JAMA Guide to Statistics and Methods
Explore this JAMA essay series that explains the basics of statistical techniques used in clinical research, to help clinicians interpret and critically appraise the medical literature.

Publication
Article type.
This JAMA Guide to Statistics and Methods article explains the test-negative study design, an observational study design routinely used to estimate vaccine effectiveness, and examines its use in a study that estimated the performance of messenger RNA boosters against the Omicron variant.
This JAMA Guide to Statistics and Methods article discusses accounting for competing risks in clinical research.
This JAMA Guide to Statistics and Methods article explains effect score analyses, an approach for evaluating the heterogeneity of treatment effects, and examines its use in a study of oxygen-saturation targets in critically ill patients.
This JAMA Guide to Statistics and Methods explains the use of historical controls—persons who had received a specific control treatment in a previous study—when randomizing participants to that control treatment in a subsequent trial may not be practical or ethical.
This JAMA Guide to Statistics and Methods discusses the early stopping of clinical trials for futility due to lack of evidence supporting the desired benefit, evidence of harm, or practical issues that make successful completion unlikely.
This JAMA Guide to Statistics and Methods explains sequential, multiple assignment, randomized trial (SMART) study designs, in which some or all participants are randomized at 2 or more decision points depending on the participant’s response to prior treatment.
This JAMA Guide to Statistics and Methods article examines conditional power, calculated while a trial is ongoing and based on both the currently observed data and an assumed treatment effect for future patients.
This Guide to Statistics and Methods describes the use of target trial emulation to design an observational study so it preserves the advantages of a randomized clinical trial, points out the limitations of the method, and provides an example of its use.
This Guide to Statistics and Methods provides an overview of the use of adjustment for baseline characteristics in the analysis of randomized clinical trials and emphasizes several important considerations.
This Guide to Statistics and Methods provides an overview of regression models for ordinal outcomes, including an explanation of why they are used and their limitations.
This Guide to Statistics and Methods provides an overview of patient-reported outcome measures for clinical research, emphasizes several important considerations when using them, and points out their limitations.
This JAMA Guide to Statistics and Methods discusses instrumental variable analysis, a method designed to reduce or eliminate unobserved confounding in observational studies, with the goal of achieving unbiased estimation of treatment effects.
This JAMA Guide to Statistics and Methods describes collider bias, illustrates examples in directed acyclic graphs, and explains how it can threaten the internal validity of a study and the accurate estimation of causal relationships in randomized clinical trials and observational studies.
This JAMA Guide to Statistics and Methods discusses the CONSERVE guidelines, which address how to report extenuating circumstances that lead to a modification in trial design, conduct, or analysis.
This JAMA Guide to Statistics and Methods discusses the basics of causal directed acyclic graphs, which are useful tools for communicating researchers’ understanding of the potential interplay among variables and are commonly used for mediation analysis.
This JAMA Guide to Statistics and Methods discusses cardinality matching, a method for finding the largest possible number of matched pairs in an observational data set, with the goal of balanced and representative samples of study participants between groups.
This Guide to Statistics and Methods discusses the various approaches to estimating variability in treatment effects, including heterogeneity of treatment effect, which was used to assess the association between surgery to close patent foramen ovale and risk of recurrent stroke in patients who presented with a stroke in a related JAMA article.
This Guide to Statistics and Methods describes how confidence intervals can be used to help in the interpretation of nonsignificant findings across all study designs.
This JAMA Guide to Statistics and Methods describes why interim analyses are performed during group sequential trials, provides examples of the limitations of interim analyses, and provides guidance on interpreting the results of interim analyses performed during group sequential trials.
This JAMA Guide to Statistics and Methods describes how ACC/AHA guidelines are formatted to rate class (denoting strength of a recommendation) and level (indicating the level of evidence on which a recommendation is based) and summarizes the strengths and benefits of this rating system in comparison with other commonly used ones.
Select Your Interests
Customize your JAMA Network experience by selecting one or more topics from the list below.
- Academic Medicine
- Acid Base, Electrolytes, Fluids
- Allergy and Clinical Immunology
- American Indian or Alaska Natives
- Anesthesiology
- Anticoagulation
- Art and Images in Psychiatry
- Artificial Intelligence
- Assisted Reproduction
- Bleeding and Transfusion
- Caring for the Critically Ill Patient
- Challenges in Clinical Electrocardiography
- Climate and Health
- Climate Change
- Clinical Challenge
- Clinical Decision Support
- Clinical Implications of Basic Neuroscience
- Clinical Pharmacy and Pharmacology
- Complementary and Alternative Medicine
- Consensus Statements
- Coronavirus (COVID-19)
- Critical Care Medicine
- Cultural Competency
- Dental Medicine
- Dermatology
- Diabetes and Endocrinology
- Diagnostic Test Interpretation
- Drug Development
- Electronic Health Records
- Emergency Medicine
- End of Life, Hospice, Palliative Care
- Environmental Health
- Equity, Diversity, and Inclusion
- Facial Plastic Surgery
- Gastroenterology and Hepatology
- Genetics and Genomics
- Genomics and Precision Health
- Global Health
- Guide to Statistics and Methods
- Hair Disorders
- Health Care Delivery Models
- Health Care Economics, Insurance, Payment
- Health Care Quality
- Health Care Reform
- Health Care Safety
- Health Care Workforce
- Health Disparities
- Health Inequities
- Health Policy
- Health Systems Science
- History of Medicine
- Hypertension
- Images in Neurology
- Implementation Science
- Infectious Diseases
- Innovations in Health Care Delivery
- JAMA Infographic
- Law and Medicine
- Leading Change
- Less is More
- LGBTQIA Medicine
- Lifestyle Behaviors
- Medical Coding
- Medical Devices and Equipment
- Medical Education
- Medical Education and Training
- Medical Journals and Publishing
- Mobile Health and Telemedicine
- Narrative Medicine
- Neuroscience and Psychiatry
- Notable Notes
- Nutrition, Obesity, Exercise
- Obstetrics and Gynecology
- Occupational Health
- Ophthalmology
- Orthopedics
- Otolaryngology
- Pain Medicine
- Palliative Care
- Pathology and Laboratory Medicine
- Patient Care
- Patient Information
- Performance Improvement
- Performance Measures
- Perioperative Care and Consultation
- Pharmacoeconomics
- Pharmacoepidemiology
- Pharmacogenetics
- Pharmacy and Clinical Pharmacology
- Physical Medicine and Rehabilitation
- Physical Therapy
- Physician Leadership
- Population Health
- Primary Care
- Professional Well-being
- Professionalism
- Psychiatry and Behavioral Health
- Public Health
- Pulmonary Medicine
- Regulatory Agencies
- Reproductive Health
- Research, Methods, Statistics
- Resuscitation
- Rheumatology
- Risk Management
- Scientific Discovery and the Future of Medicine
- Shared Decision Making and Communication
- Sleep Medicine
- Sports Medicine
- Stem Cell Transplantation
- Substance Use and Addiction Medicine
- Surgical Innovation
- Surgical Pearls
- Teachable Moment
- Technology and Finance
- The Art of JAMA
- The Arts and Medicine
- The Rational Clinical Examination
- Tobacco and e-Cigarettes
- Translational Medicine
- Trauma and Injury
- Treatment Adherence
- Ultrasonography
- Users' Guide to the Medical Literature
- Vaccination
- Venous Thromboembolism
- Veterans Health
- Women's Health
- Workflow and Process
- Wound Care, Infection, Healing
- Register for email alerts with links to free full-text articles
- Access PDFs of free articles
- Manage your interests
- Save searches and receive search alerts
Thank you for visiting nature.com. You are using a browser version with limited support for CSS. To obtain the best experience, we recommend you use a more up to date browser (or turn off compatibility mode in Internet Explorer). In the meantime, to ensure continued support, we are displaying the site without styles and JavaScript.
- View all journals
- Explore content
- About the journal
- Publish with us
- Sign up for alerts
Statistics articles within Scientific Reports
Article 26 August 2024 | Open Access
Quantification of the time-varying epidemic growth rate and of the delays between symptom onset and presenting to healthcare for the mpox epidemic in the UK in 2022
- Robert Hinch
- , Jasmina Panovska-Griffiths
- & Christophe Fraser
Investigating the causal relationship between wealth index and ICT skills: a mediation analysis approach
- Tarikul Islam
- & Nabil Ahmed Uthso
Article 24 August 2024 | Open Access
Statistical analysis of the effect of socio-political factors on individual life satisfaction
- , Isra Hasan
- & Ayman Alzaatreh
Article 23 August 2024 | Open Access
Improving the explainability of autoencoder factors for commodities through forecast-based Shapley values
- Roy Cerqueti
- , Antonio Iovanella
- & Saverio Storani
Article 20 August 2024 | Open Access
Defect detection of printed circuit board assembly based on YOLOv5
- Minghui Shen
- , Yujie Liu
- & Ye Jiang
Breaking the silence: leveraging social interaction data to identify high-risk suicide users online using network analysis and machine learning
- Damien Lekkas
- & Nicholas C. Jacobson
Stochastic image spectroscopy: a discriminative generative approach to hyperspectral image modelling and classification
- Alvaro F. Egaña
- , Alejandro Ehrenfeld
- & Jorge F. Silva
Article 15 August 2024 | Open Access
Data-driven risk analysis of nonlinear factor interactions in road safety using Bayesian networks
- Cinzia Carrodano
Article 13 August 2024 | Open Access
Momentum prediction models of tennis match based on CatBoost regression and random forest algorithms
- Xingchen Lv
- , Dingyu Gu
- & Yanfang li
Article 12 August 2024 | Open Access
Numerical and machine learning modeling of GFRP confined concrete-steel hollow elliptical columns
- Haytham F. Isleem
- , Tang Qiong
- & Ali Jahami
Experimental investigation of the distribution patterns of micro-scratches in abrasive waterjet cutting surface
- & Quan Wen
Article 07 August 2024 | Open Access
PMANet : a time series forecasting model for Chinese stock price prediction
- , Weisi Dai
- & Yunjing Zhao
Article 06 August 2024 | Open Access
Grasshopper platform-assisted design optimization of fujian rural earthen buildings considering low-carbon emissions reduction
- & Yang Ding
Article 03 August 2024 | Open Access
Effects of dietary fish to rapeseed oil ratio on steatosis symptoms in Atlantic salmon ( Salmo salar L) of different sizes
- D. Siciliani
- & Å. Krogdahl
A model-free and distribution-free multi-omics integration approach for detecting novel lung adenocarcinoma genes
- Shaofei Zhao
- & Guifang Fu
Article 01 August 2024 | Open Access
Intrinsic dimension as a multi-scale summary statistics in network modeling
- Iuri Macocco
- , Antonietta Mira
- & Alessandro Laio
A new possibilistic-based clustering method for probability density functions and its application to detecting abnormal elements
- Hung Tran-Nam
- , Thao Nguyen-Trang
- & Ha Che-Ngoc
Article 30 July 2024 | Open Access
A dynamic customer segmentation approach by combining LRFMS and multivariate time series clustering
- Shuhai Wang
- , Linfu Sun
- & Yang Yu
Article 29 July 2024 | Open Access
Field evaluation of a volatile pyrethroid spatial repellent and etofenprox treated clothing for outdoor protection against forest malaria vectors in Cambodia
- Élodie A. Vajda
- , Amanda Ross
- & Neil F. Lobo
Study on crease recovery property of warp-knitted jacquard spacer shoe upper material
- & Shiyu Peng
Article 27 July 2024 | Open Access
Calibration estimation of population total using multi-auxiliary information in the presence of non-response
- , Anant Patel
- & Menakshi Pachori
Simulation-based prior knowledge elicitation for parametric Bayesian models
- Florence Bockting
- , Stefan T. Radev
- & Paul-Christian Bürkner
Article 26 July 2024 | Open Access
Modelling Salmonella Typhi in high-density urban Blantyre neighbourhood, Malawi, using point pattern methods
- Jessie J. Khaki
- , James E. Meiring
- & Emanuele Giorgi
Exogenous variable driven deep learning models for improved price forecasting of TOP crops in India
- G. H. Harish Nayak
- , Md Wasi Alam
- & Chandan Kumar Deb
Generalization of cut-in pre-crash scenarios for autonomous vehicles based on accident data
- , Xinyu Zhu
- & Chang Xu
Article 19 July 2024 | Open Access
Automated PD-L1 status prediction in lung cancer with multi-modal PET/CT fusion
- Ronrick Da-ano
- , Gustavo Andrade-Miranda
- & Catherine Cheze Le Rest
Article 17 July 2024 | Open Access
Optimizing decision-making with aggregation operators for generalized intuitionistic fuzzy sets and their applications in the tech industry
- Muhammad Wasim
- , Awais Yousaf
- & Hamiden Abd El-Wahed Khalifa
Article 15 July 2024 | Open Access
Putting ICAP to the test: how technology-enhanced learning activities are related to cognitive and affective-motivational learning outcomes in higher education
- Christina Wekerle
- , Martin Daumiller
- & Ingo Kollar
The impact of national savings on economic development: a focused study on the ten poorest countries in Sub-Saharan Africa
Article 13 July 2024 | Open Access
Regularized ensemble learning for prediction and risk factors assessment of students at risk in the post-COVID era
- Zardad Khan
- , Amjad Ali
- & Saeed Aldahmani
Article 12 July 2024 | Open Access
Eigen-entropy based time series signatures to support multivariate time series classification
- Abhidnya Patharkar
- , Jiajing Huang
- & Naomi Gades
Article 11 July 2024 | Open Access
Exploring usage pattern variation of free-floating bike-sharing from a night travel perspective
- , Xianke Han
- & Lili Li
Early mutational signatures and transmissibility of SARS-CoV-2 Gamma and Lambda variants in Chile
- Karen Y. Oróstica
- , Sebastian B. Mohr
- & Seba Contreras
Article 10 July 2024 | Open Access
Optimizing the location of vaccination sites to stop a zoonotic epidemic
- Ricardo Castillo-Neyra
- , Sherrie Xie
- & Michael Z. Levy
Article 08 July 2024 | Open Access
Integrating socio-psychological factors in the SEIR model optimized by a genetic algorithm for COVID-19 trend analysis
- Haonan Wang
- , Danhong Wu
- & Junhui Zhang
Article 05 July 2024 | Open Access
Research on bearing fault diagnosis based on improved genetic algorithm and BP neural network
- Zenghua Chen
- , Lingjian Zhu
- & Gang Xiong
Article 04 July 2024 | Open Access
Employees’ pro-environmental behavior in an organization: a case study in the UAE
- Nadin Alherimi
- , Zeki Marva
- & Ayman Alzaaterh
Article 03 July 2024 | Open Access
The predictive capability of several anthropometric indices for identifying the risk of metabolic syndrome and its components among industrial workers
- Ekaterina D. Konstantinova
- , Tatiana A. Maslakova
- & Svetlana Yu. Ogorodnikova
Article 02 July 2024 | Open Access
A bayesian spatio-temporal dynamic analysis of food security in Africa
- Adusei Bofa
- & Temesgen Zewotir
Research on the influencing factors of promoting flipped classroom teaching based on the integrated UTAUT model and learning engagement theory
- & Wang He
Article 28 June 2024 | Open Access
Peak response regularization for localization
- , Jinzhen Yao
- & Qintao Hu
Article 25 June 2024 | Open Access
Prediction and reliability analysis of shear strength of RC deep beams
- Khaled Megahed
Multistage time-to-event models improve survival inference by partitioning mortality processes of tracked organisms
- Suresh A. Sethi
- , Alex L. Koeberle
- & Kenneth Duren
Article 24 June 2024 | Open Access
Summarizing physical performance in professional soccer: development of a new composite index
- José M. Oliva-Lozano
- , Mattia Cefis
- & Ricardo Resta
Finding multifaceted communities in multiplex networks
- László Gadár
- & János Abonyi
Article 22 June 2024 | Open Access
Utilizing Bayesian inference in accelerated testing models under constant stress via ordered ranked set sampling and hybrid censoring with practical validation
- Atef F. Hashem
- , Naif Alotaibi
- & Alaa H. Abdel-Hamid
Predicting chronic wasting disease in white-tailed deer at the county scale using machine learning
- Md Sohel Ahmed
- , Brenda J. Hanley
- & Krysten L. Schuler
Article 21 June 2024 | Open Access
Properties, quantile regression, and application of bounded exponentiated Weibull distribution to COVID-19 data of mortality and survival rates
- Shakila Bashir
- , Bushra Masood
- & Iram Saleem
Article 20 June 2024 | Open Access
Cox proportional hazards regression in small studies of predictive biomarkers
- , V. H. Nguyen
- & M. Hauptmann
Article 17 June 2024 | Open Access
Multivariate testing and effect size measures for batch effect evaluation in radiomic features
- Hannah Horng
- , Christopher Scott
- & Russell T. Shinohara
Browse broader subjects
- Mathematics and computing
Quick links
- Explore articles by subject
- Guide to authors
- Editorial policies

Top 9 Statistical Tools Used in Research
Well-designed research requires a well-chosen study sample and a suitable statistical test selection . To plan an epidemiological study or a clinical trial, you’ll need a solid understanding of the data . Improper inferences from it could lead to false conclusions and unethical behavior . And given the ocean of data available nowadays, it’s often a daunting task for researchers to gauge its credibility and do statistical analysis on it.
With that said, thanks to all the statistical tools available in the market that help researchers make such studies much more manageable. Statistical tools are extensively used in academic and research sectors to study human, animal, and material behaviors and reactions.
Statistical tools aid in the interpretation and use of data. They can be used to evaluate and comprehend any form of data. Some statistical tools can help you see trends, forecast future sales, and create links between causes and effects. When you’re unsure where to go with your study, other tools can assist you in navigating through enormous amounts of data.
What is Statistics? And its Importance in Research
Statistics is the study of collecting, arranging, and interpreting data from samples and inferring it to the total population. Also known as the “Science of Data,” it allows us to derive conclusions from a data set. It may also assist people in all industries in answering research or business queries and forecast outcomes, such as what show you should watch next on your favorite video app.
Statistics is a technique that social scientists, such as psychologists, use to examine data and answer research questions. Scientists raise a wide range of questions that statistics can answer. Moreover, it provides credibility and legitimacy to research. If two research publications are presented, one without statistics and the other with statistical analysis supporting each assertion, people will choose the latter.
Statistical Tools Used in Research
Researchers often cannot discern a simple truth from a set of data. They can only draw conclusions from data after statistical analysis. On the other hand, creating a statistical analysis is a difficult task. This is when statistical tools come into play. Researchers can use statistical tools to back up their claims, make sense of a vast set of data, graphically show complex data, or help clarify many things in a short period.
Let’s go through the top 9 best statistical tools used in research below:
SPSS first stores and organizes the data, then compile the data set to generate appropriate output. SPSS is intended to work with a wide range of variable data formats.
R is a statistical computing and graphics programming language that you may use to clean, analyze and graph your data. It is frequently used to estimate and display results by researchers from various fields and lecturers of statistics and research methodologies. It’s free, making it an appealing option, but it relies upon programming code rather than drop-down menus or buttons.
Many big tech companies are using SAS due to its support and integration for vast teams. Setting up the tool might be a bit time-consuming initially, but once it’s up and running, it’ll surely streamline your statistical processes.
Moreover, MATLAB provides a multi-paradigm numerical computing environment, which means that the language may be used for both procedural and object-oriented programming. MATLAB is ideal for matrix manipulation, including data function plotting, algorithm implementation, and user interface design, among other things. Last but not least, MATLAB can also run programs written in other programming languages.
Tableau is a data visualization program that is among the most competent on the market. In data analytics, the approach of data visualization is commonly employed. In only a few minutes, you can use Tableau to produce the best data visualization for a large amount of data. As a result, it aids the data analyst in making quick decisions. It has a large number of online analytical processing cubes, cloud databases, spreadsheets, and other tools. It also provides users with a drag-and-drop interface. As a result, the user must drag and drop the data set sheet into Tableau and set the filters according to their needs.
Some of the highlights of Tableau are:
7. MS EXCEL:
Microsoft Excel is undoubtedly one of the best and most used statistical tools for beginners looking to do basic data analysis. It provides data analytics specialists with cutting-edge solutions and can be used for both data visualization and simple statistics. Furthermore, it is the most suitable statistical tool for individuals who wish to apply fundamental data analysis approaches to their data.
You can apply various formulas and functions to your data in Excel without prior knowledge of statistics. The learning curve is great, and even freshers can achieve great results quickly since everything is just a click away. This makes Excel a great choice not only for amateurs but beginners as well.
8. RAPIDMINER:
RapidMiner is a valuable platform for data preparation, machine learning, and the deployment of predictive models. RapidMiner makes it simple to develop a data model from the beginning to the end. It comes with a complete data science suite. Machine learning, deep learning, text mining, and predictive analytics are all possible with it.
9. APACHE HADOOP:
So, if you have massive data on your hands and want something that doesn’t slow you down and works in a distributed way, Hadoop is the way to go.
Learn more about Statistics and Key Tools
Elasticity of Demand Explained in Plain Terms
An introduction to statistical power and a/b testing.
Statistical power is an integral part of A/B testing. And in this article, you will learn everything you need to know about it and how it is applied in A/B testing. A/B
What Data Analytics Tools Are And How To Use Them
When it comes to improving the quality of your products and services, data analytic tools are the antidotes. Regardless, people often have questions. What are data analytic tools? Why are
Learn More…
As an IT Engineer, who is passionate about learning and sharing. I have worked and learned quite a bit from Data Engineers, Data Analysts, Business Analysts, and Key Decision Makers almost for the past 5 years. Interested in learning more about Data Science and How to leverage it for better decision-making in my business and hopefully help you do the same in yours.
Recent Posts

An official website of the United States government
The .gov means it’s official. Federal government websites often end in .gov or .mil. Before sharing sensitive information, make sure you’re on a federal government site.
The site is secure. The https:// ensures that you are connecting to the official website and that any information you provide is encrypted and transmitted securely.
- Publications
- Account settings
- My Bibliography
- Collections
- Citation manager
Save citation to file
Email citation, add to collections.
- Create a new collection
- Add to an existing collection
Add to My Bibliography
Your saved search, create a file for external citation management software, your rss feed.
- Search in PubMed
- Search in NLM Catalog
- Add to Search
Descriptive Statistics: Reporting the Answers to the 5 Basic Questions of Who, What, Why, When, Where, and a Sixth, So What?
Affiliation.
- 1 From the Department of Surgery and Perioperative Care, Dell Medical School at the University of Texas at Austin, Austin, Texas.
- PMID: 28891910
- DOI: 10.1213/ANE.0000000000002471
Descriptive statistics are specific methods basically used to calculate, describe, and summarize collected research data in a logical, meaningful, and efficient way. Descriptive statistics are reported numerically in the manuscript text and/or in its tables, or graphically in its figures. This basic statistical tutorial discusses a series of fundamental concepts about descriptive statistics and their reporting. The mean, median, and mode are 3 measures of the center or central tendency of a set of data. In addition to a measure of its central tendency (mean, median, or mode), another important characteristic of a research data set is its variability or dispersion (ie, spread). In simplest terms, variability is how much the individual recorded scores or observed values differ from one another. The range, standard deviation, and interquartile range are 3 measures of variability or dispersion. The standard deviation is typically reported for a mean, and the interquartile range for a median. Testing for statistical significance, along with calculating the observed treatment effect (or the strength of the association between an exposure and an outcome), and generating a corresponding confidence interval are 3 tools commonly used by researchers (and their collaborating biostatistician or epidemiologist) to validly make inferences and more generalized conclusions from their collected data and descriptive statistics. A number of journals, including Anesthesia & Analgesia, strongly encourage or require the reporting of pertinent confidence intervals. A confidence interval can be calculated for virtually any variable or outcome measure in an experimental, quasi-experimental, or observational research study design. Generally speaking, in a clinical trial, the confidence interval is the range of values within which the true treatment effect in the population likely resides. In an observational study, the confidence interval is the range of values within which the true strength of the association between the exposure and the outcome (eg, the risk ratio or odds ratio) in the population likely resides. There are many possible ways to graphically display or illustrate different types of data. While there is often latitude as to the choice of format, ultimately, the simplest and most comprehensible format is preferred. Common examples include a histogram, bar chart, line chart or line graph, pie chart, scatterplot, and box-and-whisker plot. Valid and reliable descriptive statistics can answer basic yet important questions about a research data set, namely: "Who, What, Why, When, Where, How, How Much?"
PubMed Disclaimer
Similar articles
- Fundamentals of Research Data and Variables: The Devil Is in the Details. Vetter TR. Vetter TR. Anesth Analg. 2017 Oct;125(4):1375-1380. doi: 10.1213/ANE.0000000000002370. Anesth Analg. 2017. PMID: 28787341 Review.
- Repeated Measures Designs and Analysis of Longitudinal Data: If at First You Do Not Succeed-Try, Try Again. Schober P, Vetter TR. Schober P, et al. Anesth Analg. 2018 Aug;127(2):569-575. doi: 10.1213/ANE.0000000000003511. Anesth Analg. 2018. PMID: 29905618 Free PMC article.
- Preparing for the first meeting with a statistician. De Muth JE. De Muth JE. Am J Health Syst Pharm. 2008 Dec 15;65(24):2358-66. doi: 10.2146/ajhp070007. Am J Health Syst Pharm. 2008. PMID: 19052282 Review.
- Summarizing and presenting numerical data. Pupovac V, Petrovecki M. Pupovac V, et al. Biochem Med (Zagreb). 2011;21(2):106-10. doi: 10.11613/bm.2011.018. Biochem Med (Zagreb). 2011. PMID: 22135849
- Introduction to biostatistics: Part 2, Descriptive statistics. Gaddis GM, Gaddis ML. Gaddis GM, et al. Ann Emerg Med. 1990 Mar;19(3):309-15. doi: 10.1016/s0196-0644(05)82052-9. Ann Emerg Med. 1990. PMID: 2310070
- Canadian midwives' perspectives on the clinical impacts of point of care ultrasound in obstetrical care: A concurrent mixed-methods study. Johnston BK, Darling EK, Malott A, Thomas L, Murray-Davis B. Johnston BK, et al. Heliyon. 2024 Mar 5;10(6):e27512. doi: 10.1016/j.heliyon.2024.e27512. eCollection 2024 Mar 30. Heliyon. 2024. PMID: 38533003 Free PMC article.
- Validation and psychometric testing of the Chinese version of the prenatal body image questionnaire. Wang Q, Lin J, Zheng Q, Kang L, Zhang X, Zhang K, Lin R, Lin R. Wang Q, et al. BMC Pregnancy Childbirth. 2024 Feb 1;24(1):102. doi: 10.1186/s12884-024-06281-w. BMC Pregnancy Childbirth. 2024. PMID: 38302902 Free PMC article.
- Cracking the code: uncovering the factors that drive COVID-19 standard operating procedures compliance among school management in Malaysia. Ahmad NS, Karuppiah K, Praveena SM, Ali NF, Ramdas M, Mohammad Yusof NAD. Ahmad NS, et al. Sci Rep. 2024 Jan 4;14(1):556. doi: 10.1038/s41598-023-49968-4. Sci Rep. 2024. PMID: 38177620 Free PMC article.
- Comparison of Nonneurological Structures at Risk During Anterior-to-Psoas Versus Transpsoas Surgical Approaches Using Abdominal CT Imaging From L1 to S1. Razzouk J, Ramos O, Harianja G, Carter M, Mehta S, Wycliffe N, Danisa O, Cheng W. Razzouk J, et al. Int J Spine Surg. 2023 Dec 26;17(6):809-815. doi: 10.14444/8542. Int J Spine Surg. 2023. PMID: 37748918 Free PMC article.
- CT-based analysis of oblique lateral interbody fusion from L1 to L5: location of incision, feasibility of safe corridor approach, and influencing factors. Razzouk J, Ramos O, Mehta S, Harianja G, Wycliffe N, Danisa O, Cheng W. Razzouk J, et al. Eur Spine J. 2023 Jun;32(6):1947-1952. doi: 10.1007/s00586-023-07555-1. Epub 2023 Apr 28. Eur Spine J. 2023. PMID: 37118479
- Search in MeSH
Related information
- Cited in Books
LinkOut - more resources
Full text sources.
- Ingenta plc
- Ovid Technologies, Inc.
- Wolters Kluwer
Other Literature Sources
- scite Smart Citations
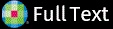
- Citation Manager
NCBI Literature Resources
MeSH PMC Bookshelf Disclaimer
The PubMed wordmark and PubMed logo are registered trademarks of the U.S. Department of Health and Human Services (HHS). Unauthorized use of these marks is strictly prohibited.

Effective Use of Statistics in Research – Methods and Tools for Data Analysis
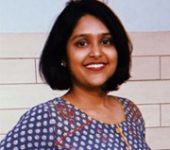
Remember that impending feeling you get when you are asked to analyze your data! Now that you have all the required raw data, you need to statistically prove your hypothesis. Representing your numerical data as part of statistics in research will also help in breaking the stereotype of being a biology student who can’t do math.
Statistical methods are essential for scientific research. In fact, statistical methods dominate the scientific research as they include planning, designing, collecting data, analyzing, drawing meaningful interpretation and reporting of research findings. Furthermore, the results acquired from research project are meaningless raw data unless analyzed with statistical tools. Therefore, determining statistics in research is of utmost necessity to justify research findings. In this article, we will discuss how using statistical methods for biology could help draw meaningful conclusion to analyze biological studies.
Table of Contents
Role of Statistics in Biological Research
Statistics is a branch of science that deals with collection, organization and analysis of data from the sample to the whole population. Moreover, it aids in designing a study more meticulously and also give a logical reasoning in concluding the hypothesis. Furthermore, biology study focuses on study of living organisms and their complex living pathways, which are very dynamic and cannot be explained with logical reasoning. However, statistics is more complex a field of study that defines and explains study patterns based on the sample sizes used. To be precise, statistics provides a trend in the conducted study.
Biological researchers often disregard the use of statistics in their research planning, and mainly use statistical tools at the end of their experiment. Therefore, giving rise to a complicated set of results which are not easily analyzed from statistical tools in research. Statistics in research can help a researcher approach the study in a stepwise manner, wherein the statistical analysis in research follows –
1. Establishing a Sample Size
Usually, a biological experiment starts with choosing samples and selecting the right number of repetitive experiments. Statistics in research deals with basics in statistics that provides statistical randomness and law of using large samples. Statistics teaches how choosing a sample size from a random large pool of sample helps extrapolate statistical findings and reduce experimental bias and errors.
2. Testing of Hypothesis
When conducting a statistical study with large sample pool, biological researchers must make sure that a conclusion is statistically significant. To achieve this, a researcher must create a hypothesis before examining the distribution of data. Furthermore, statistics in research helps interpret the data clustered near the mean of distributed data or spread across the distribution. These trends help analyze the sample and signify the hypothesis.
3. Data Interpretation Through Analysis
When dealing with large data, statistics in research assist in data analysis. This helps researchers to draw an effective conclusion from their experiment and observations. Concluding the study manually or from visual observation may give erroneous results; therefore, thorough statistical analysis will take into consideration all the other statistical measures and variance in the sample to provide a detailed interpretation of the data. Therefore, researchers produce a detailed and important data to support the conclusion.
Types of Statistical Research Methods That Aid in Data Analysis
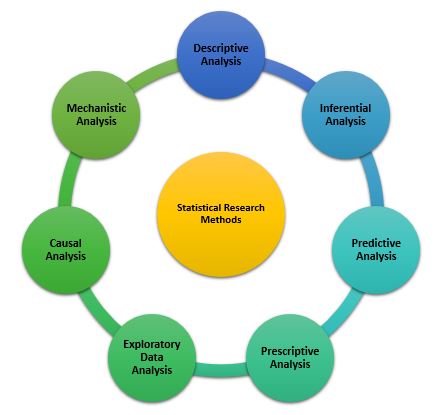
Statistical analysis is the process of analyzing samples of data into patterns or trends that help researchers anticipate situations and make appropriate research conclusions. Based on the type of data, statistical analyses are of the following type:
1. Descriptive Analysis
The descriptive statistical analysis allows organizing and summarizing the large data into graphs and tables . Descriptive analysis involves various processes such as tabulation, measure of central tendency, measure of dispersion or variance, skewness measurements etc.
2. Inferential Analysis
The inferential statistical analysis allows to extrapolate the data acquired from a small sample size to the complete population. This analysis helps draw conclusions and make decisions about the whole population on the basis of sample data. It is a highly recommended statistical method for research projects that work with smaller sample size and meaning to extrapolate conclusion for large population.
3. Predictive Analysis
Predictive analysis is used to make a prediction of future events. This analysis is approached by marketing companies, insurance organizations, online service providers, data-driven marketing, and financial corporations.
4. Prescriptive Analysis
Prescriptive analysis examines data to find out what can be done next. It is widely used in business analysis for finding out the best possible outcome for a situation. It is nearly related to descriptive and predictive analysis. However, prescriptive analysis deals with giving appropriate suggestions among the available preferences.
5. Exploratory Data Analysis
EDA is generally the first step of the data analysis process that is conducted before performing any other statistical analysis technique. It completely focuses on analyzing patterns in the data to recognize potential relationships. EDA is used to discover unknown associations within data, inspect missing data from collected data and obtain maximum insights.
6. Causal Analysis
Causal analysis assists in understanding and determining the reasons behind “why” things happen in a certain way, as they appear. This analysis helps identify root cause of failures or simply find the basic reason why something could happen. For example, causal analysis is used to understand what will happen to the provided variable if another variable changes.

7. Mechanistic Analysis
This is a least common type of statistical analysis. The mechanistic analysis is used in the process of big data analytics and biological science. It uses the concept of understanding individual changes in variables that cause changes in other variables correspondingly while excluding external influences.
Important Statistical Tools In Research
Researchers in the biological field find statistical analysis in research as the scariest aspect of completing research. However, statistical tools in research can help researchers understand what to do with data and how to interpret the results, making this process as easy as possible.
1. Statistical Package for Social Science (SPSS)
It is a widely used software package for human behavior research. SPSS can compile descriptive statistics, as well as graphical depictions of result. Moreover, it includes the option to create scripts that automate analysis or carry out more advanced statistical processing.
2. R Foundation for Statistical Computing
This software package is used among human behavior research and other fields. R is a powerful tool and has a steep learning curve. However, it requires a certain level of coding. Furthermore, it comes with an active community that is engaged in building and enhancing the software and the associated plugins.
3. MATLAB (The Mathworks)
It is an analytical platform and a programming language. Researchers and engineers use this software and create their own code and help answer their research question. While MatLab can be a difficult tool to use for novices, it offers flexibility in terms of what the researcher needs.
4. Microsoft Excel
Not the best solution for statistical analysis in research, but MS Excel offers wide variety of tools for data visualization and simple statistics. It is easy to generate summary and customizable graphs and figures. MS Excel is the most accessible option for those wanting to start with statistics.
5. Statistical Analysis Software (SAS)
It is a statistical platform used in business, healthcare, and human behavior research alike. It can carry out advanced analyzes and produce publication-worthy figures, tables and charts .
6. GraphPad Prism
It is a premium software that is primarily used among biology researchers. But, it offers a range of variety to be used in various other fields. Similar to SPSS, GraphPad gives scripting option to automate analyses to carry out complex statistical calculations.
This software offers basic as well as advanced statistical tools for data analysis. However, similar to GraphPad and SPSS, minitab needs command over coding and can offer automated analyses.
Use of Statistical Tools In Research and Data Analysis
Statistical tools manage the large data. Many biological studies use large data to analyze the trends and patterns in studies. Therefore, using statistical tools becomes essential, as they manage the large data sets, making data processing more convenient.
Following these steps will help biological researchers to showcase the statistics in research in detail, and develop accurate hypothesis and use correct tools for it.
There are a range of statistical tools in research which can help researchers manage their research data and improve the outcome of their research by better interpretation of data. You could use statistics in research by understanding the research question, knowledge of statistics and your personal experience in coding.
Have you faced challenges while using statistics in research? How did you manage it? Did you use any of the statistical tools to help you with your research data? Do write to us or comment below!
Frequently Asked Questions
Statistics in research can help a researcher approach the study in a stepwise manner: 1. Establishing a sample size 2. Testing of hypothesis 3. Data interpretation through analysis
Statistical methods are essential for scientific research. In fact, statistical methods dominate the scientific research as they include planning, designing, collecting data, analyzing, drawing meaningful interpretation and reporting of research findings. Furthermore, the results acquired from research project are meaningless raw data unless analyzed with statistical tools. Therefore, determining statistics in research is of utmost necessity to justify research findings.
Statistical tools in research can help researchers understand what to do with data and how to interpret the results, making this process as easy as possible. They can manage large data sets, making data processing more convenient. A great number of tools are available to carry out statistical analysis of data like SPSS, SAS (Statistical Analysis Software), and Minitab.

nice article to read
Holistic but delineating. A very good read.
Rate this article Cancel Reply
Your email address will not be published.
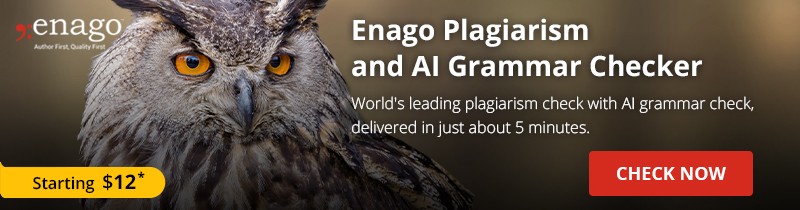
Enago Academy's Most Popular Articles
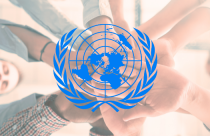
- Promoting Research
- Thought Leadership
- Trending Now
How Enago Academy Contributes to Sustainable Development Goals (SDGs) Through Empowering Researchers
The United Nations Sustainable Development Goals (SDGs) are a universal call to action to end…
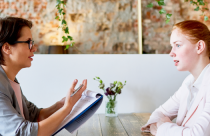
- Reporting Research
Research Interviews: An effective and insightful way of data collection
Research interviews play a pivotal role in collecting data for various academic, scientific, and professional…
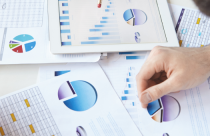
Planning Your Data Collection: Designing methods for effective research
Planning your research is very important to obtain desirable results. In research, the relevance of…
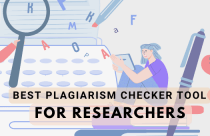
- Language & Grammar
Best Plagiarism Checker Tool for Researchers — Top 4 to choose from!
While common writing issues like language enhancement, punctuation errors, grammatical errors, etc. can be dealt…
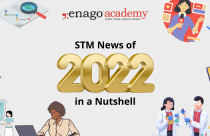
- Industry News
- Publishing News
2022 in a Nutshell — Reminiscing the year when opportunities were seized and feats were achieved!
It’s beginning to look a lot like success! Some of the greatest opportunities to research…
2022 in a Nutshell — Reminiscing the year when opportunities were seized and feats…

Sign-up to read more
Subscribe for free to get unrestricted access to all our resources on research writing and academic publishing including:
- 2000+ blog articles
- 50+ Webinars
- 10+ Expert podcasts
- 50+ Infographics
- 10+ Checklists
- Research Guides
We hate spam too. We promise to protect your privacy and never spam you.
- Publishing Research
- AI in Academia
- Career Corner
- Diversity and Inclusion
- Infographics
- Expert Video Library
- Other Resources
- Enago Learn
- Upcoming & On-Demand Webinars
- Peer Review Week 2024
- Open Access Week 2023
- Conference Videos
- Enago Report
- Journal Finder
- Enago Plagiarism & AI Grammar Check
- Editing Services
- Publication Support Services
- Research Impact
- Translation Services
- Publication solutions
- AI-Based Solutions
- Call for Articles
- Call for Speakers
- Author Training
- Edit Profile
I am looking for Editing/ Proofreading services for my manuscript Tentative date of next journal submission:
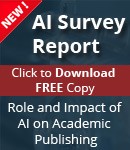
In your opinion, what is the most effective way to improve integrity in the peer review process?
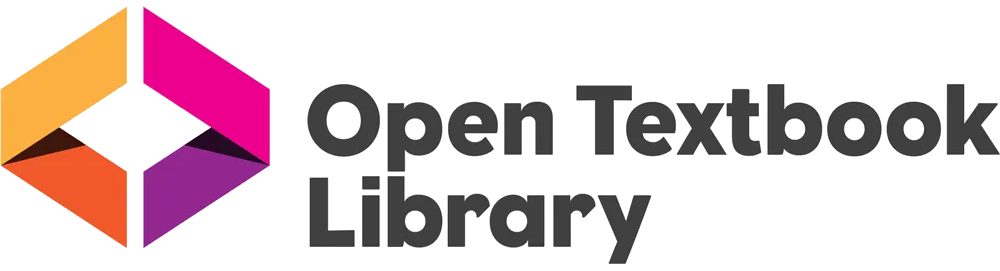
Statistics for Research Students
(2 reviews)

Erich C Fein, Toowoomba, Australia
John Gilmour, Toowoomba, Australia
Tayna Machin, Toowoomba, Australia
Liam Hendry, Toowoomba, Australia
Copyright Year: 2022
ISBN 13: 9780645326109
Publisher: University of Southern Queensland
Language: English
Formats Available
Conditions of use.

Learn more about reviews.
Reviewed by Sojib Bin Zaman, Assistant Professor, James Madison University on 3/18/24
From exploring data in Chapter One to learning advanced methodologies such as moderation and mediation in Chapter Seven, the reader is guided through the entire process of statistical methodology. With each chapter covering a different statistical... read more
Comprehensiveness rating: 5 see less
From exploring data in Chapter One to learning advanced methodologies such as moderation and mediation in Chapter Seven, the reader is guided through the entire process of statistical methodology. With each chapter covering a different statistical technique and methodology, students gain a comprehensive understanding of statistical research techniques.
Content Accuracy rating: 5
During my review of the textbook, I did not find any notable errors or omissions. In my opinion, the material was comprehensive, resulting in an enjoyable learning experience.
Relevance/Longevity rating: 5
A majority of the textbook's content is aligned with current trends, advancements, and enduring principles in the field of statistics. Several emerging methodologies and technologies are incorporated into this textbook to enhance students' statistical knowledge. It will be a valuable resource in the long run if students and researchers can properly utilize this textbook.
Clarity rating: 5
A clear explanation of complex statistical concepts such as moderation and mediation is provided in the writing style. Examples and problem sets are provided in the textbook in a comprehensive and well-explained manner.
Consistency rating: 5
Each chapter maintains consistent formatting and language, with resources organized consistently. Headings and subheadings worked well.
Modularity rating: 5
The textbook is well-structured, featuring cohesive chapters that flow smoothly from one to another. It is carefully crafted with a focus on defining terms clearly, facilitating understanding, and ensuring logical flow.
Organization/Structure/Flow rating: 5
From basic to advanced concepts, this book provides clarity of progression, logical arranging of sections and chapters, and effective headings and subheadings that guide readers. Further, the organization provides students with a lot of information on complex statistical methodologies.
Interface rating: 5
The available formats included PDFs, online access, and e-books. The e-book interface was particularly appealing to me, as it provided seamless navigation and viewing of content without compromising usability.
Grammatical Errors rating: 5
I found no significant errors in this document, and the overall quality of the writing was commendable. There was a high level of clarity and coherence in the text, which contributed to a positive reading experience.
Cultural Relevance rating: 5
The content of the book, as well as its accompanying examples, demonstrates a dedication to inclusivity by taking into account cultural diversity and a variety of perspectives. Furthermore, the material actively promotes cultural diversity, which enables readers to develop a deeper understanding of various cultural contexts and experiences.
In summary, this textbook provides a comprehensive resource tailored for advanced statistics courses, characterized by meticulous organization and practical supplementary materials. This book also provides valuable insights into the interpretation of computer output that enhance a greater understanding of each concept presented.
Reviewed by Zhuanzhuan Ma, Assistant Professor, University of Texas Rio Grande Valley on 3/7/24
The textbook covers all necessary areas and topics for students who want to conduct research in statistics. It includes foundational concepts, application methods, and advanced statistical techniques relevant to research methodologies. read more
The textbook covers all necessary areas and topics for students who want to conduct research in statistics. It includes foundational concepts, application methods, and advanced statistical techniques relevant to research methodologies.
The textbook presents statistical methods and data accurately, with up-to-date statistical practices and examples.
Relevance/Longevity rating: 4
The textbook's content is relevant to current research practices. The book includes contemporary examples and case studies that are currently prevalent in research communities. One small drawback is that the textbook did not include the example code for conduct data analysis.
The textbook break down complex statistical methods into understandable segments. All the concepts are clearly explained. Authors used diagrams, examples, and all kinds of explanations to facilitate learning for students with varying levels of background knowledge.
The terminology, framework, and presentation style (e.g. concepts, methodologies, and examples) seem consistent throughout the book.
The textbook is well organized that each chapter and section can be used independently without losing the context necessary for understanding. Also, the modular structure allows instructors and students to adapt the materials for different study plans.
The textbook is well-organized and progresses from basic concepts to more complex methods, making it easier for students to follow along. There is a logical flow of the content.
The digital format of the textbook has an interface that includes the design, layout, and navigational features. It is easier to use for readers.
The quality of writing is very high. The well-written texts help both instructors and students to follow the ideas clearly.
The textbook does not perpetuate stereotypes or biases and are inclusive in their examples, language, and perspectives.
Table of Contents
- Acknowledgement of Country
- Accessibility Information
- About the Authors
- Introduction
- I. Chapter One - Exploring Your Data
- II. Chapter Two - Test Statistics, p Values, Confidence Intervals and Effect Sizes
- III. Chapter Three- Comparing Two Group Means
- IV. Chapter Four - Comparing Associations Between Two Variables
- V. Chapter Five- Comparing Associations Between Multiple Variables
- VI. Chapter Six- Comparing Three or More Group Means
- VII. Chapter Seven- Moderation and Mediation Analyses
- VIII. Chapter Eight- Factor Analysis and Scale Reliability
- IX. Chapter Nine- Nonparametric Statistics
Ancillary Material
About the book.
This book aims to help you understand and navigate statistical concepts and the main types of statistical analyses essential for research students.
About the Contributors
Dr Erich C. Fein is an Associate Professor at the University of Southern Queensland. He received substantial training in research methods and statistics during his PhD program at Ohio State University. He currently teaches four courses in research methods and statistics. His research involves leadership, occupational health, and motivation, as well as issues related to research methods such as the following article: “ Safeguarding Access and Safeguarding Meaning as Strategies for Achieving Confidentiality .” Click here to link to his Google Scholar profile.
Dr John Gilmour is a Lecturer at the University of Southern Queensland and a Postdoctoral Research Fellow at the University of Queensland, His research focuses on the locational and temporal analyses of crime, and the evaluation of police training and procedures. John has worked across many different sectors including PTSD, social media, criminology, and medicine.
Dr Tanya Machin is a Senior Lecturer and Associate Dean at the University of Southern Queensland. Her research focuses on social media and technology across the lifespan. Tanya has co-taught Honours research methods with Erich, and is also interested in ethics and qualitative research methods. Tanya has worked across many different sectors including primary schools, financial services, and mental health.
Dr Liam Hendry is a Lecturer at the University of Southern Queensland. His research interests focus on long-term and short-term memory, measurement of human memory, attention, learning & diverse aspects of cognitive psychology.
Contribute to this Page
Introduction: Statistics as a Research Tool
- First Online: 24 February 2021
Cite this chapter
- David Weisburd 5 , 6 ,
- Chester Britt 7 ,
- David B. Wilson 5 &
- Alese Wooditch 8
2539 Accesses
Statistics seem intimidating because they are associated with complex mathematical formulas and computations. Although some knowledge of math is required, an understanding of the concepts is much more important than an in-depth understanding of the computations. The researcher’s aim in using statistics is to communicate findings in a clear and simple form. As a result, the researcher should always choose the simplest statistic appropriate for answering the research question. Statistics offer commonsense solutions to research problems. The following principles apply to all types of statistics: (1) in developing statistics, we seek to reduce the level of error as much as possible; (2) statistics based on more information are generally preferred over those based on less information; (3) outliers present a significant problem in choosing and interpreting statistics; and (4) the researcher must strive to systematize the procedures used in data collection and analysis. There are two principal uses of statistics discussed in this book. In descriptive statistics, the researcher summarizes large amounts of information in an efficient manner. Two types of descriptive statistics that go hand in hand are measures of central tendency, which describe the characteristics of the average case, and measures of dispersion, which tell us just how typical this average case is. We use inferential statistics to make statements about a population on the basis of a sample drawn from that population.
This is a preview of subscription content, log in via an institution to check access.
Access this chapter
Subscribe and save.
- Get 10 units per month
- Download Article/Chapter or eBook
- 1 Unit = 1 Article or 1 Chapter
- Cancel anytime
- Available as PDF
- Read on any device
- Instant download
- Own it forever
- Available as EPUB and PDF
- Compact, lightweight edition
- Dispatched in 3 to 5 business days
- Free shipping worldwide - see info
- Durable hardcover edition
Tax calculation will be finalised at checkout
Purchases are for personal use only
Institutional subscriptions
Reiss, A. J., Jr. (1971). Systematic Observation of Natural Social Phenomena. Sociological Methodology, 3, 3–33. doi:10.2307/270816
Google Scholar
National Institute of Justice (2016). National Institute of Justice Annual Report: 2016. Washington, DC: U.S. Department of Justice, Office of Justice Programs, National Institute of Justice.
Download references
Author information
Authors and affiliations.
Department of Criminology, Law and Society, George Mason University, Fairfax, VA, USA
David Weisburd & David B. Wilson
Institute of Criminology, Faculty of Law, Hebrew University of Jerusalem, Jerusalem, Israel
David Weisburd
Iowa State University, Ames, IA, USA
Chester Britt
Department of Criminal Justice, Temple University, Philadelphia, PA, USA
Alese Wooditch
You can also search for this author in PubMed Google Scholar
Rights and permissions
Reprints and permissions
Copyright information
© 2020 Springer Nature Switzerland AG
About this chapter
Weisburd, D., Britt, C., Wilson, D.B., Wooditch, A. (2020). Introduction: Statistics as a Research Tool. In: Basic Statistics in Criminology and Criminal Justice. Springer, Cham. https://doi.org/10.1007/978-3-030-47967-1_1
Download citation
DOI : https://doi.org/10.1007/978-3-030-47967-1_1
Published : 24 February 2021
Publisher Name : Springer, Cham
Print ISBN : 978-3-030-47966-4
Online ISBN : 978-3-030-47967-1
eBook Packages : Law and Criminology Law and Criminology (R0)
Share this chapter
Anyone you share the following link with will be able to read this content:
Sorry, a shareable link is not currently available for this article.
Provided by the Springer Nature SharedIt content-sharing initiative
- Publish with us
Policies and ethics
- Find a journal
- Track your research

Understanding and Using Statistical Methods
Statistics is a set of tools used to organize and analyze data. Data must either be numeric in origin or transformed by researchers into numbers. For instance, statistics could be used to analyze percentage scores English students receive on a grammar test: the percentage scores ranging from 0 to 100 are already in numeric form. Statistics could also be used to analyze grades on an essay by assigning numeric values to the letter grades, e.g., A=4, B=3, C=2, D=1, and F=0.
Employing statistics serves two purposes, (1) description and (2) prediction. Statistics are used to describe the characteristics of groups. These characteristics are referred to as variables . Data is gathered and recorded for each variable. Descriptive statistics can then be used to reveal the distribution of the data in each variable.
Statistics is also frequently used for purposes of prediction. Prediction is based on the concept of generalizability : if enough data is compiled about a particular context (e.g., students studying writing in a specific set of classrooms), the patterns revealed through analysis of the data collected about that context can be generalized (or predicted to occur in) similar contexts. The prediction of what will happen in a similar context is probabilistic . That is, the researcher is not certain that the same things will happen in other contexts; instead, the researcher can only reasonably expect that the same things will happen.
Prediction is a method employed by individuals throughout daily life. For instance, if writing students begin class every day for the first half of the semester with a five-minute freewriting exercise, then they will likely come to class the first day of the second half of the semester prepared to again freewrite for the first five minutes of class. The students will have made a prediction about the class content based on their previous experiences in the class: Because they began all previous class sessions with freewriting, it would be probable that their next class session will begin the same way. Statistics is used to perform the same function; the difference is that precise probabilities are determined in terms of the percentage chance that an outcome will occur, complete with a range of error. Prediction is a primary goal of inferential statistics.
Revealing Patterns Using Descriptive Statistics
Descriptive statistics, not surprisingly, "describe" data that have been collected. Commonly used descriptive statistics include frequency counts, ranges (high and low scores or values), means, modes, median scores, and standard deviations. Two concepts are essential to understanding descriptive statistics: variables and distributions .
Statistics are used to explore numerical data (Levin, 1991). Numerical data are observations which are recorded in the form of numbers (Runyon, 1976). Numbers are variable in nature, which means that quantities vary according to certain factors. For examples, when analyzing the grades on student essays, scores will vary for reasons such as the writing ability of the student, the students' knowledge of the subject, and so on. In statistics, these reasons are called variables. Variables are divided into three basic categories:
Nominal Variables
Nominal variables classify data into categories. This process involves labeling categories and then counting frequencies of occurrence (Runyon, 1991). A researcher might wish to compare essay grades between male and female students. Tabulations would be compiled using the categories "male" and "female." Sex would be a nominal variable. Note that the categories themselves are not quantified. Maleness or femaleness are not numerical in nature, rather the frequencies of each category results in data that is quantified -- 11 males and 9 females.
Ordinal Variables
Ordinal variables order (or rank) data in terms of degree. Ordinal variables do not establish the numeric difference between data points. They indicate only that one data point is ranked higher or lower than another (Runyon, 1991). For instance, a researcher might want to analyze the letter grades given on student essays. An A would be ranked higher than a B, and a B higher than a C. However, the difference between these data points, the precise distance between an A and a B, is not defined. Letter grades are an example of an ordinal variable.
Interval Variables
Interval variables score data. Thus the order of data is known as well as the precise numeric distance between data points (Runyon, 1991). A researcher might analyze the actual percentage scores of the essays, assuming that percentage scores are given by the instructor. A score of 98 (A) ranks higher than a score of 87 (B), which ranks higher than a score of 72 (C). Not only is the order of these three data points known, but so is the exact distance between them -- 11 percentage points between the first two, 15 percentage points between the second two and 26 percentage points between the first and last data points.
Distributions
A distribution is a graphic representation of data. The line formed by connecting data points is called a frequency distribution. This line may take many shapes. The single most important shape is that of the bell-shaped curve, which characterizes the distribution as "normal." A perfectly normal distribution is only a theoretical ideal. This ideal, however, is an essential ingredient in statistical decision-making (Levin, 1991). A perfectly normal distribution is a mathematical construct which carries with it certain mathematical properties helpful in describing the attributes of the distribution. Although frequency distribution based on actual data points seldom, if ever, completely matches a perfectly normal distribution, a frequency distribution often can approach such a normal curve.
The closer a frequency distribution resembles a normal curve, the more probable that the distribution maintains those same mathematical properties as the normal curve. This is an important factor in describing the characteristics of a frequency distribution. As a frequency distribution approaches a normal curve, generalizations about the data set from which the distribution was derived can be made with greater certainty. And it is this notion of generalizability upon which statistics is founded. It is important to remember that not all frequency distributions approach a normal curve. Some are skewed. When a frequency distribution is skewed, the characteristics inherent to a normal curve no longer apply.
Making Predictions Using Inferential Statistics
Inferential statistics are used to draw conclusions and make predictions based on the descriptions of data. In this section, we explore inferential statistics by using an extended example of experimental studies. Key concepts used in our discussion are probability, populations, and sampling.
Experiments
A typical experimental study involves collecting data on the behaviors, attitudes, or actions of two or more groups and attempting to answer a research question (often called a hypothesis). Based on the analysis of the data, a researcher might then attempt to develop a causal model that can be generalized to populations.
A question that might be addressed through experimental research might be "Does grammar-based writing instruction produce better writers than process-based writing instruction?" Because it would be impossible and impractical to observe, interview, survey, etc. all first-year writing students and instructors in classes using one or the other of these instructional approaches, a researcher would study a sample – or a subset – of a population. Sampling – or the creation of this subset of a population – is used by many researchers who desire to make sense of some phenomenon.
To analyze differences in the ability of student writers who are taught in each type of classroom, the researcher would compare the writing performance of the two groups of students.
Dependent Variables
In an experimental study, a variable whose score depends on (or is determined or caused by) another variable is called a dependent variable. For instance, an experiment might explore the extent to which the writing quality of final drafts of student papers is affected by the kind of instruction they received. In this case, the dependent variable would be writing quality of final drafts.
Independent Variables
In an experimental study, a variable that determines (or causes) the score of a dependent variable is called an independent variable. For instance, an experiment might explore the extent to which the writing quality of final drafts of student papers is affected by the kind of instruction they received. In this case, the independent variable would be the kind of instruction students received.
Probability
Beginning researchers most often use the word probability to express a subjective judgment about the likelihood, or degree of certainty, that a particular event will occur. People say such things as: "It will probably rain tomorrow." "It is unlikely that we will win the ball game." It is possible to assign a number to the event being predicted, a number between 0 and 1, which represents degree of confidence that the event will occur. For example, a student might say that the likelihood an instructor will give an exam next week is about 90 percent, or .9. Where 100 percent, or 1.00, represents certainty, .9 would mean the student is almost certain the instructor will give an exam. If the student assigned the number .6, the likelihood of an exam would be just slightly greater than the likelihood of no exam. A rating of 0 would indicate complete certainty that no exam would be given(Shoeninger, 1971).
The probability of a particular outcome or set of outcomes is called a p-value . In our discussion, a p-value will be symbolized by a p followed by parentheses enclosing a symbol of the outcome or set of outcomes. For example, p(X) should be read, "the probability of a given X score" (Shoeninger). Thus p(exam) should be read, "the probability an instructor will give an exam next week."
A population is a group which is studied. In educational research, the population is usually a group of people. Researchers seldom are able to study every member of a population. Usually, they instead study a representative sample – or subset – of a population. Researchers then generalize their findings about the sample to the population as a whole.
Sampling is performed so that a population under study can be reduced to a manageable size. This can be accomplished via random sampling, discussed below, or via matching.
Random sampling is a procedure used by researchers in which all samples of a particular size have an equal chance to be chosen for an observation, experiment, etc (Runyon and Haber, 1976). There is no predetermination as to which members are chosen for the sample. This type of sampling is done in order to minimize scientific biases and offers the greatest likelihood that a sample will indeed be representative of the larger population. The aim here is to make the sample as representative of the population as possible. Note that the closer a sample distribution approximates the population distribution, the more generalizable the results of the sample study are to the population. Notions of probability apply here. Random sampling provides the greatest probability that the distribution of scores in a sample will closely approximate the distribution of scores in the overall population.
Matching is a method used by researchers to gain accurate and precise results of a study so that they may be applicable to a larger population. After a population has been examined and a sample has been chosen, a researcher must then consider variables, or extrinsic factors, that might affect the study. Matching methods apply when researchers are aware of extrinsic variables before conducting a study. Two methods used to match groups are:
Precision Matching
In precision matching , there is an experimental group that is matched with a control group. Both groups, in essence, have the same characteristics. Thus, the proposed causal relationship/model being examined allows for the probabilistic assumption that the result is generalizable.
Frequency Distribution
Frequency distribution is more manageable and efficient than precision matching. Instead of one-to-one matching that must be administered in precision matching, frequency distribution allows the comparison of an experimental and control group through relevant variables. If three Communications majors and four English majors are chosen for the control group, then an equal proportion of three Communications major and four English majors should be allotted to the experiment group. Of course, beyond their majors, the characteristics of the matched sets of participants may in fact be vastly different.
Although, in theory, matching tends to produce valid conclusions, a rather obvious difficulty arises in finding subjects which are compatible. Researchers may even believe that experimental and control groups are identical when, in fact, a number of variables have been overlooked. For these reasons, researchers tend to reject matching methods in favor of random sampling.
Statistics can be used to analyze individual variables, relationships among variables, and differences between groups. In this section, we explore a range of statistical methods for conducting these analyses.
Statistics can be used to analyze individual variables, relationships among variables, and differences between groups.
Analyzing Individual Variables
The statistical procedures used to analyze a single variable describing a group (such as a population or representative sample) involve measures of central tendency and measures of variation . To explore these measures, a researcher first needs to consider the distribution , or range of values of a particular variable in a population or sample. Normal distribution occurs if the distribution of a population is completely normal. When graphed, this type of distribution will look like a bell curve; it is symmetrical and most of the scores cluster toward the middle. Skewed Distribution simply means the distribution of a population is not normal. The scores might cluster toward the right or the left side of the curve, for instance. Or there might be two or more clusters of scores, so that the distribution looks like a series of hills.
Once frequency distributions have been determined, researchers can calculate measures of central tendency and measures of variation. Measures of central tendency indicate averages of the distribution, and measures of variation indicate the spread, or range, of the distribution (Hinkle, Wiersma and Jurs 1988).
Measures of Central Tendency
Central tendency is measured in three ways: mean , median and mode . The mean is simply the average score of a distribution. The median is the center, or middle score within a distribution. The mode is the most frequent score within a distribution. In a normal distribution, the mean, median and mode are identical.
Student | # of Crayons | |
---|---|---|
A | 8 | |
B | 16 | |
C | 16 | |
D | 32 | |
E | 32 | |
F | 32 | |
G | 48 | |
H | 48 | |
J | 56 |
Measures of Variation
Measures of variation determine the range of the distribution, relative to the measures of central tendency. Where the measures of central tendency are specific data points, measures of variation are lengths between various points within the distribution. Variation is measured in terms of range, mean deviation, variance, and standard deviation (Hinkle, Wiersma and Jurs 1988).
The range is the distance between the lowest data point and the highest data point. Deviation scores are the distances between each data point and the mean.
Mean deviation is the average of the absolute values of the deviation scores; that is, mean deviation is the average distance between the mean and the data points. Closely related to the measure of mean deviation is the measure of variance .
Variance also indicates a relationship between the mean of a distribution and the data points; it is determined by averaging the sum of the squared deviations. Squaring the differences instead of taking the absolute values allows for greater flexibility in calculating further algebraic manipulations of the data. Another measure of variation is the standard deviation .
Standard deviation is the square root of the variance. This calculation is useful because it allows for the same flexibility as variance regarding further calculations and yet also expresses variation in the same units as the original measurements (Hinkle, Wiersma and Jurs 1988).
Analyzing Differences Between Groups
Statistical tests can be used to analyze differences in the scores of two or more groups. The following statistical tests are commonly used to analyze differences between groups:
A t-test is used to determine if the scores of two groups differ on a single variable. A t-test is designed to test for the differences in mean scores. For instance, you could use a t-test to determine whether writing ability differs among students in two classrooms.
Note: A t-test is appropriate only when looking at paired data. It is useful in analyzing scores of two groups of participants on a particular variable or in analyzing scores of a single group of participants on two variables.
Matched Pairs T-Test
This type of t-test could be used to determine if the scores of the same participants in a study differ under different conditions. For instance, this sort of t-test could be used to determine if people write better essays after taking a writing class than they did before taking the writing class.
Analysis of Variance (ANOVA)
The ANOVA (analysis of variance) is a statistical test which makes a single, overall decision as to whether a significant difference is present among three or more sample means (Levin 484). An ANOVA is similar to a t-test. However, the ANOVA can also test multiple groups to see if they differ on one or more variables. The ANOVA can be used to test between-groups and within-groups differences. There are two types of ANOVAs:
One-Way ANOVA: This tests a group or groups to determine if there are differences on a single set of scores. For instance, a one-way ANOVA could determine whether freshmen, sophomores, juniors, and seniors differed in their reading ability.
Multiple ANOVA (MANOVA): This tests a group or groups to determine if there are differences on two or more variables. For instance, a MANOVA could determine whether freshmen, sophomores, juniors, and seniors differed in reading ability and whether those differences were reflected by gender. In this case, a researcher could determine (1) whether reading ability differed across class levels, (2) whether reading ability differed across gender, and (3) whether there was an interaction between class level and gender.
Analyzing Relationships Among Variables
Statistical relationships between variables rely on notions of correlation and regression. These two concepts aim to describe the ways in which variables relate to one another:
Correlation
Correlation tests are used to determine how strongly the scores of two variables are associated or correlated with each other. A researcher might want to know, for instance, whether a correlation exists between students' writing placement examination scores and their scores on a standardized test such as the ACT or SAT. Correlation is measured using values between +1.0 and -1.0. Correlations close to 0 indicate little or no relationship between two variables, while correlations close to +1.0 (or -1.0) indicate strong positive (or negative) relationships (Hayes et al. 554).
Correlation denotes positive or negative association between variables in a study. Two variables are positively associated when larger values of one tend to be accompanied by larger values of the other. The variables are negatively associated when larger values of one tend to be accompanied by smaller values of the other (Moore 208).
An example of a strong positive correlation would be the correlation between age and job experience. Typically, the longer people are alive, the more job experience they might have.
An example of a strong negative relationship might occur between the strength of people's party affiliations and their willingness to vote for a candidate from different parties. In many elections, Democrats are unlikely to vote for Republicans, and vice versa.
Regression analysis attempts to determine the best "fit" between two or more variables. The independent variable in a regression analysis is a continuous variable, and thus allows you to determine how one or more independent variables predict the values of a dependent variable.
Simple Linear Regression is the simplest form of regression. Like a correlation, it determines the extent to which one independent variables predicts a dependent variable. You can think of a simple linear regression as a correlation line. Regression analysis provides you with more information than correlation does, however. It tells you how well the line "fits" the data. That is, it tells you how closely the line comes to all of your data points. The line in the figure indicates the regression line drawn to find the best fit among a set of data points. Each dot represents a person and the axes indicate the amount of job experience and the age of that person. The dotted lines indicate the distance from the regression line. A smaller total distance indicates a better fit. Some of the information provided in a regression analysis, as a result, indicates the slope of the regression line, the R value (or correlation), and the strength of the fit (an indication of the extent to which the line can account for variations among the data points).
Multiple Linear Regression allows one to determine how well multiple independent variables predict the value of a dependent variable. A researcher might examine, for instance, how well age and experience predict a person's salary. The interesting thing here is that one would no longer be dealing with a regression "line." Instead, since the study deals with three dimensions (age, experience, and salary), it would be dealing with a plane, that is, with a two-dimensional figure. If a fourth variable was added to the equations, one would be dealing with a three-dimensional figure, and so on.
Misuses of Statistics
Statistics consists of tests used to analyze data. These tests provide an analytic framework within which researchers can pursue their research questions. This framework provides one way of working with observable information. Like other analytic frameworks, statistical tests can be misused, resulting in potential misinterpretation and misrepresentation. Researchers decide which research questions to ask, which groups to study, how those groups should be divided, which variables to focus upon, and how best to categorize and measure such variables. The point is that researchers retain the ability to manipulate any study even as they decide what to study and how to study it.
Potential Misuses:
- Manipulating scale to change the appearance of the distribution of data
- Eliminating high/low scores for more coherent presentation
- Inappropriately focusing on certain variables to the exclusion of other variables
- Presenting correlation as causation
Measures Against Potential Misuses:
- Testing for reliability and validity
- Testing for statistical significance
- Critically reading statistics
Annotated Bibliography
Dear, K. (1997, August 28). SurfStat australia . Available: http://surfstat.newcastle.edu.au/surfstat/main/surfstat-main.html
A comprehensive site contain an online textbook, links together statistics sites, exercises, and a hotlist for Java applets.
de Leeuw, J. (1997, May 13 ). Statistics: The study of stability in variation . Available: http://www.stat.ucla.edu/textbook/ [1997, December 8].
An online textbook providing discussions specifically regarding variability.
Ewen, R.B. (1988). The workbook for introductory statistics for the behavioral sciences. Orlando, FL: Harcourt Brace Jovanovich.
A workbook providing sample problems typical of the statistical applications in social sciences.
Glass, G. (1996, August 26). COE 502: Introduction to quantitative methods . Available: http://seamonkey.ed.asu.edu/~gene/502/home.html
Outline of a basic statistics course in the college of education at Arizona State University, including a list of statistic resources on the Internet and access to online programs using forms and PERL to analyze data.
Hartwig, F., Dearing, B.E. (1979). Exploratory data analysis . Newberry Park, CA: Sage Publications, Inc.
Hayes, J. R., Young, R.E., Matchett, M.L., McCaffrey, M., Cochran, C., and Hajduk, T., eds. (1992). Reading empirical research studies: The rhetoric of research . Hillsdale, NJ: Lawrence Erlbaum Associates.
A text focusing on the language of research. Topics vary from "Communicating with Low-Literate Adults" to "Reporting on Journalists."
Hinkle, Dennis E., Wiersma, W. and Jurs, S.G. (1988). Applied statistics for the behavioral sciences . Boston: Houghton.
This is an introductory text book on statistics. Each of 22 chapters includes a summary, sample exercises and highlighted main points. The book also includes an index by subject.
Kleinbaum, David G., Kupper, L.L. and Muller K.E. Applied regression analysis and other multivariable methods 2nd ed . Boston: PWS-KENT Publishing Company.
An introductory text with emphasis on statistical analyses. Chapters contain exercises.
Kolstoe, R.H. (1969). Introduction to statistics for the behavioral sciences . Homewood, ILL: Dorsey.
Though more than 25-years-old, this textbook uses concise chapters to explain many essential statistical concepts. Information is organized in a simple and straightforward manner.
Levin, J., and James, A.F. (1991). Elementary statistics in social research, 5th ed . New York: HarperCollins.
This textbook presents statistics in three major sections: Description, From Description to Decision Making and Decision Making. The first chapter underlies reasons for using statistics in social research. Subsequent chapters detail the process of conducting and presenting statistics.
Liebetrau, A.M. (1983). Measures of association . Newberry Park, CA: Sage Publications, Inc.
Mendenhall, W.(1975). Introduction to probability and statistics, 4th ed. North Scltuate, MA: Duxbury Press.
An introductory textbook. A good overview of statistics. Includes clear definitions and exercises.
Moore, David S. (1979). Statistics: Concepts and controversies , 2nd ed . New York: W. H. Freeman and Company.
Introductory text. Basic overview of statistical concepts. Includes discussions of concrete applications such as opinion polls and Consumer Price Index.
Mosier, C.T. (1997). MG284 Statistics I - notes. Available: http://phoenix.som.clarkson.edu/~cmosier/statistics/main/outline/index.html
Explanations of fundamental statistical concepts.
Newton, H.J., Carrol, J.H., Wang, N., & Whiting, D.(1996, Fall). Statistics 30X class notes. Available: http://stat.tamu.edu/stat30x/trydouble2.html [1997, December 10].
This site contains a hyperlinked list of very comprehensive course notes from and introductory statistics class. A large variety of statistical concepts are covered.
Runyon, R.P., and Haber, A. (1976). Fundamentals of behavioral statistics , 3rd ed . Reading, MA: Addison-Wesley Publishing Company.
This is a textbook that divides statistics into categories of descriptive statistics and inferential statistics. It presents statistical procedures primarily through examples. This book includes sectional reviews, reviews of basic mathematics and also a glossary of symbols common to statistics.
Schoeninger, D.W. and Insko, C.A. (1971). Introductory statistics for the behavioral sciences . Boston: Allyn and Bacon, Inc.
An introductory text including discussions of correlation, probability, distribution, and variance. Includes statistical tables in the appendices.
Stevens, J. (1986). Applied multivariate statistics for the social sciences . Hillsdale, NJ: Lawrence Erlbaum Associates.
Stockberger, D. W. (1996). Introductory statistics: Concepts, models and applications . Available: http://www.psychstat.smsu.edu/ [1997, December 8].
Describes various statistical analyses. Includes statistical tables in the appendix.
Local Resources
If you are a member of the Colorado State University community and seek more in-depth help with analyzing data from your research (e.g., from an undergraduate or graduate research project), please contact CSU's Graybill Statistical Laboratory for statistical consulting assistance at http://www.stat.colostate.edu/statlab.html .
Jackson, Shawna, Karen Marcus, Cara McDonald, Timothy Wehner, & Mike Palmquist. (2005). Statistics: An Introduction. Writing@CSU . Colorado State University. https://writing.colostate.edu/guides/guide.cfm?guideid=67
Frontiers for Young Minds
- Download PDF
How Scientists Use Statistics, Samples, and Probability to Answer Research Questions
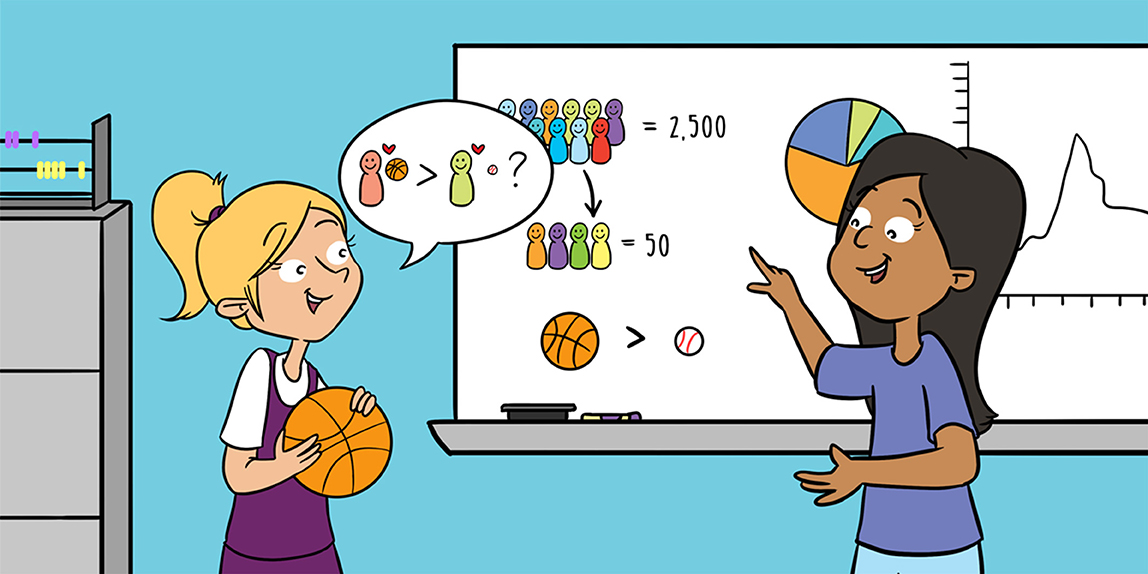
Studies show that the average person asks about 20 questions per day! Of course, some of these questions can be simple, like asking your teacher if you can use the bathroom, but some can be more complex and challenging to find an answer. That is where statistics comes in handy! Statistics allows us to draw conclusions from a set of data and is often called the “Science of Data.” It can also help people in every industry answer their research or business questions, and can help predict outcomes, such as what show you might want to watch next on your favorite video app. For social scientists like psychologists, statistics is a tool that helps us analyze data and answer our research questions.
Asking Research Questions
Scientists ask a variety of questions that can be answered with statistics. For example, a research psychologist might be interested in how test performance is affected by the amount of sleep a student gets the night before the test. Psychologists, biologists, and many other types of scientists are interested in answering questions about a population , or a group of individuals. For example, a biologist might be interested in studying a specific type of bird as a research population, a developmental psychologist might be interested in researching children ages 3–6 years old, or a clinical scientist might be interested in patients with a certain type of disease. The type of statistical analysis that should be conducted depends on the question asked and which variables are being measured. Variables are factors, traits, or conditions that can exist in different amounts or types, like height, age, or temperature.
Sampling From a Population
When answering our research questions, often it is not possible to collect information from everyone in the population we are interested in. For example, when seeing if sleep affects test performance, we cannot possibly collect sleep information and test scores from every student in the world! That is why we collect data from a sample of individuals that best represents the population. It is important that the characteristics of our sample are similar to the characteristics of the whole population. Social scientists make sure their samples have similar age or ethnic groups that are typical of the whole population. If we do not make sure that our samples have the same sort of characteristics that the overall population has, there could be problems answering our research questions Figure 1 .
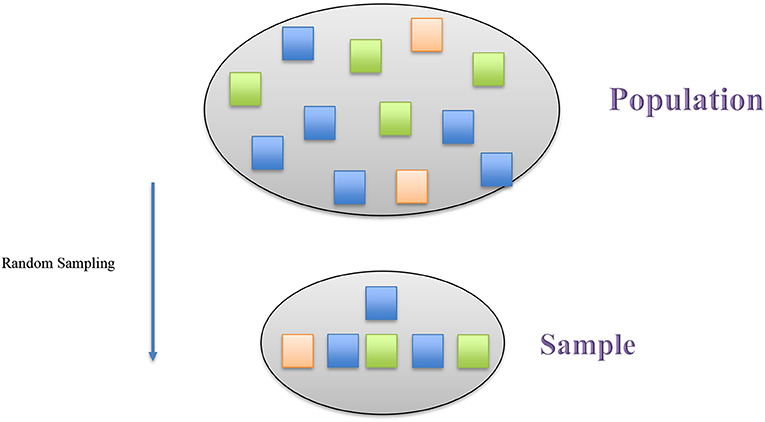
- Figure 1 - Random sampling is a way that a sample can be chosen so that it accurately represents the population.
- In random sampling, every individual in the population has an equal chance of being selected for the sample. In this example, each color within the population is also present in the sample, and the proportions of each color are represented in the sample, as well.
For example, let us say a veterinarian is interested in calculating the average weight of all dogs. She collects a sample of five dogs to weigh, finds the average weight of her sample and concludes that the average weight of all dogs is between 10 and 15 pounds. If you are a dog lover, you might suspect that something does not seem right with this number. Some dogs are rather large, so you may expect the average to be higher. What if the veterinarian collected a sample only of Chihuahuas? In that case, we certainly cannot say that all dogs weigh between 10 and 15 pounds; the sample contained only one breed and was not representative of all types of dogs. If the veterinarian had a sample that better represented the population of all dogs, the average weight she measured from the sample would likely be very different.
A key method of selecting individuals to be in a sample to best represent a population is called random sampling . Scientists use random sampling to guarantee that every individual in the population has an equal probability of being selected, and this ensures that the sample is most similar to the overall population.
Estimating From a Sample
Once the scientist gathers the sample, he or she might want to make conclusions about that sample and generalize the findings to the broader population. For example, a scientist might want to know the average number of hours of sleep 12-years old children get each night, or the average height of high schoolers in the US. In order to estimate the value of a variable in a population (like average height), scientists calculate a point estimate from the sample. A point estimate is a number that estimates the true value of a variable in a population, and often the point estimate is an average. For example, if we want to find out the average number of children per household in the city of Chicago, we would gather a random sample of families in Chicago and ask each family how many kids live in their house. Then, using this information, we could calculate the average number of children from these houses to calculate our point estimate. We can then assume that the average number of children in our sample is very similar to the average number of children in all Chicago households ( Figure 2 ).
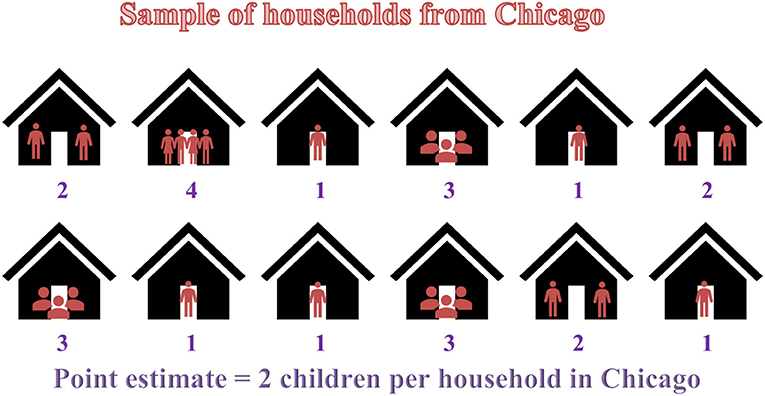
- Figure 2 - Rather than going to every house in Chicago to figure out the average number of children per home, scientists can take a sample.
- Here, the number of children from each household in the sample was collected and the sample mean was calculated. The scientist found that Chicago has an average of two children per household, which is called the point estimate.
Measurements and sampling methods can never be exact, so scientists often use confidence intervals around point estimates, to show a range of values that likely contain the true average of a variable in the population. In order to calculate the confidence interval, the scientist must first calculate the margin of error . The margin of error is a calculated amount that is added to and subtracted from a point estimate. It is a way of numerically representing miscalculations or errors in sampling from the population (such as when a sample is not totally representative of the population).
Let us practice calculating a confidence interval! Imagine we collect a sample of 49 students for a sleep study, and we find the average amount of sleep for the students is 10.5 h (our point estimate). Next, we need to figure out the standard deviation , which is the average distance between each person's data point and the total average. When the standard deviation is small, that means most data are close in value to the average, and a large standard deviation means the data are more spread out over more values. In our sample, let us say the standard deviation is 1.5 h. We then need to calculate the margin of error using this formula:
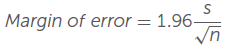
In this formula, s represents the standard deviation (1.5 h) and n refers to the number of data points in our sample (49 people). We replace the symbols with their corresponding values and calculate our margin of error to be 0.42 h of sleep. To complete the confidence intervals, we add and subtract the margin of error from our point estimate, to get the lower and upper bounds of the confidence interval. Psychologists typically use a 95% confidence interval to calculate the margin of error, which means that we can be confident that, 95% of the time, our confidence interval contains the actual population average. Our confidence interval for the point estimate in our example would be 10.5 ± 0.42 h, or 10.08 and 10.92. This means that, 95% of the time, the number of hours of sleep students in the overall population get is between 10.08 and 10.92 h ( Figure 3 ).
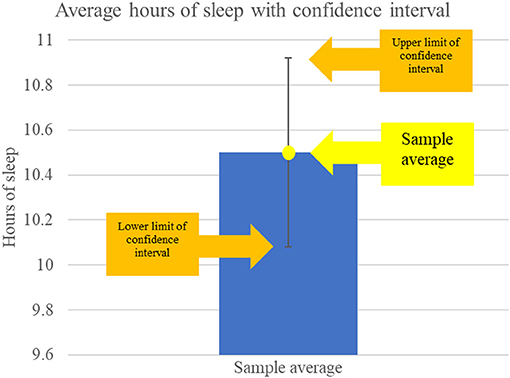
- Figure 3 - Confidence intervals show us a range of values that likely contain the true population value of a variable.
- This example shows the average hours of sleep in our sample (10.5 h). The bar shows the 95% confidence interval around the average, with 0.42 h of sleep added to and subtracted from the average to give the margin of error. The confidence interval shows that the true average hours of sleep students in the overall population get is somewhere between 10.08 and 10.92 h of sleep.
Scientists can reduce the margin of error in several ways to make their estimate of the population more precise. One way is to get more individuals in the sample, so that the sample is more representative of the population. Another way to reduce the margin of error is to make sure data collection is as error-free as possible to reduce variability in data, such as making sure all measurement tools (like scales, surveys, rulers, etc.) are accurate in what they are measuring. The more accurately the sample represents the population, by using random sampling and good data-collection practices, the smaller the margin of error and the more precise the confidence interval will be for estimating the true population value.
Asking More Complex Research Questions
Sometimes scientists want to go beyond describing simple calculations like average heights or age in their populations, to understanding aspects of their populations that are more complex. Let us say we are not only interested in seeing how much sleep students get, but we want to know how much test scores will drop after losing a few hours of sleep. Effect sizes are values that estimate the magnitude of a phenomenon, or the degree to which one variable (like hours of sleep) impacts another variable (like test scores). For example, if getting only 3 h of sleep lowers your test grade by a few points compared with when you get 9 h of sleep, you might not “lose sleep” about losing sleep. While there is a difference in the score, that is not a large difference. However, if after losing 6 h of sleep, you drop many points on a test, that could have a major impact on your grade. In this case, you would likely agree that the effect of losing sleep on your grades is an important one.
There are different ways to calculate effect size, depending on the research question and which type of statistics a scientist uses. Once a scientist calculates an effect size, he can determine if the effect is small, medium, or large. Effect sizes allow the scientist, as well as other people reviewing the findings, to better understand the effects certain variables have on other variables in the population.
Conclusions
Scientists ask many different types of questions, and there are many ways statistics can answer those questions. The examples of statistics we discussed in this article are ways that social scientists can answer simple questions from samples. But, statistics is not limited to any field or area of scientific research. Statistics has helped scientists know if medicines are likely to cure diseases, and has helped engineers understand the safety of the vehicle you ride in. It does not stop there; there are endless questions we can answer with statistics.
Population : ↑ An identified group of individuals that scientists want to answer questions about.
Variable : ↑ A factor, trait, or condition that exists in different amounts or types that is measured in research.
Random Sampling : ↑ A way of selecting individuals from a population that ensures that every individual has an equal probability of being selected.
Point Estimate : ↑ An estimate of some value in a population, such as an average.
Confidence Intervals : ↑ A range of values around point estimates that likely contain the true value of a variable in the population.
Margin of Error : ↑ A calculated amount added and subtracted to a point estimate, which is taken into account for miscalculation or errors.
Standard Deviation : ↑ The average distance between each data point and the total average.
Effect Size : ↑ Tells us how much difference there is between averages of variables.
Conflict of Interest Statement
The authors declare that the research was conducted in the absence of any commercial or financial relationships that could be construed as a potential conflict of interest.
Further Reading
Cumming, G. 2013. Understanding the New Statistics: Effect Sizes, Confidence Intervals, and Meta-Analysis . New York, NY: Routledge.
Have a language expert improve your writing
Run a free plagiarism check in 10 minutes, generate accurate citations for free.
- Knowledge Base
Methodology
Research Methods | Definitions, Types, Examples
Research methods are specific procedures for collecting and analyzing data. Developing your research methods is an integral part of your research design . When planning your methods, there are two key decisions you will make.
First, decide how you will collect data . Your methods depend on what type of data you need to answer your research question :
- Qualitative vs. quantitative : Will your data take the form of words or numbers?
- Primary vs. secondary : Will you collect original data yourself, or will you use data that has already been collected by someone else?
- Descriptive vs. experimental : Will you take measurements of something as it is, or will you perform an experiment?
Second, decide how you will analyze the data .
- For quantitative data, you can use statistical analysis methods to test relationships between variables.
- For qualitative data, you can use methods such as thematic analysis to interpret patterns and meanings in the data.
Table of contents
Methods for collecting data, examples of data collection methods, methods for analyzing data, examples of data analysis methods, other interesting articles, frequently asked questions about research methods.
Data is the information that you collect for the purposes of answering your research question . The type of data you need depends on the aims of your research.
Qualitative vs. quantitative data
Your choice of qualitative or quantitative data collection depends on the type of knowledge you want to develop.
For questions about ideas, experiences and meanings, or to study something that can’t be described numerically, collect qualitative data .
If you want to develop a more mechanistic understanding of a topic, or your research involves hypothesis testing , collect quantitative data .
Qualitative | to broader populations. . | |
---|---|---|
Quantitative | . |
You can also take a mixed methods approach , where you use both qualitative and quantitative research methods.
Primary vs. secondary research
Primary research is any original data that you collect yourself for the purposes of answering your research question (e.g. through surveys , observations and experiments ). Secondary research is data that has already been collected by other researchers (e.g. in a government census or previous scientific studies).
If you are exploring a novel research question, you’ll probably need to collect primary data . But if you want to synthesize existing knowledge, analyze historical trends, or identify patterns on a large scale, secondary data might be a better choice.
Primary | . | methods. |
---|---|---|
Secondary |
Descriptive vs. experimental data
In descriptive research , you collect data about your study subject without intervening. The validity of your research will depend on your sampling method .
In experimental research , you systematically intervene in a process and measure the outcome. The validity of your research will depend on your experimental design .
To conduct an experiment, you need to be able to vary your independent variable , precisely measure your dependent variable, and control for confounding variables . If it’s practically and ethically possible, this method is the best choice for answering questions about cause and effect.
Descriptive | . . | |
---|---|---|
Experimental |
Prevent plagiarism. Run a free check.
Research method | Primary or secondary? | Qualitative or quantitative? | When to use |
---|---|---|---|
Primary | Quantitative | To test cause-and-effect relationships. | |
Primary | Quantitative | To understand general characteristics of a population. | |
Interview/focus group | Primary | Qualitative | To gain more in-depth understanding of a topic. |
Observation | Primary | Either | To understand how something occurs in its natural setting. |
Secondary | Either | To situate your research in an existing body of work, or to evaluate trends within a research topic. | |
Either | Either | To gain an in-depth understanding of a specific group or context, or when you don’t have the resources for a large study. |
Your data analysis methods will depend on the type of data you collect and how you prepare it for analysis.
Data can often be analyzed both quantitatively and qualitatively. For example, survey responses could be analyzed qualitatively by studying the meanings of responses or quantitatively by studying the frequencies of responses.
Qualitative analysis methods
Qualitative analysis is used to understand words, ideas, and experiences. You can use it to interpret data that was collected:
- From open-ended surveys and interviews , literature reviews , case studies , ethnographies , and other sources that use text rather than numbers.
- Using non-probability sampling methods .
Qualitative analysis tends to be quite flexible and relies on the researcher’s judgement, so you have to reflect carefully on your choices and assumptions and be careful to avoid research bias .
Quantitative analysis methods
Quantitative analysis uses numbers and statistics to understand frequencies, averages and correlations (in descriptive studies) or cause-and-effect relationships (in experiments).
You can use quantitative analysis to interpret data that was collected either:
- During an experiment .
- Using probability sampling methods .
Because the data is collected and analyzed in a statistically valid way, the results of quantitative analysis can be easily standardized and shared among researchers.
Research method | Qualitative or quantitative? | When to use |
---|---|---|
Quantitative | To analyze data collected in a statistically valid manner (e.g. from experiments, surveys, and observations). | |
Meta-analysis | Quantitative | To statistically analyze the results of a large collection of studies. Can only be applied to studies that collected data in a statistically valid manner. |
Qualitative | To analyze data collected from interviews, , or textual sources. To understand general themes in the data and how they are communicated. | |
Either | To analyze large volumes of textual or visual data collected from surveys, literature reviews, or other sources. Can be quantitative (i.e. frequencies of words) or qualitative (i.e. meanings of words). |
If you want to know more about statistics , methodology , or research bias , make sure to check out some of our other articles with explanations and examples.
- Chi square test of independence
- Statistical power
- Descriptive statistics
- Degrees of freedom
- Pearson correlation
- Null hypothesis
- Double-blind study
- Case-control study
- Research ethics
- Data collection
- Hypothesis testing
- Structured interviews
Research bias
- Hawthorne effect
- Unconscious bias
- Recall bias
- Halo effect
- Self-serving bias
- Information bias
Quantitative research deals with numbers and statistics, while qualitative research deals with words and meanings.
Quantitative methods allow you to systematically measure variables and test hypotheses . Qualitative methods allow you to explore concepts and experiences in more detail.
In mixed methods research , you use both qualitative and quantitative data collection and analysis methods to answer your research question .
A sample is a subset of individuals from a larger population . Sampling means selecting the group that you will actually collect data from in your research. For example, if you are researching the opinions of students in your university, you could survey a sample of 100 students.
In statistics, sampling allows you to test a hypothesis about the characteristics of a population.
The research methods you use depend on the type of data you need to answer your research question .
- If you want to measure something or test a hypothesis , use quantitative methods . If you want to explore ideas, thoughts and meanings, use qualitative methods .
- If you want to analyze a large amount of readily-available data, use secondary data. If you want data specific to your purposes with control over how it is generated, collect primary data.
- If you want to establish cause-and-effect relationships between variables , use experimental methods. If you want to understand the characteristics of a research subject, use descriptive methods.
Methodology refers to the overarching strategy and rationale of your research project . It involves studying the methods used in your field and the theories or principles behind them, in order to develop an approach that matches your objectives.
Methods are the specific tools and procedures you use to collect and analyze data (for example, experiments, surveys , and statistical tests ).
In shorter scientific papers, where the aim is to report the findings of a specific study, you might simply describe what you did in a methods section .
In a longer or more complex research project, such as a thesis or dissertation , you will probably include a methodology section , where you explain your approach to answering the research questions and cite relevant sources to support your choice of methods.
Is this article helpful?
Other students also liked, writing strong research questions | criteria & examples.
- What Is a Research Design | Types, Guide & Examples
- Data Collection | Definition, Methods & Examples
More interesting articles
- Between-Subjects Design | Examples, Pros, & Cons
- Cluster Sampling | A Simple Step-by-Step Guide with Examples
- Confounding Variables | Definition, Examples & Controls
- Construct Validity | Definition, Types, & Examples
- Content Analysis | Guide, Methods & Examples
- Control Groups and Treatment Groups | Uses & Examples
- Control Variables | What Are They & Why Do They Matter?
- Correlation vs. Causation | Difference, Designs & Examples
- Correlational Research | When & How to Use
- Critical Discourse Analysis | Definition, Guide & Examples
- Cross-Sectional Study | Definition, Uses & Examples
- Descriptive Research | Definition, Types, Methods & Examples
- Ethical Considerations in Research | Types & Examples
- Explanatory and Response Variables | Definitions & Examples
- Explanatory Research | Definition, Guide, & Examples
- Exploratory Research | Definition, Guide, & Examples
- External Validity | Definition, Types, Threats & Examples
- Extraneous Variables | Examples, Types & Controls
- Guide to Experimental Design | Overview, Steps, & Examples
- How Do You Incorporate an Interview into a Dissertation? | Tips
- How to Do Thematic Analysis | Step-by-Step Guide & Examples
- How to Write a Literature Review | Guide, Examples, & Templates
- How to Write a Strong Hypothesis | Steps & Examples
- Inclusion and Exclusion Criteria | Examples & Definition
- Independent vs. Dependent Variables | Definition & Examples
- Inductive Reasoning | Types, Examples, Explanation
- Inductive vs. Deductive Research Approach | Steps & Examples
- Internal Validity in Research | Definition, Threats, & Examples
- Internal vs. External Validity | Understanding Differences & Threats
- Longitudinal Study | Definition, Approaches & Examples
- Mediator vs. Moderator Variables | Differences & Examples
- Mixed Methods Research | Definition, Guide & Examples
- Multistage Sampling | Introductory Guide & Examples
- Naturalistic Observation | Definition, Guide & Examples
- Operationalization | A Guide with Examples, Pros & Cons
- Population vs. Sample | Definitions, Differences & Examples
- Primary Research | Definition, Types, & Examples
- Qualitative vs. Quantitative Research | Differences, Examples & Methods
- Quasi-Experimental Design | Definition, Types & Examples
- Questionnaire Design | Methods, Question Types & Examples
- Random Assignment in Experiments | Introduction & Examples
- Random vs. Systematic Error | Definition & Examples
- Reliability vs. Validity in Research | Difference, Types and Examples
- Reproducibility vs Replicability | Difference & Examples
- Reproducibility vs. Replicability | Difference & Examples
- Sampling Methods | Types, Techniques & Examples
- Semi-Structured Interview | Definition, Guide & Examples
- Simple Random Sampling | Definition, Steps & Examples
- Single, Double, & Triple Blind Study | Definition & Examples
- Stratified Sampling | Definition, Guide & Examples
- Structured Interview | Definition, Guide & Examples
- Survey Research | Definition, Examples & Methods
- Systematic Review | Definition, Example, & Guide
- Systematic Sampling | A Step-by-Step Guide with Examples
- Textual Analysis | Guide, 3 Approaches & Examples
- The 4 Types of Reliability in Research | Definitions & Examples
- The 4 Types of Validity in Research | Definitions & Examples
- Transcribing an Interview | 5 Steps & Transcription Software
- Triangulation in Research | Guide, Types, Examples
- Types of Interviews in Research | Guide & Examples
- Types of Research Designs Compared | Guide & Examples
- Types of Variables in Research & Statistics | Examples
- Unstructured Interview | Definition, Guide & Examples
- What Is a Case Study? | Definition, Examples & Methods
- What Is a Case-Control Study? | Definition & Examples
- What Is a Cohort Study? | Definition & Examples
- What Is a Conceptual Framework? | Tips & Examples
- What Is a Controlled Experiment? | Definitions & Examples
- What Is a Double-Barreled Question?
- What Is a Focus Group? | Step-by-Step Guide & Examples
- What Is a Likert Scale? | Guide & Examples
- What Is a Prospective Cohort Study? | Definition & Examples
- What Is a Retrospective Cohort Study? | Definition & Examples
- What Is Action Research? | Definition & Examples
- What Is an Observational Study? | Guide & Examples
- What Is Concurrent Validity? | Definition & Examples
- What Is Content Validity? | Definition & Examples
- What Is Convenience Sampling? | Definition & Examples
- What Is Convergent Validity? | Definition & Examples
- What Is Criterion Validity? | Definition & Examples
- What Is Data Cleansing? | Definition, Guide & Examples
- What Is Deductive Reasoning? | Explanation & Examples
- What Is Discriminant Validity? | Definition & Example
- What Is Ecological Validity? | Definition & Examples
- What Is Ethnography? | Definition, Guide & Examples
- What Is Face Validity? | Guide, Definition & Examples
- What Is Non-Probability Sampling? | Types & Examples
- What Is Participant Observation? | Definition & Examples
- What Is Peer Review? | Types & Examples
- What Is Predictive Validity? | Examples & Definition
- What Is Probability Sampling? | Types & Examples
- What Is Purposive Sampling? | Definition & Examples
- What Is Qualitative Observation? | Definition & Examples
- What Is Qualitative Research? | Methods & Examples
- What Is Quantitative Observation? | Definition & Examples
- What Is Quantitative Research? | Definition, Uses & Methods
"I thought AI Proofreading was useless but.."
I've been using Scribbr for years now and I know it's a service that won't disappoint. It does a good job spotting mistakes”
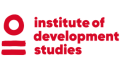
How to generate statistics and influence policy using participatory methods in research : reflections on work in Malawi, 1999-2002
Ids item types, copyright holder, usage metrics.
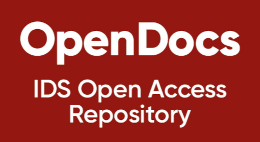
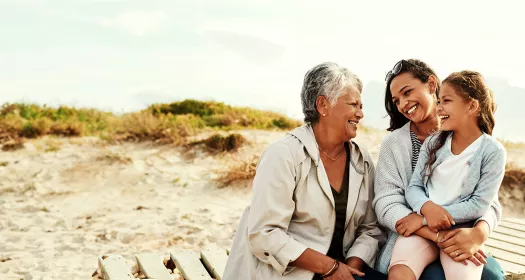
Research Scientist, Comparative Medicine- Research Collaborations
About the role.
Your Key Responsibilities:
- Perform state-of-the-art animal experimentation with the highest ethical and technical standards, setting up and animal models independently or through the team.
- Able to perform advanced, intermediate, and basic technical skills (compound administration by PO, IV, SC, IP, etc. routes, blood sample collection via different routes (ex: tail snip/vein, saphenous, abdominal aorta, vena cava, etc.). Tissue collection, clinical observation, necropsy etc.) on applicable in-house species (Mouse, Rat, Rabbit)
- Participate in scientific discussions, performing critical review of experimental protocols and identifying the best approach for in-vivo studies according to project needs.
- Continuously evaluate, and train on newest techniques and build scientific knowledge to ensure state-of-the-art in-vivo work.
- Closely interact with scientific customer base, providing optimal support, ability to build up a robust professional network and generate high quality data.
- Interest in data interpretation, statistics, and presentation, to propose next steps to customer.
- Be an excellent team player and show potential to operate within the Team to ensure appropriate performance, training, and professional development within the Team.
- Rota, weekend duties, early morning, or late evening dosing
- Routinely responsible for ensuring own compliance with all institutional and regulatory protocols, policies, and guidelines.
- Responsible for pursuing continuous learning/professional development opportunities, enhancing/expanding skill set, and reviewing CM techniques.
Role requirements:
- BS or Master’s in Life Sciences or equivalent experience
- Experience in a technical skills tract laboratory animal program or 3+ years preferred.
- Strong commitment to animal welfare, research support, and high quality in-vivo focused science
- Theoretical and practical expertise in animal experimentation with focus on small animals.
- Hands on expertise with multiple animal disease and mechanistic models
- High ethical approach, committed to high standards of animal welfare.
- Strong customer-orientation, used to communicate with a broad range of stakeholders, strong aptitudes as a team player.
- Fluency in English.
- Must be able to lift 50 lbs., be able to work from a standing position for prolonged periods of time and perform repetitive motion tasks.
Schedule: full time, shifted schedule
Includes either Saturday or Sunday
Some weekend on call, BR holiday coverage required.
Commitment to Diversity & Inclusion: The Novartis Group of Companies are Equal Opportunity Employers and take pride in maintaining a diverse environment. We do not discriminate in recruitment, hiring, training, promotion or other employment practices for reasons of race, color, religion, gender, national origin, age, sexual orientation, gender identity or expression, marital or veteran status, disability, or any other legally protected status. We are committed to building diverse teams, representative of the patients and communities we serve, and we strive to create an inclusive workplace that cultivates bold innovation through collaboration and empowers our people to unleash their full potential.
Novartis Compensation and Benefit Summary: The pay range for this position at commencement of employment is expected to be between $39.13- $58.72/year ; however, w hile salary ranges are effective from 1/1/24 through 12/31/24, fluctuations in the job market may necessitate adjustments to pay ranges during this period. Further, final pay determinations will depend on various factors, including, but not limited to geographical location, experience level, knowledge, skills, and abilities. The total compensation package for this position may also include other elements, including a sign-on bonus, restricted stock units, and discretionary awards in addition to a full range of medical, financial, and/or other benefits (including 401(k) eligibility and various paid time off benefits, such as vacation, sick time, and parental leave), dependent on the position offered. Details of participation in these benefit plans will be provided if an employee receives an offer of employment. If hired, employee will be in an “at-will position” and the Company reserves the right to modify base salary (as well as any other discretionary payment or compensation program) at any time, including for reasons related to individual performance, Company or individual department/team performance, and market factors.
Why Novartis: Helping people with disease and their families takes more than innovative science. It takes a community of smart, passionate people like you. Collaborating, supporting and inspiring each other. Combining to achieve breakthroughs that change patients’ lives. Ready to create a brighter future together? https://www.novartis.com/about/strategy/people-and-culture
Join our Novartis Network: Not the right Novartis role for you? Sign up to our talent community to stay connected and learn about suitable career opportunities as soon as they come up: https://talentnetwork.novartis.com/network
Benefits and Rewards: Read our handbook to learn about all the ways we’ll help you thrive personally and professionally: https://www.novartis.com/careers/benefits-rewards
EEO Statement:
The Novartis Group of Companies are Equal Opportunity Employers who are focused on building and advancing a culture of inclusion that values and celebrates individual differences, uniqueness, backgrounds and perspectives. We do not discriminate in recruitment, hiring, training, promotion or other employment practices for reasons of race, color, religion, sex, national origin, age, sexual orientation, gender identity or expression, marital or veteran status, disability, or any other legally protected status. We are committed to fostering a diverse and inclusive workplace that reflects the world around us and connects us to the patients, customers and communities we serve.
Accessibility & Reasonable Accommodations
The Novartis Group of Companies are committed to working with and providing reasonable accommodation to individuals with disabilities. If, because of a medical condition or disability, you need a reasonable accommodation for any part of the application process, or to perform the essential functions of a position, please send an e-mail to [email protected] or call +1(877)395-2339 and let us know the nature of your request and your contact information. Please include the job requisition number in your message.
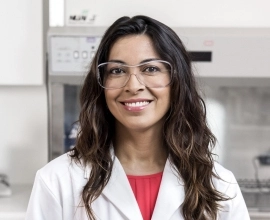

An official website of the United States government
The .gov means it’s official. Federal government websites often end in .gov or .mil. Before sharing sensitive information, make sure you’re on a federal government site.
The site is secure. The https:// ensures that you are connecting to the official website and that any information you provide is encrypted and transmitted securely.
- Publications
- Account settings
Preview improvements coming to the PMC website in October 2024. Learn More or Try it out now .
- Advanced Search
- Journal List
- Transl Med UniSa
- v.5; Jan-Apr 2013
Statistics and Medicine: the Indispensable Know-How of the Researcher
1 Department of Medicine, University of Salerno
2 Department of UTTP-FOS, ENEA
Statistics has often been misunderstood in Medicine, but it is indispensable knowledge both for the experimenter and the reader. Statistical methods allow to study diseases, patients, and epidemiological events. The modern researcher cannot refuse to know and to use statistics. A deeper understanding is required to prepare a research project and to avoid colossal mistakes of misleading.
The aim of this paper is to provide an organized and structured point of view on the use of statistics in Medicine and Research, showing the principal resources to organize a scientific study from the declaration of a hypothesis to the report of the results.
“Statistical thinking will one day be as necessary for efficient citizenship as the ability to read or write.” H. G. Wells
I. INTRODUCTION
Statistics represents the heel of Achilles for the modern researcher. The necessity to apply statistics to any scientific problem leads the researcher to assume the burden of a deeper knowledge in this science.
Medicine often uses probabilistic statistics that could be far away from the scientific method. Data are used and analysed in order to highlight trends or to make a prevision for the validity of a diagnostic method, a therapy or a prognosis for a disease. When an event is pointed out in a large amount of cases, a descriptive method is used to identify the potential basic mechanism. Starting from this point, the aim of the researcher should be the precise and reasoned planning of a scientific project. Any aspect of a research project should be provided at the beginning and then verified.
The data from the study of a subset of the population, the sample, are collected and then subjected to statistical analysis, in order to attend to two purposes: description and inference.
Descriptive statistics uses data to describe numerically and graphically the observations in the sample. Instead, inferential statistics uses the identification of patterns in data to draw inferences about the population.
Statistical analysis might identify the presence of a relationship between variables.
The need for greater knowledge in statistics is crucial both for the researcher, who is planning a study or elaborating data from a study, and for readers to understand the plan of a study and the validity of its conclusion.
II. STATISTICAL METHODS
A) experimental and observational studies.
The use of statistical studies allows to evaluate the causality and to draw a conclusion about the effect of an independent variable on the dependent variables examined. We consider two types of statistical study: experimental and observational ( Table 1 ). They differ in how studies are conducted and in their aims [ 1 , 2 ].
Classification of observational and experimental studies.
CLASSIFICATION OF EPIDEMIOLOGIC STUDIES | |
---|---|
Observational Studies | Cohort |
Cross-sectional | |
Case- Control | |
Ecological | |
Experimental Studies | Preventing Trial |
Clinical Trial |
An observational study evaluates the inferences about the effect of a treatment on patients, when the option of giving a patient to a treated group versus a control group is not an option for the investigator. Data are already available, researchers can explore any similarities among them. So, it is characterized by the observation of the interaction between two or more variables: the independent variable (or the risk factor) and, on the other hand, the dependent variables (or the response). This type of study might be prospective (with the evaluation of the relative risk) or retro-prospective (with the evaluation of the odds ratio). It is essential to consider the presence of confounding variables that influenced the relation between the risk factor and the response causing a bias. This study might be preliminary to an experimental study, the starting point to plan a research.
The experimental model is fundamental for the scientific model: after the observation of a phenomenon, the hypothesis is declared and then verified. Nowadays, planning a research project and identifying a hypothesis clearly, it is necessary to check its innovation. Only after that the researcher can design and perform the experiment and at the end evaluate the validity of the hypothesis.
The substantial difference between the observational and experimental study is in the role of the researcher. In an experimental study researchers are involved in taking measurements, manipulating the system, and then taking again measurements using the same procedure in order to understand the impact of the manipulation on the system. Instead, in an observational study there is no experimental manipulation but only the data collection and their analysis.
Designing an experimental study, the researcher needs to:
- consider the initial information about the entity of the effects, the alternative hypothesis and the experimental variability;
- plan the research and design experiments, choosing the quantity to measure, the independent and the dependent variables, the method of measurement, the acceptable error of measure, the variability range, the acceptable influence of confounding variables;
- perform the experiment and measurement;
- analyse data following strict determined before;
- present the results of the study.
Planning a study the researcher decides to support an “hypothesis”, a conjecture about the variables taken into account in a population. This hypothesis can be verified with statistical method, e.g. confirmatory tests and χ 2 test.
B) The choice between parametric and non-parametric methods
A parametric model is a distribution that can be described using a finite number of parameters. Many basic statistical methods are parametric. The use of parametric methods leads to make more assumptions than using non-parametric methods. If these assumptions are correct, parametric methods can be accurate and precise.
The necessity to make assumptions is a risk, because if they are wrong, the parametric method will be misleading. This is the reason why they are considered statistical powerful, but not robust.
In addition to parametric models, there are also semi-parametric, semi-nonparametric, and non-parametric models. A non-parametric model is a model where all parameters are in infinite-dimensional parameter spaces. It considers data belonging to any particular distribution or techniques.
The use of non-parametric methods may be indispensable when data are characterized by a ranking without an useful numerical interpretation. Non-parametric methods make fewer assumptions than parametric methods. So they can be applied widely and be considered robust. Nonparametric tests have less power where a parametric test would be appropriate [ 3 ].
C) The evaluation and control of the error
The choice of a sample might be extremely difficult. The sample should be representative of an entire population to be used as a guide. The use of a representative sample assures that the inferences and conclusions can be safely extended from the sample to all the population. A crucial problem is in determining the adequate size of the sample. The sample should be large enough to be representative, but it does not need to be oversize to reduce the costs of the study.
There are two large groups of errors:
- the error due to measurement instrumentation and to the operator (for example, systematic error, sensitivity error). It is a controllable but often unavoidable error, due to a discretional evaluation of the measurement. In the medical field, much emphasis is given to error “bias” is an error of this kind due to experimenter’s choices.
- the statistical error, that is always present when there is a measurement, has many causes, even inherent to the experiment itself. This error can be reduced increasing the number of measurements, but it cannot be eliminated. It is the measure of the amplitude of data sheet dispersion. The normal distribution is frequently used to describe random variables to real value. It is considered the most prominent distribution in statistics because it is a distribution that can be used for a large number of random variables. It is commonly used in practice as a simple model for complex phenomena both in natural science and in social science. The error in an experiment is usually assumed to follow a normal distribution. This assumption is used to calculate the propagation of uncertainty. When the distribution is a Gaussian distribution the error α can be calculate as (1) Δ x = ∑ ( x i − μ ) 2 ( n − 1 ) (1) where n is the sample size and μ is the mean of the distribution [ 3 ].
Many of the errors derived from the measurements are classified as “noise” if they are random. They can lead to statistic misleading. Misuse of statistics can produce subtle, but serious errors in description and interpretation. Even experienced professionals make such errors, but they can lead to enormous decision errors.
D) Significance levels
Statistics offers some instruments to understand if the results of the measurements is that expected by the hypothesis. One of these is the significance test.
A significance test evaluates the plausibility of the observed data when a “null hypothesis” is true. It is expressed by a significance level α that is the probability of rejecting a null hypothesis that is true [ 3 ].
In the interpretation of the statistical information, it is possible to evaluate the null hypothesis wrongly.
As reported in Table 2 , there are two types of error:
- Type I error or α, when the null hypothesis is rejected, but it is true. It is a “false positive”.
- Type II error or β, when the null hypothesis is false, but it is not rejected. It is a “false negative”.
The null hypothesis and the possible errors.
H | H false | |
H non rejected | True positive | Error β |
H rejected | Error α | True negative |
Rejecting a true null hypothesis is an error that should be as small as possible. It is necessary to choose the smallest value: 0.01, 0.05 or 0.001. This significance level is named P value. It is used to express the chance that the observed data are due to chance. The relevance of the chosen P value might depend on the sample size. Furthermore, reporting results it is important to report the real P value.
It is difficult to choose a significance level, but it is extremely difficult interpreting results using the P value. If P value of observed data is small enough (P< 0.05 or P< 0.01 or P< 0.001), the null hypothesis could be rejected, and the alternative hypothesis could be accepted. But if P value is not small enough, there is no statistical significance. The null hypothesis cannot be rejected, but it does not mean anything else.
E) Survival statistics
Survival statistics is a branch of statistics that deals with death in a biological organism. Time is a fundamental character of this branch and the main “events” are death and failure. Survival models can be usefully considered as ordinary regression models in which time is the response variable.
Among the estimators used to estimating survival data, the Kaplan–Meier estimator is extremely important. In the medical literature, it is frequently used to measure the rate of patients living after a therapy or after the exposition to a risk factor. A plot of the Kaplan–Meier estimate of patients’ survival is made by horizontal steps of declining magnitude. The value of the survival function is constant between clicks that are successive distinct sampled observations.
Among survival analysis, it is necessary to consider the survival rate. It is percentage of people alive after 1, 5 or 10 years after the diagnosis of disease. It can be expressed as relative survival, cause specific survival, disease specific survival. The interval considered can be one, five, and ten years. Survival rates can be used to compare the effectiveness of treatments and to evaluated the prognosis of patients [ 1 ].
III. REPORTING STATISTICAL RESULTS
Planning and reporting an observational or an experimental study it is important to declare the aim of the study, the statistical method that will be used and the endpoints. The characteristics of subjects and of the planning should be clearly expressed. It is important if the study is blinding (masking). The endpoints are the events that will be considered. It is possible to differentiate primary and secondary endpoints. Examples of endpoints can be death (in a study survival rate), adverse events and morbidity, toxicity, maximum tolerated dose, response to a therapy. Every adverse event should be reported.
The software used for analysing data should be reported, e.g. SPSS or SAS. So, statistical tests should be described. There should be the type of statistical test, if it is a confirmatory analysis or if it is an exploratory test that need to be strengthen with cross validation [ 1 , 4 ].
Criteria of inclusion or exclusion are indispensable to clarify the validity of interpretation of the data and the extent to which the results can be applied.
Data should be enlighten and so their sources [ 4 ].
Reporting results, any exclusion during analysis should be expressed. Data analysis should be reported with appropriate confidence intervals or P value [ 3 , 4 ].
The interpretation of the P value is disputable. The presence of a P value less than a predefined value determines statistical significance, but its absence is not a reason to reject the alternative hypothesis. Statistical significance does not mean equivalence of treatment or techniques because the clinical importance of a result depends on several variables. Careful statisticians distinguish between statistical and medical significance.
To avoid problems in the interpretation of analysis, it is necessary to choose easy endpoints, to avoid using the term significant, to use correction for data when it is due, e.g. the correction of Bonferroni.
Results and data should be reported in a clear and not confused way, using tables and graphs. The estimation of a valuable and important relation among data is facilitated using graphs.
Graphs give a visual perception of results and catch attention quickly. Understanding of the context is immediate if a graph is well-organized. A graph has two component: content and format. The content is what is reported in a graph while the format is the way that it is reported. It is fundamental to avoid the overlapping of data symbols. If the graph requires the use of many lines, choose patterns that are distinguished easily from each other [ 5 ].
If visualizing distributions is essential, show the distribution of data with scatter plots or one-way plots, but avoid graphs showing summaries of data such as bar graphs showing means with standard deviation. Good work is a work well reported.

IMAGES
COMMENTS
Introduction. Statistical analysis is necessary for any research project seeking to make quantitative conclusions. The following is a primer for research-based statistical analysis. It is intended to be a high-level overview of appropriate statistical testing, while not diving too deep into any specific methodology.
Table of contents. Step 1: Write your hypotheses and plan your research design. Step 2: Collect data from a sample. Step 3: Summarize your data with descriptive statistics. Step 4: Test hypotheses or make estimates with inferential statistics.
In the field of research, statistics is important for the following reasons: Reason 1: Statistics allows researchers to design studies such that the findings from the studies can be extrapolated to a larger population. Reason 2: Statistics allows researchers to perform hypothesis tests to determine if some claim about a new drug, new procedure ...
Statistics is the application of mathematical concepts to understanding and analysing large collections of data. A central tenet of statistics is to describe the variations in a data set or ...
Table 1 summarises commonly used descriptive statistics. For consistency, I use the terms independent variable and dependent variable, but in some fields and types of research such as correlational studies the preferred terms may be predictor and outcome variable. An independent variable influences, affects or predicts a dependent variable.
Abstract. Statistical methods involved in carrying out a study include planning, designing, collecting data, analysing, drawing meaningful interpretation and reporting of the research findings. The statistical analysis gives meaning to the meaningless numbers, thereby breathing life into a lifeless data. The results and inferences are precise ...
JAMA. Review. December 12, 2022. This Guide to Statistics and Methods describes the use of target trial emulation to design an observational study so it preserves the advantages of a randomized clinical trial, points out the limitations of the method, and provides an example of its use. Research, Methods, Statistics.
Quantitative research methods. You can use quantitative research methods for descriptive, correlational or experimental research. In descriptive research, you simply seek an overall summary of your study variables.; In correlational research, you investigate relationships between your study variables.; In experimental research, you systematically examine whether there is a cause-and-effect ...
Read the latest Research articles in Statistics from Scientific Reports. ... Employees' pro-environmental behavior in an organization: a case study in the UAE. Nadin Alherimi, Zeki Marva
Let's go through the top 9 best statistical tools used in research below: 1. SPSS: SPSS (Statistical Package for the Social Sciences) is a collection of software tools compiled as a single package. This program's primary function is to analyze scientific data in social science. This information can be utilized for market research, surveys ...
Descriptive statistics are specific methods basically used to calculate, describe, and summarize collected research data in a logical, meaningful, and efficient way. Descriptive statistics are reported numerically in the manuscript text and/or in its tables, or graphically in its figures. This basic …
Example: Inferential statistics. You randomly select a sample of 11th graders in your state and collect data on their SAT scores and other characteristics. You can use inferential statistics to make estimates and test hypotheses about the whole population of 11th graders in the state based on your sample data.
However, statistics is more complex a field of study that defines and explains study patterns based on the sample sizes used. To be precise, statistics provides a trend in the conducted study. Biological researchers often disregard the use of statistics in their research planning, and mainly use statistical tools at the end of their experiment.
The textbook covers all necessary areas and topics for students who want to conduct research in statistics. It includes foundational concepts, application methods, and advanced statistical techniques relevant to research methodologies. ... Also, the modular structure allows instructors and students to adapt the materials for different study ...
The Purpose of Statistics Is to Clarify. It sometimes seems as if researchers use statistics as a kind of secret language. In this sense, statistics provide a way for the initiated to share ideas and concepts without including the rest of us. Of course, it is necessary to use a common language to report research results.
In a previous article in this series, we looked at different types of data and ways to summarise them. 1 At the end of the research study, statistical analyses are performed to test the hypothesis and either prove or disprove it. The choice of statistical test needs to be carefully performed since the use of incorrect tests could lead to misleading conclusions.
Understanding and Using Statistical Methods. Statistics is a set of tools used to organize and analyze data. Data must either be numeric in origin or transformed by researchers into numbers. For instance, statistics could be used to analyze percentage scores English students receive on a grammar test: the percentage scores ranging from 0 to 100 ...
Types of descriptive statistics. There are 3 main types of descriptive statistics: The distribution concerns the frequency of each value. The central tendency concerns the averages of the values. The variability or dispersion concerns how spread out the values are. You can apply these to assess only one variable at a time, in univariate ...
Studies show that the average person asks about 20 questions per day! Of course, some of these questions can be simple, like asking your teacher if you can use the bathroom, but some can be more complex and challenging to find an answer. That is where statistics comes in handy! Statistics allows us to draw conclusions from a set of data and is often called the "Science of Data." It can ...
1. Describe the application of the following statistical areas to educational interventions: study designs, variable types, exploratory data analysis, confirmatory (inferential) data analysis, and basic interpretation of results. 2. Use a four-step approach to choosing a statistical test for educational cases. Go to:
This study explored relationships between social media use (active and passive), body image, and drive for muscularity in physically active men. A questionnaire containing measures of body image (appearance valence, appearance salience), drive for muscularity, and social media use was completed by 224 men aged 18-50 years.
Quantitative analysis uses numbers and statistics to understand frequencies, averages and correlations (in descriptive studies) or cause-and-effect relationships (in experiments). You can use quantitative analysis to interpret data that was collected either: During an experiment. Using probability sampling methods.
In Malawi in 1999-2002 we conducted research studies using participatory methods to generate population estimates; estimates of the proportion of people in a population with certain characteristics (e.g. the very food insecure); and estimates of the proportion of people in a population that should be targeted by an intervention. We claim that the statistics generated in these studies are at ...
Research Collaborations (RC) is a centralized core unit within In vivo Science & Technology (IST), Comparative Medicine (CM). RC is responsible for performing best-in-class in-vivo studies together with developing key animal models and cutting-edge technologies needed for Biomedical Research (BR) drug discovery and development process.
Statistics has often been misunderstood in Medicine, but it is indispensable knowledge both for the experimenter and the reader. Statistical methods allow to study diseases, patients, and epidemiological events. The modern researcher cannot refuse to know and to use statistics. A deeper understanding is required to prepare a research project ...