribution.
The chi square test of independence is the only test that can be used with nominal variables.
Pearson’s | Yes | Interval/ratio variables |
---|---|---|
Spearman’s | No | Ordinal/interval/ratio variables |
Chi square test of independence | No | Nominal/ordinal variables |
Regression tests
Regression tests demonstrate whether changes in predictor variables cause changes in an outcome variable. You can decide which regression test to use based on the number and types of variables you have as predictors and outcomes.
Most of the commonly used regression tests are parametric. If your data is not normally distributed, you can perform data transformations.
Data transformations help you make your data normally distributed using mathematical operations, like taking the square root of each value.
1 interval/ratio variable | 1 interval/ratio variable | |
2+ interval/ratio variable(s) | 1 interval/ratio variable | |
Logistic regression | 1+ any variable(s) | 1 binary variable |
Nominal regression | 1+ any variable(s) | 1 nominal variable |
Ordinal regression | 1+ any variable(s) | 1 ordinal variable |
If you want to know more about statistics , methodology , or research bias , make sure to check out some of our other articles with explanations and examples.
- Confidence interval
- Measures of central tendency
- Correlation coefficient
Methodology
- Cluster sampling
- Stratified sampling
- Types of interviews
- Cohort study
- Thematic analysis
Research bias
- Implicit bias
- Cognitive bias
- Survivorship bias
- Availability heuristic
- Nonresponse bias
- Regression to the mean
Receive feedback on language, structure, and formatting
Professional editors proofread and edit your paper by focusing on:
- Academic style
- Vague sentences
- Style consistency
See an example
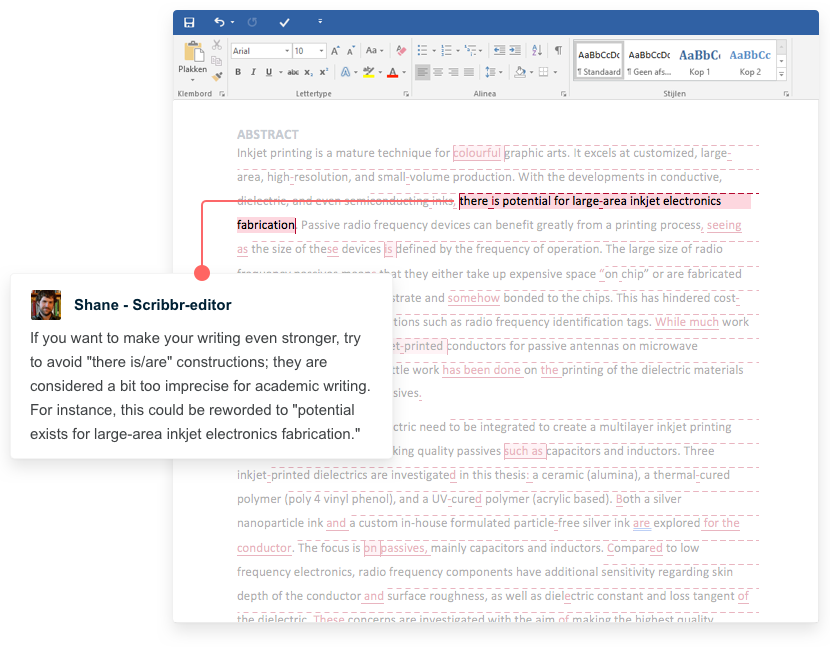
Descriptive statistics summarize the characteristics of a data set. Inferential statistics allow you to test a hypothesis or assess whether your data is generalizable to the broader population.
A statistic refers to measures about the sample , while a parameter refers to measures about the population .
A sampling error is the difference between a population parameter and a sample statistic .
Hypothesis testing is a formal procedure for investigating our ideas about the world using statistics. It is used by scientists to test specific predictions, called hypotheses , by calculating how likely it is that a pattern or relationship between variables could have arisen by chance.
Cite this Scribbr article
If you want to cite this source, you can copy and paste the citation or click the “Cite this Scribbr article” button to automatically add the citation to our free Citation Generator.
Bhandari, P. (2023, June 22). Inferential Statistics | An Easy Introduction & Examples. Scribbr. Retrieved September 9, 2024, from https://www.scribbr.com/statistics/inferential-statistics/
Is this article helpful?
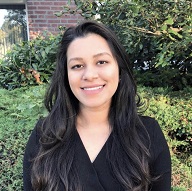
Pritha Bhandari
Other students also liked, parameter vs statistic | definitions, differences & examples, descriptive statistics | definitions, types, examples, hypothesis testing | a step-by-step guide with easy examples, what is your plagiarism score.
- Search Search Please fill out this field.
What Are Descriptive Statistics?
- How They Work
Univariate vs. Bivariate
Descriptive statistics and visualizations, descriptive statistics and outliers.
- Descriptive vs. Inferential
The Bottom Line
- Corporate Finance
- Financial Analysis
Descriptive Statistics: Definition, Overview, Types, and Examples
Adam Hayes, Ph.D., CFA, is a financial writer with 15+ years Wall Street experience as a derivatives trader. Besides his extensive derivative trading expertise, Adam is an expert in economics and behavioral finance. Adam received his master's in economics from The New School for Social Research and his Ph.D. from the University of Wisconsin-Madison in sociology. He is a CFA charterholder as well as holding FINRA Series 7, 55 & 63 licenses. He currently researches and teaches economic sociology and the social studies of finance at the Hebrew University in Jerusalem.
:max_bytes(150000):strip_icc():format(webp)/adam_hayes-5bfc262a46e0fb005118b414.jpg)
Descriptive statistics are brief informational coefficients that summarize a given data set, which can be either a representation of the entire population or a sample of a population. Descriptive statistics are broken down into measures of central tendency and measures of variability (spread). Measures of central tendency include the mean , median , and mode , while measures of variability include standard deviation , variance , minimum and maximum variables, kurtosis , and skewness .
Key Takeaways
- Descriptive statistics summarizes or describes the characteristics of a data set.
- Descriptive statistics consists of three basic categories of measures: measures of central tendency, measures of variability (or spread), and frequency distribution.
- Measures of central tendency describe the center of the data set (mean, median, mode).
- Measures of variability describe the dispersion of the data set (variance, standard deviation).
- Measures of frequency distribution describe the occurrence of data within the data set (count).
Jessica Olah
Understanding Descriptive Statistics
Descriptive statistics help describe and explain the features of a specific data set by giving short summaries about the sample and measures of the data. The most recognized types of descriptive statistics are measures of center. For example, the mean, median, and mode, which are used at almost all levels of math and statistics, are used to define and describe a data set. The mean, or the average, is calculated by adding all the figures within the data set and then dividing by the number of figures within the set.
For example, the sum of the following data set is 20: (2, 3, 4, 5, 6). The mean is 4 (20/5). The mode of a data set is the value appearing most often, and the median is the figure situated in the middle of the data set. It is the figure separating the higher figures from the lower figures within a data set. However, there are less common types of descriptive statistics that are still very important.
People use descriptive statistics to repurpose hard-to-understand quantitative insights across a large data set into bite-sized descriptions. A student's grade point average (GPA), for example, provides a good understanding of descriptive statistics. The idea of a GPA is that it takes data points from a range of individual course grades, and averages them together to provide a general understanding of a student's overall academic performance. A student's personal GPA reflects their mean academic performance.
Descriptive statistics, especially in fields such as medicine, often visually depict data using scatter plots, histograms, line graphs, or stem and leaf displays. We'll talk more about visuals later in this article.
Types of Descriptive Statistics
All descriptive statistics are either measures of central tendency or measures of variability , also known as measures of dispersion.
Central Tendency
Measures of central tendency focus on the average or middle values of data sets, whereas measures of variability focus on the dispersion of data. These two measures use graphs, tables, and general discussions to help people understand the meaning of the analyzed data.
Measures of central tendency describe the center position of a distribution for a data set. A person analyzes the frequency of each data point in the distribution and describes it using the mean, median, or mode, which measures the most common patterns of the analyzed data set.
Measures of Variability
Measures of variability (or measures of spread) aid in analyzing how dispersed the distribution is for a set of data. For example, while the measures of central tendency may give a person the average of a data set, it does not describe how the data is distributed within the set.
So while the average of the data might be 65 out of 100, there can still be data points at both 1 and 100. Measures of variability help communicate this by describing the shape and spread of the data set. Range, quartiles , absolute deviation, and variance are all examples of measures of variability.
Consider the following data set: 5, 19, 24, 62, 91, 100. The range of that data set is 95, which is calculated by subtracting the lowest number (5) in the data set from the highest (100).
Distribution
Distribution (or frequency distribution) refers to the number of times a data point occurs. Alternatively, it can be how many times a data point fails to occur. Consider this data set: male, male, female, female, female, other. The distribution of this data can be classified as:
- The number of males in the data set is 2.
- The number of females in the data set is 3.
- The number of individuals identifying as other is 1.
- The number of non-males is 4.
In descriptive statistics, univariate data analyzes only one variable. It is used to identify characteristics of a single trait and is not used to analyze any relationships or causations.
For example, imagine a room full of high school students. Say you wanted to gather the average age of the individuals in the room. This univariate data is only dependent on one factor: each person's age. By gathering this one piece of information from each person and dividing by the total number of people, you can determine the average age.
Bivariate data, on the other hand, attempts to link two variables by searching for correlation. Two types of data are collected, and the relationship between the two pieces of information is analyzed together. Because multiple variables are analyzed, this approach may also be referred to as multivariate .
Let's say each high school student in the example above takes a college assessment test, and we want to see whether older students are testing better than younger students. In addition to gathering the ages of the students, we need to find out each student's test score. Then, using data analytics, we mathematically or graphically depict whether there is a relationship between student age and test scores.
The preparation and reporting of financial statements is an example of descriptive statistics. Analyzing that financial information to make decisions on the future is inferential statistics.
One essential aspect of descriptive statistics is graphical representation. Visualizing data distributions effectively can be incredibly powerful, and this is done in several ways.
Histograms are tools for displaying the distribution of numerical data. They divide the data into bins or intervals and represent the frequency or count of data points falling into each bin through bars of varying heights. Histograms help identify the shape of the distribution, central tendency, and variability of the data.
Another visualization is boxplots. Boxplots, also known as box-and-whisker plots, provide a concise summary of a data distribution by highlighting key summary statistics including the median (middle line inside the box), quartiles (edges of the box), and potential outliers (points outside, or the "whiskers"). Boxplots visually depict the spread and skewness of the data and are particularly useful for comparing distributions across different groups or variables.
Whenever descriptive statistics are being discussed, it's important to note outliers. Outliers are data points that significantly differ from other observations in a dataset. These could be errors, anomalies, or rare events within the data.
Detecting and managing outliers is a step in descriptive statistics to ensure accurate and reliable data analysis. To identify outliers, you can use graphical techniques (such as boxplots or scatter plots) or statistical methods (such as Z-score or IQR method). These approaches help pinpoint observations that deviate substantially from the overall pattern of the data.
The presence of outliers can have a notable impact on descriptive statistics, skewing results and affecting the interpretation of data. Outliers can disproportionately influence measures of central tendency, such as the mean, pulling it towards their extreme values. For example, the dataset of (1, 1, 1, 997) is 250, even though that is hardly representative of the dataset. This distortion can lead to misleading conclusions about the typical behavior of the dataset.
Depending on the context, outliers can often be treated by removing them (if they are genuinely erroneous or irrelevant). Alternatively, outliers may hold important information and should be kept for the value they may be able to demonstrate. As you analyze your data, consider the relevance of what outliers can contribute and whether it makes more sense to just strike those data points from your descriptive statistic calculations.
Descriptive Statistics vs. Inferential Statistics
Descriptive statistics have a different function from inferential statistics, which are data sets that are used to make decisions or apply characteristics from one data set to another.
Imagine another example where a company sells hot sauce. The company gathers data such as the count of sales , average quantity purchased per transaction , and average sale per day of the week. All of this information is descriptive, as it tells a story of what actually happened in the past. In this case, it is not being used beyond being informational.
Now let's say that the company wants to roll out a new hot sauce. It gathers the same sales data above, but it uses the information to make predictions about what the sales of the new hot sauce will be. The act of using descriptive statistics and applying characteristics to a different data set makes the data set inferential statistics. We are no longer simply summarizing data; we are using it to predict what will happen regarding an entirely different body of data (in this case, the new hot sauce product).
What Is Descriptive Statistics?
Descriptive statistics is a means of describing features of a data set by generating summaries about data samples. For example, a population census may include descriptive statistics regarding the ratio of men and women in a specific city.
What Are Examples of Descriptive Statistics?
In recapping a Major League Baseball season, for example, descriptive statistics might include team batting averages, the number of runs allowed per team, and the average wins per division.
What Is the Main Purpose of Descriptive Statistics?
The main purpose of descriptive statistics is to provide information about a data set. In the example above, there are dozens of baseball teams, hundreds of players, and thousands of games. Descriptive statistics summarizes large amounts of data into useful bits of information.
What Are the Types of Descriptive Statistics?
The three main types of descriptive statistics are frequency distribution, central tendency, and variability of a data set. The frequency distribution records how often data occurs, central tendency records the data's center point of distribution, and variability of a data set records its degree of dispersion.
Can Descriptive Statistics Be Used to Make Inferences or Predictions?
Technically speaking, descriptive statistics only serves to help understand historical data attributes. Inferential statistics—a separate branch of statistics—is used to understand how variables interact with one another in a data set and possibly predict what might happen in the future.
Descriptive statistics refers to the analysis, summary, and communication of findings that describe a data set. Often not useful for decision-making, descriptive statistics still hold value in explaining high-level summaries of a set of information such as the mean, median, mode, variance, range, and count of information.
Purdue Online Writing Lab. " Writing with Statistics: Descriptive Statistics ."
National Library of Medicine. " Descriptive Statistics for Summarizing Data ."
CSUN.edu. " Measures of Variability, Descriptive Statistics Part 2 ."
Math.Kent.edu. " Summary: Differences Between Univariate and Bivariate Data ."
Purdue Online Writing Lab. " Writing with Statistics: Basic Inferential Statistics: Theory and Application ."
:max_bytes(150000):strip_icc():format(webp)/durbin-watson-statistic-4197394-final-1-1598921e038a404aa070411c9ca2cf9f.png)
- Terms of Service
- Editorial Policy
- Privacy Policy
- Your Privacy Choices
- School Guide
- Mathematics
- Number System and Arithmetic
- Trigonometry
- Probability
- Mensuration
- Maths Formulas
- Class 8 Maths Notes
- Class 9 Maths Notes
- Class 10 Maths Notes
- Class 11 Maths Notes
- Class 12 Maths Notes
Descriptive Statistics
Descriptive statistics is a subfield of statistics that deals with characterizing the features of known data. Descriptive statistics give summaries of either population or sample data. Aside from descriptive statistics, inferential statistics is another important discipline of statistics used to draw conclusions about population data.
Descriptive statistics is divided into two categories:
Measures of Central Tendency
Measures of dispersion.
In this article, we will learn about descriptive statistics, including their many categories, formulae, and examples in detail.
What is Descriptive Statistics?
Descriptive statistics is a branch of statistics focused on summarizing, organizing, and presenting data in a clear and understandable way. Its primary aim is to define and analyze the fundamental characteristics of a dataset without making sweeping generalizations or assumptions about the entire data set.
The main purpose of descriptive statistics is to provide a straightforward and concise overview of the data, enabling researchers or analysts to gain insights and understand patterns, trends, and distributions within the dataset.
Descriptive statistics typically involve measures of central tendency (such as mean, median, mode), dispersion (such as range, variance, standard deviation), and distribution shape (including skewness and kurtosis). Additionally, graphical representations like charts, graphs, and tables are commonly used to visualize and interpret the data.
Histograms, bar charts, pie charts, scatter plots, and box plots are some examples of widely used graphical techniques in descriptive statistics.
Descriptive Statistics Definition
Descriptive statistics is a type of statistical analysis that uses quantitative methods to summarize the features of a population sample. It is useful to present easy and exact summaries of the sample and observations using metrics such as mean, median, variance, graphs, and charts.
Types of Descriptive Statistics
There are three types of descriptive statistics:
Measures of Frequency Distribution
The central tendency is defined as a statistical measure that may be used to describe a complete distribution or dataset with a single value, known as a measure of central tendency. Any of the central tendency measures accurately describes the whole data distribution. In the following sections, we will look at the central tendency measures, their formulae, applications, and kinds in depth.
Mean is the sum of all the components in a group or collection divided by the number of items in that group or collection. Mean of a data collection is typically represented as x̄ (pronounced “x bar”). The formula for calculating the mean for ungrouped data to express it as the measure is given as follows:
For a series of observations:
x̄ = Σx / n
- x̄ = Mean Value of Provided Dataset
- Σx = Sum of All Terms
- n = Number of Terms
Example: Weights of 7 girls in kg are 54, 32, 45, 61, 20, 66 and 50. Determine the mean weight for the provided collection of data.
Mean = Σx/n = (54 + 32 + 45 + 61 + 20 + 66 + 50)/7 = 328 / 7 = 46.85 Thus, the group’s mean weight is 46.85 kg.
Median of a data set is the value of the middle-most observation obtained after organizing the data in ascending order, which is one of the measures of central tendency. Median formula may be used to compute the median for many types of data, such as grouped and ungrouped data.
Ungrouped Data Median (n is odd): [(n + 1)/2] th term Ungrouped Data Median (n is even): [(n / 2) th term + ((n / 2) + 1) th term]/2
Example: Weights of 7 girls in kg are 54, 32, 45, 61, 20, 66 and 50. Determine the median weight for the provided collection of data.
Arrange the provided data collection in ascending order: 20, 32, 45, 50, 54, 61, 66 Median = [(n + 1) / 2] th term = [(7 + 1) / 2] th term = 4 th term = 50 Thus, group’s median weight is 50 kg.
Mode is one of the measures of central tendency, defined as the value that appears the most frequently in the provided data, i.e. the observation with the highest frequency is known as the mode of data. The mode formulae provided below can be used to compute the mode for ungrouped data.
Mode of Ungrouped Data: Most Repeated Observation in Dataset
Example: Weights of 7 girls in kg are 54, 32, 45, 61, 20, 45 and 50. Determine the mode weight for the provided collection of data.
Mode = Most repeated observation in Dataset = 45 Thus, group’s mode weight is 45 kg.
If the variability of data within an experiment must be established, absolute measures of variability should be employed. These metrics often reflect differences in a data collection in terms of the average deviations of the observations. The most prevalent absolute measurements of deviation are mentioned below. In the following sections, we will look at the variability measures, their formulae in depth.
Standard Deviation
The range represents the spread of your data from the lowest to the highest value in the distribution. It is the most straightforward measure of variability to compute. To get the range, subtract the data set’s lowest and highest values.
Range = Highest Value – Lowest Value
Example: Calculate the range of the following data series: 5, 13, 32, 42, 15, 84
Arrange the provided data series in ascending order: 5, 13, 15, 32, 42, 84 Range = H – L = 84 – 5 = 79 So, the range is 79.
Standard deviation (s or SD) represents the average level of variability in your dataset. It represents the average deviation of each score from the mean. The higher the standard deviation, the more varied the dataset is.
To calculate standard deviation, follow these six steps:
Step 1: Make a list of each score and calculate the mean.
Step 2: Calculate deviation from the mean, by subtracting the mean from each score.
Step 3: Square each of these differences.
Step 4: Sum up all squared variances.
Step 5: Divide the total of squared variances by N-1.
Step 6: Find the square root of the number that you discovered.
Example: Calculate standard deviation of the following data series: 5, 13, 32, 42, 15, 84.
Step 1: First we have to calculate the mean of following series using formula: Σx / n
Step 2: Now calculate the deviation from mean, subtract the mean from each series.
Step 3: Squared the deviation from mean and then add all the deviation.
Series | Deviation from Mean | Squared Deviation |
---|---|---|
5 | 5-31.83 = -26.83 | 719.85 |
13 | 13-31.83 = -18.83 | 354.57 |
32 | 32-31.83 = 0.17 | 0.0289 |
42 | 42-31.83 = 10.17 | 103.43 |
15 | 15-31.83 = -16.83 | 283.25 |
84 | 84-31.83 = 52.17 | 2721.71 |
Mean = 191/6 = 31.83 | sum = 0 | Sum = 4182.84 |
Step 4: Divide the squared deviation with N-1 => 4182.84 / 5 = 836.57
Step 5: √836.57 = 28.92
So, the standard deviation is 28.92
Variance is calculated as average of squared departures from the mean. Variance measures the degree of dispersion in a data collection. The more scattered the data, the larger the variance in relation to the mean. To calculate the variance, square the standard deviation.
Symbol for variance is s 2
Example: Calculate the variance of the following data series: 5, 13, 32, 42, 15, 84.
First we have to calculate the standard deviation, that we calculate above i.e. SD = 28.92 s 2 = (SD) 2 = (28.92) 2 = 836.37 So, the variance is 836.37
Mean Deviation
Mean Deviation is used to find the average of the absolute value of the data about the mean, median, or mode. Mean Deviation is some times also known as absolute deviation. The formula mean deviation is given as follows:
Mean Deviation = ∑ n 1 |X – μ|/n
- μ is Central Value
Quartile Deviation
Quartile Deviation is the Half of difference between the third and first quartile. The formula for quartile deviation is given as follows:
Quartile Deviation = (Q 3 − Q 1 )/2
- Q 3 is Third Quartile
- Q 1 is First Quartile
Other measures of dispersion include the relative measures also known as the coefficients of dispersion.
Datasets consist of various scores or values. Statisticians employ graphs and tables to summarize the occurrence of each possible value of a variable, often presented in percentages or numerical figures.
For instance, suppose you were conducting a poll to determine people’s favorite Beatles. You would create one column listing all potential options (John, Paul, George, and Ringo) and another column indicating the number of votes each received. Statisticians represent these frequency distributions through graphs or tables
Univariate Descriptive Statistics
Univariate descriptive statistics focus on one thing at a time. We look at each thing individually and use different ways to understand it better. Programs like SPSS and Excel can help us with this.
If we only look at the average (mean) of something, like how much people earn, it might not give us the true picture, especially if some people earn a lot more or less than others. Instead, we can also look at other things like the middle value (median) or the one that appears most often (mode). And to understand how spread out the values are, we use things like standard deviation and variance along with the range.
Bivariate Descriptive Statistics
When we have information about more than one thing, we can use bivariate or multivariate descriptive statistics to see if they are related. Bivariate analysis compares two things to see if they change together. Before doing any more complicated tests, it’s important to look at how the two things compare in the middle.
Multivariate analysis is similar to bivariate analysis, but it looks at more than two things at once, which helps us understand relationships even better.
Representations of Data in Descriptive Statistics
Descriptive statistics use a variety of ways to summarize and present data in an understandable manner. This helps us grasp the data set’s patterns, trends, and properties.
Frequency Distribution Tables: Frequency distribution tables divide data into categories or intervals and display the number of observations (frequency) that fall into each one. For example, suppose we have a class of 20 students and are tracking their test scores. We may make a frequency distribution table that contains score ranges (e.g., 0-10, 11-20) and displays how many students scored in each range.
Graphs and Charts: Graphs and charts graphically display data, making it simpler to understand and analyze. For example, using the same test score data, we may generate a bar graph with the x-axis representing score ranges and the y-axis representing the number of students. Each bar on the graph represents a score range, and its height shows the number of students scoring within that range.
These approaches help us summarize and visualize data, making it easier to discover trends, patterns, and outliers, which is critical for making informed decisions and reaching meaningful conclusions in a variety of sectors.
Descriptive Statistics Applications
Descriptive statistics are used in a variety of sectors to summarize, organize, and display data in a meaningful and intelligible way. Here are a few popular applications:
- Business and Economics: Descriptive statistics are useful for analyzing sales data, market trends, and customer behaviour. They are used to generate averages, medians, and standard deviations in order to better evaluate product performance, pricing strategies, and financial metrics.
- Healthcare: Descriptive statistics are used to analyze patient data such as demographics, medical histories, and treatment outcomes. They assist healthcare workers in determining illness prevalence, assessing treatment efficacy, and identifying risk factors.
- Education: Descriptive statistics are useful in education since they summarize student performance on tests and examinations. They assist instructors in assessing instructional techniques, identifying areas for improvement, and monitoring student growth over time.
- Market Research: Descriptive statistics are used to analyze customer preferences, product demand, and market trends. They enable businesses to make educated decisions about product development, advertising campaigns, and market segmentation.
- Finance and investment: Descriptive statistics are used to analyze stock market data, portfolio performance, and risk management. They assist investors in determining investment possibilities, tracking asset values, and evaluating financial instruments.
Difference Between Descriptive Statistics and Inferential Statistics
Difference between Descriptive Statistics and Inferential Statistics is studied using the table added below as,
Descriptive Statistics vs Inferential Statistics | |
---|---|
Descriptive Statistics |
|
Does not need making predictions or generalizations outside the dataset. | This involves making forecasts or generalizations about a wider population. |
Gives basic summary of the sample. | Concludes about the population based on the sample. |
include mean, median, mode, standard deviation, etc. | include hypothesis testing, confidence intervals, regression analysis, etc. |
Focuses on the properties of the current dataset. | Concentrates on drawing conclusions about the population from sample data. |
Helpful for comprehending data patterns and linkages. | Useful for making judgements, predictions, and drawing inferences that go beyond the observed facts. |
Example of Descriptive Statistics Examples
Example 1: Calculate the Mean, Median and Mode for the following series: {4, 8, 9, 10, 6, 12, 14, 4, 5, 3, 4}
First, we are going to calculate the mean. Mean = Σx / n = (4 + 8 + 9 + 10 + 6 + 12 + 14 + 4 + 5 + 3 + 4)/11 = 79 / 11 = 7.1818 Thus, the Mean is 7.1818. Now, we are going to calculate the median. Arrange the provided data collection in ascending order: 3, 4, 4, 4, 5, 6, 8, 9, 10, 12, 14 Median = [(n + 1) / 2] th term = [(11 + 1) / 2] th term = 6 th term = 6 Thus, the median is 6. Now, we are going to calculate the mode. Mode = The most repeated observation in the dataset = 4 Thus, the mode is 4.
Example 2: Calculate the Range for the following series: {4, 8, 9, 10, 6, 12, 14, 4, 5, 3, 4}
Arrange the provided data series in ascending order: 3, 4, 4, 4, 5, 6, 8, 9, 10, 12, 14 Range = H – L = 14 – 3 = 11 So, the range is 11.
Example 3: Calculate the standard deviation and variance of following data: {12, 24, 36, 48, 10, 18}
First we are going to compute standard deviation. For standard deviation calculate the mean, deviation from mean and squared deviation.
Series | Deviation from Mean | Squared Deviation |
---|---|---|
12 | 12-24.66 = -12.66 | 160.28 |
24 | 24-24.66 = -0.66 | 0.436 |
36 | 36-24.66 = 11.34 | 128.595 |
48 | 48-24.66 = 23.34 | 544.76 |
10 | 10-24.66 = -14.66 | 214.92 |
18 | 18-24.66 = -6.66 | 44.36 |
Mean = 148/6 = 24.66 | sum = 0 | Sum = 1093.351 |
Dividing squared deviation with N-1 => 1093.351 / 5 = 218.67
√(218.67) = 14.79
So, the standard deviation is 14.79.
Now we are going to calculate the variance.
s 2 = 218.744
So, the variance is 218.744
Practice Problems on Descriptive Statistics
P1) Determine the sample variance of the following series: {17, 21, 52, 28, 26, 23}
P2) Determine the mean and mode of the following series: {21, 14, 56, 41, 18, 15, 18, 21, 15, 18}
P3) Find the median of the following series: {7, 24, 12, 8, 6, 23, 11}
P4) Find the standard deviation and variance of the following series: {17, 28, 42, 48, 36, 42, 20}
FAQs of Descriptive Statistics
What is meant by descriptive statistics.
Descriptive statistics seek to summarize, organize, and display data in an accessible manner while avoiding making sweeping generalizations about the whole population. It aids in discovering patterns, trends, and distributions within the collection.
How is the mean computed in descriptive statistics?
Mean is computed by adding together all of the values in the dataset and dividing them by the total number of observations. It measures the dataset’s central tendency or average value.
What role do measures of variability play in descriptive statistics?
Measures of variability, such as range, standard deviation, and variance, aid in quantifying the spread or dispersion of data points around the mean. They give insights on the dataset’s variety and consistency.
Can you explain the median in descriptive statistics?
The median is the midpoint value of a dataset whether sorted ascending or descending. It measures central tendency and is important when dealing with skewed data or outliers.
How can frequency distribution measurements contribute to descriptive statistics?
Measures of frequency distribution summarize the incidence of various values or categories within a dataset. They give insights into the distribution pattern of the data and are commonly represented by graphs or tables.
How are inferential statistics distinguished from descriptive statistics?
Inferential statistics use sample data to draw inferences or make predictions about a wider population, whereas descriptive statistics summarize aspects of known data. Descriptive statistics concentrate on the present dataset, whereas inferential statistics go beyond the observable data.
Why are descriptive statistics necessary in data analysis?
Descriptive statistics give researchers and analysts a clear and straightforward summary of the dataset, helping them to identify patterns, trends, and distributions. It aids in making educated judgements and gaining valuable insights from data.
What are the four types of descriptive statistics?
There are four major types of descriptive statistics: Measures of Frequency Measures of Central Tendency Measures of Dispersion or Variation Measures of Position
Which is an example of descriptive statistics?
Descriptive statistics examples include the study of mean, median, and mode.
Please Login to comment...
Similar reads.
- School Learning
- Math-Statistics
- 105 Funny Things to Do to Make Someone Laugh
- Best PS5 SSDs in 2024: Top Picks for Expanding Your Storage
- Best Nintendo Switch Controllers in 2024
- Xbox Game Pass Ultimate: Features, Benefits, and Pricing in 2024
- #geekstreak2024 – 21 Days POTD Challenge Powered By Deutsche Bank
Improve your Coding Skills with Practice
What kind of Experience do you want to share?
Statistical Research Questions: Five Examples for Quantitative Analysis
Table of contents, introduction.
How are statistical research questions for quantitative analysis written? This article provides five examples of statistical research questions that will allow statistical analysis to take place.
Thus, before writing your statistical research questions and reading the examples in this article, read first the article that enumerates the four types of measurement scales . Knowing the four types of measurement scales will enable you to appreciate the formulation or structuring of research questions.
Five Examples of Statistical Research Questions
In writing the statistical research questions, I provide a topic that shows the variables of the study, the study description, and a link to the original scientific article to give you a glimpse of the real-world examples.
Topic 1: Physical Fitness and Academic Achievement
Statistical research question no. 1.
Is there a significant relationship between physical fitness and academic achievement?
On the other hand, the researchers measured academic achievement in terms of a passing score in Mathematics and English. The variable is the number of passing scores in both Mathematics and English.
Most of what I discuss in the statistics articles I wrote came from self-study. It’s easier to understand concepts now as there are a lot of resource materials available online. Videos and ebooks from places like Youtube, Veoh, The Internet Archives, among others, provide free educational materials. Online education will be the norm of the future. I describe this situation in my post about Education 4.0 .
Topic 2: Climate Conditions and Consumption of Bottled Water
Statistical research question no. 2.
Is there a significant relationship between average temperature and amount of bottled water consumed?
Now, it’s easy to identify the statistical test to analyze the relationship between the two variables. You may refer to my previous post titled Parametric Statistics: Four Widely Used Parametric Tests and When to Use Them . Using the figure supplied in that article, the appropriate test to use is, again, Pearson’s Correlation Coefficient.
Topic 3: Nursing Home Staff Size and Number of COVID-19 Cases
Statistical research question no. 3.
Note that this study on COVID-19 looked into three variables, namely 1) number of unique employees working in skilled nursing homes, 2) number of weekly confirmed cases among residents and staff, and 3) number of weekly COVID-19 deaths among residents.
A simple Pearson test may be used to correlate one variable with another variable. But the study used multiple variables. Hence, they produced regression models that show how multiple variables affect the outcome. Some of the variables in the study may be redundant, meaning, those variables may represent the same attribute of a population. Stepwise multiple regression models take care of those redundancies. Using this statistical test requires further study and experience.
Topic 4: Surrounding Greenness, Stress, and Memory
Statistical research question no. 4.
As this article is behind a paywall and we cannot see the full article, we can content ourselves with the knowledge that three major variables were explored in this study. These are 1) exposure to and use of natural environments, 2) stress, and 3) memory performance.
Topic 5: Income and Happiness
This recent finding is an interesting read and is available online. Just click on the link I provide as the source below. The study sought to determine if income plays a role in people’s happiness across three age groups: young (18-30 years), middle (31-64 years), and old (65 or older). The literature review suggests that income has a positive effect on an individual’s sense of happiness. That’s because more money increases opportunities to fulfill dreams and buy more goods and services.
An investigation was conducted to determine if the size of nursing home staff and the number of COVID-19 cases are correlated. Specifically, they looked into the number of unique employees working daily, and the outcomes include weekly counts of confirmed COVID-19 cases among residents and staff and weekly COVID-19 deaths among residents.
Statistical Research Question No. 5
I do hope that upon reaching this part of the article, you are now well familiar on how to write statistical research questions. Practice makes perfect.
References:
Måseide, H. (2021). Income and Happiness: Does the relationship vary with age?
© P. A. Regoniel 12 October 2021 | Updated 08 January 2024
Related Posts
What is the difference between theory testing and theory building, heart rate analysis: example of t-test using ms excel analysis toolpak, honing research skills through self-study: 3 key ideas, about the author, patrick regoniel, simplyeducate.me privacy policy.
educational research techniques
Research techniques and education.

Research Questions, Variables, and Statistics
Working with students over the years has led me to the conclusion that often students do not understand the connection between variables, quantitative research questions and the statistical tools
used to answer these questions. In other words, students will take statistics and pass the class. Then they will take research methods, collect data, and have no idea how to analyze the data even though they have the necessary skills in statistics to succeed.
This means that the students have a theoretical understanding of statistics but struggle in the application of it. In this post, we will look at some of the connections between research questions and statistics.
Variables are important because how they are measured affects the type of question you can ask and get answers to. Students often have no clue how they will measure a variable and therefore have no idea how they will answer any research questions they may have.
Another aspect that can make this confusing is that many variables can be measured more than one way. Sometimes the variable “salary” can be measured in a continuous manner or in a categorical manner. The superiority of one or the other depends on the goals of the research.
It is critical to support students to have a thorough understanding of variables in order to support their research.
Types of Research Questions
In general, there are two types of research questions. These two types are descriptive and relational questions. Descriptive questions involve the use of descriptive statistic such as the mean, median, mode, skew, kurtosis, etc. The purpose is to describe the sample quantitatively with numbers (ie the average height is 172cm) rather than relying on qualitative descriptions of it (ie the people are tall).
Below are several example research questions that are descriptive in nature.
- What is the average height of the participants in the study?
- What proportion of the sample is passed the exam?
- What are the respondents perceptions towards the cafeteria?
These questions are not intellectually sophisticated but they are all answerable with descriptive statistical tools. Question 1 can be answered by calculating the mean. Question 2 can be answered by determining how many passed the exam and dividing by the total sample size. Question 3 can be answered by calculating the mean of all the survey items that are used to measure respondents perception of the cafeteria.
Understanding the link between research question and statistical tool is critical. However, many people seem to miss the connection between the type of question and the tools to use.
Relational questions look for the connection or link between variables. Within this type there are two sub-types. Comparison question involve comparing groups. The other sub-type is called relational or an association question.
Comparison questions involve comparing groups on a continuous variable. For example, comparing men and women by height. What you want to know is whether there is a difference in the height of men and women. The comparison here is trying to determine if gender is related to height. Therefore, it is looking for a relationship just not in the way that many student understand. Common comparison questions include the following.male
- Is there a difference in height by gender among the participants?
- Is there a difference in reading scores by grade level?
- Is there a difference in job satisfaction in based on major?
Each of these questions can be answered using ANOVA or if we want to get technical and there are only two groups (ie gender) we can use t-test. This is a broad overview and does not include the complexities of one-sample test and or paired t-test.
Relational or association question involve continuous variables primarily. The goal is to see how variables move together. For example, you may look for the relationship between height and weight of students. Common questions include the following.
- Is there a relationship between height and weight?
- Does height and show size explain weight?
Questions 1 can be answered by calculating the correlation. Question 2 requires the use of linear regression in order to answer the question.
The challenging as a teacher is showing the students the connection between statistics and research questions from the real world. It takes time for students to see how the question inspire the type of statistical tool to use. Understanding this is critical because it helps to frame the possibilities of what to do in research based on the statistical knowledge one has.
Share this:
Leave a reply cancel reply, discover more from educational research techniques.
Subscribe now to keep reading and get access to the full archive.
Type your email…
Continue reading
Want to create or adapt books like this? Learn more about how Pressbooks supports open publishing practices.
Descriptive Research and Case Studies
Learning objectives.
- Explain the importance and uses of descriptive research, especially case studies, in studying abnormal behavior
Types of Research Methods
There are many research methods available to psychologists in their efforts to understand, describe, and explain behavior and the cognitive and biological processes that underlie it. Some methods rely on observational techniques. Other approaches involve interactions between the researcher and the individuals who are being studied—ranging from a series of simple questions; to extensive, in-depth interviews; to well-controlled experiments.
The three main categories of psychological research are descriptive, correlational, and experimental research. Research studies that do not test specific relationships between variables are called descriptive, or qualitative, studies . These studies are used to describe general or specific behaviors and attributes that are observed and measured. In the early stages of research, it might be difficult to form a hypothesis, especially when there is not any existing literature in the area. In these situations designing an experiment would be premature, as the question of interest is not yet clearly defined as a hypothesis. Often a researcher will begin with a non-experimental approach, such as a descriptive study, to gather more information about the topic before designing an experiment or correlational study to address a specific hypothesis. Descriptive research is distinct from correlational research , in which psychologists formally test whether a relationship exists between two or more variables. Experimental research goes a step further beyond descriptive and correlational research and randomly assigns people to different conditions, using hypothesis testing to make inferences about how these conditions affect behavior. It aims to determine if one variable directly impacts and causes another. Correlational and experimental research both typically use hypothesis testing, whereas descriptive research does not.
Each of these research methods has unique strengths and weaknesses, and each method may only be appropriate for certain types of research questions. For example, studies that rely primarily on observation produce incredible amounts of information, but the ability to apply this information to the larger population is somewhat limited because of small sample sizes. Survey research, on the other hand, allows researchers to easily collect data from relatively large samples. While surveys allow results to be generalized to the larger population more easily, the information that can be collected on any given survey is somewhat limited and subject to problems associated with any type of self-reported data. Some researchers conduct archival research by using existing records. While existing records can be a fairly inexpensive way to collect data that can provide insight into a number of research questions, researchers using this approach have no control on how or what kind of data was collected.
Correlational research can find a relationship between two variables, but the only way a researcher can claim that the relationship between the variables is cause and effect is to perform an experiment. In experimental research, which will be discussed later, there is a tremendous amount of control over variables of interest. While performing an experiment is a powerful approach, experiments are often conducted in very artificial settings, which calls into question the validity of experimental findings with regard to how they would apply in real-world settings. In addition, many of the questions that psychologists would like to answer cannot be pursued through experimental research because of ethical concerns.
The three main types of descriptive studies are case studies, naturalistic observation, and surveys.
Clinical or Case Studies
Psychologists can use a detailed description of one person or a small group based on careful observation. Case studies are intensive studies of individuals and have commonly been seen as a fruitful way to come up with hypotheses and generate theories. Case studies add descriptive richness. Case studies are also useful for formulating concepts, which are an important aspect of theory construction. Through fine-grained knowledge and description, case studies can fully specify the causal mechanisms in a way that may be harder in a large study.
Sigmund Freud developed many theories from case studies (Anna O., Little Hans, Wolf Man, Dora, etc.). F or example, he conducted a case study of a man, nicknamed “Rat Man,” in which he claimed that this patient had been cured by psychoanalysis. T he nickname derives from the fact that among the patient’s many compulsions, he had an obsession with nightmarish fantasies about rats.
Today, more commonly, case studies reflect an up-close, in-depth, and detailed examination of an individual’s course of treatment. Case studies typically include a complete history of the subject’s background and response to treatment. From the particular client’s experience in therapy, the therapist’s goal is to provide information that may help other therapists who treat similar clients.
Case studies are generally a single-case design, but can also be a multiple-case design, where replication instead of sampling is the criterion for inclusion. Like other research methodologies within psychology, the case study must produce valid and reliable results in order to be useful for the development of future research. Distinct advantages and disadvantages are associated with the case study in psychology.
A commonly described limit of case studies is that they do not lend themselves to generalizability . The other issue is that the case study is subject to the bias of the researcher in terms of how the case is written, and that cases are chosen because they are consistent with the researcher’s preconceived notions, resulting in biased research. Another common problem in case study research is that of reconciling conflicting interpretations of the same case history.
Despite these limitations, there are advantages to using case studies. One major advantage of the case study in psychology is the potential for the development of novel hypotheses of the cause of abnormal behavior for later testing. Second, the case study can provide detailed descriptions of specific and rare cases and help us study unusual conditions that occur too infrequently to study with large sample sizes. The major disadvantage is that case studies cannot be used to determine causation, as is the case in experimental research, where the factors or variables hypothesized to play a causal role are manipulated or controlled by the researcher.
Link to Learning: Famous Case Studies
Some well-known case studies that related to abnormal psychology include the following:
- Harlow— Phineas Gage
- Breuer & Freud (1895)— Anna O.
- Cleckley’s case studies: on psychopathy ( The Mask of Sanity ) (1941) and multiple personality disorder ( The Three Faces of Eve ) (1957)
- Freud and Little Hans
- Freud and the Rat Man
- John Money and the John/Joan case
- Genie (feral child)
- Piaget’s studies
- Rosenthal’s book on the murder of Kitty Genovese
- Washoe (sign language)
- Patient H.M.
Naturalistic Observation
If you want to understand how behavior occurs, one of the best ways to gain information is to simply observe the behavior in its natural context. However, people might change their behavior in unexpected ways if they know they are being observed. How do researchers obtain accurate information when people tend to hide their natural behavior? As an example, imagine that your professor asks everyone in your class to raise their hand if they always wash their hands after using the restroom. Chances are that almost everyone in the classroom will raise their hand, but do you think hand washing after every trip to the restroom is really that universal?
This is very similar to the phenomenon mentioned earlier in this module: many individuals do not feel comfortable answering a question honestly. But if we are committed to finding out the facts about handwashing, we have other options available to us.
Suppose we send a researcher to a school playground to observe how aggressive or socially anxious children interact with peers. Will our observer blend into the playground environment by wearing a white lab coat, sitting with a clipboard, and staring at the swings? We want our researcher to be inconspicuous and unobtrusively positioned—perhaps pretending to be a school monitor while secretly recording the relevant information. This type of observational study is called naturalistic observation : observing behavior in its natural setting. To better understand peer exclusion, Suzanne Fanger collaborated with colleagues at the University of Texas to observe the behavior of preschool children on a playground. How did the observers remain inconspicuous over the duration of the study? They equipped a few of the children with wireless microphones (which the children quickly forgot about) and observed while taking notes from a distance. Also, the children in that particular preschool (a “laboratory preschool”) were accustomed to having observers on the playground (Fanger, Frankel, & Hazen, 2012).
It is critical that the observer be as unobtrusive and as inconspicuous as possible: when people know they are being watched, they are less likely to behave naturally. For example, psychologists have spent weeks observing the behavior of homeless people on the streets, in train stations, and bus terminals. They try to ensure that their naturalistic observations are unobtrusive, so as to minimize interference with the behavior they observe. Nevertheless, the presence of the observer may distort the behavior that is observed, and this must be taken into consideration (Figure 1).
The greatest benefit of naturalistic observation is the validity, or accuracy, of information collected unobtrusively in a natural setting. Having individuals behave as they normally would in a given situation means that we have a higher degree of ecological validity, or realism, than we might achieve with other research approaches. Therefore, our ability to generalize the findings of the research to real-world situations is enhanced. If done correctly, we need not worry about people modifying their behavior simply because they are being observed. Sometimes, people may assume that reality programs give us a glimpse into authentic human behavior. However, the principle of inconspicuous observation is violated as reality stars are followed by camera crews and are interviewed on camera for personal confessionals. Given that environment, we must doubt how natural and realistic their behaviors are.
The major downside of naturalistic observation is that they are often difficult to set up and control. Although something as simple as observation may seem like it would be a part of all research methods, participant observation is a distinct methodology that involves the researcher embedding themselves into a group in order to study its dynamics. For example, Festinger, Riecken, and Shacter (1956) were very interested in the psychology of a particular cult. However, this cult was very secretive and wouldn’t grant interviews to outside members. So, in order to study these people, Festinger and his colleagues pretended to be cult members, allowing them access to the behavior and psychology of the cult. Despite this example, it should be noted that the people being observed in a participant observation study usually know that the researcher is there to study them. [1]
Another potential problem in observational research is observer bias . Generally, people who act as observers are closely involved in the research project and may unconsciously skew their observations to fit their research goals or expectations. To protect against this type of bias, researchers should have clear criteria established for the types of behaviors recorded and how those behaviors should be classified. In addition, researchers often compare observations of the same event by multiple observers, in order to test inter-rater reliability : a measure of reliability that assesses the consistency of observations by different observers.
Often, psychologists develop surveys as a means of gathering data. Surveys are lists of questions to be answered by research participants, and can be delivered as paper-and-pencil questionnaires, administered electronically, or conducted verbally (Figure 3). Generally, the survey itself can be completed in a short time, and the ease of administering a survey makes it easy to collect data from a large number of people.
Surveys allow researchers to gather data from larger samples than may be afforded by other research methods . A sample is a subset of individuals selected from a population , which is the overall group of individuals that the researchers are interested in. Researchers study the sample and seek to generalize their findings to the population.
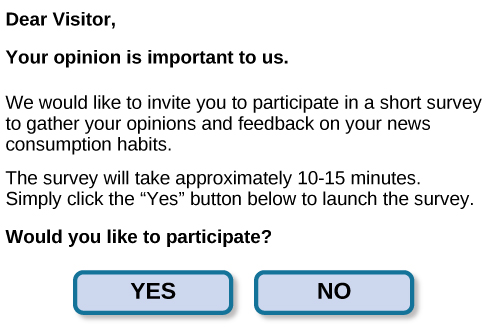
There is both strength and weakness in surveys when compared to case studies. By using surveys, we can collect information from a larger sample of people. A larger sample is better able to reflect the actual diversity of the population, thus allowing better generalizability. Therefore, if our sample is sufficiently large and diverse, we can assume that the data we collect from the survey can be generalized to the larger population with more certainty than the information collected through a case study. However, given the greater number of people involved, we are not able to collect the same depth of information on each person that would be collected in a case study.
Another potential weakness of surveys is something we touched on earlier in this module: people do not always give accurate responses. They may lie, misremember, or answer questions in a way that they think makes them look good. For example, people may report drinking less alcohol than is actually the case.
Any number of research questions can be answered through the use of surveys. One real-world example is the research conducted by Jenkins, Ruppel, Kizer, Yehl, and Griffin (2012) about the backlash against the U.S. Arab-American community following the terrorist attacks of September 11, 2001. Jenkins and colleagues wanted to determine to what extent these negative attitudes toward Arab-Americans still existed nearly a decade after the attacks occurred. In one study, 140 research participants filled out a survey with 10 questions, including questions asking directly about the participant’s overt prejudicial attitudes toward people of various ethnicities. The survey also asked indirect questions about how likely the participant would be to interact with a person of a given ethnicity in a variety of settings (such as, “How likely do you think it is that you would introduce yourself to a person of Arab-American descent?”). The results of the research suggested that participants were unwilling to report prejudicial attitudes toward any ethnic group. However, there were significant differences between their pattern of responses to questions about social interaction with Arab-Americans compared to other ethnic groups: they indicated less willingness for social interaction with Arab-Americans compared to the other ethnic groups. This suggested that the participants harbored subtle forms of prejudice against Arab-Americans, despite their assertions that this was not the case (Jenkins et al., 2012).
Think it Over
Research has shown that parental depressive symptoms are linked to a number of negative child outcomes. A classmate of yours is interested in the associations between parental depressive symptoms and actual child behaviors in everyday life [2] because this associations remains largely unknown. After reading this section, what do you think is the best way to better understand such associations? Which method might result in the most valid data?
clinical or case study: observational research study focusing on one or a few people
correlational research: tests whether a relationship exists between two or more variables
descriptive research: research studies that do not test specific relationships between variables; they are used to describe general or specific behaviors and attributes that are observed and measured
experimental research: tests a hypothesis to determine cause-and-effect relationships
generalizability: inferring that the results for a sample apply to the larger population
inter-rater reliability: measure of agreement among observers on how they record and classify a particular event
naturalistic observation: observation of behavior in its natural setting
observer bias: when observations may be skewed to align with observer expectations
population: overall group of individuals that the researchers are interested in
sample: subset of individuals selected from the larger population
survey: list of questions to be answered by research participants—given as paper-and-pencil questionnaires, administered electronically, or conducted verbally—allowing researchers to collect data from a large number of people
CC Licensed Content, Shared Previously
- Descriptive Research and Case Studies . Authored by : Sonja Ann Miller for Lumen Learning. Provided by : Lumen Learning. License : CC BY-SA: Attribution-ShareAlike
- Approaches to Research. Authored by : OpenStax College. Located at : http://cnx.org/contents/[email protected]:iMyFZJzg@5/Approaches-to-Research . License : CC BY: Attribution . License Terms : Download for free at http://cnx.org/contents/[email protected]
- Descriptive Research. Provided by : Boundless. Located at : https://www.boundless.com/psychology/textbooks/boundless-psychology-textbook/researching-psychology-2/types-of-research-studies-27/descriptive-research-124-12659/ . License : CC BY-SA: Attribution-ShareAlike
- Case Study. Provided by : Wikipedia. Located at : https://en.wikipedia.org/wiki/Case_study . License : CC BY-SA: Attribution-ShareAlike
- Rat man. Provided by : Wikipedia. Located at : https://en.wikipedia.org/wiki/Rat_Man#Legacy . License : CC BY-SA: Attribution-ShareAlike
- Case study in psychology. Provided by : Wikipedia. Located at : https://en.wikipedia.org/wiki/Case_study_in_psychology . License : CC BY-SA: Attribution-ShareAlike
- Research Designs. Authored by : Christie Napa Scollon. Provided by : Singapore Management University. Located at : https://nobaproject.com/modules/research-designs#reference-6 . Project : The Noba Project. License : CC BY-NC-SA: Attribution-NonCommercial-ShareAlike
- Single subject design. Provided by : Wikipedia. Located at : https://en.wikipedia.org/wiki/Single-subject_design . License : CC BY-SA: Attribution-ShareAlike
- Single subject research. Provided by : Wikipedia. Located at : https://en.wikipedia.org/wiki/Single-subject_research#A-B-A-B . License : Public Domain: No Known Copyright
- Pills. Authored by : qimono. Provided by : Pixabay. Located at : https://pixabay.com/illustrations/pill-capsule-medicine-medical-1884775/ . License : CC0: No Rights Reserved
- ABAB Design. Authored by : Doc. Yu. Provided by : Wikimedia. Located at : https://commons.wikimedia.org/wiki/File:A-B-A-B_Design.png . License : CC BY-SA: Attribution-ShareAlike
- Scollon, C. N. (2020). Research designs. In R. Biswas-Diener & E. Diener (Eds), Noba textbook series: Psychology. Champaign, IL: DEF publishers. Retrieved from http://noba.to/acxb2thy ↵
- Slatcher, R. B., & Trentacosta, C. J. (2011). A naturalistic observation study of the links between parental depressive symptoms and preschoolers' behaviors in everyday life. Journal of family psychology : JFP : journal of the Division of Family Psychology of the American Psychological Association (Division 43), 25(3), 444–448. https://doi.org/10.1037/a0023728 ↵
Descriptive Research and Case Studies Copyright © by Meredith Palm is licensed under a Creative Commons Attribution 4.0 International License , except where otherwise noted.
Share This Book
- Open access
- Published: 04 September 2024
Barriers of access to primary healthcare services by National Health Insurance Fund capitated members in Uasin Gishu county, Kenya
- Barbara Nawire Were 1 ,
- Eunice Muthoni Mwangi 2 &
- Lillian Wambui Muiruri 1
BMC Health Services Research volume 24 , Article number: 1025 ( 2024 ) Cite this article
13 Accesses
Metrics details
The study identifies provision of primary healthcare services using the capitated health model as a prerequisite for promoting positive healthcare outcomes for a country’s population. However, capitated members have continued to face challenges in accessing primary healthcare services despite enrolment in the National Health Insurance Fund (NHIF). This study sought to determine if variables such as patient knowledge of the NHIF benefit package, NHIF Premium Payment processes, selecting NHIF capitated health facilities, and NHIF Communication to citizens’ influences access to primary healthcare services.
A cross-sectional analytical research design was adopted. Data was collected from patients who were using NHIF cards, who were drawn from health facilities. Data was collected using a structured questionnaire where some of the questions were rated using the Likert scale to enable the generation of descriptive statistics. Data was analysed using descriptive and inferential statistics. Logistic regression was conducted to determine the relationship between the independent and the dependent variables.
The study found that four independent variables (Patient knowledge of NHIF Benefit Package, NHIF Premium Payment processes, Selecting NHIF capitated Health Facility, and NHIF Communication to citizens) were significant predictors of access to capitated healthcare services with significance values of .001, .001, .001 and .001 respectively at 95% significance level.
Conclusions
The study found that familiarity with the NHIF benefit package significantly influenced NHIF capitated members' access to primary healthcare services in Uasin Gishu County. While most members were aware of their healthcare entitlements, there's a need for increased awareness regarding access to surgical services and dependents' inclusion. Facility selection also played a crucial role, influenced by factors like freedom of choice, NHIF facility selection rules, facility appearance, and proximity to members' homes. NHIF communication positively impacted access, with effective communication channels aiding service accessibility. Premium payment processes also significantly linked with service access, influenced by factors such as payment procedures, premium awareness, payment schedules, registration waiting periods, and penalties for defaults. Overall, patient knowledge, NHIF communication, premium payment processes, and facility selection all contributed positively to NHIF capitated members' access to primary healthcare services in Uasin Gishu County.
Peer Review reports
Introduction
Health financing plays a critical role in the functioning of healthcare systems, encompassing the acquisition, pooling, and allocation of funds to address people’s healthcare needs [ 1 ]. An effective health financing system should be capable of consistently generating sufficient funds to facilitate access to high-quality health services without imposing financial burdens [ 2 , 3 ]. The core functions of health financing comprise revenue collection, risk pooling, and the purchasing of health services [ 4 , 5 ]. Purchasing can take either a passive or strategic approach. Strategic purchasing aims to optimize health system performance, while passive purchasing operates within predetermined budgetary constraints. Strategic purchasing, integral to achieving Universal Health Coverage (UHC), prioritizes both adequate resource mobilization and efficient resource utilization [ 6 , 7 , 8 ]. Provider payment mechanisms within healthcare systems primarily include fee-for-service, salary, and capitation. Strategic employment of capitation holds promise for advancing UHC objectives. Capitation involves providers receiving fixed payments per enrolled individual over a defined period, fostering a shift towards preventive care [ 9 ]. Simplified billing procedures under capitation streamline administrative processes and incentivize preventive healthcare, thus reducing reliance on costly interventions [ 10 , 11 , 12 ].
Globally, the capitation model predominates in tax-funded health systems like those of Italy and the UK, where general practitioners deliver primary care [ 13 , 14 ]. As countries commit to UHC, many are considering integrating capitation with other payment models to enhance healthcare efficiency and performance [ 13 , 15 ]. However, challenges such as inadequate incentive structures and concerns about limiting consumer choice hinder widespread adoption [ 16 , 17 ].
Kenya's National Hospital Insurance Fund (NHIF) predominantly employs capitation to finance primary healthcare services, contracting various organizations to provide outpatient care. Despite the theoretical benefits of capitation in promoting preventive care and reducing hospitalization rates, challenges persist, including inadequate funding and delays in payment [ 18 , 19 , 20 ]. Kenya's efforts to achieve UHC through NHIF expansion face obstacles such as reliance on out-of-pocket payments and inconsistencies in service provision [ 21 , 22 , 23 ].
To address these challenges, this study was conducted in Uasin Gishu County, Kenya, to explore the barriers of access to primary healthcare services by national health insurance fund capitated members in Uasin Gishu county, Kenya. The findings aim to inform decision-makers and stakeholders on measures to enhance access to primary healthcare services within the national scheme.
This cross-sectional analytical research was conducted in Uasin Gishu County, Kenya, chosen due to its large population exceeding 1.3 million in 2019 and a notable inter-censual growth rate of 3.6%, surpassing the national rate of 2.9% [ 24 ]. The county's poverty rate, at 44.6% as of 2006, highlights socio-economic disparities affecting the purchasing power of primary healthcare services. Among its 125 public health facilities, there's one national referral hospital, two district hospitals, 11 sub-district hospitals, 88 dispensaries, and 23 health centres. Utilizing a multistage sampling technique, 90 facilities were selected, targeting over 10,000 capitated members [ 25 ]. Based on Krejcie and Morgan's formula [ 14 ], a sample of 384 respondents was determined, proportionately allocated to the facilities according to NHIF registration. Within each facility, simple random sampling was employed.
Data collection utilized a structured questionnaire, employing a three-point Likert scale, administered in English, covering socio-demographic characteristics, independent variables (NHIF benefit package, premium payment processes, communication to citizens, and healthcare provider selection), and the dependent variable (access to NHIF primary healthcare services) [ 26 ]. Logistic regression analysed the relationship between independent and dependent variables, with Likert scale responses converted into binary variables, where agreement was coded as 1 and disagreement or not sure as 0. This binary coding facilitated the analysis, reflecting patients' access or lack thereof to primary healthcare services.
Two hundred eighty-two out of 384 participants responded, contributing to a 73% response rate, ideal for analysing socio-demographic characteristics such as gender, age, number of children, marital status, education, employment, household income, and NHIF contributions as presented in Table 1 .
The primary age group of respondents was 25 to 34 years, typically productive and focused on personal and organizational growth. They exhibited a high likelihood of seeking healthcare services, with many having young families, leading to increased capitation subscription, contribution, and utilization rates. The majority had partners, had 1–2 children, had acquired tertiary education, had employment, and had a household income adequate for NHIF premiums.
Access to NHIF primary care health services
The study dependent variable was patient’s access to NHIF Primary Care Health Services. Descriptive results are presented in Table 2 [ 27 ].
Over 70% agreement was observed regarding positive staff attitude, feeling safe while with staffs, staff competence and willingness to assist patients, NHIF service availability, and manageable waiting times. However, 226(80%) agreed to paying out-of-pocket for registration, consultation, medications, or laboratory services despite having prepaid for the services.
Logistic regression
The independent variable in this study were (NHIF Benefit Package, premium payment processes, communication to citizens, and healthcare provider selection) and the dependent variable was access to NHIF primary healthcare services. Logistic regression was undertaken of the variables to determine the barriers of access to primary healthcare services by national health insurance fund capitated members in Uasin Gishu county, Kenya.
The model used in this study was as follows:
where Z is a linear combination of the covariates expressed as:
The model employed in this study was formulated as follows:
where Z represents a linear combination of the covariates, with X1, X2, X3, and X4, being the independent variables (NHIF Benefit Package, premium payment processes, communication to citizens, and healthcare provider selection). The intercept is represented by β0, whereas β1, β2, β3 and β4 denote the estimates of the increase in log odds of the dependent variable (access to NHIF primary care health services) for each unit increase in the respective independent variables. An odds ratio of 1 indicates that the independent variable has no effect on the dependent variable. An odds ratio greater than one suggests a greater risk association, while ratio less than one indicates a reduced risk or the ability of the independent variable to mitigate the risk of access to NHIF primary care health services [ 28 ].
A logistic regression analysis was conducted to examine the impact of patients’ knowledge of the NHIF benefit package, premium payment process, facility selection, and communication from NHIF on their access to services. The results are presented in Table 3 [ 29 ]
The results reveal that patients who knew the NHIF benefit package were 9.274 times more likely to receive the healthcare services compared to those unaware of the benefit package. In addition, patients who knew about the NHIF premium payment process were 5.339 times more likely to visit the NHIF recommended health facilities compared to those unaware of the process. The NHIF members who knew how to select a health facility were 6.101 times willing to access NHIF services compared to their counterparts who had no knowledge of health care facility selection. Members who receive communication were also more likely to access primary healthcare services.
The predominant age group among respondents was between 25 and 34 years, representing individuals at their peak productivity and deeply invested in personal and professional growth. This cohort exhibited the highest likelihood of seeking healthcare services, leading to increased rates of capitation subscription, contribution, and utilization [ 30 ]. Married couples showed a higher rate of insurance coverage, attributed to their responsibilities towards dependents and a comparatively higher household income, facilitating premium payments [ 31 ]. Moreover, a majority of respondents had attained at least minimum academic and professional qualifications, enhancing their understanding of NHIF procedures and terms. They also boasted household incomes adequate for monthly NHIF contributions [ 32 ].
However, patient awareness of NHIF primary health service benefits remained limited, with approximately 25% expressing disagreement or uncertainty regarding their entitlements [ 23 , 33 ]. Understanding of NHIF benefits significantly influenced access to capitated health services positively [ 34 ]. While NHIF continuously reviews its benefit package, many members only become aware of the outpatient services when they necessitate them, possibly due to the on-demand nature of outpatient care utilization [ 35 , 36 ].
Effective communication from NHIF positively impacted access to primary care health services under capitation. Despite positive feedback regarding NHIF's provision of necessary information, a significant portion of respondents expressed dissatisfaction with NHIF's responsiveness to public complaints and its clarity regarding service packages. NHIF primarily communicates through its website and media advertisements, but the limited reach of these channels potentially hinders members' awareness of their entitlements, affecting healthcare access and potentially leading to under or over-provision of services [ 18 , 23 , 37 , 38 , 39 ]. Equity and efficiency in healthcare provision can be achieved by empowering the service providers and the members with the information by adopting effective communication channels [ 40 , 41 ]. The findings agree with other studies which show that some of the communication media used by the NHIF included television, radio, newspaper, social media, mobile phones, billboards, and sensitization campaigns [ 33 , 42 ]. They agree with the findings of another study where reportedly 57% of the respondents are provided information by NHIF while 43% are not receiving any communication from NHIF regarding the health services covered [ 23 , 33 , 43 ]. In addition, the results also highlight that no legislation provides for feedback or complaints mechanism from members or beneficiaries [ 18 , 23 , 44 ].
Approximately 20% of respondents were unaware of the premium payment process, indicating a lack of clarity regarding NHIF contributions [ 45 , 46 , 47 , 48 ]. The perception of NHIF-accredited health facilities significantly influenced service utilization, with patients associating the facilities' image with service quality [ 35 , 36 , 49 ]. However, the accreditation status varied among clinics and higher-level facilities, potentially impacting service uptake [ 50 , 51 ]. Additionally, individuals diagnosed with chronic illnesses exhibited a higher likelihood of selecting healthcare providers and utilizing services, with private providers restricted to specific service categories compared to government hospitals [ 52 , 53 ].
Limitations
Using structured questions to collect self-reported data. Participants may provide inaccurate or biased responses due to social desirability bias or recall bias. Additionally, respondents' comprehension of the questions or their willingness to disclose certain information could vary, leading to inconsistencies in the data collected. This could affect the validity and reliability of the study's findings.
The findings of the research may have limited generalizability beyond the specific context of Uasin Gishu County. Factors influencing access to primary healthcare services can vary significantly depending on geographical location, cultural norms, healthcare infrastructure, and other contextual factors. Therefore, the determinants identified in this study may not be applicable to capitated members in other regions of Kenya or in different counties; limiting the broader applicability of the research findings and the study also targeted NHIF accredited public health facilities in Uasin Gishu County, Kenya. Privately owned hospitals were excluded. Hence the study findings can be generalized to public facilities.
Out of pocket payment despite prepayment remains a key barrier of access to primary healthcare services. NHIF capitated members are generally aware of their healthcare entitlement. However, efforts are needed to enhance more awareness regarding entitlement to surgical services, inclusion of dependents, access to information about the benefit package, feedback and complaint mechanisms, premium payment process, awareness of the premium to be paid, payment schedule, the waiting period before registration and accessing services, and penalties in the event of default. This information could be used to advocate for the implementation of effective communication systems that allow for real-time dissemination of information and feedback, as well as to conduct regular in-service training and recruiting a well-educated workforce that is familiar with NHIF procedures and terms in order to promote the NHIF in the face of capitated members' social-demographic profiles, and to increase knowledge of the health coverage plan for the informal sector and flexible payment platforms. Utilizing vernacular to reach more communities, particularly in rural areas could broaden outreach.
Availability of data and materials
The datasets used and/or analysed during the study are available from the corresponding author on reasonable request.
World Health Organization. The world health report: health systems financing: the path to universal coverage: executive summary. World Health Organization; 2010.
Liaropoulos L, Goranitis I. Health care financing and the sustainability of health systems. Int J Equity Health. 2015;14(1):1–4.
Lagomarsino G, Garabrant A, Adyas A, Muga R, Otoo N. Moving towards universal health coverage: health insurance reforms in nine developing countries in Africa and Asia. The Lancet. 2012;380(9845):933–43.
Article Google Scholar
World Health Organization. An empirical model of access to health care, health care expenditure and impoverishment in Kenya: learning from past reforms and lessons for the future. World Health Organization; 2006.
Abiiro GA, Alatinga KA, Yamey G. Why did Ghana’s national health insurance capitation payment model fall off the policy agenda? A regional level policy analysis. Health Policy Plan. 2021;36(6):869–80.
Article PubMed PubMed Central Google Scholar
Honda A. What is strategic purchasing for health? World Health Organization; 2014.
Alawode GO, Adewole DA. Assessment of the design and implementation challenges of the National Health Insurance Scheme in Nigeria: a qualitative study among sub-national level actors, healthcare and insurance providers. BMC Public Health. 2021;21(1):1–2.
Nyandekwe M, Nzayirambaho M, Kakoma JB. Universal health insurance in Rwanda: major challenges and solutions for financial sustainability case study of Rwanda community-based health insurance part I. Pan Afr Med J. 2020;37(1).
Langenbrunner J, Cashin C, O’Dougherty S, editors. Designing and implementing health care provider payment systems: how-to manuals. World Bank Publications; 2009.
James BC. The Case for Capitation. Harvard Business Review. 2016. Retrieved from https://hbr.org/2016/07/the-case-for-capitation .
Busby M, Chapple L, Clover H, McCreanor J, Chapple I. Capitation care fee banding: aspects of reliability and validity of an online tool. Br Dent J. 2018;225(8):751–5.
Obadha M, Chuma J, Whongu J. Preferences of healthcare providers for capitation payment in Kenya: a discrete choice experiment. Health Policy Plan. 2020;35(7):842–54.
Park M, Braun T, Carrin G, Evans DB, World Health Organization. Provider payments and cost-containment: lessons from OECD countries. World Health Organization; 2007.
Krejcie RV, Morgan DW. Determining sample size for research activities. Educ Psychol Measur. 1970;30(3):607–10.
Feldhaus I, Mathauer I. Effects of mixed provider payment systems and aligned cost sharing practices on expenditure growth management, efficiency, and equity: a structured review of the literature. BMC Health Serv Res. 2018;18:1–4.
Koenecke A. A game theoretic setting of capitation versus fee-for-service payment systems. PLoS ONE. 2019;14(10): e0223672.
Article CAS PubMed PubMed Central Google Scholar
Sachs JD. Achieving universal health coverage in low-income settings. The Lancet. 2012;380(9845):944–7.
Munge K, Mulupi S, Barasa EW, Chuma J. A critical analysis of purchasing arrangements in Kenya: the case of the National Hospital Insurance Fund. Int J Health Policy Manag. 2018;7(3):244.
Article PubMed Google Scholar
Obadha M, Chuma J, Kazungu J, Barasa E. Health care purchasing in Kenya: Experiences of health care providers with capitation and fee-for-service provider payment mechanisms. Int J Health Plann Manage. 2019;34(1):e917–33.
Xu W, Cai GJ, Li GN, Cao JJ, Shi QH, Bai J. Age or health status: which influences medical insurance enrollment greater? J Global Health. 2016;6(2).
Barasa EW, Maina T, Ravishankar N. Assessing the impoverishing effects, and factors associated with the incidence of catastrophic health care payments in Kenya. Int J Equity Health. 2017;16(1):1–4.
Barasa E, Nguhiu P, McIntyre D. Measuring progress towards sustainable development goal 3.8 on universal health coverage in Kenya. BMJ Global Health. 2018;3(3):e000904.
Mwangi EM, Tenambergen W, Mapesa J, Mutai I. Citizen engagement in social health insurance purchasing, in selected counties in Kenya. Int J Community Med Public Heal. 2019;6(10):4145–53.
KNBS K. Kenya Population and Housing Census Volume I: Population by County and Sub-County. Vol. I. 2019;2019.
Salari P, Di Giorgio L, Ilinca S, Chuma J. The catastrophic and impoverishing effects of out-of-pocket healthcare payments in Kenya, 2018. BMJ Glob Health. 2019;4(6):e001809.
World Health Organization. (2008). Health System Financing. Retrieved from https://www.who.int/healthinfo/statistics/toolkit_hss/EN_PDF_Toolkit_HSS_Financing.pdf .
Explanation of the benefit package for the National Scheme. 2015. Retrieved from http://publications.universalhealth2030.org/uploads/nhif_benefits_package.pdf .
Mulupi S, Kirigia D, Chuma J. Community perceptions of health insurance and their preferred design features: implications for the design of universal health coverage reforms in Kenya. BMC Health Serv Res. 2013;13(1):1–2.
Ongiri IS, Kubani JA. Unions demand talks on NHIF rates. Daily Nation. 2015:17.
Orangi S, Kairu A, Malla L, Ondera J, Mbuthia B, Ravishankar N, Barasa E. Impact of free maternity policies in Kenya: an interrupted time-series analysis. BMJ Glob Health. 2021;6(6):e003649. https://doi.org/10.1136/bmjgh-2021-003649 .
Nyman JA. The theory of demand for health insurance. Stanford University Press; 2003.
Jowett M. Theoretical insights into the development of health insurance in low-income countries. 2004.
Google Scholar
Chuma J, Maina T. Catastrophic health care spending and impoverishment in Kenya. BMC Health Serv Res. 2012;12(1):1–9. https://doi.org/10.1186/1472-6963-12-413 .
Ilinca S, Di Giorgio L, Salari P, Chuma J. Socio-economic inequality and inequity in use of health care services in Kenya: evidence from the fourth Kenya household health expenditure and utilization survey. Int J Equity Health. 2019;18:1–3. https://doi.org/10.1186/s12939-019-1080-2 .
Mwaura GW, Kamano JH. Effects of Perceived Image of NHIF Outpatient Facilities on Utilization of Primary Care Services by Private University Employees in Nairobi County. n.d.
Njagi P, Arsenijevic J, Groot W. Decomposition of changes in socioeconomic inequalities in catastrophic health expenditure in Kenya. PLoS ONE. 2020;15(12):e0244428.
Pauly MV. The economics of moral hazard: comment. Am Econ Rev. 1968;58(3):531–7.
Suchman L. Accrediting private providers with National Health Insurance to better serve low-income populations in Kenya and Ghana: a qualitative study. Int J Equity Health. 2018;17:1–8. https://doi.org/10.1186/s12939-018-0900-8 .
Einav L, Finkelstein A. Moral hazard in health insurance: what we know and how we know it. J Eur Econ Assoc. 2018;16(4):957–82. https://doi.org/10.1093/jeea/jvx050 .
Carrin G, James C. Key Performance Indicators for the Implementation of Social Health Insurance. Appl Health Econ Health Policy. 2005;4(1):15–22. https://doi.org/10.2165/00148365-200504010-00004 .
Ndungu TT. Factors influencing uptake of national health insurance in the informal sector: a case of ithanga division in murang’a county. Kenya: University of Nairobi; 2015.
Mbau R, Kabia E, Honda A, Hanson K, Barasa E. Examining purchasing reforms towards universal health coverage by the National Hospital Insurance Fund in Kenya. International journal for equity in health. 2020;19:1–8.
World Health Organization. Monitoring the building blocks of health systems: a handbook of indicators and their measurement strategies. World Health Organization; 2010.
Morris S, Devlin N, Parkin D, Spencer A. Health insurance and healthcare financing. Economic Analysis in Health Care. Chichester: John Wiley and Sons Ltd; 2007. p. 149–82.
Namuhisa JA. Determinants of uptake of National Hospital Insurance Fund scheme by the informal sector in Nairobi county. Kenya: Unpubl MBA Proj Kenyatta Univ Kenya; 2014.
Ochieng DL. Factors influencing uptake of national hospital insurance fund cover by rural households in Kasipul division, Rachuonyo south sub county, Homa Bay County (Doctoral dissertation, University of Nairobi). n.d.
Mugenda OM, Mugenda AG. Research methods: Quantitative & qualitative approaches. Nairobi: Acts press; 2003.
Kituku AM, Amata E, Wachira M. Determinants of the uptake of NHIF medical cover by informal sector workers: A case of UNAITAS SACCO members in Muranga County. 2016.
Otieno PO, Wambiya EO, Mohamed SM, Mutua MK, Kibe PM, Mwangi B, Donfouet HP. Access to primary healthcare services and associated factors in urban slums in Nairobi-Kenya. BMC Public Health. n.d.;20:1–9. https://doi.org/10.1186/s12889-020-09379-2 .
Sieverding M, Onyango C, Suchman L. Private healthcare provider experiences with social health insurance schemes: findings from a qualitative study in Ghana and Kenya. PLoS ONE. 2018;13(2):e0192973. https://doi.org/10.1371/journal.pone.0192973 .
Clark T, Foster L, Bryman A, Sloan L. Bryman's social research methods. Oxford University Press; 2021.
Mwaura GW, Kamano JH, Mwangi AW, Lusimbo L, Mugo R, Too K, Andale T, Itsura P, Kibachio J, Gathecha G, Aruasa W. Awareness, Uptake and factors associated with NHIF uptake in Western Kenya: A case of 4 counties-Busia, Trans Nzoia, Vihiga and Siaya. n.d.
Rono GJ. Out-Of-Pocket Payment for Healthcare and Its Effects on Household Welfare in Rural and Urban Areas of Kenya [Internet]. 2017. Available from: https://ir-library.ku.ac.ke/handle/123456789/17969 .
Download references
Acknowledgements
I thank the county government of Uasin Gishu-county directors, the healthcare facility managers, service providers and their clients for their support in the study. I am also gratefully to my supervisors Dr. Eunice Muthoni Mwangi and Ms. Lillian Wambui Muiruri for their guidance through the process from inception to ensuring the objective of this study is achieved.
The author received no financial support for the research, authorship and /or publication of this manuscript.
Author information
Authors and affiliations.
Department of Health Systems Management, Kenya Methodist University-Nairobi, Nairobi, Kenya
Barbara Nawire Were & Lillian Wambui Muiruri
Department of Population Health - Medical College, Aga Khan University-Nairobi, Nairobi, Kenya
Eunice Muthoni Mwangi
You can also search for this author in PubMed Google Scholar
Contributions
The author confirms sole responsibility for the following: study conception and design, data collection, analysis and interpretation of results and manuscript preparation.
Corresponding author
Correspondence to Barbara Nawire Were .
Ethics declarations
Ethics approval and consent to participate.
Ethical clearance was obtained from Kenya Methodist University Scientific and Ethics Review Committee (KeMU/SERC/HSM/22/2022), the National Commission of Science and Technology and Innovation (NACOSTI/P/22/19153), and the County Director of Health. Participants provided informed consent, assured of confidentiality and anonymity. They were informed of the voluntary nature of participation and their right to withdraw at any time.
Consent for publication
Not applicable.
Competing interests
The authors declare no competing interests.
Additional information
Publisher’s note.
Springer Nature remains neutral with regard to jurisdictional claims in published maps and institutional affiliations.
Supplementary Information
Supplementary material 1., supplementary material 2., rights and permissions.
Open Access This article is licensed under a Creative Commons Attribution 4.0 International License, which permits use, sharing, adaptation, distribution and reproduction in any medium or format, as long as you give appropriate credit to the original author(s) and the source, provide a link to the Creative Commons licence, and indicate if changes were made. The images or other third party material in this article are included in the article's Creative Commons licence, unless indicated otherwise in a credit line to the material. If material is not included in the article's Creative Commons licence and your intended use is not permitted by statutory regulation or exceeds the permitted use, you will need to obtain permission directly from the copyright holder. To view a copy of this licence, visit http://creativecommons.org/licenses/by/4.0/ . The Creative Commons Public Domain Dedication waiver ( http://creativecommons.org/publicdomain/zero/1.0/ ) applies to the data made available in this article, unless otherwise stated in a credit line to the data.
Reprints and permissions
About this article
Cite this article.
Were, B.N., Mwangi, E.M. & Muiruri, L.W. Barriers of access to primary healthcare services by National Health Insurance Fund capitated members in Uasin Gishu county, Kenya. BMC Health Serv Res 24 , 1025 (2024). https://doi.org/10.1186/s12913-024-11282-8
Download citation
Received : 11 February 2024
Accepted : 04 July 2024
Published : 04 September 2024
DOI : https://doi.org/10.1186/s12913-024-11282-8
Share this article
Anyone you share the following link with will be able to read this content:
Sorry, a shareable link is not currently available for this article.
Provided by the Springer Nature SharedIt content-sharing initiative
- National Hospital Insurance Fund
- Universal Health Coverage
BMC Health Services Research
ISSN: 1472-6963
- General enquiries: [email protected]

COMMENTS
Descriptive research questions are a systematic methodology that helps in understanding the what, where, when and how. Important variables can be rigidly defined using descriptive research, unlike qualitative research where the subjectivity in responses makes it relatively difficult to get a grasp on the overall picture.
Descriptive statistics summarize and organize characteristics of a data set. A data set is a collection of responses or observations from a sample or entire population.
This chapter discusses and illustrates descriptive statistics. The purpose of the procedures and fundamental concepts reviewed in this chapter is quite straightforward: to facilitate the description and summarisation of data. By 'describe' ...
At the simplest level, descriptive statistics summarise and describe relatively basic but essential features of a quantitative dataset - for example, a set of survey responses. They provide a snapshot of the characteristics of your dataset and allow you to better understand, roughly, how the data are "shaped" (more on this later).
It's also important to note that descriptive statistics can employ and use both quantitative and qualitative research. Describing data is undoubtedly the most critical first step in research as it enables the subsequent organization, simplification and summarization of information — and every survey question and population has summary ...
Descriptive statistics summarise and organise characteristics of a data set. A data set is a collection of responses or observations from a sample or
Effective presentation of study results, in presentation or manuscript form, typically starts with frequencies and descriptive statistics (ie, mean, medians, standard deviations). One can get a better sense of the variables by examining these data to determine whether a balanced and sufficient research design exists.
This handout explains how to write with statistics including quick tips, writing descriptive statistics, writing inferential statistics, and using visuals with statistics.
Data analysis is dependent on the type of data collected and on utilizing an appropriate statistical analysis to answer research questions and to satisfy the purpose, objectives, and goals of the study. As we proceed, pertinent statistical analyses will be discussed to answer your research questions. Statistics discussed range from basic (e.g., frequency, average) to complex (e.g., canonical ...
Descriptive research aims to accurately and systematically describe a population, situation or phenomenon. It can answer what, where, when and how questions, but not why questions. A descriptive research design can use a wide variety of research methods to investigate one or more variables. Unlike in experimental research, the researcher does ...
Descriptive statistics are specific methods basically used to calculate, describe, and summarize collected research data in a logical, meaningful, and efficient way. Descriptive statistics are reported numerically in the manuscript text and/or in its tables, or graphically in its figures. This basic …
Descriptive Statistics Descriptive statistics is a branch of statistics that deals with the summarization and description of collected data. This type of statistics is used to simplify and present data in a manner that is easy to understand, often through visual or numerical methods. Descriptive statistics is primarily concerned with measures of central tendency, variability, and distribution ...
Descriptive statistics are used to describe the basic features of the data in a study. They provide simple summaries about the sample and the measures. Together with simple graphics analysis, they form the basis of virtually every quantitative analysis of data. Descriptive statistics are typically distinguished from inferential statistics.
Descriptive statistics is the study of numerical and graphical ways of describing and displaying data. Here are some important concepts.
When to use descriptive Statistics to answer RQs. Descriptive statistics are the appropriate analyses when the goal of the research is to present the participants' responses to survey items in order to address the research questions. There are no hypotheses in descriptive statistics. Descriptive statistics include: frequencies and percentages ...
This handout explains how to write with statistics including quick tips, writing descriptive statistics, writing inferential statistics, and using visuals with statistics.
Numerical descriptors consist of summary statistics (typically calculated from a sample) that represent important aspects such as the central tendency and variability of a distribution or the relative standing of a single observation with regard to the rest of the distribution. Graphical descriptive methods consist of chart, tables, and graphs.
Descriptive Statistics - Science topic Explore the latest questions and answers in Descriptive Statistics, and find Descriptive Statistics experts.
Descriptive and Inferential Statistics Read these sections and complete the questions at the end of each section. Here, we introduce descriptive statistics using examples and discuss the difference between descriptive and inferential statistics. We also talk about samples and populations, explain how you can identify biased samples, and define differential statistics.
While descriptive statistics can only summarize a sample's characteristics, inferential statistics use your sample to make reasonable guesses about the larger population. With inferential statistics, it's important to use random and unbiased sampling methods.
Descriptive statistics help describe and explain the features of a specific data set by giving short summaries about the sample and measures of the data. The most recognized types of descriptive ...
Descriptive Statistics Definition. Descriptive statistics is a type of statistical analysis that uses quantitative methods to summarize the features of a population sample. It is useful to present easy and exact summaries of the sample and observations using metrics such as mean, median, variance, graphs, and charts.
Introduction How are statistical research questions for quantitative analysis written? This article provides five examples of statistical research questions that will allow statistical analysis to take place. In quantitative research projects, writing statistical research questions requires a good understanding and the ability to discern the type of data that you will analyze. This knowledge ...
Descriptive questions involve the use of descriptive statistic such as the mean, median, mode, skew, kurtosis, etc. The purpose is to describe the sample quantitatively with numbers (ie the average height is 172cm) rather than relying on qualitative descriptions of it (ie the people are tall).
Surveys. Often, psychologists develop surveys as a means of gathering data. Surveys are lists of questions to be answered by research participants, and can be delivered as paper-and-pencil questionnaires, administered electronically, or conducted verbally (Figure 3). Generally, the survey itself can be completed in a short time, and the ease of administering a survey makes it easy to collect ...
A cross-sectional analytical research design was adopted. Data was collected from patients who were using NHIF cards, who were drawn from health facilities. Data was collected using a structured questionnaire where some of the questions were rated using the Likert scale to enable the generation of descriptive statistics.