
Statistical Papers
Statistical Papers is a forum for presentation and critical assessment of statistical methods encouraging the discussion of methodological foundations and potential applications.
- The Journal stresses statistical methods that have broad applications, giving special attention to those relevant to the economic and social sciences.
- Covers all topics of modern data science, such as frequentist and Bayesian design and inference as well as statistical learning.
- Contains original research papers (regular articles), survey articles, short communications, reports on statistical software, and book reviews.
- High author satisfaction with 90% likely to publish in the journal again.
- Werner G. Müller,
- Carsten Jentsch,
- Shuangzhe Liu,
- Ulrike Schneider
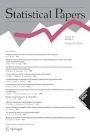
Latest issue
Volume 65, Issue 6
Latest articles
Confidence intervals for overall response rate difference in the sequential parallel comparison design.
- Guogen Shan
- Samuel S. Wu
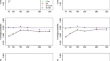
Bayesian and frequentist inference derived from the maximum entropy principle with applications to propagating uncertainty about statistical methods
- David R. Bickel
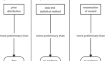
Reduced bias estimation of the log odds ratio
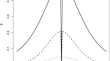
A critical note on the exponentiated EWMA chart
- William H. Woodall
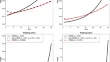
Hadamard matrices, quaternions, and the Pearson chi-square statistic
- Abbas Alhakim
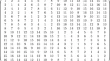
Journal updates
Write & submit: overleaf latex template.
Overleaf LaTeX Template
Journal information
- Australian Business Deans Council (ABDC) Journal Quality List
- Current Index to Statistics
- Google Scholar
- Japanese Science and Technology Agency (JST)
- Mathematical Reviews
- Norwegian Register for Scientific Journals and Series
- OCLC WorldCat Discovery Service
- Research Papers in Economics (RePEc)
- Science Citation Index Expanded (SCIE)
- TD Net Discovery Service
- UGC-CARE List (India)
Rights and permissions
Editorial policies
© Springer-Verlag GmbH Germany, part of Springer Nature
- Find a journal
- Publish with us
- Track your research

An official website of the United States government
The .gov means it’s official. Federal government websites often end in .gov or .mil. Before sharing sensitive information, make sure you’re on a federal government site.
The site is secure. The https:// ensures that you are connecting to the official website and that any information you provide is encrypted and transmitted securely.
- Publications
- Account settings
- My Bibliography
- Collections
- Citation manager
Save citation to file
Email citation, add to collections.
- Create a new collection
- Add to an existing collection
Add to My Bibliography
Your saved search, create a file for external citation management software, your rss feed.
- Search in PubMed
- Search in NLM Catalog
- Add to Search
Comprehensive guidelines for appropriate statistical analysis methods in research
Affiliations.
- 1 Department of Anesthesiology and Pain Medicine, Daegu Catholic University School of Medicine, Daegu, Korea.
- 2 Department of Medical Statistics, Daegu Catholic University School of Medicine, Daegu, Korea.
- PMID: 39210669
- DOI: 10.4097/kja.24016
Background: The selection of statistical analysis methods in research is a critical and nuanced task that requires a scientific and rational approach. Aligning the chosen method with the specifics of the research design and hypothesis is paramount, as it can significantly impact the reliability and quality of the research outcomes.
Methods: This study explores a comprehensive guideline for systematically choosing appropriate statistical analysis methods, with a particular focus on the statistical hypothesis testing stage and categorization of variables. By providing a detailed examination of these aspects, this study aims to provide researchers with a solid foundation for informed methodological decision making. Moving beyond theoretical considerations, this study delves into the practical realm by examining the null and alternative hypotheses tailored to specific statistical methods of analysis. The dynamic relationship between these hypotheses and statistical methods is thoroughly explored, and a carefully crafted flowchart for selecting the statistical analysis method is proposed.
Results: Based on the flowchart, we examined whether exemplary research papers appropriately used statistical methods that align with the variables chosen and hypotheses built for the research. This iterative process ensures the adaptability and relevance of this flowchart across diverse research contexts, contributing to both theoretical insights and tangible tools for methodological decision-making.
Conclusions: This study emphasizes the importance of a scientific and rational approach for the selection of statistical analysis methods. By providing comprehensive guidelines, insights into the null and alternative hypotheses, and a practical flowchart, this study aims to empower researchers and enhance the overall quality and reliability of scientific studies.
Keywords: Algorithms; Biostatistics; Data analysis; Guideline; Statistical data interpretation; Statistical model..
PubMed Disclaimer
- Citation Manager
NCBI Literature Resources
MeSH PMC Bookshelf Disclaimer
The PubMed wordmark and PubMed logo are registered trademarks of the U.S. Department of Health and Human Services (HHS). Unauthorized use of these marks is strictly prohibited.

Statistical Analysis in Research: Meaning, Methods and Types
Home » Videos » Statistical Analysis in Research: Meaning, Methods and Types
The scientific method is an empirical approach to acquiring new knowledge by making skeptical observations and analyses to develop a meaningful interpretation. It is the basis of research and the primary pillar of modern science. Researchers seek to understand the relationships between factors associated with the phenomena of interest. In some cases, research works with vast chunks of data, making it difficult to observe or manipulate each data point. As a result, statistical analysis in research becomes a means of evaluating relationships and interconnections between variables with tools and analytical techniques for working with large data. Since researchers use statistical power analysis to assess the probability of finding an effect in such an investigation, the method is relatively accurate. Hence, statistical analysis in research eases analytical methods by focusing on the quantifiable aspects of phenomena.
What is Statistical Analysis in Research? A Simplified Definition
Statistical analysis uses quantitative data to investigate patterns, relationships, and patterns to understand real-life and simulated phenomena. The approach is a key analytical tool in various fields, including academia, business, government, and science in general. This statistical analysis in research definition implies that the primary focus of the scientific method is quantitative research. Notably, the investigator targets the constructs developed from general concepts as the researchers can quantify their hypotheses and present their findings in simple statistics.
When a business needs to learn how to improve its product, they collect statistical data about the production line and customer satisfaction. Qualitative data is valuable and often identifies the most common themes in the stakeholders’ responses. On the other hand, the quantitative data creates a level of importance, comparing the themes based on their criticality to the affected persons. For instance, descriptive statistics highlight tendency, frequency, variation, and position information. While the mean shows the average number of respondents who value a certain aspect, the variance indicates the accuracy of the data. In any case, statistical analysis creates simplified concepts used to understand the phenomenon under investigation. It is also a key component in academia as the primary approach to data representation, especially in research projects, term papers and dissertations.
Most Useful Statistical Analysis Methods in Research
Using statistical analysis methods in research is inevitable, especially in academic assignments, projects, and term papers. It’s always advisable to seek assistance from your professor or you can try research paper writing by CustomWritings before you start your academic project or write statistical analysis in research paper. Consulting an expert when developing a topic for your thesis or short mid-term assignment increases your chances of getting a better grade. Most importantly, it improves your understanding of research methods with insights on how to enhance the originality and quality of personalized essays. Professional writers can also help select the most suitable statistical analysis method for your thesis, influencing the choice of data and type of study.
Descriptive Statistics
Descriptive statistics is a statistical method summarizing quantitative figures to understand critical details about the sample and population. A description statistic is a figure that quantifies a specific aspect of the data. For instance, instead of analyzing the behavior of a thousand students, research can identify the most common actions among them. By doing this, the person utilizes statistical analysis in research, particularly descriptive statistics.
- Measures of central tendency . Central tendency measures are the mean, mode, and media or the averages denoting specific data points. They assess the centrality of the probability distribution, hence the name. These measures describe the data in relation to the center.
- Measures of frequency . These statistics document the number of times an event happens. They include frequency, count, ratios, rates, and proportions. Measures of frequency can also show how often a score occurs.
- Measures of dispersion/variation . These descriptive statistics assess the intervals between the data points. The objective is to view the spread or disparity between the specific inputs. Measures of variation include the standard deviation, variance, and range. They indicate how the spread may affect other statistics, such as the mean.
- Measures of position . Sometimes researchers can investigate relationships between scores. Measures of position, such as percentiles, quartiles, and ranks, demonstrate this association. They are often useful when comparing the data to normalized information.
Inferential Statistics
Inferential statistics is critical in statistical analysis in quantitative research. This approach uses statistical tests to draw conclusions about the population. Examples of inferential statistics include t-tests, F-tests, ANOVA, p-value, Mann-Whitney U test, and Wilcoxon W test. This
Common Statistical Analysis in Research Types
Although inferential and descriptive statistics can be classified as types of statistical analysis in research, they are mostly considered analytical methods. Types of research are distinguishable by the differences in the methodology employed in analyzing, assembling, classifying, manipulating, and interpreting data. The categories may also depend on the type of data used.
Predictive Analysis
Predictive research analyzes past and present data to assess trends and predict future events. An excellent example of predictive analysis is a market survey that seeks to understand customers’ spending habits to weigh the possibility of a repeat or future purchase. Such studies assess the likelihood of an action based on trends.
Prescriptive Analysis
On the other hand, a prescriptive analysis targets likely courses of action. It’s decision-making research designed to identify optimal solutions to a problem. Its primary objective is to test or assess alternative measures.
Causal Analysis
Causal research investigates the explanation behind the events. It explores the relationship between factors for causation. Thus, researchers use causal analyses to analyze root causes, possible problems, and unknown outcomes.
Mechanistic Analysis
This type of research investigates the mechanism of action. Instead of focusing only on the causes or possible outcomes, researchers may seek an understanding of the processes involved. In such cases, they use mechanistic analyses to document, observe, or learn the mechanisms involved.
Exploratory Data Analysis
Similarly, an exploratory study is extensive with a wider scope and minimal limitations. This type of research seeks insight into the topic of interest. An exploratory researcher does not try to generalize or predict relationships. Instead, they look for information about the subject before conducting an in-depth analysis.
The Importance of Statistical Analysis in Research
As a matter of fact, statistical analysis provides critical information for decision-making. Decision-makers require past trends and predictive assumptions to inform their actions. In most cases, the data is too complex or lacks meaningful inferences. Statistical tools for analyzing such details help save time and money, deriving only valuable information for assessment. An excellent statistical analysis in research example is a randomized control trial (RCT) for the Covid-19 vaccine. You can download a sample of such a document online to understand the significance such analyses have to the stakeholders. A vaccine RCT assesses the effectiveness, side effects, duration of protection, and other benefits. Hence, statistical analysis in research is a helpful tool for understanding data.
Sources and links For the articles and videos I use different databases, such as Eurostat, OECD World Bank Open Data, Data Gov and others. You are free to use the video I have made on your site using the link or the embed code. If you have any questions, don’t hesitate to write to me!
Support statistics and data, if you have reached the end and like this project, you can donate a coffee to “statistics and data”..
Copyright © 2022 Statistics and Data
SPSS: An Imperative Quantitative Data Analysis Tool for Social Science Research
- October 2021
- V(X):300-302

- Begum Rokeya University, Rangpur
- This person is not on ResearchGate, or hasn't claimed this research yet.
Discover the world's research
- 25+ million members
- 160+ million publication pages
- 2.3+ billion citations
- Naila Rajiha
- Noor Malihah
- Nur Muthmainnah
- Rubisha Adhikari
- Rajani Shah
- Kamal Ghimire

- Raghavendra R

- INT J ENVIRON HEAL R

- Umaiza Bashir
- OPHTHAL EPIDEMIOL
- Yu-Bai Chou
- Hsin-Ho Chang
- Hsun-I Chiu
- Amirah Iryani Hayatuddin
- A’dawiyah Ismail
- Seyievono Savi

- Sabine Landau
- Brian S. Everitt

- Dorothy Giles Williams
- Earl R. Babbie

- Recruit researchers
- Join for free
- Login Email Tip: Most researchers use their institutional email address as their ResearchGate login Password Forgot password? Keep me logged in Log in or Continue with Google Welcome back! Please log in. Email · Hint Tip: Most researchers use their institutional email address as their ResearchGate login Password Forgot password? Keep me logged in Log in or Continue with Google No account? Sign up
Thank you for visiting nature.com. You are using a browser version with limited support for CSS. To obtain the best experience, we recommend you use a more up to date browser (or turn off compatibility mode in Internet Explorer). In the meantime, to ensure continued support, we are displaying the site without styles and JavaScript.
- View all journals
- Explore content
- About the journal
- Publish with us
- Sign up for alerts
Statistics articles within Scientific Reports
Article 29 August 2024 | Open Access
Effective weight optimization strategy for precise deep learning forecasting models using EvoLearn approach
- , Ashima Anand
- & Rajender Parsad
Article 26 August 2024 | Open Access
Quantification of the time-varying epidemic growth rate and of the delays between symptom onset and presenting to healthcare for the mpox epidemic in the UK in 2022
- Robert Hinch
- , Jasmina Panovska-Griffiths
- & Christophe Fraser
Investigating the causal relationship between wealth index and ICT skills: a mediation analysis approach
- Tarikul Islam
- & Nabil Ahmed Uthso
Article 24 August 2024 | Open Access
Statistical analysis of the effect of socio-political factors on individual life satisfaction
- , Isra Hasan
- & Ayman Alzaatreh
Article 23 August 2024 | Open Access
Improving the explainability of autoencoder factors for commodities through forecast-based Shapley values
- Roy Cerqueti
- , Antonio Iovanella
- & Saverio Storani
Article 20 August 2024 | Open Access
Defect detection of printed circuit board assembly based on YOLOv5
- Minghui Shen
- , Yujie Liu
- & Ye Jiang
Breaking the silence: leveraging social interaction data to identify high-risk suicide users online using network analysis and machine learning
- Damien Lekkas
- & Nicholas C. Jacobson
Stochastic image spectroscopy: a discriminative generative approach to hyperspectral image modelling and classification
- Alvaro F. Egaña
- , Alejandro Ehrenfeld
- & Jorge F. Silva
Article 15 August 2024 | Open Access
Data-driven risk analysis of nonlinear factor interactions in road safety using Bayesian networks
- Cinzia Carrodano
Article 13 August 2024 | Open Access
Momentum prediction models of tennis match based on CatBoost regression and random forest algorithms
- Xingchen Lv
- , Dingyu Gu
- & Yanfang li
Article 12 August 2024 | Open Access
Numerical and machine learning modeling of GFRP confined concrete-steel hollow elliptical columns
- Haytham F. Isleem
- , Tang Qiong
- & Ali Jahami
Experimental investigation of the distribution patterns of micro-scratches in abrasive waterjet cutting surface
- & Quan Wen
Article 07 August 2024 | Open Access
PMANet : a time series forecasting model for Chinese stock price prediction
- , Weisi Dai
- & Yunjing Zhao
Article 06 August 2024 | Open Access
Grasshopper platform-assisted design optimization of fujian rural earthen buildings considering low-carbon emissions reduction
- & Yang Ding
Article 03 August 2024 | Open Access
Effects of dietary fish to rapeseed oil ratio on steatosis symptoms in Atlantic salmon ( Salmo salar L) of different sizes
- D. Siciliani
- & Å. Krogdahl
A model-free and distribution-free multi-omics integration approach for detecting novel lung adenocarcinoma genes
- Shaofei Zhao
- & Guifang Fu
Article 01 August 2024 | Open Access
Intrinsic dimension as a multi-scale summary statistics in network modeling
- Iuri Macocco
- , Antonietta Mira
- & Alessandro Laio
A new possibilistic-based clustering method for probability density functions and its application to detecting abnormal elements
- Hung Tran-Nam
- , Thao Nguyen-Trang
- & Ha Che-Ngoc
Article 30 July 2024 | Open Access
A dynamic customer segmentation approach by combining LRFMS and multivariate time series clustering
- Shuhai Wang
- , Linfu Sun
- & Yang Yu
Article 29 July 2024 | Open Access
Field evaluation of a volatile pyrethroid spatial repellent and etofenprox treated clothing for outdoor protection against forest malaria vectors in Cambodia
- Élodie A. Vajda
- , Amanda Ross
- & Neil F. Lobo
Study on crease recovery property of warp-knitted jacquard spacer shoe upper material
- & Shiyu Peng
Article 27 July 2024 | Open Access
Calibration estimation of population total using multi-auxiliary information in the presence of non-response
- , Anant Patel
- & Menakshi Pachori
Simulation-based prior knowledge elicitation for parametric Bayesian models
- Florence Bockting
- , Stefan T. Radev
- & Paul-Christian Bürkner
Article 26 July 2024 | Open Access
Modelling Salmonella Typhi in high-density urban Blantyre neighbourhood, Malawi, using point pattern methods
- Jessie J. Khaki
- , James E. Meiring
- & Emanuele Giorgi
Exogenous variable driven deep learning models for improved price forecasting of TOP crops in India
- G. H. Harish Nayak
- , Md Wasi Alam
- & Chandan Kumar Deb
Generalization of cut-in pre-crash scenarios for autonomous vehicles based on accident data
- , Xinyu Zhu
- & Chang Xu
Article 19 July 2024 | Open Access
Automated PD-L1 status prediction in lung cancer with multi-modal PET/CT fusion
- Ronrick Da-ano
- , Gustavo Andrade-Miranda
- & Catherine Cheze Le Rest
Article 17 July 2024 | Open Access
Optimizing decision-making with aggregation operators for generalized intuitionistic fuzzy sets and their applications in the tech industry
- Muhammad Wasim
- , Awais Yousaf
- & Hamiden Abd El-Wahed Khalifa
Article 15 July 2024 | Open Access
Putting ICAP to the test: how technology-enhanced learning activities are related to cognitive and affective-motivational learning outcomes in higher education
- Christina Wekerle
- , Martin Daumiller
- & Ingo Kollar
The impact of national savings on economic development: a focused study on the ten poorest countries in Sub-Saharan Africa
Article 13 July 2024 | Open Access
Regularized ensemble learning for prediction and risk factors assessment of students at risk in the post-COVID era
- Zardad Khan
- , Amjad Ali
- & Saeed Aldahmani
Article 12 July 2024 | Open Access
Eigen-entropy based time series signatures to support multivariate time series classification
- Abhidnya Patharkar
- , Jiajing Huang
- & Naomi Gades
Article 11 July 2024 | Open Access
Exploring usage pattern variation of free-floating bike-sharing from a night travel perspective
- , Xianke Han
- & Lili Li
Early mutational signatures and transmissibility of SARS-CoV-2 Gamma and Lambda variants in Chile
- Karen Y. Oróstica
- , Sebastian B. Mohr
- & Seba Contreras
Article 10 July 2024 | Open Access
Optimizing the location of vaccination sites to stop a zoonotic epidemic
- Ricardo Castillo-Neyra
- , Sherrie Xie
- & Michael Z. Levy
Article 08 July 2024 | Open Access
Integrating socio-psychological factors in the SEIR model optimized by a genetic algorithm for COVID-19 trend analysis
- Haonan Wang
- , Danhong Wu
- & Junhui Zhang
Article 05 July 2024 | Open Access
Research on bearing fault diagnosis based on improved genetic algorithm and BP neural network
- Zenghua Chen
- , Lingjian Zhu
- & Gang Xiong
Article 04 July 2024 | Open Access
Employees’ pro-environmental behavior in an organization: a case study in the UAE
- Nadin Alherimi
- , Zeki Marva
- & Ayman Alzaaterh
Article 03 July 2024 | Open Access
The predictive capability of several anthropometric indices for identifying the risk of metabolic syndrome and its components among industrial workers
- Ekaterina D. Konstantinova
- , Tatiana A. Maslakova
- & Svetlana Yu. Ogorodnikova
Article 02 July 2024 | Open Access
A bayesian spatio-temporal dynamic analysis of food security in Africa
- Adusei Bofa
- & Temesgen Zewotir
Research on the influencing factors of promoting flipped classroom teaching based on the integrated UTAUT model and learning engagement theory
- & Wang He
Article 28 June 2024 | Open Access
Peak response regularization for localization
- , Jinzhen Yao
- & Qintao Hu
Article 25 June 2024 | Open Access
Prediction and reliability analysis of shear strength of RC deep beams
- Khaled Megahed
Multistage time-to-event models improve survival inference by partitioning mortality processes of tracked organisms
- Suresh A. Sethi
- , Alex L. Koeberle
- & Kenneth Duren
Article 24 June 2024 | Open Access
Summarizing physical performance in professional soccer: development of a new composite index
- José M. Oliva-Lozano
- , Mattia Cefis
- & Ricardo Resta
Finding multifaceted communities in multiplex networks
- László Gadár
- & János Abonyi
Article 22 June 2024 | Open Access
Utilizing Bayesian inference in accelerated testing models under constant stress via ordered ranked set sampling and hybrid censoring with practical validation
- Atef F. Hashem
- , Naif Alotaibi
- & Alaa H. Abdel-Hamid
Predicting chronic wasting disease in white-tailed deer at the county scale using machine learning
- Md Sohel Ahmed
- , Brenda J. Hanley
- & Krysten L. Schuler
Article 21 June 2024 | Open Access
Properties, quantile regression, and application of bounded exponentiated Weibull distribution to COVID-19 data of mortality and survival rates
- Shakila Bashir
- , Bushra Masood
- & Iram Saleem
Article 20 June 2024 | Open Access
Cox proportional hazards regression in small studies of predictive biomarkers
- , V. H. Nguyen
- & M. Hauptmann
Browse broader subjects
- Mathematics and computing
Quick links
- Explore articles by subject
- Guide to authors
- Editorial policies


An official website of the United States government
The .gov means it’s official. Federal government websites often end in .gov or .mil. Before sharing sensitive information, make sure you’re on a federal government site.
The site is secure. The https:// ensures that you are connecting to the official website and that any information you provide is encrypted and transmitted securely.
- Publications
- Account settings
Preview improvements coming to the PMC website in October 2024. Learn More or Try it out now .
- Advanced Search
- Journal List
- BMJ Open Access

How to write statistical analysis section in medical research
Alok kumar dwivedi.
Department of Molecular and Translational Medicine, Division of Biostatistics and Epidemiology, Texas Tech University Health Sciences Center El Paso, El Paso, Texas, USA
Associated Data
jim-2022-002479supp001.pdf
Data sharing not applicable as no datasets generated and/or analyzed for this study.
Reporting of statistical analysis is essential in any clinical and translational research study. However, medical research studies sometimes report statistical analysis that is either inappropriate or insufficient to attest to the accuracy and validity of findings and conclusions. Published works involving inaccurate statistical analyses and insufficient reporting influence the conduct of future scientific studies, including meta-analyses and medical decisions. Although the biostatistical practice has been improved over the years due to the involvement of statistical reviewers and collaborators in research studies, there remain areas of improvement for transparent reporting of the statistical analysis section in a study. Evidence-based biostatistics practice throughout the research is useful for generating reliable data and translating meaningful data to meaningful interpretation and decisions in medical research. Most existing research reporting guidelines do not provide guidance for reporting methods in the statistical analysis section that helps in evaluating the quality of findings and data interpretation. In this report, we highlight the global and critical steps to be reported in the statistical analysis of grants and research articles. We provide clarity and the importance of understanding study objective types, data generation process, effect size use, evidence-based biostatistical methods use, and development of statistical models through several thematic frameworks. We also provide published examples of adherence or non-adherence to methodological standards related to each step in the statistical analysis and their implications. We believe the suggestions provided in this report can have far-reaching implications for education and strengthening the quality of statistical reporting and biostatistical practice in medical research.
Introduction
Biostatistics is the overall approach to how we realistically and feasibly execute a research idea to produce meaningful data and translate data to meaningful interpretation and decisions. In this era of evidence-based medicine and practice, basic biostatistical knowledge becomes essential for critically appraising research articles and implementing findings for better patient management, improving healthcare, and research planning. 1 However, it may not be sufficient for the proper execution and reporting of statistical analyses in studies. 2 3 Three things are required for statistical analyses, namely knowledge of the conceptual framework of variables, research design, and evidence-based applications of statistical analysis with statistical software. 4 5 The conceptual framework provides possible biological and clinical pathways between independent variables and outcomes with role specification of variables. The research design provides a protocol of study design and data generation process (DGP), whereas the evidence-based statistical analysis approach provides guidance for selecting and implementing approaches after evaluating data with the research design. 2 5 Ocaña-Riola 6 reported a substantial percentage of articles from high-impact medical journals contained errors in statistical analysis or data interpretation. These errors in statistical analyses and interpretation of results do not only impact the reliability of research findings but also influence the medical decision-making and planning and execution of other related studies. A survey of consulting biostatisticians in the USA reported that researchers frequently request biostatisticians for performing inappropriate statistical analyses and inappropriate reporting of data. 7 This implies that there is a need to enforce standardized reporting of the statistical analysis section in medical research which can also help rreviewers and investigators to improve the methodological standards of the study.
Biostatistical practice in medicine has been improving over the years due to continuous efforts in promoting awareness and involving expert services on biostatistics, epidemiology, and research design in clinical and translational research. 8–11 Despite these efforts, the quality of reporting of statistical analysis in research studies has often been suboptimal. 12 13 We noticed that none of the methods reporting documents were developed using evidence-based biostatistics (EBB) theory and practice. The EBB practice implies that the selection of statistical analysis methods for statistical analyses and the steps of results reporting and interpretation should be grounded based on the evidence generated in the scientific literature and according to the study objective type and design. 5 Previous works have not properly elucidated the importance of understanding EBB concepts and related reporting in the write-up of statistical analyses. As a result, reviewers sometimes ask to present data or execute analyses that do not match the study objective type. 14 We summarize the statistical analysis steps to be reported in the statistical analysis section based on review and thematic frameworks.
We identified articles describing statistical reporting problems in medicine using different search terms ( online supplemental table 1 ). Based on these studies, we prioritized commonly reported statistical errors in analytical strategies and developed essential components to be reported in the statistical analysis section of research grants and studies. We also clarified the purpose and the overall implication of reporting each step in statistical analyses through various examples.
Supplementary data
Although biostatistical inputs are critical for the entire research study ( online supplemental table 2 ), biostatistical consultations were mostly used for statistical analyses only 15 . Even though the conduct of statistical analysis mismatched with the study objective and DGP was identified as the major problem in articles submitted to high-impact medical journals. 16 In addition, multivariable analyses were often inappropriately conducted and reported in published studies. 17 18 In light of these statistical errors, we describe the reporting of the following components in the statistical analysis section of the study.
Step 1: specify study objective type and outcomes (overall approach)
The study objective type provides the role of important variables for a specified outcome in statistical analyses and the overall approach of the model building and model reporting steps in a study. In the statistical framework, the problems are classified into descriptive and inferential/analytical/confirmatory objectives. In the epidemiological framework, the analytical and prognostic problems are broadly classified into association, explanatory, and predictive objectives. 19 These study objectives ( figure 1 ) may be classified into six categories: (1) exploratory, (2) association, (3) causal, (4) intervention, (5) prediction and (6) clinical decision models in medical research. 20

Comparative assessments of developing and reporting of study objective types and models. Association measures include odds ratio, risk ratio, or hazard ratio. AUC, area under the curve; C, confounder; CI, confidence interval; E, exposure; HbA1C: hemoglobin A1c; M, mediator; MFT, model fit test; MST, model specification test; PI, predictive interval; R 2 , coefficient of determinant; X, independent variable; Y, outcome.
The exploratory objective type is a specific type of determinant study and is commonly known as risk factors or correlates study in medical research. In an exploratory study, all covariates are considered equally important for the outcome of interest in the study. The goal of the exploratory study is to present the results of a model which gives higher accuracy after satisfying all model-related assumptions. In the association study, the investigator identifies predefined exposures of interest for the outcome, and variables other than exposures are also important for the interpretation and considered as covariates. The goal of an association study is to present the adjusted association of exposure with outcome. 20 In the causal objective study, the investigator is interested in determining the impact of exposure(s) on outcome using the conceptual framework. In this study objective, all variables should have a predefined role (exposures, confounders, mediators, covariates, and predictors) in a conceptual framework. A study with a causal objective is known as an explanatory or a confirmatory study in medical research. The goal is to present the direct or indirect effects of exposure(s) on an outcome after assessing the model’s fitness in the conceptual framework. 19 21 The objective of an interventional study is to determine the effect of an intervention on outcomes and is often known as randomized or non-randomized clinical trials in medical research. In the intervention objective model, all variables other than the intervention are treated as nuisance variables for primary analyses. The goal is to present the direct effect of the intervention on the outcomes by eliminating biases. 22–24 In the predictive study, the goal is to determine an optimum set of variables that can predict the outcome, particularly in external settings. The clinical decision models are a special case of prognostic models in which high dimensional data at various levels are used for risk stratification, classification, and prediction. In this model, all variables are considered input features. The goal is to present a decision tool that has high accuracy in training, testing, and validation data sets. 20 25 Biostatisticians or applied researchers should properly discuss the intention of the study objective type before proceeding with statistical analyses. In addition, it would be a good idea to prepare a conceptual model framework regardless of study objective type to understand study concepts.
A study 26 showed a favorable effect of the beta-blocker intervention on survival outcome in patients with advanced human epidermal growth factor receptor (HER2)-negative breast cancer without adjusting for all the potential confounding effects (age or menopausal status and Eastern Cooperative Oncology Performance Status) in primary analyses or validation analyses or using a propensity score-adjusted analysis, which is an EBB preferred method for analyzing non-randomized studies. 27 Similarly, another study had the goal of developing a predictive model for prediction of Alzheimer’s disease progression. 28 However, this study did not internally or externally validate the performance of the model as per the requirement of a predictive objective study. In another study, 29 investigators were interested in determining an association between metabolic syndrome and hepatitis C virus. However, the authors did not clearly specify the outcome in the analysis and produced conflicting associations with different analyses. 30 Thus, the outcome should be clearly specified as per the study objective type.
Step 2: specify effect size measure according to study design (interpretation and practical value)
The study design provides information on the selection of study participants and the process of data collection conditioned on either exposure or outcome ( figure 2 ). The appropriate use of effect size measure, tabular presentation of results, and the level of evidence are mostly determined by the study design. 31 32 In cohort or clinical trial study designs, the participants are selected based on exposure status and are followed up for the development of the outcome. These study designs can provide multiple outcomes, produce incidence or incidence density, and are preferred to be analyzed with risk ratio (RR) or hazards models. In a case–control study, the selection of participants is conditioned on outcome status. This type of study can have only one outcome and is preferred to be analyzed with an odds ratio (OR) model. In a cross-sectional study design, there is no selection restriction on outcomes or exposures. All data are collected simultaneously and can be analyzed with a prevalence ratio model, which is mathematically equivalent to the RR model. 33 The reporting of effect size measure also depends on the study objective type. For example, predictive models typically require reporting of regression coefficients or weight of variables in the model instead of association measures, which are required in other objective types. There are agreements and disagreements between OR and RR measures. Due to the constancy and symmetricity properties of OR, some researchers prefer to use OR in studies with common events. Similarly, the collapsibility and interpretability properties of RR make it more appealing to use in studies with common events. 34 To avoid variable practice and interpretation issues with OR, it is recommended to use RR models in all studies except for case–control and nested case–control studies, where OR approximates RR and thus OR models should be used. Otherwise, investigators may report sufficient data to compute any ratio measure. Biostatisticians should educate investigators on the proper interpretation of ratio measures in the light of study design and their reporting. 34 35

Effect size according to study design.
Investigators sometimes either inappropriately label their study design 36 37 or report effect size measures not aligned with the study design, 38 39 leading to difficulty in results interpretation and evaluation of the level of evidence. The proper labeling of study design and the appropriate use of effect size measure have substantial implications for results interpretation, including the conduct of systematic review and meta-analysis. 40 A study 31 reviewed the frequency of reporting OR instead of RR in cohort studies and randomized clinical trials (RCTs) and found that one-third of the cohort studies used an OR model, whereas 5% of RCTs used an OR model. The majority of estimated ORs from these studies had a 20% or higher deviation from the corresponding RR.
Step 3: specify study hypothesis, reporting of p values, and interval estimates (interpretation and decision)
The clinical hypothesis provides information for evaluating formal claims specified in the study objectives, while the statistical hypothesis provides information about the population parameters/statistics being used to test the formal claims. The inference about the study hypothesis is typically measured by p value and confidence interval (CI). A smaller p value indicates that the data support against the null hypothesis. Since the p value is a conditional probability, it can never tell about the acceptance or rejection of the null hypothesis. Therefore, multiple alternative strategies of p values have been proposed to strengthen the credibility of conclusions. 41 42 Adaption of these alternative strategies is only needed in the explanatory objective studies. Although exact p values are recommended to be reported in research studies, p values do not provide any information about the effect size. Compared with p values, the CI provides a confidence range of the effect size that contains the true effect size if the study were repeated and can be used to determine whether the results are statistically significant or not. 43 Both p value and 95% CI provide complementary information and thus need to be specified in the statistical analysis section. 24 44
Researchers often test one or more comparisons or hypotheses. Accordingly, the side and the level of significance for considering results to be statistically significant may change. Furthermore, studies may include more than one primary outcome that requires an adjustment in the level of significance for multiplicity. All studies should provide the interval estimate of the effect size/regression coefficient in the primary analyses. Since the interpretation of data analysis depends on the study hypothesis, researchers are required to specify the level of significance along with the side (one-sided or two-sided) of the p value in the test for considering statistically significant results, adjustment of the level of significance due to multiple comparisons or multiplicity, and reporting of interval estimates of the effect size in the statistical analysis section. 45
A study 46 showed a significant effect of fluoxetine on relapse rates in obsessive-compulsive disorder based on a one-sided p value of 0.04. Clearly, there was no reason for using a one-sided p value as opposed to a two-sided p value. A review of the appropriate use of multiple test correction methods in multiarm clinical trials published in major medical journals in 2012 identified over 50% of the articles did not perform multiple-testing correction. 47 Similar to controlling a familywise error rate due to multiple comparisons, adjustment of the false discovery rate is also critical in studies involving multiple related outcomes. A review of RCTs for depression between 2007 and 2008 from six journals reported that only limited studies (5.8%) accounted for multiplicity in the analyses due to multiple outcomes. 48
Step 4: account for DGP in the statistical analysis (accuracy)
The study design also requires the specification of the selection of participants and outcome measurement processes in different design settings. We referred to this specific design feature as DGP. Understanding DGP helps in determining appropriate modeling of outcome distribution in statistical analyses and setting up model premises and units of analysis. 4 DGP ( figure 3 ) involves information on data generation and data measures, including the number of measurements after random selection, complex selection, consecutive selection, pragmatic selection, or systematic selection. Specifically, DGP depends on a sampling setting (participants are selected using survey sampling methods and one subject may represent multiple participants in the population), clustered setting (participants are clustered through a recruitment setting or hierarchical setting or multiple hospitals), pragmatic setting (participants are selected through mixed approaches), or systematic review setting (participants are selected from published studies). DGP also depends on the measurements of outcomes in an unpaired setting (measured on one occasion only in independent groups), paired setting (measured on more than one occasion or participants are matched on certain subject characteristics), or mixed setting (measured on more than one occasion but interested in comparing independent groups). It also involves information regarding outcomes or exposure generation processes using quantitative or categorical variables, quantitative values using labs or validated instruments, and self-reported or administered tests yielding a variety of data distributions, including individual distribution, mixed-type distribution, mixed distributions, and latent distributions. Due to different DGPs, study data may include messy or missing data, incomplete/partial measurements, time-varying measurements, surrogate measures, latent measures, imbalances, unknown confounders, instrument variables, correlated responses, various levels of clustering, qualitative data, or mixed data outcomes, competing events, individual and higher-level variables, etc. The performance of statistical analysis, appropriate estimation of standard errors of estimates and subsequently computation of p values, the generalizability of findings, and the graphical display of data rely on DGP. Accounting for DGP in the analyses requires proper communication between investigators and biostatisticians about each aspect of participant selection and data collection, including measurements, occasions of measurements, and instruments used in the research study.

Common features of the data generation process.
A study 49 compared the intake of fresh fruit and komatsuna juice with the intake of commercial vegetable juice on metabolic parameters in middle-aged men using an RCT. The study was criticized for many reasons, but primarily for incorrect statistical methods not aligned with the study DGP. 50 Similarly, another study 51 highlighted that 80% of published studies using the Korean National Health and Nutrition Examination Survey did not incorporate survey sampling structure in statistical analyses, producing biased estimates and inappropriate findings. Likewise, another study 52 highlighted the need for maintaining methodological standards while analyzing data from the National Inpatient Sample. A systematic review 53 identified that over 50% of studies did not specify whether a paired t-test or an unpaired t-test was performed in statistical analysis in the top 25% of physiology journals, indicating poor transparency in reporting of statistical analysis as per the data type. Another study 54 also highlighted the data displaying errors not aligned with DGP. As per DGP, delay in treatment initiation of patients with cancer defined from the onset of symptom to treatment initiation should be analyzed into three components: patient/primary delay, secondary delay, and tertiary delay. 55 Similarly, the number of cancerous nodes should be analyzed with count data models. 56 However, several studies did not analyze such data according to DGP. 57 58
Step 5: apply EBB methods specific to study design features and DGP (efficiency and robustness)
The continuous growth in the development of robust statistical methods for dealing with a specific problem produced various methods to analyze specific data types. Since multiple methods are available for handling a specific problem yet with varying performances, heterogeneous practices among applied researchers have been noticed. Variable practices could also be due to a lack of consensus on statistical methods in literature, unawareness, and the unavailability of standardized statistical guidelines. 2 5 59 However, it becomes sometimes difficult to differentiate whether a specific method was used due to its robustness, lack of awareness, lack of accessibility of statistical software to apply an alternative appropriate method, intention to produce expected results, or ignorance of model diagnostics. To avoid heterogeneous practices, the selection of statistical methodology and their reporting at each stage of data analysis should be conducted using methods according to EBB practice. 5 Since it is hard for applied researchers to optimally select statistical methodology at each step, we encourage investigators to involve biostatisticians at the very early stage in basic, clinical, population, translational, and database research. We also appeal to biostatisticians to develop guidelines, checklists, and educational tools to promote the concept of EBB. As an effort, we developed the statistical analysis and methods in biomedical research (SAMBR) guidelines for applied researchers to use EBB methods for data analysis. 5 The EBB practice is essential for applying recent cutting-edge robust methodologies to yield accurate and unbiased results. The efficiency of statistical methodologies depends on the assumptions and DGP. Therefore, investigators may attempt to specify the choice of specific models in the primary analysis as per the EBB.
Although details of evidence-based preferred methods are provided in the SAMBR checklists for each study design/objective, 5 we have presented a simplified version of evidence-based preferred methods for common statistical analysis ( online supplemental table 3 ). Several examples are available in the literature where inefficient methods not according to EBB practice have been used. 31 57 60
Step 6: report variable selection method in the multivariable analysis according to study objective type (unbiased)
Multivariable analysis can be used for association, prediction or classification or risk stratification, adjustment, propensity score development, and effect size estimation. 61 Some biological, clinical, behavioral, and environmental factors may directly associate or influence the relationship between exposure and outcome. Therefore, almost all health studies require multivariable analyses for accurate and unbiased interpretations of findings ( figure 1 ). Analysts should develop an adjusted model if the sample size permits. It is a misconception that the analysis of RCT does not require adjusted analysis. Analysis of RCT may require adjustment for prognostic variables. 23 The foremost step in model building is the entry of variables after finalizing the appropriate parametric or non-parametric regression model. In the exploratory model building process due to no preference of exposures, a backward automated approach after including any variables that are significant at 25% in the unadjusted analysis can be used for variable selection. 62 63 In the association model, a manual selection of covariates based on the relevance of the variables should be included in a fully adjusted model. 63 In a causal model, clinically guided methods should be used for variable selection and their adjustments. 20 In a non-randomized interventional model, efforts should be made to eliminate confounding effects through propensity score methods and the final propensity score-adjusted multivariable model may adjust any prognostic variables, while a randomized study simply should adjust any prognostic variables. 27 Maintaining the event per variable (EVR) is important to avoid overfitting in any type of modeling; therefore, screening of variables may be required in some association and explanatory studies, which may be accomplished using a backward stepwise method that needs to be clarified in the statistical analyses. 10 In a predictive study, a model with an optimum set of variables producing the highest accuracy should be used. The optimum set of variables may be screened with the random forest method or bootstrap or machine learning methods. 64 65 Different methods of variable selection and adjustments may lead to different results. The screening process of variables and their adjustments in the final multivariable model should be clearly mentioned in the statistical analysis section.
A study 66 evaluating the effect of hydroxychloroquine (HDQ) showed unfavorable events (intubation or death) in patients who received HDQ compared with those who did not (hazard ratio (HR): 2.37, 95% CI 1.84 to 3.02) in an unadjusted analysis. However, the propensity score-adjusted analyses as appropriate with the interventional objective model showed no significant association between HDQ use and unfavorable events (HR: 1.04, 95% CI 0.82 to 1.32), which was also confirmed in multivariable and other propensity score-adjusted analyses. This study clearly suggests that results interpretation should be based on a multivariable analysis only in observational studies if feasible. A recent study 10 noted that approximately 6% of multivariable analyses based on either logistic or Cox regression used an inappropriate selection method of variables in medical research. This practice was more commonly noted in studies that did not involve an expert biostatistician. Another review 61 of 316 articles from high-impact Chinese medical journals revealed that 30.7% of articles did not report the selection of variables in multivariable models. Indeed, this inappropriate practice could have been identified more commonly if classified according to the study objective type. 18 In RCTs, it is uncommon to report an adjusted analysis based on prognostic variables, even though an adjusted analysis may produce an efficient estimate compared with an unadjusted analysis. A study assessing the effect of preemptive intervention on development outcomes showed a significant effect of an intervention on reducing autism spectrum disorder symptoms. 67 However, this study was criticized by Ware 68 for not reporting non-significant results in unadjusted analyses. If possible, unadjusted estimates should also be reported in any study, particularly in RCTs. 23 68
Step 7: provide evidence for exploring effect modifiers (applicability)
Any variable that modifies the effect of exposure on the outcome is called an effect modifier or modifier or an interacting variable. Exploring the effect modifiers in multivariable analyses helps in (1) determining the applicability/generalizability of findings in the overall or specific subpopulation, (2) generating ideas for new hypotheses, (3) explaining uninterpretable findings between unadjusted and adjusted analyses, (4) guiding to present combined or separate models for each specific subpopulation, and (5) explaining heterogeneity in treatment effect. Often, investigators present adjusted stratified results according to the presence or absence of an effect modifier. If the exposure interacts with multiple variables statistically or conceptually in the model, then the stratified findings (subgroup) according to each effect modifier may be presented. Otherwise, stratified analysis substantially reduces the power of the study due to the lower sample size in each stratum and may produce significant results by inflating type I error. 69 Therefore, a multivariable analysis involving an interaction term as opposed to a stratified analysis may be presented in the presence of an effect modifier. 70 Sometimes, a quantitative variable may emerge as a potential effect modifier for exposure and an outcome relationship. In such a situation, the quantitative variable should not be categorized unless a clinically meaningful threshold is not available in the study. In fact, the practice of categorizing quantitative variables should be avoided in the analysis unless a clinically meaningful cut-off is available or a hypothesis requires for it. 71 In an exploratory objective type, any possible interaction may be obtained in a study; however, the interpretation should be guided based on clinical implications. Similarly, some objective models may have more than one exposure or intervention and the association of each exposure according to the level of other exposure should be presented through adjusted analyses as suggested in the presence of interaction effects. 70
A review of 428 articles from MEDLINE on the quality of reporting from statistical analyses of three (linear, logistic, and Cox) commonly used regression models reported that only 18.5% of the published articles provided interaction analyses, 17 even though interaction analyses can provide a lot of useful information.
Step 8: assessment of assumptions, specifically the distribution of outcome, linearity, multicollinearity, sparsity, and overfitting (reliability)
The assessment and reporting of model diagnostics are important in assessing the efficiency, validity, and usefulness of the model. Model diagnostics include satisfying model-specific assumptions and the assessment of sparsity, linearity, distribution of outcome, multicollinearity, and overfitting. 61 72 Model-specific assumptions such as normal residuals, heteroscedasticity and independence of errors in linear regression, proportionality in Cox regression, proportionality odds assumption in ordinal logistic regression, and distribution fit in other types of continuous and count models are required. In addition, sparsity should also be examined prior to selecting an appropriate model. Sparsity indicates many zero observations in the data set. 73 In the presence of sparsity, the effect size is difficult to interpret. Except for machine learning models, most of the parametric and semiparametric models require a linear relationship between independent variables and a functional form of an outcome. Linearity should be assessed using a multivariable polynomial in all model objectives. 62 Similarly, the appropriate choice of the distribution of outcome is required for model building in all study objective models. Multicollinearity assessment is also useful in all objective models. Assessment of EVR in multivariable analysis can be used to avoid the overfitting issue of a multivariable model. 18
Some review studies highlighted that 73.8%–92% of the articles published in MEDLINE had not assessed the model diagnostics of the multivariable regression models. 17 61 72 Contrary to the monotonically, linearly increasing relationship between systolic blood pressure (SBP) and mortality established using the Framingham’s study, 74 Port et al 75 reported a non-linear relationship between SBP and all-cause mortality or cardiovascular deaths by reanalysis of the Framingham’s study data set. This study identified a different threshold for treating hypertension, indicating the role of linearity assessment in multivariable models. Although a non-Gaussian distribution model may be required for modeling patient delay outcome data in cancer, 55 a study analyzed patient delay data using an ordinary linear regression model. 57 An investigation of the development of predictive models and their reporting in medical journals identified that 53% of the articles had fewer EVR than the recommended EVR, indicating over half of the published articles may have an overfitting model. 18 Another study 76 attempted to identify the anthropometric variables associated with non-insulin-dependent diabetes and found that none of the anthropometric variables were significant after adjusting for waist circumference, age, and sex, indicating the presence of collinearity. A study reported detailed sparse data problems in published studies and potential solutions. 73
Step 9: report type of primary and sensitivity analyses (consistency)
Numerous considerations and assumptions are made throughout the research processes that require assessment, evaluation, and validation. Some assumptions, executions, and errors made at the beginning of the study data collection may not be fixable 13 ; however, additional information collected during the study and data processing, including data distribution obtained at the end of the study, may facilitate additional considerations that need to be verified in the statistical analyses. Consistencies in the research findings via modifications in the outcome or exposure definition, study population, accounting for missing data, model-related assumptions, variables and their forms, and accounting for adherence to protocol in the models can be evaluated and reported in research studies using sensitivity analyses. 77 The purpose and type of supporting analyses need to be specified clearly in the statistical analyses to differentiate the main findings from the supporting findings. Sensitivity analyses are different from secondary or interim or subgroup analyses. 78 Data analyses for secondary outcomes are often referred to as secondary analyses, while data analyses of an ongoing study are called interim analyses and data analyses according to groups based on patient characteristics are known as subgroup analyses.
Almost all studies require some form of sensitivity analysis to validate the findings under different conditions. However, it is often underutilized in medical journals. Only 18%–20.3% of studies reported some forms of sensitivity analyses. 77 78 A review of nutritional trials from high-quality journals reflected that 17% of the conclusions were reported inappropriately using findings from sensitivity analyses not based on the primary/main analyses. 77
Step 10: provide methods for summarizing, displaying, and interpreting data (transparency and usability)
Data presentation includes data summary, data display, and data from statistical model analyses. The primary purpose of the data summary is to understand the distribution of outcome status and other characteristics in the total sample and by primary exposure status or outcome status. Column-wise data presentation should be preferred according to exposure status in all study designs, while row-wise data presentation for the outcome should be preferred in all study designs except for a case–control study. 24 32 Summary statistics should be used to provide maximum information on data distribution aligned with DGP and variable type. The purpose of results presentation primarily from regression analyses or statistical models is to convey results interpretation and implications of findings. The results should be presented according to the study objective type. Accordingly, the reporting of unadjusted and adjusted associations of each factor with the outcome may be preferred in the determinant objective model, while unadjusted and adjusted effects of primary exposure on the outcome may be preferred in the explanatory objective model. In prognostic models, the final predictive models may be presented in such a way that users can use models to predict an outcome. In the exploratory objective model, a final multivariable model should be reported with R 2 or area under the curve (AUC). In the association and interventional models, the assessment of internal validation is critically important through various sensitivity and validation analyses. A model with better fit indices (in terms of R 2 or AUC, Akaike information criterion, Bayesian information criterion, fit index, root mean square error) should be finalized and reported in the causal model objective study. In the predictive objective type, the model performance in terms of R 2 or AUC in training and validation data sets needs to be reported ( figure 1 ). 20 21 There are multiple purposes of data display, including data distribution using bar diagram or histogram or frequency polygons or box plots, comparisons using cluster bar diagram or scatter dot plot or stacked bar diagram or Kaplan-Meier plot, correlation or model assessment using scatter plot or scatter matrix, clustering or pattern using heatmap or line plots, the effect of predictors with fitted models using marginsplot, and comparative evaluation of effect sizes from regression models using forest plot. Although the key purpose of data display is to highlight critical issues or findings in the study, data display should essentially follow DGP and variable types and should be user-friendly. 54 79 Data interpretation heavily relies on the effect size measure along with study design and specified hypotheses. Sometimes, variables require standardization for descriptive comparison of effect sizes among exposures or interpreting small effect size, or centralization for interpreting intercept or avoiding collinearity due to interaction terms, or transformation for achieving model-related assumptions. 80 Appropriate methods of data reporting and interpretation aligned with study design, study hypothesis, and effect size measure should be specified in the statistical analysis section of research studies.
Published articles from reputed journals inappropriately summarized a categorized variable with mean and range, 81 summarized a highly skewed variable with mean and standard deviation, 57 and treated a categorized variable as a continuous variable in regression analyses. 82 Similarly, numerous examples from published studies reporting inappropriate graphical display or inappropriate interpretation of data not aligned with DGP or variable types are illustrated in a book published by Bland and Peacock. 83 84 A study used qualitative data on MRI but inappropriately presented with a Box-Whisker plot. 81 Another study reported unusually high OR for an association between high breast parenchymal enhancement and breast cancer in both premenopausal and postmenopausal women. 85 This reporting makes suspicious findings and may include sparse data bias. 86 A poor tabular presentation without proper scaling or standardization of a variable, missing CI for some variables, missing unit and sample size, and inconsistent reporting of decimal places could be easily noticed in table 4 of a published study. 29 Some published predictive models 87 do not report intercept or baseline survival estimates to use their predictive models in clinical use. Although a direct comparison of effect sizes obtained from the same model may be avoided if the units are different among variables, 35 a study had an objective to compare effect sizes across variables but the authors performed comparisons without standardization of variables or using statistical tests. 88
A sample for writing statistical analysis section in medical journals/research studies
Our primary study objective type was to develop a (select from figure 1 ) model to assess the relationship of risk factors (list critical variables or exposures) with outcomes (specify type from continuous/discrete/count/binary/polytomous/time-to-event). To address this objective, we conducted a (select from figure 2 or any other) study design to test the hypotheses of (equality or superiority or non-inferiority or equivalence or futility) or develop prediction. Accordingly, the other variables were adjusted or considered as (specify role of variables from confounders, covariates, or predictors or independent variables) as reflected in the conceptual framework. In the unadjusted or preliminary analyses as per the (select from figure 3 or any other design features) DGP, (specify EBB preferred tests from online supplemental table 3 or any other appropriate tests) were used for (specify variables and types) in unadjusted analyses. According to the EBB practice for the outcome (specify type) and DGP of (select from figure 3 or any other), we used (select from online supplemental table 1 or specify a multivariable approach) as the primary model in the multivariable analysis. We used (select from figure 1 ) variable selection method in the multivariable analysis and explored the interaction effects between (specify variables). The model diagnostics including (list all applicable, including model-related assumptions, linearity, or multicollinearity or overfitting or distribution of outcome or sparsity) were also assessed using (specify appropriate methods) respectively. In such exploration, we identified (specify diagnostic issues if any) and therefore the multivariable models were developed using (specify potential methods used to handle diagnostic issues). The other outcomes were analyzed with (list names of multivariable approaches with respective outcomes). All the models used the same procedure (or specify from figure 1 ) for variable selection, exploration of interaction effects, and model diagnostics using (specify statistical approaches) depending on the statistical models. As per the study design, hypothesis, and multivariable analysis, the results were summarized with effect size (select as appropriate or from figure 2 ) along with (specify 95% CI or other interval estimates) and considered statistically significant using (specify the side of p value or alternatives) at (specify the level of significance) due to (provide reasons for choosing a significance level). We presented unadjusted and/or adjusted estimates of primary outcome according to (list primary exposures or variables). Additional analyses were conducted for (specific reasons from step 9) using (specify methods) to validate findings obtained in the primary analyses. The data were summarized with (list summary measures and appropriate graphs from step 10), whereas the final multivariable model performance was summarized with (fit indices if applicable from step 10). We also used (list graphs) as appropriate with DGP (specify from figure 3 ) to present the critical findings or highlight (specify data issues) using (list graphs/methods) in the study. The exposures or variables were used in (specify the form of the variables) and therefore the effect or association of (list exposures or variables) on outcome should be interpreted in terms of changes in (specify interpretation unit) exposures/variables. List all other additional analyses if performed (with full details of all models in a supplementary file along with statistical codes if possible).
Concluding remarks
We highlighted 10 essential steps to be reported in the statistical analysis section of any analytical study ( figure 4 ). Adherence to minimum reporting of the steps specified in this report may enforce investigators to understand concepts and approach biostatisticians timely to apply these concepts in their study to improve the overall quality of methodological standards in grant proposals and research studies. The order of reporting information in statistical analyses specified in this report is not mandatory; however, clear reporting of analytical steps applicable to the specific study type should be mentioned somewhere in the manuscript. Since the entire approach of statistical analyses is dependent on the study objective type and EBB practice, proper execution and reporting of statistical models can be taught to the next generation of statisticians by the study objective type in statistical education courses. In fact, some disciplines ( figure 5 ) are strictly aligned with specific study objective types. Bioinformaticians are oriented in studying determinant and prognostic models toward precision medicine, while epidemiologists are oriented in studying association and causal models, particularly in population-based observational and pragmatic settings. Data scientists are heavily involved in prediction and classification models in personalized medicine. A common thing across disciplines is using biostatistical principles and computation tools to address any research question. Sometimes, one discipline expert does the part of others. 89 We strongly recommend using a team science approach that includes an epidemiologist, biostatistician, data scientist, and bioinformatician depending on the study objectives and needs. Clear reporting of data analyses as per the study objective type should be encouraged among all researchers to minimize heterogeneous practices and improve scientific quality and outcomes. In addition, we also encourage investigators to strictly follow transparent reporting and quality assessment guidelines according to the study design ( https://www.equator-network.org/ ) to improve the overall quality of the study, accordingly STROBE (Strengthening the Reporting of Observational Studies in Epidemiology) for observational studies, CONSORT (Consolidated Standards of Reporting Trials) for clinical trials, STARD (Standards for Reporting Diagnostic Accuracy Studies) for diagnostic studies, TRIPOD (Transparent Reporting of a multivariable prediction model for Individual Prognosis OR Diagnosis) for prediction modeling, and ARRIVE (Animal Research: Reporting of In Vivo Experiments) for preclinical studies. The steps provided in this document for writing the statistical analysis section is essentially different from other guidance documents, including SAMBR. 5 SAMBR provides a guidance document for selecting evidence-based preferred methods of statistical analysis according to different study designs, while this report suggests the global reporting of essential information in the statistical analysis section according to study objective type. In this guidance report, our suggestion strictly pertains to the reporting of methods in the statistical analysis section and their implications on the interpretation of results. Our document does not provide guidance on the reporting of sample size or results or statistical analysis section for meta-analysis. The examples and reviews reported in this study may be used to emphasize the concepts and related implications in medical research.

Summary of reporting steps, purpose, and evaluation measures in the statistical analysis section.

Role of interrelated disciplines according to study objective type.
Acknowledgments
The author would like to thank the reviewers for their careful review and insightful suggestions.
Contributors: AKD developed the concept and design and wrote the manuscript.
Funding: The authors have not declared a specific grant for this research from any funding agency in the public, commercial or not-for-profit sectors.
Competing interests: AKD is a Journal of Investigative Medicine Editorial Board member. No other competing interests declared.
Provenance and peer review: Commissioned; externally peer reviewed.
Supplemental material: This content has been supplied by the author(s). It has not been vetted by BMJ Publishing Group Limited (BMJ) and may not have been peer-reviewed. Any opinions or recommendations discussed are solely those of the author(s) and are not endorsed by BMJ. BMJ disclaims all liability and responsibility arising from any reliance placed on the content. Where the content includes any translated material, BMJ does not warrant the accuracy and reliability of the translations (including but not limited to local regulations, clinical guidelines, terminology, drug names and drug dosages), and is not responsible for any error and/or omissions arising from translation and adaptation or otherwise.
Data availability statement
Ethics statements, patient consent for publication.
Not required.
Table of Contents
Types of statistical analysis, importance of statistical analysis, benefits of statistical analysis, statistical analysis process, statistical analysis methods, statistical analysis software, statistical analysis examples, career in statistical analysis, choose the right program, become proficient in statistics today, understanding statistical analysis: techniques and applications.
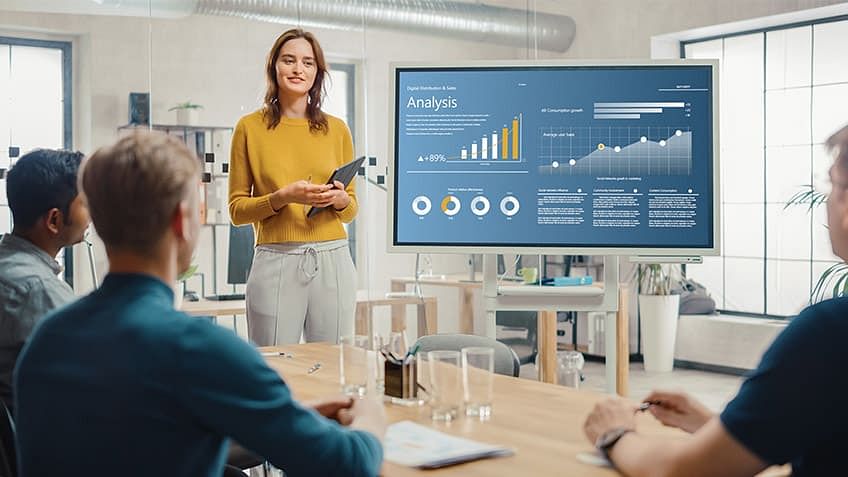
Statistical analysis is the process of collecting and analyzing data in order to discern patterns and trends. It is a method for removing bias from evaluating data by employing numerical analysis. This technique is useful for collecting the interpretations of research, developing statistical models, and planning surveys and studies.
Statistical analysis is a scientific tool in AI and ML that helps collect and analyze large amounts of data to identify common patterns and trends to convert them into meaningful information. In simple words, statistical analysis is a data analysis tool that helps draw meaningful conclusions from raw and unstructured data.
The conclusions are drawn using statistical analysis facilitating decision-making and helping businesses make future predictions on the basis of past trends. It can be defined as a science of collecting and analyzing data to identify trends and patterns and presenting them. Statistical analysis involves working with numbers and is used by businesses and other institutions to make use of data to derive meaningful information.
Given below are the 6 types of statistical analysis:
Descriptive Analysis
Descriptive statistical analysis involves collecting, interpreting, analyzing, and summarizing data to present them in the form of charts, graphs, and tables. Rather than drawing conclusions, it simply makes the complex data easy to read and understand.
Inferential Analysis
The inferential statistical analysis focuses on drawing meaningful conclusions on the basis of the data analyzed. It studies the relationship between different variables or makes predictions for the whole population.
Predictive Analysis
Predictive statistical analysis is a type of statistical analysis that analyzes data to derive past trends and predict future events on the basis of them. It uses machine learning algorithms, data mining , data modelling , and artificial intelligence to conduct the statistical analysis of data.
Prescriptive Analysis
The prescriptive analysis conducts the analysis of data and prescribes the best course of action based on the results. It is a type of statistical analysis that helps you make an informed decision.
Exploratory Data Analysis
Exploratory analysis is similar to inferential analysis, but the difference is that it involves exploring the unknown data associations. It analyzes the potential relationships within the data.
Causal Analysis
The causal statistical analysis focuses on determining the cause and effect relationship between different variables within the raw data. In simple words, it determines why something happens and its effect on other variables. This methodology can be used by businesses to determine the reason for failure.
Statistical analysis eliminates unnecessary information and catalogs important data in an uncomplicated manner, making the monumental work of organizing inputs appear so serene. Once the data has been collected, statistical analysis may be utilized for a variety of purposes. Some of them are listed below:
- The statistical analysis aids in summarizing enormous amounts of data into clearly digestible chunks.
- The statistical analysis aids in the effective design of laboratory, field, and survey investigations.
- Statistical analysis may help with solid and efficient planning in any subject of study.
- Statistical analysis aid in establishing broad generalizations and forecasting how much of something will occur under particular conditions.
- Statistical methods, which are effective tools for interpreting numerical data, are applied in practically every field of study. Statistical approaches have been created and are increasingly applied in physical and biological sciences, such as genetics.
- Statistical approaches are used in the job of a businessman, a manufacturer, and a researcher. Statistics departments can be found in banks, insurance businesses, and government agencies.
- A modern administrator, whether in the public or commercial sector, relies on statistical data to make correct decisions.
- Politicians can utilize statistics to support and validate their claims while also explaining the issues they address.
Become a Data Science & Business Analytics Professional
- 11.5 M Expected New Jobs For Data Science And Analytics
- 28% Annual Job Growth By 2026
- $46K-$100K Average Annual Salary
Post Graduate Program in Data Analytics
- Post Graduate Program certificate and Alumni Association membership
- Exclusive hackathons and Ask me Anything sessions by IBM
Data Analyst
- Industry-recognized Data Analyst Master’s certificate from Simplilearn
- Dedicated live sessions by faculty of industry experts
Here's what learners are saying regarding our programs:
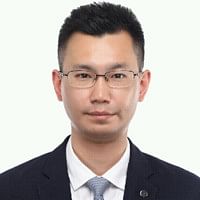
Felix Chong
Project manage , codethink.
After completing this course, I landed a new job & a salary hike of 30%. I now work with Zuhlke Group as a Project Manager.

Gayathri Ramesh
Associate data engineer , publicis sapient.
The course was well structured and curated. The live classes were extremely helpful. They made learning more productive and interactive. The program helped me change my domain from a data analyst to an Associate Data Engineer.
Statistical analysis can be called a boon to mankind and has many benefits for both individuals and organizations. Given below are some of the reasons why you should consider investing in statistical analysis:
- It can help you determine the monthly, quarterly, yearly figures of sales profits, and costs making it easier to make your decisions.
- It can help you make informed and correct decisions.
- It can help you identify the problem or cause of the failure and make corrections. For example, it can identify the reason for an increase in total costs and help you cut the wasteful expenses.
- It can help you conduct market research or analysis and make an effective marketing and sales strategy.
- It helps improve the efficiency of different processes.
Given below are the 5 steps to conduct a statistical analysis that you should follow:
- Step 1: Identify and describe the nature of the data that you are supposed to analyze.
- Step 2: The next step is to establish a relation between the data analyzed and the sample population to which the data belongs.
- Step 3: The third step is to create a model that clearly presents and summarizes the relationship between the population and the data.
- Step 4: Prove if the model is valid or not.
- Step 5: Use predictive analysis to predict future trends and events likely to happen.
Although there are various methods used to perform data analysis, given below are the 5 most used and popular methods of statistical analysis:
Mean or average mean is one of the most popular methods of statistical analysis. Mean determines the overall trend of the data and is very simple to calculate. Mean is calculated by summing the numbers in the data set together and then dividing it by the number of data points. Despite the ease of calculation and its benefits, it is not advisable to resort to mean as the only statistical indicator as it can result in inaccurate decision making.
Standard Deviation
Standard deviation is another very widely used statistical tool or method. It analyzes the deviation of different data points from the mean of the entire data set. It determines how data of the data set is spread around the mean. You can use it to decide whether the research outcomes can be generalized or not.
Regression is a statistical tool that helps determine the cause and effect relationship between the variables. It determines the relationship between a dependent and an independent variable. It is generally used to predict future trends and events.

Hypothesis Testing
Hypothesis testing can be used to test the validity or trueness of a conclusion or argument against a data set. The hypothesis is an assumption made at the beginning of the research and can hold or be false based on the analysis results.
Sample Size Determination
Sample size determination or data sampling is a technique used to derive a sample from the entire population, which is representative of the population. This method is used when the size of the population is very large. You can choose from among the various data sampling techniques such as snowball sampling, convenience sampling, and random sampling.
Everyone can't perform very complex statistical calculations with accuracy making statistical analysis a time-consuming and costly process. Statistical software has become a very important tool for companies to perform their data analysis. The software uses Artificial Intelligence and Machine Learning to perform complex calculations, identify trends and patterns, and create charts, graphs, and tables accurately within minutes.
Look at the standard deviation sample calculation given below to understand more about statistical analysis.
The weights of 5 pizza bases in cms are as follows:
9 | 9-6.4 = 2.6 | (2.6)2 = 6.76 |
2 | 2-6.4 = - 4.4 | (-4.4)2 = 19.36 |
5 | 5-6.4 = - 1.4 | (-1.4)2 = 1.96 |
4 | 4-6.4 = - 2.4 | (-2.4)2 = 5.76 |
12 | 12-6.4 = 5.6 | (5.6)2 = 31.36 |
Calculation of Mean = (9+2+5+4+12)/5 = 32/5 = 6.4
Calculation of mean of squared mean deviation = (6.76+19.36+1.96+5.76+31.36)/5 = 13.04
Sample Variance = 13.04
Standard deviation = √13.04 = 3.611
A Statistical Analyst's career path is determined by the industry in which they work. Anyone interested in becoming a Data Analyst may usually enter the profession and qualify for entry-level Data Analyst positions right out of high school or a certificate program — potentially with a Bachelor's degree in statistics, computer science, or mathematics. Some people go into data analysis from a similar sector such as business, economics, or even the social sciences, usually by updating their skills mid-career with a statistical analytics course.
Statistical Analyst is also a great way to get started in the normally more complex area of data science. A Data Scientist is generally a more senior role than a Data Analyst since it is more strategic in nature and necessitates a more highly developed set of technical abilities, such as knowledge of multiple statistical tools, programming languages, and predictive analytics models.
Aspiring Data Scientists and Statistical Analysts generally begin their careers by learning a programming language such as R or SQL. Following that, they must learn how to create databases, do basic analysis, and make visuals using applications such as Tableau. However, not every Statistical Analyst will need to know how to do all of these things, but if you want to advance in your profession, you should be able to do them all.
Based on your industry and the sort of work you do, you may opt to study Python or R , become an expert at data cleaning, or focus on developing complicated statistical models.
You could also learn a little bit of everything, which might help you take on a leadership role and advance to the position of Senior Data Analyst. A Senior Statistical Analyst with vast and deep knowledge might take on a leadership role leading a team of other Statistical Analysts. Statistical Analysts with extra skill training may be able to advance to Data Scientists or other more senior data analytics positions.
Supercharge your career in AI and ML with Simplilearn's comprehensive courses. Gain the skills and knowledge to transform industries and unleash your true potential. Enroll now and unlock limitless possibilities!
Program Name AI Engineer Post Graduate Program In Artificial Intelligence Post Graduate Program In Artificial Intelligence Geo All Geos All Geos IN/ROW University Simplilearn Purdue Caltech Course Duration 11 Months 11 Months 11 Months Coding Experience Required Basic Basic No Skills You Will Learn 10+ skills including data structure, data manipulation, NumPy, Scikit-Learn, Tableau and more. 16+ skills including chatbots, NLP, Python, Keras and more. 8+ skills including Supervised & Unsupervised Learning Deep Learning Data Visualization, and more. Additional Benefits Get access to exclusive Hackathons, Masterclasses and Ask-Me-Anything sessions by IBM Applied learning via 3 Capstone and 12 Industry-relevant Projects Purdue Alumni Association Membership Free IIMJobs Pro-Membership of 6 months Resume Building Assistance Upto 14 CEU Credits Caltech CTME Circle Membership Cost $$ $$$$ $$$$ Explore Program Explore Program Explore Program
Hope this article assisted you in understanding the importance of statistical analysis in every sphere of life. Artificial Intelligence (AI) can help you perform statistical analysis and data analysis very effectively and efficiently.
If you are a science wizard and fascinated by statistical analysis, check out this amazing Post Graduate Program In Data Analytics in collaboration with Purdue. With a comprehensive syllabus and real-life projects, this course is one of the most popular courses and will help you with all that you need to know about Artificial Intelligence.
Data Science & Business Analytics Courses Duration and Fees
Data Science & Business Analytics programs typically range from a few weeks to several months, with fees varying based on program and institution.
Program Name | Duration | Fees |
---|---|---|
Cohort Starts: | 11 months | € 2,790 |
Cohort Starts: | 3 Months | € 1,999 |
Cohort Starts: | 32 weeks | € 1,790 |
Cohort Starts: | 11 months | € 2,290 |
Cohort Starts: | 8 months | € 2,790 |
11 Months | € 3,790 | |
11 months | € 1,099 | |
11 months | € 1,099 |
Get Free Certifications with free video courses
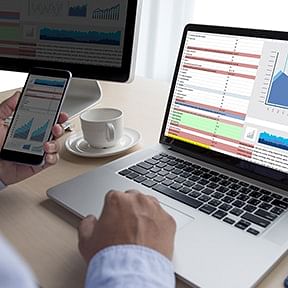
Data Science & Business Analytics
Introduction to Data Analytics Course
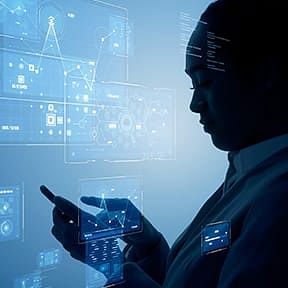
Introduction to Data Science
Learn from Industry Experts with free Masterclasses
How Can You Master the Art of Data Analysis: Uncover the Path to Career Advancement
Develop Your Career in Data Analytics with Purdue University Professional Certificate
Career Masterclass: How to Get Qualified for a Data Analytics Career
Recommended Reads
Free eBook: Guide To The CCBA And CBAP Certifications
Understanding Statistical Process Control (SPC) and Top Applications
A Complete Guide on the Types of Statistical Studies
Digital Marketing Salary Guide 2021
Top Statistical Tools For Research and Data Analysis
What Is Exploratory Data Analysis? Steps and Market Analysis
Get Affiliated Certifications with Live Class programs
- PMP, PMI, PMBOK, CAPM, PgMP, PfMP, ACP, PBA, RMP, SP, and OPM3 are registered marks of the Project Management Institute, Inc.
Frequently asked questions
What is statistical analysis.
Statistical analysis is the main method for analyzing quantitative research data . It uses probabilities and models to test predictions about a population from sample data.
Frequently asked questions: Statistics
As the degrees of freedom increase, Student’s t distribution becomes less leptokurtic , meaning that the probability of extreme values decreases. The distribution becomes more and more similar to a standard normal distribution .
The three categories of kurtosis are:
- Mesokurtosis : An excess kurtosis of 0. Normal distributions are mesokurtic.
- Platykurtosis : A negative excess kurtosis. Platykurtic distributions are thin-tailed, meaning that they have few outliers .
- Leptokurtosis : A positive excess kurtosis. Leptokurtic distributions are fat-tailed, meaning that they have many outliers.
Probability distributions belong to two broad categories: discrete probability distributions and continuous probability distributions . Within each category, there are many types of probability distributions.
Probability is the relative frequency over an infinite number of trials.
For example, the probability of a coin landing on heads is .5, meaning that if you flip the coin an infinite number of times, it will land on heads half the time.
Since doing something an infinite number of times is impossible, relative frequency is often used as an estimate of probability. If you flip a coin 1000 times and get 507 heads, the relative frequency, .507, is a good estimate of the probability.
Categorical variables can be described by a frequency distribution. Quantitative variables can also be described by a frequency distribution, but first they need to be grouped into interval classes .
A histogram is an effective way to tell if a frequency distribution appears to have a normal distribution .
Plot a histogram and look at the shape of the bars. If the bars roughly follow a symmetrical bell or hill shape, like the example below, then the distribution is approximately normally distributed.
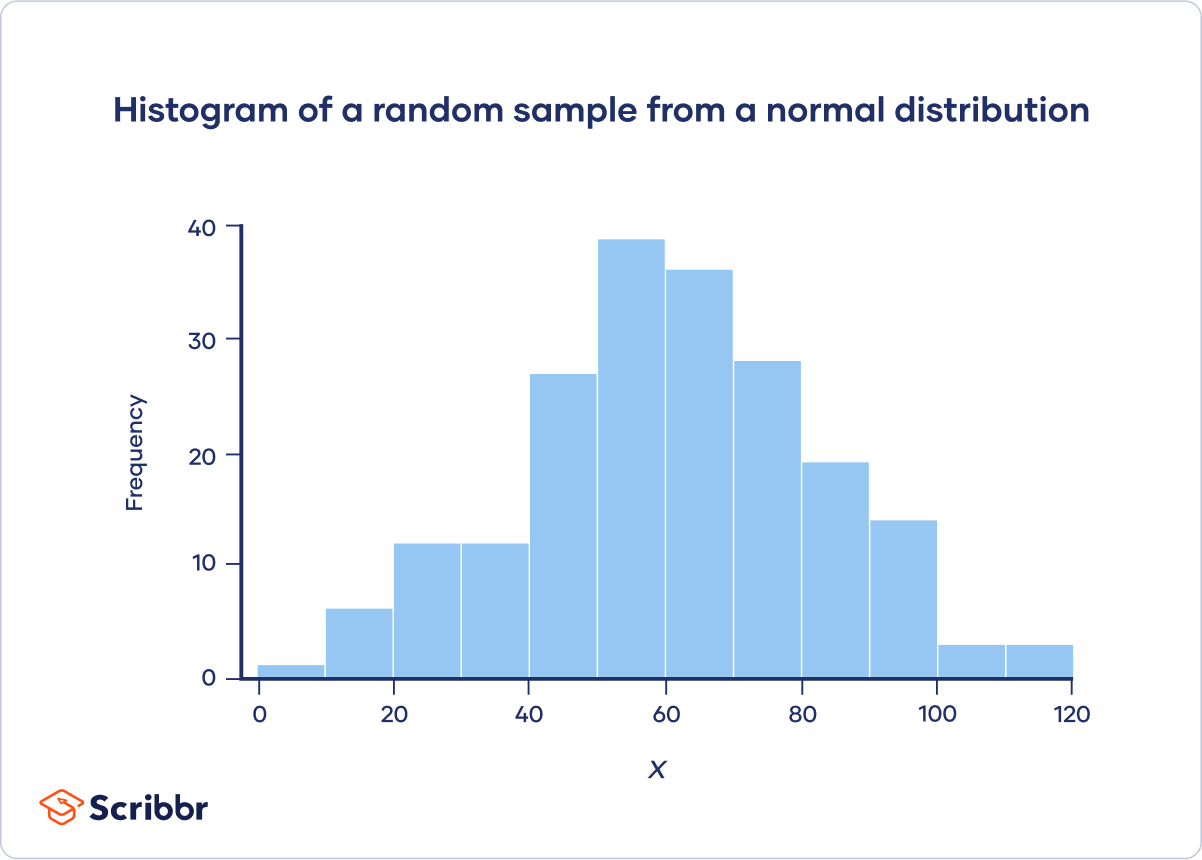
You can use the CHISQ.INV.RT() function to find a chi-square critical value in Excel.
For example, to calculate the chi-square critical value for a test with df = 22 and α = .05, click any blank cell and type:
=CHISQ.INV.RT(0.05,22)
You can use the qchisq() function to find a chi-square critical value in R.
For example, to calculate the chi-square critical value for a test with df = 22 and α = .05:
qchisq(p = .05, df = 22, lower.tail = FALSE)
You can use the chisq.test() function to perform a chi-square test of independence in R. Give the contingency table as a matrix for the “x” argument. For example:
m = matrix(data = c(89, 84, 86, 9, 8, 24), nrow = 3, ncol = 2)
chisq.test(x = m)
You can use the CHISQ.TEST() function to perform a chi-square test of independence in Excel. It takes two arguments, CHISQ.TEST(observed_range, expected_range), and returns the p value.
Chi-square goodness of fit tests are often used in genetics. One common application is to check if two genes are linked (i.e., if the assortment is independent). When genes are linked, the allele inherited for one gene affects the allele inherited for another gene.
Suppose that you want to know if the genes for pea texture (R = round, r = wrinkled) and color (Y = yellow, y = green) are linked. You perform a dihybrid cross between two heterozygous ( RY / ry ) pea plants. The hypotheses you’re testing with your experiment are:
- This would suggest that the genes are unlinked.
- This would suggest that the genes are linked.
You observe 100 peas:
- 78 round and yellow peas
- 6 round and green peas
- 4 wrinkled and yellow peas
- 12 wrinkled and green peas
Step 1: Calculate the expected frequencies
To calculate the expected values, you can make a Punnett square. If the two genes are unlinked, the probability of each genotypic combination is equal.
RRYY | RrYy | RRYy | RrYY | |
RrYy | rryy | Rryy | rrYy | |
RRYy | Rryy | RRyy | RrYy | |
RrYY | rrYy | RrYy | rrYY |
The expected phenotypic ratios are therefore 9 round and yellow: 3 round and green: 3 wrinkled and yellow: 1 wrinkled and green.
From this, you can calculate the expected phenotypic frequencies for 100 peas:
Round and yellow | 78 | 100 * (9/16) = 56.25 |
Round and green | 6 | 100 * (3/16) = 18.75 |
Wrinkled and yellow | 4 | 100 * (3/16) = 18.75 |
Wrinkled and green | 12 | 100 * (1/16) = 6.21 |
Step 2: Calculate chi-square
− | − | ||||
Round and yellow | 78 | 56.25 | 21.75 | 473.06 | 8.41 |
Round and green | 6 | 18.75 | −12.75 | 162.56 | 8.67 |
Wrinkled and yellow | 4 | 18.75 | −14.75 | 217.56 | 11.6 |
Wrinkled and green | 12 | 6.21 | 5.79 | 33.52 | 5.4 |
Χ 2 = 8.41 + 8.67 + 11.6 + 5.4 = 34.08
Step 3: Find the critical chi-square value
Since there are four groups (round and yellow, round and green, wrinkled and yellow, wrinkled and green), there are three degrees of freedom .
For a test of significance at α = .05 and df = 3, the Χ 2 critical value is 7.82.
Step 4: Compare the chi-square value to the critical value
Χ 2 = 34.08
Critical value = 7.82
The Χ 2 value is greater than the critical value .
Step 5: Decide whether the reject the null hypothesis
The Χ 2 value is greater than the critical value, so we reject the null hypothesis that the population of offspring have an equal probability of inheriting all possible genotypic combinations. There is a significant difference between the observed and expected genotypic frequencies ( p < .05).
The data supports the alternative hypothesis that the offspring do not have an equal probability of inheriting all possible genotypic combinations, which suggests that the genes are linked
You can use the chisq.test() function to perform a chi-square goodness of fit test in R. Give the observed values in the “x” argument, give the expected values in the “p” argument, and set “rescale.p” to true. For example:
chisq.test(x = c(22,30,23), p = c(25,25,25), rescale.p = TRUE)
You can use the CHISQ.TEST() function to perform a chi-square goodness of fit test in Excel. It takes two arguments, CHISQ.TEST(observed_range, expected_range), and returns the p value .
Both correlations and chi-square tests can test for relationships between two variables. However, a correlation is used when you have two quantitative variables and a chi-square test of independence is used when you have two categorical variables.
Both chi-square tests and t tests can test for differences between two groups. However, a t test is used when you have a dependent quantitative variable and an independent categorical variable (with two groups). A chi-square test of independence is used when you have two categorical variables.
The two main chi-square tests are the chi-square goodness of fit test and the chi-square test of independence .
A chi-square distribution is a continuous probability distribution . The shape of a chi-square distribution depends on its degrees of freedom , k . The mean of a chi-square distribution is equal to its degrees of freedom ( k ) and the variance is 2 k . The range is 0 to ∞.
As the degrees of freedom ( k ) increases, the chi-square distribution goes from a downward curve to a hump shape. As the degrees of freedom increases further, the hump goes from being strongly right-skewed to being approximately normal.
To find the quartiles of a probability distribution, you can use the distribution’s quantile function.
You can use the quantile() function to find quartiles in R. If your data is called “data”, then “quantile(data, prob=c(.25,.5,.75), type=1)” will return the three quartiles.
You can use the QUARTILE() function to find quartiles in Excel. If your data is in column A, then click any blank cell and type “=QUARTILE(A:A,1)” for the first quartile, “=QUARTILE(A:A,2)” for the second quartile, and “=QUARTILE(A:A,3)” for the third quartile.
You can use the PEARSON() function to calculate the Pearson correlation coefficient in Excel. If your variables are in columns A and B, then click any blank cell and type “PEARSON(A:A,B:B)”.
There is no function to directly test the significance of the correlation.
You can use the cor() function to calculate the Pearson correlation coefficient in R. To test the significance of the correlation, you can use the cor.test() function.
You should use the Pearson correlation coefficient when (1) the relationship is linear and (2) both variables are quantitative and (3) normally distributed and (4) have no outliers.
The Pearson correlation coefficient ( r ) is the most common way of measuring a linear correlation. It is a number between –1 and 1 that measures the strength and direction of the relationship between two variables.
This table summarizes the most important differences between normal distributions and Poisson distributions :
Characteristic | Normal | Poisson |
---|---|---|
Continuous | ||
Mean (µ) and standard deviation (σ) | Lambda (λ) | |
Shape | Bell-shaped | Depends on λ |
Symmetrical | Asymmetrical (right-skewed). As λ increases, the asymmetry decreases. | |
Range | −∞ to ∞ | 0 to ∞ |
When the mean of a Poisson distribution is large (>10), it can be approximated by a normal distribution.
In the Poisson distribution formula, lambda (λ) is the mean number of events within a given interval of time or space. For example, λ = 0.748 floods per year.
The e in the Poisson distribution formula stands for the number 2.718. This number is called Euler’s constant. You can simply substitute e with 2.718 when you’re calculating a Poisson probability. Euler’s constant is a very useful number and is especially important in calculus.
The three types of skewness are:
- Right skew (also called positive skew ) . A right-skewed distribution is longer on the right side of its peak than on its left.
- Left skew (also called negative skew). A left-skewed distribution is longer on the left side of its peak than on its right.
- Zero skew. It is symmetrical and its left and right sides are mirror images.
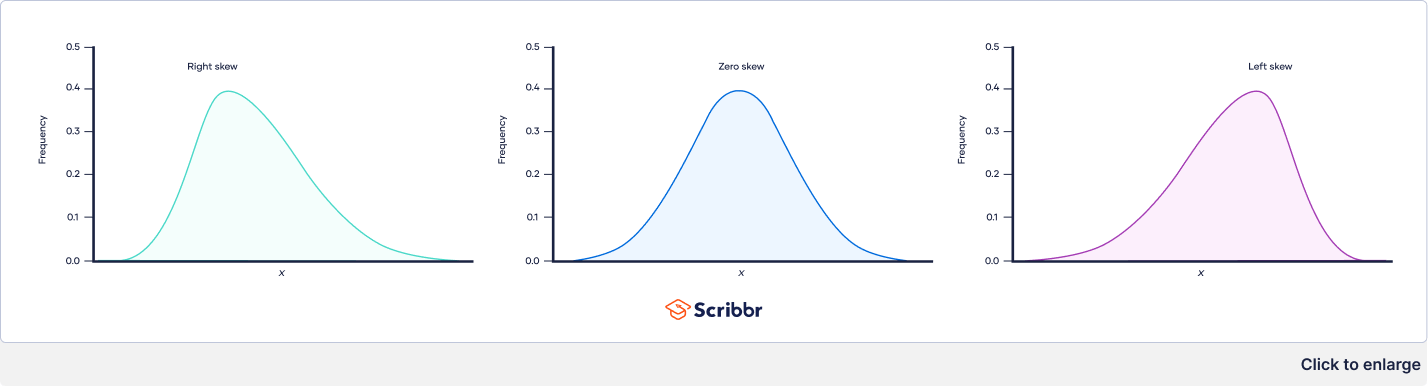
Skewness and kurtosis are both important measures of a distribution’s shape.
- Skewness measures the asymmetry of a distribution.
- Kurtosis measures the heaviness of a distribution’s tails relative to a normal distribution .
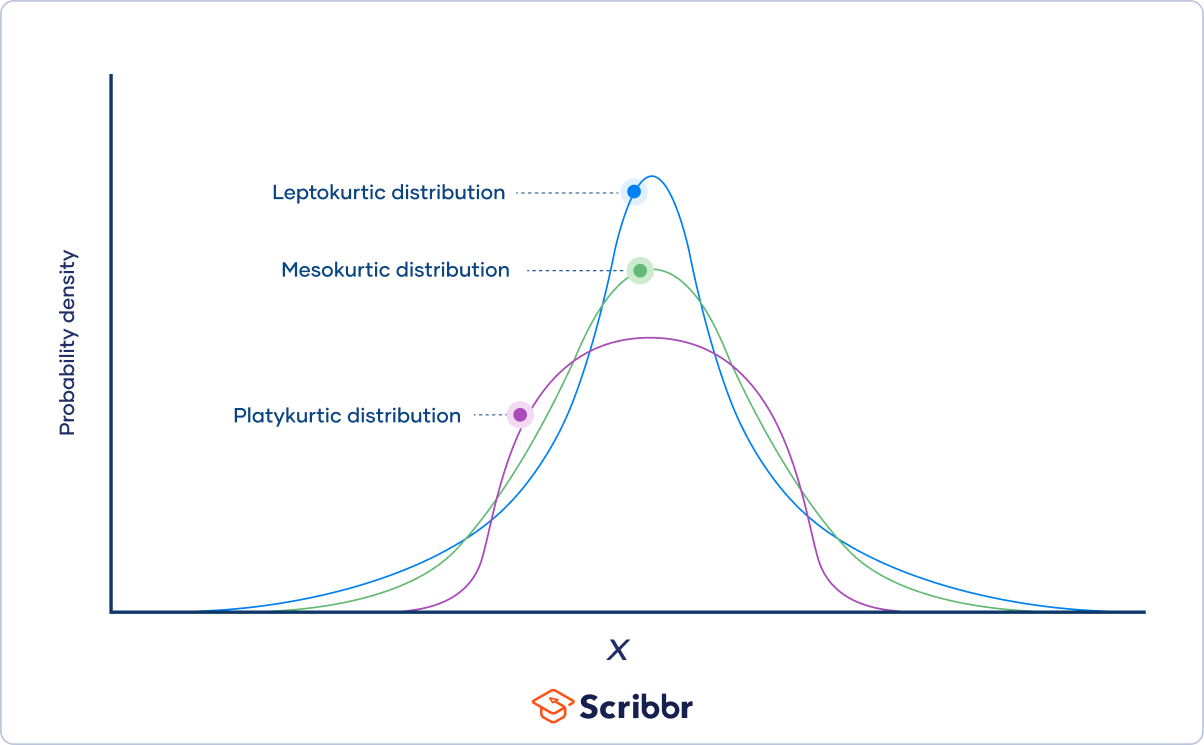
A research hypothesis is your proposed answer to your research question. The research hypothesis usually includes an explanation (“ x affects y because …”).
A statistical hypothesis, on the other hand, is a mathematical statement about a population parameter. Statistical hypotheses always come in pairs: the null and alternative hypotheses . In a well-designed study , the statistical hypotheses correspond logically to the research hypothesis.
The alternative hypothesis is often abbreviated as H a or H 1 . When the alternative hypothesis is written using mathematical symbols, it always includes an inequality symbol (usually ≠, but sometimes < or >).
The null hypothesis is often abbreviated as H 0 . When the null hypothesis is written using mathematical symbols, it always includes an equality symbol (usually =, but sometimes ≥ or ≤).
The t distribution was first described by statistician William Sealy Gosset under the pseudonym “Student.”
To calculate a confidence interval of a mean using the critical value of t , follow these four steps:
- Choose the significance level based on your desired confidence level. The most common confidence level is 95%, which corresponds to α = .05 in the two-tailed t table .
- Find the critical value of t in the two-tailed t table.
- Multiply the critical value of t by s / √ n .
- Add this value to the mean to calculate the upper limit of the confidence interval, and subtract this value from the mean to calculate the lower limit.
To test a hypothesis using the critical value of t , follow these four steps:
- Calculate the t value for your sample.
- Find the critical value of t in the t table .
- Determine if the (absolute) t value is greater than the critical value of t .
- Reject the null hypothesis if the sample’s t value is greater than the critical value of t . Otherwise, don’t reject the null hypothesis .
You can use the T.INV() function to find the critical value of t for one-tailed tests in Excel, and you can use the T.INV.2T() function for two-tailed tests.
You can use the qt() function to find the critical value of t in R. The function gives the critical value of t for the one-tailed test. If you want the critical value of t for a two-tailed test, divide the significance level by two.
You can use the RSQ() function to calculate R² in Excel. If your dependent variable is in column A and your independent variable is in column B, then click any blank cell and type “RSQ(A:A,B:B)”.
You can use the summary() function to view the R² of a linear model in R. You will see the “R-squared” near the bottom of the output.
There are two formulas you can use to calculate the coefficient of determination (R²) of a simple linear regression .

The coefficient of determination (R²) is a number between 0 and 1 that measures how well a statistical model predicts an outcome. You can interpret the R² as the proportion of variation in the dependent variable that is predicted by the statistical model.
There are three main types of missing data .
Missing completely at random (MCAR) data are randomly distributed across the variable and unrelated to other variables .
Missing at random (MAR) data are not randomly distributed but they are accounted for by other observed variables.
Missing not at random (MNAR) data systematically differ from the observed values.
To tidy up your missing data , your options usually include accepting, removing, or recreating the missing data.
- Acceptance: You leave your data as is
- Listwise or pairwise deletion: You delete all cases (participants) with missing data from analyses
- Imputation: You use other data to fill in the missing data
Missing data are important because, depending on the type, they can sometimes bias your results. This means your results may not be generalizable outside of your study because your data come from an unrepresentative sample .
Missing data , or missing values, occur when you don’t have data stored for certain variables or participants.
In any dataset, there’s usually some missing data. In quantitative research , missing values appear as blank cells in your spreadsheet.
There are two steps to calculating the geometric mean :
- Multiply all values together to get their product.
- Find the n th root of the product ( n is the number of values).
Before calculating the geometric mean, note that:
- The geometric mean can only be found for positive values.
- If any value in the data set is zero, the geometric mean is zero.
The arithmetic mean is the most commonly used type of mean and is often referred to simply as “the mean.” While the arithmetic mean is based on adding and dividing values, the geometric mean multiplies and finds the root of values.
Even though the geometric mean is a less common measure of central tendency , it’s more accurate than the arithmetic mean for percentage change and positively skewed data. The geometric mean is often reported for financial indices and population growth rates.
The geometric mean is an average that multiplies all values and finds a root of the number. For a dataset with n numbers, you find the n th root of their product.
Outliers are extreme values that differ from most values in the dataset. You find outliers at the extreme ends of your dataset.
It’s best to remove outliers only when you have a sound reason for doing so.
Some outliers represent natural variations in the population , and they should be left as is in your dataset. These are called true outliers.
Other outliers are problematic and should be removed because they represent measurement errors , data entry or processing errors, or poor sampling.
You can choose from four main ways to detect outliers :
- Sorting your values from low to high and checking minimum and maximum values
- Visualizing your data with a box plot and looking for outliers
- Using the interquartile range to create fences for your data
- Using statistical procedures to identify extreme values
Outliers can have a big impact on your statistical analyses and skew the results of any hypothesis test if they are inaccurate.
These extreme values can impact your statistical power as well, making it hard to detect a true effect if there is one.
No, the steepness or slope of the line isn’t related to the correlation coefficient value. The correlation coefficient only tells you how closely your data fit on a line, so two datasets with the same correlation coefficient can have very different slopes.
To find the slope of the line, you’ll need to perform a regression analysis .
Correlation coefficients always range between -1 and 1.
The sign of the coefficient tells you the direction of the relationship: a positive value means the variables change together in the same direction, while a negative value means they change together in opposite directions.
The absolute value of a number is equal to the number without its sign. The absolute value of a correlation coefficient tells you the magnitude of the correlation: the greater the absolute value, the stronger the correlation.
These are the assumptions your data must meet if you want to use Pearson’s r :
- Both variables are on an interval or ratio level of measurement
- Data from both variables follow normal distributions
- Your data have no outliers
- Your data is from a random or representative sample
- You expect a linear relationship between the two variables
A correlation coefficient is a single number that describes the strength and direction of the relationship between your variables.
Different types of correlation coefficients might be appropriate for your data based on their levels of measurement and distributions . The Pearson product-moment correlation coefficient (Pearson’s r ) is commonly used to assess a linear relationship between two quantitative variables.
There are various ways to improve power:
- Increase the potential effect size by manipulating your independent variable more strongly,
- Increase sample size,
- Increase the significance level (alpha),
- Reduce measurement error by increasing the precision and accuracy of your measurement devices and procedures,
- Use a one-tailed test instead of a two-tailed test for t tests and z tests.
A power analysis is a calculation that helps you determine a minimum sample size for your study. It’s made up of four main components. If you know or have estimates for any three of these, you can calculate the fourth component.
- Statistical power : the likelihood that a test will detect an effect of a certain size if there is one, usually set at 80% or higher.
- Sample size : the minimum number of observations needed to observe an effect of a certain size with a given power level.
- Significance level (alpha) : the maximum risk of rejecting a true null hypothesis that you are willing to take, usually set at 5%.
- Expected effect size : a standardized way of expressing the magnitude of the expected result of your study, usually based on similar studies or a pilot study.
Null and alternative hypotheses are used in statistical hypothesis testing . The null hypothesis of a test always predicts no effect or no relationship between variables, while the alternative hypothesis states your research prediction of an effect or relationship.
The risk of making a Type II error is inversely related to the statistical power of a test. Power is the extent to which a test can correctly detect a real effect when there is one.
To (indirectly) reduce the risk of a Type II error, you can increase the sample size or the significance level to increase statistical power.
The risk of making a Type I error is the significance level (or alpha) that you choose. That’s a value that you set at the beginning of your study to assess the statistical probability of obtaining your results ( p value ).
The significance level is usually set at 0.05 or 5%. This means that your results only have a 5% chance of occurring, or less, if the null hypothesis is actually true.
To reduce the Type I error probability, you can set a lower significance level.
In statistics, a Type I error means rejecting the null hypothesis when it’s actually true, while a Type II error means failing to reject the null hypothesis when it’s actually false.
In statistics, power refers to the likelihood of a hypothesis test detecting a true effect if there is one. A statistically powerful test is more likely to reject a false negative (a Type II error).
If you don’t ensure enough power in your study, you may not be able to detect a statistically significant result even when it has practical significance. Your study might not have the ability to answer your research question.
While statistical significance shows that an effect exists in a study, practical significance shows that the effect is large enough to be meaningful in the real world.
Statistical significance is denoted by p -values whereas practical significance is represented by effect sizes .
There are dozens of measures of effect sizes . The most common effect sizes are Cohen’s d and Pearson’s r . Cohen’s d measures the size of the difference between two groups while Pearson’s r measures the strength of the relationship between two variables .
Effect size tells you how meaningful the relationship between variables or the difference between groups is.
A large effect size means that a research finding has practical significance, while a small effect size indicates limited practical applications.
Using descriptive and inferential statistics , you can make two types of estimates about the population : point estimates and interval estimates.
- A point estimate is a single value estimate of a parameter . For instance, a sample mean is a point estimate of a population mean.
- An interval estimate gives you a range of values where the parameter is expected to lie. A confidence interval is the most common type of interval estimate.
Both types of estimates are important for gathering a clear idea of where a parameter is likely to lie.
Standard error and standard deviation are both measures of variability . The standard deviation reflects variability within a sample, while the standard error estimates the variability across samples of a population.
The standard error of the mean , or simply standard error , indicates how different the population mean is likely to be from a sample mean. It tells you how much the sample mean would vary if you were to repeat a study using new samples from within a single population.
To figure out whether a given number is a parameter or a statistic , ask yourself the following:
- Does the number describe a whole, complete population where every member can be reached for data collection ?
- Is it possible to collect data for this number from every member of the population in a reasonable time frame?
If the answer is yes to both questions, the number is likely to be a parameter. For small populations, data can be collected from the whole population and summarized in parameters.
If the answer is no to either of the questions, then the number is more likely to be a statistic.
The arithmetic mean is the most commonly used mean. It’s often simply called the mean or the average. But there are some other types of means you can calculate depending on your research purposes:
- Weighted mean: some values contribute more to the mean than others.
- Geometric mean : values are multiplied rather than summed up.
- Harmonic mean: reciprocals of values are used instead of the values themselves.
You can find the mean , or average, of a data set in two simple steps:
- Find the sum of the values by adding them all up.
- Divide the sum by the number of values in the data set.
This method is the same whether you are dealing with sample or population data or positive or negative numbers.
The median is the most informative measure of central tendency for skewed distributions or distributions with outliers. For example, the median is often used as a measure of central tendency for income distributions, which are generally highly skewed.
Because the median only uses one or two values, it’s unaffected by extreme outliers or non-symmetric distributions of scores. In contrast, the mean and mode can vary in skewed distributions.
To find the median , first order your data. Then calculate the middle position based on n , the number of values in your data set.

A data set can often have no mode, one mode or more than one mode – it all depends on how many different values repeat most frequently.
Your data can be:
- without any mode
- unimodal, with one mode,
- bimodal, with two modes,
- trimodal, with three modes, or
- multimodal, with four or more modes.
To find the mode :
- If your data is numerical or quantitative, order the values from low to high.
- If it is categorical, sort the values by group, in any order.
Then you simply need to identify the most frequently occurring value.
The interquartile range is the best measure of variability for skewed distributions or data sets with outliers. Because it’s based on values that come from the middle half of the distribution, it’s unlikely to be influenced by outliers .
The two most common methods for calculating interquartile range are the exclusive and inclusive methods.
The exclusive method excludes the median when identifying Q1 and Q3, while the inclusive method includes the median as a value in the data set in identifying the quartiles.
For each of these methods, you’ll need different procedures for finding the median, Q1 and Q3 depending on whether your sample size is even- or odd-numbered. The exclusive method works best for even-numbered sample sizes, while the inclusive method is often used with odd-numbered sample sizes.
While the range gives you the spread of the whole data set, the interquartile range gives you the spread of the middle half of a data set.
Homoscedasticity, or homogeneity of variances, is an assumption of equal or similar variances in different groups being compared.
This is an important assumption of parametric statistical tests because they are sensitive to any dissimilarities. Uneven variances in samples result in biased and skewed test results.
Statistical tests such as variance tests or the analysis of variance (ANOVA) use sample variance to assess group differences of populations. They use the variances of the samples to assess whether the populations they come from significantly differ from each other.
Variance is the average squared deviations from the mean, while standard deviation is the square root of this number. Both measures reflect variability in a distribution, but their units differ:
- Standard deviation is expressed in the same units as the original values (e.g., minutes or meters).
- Variance is expressed in much larger units (e.g., meters squared).
Although the units of variance are harder to intuitively understand, variance is important in statistical tests .
The empirical rule, or the 68-95-99.7 rule, tells you where most of the values lie in a normal distribution :
- Around 68% of values are within 1 standard deviation of the mean.
- Around 95% of values are within 2 standard deviations of the mean.
- Around 99.7% of values are within 3 standard deviations of the mean.
The empirical rule is a quick way to get an overview of your data and check for any outliers or extreme values that don’t follow this pattern.
In a normal distribution , data are symmetrically distributed with no skew. Most values cluster around a central region, with values tapering off as they go further away from the center.
The measures of central tendency (mean, mode, and median) are exactly the same in a normal distribution.
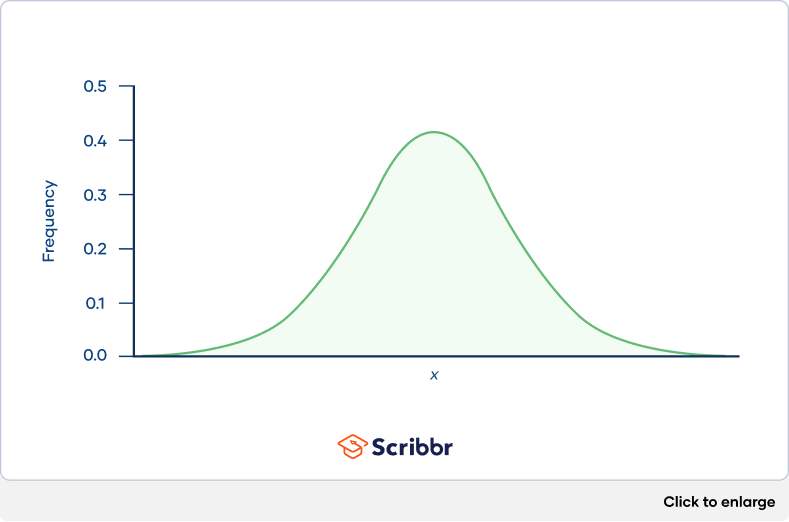
The standard deviation is the average amount of variability in your data set. It tells you, on average, how far each score lies from the mean .
In normal distributions, a high standard deviation means that values are generally far from the mean, while a low standard deviation indicates that values are clustered close to the mean.
No. Because the range formula subtracts the lowest number from the highest number, the range is always zero or a positive number.
In statistics, the range is the spread of your data from the lowest to the highest value in the distribution. It is the simplest measure of variability .
While central tendency tells you where most of your data points lie, variability summarizes how far apart your points from each other.
Data sets can have the same central tendency but different levels of variability or vice versa . Together, they give you a complete picture of your data.
Variability is most commonly measured with the following descriptive statistics :
- Range : the difference between the highest and lowest values
- Interquartile range : the range of the middle half of a distribution
- Standard deviation : average distance from the mean
- Variance : average of squared distances from the mean
Variability tells you how far apart points lie from each other and from the center of a distribution or a data set.
Variability is also referred to as spread, scatter or dispersion.
While interval and ratio data can both be categorized, ranked, and have equal spacing between adjacent values, only ratio scales have a true zero.
For example, temperature in Celsius or Fahrenheit is at an interval scale because zero is not the lowest possible temperature. In the Kelvin scale, a ratio scale, zero represents a total lack of thermal energy.
A critical value is the value of the test statistic which defines the upper and lower bounds of a confidence interval , or which defines the threshold of statistical significance in a statistical test. It describes how far from the mean of the distribution you have to go to cover a certain amount of the total variation in the data (i.e. 90%, 95%, 99%).
If you are constructing a 95% confidence interval and are using a threshold of statistical significance of p = 0.05, then your critical value will be identical in both cases.
The t -distribution gives more probability to observations in the tails of the distribution than the standard normal distribution (a.k.a. the z -distribution).
In this way, the t -distribution is more conservative than the standard normal distribution: to reach the same level of confidence or statistical significance , you will need to include a wider range of the data.
A t -score (a.k.a. a t -value) is equivalent to the number of standard deviations away from the mean of the t -distribution .
The t -score is the test statistic used in t -tests and regression tests. It can also be used to describe how far from the mean an observation is when the data follow a t -distribution.
The t -distribution is a way of describing a set of observations where most observations fall close to the mean , and the rest of the observations make up the tails on either side. It is a type of normal distribution used for smaller sample sizes, where the variance in the data is unknown.
The t -distribution forms a bell curve when plotted on a graph. It can be described mathematically using the mean and the standard deviation .
In statistics, ordinal and nominal variables are both considered categorical variables .
Even though ordinal data can sometimes be numerical, not all mathematical operations can be performed on them.
Ordinal data has two characteristics:
- The data can be classified into different categories within a variable.
- The categories have a natural ranked order.
However, unlike with interval data, the distances between the categories are uneven or unknown.
Nominal and ordinal are two of the four levels of measurement . Nominal level data can only be classified, while ordinal level data can be classified and ordered.
Nominal data is data that can be labelled or classified into mutually exclusive categories within a variable. These categories cannot be ordered in a meaningful way.
For example, for the nominal variable of preferred mode of transportation, you may have the categories of car, bus, train, tram or bicycle.
If your confidence interval for a difference between groups includes zero, that means that if you run your experiment again you have a good chance of finding no difference between groups.
If your confidence interval for a correlation or regression includes zero, that means that if you run your experiment again there is a good chance of finding no correlation in your data.
In both of these cases, you will also find a high p -value when you run your statistical test, meaning that your results could have occurred under the null hypothesis of no relationship between variables or no difference between groups.
If you want to calculate a confidence interval around the mean of data that is not normally distributed , you have two choices:
- Find a distribution that matches the shape of your data and use that distribution to calculate the confidence interval.
- Perform a transformation on your data to make it fit a normal distribution, and then find the confidence interval for the transformed data.
The standard normal distribution , also called the z -distribution, is a special normal distribution where the mean is 0 and the standard deviation is 1.
Any normal distribution can be converted into the standard normal distribution by turning the individual values into z -scores. In a z -distribution, z -scores tell you how many standard deviations away from the mean each value lies.
The z -score and t -score (aka z -value and t -value) show how many standard deviations away from the mean of the distribution you are, assuming your data follow a z -distribution or a t -distribution .
These scores are used in statistical tests to show how far from the mean of the predicted distribution your statistical estimate is. If your test produces a z -score of 2.5, this means that your estimate is 2.5 standard deviations from the predicted mean.
The predicted mean and distribution of your estimate are generated by the null hypothesis of the statistical test you are using. The more standard deviations away from the predicted mean your estimate is, the less likely it is that the estimate could have occurred under the null hypothesis .
To calculate the confidence interval , you need to know:
- The point estimate you are constructing the confidence interval for
- The critical values for the test statistic
- The standard deviation of the sample
- The sample size
Then you can plug these components into the confidence interval formula that corresponds to your data. The formula depends on the type of estimate (e.g. a mean or a proportion) and on the distribution of your data.
The confidence level is the percentage of times you expect to get close to the same estimate if you run your experiment again or resample the population in the same way.
The confidence interval consists of the upper and lower bounds of the estimate you expect to find at a given level of confidence.
For example, if you are estimating a 95% confidence interval around the mean proportion of female babies born every year based on a random sample of babies, you might find an upper bound of 0.56 and a lower bound of 0.48. These are the upper and lower bounds of the confidence interval. The confidence level is 95%.
The mean is the most frequently used measure of central tendency because it uses all values in the data set to give you an average.
For data from skewed distributions, the median is better than the mean because it isn’t influenced by extremely large values.
The mode is the only measure you can use for nominal or categorical data that can’t be ordered.
The measures of central tendency you can use depends on the level of measurement of your data.
- For a nominal level, you can only use the mode to find the most frequent value.
- For an ordinal level or ranked data, you can also use the median to find the value in the middle of your data set.
- For interval or ratio levels, in addition to the mode and median, you can use the mean to find the average value.
Measures of central tendency help you find the middle, or the average, of a data set.
The 3 most common measures of central tendency are the mean, median and mode.
- The mode is the most frequent value.
- The median is the middle number in an ordered data set.
- The mean is the sum of all values divided by the total number of values.
Some variables have fixed levels. For example, gender and ethnicity are always nominal level data because they cannot be ranked.
However, for other variables, you can choose the level of measurement . For example, income is a variable that can be recorded on an ordinal or a ratio scale:
- At an ordinal level , you could create 5 income groupings and code the incomes that fall within them from 1–5.
- At a ratio level , you would record exact numbers for income.
If you have a choice, the ratio level is always preferable because you can analyze data in more ways. The higher the level of measurement, the more precise your data is.
The level at which you measure a variable determines how you can analyze your data.
Depending on the level of measurement , you can perform different descriptive statistics to get an overall summary of your data and inferential statistics to see if your results support or refute your hypothesis .
Levels of measurement tell you how precisely variables are recorded. There are 4 levels of measurement, which can be ranked from low to high:
- Nominal : the data can only be categorized.
- Ordinal : the data can be categorized and ranked.
- Interval : the data can be categorized and ranked, and evenly spaced.
- Ratio : the data can be categorized, ranked, evenly spaced and has a natural zero.
No. The p -value only tells you how likely the data you have observed is to have occurred under the null hypothesis .
If the p -value is below your threshold of significance (typically p < 0.05), then you can reject the null hypothesis, but this does not necessarily mean that your alternative hypothesis is true.
The alpha value, or the threshold for statistical significance , is arbitrary – which value you use depends on your field of study.
In most cases, researchers use an alpha of 0.05, which means that there is a less than 5% chance that the data being tested could have occurred under the null hypothesis.
P -values are usually automatically calculated by the program you use to perform your statistical test. They can also be estimated using p -value tables for the relevant test statistic .
P -values are calculated from the null distribution of the test statistic. They tell you how often a test statistic is expected to occur under the null hypothesis of the statistical test, based on where it falls in the null distribution.
If the test statistic is far from the mean of the null distribution, then the p -value will be small, showing that the test statistic is not likely to have occurred under the null hypothesis.
A p -value , or probability value, is a number describing how likely it is that your data would have occurred under the null hypothesis of your statistical test .
The test statistic you use will be determined by the statistical test.
You can choose the right statistical test by looking at what type of data you have collected and what type of relationship you want to test.
The test statistic will change based on the number of observations in your data, how variable your observations are, and how strong the underlying patterns in the data are.
For example, if one data set has higher variability while another has lower variability, the first data set will produce a test statistic closer to the null hypothesis , even if the true correlation between two variables is the same in either data set.
The formula for the test statistic depends on the statistical test being used.
Generally, the test statistic is calculated as the pattern in your data (i.e. the correlation between variables or difference between groups) divided by the variance in the data (i.e. the standard deviation ).
- Univariate statistics summarize only one variable at a time.
- Bivariate statistics compare two variables .
- Multivariate statistics compare more than two variables .
The 3 main types of descriptive statistics concern the frequency distribution, central tendency, and variability of a dataset.
- Distribution refers to the frequencies of different responses.
- Measures of central tendency give you the average for each response.
- Measures of variability show you the spread or dispersion of your dataset.
Descriptive statistics summarize the characteristics of a data set. Inferential statistics allow you to test a hypothesis or assess whether your data is generalizable to the broader population.
In statistics, model selection is a process researchers use to compare the relative value of different statistical models and determine which one is the best fit for the observed data.
The Akaike information criterion is one of the most common methods of model selection. AIC weights the ability of the model to predict the observed data against the number of parameters the model requires to reach that level of precision.
AIC model selection can help researchers find a model that explains the observed variation in their data while avoiding overfitting.
In statistics, a model is the collection of one or more independent variables and their predicted interactions that researchers use to try to explain variation in their dependent variable.
You can test a model using a statistical test . To compare how well different models fit your data, you can use Akaike’s information criterion for model selection.
The Akaike information criterion is calculated from the maximum log-likelihood of the model and the number of parameters (K) used to reach that likelihood. The AIC function is 2K – 2(log-likelihood) .
Lower AIC values indicate a better-fit model, and a model with a delta-AIC (the difference between the two AIC values being compared) of more than -2 is considered significantly better than the model it is being compared to.
The Akaike information criterion is a mathematical test used to evaluate how well a model fits the data it is meant to describe. It penalizes models which use more independent variables (parameters) as a way to avoid over-fitting.
AIC is most often used to compare the relative goodness-of-fit among different models under consideration and to then choose the model that best fits the data.
A factorial ANOVA is any ANOVA that uses more than one categorical independent variable . A two-way ANOVA is a type of factorial ANOVA.
Some examples of factorial ANOVAs include:
- Testing the combined effects of vaccination (vaccinated or not vaccinated) and health status (healthy or pre-existing condition) on the rate of flu infection in a population.
- Testing the effects of marital status (married, single, divorced, widowed), job status (employed, self-employed, unemployed, retired), and family history (no family history, some family history) on the incidence of depression in a population.
- Testing the effects of feed type (type A, B, or C) and barn crowding (not crowded, somewhat crowded, very crowded) on the final weight of chickens in a commercial farming operation.
In ANOVA, the null hypothesis is that there is no difference among group means. If any group differs significantly from the overall group mean, then the ANOVA will report a statistically significant result.
Significant differences among group means are calculated using the F statistic, which is the ratio of the mean sum of squares (the variance explained by the independent variable) to the mean square error (the variance left over).
If the F statistic is higher than the critical value (the value of F that corresponds with your alpha value, usually 0.05), then the difference among groups is deemed statistically significant.
The only difference between one-way and two-way ANOVA is the number of independent variables . A one-way ANOVA has one independent variable, while a two-way ANOVA has two.
- One-way ANOVA : Testing the relationship between shoe brand (Nike, Adidas, Saucony, Hoka) and race finish times in a marathon.
- Two-way ANOVA : Testing the relationship between shoe brand (Nike, Adidas, Saucony, Hoka), runner age group (junior, senior, master’s), and race finishing times in a marathon.
All ANOVAs are designed to test for differences among three or more groups. If you are only testing for a difference between two groups, use a t-test instead.
Multiple linear regression is a regression model that estimates the relationship between a quantitative dependent variable and two or more independent variables using a straight line.
Linear regression most often uses mean-square error (MSE) to calculate the error of the model. MSE is calculated by:
- measuring the distance of the observed y-values from the predicted y-values at each value of x;
- squaring each of these distances;
- calculating the mean of each of the squared distances.
Linear regression fits a line to the data by finding the regression coefficient that results in the smallest MSE.
Simple linear regression is a regression model that estimates the relationship between one independent variable and one dependent variable using a straight line. Both variables should be quantitative.
For example, the relationship between temperature and the expansion of mercury in a thermometer can be modeled using a straight line: as temperature increases, the mercury expands. This linear relationship is so certain that we can use mercury thermometers to measure temperature.
A regression model is a statistical model that estimates the relationship between one dependent variable and one or more independent variables using a line (or a plane in the case of two or more independent variables).
A regression model can be used when the dependent variable is quantitative, except in the case of logistic regression, where the dependent variable is binary.
A t-test should not be used to measure differences among more than two groups, because the error structure for a t-test will underestimate the actual error when many groups are being compared.
If you want to compare the means of several groups at once, it’s best to use another statistical test such as ANOVA or a post-hoc test.
A one-sample t-test is used to compare a single population to a standard value (for example, to determine whether the average lifespan of a specific town is different from the country average).
A paired t-test is used to compare a single population before and after some experimental intervention or at two different points in time (for example, measuring student performance on a test before and after being taught the material).
A t-test measures the difference in group means divided by the pooled standard error of the two group means.
In this way, it calculates a number (the t-value) illustrating the magnitude of the difference between the two group means being compared, and estimates the likelihood that this difference exists purely by chance (p-value).
Your choice of t-test depends on whether you are studying one group or two groups, and whether you care about the direction of the difference in group means.
If you are studying one group, use a paired t-test to compare the group mean over time or after an intervention, or use a one-sample t-test to compare the group mean to a standard value. If you are studying two groups, use a two-sample t-test .
If you want to know only whether a difference exists, use a two-tailed test . If you want to know if one group mean is greater or less than the other, use a left-tailed or right-tailed one-tailed test .
A t-test is a statistical test that compares the means of two samples . It is used in hypothesis testing , with a null hypothesis that the difference in group means is zero and an alternate hypothesis that the difference in group means is different from zero.
Statistical significance is a term used by researchers to state that it is unlikely their observations could have occurred under the null hypothesis of a statistical test . Significance is usually denoted by a p -value , or probability value.
Statistical significance is arbitrary – it depends on the threshold, or alpha value, chosen by the researcher. The most common threshold is p < 0.05, which means that the data is likely to occur less than 5% of the time under the null hypothesis .
When the p -value falls below the chosen alpha value, then we say the result of the test is statistically significant.
A test statistic is a number calculated by a statistical test . It describes how far your observed data is from the null hypothesis of no relationship between variables or no difference among sample groups.
The test statistic tells you how different two or more groups are from the overall population mean , or how different a linear slope is from the slope predicted by a null hypothesis . Different test statistics are used in different statistical tests.
Statistical tests commonly assume that:
- the data are normally distributed
- the groups that are being compared have similar variance
- the data are independent
If your data does not meet these assumptions you might still be able to use a nonparametric statistical test , which have fewer requirements but also make weaker inferences.
Ask our team
Want to contact us directly? No problem. We are always here for you.
- Email [email protected]
- Start live chat
- Call +1 (510) 822-8066
- WhatsApp +31 20 261 6040
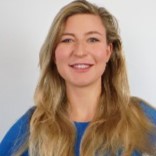
Our team helps students graduate by offering:
- A world-class citation generator
- Plagiarism Checker software powered by Turnitin
- Innovative Citation Checker software
- Professional proofreading services
- Over 300 helpful articles about academic writing, citing sources, plagiarism, and more
Scribbr specializes in editing study-related documents . We proofread:
- PhD dissertations
- Research proposals
- Personal statements
- Admission essays
- Motivation letters
- Reflection papers
- Journal articles
- Capstone projects
Scribbr’s Plagiarism Checker is powered by elements of Turnitin’s Similarity Checker , namely the plagiarism detection software and the Internet Archive and Premium Scholarly Publications content databases .
The add-on AI detector is powered by Scribbr’s proprietary software.
The Scribbr Citation Generator is developed using the open-source Citation Style Language (CSL) project and Frank Bennett’s citeproc-js . It’s the same technology used by dozens of other popular citation tools, including Mendeley and Zotero.
You can find all the citation styles and locales used in the Scribbr Citation Generator in our publicly accessible repository on Github .
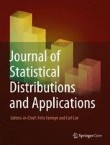
- Search by keyword
- Search by citation
Page 1 of 3
A generalization to the log-inverse Weibull distribution and its applications in cancer research
In this paper we consider a generalization of a log-transformed version of the inverse Weibull distribution. Several theoretical properties of the distribution are studied in detail including expressions for i...
- View Full Text
Approximations of conditional probability density functions in Lebesgue spaces via mixture of experts models
Mixture of experts (MoE) models are widely applied for conditional probability density estimation problems. We demonstrate the richness of the class of MoE models by proving denseness results in Lebesgue space...
Structural properties of generalised Planck distributions
A family of generalised Planck (GP) laws is defined and its structural properties explored. Sometimes subject to parameter restrictions, a GP law is a randomly scaled gamma law; it arises as the equilibrium la...
New class of Lindley distributions: properties and applications
A new generalized class of Lindley distribution is introduced in this paper. This new class is called the T -Lindley{ Y } class of distributions, and it is generated by using the quantile functions of uniform, expon...
Tolerance intervals in statistical software and robustness under model misspecification
A tolerance interval is a statistical interval that covers at least 100 ρ % of the population of interest with a 100(1− α ) % confidence, where ρ and α are pre-specified values in (0, 1). In many scientific fields, su...
Combining assumptions and graphical network into gene expression data analysis
Analyzing gene expression data rigorously requires taking assumptions into consideration but also relies on using information about network relations that exist among genes. Combining these different elements ...
A comparison of zero-inflated and hurdle models for modeling zero-inflated count data
Counts data with excessive zeros are frequently encountered in practice. For example, the number of health services visits often includes many zeros representing the patients with no utilization during a follo...
A general stochastic model for bivariate episodes driven by a gamma sequence
We propose a new stochastic model describing the joint distribution of ( X , N ), where N is a counting variable while X is the sum of N independent gamma random variables. We present the main properties of this gene...
A flexible multivariate model for high-dimensional correlated count data
We propose a flexible multivariate stochastic model for over-dispersed count data. Our methodology is built upon mixed Poisson random vectors ( Y 1 ,…, Y d ), where the { Y i } are conditionally independent Poisson random...
Generalized fiducial inference on the mean of zero-inflated Poisson and Poisson hurdle models
Zero-inflated and hurdle models are widely applied to count data possessing excess zeros, where they can simultaneously model the process from how the zeros were generated and potentially help mitigate the eff...
Multivariate distributions of correlated binary variables generated by pair-copulas
Correlated binary data are prevalent in a wide range of scientific disciplines, including healthcare and medicine. The generalized estimating equations (GEEs) and the multivariate probit (MP) model are two of ...
On two extensions of the canonical Feller–Spitzer distribution
We introduce two extensions of the canonical Feller–Spitzer distribution from the class of Bessel densities, which comprise two distinct stochastically decreasing one-parameter families of positive absolutely ...
A new trivariate model for stochastic episodes
We study the joint distribution of stochastic events described by ( X , Y , N ), where N has a 1-inflated (or deflated) geometric distribution and X , Y are the sum and the maximum of N exponential random variables. Mod...
A flexible univariate moving average time-series model for dispersed count data
Al-Osh and Alzaid ( 1988 ) consider a Poisson moving average (PMA) model to describe the relation among integer-valued time series data; this model, however, is constrained by the underlying equi-dispersion assumpt...
Spatio-temporal analysis of flood data from South Carolina
To investigate the relationship between flood gage height and precipitation in South Carolina from 2012 to 2016, we built a conditional autoregressive (CAR) model using a Bayesian hierarchical framework. This ...
Affine-transformation invariant clustering models
We develop a cluster process which is invariant with respect to unknown affine transformations of the feature space without knowing the number of clusters in advance. Specifically, our proposed method can iden...
Distributions associated with simultaneous multiple hypothesis testing
We develop the distribution for the number of hypotheses found to be statistically significant using the rule from Simes (Biometrika 73: 751–754, 1986) for controlling the family-wise error rate (FWER). We fin...
New families of bivariate copulas via unit weibull distortion
This paper introduces a new family of bivariate copulas constructed using a unit Weibull distortion. Existing copulas play the role of the base or initial copulas that are transformed or distorted into a new f...
Generalized logistic distribution and its regression model
A new generalized asymmetric logistic distribution is defined. In some cases, existing three parameter distributions provide poor fit to heavy tailed data sets. The proposed new distribution consists of only t...
The spherical-Dirichlet distribution
Today, data mining and gene expressions are at the forefront of modern data analysis. Here we introduce a novel probability distribution that is applicable in these fields. This paper develops the proposed sph...
Item fit statistics for Rasch analysis: can we trust them?
To compare fit statistics for the Rasch model based on estimates of unconditional or conditional response probabilities.
Exact distributions of statistics for making inferences on mixed models under the default covariance structure
At this juncture when mixed models are heavily employed in applications ranging from clinical research to business analytics, the purpose of this article is to extend the exact distributional result of Wald (A...
A new discrete pareto type (IV) model: theory, properties and applications
Discrete analogue of a continuous distribution (especially in the univariate domain) is not new in the literature. The work of discretizing continuous distributions begun with the paper by Nakagawa and Osaki (197...
Density deconvolution for generalized skew-symmetric distributions
The density deconvolution problem is considered for random variables assumed to belong to the generalized skew-symmetric (GSS) family of distributions. The approach is semiparametric in that the symmetric comp...
The unifed distribution
We introduce a new distribution with support on (0,1) called unifed. It can be used as the response distribution for a GLM and it is suitable for data aggregation. We make a comparison to the beta regression. ...
On Burr III Marshal Olkin family: development, properties, characterizations and applications
In this paper, a flexible family of distributions with unimodel, bimodal, increasing, increasing and decreasing, inverted bathtub and modified bathtub hazard rate called Burr III-Marshal Olkin-G (BIIIMO-G) fam...
The linearly decreasing stress Weibull (LDSWeibull): a new Weibull-like distribution
Motivated by an engineering pullout test applied to a steel strip embedded in earth, we show how the resulting linearly decreasing force leads naturally to a new distribution, if the force under constant stress i...
Meta analysis of binary data with excessive zeros in two-arm trials
We present a novel Bayesian approach to random effects meta analysis of binary data with excessive zeros in two-arm trials. We discuss the development of likelihood accounting for excessive zeros, the prior, a...
On ( p 1 ,…, p k )-spherical distributions
The class of ( p 1 ,…, p k )-spherical probability laws and a method of simulating random vectors following such distributions are introduced using a new stochastic vector representation. A dynamic geometric disintegra...
A new class of survival distribution for degradation processes subject to shocks
Many systems experience gradual degradation while simultaneously being exposed to a stream of random shocks of varying magnitudes that eventually cause failure when a shock exceeds the residual strength of the...
A new extended normal regression model: simulations and applications
Various applications in natural science require models more accurate than well-known distributions. In this context, several generators of distributions have been recently proposed. We introduce a new four-par...
Multiclass analysis and prediction with network structured covariates
Technological advances associated with data acquisition are leading to the production of complex structured data sets. The recent development on classification with multiclass responses makes it possible to in...
High-dimensional star-shaped distributions
Stochastic representations of star-shaped distributed random vectors having heavy or light tail density generating function g are studied for increasing dimensions along with corresponding geometric measure repre...
A unified complex noncentral Wishart type distribution inspired by massive MIMO systems
The eigenvalue distributions from a complex noncentral Wishart matrix S = X H X has been the subject of interest in various real world applications, where X is assumed to be complex matrix variate normally distribute...
Particle swarm based algorithms for finding locally and Bayesian D -optimal designs
When a model-based approach is appropriate, an optimal design can guide how to collect data judiciously for making reliable inference at minimal cost. However, finding optimal designs for a statistical model w...
Admissible Bernoulli correlations
A multivariate symmetric Bernoulli distribution has marginals that are uniform over the pair {0,1}. Consider the problem of sampling from this distribution given a prescribed correlation between each pair of v...
On p -generalized elliptical random processes
We introduce rank- k -continuous axis-aligned p -generalized elliptically contoured distributions and study their properties such as stochastic representations, moments, and density-like representations. Applying th...
Parameters of stochastic models for electroencephalogram data as biomarkers for child’s neurodevelopment after cerebral malaria
The objective of this study was to test statistical features from the electroencephalogram (EEG) recordings as predictors of neurodevelopment and cognition of Ugandan children after coma due to cerebral malari...
A new generalization of generalized half-normal distribution: properties and regression models
In this paper, a new extension of the generalized half-normal distribution is introduced and studied. We assess the performance of the maximum likelihood estimators of the parameters of the new distribution vi...
Analytical properties of generalized Gaussian distributions
The family of Generalized Gaussian (GG) distributions has received considerable attention from the engineering community, due to the flexible parametric form of its probability density function, in modeling ma...
A new Weibull- X family of distributions: properties, characterizations and applications
We propose a new family of univariate distributions generated from the Weibull random variable, called a new Weibull-X family of distributions. Two special sub-models of the proposed family are presented and t...
The transmuted geometric-quadratic hazard rate distribution: development, properties, characterizations and applications
We propose a five parameter transmuted geometric quadratic hazard rate (TG-QHR) distribution derived from mixture of quadratic hazard rate (QHR), geometric and transmuted distributions via the application of t...
A nonparametric approach for quantile regression
Quantile regression estimates conditional quantiles and has wide applications in the real world. Estimating high conditional quantiles is an important problem. The regular quantile regression (QR) method often...
Mean and variance of ratios of proportions from categories of a multinomial distribution
Ratio distribution is a probability distribution representing the ratio of two random variables, each usually having a known distribution. Currently, there are results when the random variables in the ratio fo...
The power-Cauchy negative-binomial: properties and regression
We propose and study a new compounded model to extend the half-Cauchy and power-Cauchy distributions, which offers more flexibility in modeling lifetime data. The proposed model is analytically tractable and c...
Families of distributions arising from the quantile of generalized lambda distribution
In this paper, the class of T-R { generalized lambda } families of distributions based on the quantile of generalized lambda distribution has been proposed using the T-R { Y } framework. In the development of the T - R {
Risk ratios and Scanlan’s HRX
Risk ratios are distribution function tail ratios and are widely used in health disparities research. Let A and D denote advantaged and disadvantaged populations with cdfs F ...
Joint distribution of k -tuple statistics in zero-one sequences of Markov-dependent trials
We consider a sequence of n , n ≥3, zero (0) - one (1) Markov-dependent trials. We focus on k -tuples of 1s; i.e. runs of 1s of length at least equal to a fixed integer number k , 1≤ k ≤ n . The statistics denoting the n...
Quantile regression for overdispersed count data: a hierarchical method
Generalized Poisson regression is commonly applied to overdispersed count data, and focused on modelling the conditional mean of the response. However, conditional mean regression models may be sensitive to re...
Describing the Flexibility of the Generalized Gamma and Related Distributions
The generalized gamma (GG) distribution is a widely used, flexible tool for parametric survival analysis. Many alternatives and extensions to this family have been proposed. This paper characterizes the flexib...
- ISSN: 2195-5832 (electronic)
Social Security
Research, statistics & policy analysis, monthly statistical snapshot, july 2024.
Next Expected Update
Subscribe to Updates
Publishing Schedule
Download snapshot (0.8 MB )
Download all tables (0.2 MB )
Table of Contents
Type of beneficiary | Total | Social Security only | only | Both Social Security and |
---|---|---|---|---|
All beneficiaries | 72,452 | 65,043 | 4,902 | 2,507 |
Aged 65 or older | 55,479 | 53,041 | 1,052 | 1,386 |
Disabled, under age 65 | 11,414 | 6,442 | 3,851 | 1,121 |
Other | 5,560 | 5,560 | . . . | . . . |
SOURCES: Social Security Administration, Master Beneficiary Record and Supplemental Security Record, 100 percent data. | ||||
NOTES: Social Security beneficiaries who are entitled to a primary and a secondary benefit (dual entitlement) are counted only once in this table. counts include recipients of federal , federally administered state supplementation, or both. | ||||
. . . = not applicable. | ||||
a. Includes children receiving based on their own disability. | ||||
b. Social Security beneficiaries who are neither aged nor disabled (for example, early retirees, young survivors). | ||||
CONTACT: . |
For detailed annual and time series program data, see our Annual Statistical Supplement .
Type of beneficiary | Beneficiaries | Total monthly benefits (millions of dollars) | Average monthly benefit (dollars) | |
---|---|---|---|---|
Number (thousands) | Percent | |||
Total | 67,945 | 100.0 | 121,129 | 1,782.74 |
Old-Age and Survivors Insurance | 59,615 | 87.7 | 109,456 | 1,836.04 |
Retirement benefits | 53,837 | 79.2 | 100,733 | 1,871.09 |
Retired workers | 51,271 | 75.5 | 98,410 | 1,919.40 |
Spouses of retired workers | 1,878 | 2.8 | 1,710 | 910.42 |
Children of retired workers | 687 | 1.0 | 614 | 892.39 |
Survivor benefits | 5,779 | 8.5 | 8,723 | 1,509.50 |
Children of deceased workers | 2,009 | 3.0 | 2,217 | 1,103.54 |
Widowed mothers and fathers | 103 | 0.2 | 132 | 1,284.42 |
Nondisabled widow(er)s | 3,469 | 5.1 | 6,189 | 1,784.31 |
Disabled widow(er)s | 197 | 0.3 | 183 | 928.25 |
Parents of deceased workers | 1 | (L) | 1 | 1,612.94 |
Disability Insurance | 8,330 | 12.3 | 11,673 | 1,401.30 |
Disabled workers | 7,242 | 10.7 | 11,144 | 1,538.85 |
Spouses of disabled workers | 86 | 0.1 | 36 | 421.34 |
Children of disabled workers | 1,003 | 1.5 | 493 | 491.41 |
SOURCE: Social Security Administration, Master Beneficiary Record, 100 percent data. | ||||
NOTE: (L) = less than 0.05 percent. | ||||
CONTACT: . |
For more detailed monthly OASDI information, visit the Actuary's website for Beneficiary Data .
Age | Recipients | Total payments (millions of dollars) | Average monthly payment (dollars) | |
---|---|---|---|---|
Number (thousands) | Percent | |||
All recipients | 7,410 | 100.0 | 5,461 | 695.84 |
Under 18 | 992 | 13.4 | 877 | 805.39 |
18–64 | 3,979 | 53.7 | 3,172 | 742.70 |
65 or older | 2,438 | 32.9 | 1,412 | 574.90 |
SOURCE: Social Security Administration, Supplemental Security Record, 100 percent data. | ||||
a. Includes retroactive payments. | ||||
b. Excludes retroactive payments. | ||||
CONTACT: . |
For more detailed monthly SSI information, see SSI Monthly Statistics .
Information
- Author Services
Initiatives
You are accessing a machine-readable page. In order to be human-readable, please install an RSS reader.
All articles published by MDPI are made immediately available worldwide under an open access license. No special permission is required to reuse all or part of the article published by MDPI, including figures and tables. For articles published under an open access Creative Common CC BY license, any part of the article may be reused without permission provided that the original article is clearly cited. For more information, please refer to https://www.mdpi.com/openaccess .
Feature papers represent the most advanced research with significant potential for high impact in the field. A Feature Paper should be a substantial original Article that involves several techniques or approaches, provides an outlook for future research directions and describes possible research applications.
Feature papers are submitted upon individual invitation or recommendation by the scientific editors and must receive positive feedback from the reviewers.
Editor’s Choice articles are based on recommendations by the scientific editors of MDPI journals from around the world. Editors select a small number of articles recently published in the journal that they believe will be particularly interesting to readers, or important in the respective research area. The aim is to provide a snapshot of some of the most exciting work published in the various research areas of the journal.
Original Submission Date Received: .
- Active Journals
- Find a Journal
- Proceedings Series
- For Authors
- For Reviewers
- For Editors
- For Librarians
- For Publishers
- For Societies
- For Conference Organizers
- Open Access Policy
- Institutional Open Access Program
- Special Issues Guidelines
- Editorial Process
- Research and Publication Ethics
- Article Processing Charges
- Testimonials
- Preprints.org
- SciProfiles
- Encyclopedia
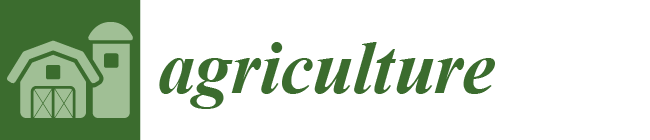
Article Menu

- Subscribe SciFeed
- Recommended Articles
- Google Scholar
- on Google Scholar
- Table of Contents
Find support for a specific problem in the support section of our website.
Please let us know what you think of our products and services.
Visit our dedicated information section to learn more about MDPI.
JSmol Viewer
Global trends and current advances in slow/controlled-release fertilizers: a bibliometric analysis from 1990 to 2023.
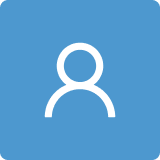
1. Introduction
- Provide a concise overview of the predominant types of SRFs/CRFs in contemporary use while detailing the challenges associated with these specific categories of fertilizers. Additionally, it highlights potential future developments in preparation techniques and emerging materials in the field.
- Generate and visualize the publication trends regarding the literature associated with SRFs/CRFs.
- Examine the collaborative networks among leading groups, as characterized by their geographic distribution, prominent collaborations, and highly productive authors in the field.
- Examine the research focus and evolution of slow-release and controlled-release fertilizers (SRFs/CRFs) from 1990 to 2023.
- Identify current research limitations and explore potential future directions in the field of SRFs/CRFs.
2. Methodology
2.1. database and search strategy, 2.2. data analysis, 3.1. publication trends, 3.2. subject categories, 3.3. contribution of countries and institutions, 3.4. contribution of journals, 3.5. contribution of authors, 3.6. highly impactful and highly cited publications, 3.7. keyword analysis, 3.7.1. high-frequency keywords, 3.7.2. keywords co-occurrence analysis, 3.7.3. keyword burst analysis, 4. challenges and future research prospects, 4.1. emerging preparation techniques and materials for srfs/crfs, 4.2. mechanism and model simulation of nutrient release from srfs/crfs, 5. conclusions and limitations, author contributions, data availability statement, conflicts of interest.
- El-Naggar, A.; Lee, S.S.; Rinklebe, J.; Farooq, M.; Song, H.; Sarmah, A.K.; Zimmerman, A.R.; Ahmad, M.; Shaheen, S.M.; Ok, Y.S. Biochar application to low fertility soils: A review of current status, and future prospects. Geoderma 2019 , 337 , 536–554. [ Google Scholar ] [ CrossRef ]
- Sim, D.H.H.; Tan, I.A.W.; Lim, L.L.P.; Hameed, B.H. Encapsulated biochar-based sustained release fertilizer for precision agriculture: A review. J. Clean. Prod. 2021 , 303 , 127018. [ Google Scholar ] [ CrossRef ]
- Cole, M.B.; Augustin, M.A.; Robertson, M.J.; Manners, J.M. The science of food security. npj Sci. Food 2018 , 2 , 14. [ Google Scholar ] [ CrossRef ] [ PubMed ]
- Wesolowska, M.; Rymarczyk, J.; Gora, R.; Baranowski, P.; Slawinski, C.; Klimczyk, M.; Supryn, G.; Schimmelpfennig, L. New slow-release fertilizers—Economic, legal and practical aspects: A Review. Int. Agrophys. 2021 , 35 , 11–24. [ Google Scholar ] [ CrossRef ]
- Bi, S.; Barinelli, V.; Sobkowicz, M.J. Degradable controlled release fertilizer composite prepared via extrusion: Fabrication, characterization, and release mechanisms. Polymers 2020 , 12 , 301. [ Google Scholar ] [ CrossRef ]
- Li, Y.; Zhang, W.; Ma, L.; Huang, G.; Oenema, O.; Zhang, F.; Dou, Z. An analysis of China’s fertilizer policies: Impacts on the industry, food security, and the environment. J. Environ. Qual. 2013 , 42 , 972–981. [ Google Scholar ] [ CrossRef ]
- Timilsena, Y.P.; Adhikari, R.; Casey, P.; Muster, T.; Gill, H.; Adhikari, B. Enhanced efficiency fertilisers: A review of formulation and nutrient release patterns. J. Sci. Food Agric. 2015 , 95 , 1131–1142. [ Google Scholar ] [ CrossRef ]
- Fu, J.; Wang, C.; Chen, X.; Huang, Z.; Chen, D. Classification research and types of slow controlled release fertilizers (SRFs) used—A review. Commun. Soil Sci. Plant Anal. 2018 , 49 , 2219–2230. [ Google Scholar ] [ CrossRef ]
- Liu, Y.; Wang, J.; Chen, H.; Cheng, D. Environmentally friendly hydrogel: A review of classification, preparation and application in agriculture. Sci. Total Environ. 2022 , 846 , 157303. [ Google Scholar ] [ CrossRef ] [ PubMed ]
- Beig, B.; Niazi, M.B.K.; Jahan, Z.; Hussain, A.; Zia, M.H.; Mehran, M.T. Coating materials for slow release of nitrogen from urea fertilizer: A review. J. Plant Nutr. 2020 , 43 , 1510–1533. [ Google Scholar ] [ CrossRef ]
- Chen, J.; Fan, X.; Zhang, L.; Chen, X.; Sun, S.; Sun, R.C. Research progress in lignin-based slow/controlled release fertilizer. ChemSusChem 2020 , 13 , 4356–4366. [ Google Scholar ] [ CrossRef ]
- Li, X.; Li, Z.; Du, C.; Tian, Z.; Zhu, Q.; Li, G.; Shen, Q.; Li, C.; Li, J.; Li, W.; et al. Bibliometric analysis of zerovalent iron particles research for environmental remediation from 2000 to 2019. Environ. Sci. Pollut. Res. 2021 , 28 , 34200–34210. [ Google Scholar ] [ CrossRef ] [ PubMed ]
- Rocchi, L.; Boggia, A.; Paolotti, L. Sustainable agricultural systems: A bibliometrics analysis of ecological modernization approach. Sustainability 2020 , 12 , 9635. [ Google Scholar ] [ CrossRef ]
- Zhang, Y.; Pu, S.; Lv, X.; Gao, Y.; Ge, L. Global trends and prospects in microplastics research: A bibliometric analysis. J. Hazard. Mater. 2020 , 400 , 123110. [ Google Scholar ] [ CrossRef ]
- Xu, Y.; Lyu, J.; Liu, H.; Xue, Y. A Bibliometric and visualized analysis of the global literature on black soil conservation from 1983–2022 based on CiteSpace and VOSviewer. Agronomy 2022 , 12 , 2432. [ Google Scholar ] [ CrossRef ]
- Duan, G.; Bai, Y.; Ye, D.; Lin, T.; Peng, P.; Liu, M.; Bai, S. Bibliometric evaluation of the status of Picea research and research hotspots: Comparison of China to other countries. J. For. Res. 2020 , 31 , 1103–1114. [ Google Scholar ] [ CrossRef ]
- Aria, M.; Cuccurullo, C. Bibliometrix: An r-tool for comprehensive science mapping analysis. J. Informetr. 2017 , 11 , 959–975. [ Google Scholar ] [ CrossRef ]
- van Eck, N.J.; Waltman, L. Software survey: VOSviewer, a computer program for bibliometric mapping. Scientometrics 2010 , 84 , 523–538. [ Google Scholar ] [ CrossRef ] [ PubMed ]
- Chen, C.M. CiteSpace II: Detecting and visualizing emerging trends and transient patterns in scientific literature. J. Am. Soc. Inf. Sci. Technol. 2006 , 57 , 359–377. [ Google Scholar ] [ CrossRef ]
- Akiyama, H.; Yan, X.; Yagi, K. Evaluation of effectiveness of enhanced-efficiency fertilizers as mitigation options for N 2 O and NO emissions from agricultural soils: Meta-analysis. Glob. Chang. Biol. 2010 , 16 , 1837–1846. [ Google Scholar ] [ CrossRef ]
- Yao, Y.; Gao, B.; Chen, J.; Yang, L. Engineered biochar reclaiming phosphate from aqueous solutions: Mechanisms and potential application as a slow-release fertilizer. Environ. Sci. Technol. 2013 , 47 , 8700–8708. [ Google Scholar ] [ CrossRef ] [ PubMed ]
- Yao, Y.; Gao, B.; Inyang, M.; Zimmerman, A.R.; Cao, X.D.; Pullammanappallil, P.; Yang, L.Y. Removal of phosphate from aqueous solution by biochar derived from anaerobically digested sugar beet tailings. J. Hazard. Mater. 2011 , 190 , 501–507. [ Google Scholar ] [ CrossRef ]
- Li, C.; Wu, K.; Wu, J. A bibliometric analysis of research on haze during 2000–2016. Environ. Sci. Pollut. Res. 2017 , 24 , 24733–24742. [ Google Scholar ] [ CrossRef ] [ PubMed ]
- Ghumman, A.S.M.; Shamsuddin, R.; Nasef, M.M.; Yahya, W.Z.N.; Abbasi, A.; Almohamadi, H. Sulfur enriched slow-release coated urea produced from inverse vulcanized copolymer. Sci. Total Environ. 2022 , 846 , 157417. [ Google Scholar ] [ CrossRef ] [ PubMed ]
- Guo, Y.; Ma, Z.; Ren, B.; Zhao, B.; Liu, P.; Zhang, J. Effects of humic acid added to controlled-release fertilizer on summer maize yield, nitrogen use efficiency and greenhouse gas emission. Agriculture 2022 , 12 , 448. [ Google Scholar ] [ CrossRef ]
- Dubey, A.; Mailapalli, D.R. Zeolite coated urea fertilizer using different binders: Fabrication, material properties and nitrogen release studies. Environ. Technol. Innov. 2019 , 16 , 100452. [ Google Scholar ] [ CrossRef ]
- El Assimi, T.; Lakbita, O.; El Meziane, A.; Khouloud, M.; Dahchour, A.; Beniazza, R.; Boulif, R.; Raihane, M.; Lahcini, M. Sustainable coating material based on chitosan-clay composite and paraffin wax for slow-release DAP fertilizer. Int. J. Biol. Macromol. 2020 , 161 , 492–502. [ Google Scholar ] [ CrossRef ]
- Tyagi, P.; Agate, S.; Velev, O.D.; Lucia, L.; Pal, L. A critical review of the performance and soil biodegradability profiles of biobased natural and chemically synthesized polymers in industrial applications. Environ. Sci. Technol. 2022 , 56 , 2071–2095. [ Google Scholar ] [ CrossRef ]
- Zhang, Z.; An, Y. Nanotechnology for the oil and gas industry—An overview of recent progress. Nanotechnol. Rev. 2018 , 7 , 341–353. [ Google Scholar ]
- Jakhar, A.M.; Aziz, I.; Kaleri, A.R.; Hasnain, M.; Haider, G.; Ma, J.H.; Abideen, Z. Nano-fertilizers: A sustainable technology for improving crop nutrition and food security. Nanoimpact 2022 , 27 , 100411. [ Google Scholar ] [ CrossRef ]
- Khan, H.A.; Naqvi, S.R.; Mehran, M.T.; Khoja, A.H.; Niazi, M.B.K.; Juchelkova, D.; Atabani, A. A performance evaluation study of nano-biochar as a potential slow-release nano-fertilizer from wheat straw residue for sustainable agriculture. Chemosphere 2021 , 285 , 131382. [ Google Scholar ] [ CrossRef ] [ PubMed ]
- Shen, Y.; Zhou, J.; Du, C.; Zhou, Z. Hydrophobic modification of waterborne polymer slows urea release and improves nitrogen use efficiency in rice. Sci. Total Environ. 2021 , 794 , 148612. [ Google Scholar ] [ CrossRef ] [ PubMed ]
- Ouyang, W.; Wang, Y.; Lin, C.; He, M.; Hao, F.; Liu, H.; Zhu, W. Heavy metal loss from agricultural watershed to aquatic system: A scientometrics review. Sci. Total Environ. 2018 , 637 , 208–220. [ Google Scholar ] [ CrossRef ]
- Zheng, W.; Zhang, M.; Liu, Z.; Zhou, H.; Lu, H.; Zhang, W.; Yang, Y.; Li, C.; Chen, B. Combining controlled-release urea and normal urea to improve the nitrogen use efficiency and yield under wheat-maize double cropping system. Field Crop Res. 2016 , 197 , 52–62. [ Google Scholar ] [ CrossRef ]
- Liu, L.; Shen, T.; Yang, Y.; Gao, B.; Li, Y.C.; Xie, J.; Tang, Y.; Zhang, S.; Wang, Z.; Chen, J. Bio-based large tablet controlled-release urea: Synthesis, characterization, and controlled-released mechanisms. J. Agric. Food Chem. 2018 , 66 , 11265–11272. [ Google Scholar ] [ CrossRef ] [ PubMed ]
- Zhang, W.; Liang, Z.; He, X.; Wang, X.; Shi, X.; Zou, C.; Chen, X. The effects of controlled release urea on maize productivity and reactive nitrogen losses: A meta-analysis. Environ. Pollut. 2019 , 246 , 559–565. [ Google Scholar ] [ CrossRef ]
- Tian, X.; Fan, Z.; Zhao, Y.; Sun, S.; Li, T.; Yu, N.; Zhai, S. Controlled-release urea improved cotton productivity and nitrogen use efficiency in China: A meta-analysis. Agron. J. 2021 , 113 , 2251–2259. [ Google Scholar ] [ CrossRef ]
- Khalsa, S.D.S.; Smart, D.R.; Muhammad, S.; Armstrong, C.M.; Sanden, B.L.; Houlton, B.Z.; Brown, P.H. Intensive fertilizer use increases orchard N cycling and lowers net global warming potential. Sci. Total Environ. 2020 , 722 , 137889. [ Google Scholar ] [ CrossRef ]
- Coskun, D.; Britto, D.T.; Shi, W.; Kronzucker, H.J. Nitrogen transformations in modern agriculture and the role of biological nitrification inhibition. Nat. Plants 2017 , 3 , 17074. [ Google Scholar ] [ CrossRef ]
- Wang, Y.; Guo, H.; Wang, X.; Ma, Z.; Li, X.; Li, R.; Li, Q.; Wang, R.; Jia, X. Spout fluidized bed assisted preparation of poly(tannic acid)-coated urea fertilizer. ACS Omega 2020 , 5 , 1127–1133. [ Google Scholar ] [ CrossRef ]
- Hamidi, R.M.; Siyal, A.A.; Luukkonen, T.; Shamsuddin, R.M.; Moniruzzaman, M. Fly ash geopolymer as a coating material for controlled-release fertilizer based on granulated urea. RSC Adv. 2022 , 12 , 33187–33199. [ Google Scholar ] [ CrossRef ] [ PubMed ]
- Li, B.; Ng, S.J.; Han, J.C.; Li, M.; Zeng, J.; Guo, D.; Zhou, Y.; He, Z.; Wu, X.; Huang, Y. Network evolution and risk assessment of the global phosphorus trade. Sci. Total Environ. 2023 , 860 , 160433. [ Google Scholar ] [ CrossRef ] [ PubMed ]
- Guelfi, D.; Pereira Nunes, A.P.; Sarkis, L.F.; Oliveira, D.P. Innovative phosphate fertilizer technologies to improve phosphorus use efficiency in agriculture. Sustainability 2022 , 14 , 14266. [ Google Scholar ] [ CrossRef ]
- Sharma, N.; Singh, A.; Dutta, R. Biodegradable fertilizer nanocomposite hydrogel based on poly(vinyl alcohol)/kaolin/diammonium hydrogen phosphate (DAhP) for controlled release of phosphate. Polym. Bull. 2021 , 78 , 2933–2950. [ Google Scholar ] [ CrossRef ]
- Yang, J.; Liu, T.; Liu, H.; Zhai, L.; Wang, M.; Du, Y.; Chen, Y.; Yang, C.; Xiao, H.; Wang, H. Dimethylolurea as a novel slow-release nitrogen source for nitrogen leaching mitigation and crop production. J. Agric. Food Chem. 2019 , 67 , 7616–7625. [ Google Scholar ] [ CrossRef ] [ PubMed ]
- Mustafa, A.; Athar, F.; Khan, I.; Chattha, M.U.; Nawaz, M.; Shah, A.N.; Mahmood, A.; Batool, M.; Aslam, M.T.; Jaremko, M.; et al. Improving crop productivity and nitrogen use efficiency using sulfur and zinc-coated urea: A review. Front. Plant Sci. 2022 , 13 , 942384. [ Google Scholar ] [ CrossRef ]
- Rahman, M.H.; Haque, K.M.S.; Khan, M.Z.H. A review on application of controlled released fertilizers influencing the sustainable agricultural production: A Cleaner production process. Environ. Technol. Innov. 2021 , 23 , 101697. [ Google Scholar ] [ CrossRef ]
- Lu, S.; Feng, C.; Gao, C.; Wang, X.; Xu, X.; Bai, X.; Gao, N.; Liu, M. Multifunctional environmental smart fertilizer based on L-aspartic acid for sustained nutrient release. J. Agric. Food Chem. 2016 , 64 , 4965–4974. [ Google Scholar ] [ CrossRef ]
- Guo, C.; Ren, T.; Li, P.; Wang, B.; Zou, J.; Hussain, S.; Cong, R.; Wu, L.; Lu, J.; Li, X. Producing more grain yield of rice with less ammonia volatilization and greenhouse gases emission using slow/controlled-release urea. Environ. Sci. Pollut. Res. 2019 , 26 , 2569–2579. [ Google Scholar ] [ CrossRef ]
- Tian, W.H.; Ye, J.Y.; Cui, M.Q.; Chang, J.B.; Liu, Y.; Li, G.X.; Wu, Y.R.; Xu, J.M.; Harberd, N.P.; Mao, C.Z.; et al. A transcription factor STOP1-centered pathway coordinates ammonium and phosphate acquisition in Arabidopsis . Mol. Plant 2021 , 14 , 1554–1568. [ Google Scholar ] [ CrossRef ]
- Alami, M.M.; Liu, S.; Gong, D.; Guo, S.; Shaohua, S.; Mei, Z.; Alami, M.J.; Yang, G.; Wang, X. Effects of excessive and deficient nitrogen fertilizers on triptolide, celastrol, and metabolite profile content in Tripterygium wilfordii Hook F. Ind. Crops Prod. 2023 , 206 , 117577. [ Google Scholar ] [ CrossRef ]
- Zhong, S.; Chen, R.; Song, F.; Xu, Y. Knowledge mapping of carbon footprint research in a LCA perspective: A visual analysis using CiteSpace. Processes 2019 , 7 , 818. [ Google Scholar ] [ CrossRef ]
- Rong, L.; Zheng, X.; Oba, B.T.; Shen, C.; Wang, X.; Wang, H.; Luo, Q.; Sun, L. Activating soil microbial community using bacillus and rhamnolipid to remediate TPH contaminated soil. Chemosphere 2021 , 275 , 130062. [ Google Scholar ] [ CrossRef ] [ PubMed ]
- Rehana, M.; Gladis, R.; Joseph, B. Controlled Release of Nutrients for Soil Productivity- A Review. Curr. J. Appl. Sci. Technol. 2022 , 41 , 34–46. [ Google Scholar ] [ CrossRef ]
- Ye, L.; Camps-Arbestain, M.; Shen, Q.; Lehmann, J.; Singh, B.; Sabir, M. Biochar effects on crop yields with and without fertilizer: A meta-analysis of field studies using separate controls. Soil Use Manag. 2020 , 36 , 2–18. [ Google Scholar ] [ CrossRef ]
- Liao, J.; Liu, X.; Hu, A.; Song, H.; Chen, X.; Zhang, Z. Effects of biochar-based controlled release nitrogen fertilizer on nitrogen-use efficiency of oilseed rape ( Brassica napus L.). Sci. Rep. 2020 , 10 , 11063. [ Google Scholar ] [ CrossRef ]
- Mbachu, O.; Jenkins, G.; Kaparaju, P.; Pratt, C. The rise of artificial soil carbon inputs: Reviewing microplastic pollution effects in the soil environment. Sci. Total Environ. 2021 , 780 , 146569. [ Google Scholar ] [ CrossRef ]
- Xie, J.; Yang, Y.; Gao, B.; Wang, Y.; Li, Y.C.; Cheng, D.; Xiao, T.; Li, K.; Fu, Y.; Xu, J.; et al. Magnetic-sensitive nanoparticle self-assembled superhydrophobic biopolymer-coated slow-release fertilizer: Fabrication, enhanced performance, and mechanism. ACS Nano 2019 , 13 , 3320–3333. [ Google Scholar ] [ CrossRef ]
- Li, T.; Lu, S.; Yan, J.; Bai, X.; Gao, C.; Liu, M. An environment-friendly fertilizer prepared by layer-by-layer self-assembly for pH-responsive nutrient release. ACS Appl. Mater. Interfaces 2019 , 11 , 10941–10950. [ Google Scholar ] [ CrossRef ]
- Ardali, T.R.; Ma’mani, L.; Chorom, M.; Motamedi, E.; Gharebaba, M.F. A biocompatible NPK +Fe+Zn slow release fertilizer: Synthesis and its evaluation in tomato plant growth improvement. Sci. Rep. 2024 , 14 , 4640. [ Google Scholar ]
- Jariwala, H.; Santos, R.M.; Lauzon, J.D.; Dutta, A.; Wai Chiang, Y. Controlled release fertilizers (CRFs) for climate-smart agriculture practices: A comprehensive review on release mechanism, materials, methods of preparation, and effect on environmental parameters. Environ. Sci. Pollut. Res. 2022 , 29 , 53967–53995. [ Google Scholar ] [ CrossRef ] [ PubMed ]
- Kassem, I.; Ablouh, E.H.; El Bouchtaoui, F.Z.; Kassab, Z.; Khouloud, M.; Sehaqui, H.; Ghalfi, H.; Alami, J.; El Achaby, M. Cellulose nanocrystals-filled poly (vinyl alcohol) nanocomposites as waterborne coating materials of NPK fertilizer with slow release and water retention properties. Int. J. Biol. Macromol. 2021 , 189 , 1029–1042. [ Google Scholar ] [ CrossRef ] [ PubMed ]
Click here to enlarge figure
Rank | Institution | Country and Region | Records | % of 1676 | Total Citations | Average Cited Times |
---|---|---|---|---|---|---|
1 | Univ Florida | USA | 97 | 5.79 | 3217 | 33.16 |
2 | Chinese Acad Sci | China | 79 | 4.71 | 2534 | 32.08 |
3 | Shandong Agr Univ | China | 68 | 4.06 | 1613 | 23.72 |
4 | Univ Chinese Acad Sci | China | 29 | 1.73 | 653 | 22.52 |
5 | USDA Ars | USA | 26 | 1.55 | 1279 | 49.19 |
6 | Langzhou Univ | China | 22 | 1.31 | 1333 | 60.59 |
7 | Purdue Univ | USA | 20 | 1.19 | 422 | 21.10 |
8 | Univ Fed Sao Carlos | Brazil | 20 | 1.19 | 709 | 35.45 |
9 | Chinese Acad Agr Sci | China | 19 | 1.13 | 321 | 16.89 |
10 | Univ Sao Paulo | Brazil | 19 | 1.13 | 466 | 24.53 |
Rank | Journal Name | Records | % of 1676 | IF | Total Citations | Average Cited Times |
---|---|---|---|---|---|---|
1 | Hortscience | 69 | 4.12 | 1.9 | 815 | 11.81 |
2 | Science of the Total Environment | 44 | 2.63 | 9.8 | 1243 | 28.25 |
3 | Communications in Soil Science and Plant Analysis | 37 | 2.21 | 1.8 | 672 | 18.16 |
4 | Journal of Agricultural and Food Chemistry | 37 | 2.21 | 6.1 | 1835 | 49.59 |
5 | Journal of Plant Nutrition | 37 | 2.21 | 2.1 | 480 | 12.97 |
6 | Horttechnology | 32 | 1.91 | 1.0 | 412 | 12.88 |
7 | Journal of Cleaner Production | 32 | 1.91 | 11.1 | 926 | 28.94 |
8 | Agronomy—Basel | 30 | 1.79 | 3.7 | 187 | 6.23 |
9 | International Journal of Biological Macromolecules | 28 | 1.67 | 8.2 | 614 | 21.93 |
10 | Journal of Applied Polymer Science | 28 | 1.67 | 3.0 | 722 | 25.79 |
Rank | Author | Records | Total Citations | Institution | Average Cited Times |
---|---|---|---|---|---|
1 | Zhang M | 36 | 832 | Shandong Agr Univ | 23.11 |
2 | Gao B | 23 | 1845 | Univ Florida | 80.22 |
3 | Yang YC | 23 | 765 | Shandong Agr Univ | 33.26 |
4 | Zhou JM | 21 | 446 | Chinese Acad Sci | 21.24 |
5 | Du CW | 20 | 430 | Nanjing Institute of Soil Science, Chinese Academy of Sciences | 21.50 |
6 | Shen YZ | 18 | 287 | Nanjing Institute of Soil Science, Chinese Academy of Sciences | 15.94 |
7 | Jacobs DF | 17 | 468 | Purdue Univ | 27.53 |
8 | Liu MZ | 16 | 1110 | Lanzhou Univ | 69.38 |
9 | Liu ZG | 16 | 391 | Shandong Agr Univ | 24.44 |
10 | Li YC | 15 | 730 | Shandong Agr Univ | 48.67 |
Title | First Author | Year | Journal | Total Citation | TC per Year |
---|---|---|---|---|---|
Evaluation of effectiveness of enhanced-efficiency fertilizers as mitigation options for N O and NO emissions from agricultural soils: meta-analysis | Akiyama H | 2010 | Global Change Biology | 532 | 35.47 |
Engineered biochar reclaiming phosphate from aqueous solutions: mechanisms and potential application as a slow-release fertilizer | Yao Y | 2013 | Environmental Science & Technology | 509 | 42.42 |
Removal of phosphate from aqueous solution by biochar derived from anaerobically digested sugar beet tailings | Yao Y | 2011 | Journal of Hazardous Materials | 442 | 31.57 |
Ammonia volatilization from synthetic fertilizers and its mitigation strategies: A global synthesis | Pan BB | 2016 | Agriculture Ecosystems & Environment | 346 | 38.44 |
Preparation and characterization of slow-release fertilizer encapsulated by starch-based superabsorbent polymer | Qiao DL | 2016 | Carbohydrate Polymers | 259 | 28.78 |
Fertilizer source and tillage effects on yield-scaled nitrous oxide emissions in a corn cropping system | Venterea RT | 2011 | Journal of Environmental Quality | 227 | 16.21 |
Recycling of manure nutrients: use of algal biomass from dairy manure treatment as a slow release fertilizer | Mulbry W | 2005 | Bioresource Technology | 220 | 11.00 |
Environmentally friendly slow-release nitrogen fertilizer | Ni BL | 2011 | Journal of Agricultural and Food Chemistry | 210 | 15.00 |
Use of controlled release fertilizers and nitrification inhibitors to increase nitrogen use efficiency and to conserve air and water quality | Shoji S | 2001 | Communications in Soil Science and Plant Analysis | 206 | 8.58 |
Controlled-release fertilizer encapsulated by starch/polyvinyl alcohol coating | Han XZ | 2009 | Desalination | 203 | 12.69 |
Clusters | Main Keywords | Research Topic |
---|---|---|
Red cluster | Nitrogen, plants, growth, quality, plant growth, plant nutrition, plant quality | The impact of SRFs/CRFs on crop growth and quality |
Blue cluster | Urea, slow release, super-absorbent, | Urea-based SRFs/CRFs |
Yellow cluster | Phosphorus, recovery, biomass, struvite, adsorption, kinetics, mechanism, | Phosphorus fertilizer slow and controlled SRFs/CRFs |
Green cluster | Soil, management, use efficiency, nitrous, nitrous-oxide emissions, nitrification inhibitors, ammonia volatilization | Impact of SRFs/CRFs on N use efficiency, nitrification and denitrification functions in soil–plant systems |
Purple cluster | Biogradation, bioremediation, bioavailability | Degradation aspects of SRFs/CRFs |
The statements, opinions and data contained in all publications are solely those of the individual author(s) and contributor(s) and not of MDPI and/or the editor(s). MDPI and/or the editor(s) disclaim responsibility for any injury to people or property resulting from any ideas, methods, instructions or products referred to in the content. |
Share and Cite
Li, X.; Li, Z. Global Trends and Current Advances in Slow/Controlled-Release Fertilizers: A Bibliometric Analysis from 1990 to 2023. Agriculture 2024 , 14 , 1502. https://doi.org/10.3390/agriculture14091502
Li X, Li Z. Global Trends and Current Advances in Slow/Controlled-Release Fertilizers: A Bibliometric Analysis from 1990 to 2023. Agriculture . 2024; 14(9):1502. https://doi.org/10.3390/agriculture14091502
Li, Xianhong, and Zhonghong Li. 2024. "Global Trends and Current Advances in Slow/Controlled-Release Fertilizers: A Bibliometric Analysis from 1990 to 2023" Agriculture 14, no. 9: 1502. https://doi.org/10.3390/agriculture14091502
Article Metrics
Article access statistics, further information, mdpi initiatives, follow mdpi.
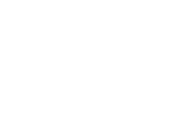
Subscribe to receive issue release notifications and newsletters from MDPI journals
- Open access
- Published: 28 August 2024
Perspectives of midwives on the use of Kaligutim (local oxytocin) for induction of labour among pregnant women in the government hospitals in Tamale
- Ahmad Sukerazu Alhassan 1 ,
- Shivera Dakurah 2 &
- Joseph Lasong 1
BMC Pregnancy and Childbirth volume 24 , Article number: 561 ( 2024 ) Cite this article
Metrics details
The use of herbal medicine and/or its products is common throughout the world. In Tamale Metropolis, pregnant women frequently use local oxytocin to induce labour, as shown by the fact that 90% of midwives reported managing patients who used kaligutim (local oxytocin) to speed up labour. Early career midwives are also aware of this and have personally observed it being used by their clients. The purpose of the study was to assess midwives’ opinions on pregnant women’s use of the well-known kaligutim (local oxytocin) for labour induction in the Tamale Metropolis.
A facility-based, quantitative, cross-sectional research design was used for the study. A total of 214 working midwives from Tamale’s three main public hospitals participated. Data for the study were gathered through a standardized questionnaire. For the analysis and presentation of the data, descriptive and analytical statistics, such as basic frequencies, percentages, Fisher’s exact test, chi square test and multivariate analysis, were employed.
According to the findings of this study, the safety, dosages, and contraindications of kaligutim during pregnancy and labour are unknown. The cessation of contractions was reported by 44 (22.4%) of the respondents whose clients used local oxytocin. The study also revealed that women in Tamale metropolis use “walgu”, a spiritual form of oxytocin, to induce and augment labour. Respondents who responded, “yes” to baby admission to the new-born care unit were 25% more likely to use kaligutim (local oxytocin) than were those who responded, “no” to baby admission to the new-born care unit (AOR = 0.25 95% CI (0.01, 0.53), P = 0.021).
Conclusions
It can be concluded that using kaligutim to start labour has negative effects on both the mother and the foetus. Additional research is required to evaluate the efficacy, effectiveness, biochemical makeup, and safety of these herbal medicines, particularly during pregnancy and delivery, as well as the spiritual significance of kaligutim (Walgu) and its forms.
Peer Review reports
Introduction
Herbal medicines, traditional treatments, and traditional practitioners are the main source of health care for many millions of people, and sometimes the only source of care [ 1 ]. Herbal medicines include herbs, herbal materials, herbal preparations and finished herbal products, that contain as active ingredients parts of plants, or other plant materials, or combinations [ 1 , 2 ]. Women in both developed and developing countries use herbal medicine before pregnancy and during pregnancy and delivery, which has several consequences [ 3 ]. The use of herbal medicine has a long history, tracing its roots back to ancient and biblical days when there was no Orthodox medicine. Currently, both developed and developing countries use herbal medicine due to the presence of many traditional medicine practitioners [ 4 ].
Many cultures worldwide use herbal medicine to induce or accelerate labour, and the incidence of labour induction to shorten the duration of labour is on the rise. Most herbal medicine users are pregnant women who have no formal education, who have a low level of income and who mostly stay far from health facilities [ 5 ]. The majority of pregnant women use herbal medicine through the oral route and have confidence in its efficacy, safety and effectiveness [ 6 ]. Herbal medicine is used by women for maternal health-related issues, such as to induce abortion and labour, to correct infertility, for the treatment of pregnancy-related issues, for breast milk secretion and for general wellbeing during pregnancy [ 5 ].
Women who use herbal medicine during pregnancy and/or labour usually have a high risk of postpartum complications [ 7 ]. The use of herbal uterotonics can lead to hyperstimulation of the uterus, foetal asphyxia and several other adverse effects of labour [ 8 ]. Moreover, traditional medicine used by pregnant women is associated with several complications, including a ruptured uterus, a fresh still birth, a macerated still birth, a caesarean section and even death [ 9 ]. These herbal medicines have both uterotonic and nonuterotonic effects on labour and delivery and are mostly used to induce or augment labour in prolonged labour or postdate or to relax or widen the pelvis for delivery [ 8 ].
Maternal and neonatal deaths are still major challenges for most developing countries, with obstetric complications, especially postpartum haemorrhage (P.P.H.) being the major cause of maternal mortality [ 10 ]. The delivery of healthcare services is still poor quality in developing nations [ 11 ]. Maternal and foetal mortality and morbidity have remained high due to inadequate health services and inadequate emergency obstetric treatment. Childbirth is accompanied by numerous customs that are subject to ethnological research and are often rooted in traditional medicine or religion. Cultural influences and sociodemographic characteristics play an important role in a woman’s decision to seek maternal and child health services.
The induction of labour is the process of artificially starting labour by stimulating the uterus with oxytocin or manually through the rupture of amniotic membranes. This process is usually not risk free, and most women find it to be uncomfortable [ 12 ]. The induction of labour is an obstetric procedure recommended when the benefits to the baby and mother outweigh the benefits of continuing the pregnancy. The procedure usually involves complications and failures and must be performed under close monitoring, proper selection of clients and good preparation [ 13 ].
Labour induction also changes the normal physiological processes that accompany childbirth and increases the risk of adverse pregnancy outcomes such as postpartum haemorrhage, neonatal mortality, foetal distress, uterine rapture and premature birth [ 14 ]. Oxytocin is a natural hormone produced by the hypothalamus and is responsible for the activation of sensory nerves during labour and breastfeeding [ 15 ]. Clinically, commercially manufactured synthetic oxytocin is administered to commence or increase uterine activity to reduce the duration of labour [ 16 ].
The induction of labour is not free from risk and must be performed with caution because the procedure involves hyperstimulation of the uterus and foetal distress. Herbal medicine used by pregnant women has long-term effects on both mothers and babies [ 17 ]. Many pregnant women in the Tamale Metropolis use prepackaged herbal medicine before and during pregnancy [ 18 ]. Health-related factors such as cost, distance, access and unavailability of medications influence the utilization of herbal medicine by pregnant women [ 17 ].
All women should be given a prophylactic dose of oxytocin as soon as they give birth. If they start to haemorrhage, they should also be given a treatment dose of oxytocin, which is greater than the prophylactic dose [ 19 ]. There is also a traditional manufactured form of oxytocin (kaligutim) that pregnant women use to start labour. Kaligutim is the local name for the mixture of some special plant parts or a combination of plants prepared and given to pregnant women to start or accelerate the process of labour in the northern part of Ghana [ 17 ].
Ideally, women should take medical drugs during pregnancy (folic acid and fersolate) to help prevent birth defects and congenital malformations such as neural tube defects of the foetus and spinal bifida during pregnancy [ 20 ]. However, in recent decades, women worldwide have used herbal medications during pregnancy and labour, with some taking both herbal medicine and orthodox medicine at the same time [ 21 ]. However, little is known about the use and safety of these medicines, especially during pregnancy, and their dosages, indications and contraindications are not known [ 22 ].
There are studies on herbal medicine use by women during pregnancy and labour, but there is currently no literature on the use of Kaligutim (local oxytocin) for labour induction among pregnant women in Ghana, but similar studies have been conducted in Uganda, Malawi, Tanzania, and Nigeria. Despite the efforts of the government and other nongovernmental organizations to ensure maximum coverage of skilled delivery to help reduce maternal and neonatal mortalities, women still use locally prepared oxytocin to induce labour. Although herbal medicine is commonly used by pregnant women, healthcare providers, especially midwives, are often unprepared to communicate effectively with patients or make proper decisions concerning complementary and alternative medicine use, especially during pregnancy and labour [ 23 ].
It is well known that herbs have played a vital role since the precolonial era during pregnancy, delivery and postpartum care in many parts of the country, but there are still few data on the use of herbs among pregnant women in Ghana [ 24 ]. Towards the end of pregnancy, many women are tired and eager to welcome their babies into the world. Moreover, as the expected date of delivery approaches, these women are given local oxytocin by their mothers’ in-laws, grandmothers, mothers, or TBAs or even by the women themselves to start labour at home before going to the health facility [ 25 ].
Medicinal plants that are used to hasten or speed up labour are mostly taken towards the end of pregnancy or the beginning of labour [ 26 ]. Even after delivery, these herbs may be found in small amounts in the mother’s breast, and some may cross the placental barrier and have harmful effects on the baby. The use of herbal medication by pregnant women is inevitable given that up to 80% of people who live in developing nations rely on traditional medicine for their healthcare needs [ 18 ].
The situation in Ghana, especially Northern Ghana, is not different, as pregnant women continue to use herbs despite the availability of health facilities [ 24 ]. The use of herbal medicine (kaligutim) among the Ghanaian population is alarming. Pregnant women in Tamale use herbal products at a rate of 42.5% prior to pregnancy and 52.7% during pregnancy [ 27 ]. Residents of Tamales who seek healthcare services in hospitals or herbal clinics are therefore at a greater risk of experiencing adverse consequences from drug-herb interactions [ 28 ].
Herbal product manufacturers should clearly state that pregnancy is a contraindication, and vendors should use caution when selling these items to pregnant women [ 27 ]. The use of Kaligutim (local oxytocin) by pregnant women is a maternal and child health problem. Herbal medicine used by pregnant women has long-term effects on both mothers and babies [ 17 ]. Unfortunately, maternal, and neonatal deaths may occur, and hence, there is a need to examine midwives’ perspectives on local oxytocin use during labour, its effects on the progress and outcome of labour, and the relationship between kaligutim use and birth outcomes among pregnant women in the three major government hospitals in Tamale Metropolis.
Theoretical foundation
This study adopted and adapted Andersen’s (1968) behavioural model of healthcare service utilization (use and nonuse of health services [ 29 ]. Andersen’s healthcare utilization model is a conceptual model aimed at demonstrating the factors that lead to the use/nonuse of health services [ 29 ]. This study was guided by Andersen’s behavioural model of health service use as a theoretical framework to identify the effects of Kaligutim on the progress and outcome of labour and to establish the relationship between the use of Kaligutim and nonuse of kaligutim and birth outcomes. The behavioural model is a multilevel model that incorporates both individual and contextual determinants of health service use.
Conceptual framework
Many people rely on products made from medicinal plants to maintain their health or treat illness, and current general development trends in developing and developed countries suggest that the consumption of medicinal plants is unlikely to decline in the short to medium term because of the benefits to consumers, producers, and society as a whole [ 29 ]. Therefore, there is a need to increase our understanding of what motivates the consumption of medicinal plants, despite the barriers to the establishment of solid evidence on the safety and efficacy of herbal medicines and related products [ 29 ].
This unified conceptual framework offers a step towards establishing a comprehensive approach to understanding the experiences midwives encounter when their clients use herbal medicine to induce their labour. The exposure variable in this study refers to kaligutim (local oxytocin) used by pregnant women in the three major government hospitals to induce labour through several routes, including oral, rectal, and vaginal routes, among others. When oxytocin is used by pregnant women, it can produce several results that can be immediate or late.
The results elicited on labour are termed the outcome variables, which can be immediate outcomes (the progress of labour) or outcomes after delivery (the outcome of labour). The progression of labour includes three stages: progressive dilatation of the cervix from 1 cm to 10 cm, delivery of the baby and expulsion of the placenta. Several factors can be used to determine the progress of labour (obstructed labour, prolonged labour, nature of uterine contractions, precipitated labour, foetal distress, and poor progress of labour).
The outcome of labour on the hand refers to what happens during the delivery of the baby, how the baby was delivered, foetal conditions and maternal conditions. The following factors were used for the purpose of this study to determine the outcome of labour (mode of delivery, postpartum haemorrhage, ruptured uterus, cervical tear, birth asphyxia, uterine atony, maternal mortality, and neonatal mortality). This study focused on the immediate effects of Kaligutim (on labour progress) and the effects of Kaligutim after delivery (on labour outcomes) and the relationship between the use of Kaligutim and birth outcomes.
The study was carried out in Tamale, which is the capital city of the northern region of Ghana. According to the 2021 World Urbanization Review, Tamales has an estimated population of 671,812 people. Tamale still has a blend of typical rural and urban communities, although it has attained the status of a metropolitan area. There are three major government hospitals in Tamale: Tamale Central Hospital, Tamale West Hospital and Tamale Teaching Hospital. The Tamale Teaching Hospital is the only tertiary facility in the northern region and serves as the main reference centre for the five regions of the north.
Study population
The main study population was midwives working in Tamale Metropolis. The sampling frame was all midwives practicing in the three major hospitals in Tamale Metropolis who were willing to participate in the study.
Study design
A facility-based cross-sectional research design was used for this study. A cross-sectional study is a type of observational study design carried out at one point in time or over a short period of time to estimate the prevalence of the outcome of interest for a given population for the purpose of public health planning [ 30 ]. This study adopted a quantitative research approach to obtain information.
Sampling technique
A purposive sampling technique was used for this study. Purposive sampling is a nonprobability sampling method in which participants are selected for inclusion in the sample based on their characteristics, knowledge, or experiences. This is because of the midwives’ knowledge, experiences, and objective of the study.
Sample size calculation
Total number of midwives = 458
Yamane formula (1967) was used with a confidence interval of 95% and a margin of error of 5%.
N = population size (458).
n = the sample size (?)
e = margin of error (5%).
n \(\:=\frac{N}{1\:+N\left(e\right)2}\)
n= \(\:\frac{458}{1\:+458\left(0.05\right)2}\)
n = 214.01 = 214.
Sample size = 214 midwives.
Inclusion criteria
All midwives practicing in the three major government hospitals in Tamale Metropolis who were willing to participate in the study were included.
All midwives in the three-government hospital with experience with kaligutim use during labour were also included in the study.
Exclusion criteria
All midwives who were not practising at the three major government hospitals in Tamale Metropolis were excluded from the study.
Midwives who were practicing at the three major government hospitals in Tamale and who were not willing to participate in the study were also excluded from the study.
All midwives who did not have experience with kaligutim use for the induction of labour were excluded from the study.
Data collection instrument
The data collection tool that was used for the study was a standardized questionnaire. The questionnaire was constructed by reviewing various documents, including existing questionnaires that have been used in previous research. Close-ended questions with few open-ended questions were used as the question format. It was designed in line with the objectives of the study to help obtain the necessary information needed for the study. The questionnaire was pretested with midwives before the actual data collection took place.
Data management and analysis
Data collected from the field were coded, cleaned, and entered into the Statistical Package for Social Services (SPSS) version 21.0. Descriptive and analytical statistics, including simple frequencies and percentages, were used for the analysis and presentation of the data. The relationships between predictor and outcome variables were assessed by means of bivariate (chi-square test) analysis to determine potential predictors of kaligutim (local oxytocin) at p values less than 0.05. Adjusted odds ratios were reported, and p values less than 0.05 were deemed to indicate statistical significance at the 95% confidence level after multivariate analysis.
Ethical consideration
The following ethical principles guided this study: respect for persons, beneficence, and justice for all. These principles are based on the human rights that must be protected during any research project, including the right to self-determination, privacy, anonymity, confidentiality, fair treatment and protection from discomfort and harm. First, an introductory letter was obtained from the University for Development Studies authorities. This letter was then presented to the authorities of the three major government hospitals in Tamale, namely, Tamale West Hospital (T.W.H.), Tamale Central Hospital (T.C.H.) and Tamale Teaching Hospital (TTH.), to seek permission to undertake the study. Ethical clearance was also obtained from the Kwame Nkrumah University of Science and Technology (KNUST) (CHRPE/AP/332/22).
Permission was once sought through a consent form to which participants were asked to consent if they were willing to participate in the study. The participants were assured of the confidentiality of all the information they were going to provide. They were also encouraged to participate in the study as much as they could but were also made aware that the study was voluntary and that they could withdraw at any point in time during the process if needed. There was no compensation for the study participants.
The study revealed that 45% of the respondents were between the ages of 20 and 30. Most of the respondents were in their twenties or thirties. Those who were in the first half of their work life constituted 73% of the respondents, while 17% were in the second half of their working life. The majority of the respondents were diploma midwives, representing 48% of the respondents; post basic midwives, constituting 32%; and degree and master’s holders, representing 19% and 1%, respectively. Staff midwives composed the largest group of respondents, while Principal Midwifery officers composed the group with the lowest participation in the study. The lowest rank in midwifery practice in the study was staff midwives, and the highest was principal midwifery officers. This is presented in Table 1 .
The experience of using local oxytocin to induce labour
Approximately 90% of the respondents have prior knowledge or heard that some of their clients take local oxytocin at home to start labour, and only 10% of respondents have no prior knowledge of that. Approximately 63.4% of the respondents encountered local oxytocin cases more than three times every week. This is presented in Fig. 1 .
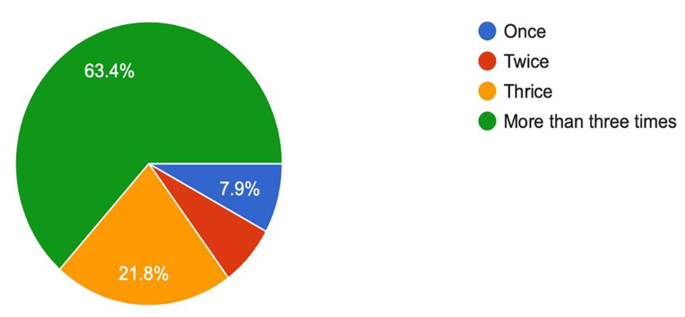
Average number of local oxytocin cases per week
Approximately 72.9% of the respondents said that their clients had ever induced labour during the previous C/S, and 59.6% of the respondents said that they met clients who also induced their labour during twin pregnancy. Another 64.5% of the respondents said that they also met clients with large babies who also induced labour using local oxytocin, while 86.2% of the respondents said that they also met clients who induced labour with local oxytocin even when they had grand multiparity. Another 11.3% of the respondents said that they met clients who used local oxytocin to induce labour during transverse lies, and 15.3% of the respondents said that they had experienced when clients with mal presentations used local oxytocin to induce labour. This is presented in Fig. 2 .
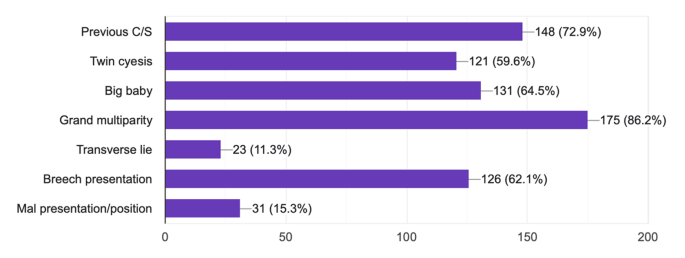
Induction of labour by clients through local oxytocin under certain conditions
The study additionally asked midwives to report on how pregnant women who had taken local oxytocin to induce labour coped during their care. Midwives were expected to respond whether the women they cared for experienced good, difficult, bad, painful, life-threatening, terrible, or normal labour. As shown in Fig. 3 , generally, the experience that pregnant women experience when they use local oxytocin to induce labour is not good. A total of 93.5% of the respondents said that the women who used local oxytocin had very bad experiences.
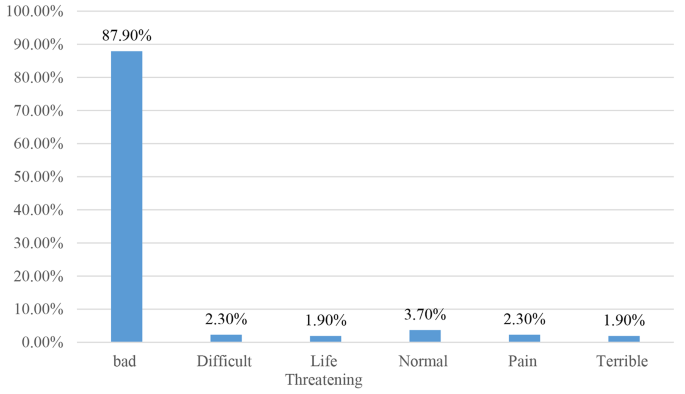
Experience of using local oxytocin to induce labour
The study further revealed that 15.2% of the respondents had experienced situations where some pregnant women died because of the use of local oxytocin.
Effects of local oxytocin on the progress of labour
The effects of local oxytocin (Kaligutim) on the progress of labour were diverse. The study revealed that the effects of Kaligutim on the progress of labour are negative, as it causes prolonged labour for some, obstructed labour for others, precipitated labour, and poor progress of labour for others. With obstructed labour being the leading effect of kaligutim on the progress of labour, most of the respondents chose caesarean section as the preferred delivery for most clients who used kaligutim at birth. The use of local oxytocin also has some effect on the amniotic fluid of pregnant women, as 99% of the midwives who responded to the study said that there were some levels of stain of the amniotic fluid, and only 1% said it was clear. It is evident from the study that for most pregnant women who use local oxytocin, there is hyperstimulation of the uterus, as most of the midwives confirmed this for the study. Most pregnant women who use kaligutim suffer excessive contractions, which could have an effect on both mothers and babies. Again, more than half (53.75) of the respondents also said that their foetal heart rate was above 160 bpm. The majority (77.65) of the respondents said that there was no cessation of the contractions for those who took the local oxytocin. The results are presented in Table 2 .
Impact of local oxytocin on the outcome of labour
To understand how local oxytocin impacts labour, the study went further to ask participants what the mode of delivery was for those who used Kaligutim. According to the data, caesarean section is the mode of delivery for most women (56.5%) who use local oxytocin, and most are unable to achieve spontaneous delivery. This has contributed to the increasing number of caesarean sections recorded daily. Most of the babies had an Apgar score of 4/10 to 6/10. Many babies born to mothers who used herbal oxytocin were born with moderate birth asphyxia (69.6%) and severe birth asphyxia (24%). The study also reported that 20.8% of midwives reported that hysterectomy was carried out on their clients who had used herbal preparations to induce or hasten labour. This is alarming because many women have their uterus removed as a result of herbal oxytocin (kaligutm) usage. Most clients who used Kaligutim experienced postpartum haemorrhage after delivery. It was also evident that some pregnant women (34.5%) had uterine atony, although it cannot be said that Kaligutim was the cause of uterine atony. Several pregnant women (65.3 years old) who used Kaligutim also developed a ruptured uterus. See Table 3 .
Relationship between kaligutim (local oxytocin) use and birth outcome
Table 4 shows the associations between kaligutim (local oxytocin) use and birth outcomes among the respondents. Fisher’s exact test and the chi-square test showed that several birth outcome variables were significantly associated with kaligutim (local oxytocin). Do women who go through the normal process of labour and those who use kaligutim to induce their labour have the same birth outcome? (P value = 0.021), what was the foetal wellbeing? (P value = 0.041), When do most neonates whose mothers have taken Kaligutim die? (P value = 0.038), was baby admitted at the Newborn Care Unit? (P value = 0.001), were significantly associated with kaligutim. Additionally, having recorded a maternal death because of the use of Kaligutim (p value = 0.002) was also significantly associated with kaligutim, as presented in Table 4 .
Multivariate analysis of birth outcome predictors of Kaligutim (local oxytocin) among pregnant women in three major government hospitals in Tamale metropolis
In Table 5 , three birth outcome variables strongly depicted kaligutim use among the respondents: foetal wellbeing, admission to the new-born care unit, and death of most neonates because of the use of Kaligutim by their mothers. Respondents who responded, “yes” to baby admission to the Newborn Care Unit were 25% more likely to use kaligutim (local oxytocin) than were those who responded “no” to baby admission to the Newborn Care Unit [(AOR = 0.25 95% CI (0.01, 0.53), P = 0.021)].
Discussions
Although the respondents cut across with regard to the number of years of experience, most of the respondents were early career midwives. The fact that these early career midwives are familiar with and have experienced the use of local oxytocin by their clients shows that it is widely used by pregnant women in the Tamale metropolis. Approximately 90% of respondents were aware of the usage of kaligutim (local oxytocin) for inducing labour at home before going to the hospital for delivery. However, a study conducted in the Ashanti region of Ghana revealed that midwives and other healthcare professionals lack proper knowledge about herbal medicine usage among pregnant women, even though this information is urgently needed so that appropriate action may be taken to address the issue [ 31 ]. The study findings also demonstrated that pregnant women frequently utilize local oxytocin and that many of them are unaware of the potential negative effects that these herbs may have on them in certain circumstances. Figure 2 shows that the use of local oxytocin was not limited to only one condition. These findings further show that the use of local oxytocin by pregnant women is widespread and that pregnant women do not know the effect that local oxytocin can have on them when they have certain conditions. Additionally, pregnant women are ignorant of the fact that local oxytocin can be contraindicated under certain conditions and must be avoided. Hence, it may put the life of the pregnant mother and her baby in danger.
Although herbal medicines are natural, not all herbs are safe to use while pregnant. Thus, expectant mothers should consult their midwives for guidance before taking herbal remedies. The experience that pregnant women have when they use local oxytocin to induce labour is not a positive one. A total of 188 respondents, or 93.5% of the respondents, stated that the women who used local oxytocin had a very unpleasant experience. This is supported by additional research results showing that between 50 and 80% of pregnant women use traditional plant remedies, which could have adverse perinatal effects [ 32 ]. The statistics indicate that local oxytocin is frequently used by pregnant women in the Tamale Metropolis. Most of the midwives reported seeing these cases virtually daily. This finding supports a study conducted in Ghana’s Ashanti region (Kumasi), which revealed that knowledge of herbal medicine is widely shared and that there is evidence of an increase in the usage of herbs [ 33 ].
The study revealed that local oxytocin (Kaligutim) has a diverse range of effects on the progress of labour, including precipitating labour, prolonging labour, obstructing labour, and slowing labour. The partograph is a great tool for keeping track of labour progress and serving as a warning system for abnormalities in normal labour, which helps to prevent obstructed labour and improves maternal and foetal outcomes [ 34 ]. This is supported by the study’s findings, which indicate that using a partograph to monitor labour progress and identify any deviations is essential [ 34 ].
According to this study, most midwives, who make up 65.2% of the respondents, also claimed that pregnant women who use local oxytocin (Kaligutim) have excessive contractions, while only 71 of them, or 34.8% of the respondents, claimed that they do not notice excessive contractions in their clients. This is supported by research performed in Zambia, which revealed that these herbal medicines also elicit greater than normal uterine contractions [ 26 ].
Most pregnant women who use kaligutim experience excessive contractions, which may have an impact on both the mother and the unborn child. Similarly, other authors have also claimed that using herbal remedies during labour causes stronger and more frequent uterine contractions, which do not necessarily result in cervical dilatation [ 35 ]. This was confirmed in the study’s findings, which also noted that herbal oxytocin not only produces excessive uterine contractions but also may cause contractions to cease, as 44 (22.4%) of the respondents reported that those who took local oxytocin had a halt in contractions. Intravenous fluids such as normal saline and Ringer’s lactate are used to flush out the local oxytocin in the system and CS in the case of an emergency. Nifedipine is also given in certain circumstances to prevent contractions.
According to the study, 121 midwives, or 59.6% of the respondents, stated that caesarean sections were the preferred method of delivery for women who used kaligutim to induce labour. Both [ 36 ] in South Africa and [ 34 ] in Western Uganda reported these findings. Moderate birth asphyxia (69.6%) and severe birth asphyxia (23%) are common in newborns whose mothers utilize herbal oxytocin. According to the survey, 20.8% of midwives said they had performed hysterectomy procedures on clients who had utilized herbal induction or hastening methods to induce labour.
One of the main causes of maternal deaths worldwide, including in Ghana, is postpartum haemorrhage [ 10 ]. 91% of midwives said that when their patients use herbal oxytocin during labour, more of them suffer from postpartum haemorrhage. This is corroborated by research by Frank (2018), who found a connection between postpartum haemorrhage and the use of herbal medications during labour [ 37 ]. In contrast, other studies [ 38 ] have shown that using herbal medication during childbirth is linked to a lower risk of postpartum haemorrhage. Individuals who experienced postpartum haemorrhage were managed with uterine massage, intravenous fluids, Cytotec, repairs to tears, expulsion of retained products, blood transfusions, cervical repairs, and catheter use.
This report supports the findings of a study conducted in the Ugandan village of Kiganda, where the researcher [ 37 ] reported that the use of herbal medicines has been linked to labour induction, which can cause significant birth canal tearing, postpartum haemorrhage, uterine atony, a raptured uterus, and, if untreated, maternal mortality. Medical experts who are aware of the dangers of herbal remedies and who are obliged to advise patients against using them do so themselves. The majority of women who use herbal preparations during pregnancy have a high school education or higher, according to evidence showing that more than 57.5% of pregnant women who use herbs have a high school diploma or higher, which is consistent with findings from Saudi Arabia by [ 39 ] that show that formal education cannot even prevent women from taking herbs during pregnancy and labour.
Kaligutim also causes excessive uterine contractions, foetal discomfort, excessive uterine stimulation, uterine atony, PPH, birth hypoxia, and premature bearing down, claims this study. This is supported by the results of a study carried out in Europe, where researchers [ 40 ] found that the majority of herbal drugs taken by pregnant women have undesirable side effects. An Iranian study, however, revealed that utilizing herbal treatments during labour can lessen discomfort, speed up the process, and enhance both the quality of a woman’s delivery experience and her odds of having a healthy baby [ 41 ].
According to the study’s findings, three birth outcome variables strongly affected kaligutim (local oxytocin) use among the respondents: foetal wellbeing, admission to the newborn care unit, and death of most neonates as a result of the use of Kaligutim by their mothers. Respondents who responded, “yes to baby” and were admitted to the new-born care unit were 25% more likely to use kaligutim (local oxytocin) than were those who responded, “no to baby” and were admitted to the new-born care unit (AOR = 0.25 95% CI (0.01, 0.53), P = 0.021). This is probably one of the effects of taking local oxytocin. These infants were hospitalized for a variety of reasons, including asphyxia, respiratory distress, and low Apgar scores.
Additionally, the study results indicated that respondents who responded that a still birth outcome affected foetal wellbeing were 1.9 times more likely to use kaligutim (local oxytocin) than those who responded no to having live births were (AOR = 1.9 95% CI (0.01, 1.21), P = 0.047)]. This finding is consistent with findings from a sub-Saharan African study that showed that herbal medications used to speed up and induce labour have uterotonic effects and increase the risk of neonatal asphyxia attributable to uterine hyperstimulation [ 42 ]. This could be ascribed to the fact that the respondents wanted fast and easy delivery, which subsequently caused this effect.
Another interesting finding was that respondents who responded that having a birth asphyxia outcome to foetal wellbeing were 0.16 times more likely to use kaligutim (local oxytocin) than were those who responded no to having live births (AOR = 0.16, 95% CI (0.08, 3.08), P = 0.047). This result is similar to that of [ 42 ], who conducted their study in sub-Saharan Africa. This could be a result of the effects of kaligutim on foetal well-being, which results in birth asphyxia.
Furthermore, newborns whose mothers used kaligutim during labour and who died within the first hour of birth were 3.4 times more likely to use kaligutim (local oxytocin) than those whose mothers used kaligutim during labour [AOR = 3.4 95% CI (0.74, 1.5), P = 0.045]. In support of the findings from this study, a study on the consumption of herbal drugs among pregnant women in rural Malawi revealed that consumption was linked to pregnancy-related issues and that users had a greater risk of neonatal mortality/morbidity within the first hour of life than nonusers [ 14 ]. This could be attributed to the dangers this herb poses to the foetus during delivery.
Newborns whose mothers used kaligutim during labor and who died within the first week of life were 2.23 times more likely to use kaligutim (local oxytocin) than those whose mothers used intrauterine kaligutim [(AOR = 2.23 95% CI (0.00, 0.02), P = 0.045)]. This is supported by findings from a Malawian study that revealed that the use of labour-inducing plants during pregnancy has negative effects on obstetric and labour outcomes, such as uterine rapture, which can cause neonatal mortality and morbidity [ 35 ]. This could be attributed to the fact that PPH, uterine rapture, cervical tear, DIC, and hypoxia were the main causes of death.
Every life matter, which is why mothers’ lives and that of their newborn babies must be safeguarded at all costs. A sufficient level of knowledge is always vital since it exacerbates doubt. Therefore, it is crucial that people are informed of their rights, their health, and the services they can utilize to maintain and improve health to have a healthy increasing population. Although herbal medicine could be effective in treating certain ailments associated with pregnancy and delivery and is easily accessible to pregnant women, especially in rural communities, the possibility of overdose, drug-herb interactions, contraindications, and the unhygienic conditions under which they are prepared may influence both maternal and neonatal conditions.
The results showed that the use of kaligutim by pregnant women in Tamale Metropolis is on the rise. This means that much needs to be done to do away with the use of kaligutim, and this must start with midwives. Pregnancies and births can be improved with a healthy and qualified midwifery care model in improving and protecting women’s and newborn health in Tamale.
It can be concluded that the use of this herbal medicine (Kaligutim) poses a greater long-term health challenge for mothers and their babies. Midwives and other healthcare workers in the Tamale Metropolis must therefore intensify their public health campaigns against the use of Kaligutim for labour induction.
Recommendations
The findings of the study have important implications for maternal and child health. The nonuse of kaligutim (local oxytocin) for the induction of labour is the best option for pregnant women. Pregnant women should visit the hospital for all their health needs during the entire pregnancy. This will help prevent adverse pregnancy and labour outcomes as well as maternal and neonatal mortalities and morbidities.
Future researchers should perform further studies on the spiritual aspects of kaligutim (Walgu) and its types. Like synthetic oxytocin, an Islamic form of oxytocin is prepared by Mallams and causes uterine contractions and dilates the cervix.
However, studies should also be conducted on the efficiency, effectiveness and biochemical composition of these herbal preparations and their safety, especially during pregnancy and delivery. Samples of these herbal preparations should be taken for laboratory investigations.
Data availability
All data generated or analysed during this study are included in this article and its supplementary information files are available from the corresponding author on reasonable request.
World Health Organization. WHO traditional medicine strategy: 2014–2023. World Health Organization;2013. Accessed 20 Nov 2023.
Fokunang CN, Ndikum V, Tabi OY, Jiofack RB, Ngameni B, Guedje NM et al. Traditional medicine: past, present and future research and development prospects and integration in the National Health System of Cameroon. Afr J Trad Complement Alt Med. 2011;8(3).
John LJ, Shantakumari N. Herbal medicines use during pregnancy: a review from the Middle East. Oman Med J. 2015;30(4):229.
Article CAS PubMed PubMed Central Google Scholar
Mekuria AB, Erku DA, Gebresillassie BM, Birru EM, Tizazu B, Ahmedin A. Prevalence and associated factors of herbal medicine use among pregnant women on antenatal care follow-up at University of Gondar referral and teaching hospital, Ethiopia: a cross-sectional study. BMC Complement Alt Med. 2017;17:1–7.
Google Scholar
Shewamene Z, Dune T, Smith CA. The use of traditional medicine in maternity care among African women in Africa and the diaspora: a systematic review. BMC Complement Alt Med. 2017;17:1–6.
Nyeko R, Tumwesigye NM, Halage AA. Prevalence and factors associated with use of herbal medicines during pregnancy among women attending postnatal clinics in Gulu district, Northern Uganda. BMC Preg Childbirth. 2016;1–12.
Fukunaga R, Morof D, Blanton C, Ruiz A, Maro G, Serbanescu F. Factors associated with local herb use during pregnancy and labor among women in Kigoma region, Tanzania, 2014–2016. BMC Preg Childbirth. 2020;20:1–1.
Article Google Scholar
Tripathi V, Stanton C, Anderson FW. Traditional preparations used as uterotonics in Sub-saharan Africa and their pharmacologic effects. Int J Gynecol Obstet. 2013;120(1):16–22.
Maliwichi-Nyirenda CP, Maliwichi LL. Medicinal plants used to induce labour and traditional techniques used in determination of onset of labour in pregnant women in Malawi: a case study of Mulanje district. J Med Plants Res. 2010;4(24):2609.
Amanuel T, Dache A, Dona A. Postpartum Hemorrhage and its Associated factors among women who gave birth at Yirgalem General Hospital, Sidama Regional State, Ethiopia. Health Res Serv Manag Epidemiol. 2021;8:1–7.
James PB, Wardle J, Steel A, Adams J. Traditional, complementary and alternative medicine use in Sub-saharan Africa: a systematic review. BMJ Glob Health. 2018;3(5).
Coates D, Homer C, Wilson A, Deady L, Mason E, Foureur M, et al. Induction of labour indications and timing: a systematic analysis of clinical guidelines. Women Birth. 2020;33(3):219–30.
Article PubMed Google Scholar
Lawani OL, Onyebuchi AK, Iyoke CA, Okafo CN, Ajah LO. Obstetric outcome and significance of labour induction in a health resource poor setting. Obstet Gynecol Int. 2014;2014(1):419621.
PubMed PubMed Central Google Scholar
Zamawe C, King C, Jennings HM, Fottrell E. Associations between the use of herbal medicines and adverse pregnancy outcomes in rural Malawi: a secondary analysis of randomised controlled trial data. BMC Complement Alt Med. 2018;18:1–8.
Roopasree B, Joseph J, Mukkadan JK. Oxytocin-functions: an overview. MOJ Anat Physiol. 2019;6:128–33.
Espada-Trespalacios X, Ojeda F, Perez-Botella M, Milà Villarroel R, Bach Martinez M, Figuls Soler H, et al. Oxytocin administration in low-risk women, a retrospective analysis of birth and neonatal outcomes. Int J Environ Res Public Health. 2021;18(8):4375.
Article PubMed PubMed Central Google Scholar
Ayelyini B, Yidana A, Ziblim SD. The Use of Indigenous Medicine among women during pregnancy and labour in rural Ghana. Cent Afr J Public Health. 2019;5(3):120–8. https://doi.org/10.11648/j.cajph.20190503.14 .
Kwame Ameade EP, Zakaria AP, Abubakar L, Sandow R. Herbal medicine usage before and during pregnancy – a study in Northern Ghana. Int J Complement Alt Med. 2018;11(4).
Jhpiego. Business Case: Investing in production of high-quality oxytocin for low-resource settings–Final report December 2014. 2014. http://www.conceptfoundation.org/wp-content/uploads/2015/06/BusinessCase_Oxytocin_web.pdf . Accesed 25 Jan 2024.
Barišić T, Pecirep A, Milićević R, Vasilj A, Tirić D. What do pregnant women know about harmful effects of medication and herbal remedies use during pregnancy? Psychiatr Danub. 2017;29(1):804–11.
PubMed Google Scholar
Ameade EPK, Ibrahim M, Ibrahim HS, Habib RH, Gbedema SY. Concurrent Use of Herbal and Orthodox Medicines among Residents of Tamale, Northern Ghana, Who Patronize Hospitals and Herbal Clinics. Evidence-based Complement Alt Med. 2018;2018(2002).
Illamola SM, Amaeze OU, Krepkova LV, Birnbaum AK, Karanam A, Job KM, et al. Use of herbal medicine by pregnant women: what physicians need to know. Front Pharmacol. 2020;10:1483.
Bahall M, Legall G. Knowledge, attitudes, and practices among health care providers regarding complementary and alternative medicine in Trinidad and Tobago. BMC Complement Alt Med. 2017;17:1–9.
Peprah P, Agyemang-duah W, Arthur-holmes F, Budu HI, Abalo EM, Okwei R et al. ‘ we are nothing without herbs ’ : a story of herbal remedies use during pregnancy in rural Ghana. BMC Complement Altern Med.2019;1–12.
Kamatenesi-Mugisha M, Oryem-Origa H. Medicinal plants used to induce labour during childbirth in western Uganda. J Ethnopharmacol. 2007;109(1):1–9.
Ngoma CM. Use of Herbal Medicines to induce labour by pregnant women: a systematic review of literature. JOJ Nurs Heal Care. 2017;2(3):7–12.
Ameade EK, Zakaria AP, Abubakar L, Sandow R. Herbal medicine usage before and during pregnancy—a study in Northern Ghana. Int J Complement Alt Med. 2018;11(4):235–42.
Ameade EP, Ibrahim M, Ibrahim HS, Habib RH, Gbedema SY. Concurrent use of herbal and orthodox medicines among residents of Tamale, Northern Ghana, who patronize hospitals and herbal clinics. Evidence-Based Complement Alt Med. 2018;2018(1):1289125.
Andersen RM. Revisiting the behavioral Model and Access to Medical Care: does it Matter? J Health Soc Behav. 1995;1–10. https://doi.org/10.2307/2137284 .
Smith-Hall C, Larsen HO, Pouliot M. People, plants and health: a conceptual framework for assessing changes in medicinal plant consumption. J Ethnobiolo Ethnomed. 2012;8:1–1.
Levin KA. Study design III: cross-sectional studies. Evid-Based Dent. 2006;7(1):24–5.
Beste J, Asanti D, Nsabimana D, Anastos K, Mutimura E, Merkatz I, et al. Use of Traditional Botanical Medicines during pregnancy in Rural Rwanda. J Glob Health Perspect. 2015;2015:1–10.
Adusi-Poku Y, Vanotoo L, Detoh E, Oduro J, Nsiah R, Natogmah A. Type of herbal medicines utilized by pregnant women attending ante-natal clinic in Offinso North district: are orthodox prescribers aware? Ghana Med J. 2016;49(4):227.
Agyei-Baffour P, Kudolo A, Quansah DY, Boateng D. Integrating herbal medicine into mainstream healthcare in Ghana: clients’ acceptability, perceptions and disclosure of use. BMC Complement Alt Med. 2017;17(1):1–9.
Mukasa PK, Kabakyenga J, Senkungu JK, Ngonzi J, Kyalimpa M, Roosmalen VJ. Uterine rupture in a teaching hospital in Mbarara, western Uganda, unmatched case-control study. Reprod Health. 2013;10(1):1–6.
Lampiao F, Maliwichi-Nyirenda C, Mponda J, Tembo L, Clements C. A preliminary investigation of the effects of labour inducing plant, cissampelos mucronata, on the outcomesof pregnancy using rat models. Malawi Med J. 2018;30(3):159–61.
Kekana LS, Sebitloane MH. Ingestion of herbal medication during pregnancy and adverse perinatal outcomes. S Afr J Obstet Gynaecol. 2020;26(2):1–5.
Buyondo BF. Use of Herbal Medicines in Preparation for Labour and its determinants among pregnant women at Kiganda Health Centre Iv-Mubende District. Angew Chemie Int Edu. 2018;6(11):951–2.
Koh LM, Percival B, Pauley T, Pathak S. Complementary therapy and alternative medicine: effects on induction of labour and pregnancy outcome in low risk post-dates women. Heliyon. 2019;5(11). https://doi.org/10.1016/j.heliyon.2019.e02787 .
Al-Ghamdi S, Aldossari K, Al-Zahrani J, Al-Shaalan F, Al-Sharif S, Al-Khurayji H, et al. Prevalence, knowledge and attitudes toward herbal medication use by Saudi women in the central region during pregnancy, during labor and after delivery. BMC Complement Alt Med. 2017;17(1):1–9.
Gruber CW, O’Brien M. Uterotonic plants and their bioactive constituents. Planta Med. 2011;77(03):207–20.
Article CAS PubMed Google Scholar
Zahra A. Lavender aromatherapy massages in reducing labor pain and duration of labor: a randomized controlled trial. Afr J Pharm Pharmacol. 2013;7(8):456–430.
Download references
Acknowledgements
We thank the Management and Healthcare Staff of the Tamale West Hospital (T.W.H), Tamale Central Hospital (T.C.H) and Tamale Teaching Hospital (TTH) for their support throughout the data collection process. We acknowledge the contributions of all the midwives who shared their knowledge and experiences with us, your efforts are well appreciated.
No funding was available for the study.
Author information
Authors and affiliations.
Department of Population and Reproductive Health, School of Public Health, University for Development Studies, P. O. Box 1883, Tamale, Northern Region, Ghana
Ahmad Sukerazu Alhassan & Joseph Lasong
Nandom Nursing and Midwifery Training College, Upper West Region, Nandom, Ghana
Shivera Dakurah
You can also search for this author in PubMed Google Scholar
Contributions
ASA and SD conceptualised and drafted the research proposal. ASA, SD, and JL performed the statistical analysis, assisted with interpretation of the results, and co-drafted the manuscript. All authors contributed to the discussion of the paper, read, and approved the final manuscript.
Corresponding author
Correspondence to Ahmad Sukerazu Alhassan .
Ethics declarations
Ethics approval and consent to participate.
An introductory letter from the University for Development Studies was presented to the three government hospitals, Tamale West Hospital (T.W.H), Tamale Central Hospital (T.C.H) and Tamale Teaching Hospital (TTH) to seek for permission to undertake the study. Ethical clearance was also obtained from the Kwame Nkrumah University of Science and Technology (KNUST) with reference number (CHRPE/AP/332/22). Permission was also sought through a consent form of which participants were asked to consent to if they were willing to participate in the study. They were assured of confidentiality of every information they were going to provide. All other methods were performed in accordance with relevant guidelines and regulations on subject selection and participation.
Consent for publication
Not applicable.
Competing interests
The authors declare no competing interests.
Additional information
Publisher’s note.
Springer Nature remains neutral with regard to jurisdictional claims in published maps and institutional affiliations.
Rights and permissions
Open Access This article is licensed under a Creative Commons Attribution-NonCommercial-NoDerivatives 4.0 International License, which permits any non-commercial use, sharing, distribution and reproduction in any medium or format, as long as you give appropriate credit to the original author(s) and the source, provide a link to the Creative Commons licence, and indicate if you modified the licensed material. You do not have permission under this licence to share adapted material derived from this article or parts of it. The images or other third party material in this article are included in the article’s Creative Commons licence, unless indicated otherwise in a credit line to the material. If material is not included in the article’s Creative Commons licence and your intended use is not permitted by statutory regulation or exceeds the permitted use, you will need to obtain permission directly from the copyright holder. To view a copy of this licence, visit http://creativecommons.org/licenses/by-nc-nd/4.0/ .
Reprints and permissions
About this article
Cite this article.
Alhassan, A.S., Dakurah, S. & Lasong, J. Perspectives of midwives on the use of Kaligutim (local oxytocin) for induction of labour among pregnant women in the government hospitals in Tamale. BMC Pregnancy Childbirth 24 , 561 (2024). https://doi.org/10.1186/s12884-024-06745-z
Download citation
Received : 15 April 2024
Accepted : 08 August 2024
Published : 28 August 2024
DOI : https://doi.org/10.1186/s12884-024-06745-z
Share this article
Anyone you share the following link with will be able to read this content:
Sorry, a shareable link is not currently available for this article.
Provided by the Springer Nature SharedIt content-sharing initiative
- Local oxytocin
- Herbal medicine
- Traditional medicine
BMC Pregnancy and Childbirth
ISSN: 1471-2393
- Submission enquiries: [email protected]
- General enquiries: [email protected]

IMAGES
VIDEO
COMMENTS
Table of contents. Step 1: Write your hypotheses and plan your research design. Step 2: Collect data from a sample. Step 3: Summarize your data with descriptive statistics. Step 4: Test hypotheses or make estimates with inferential statistics.
[email protected]. August 21, 2019. Abstract. The use of statistical software in academia and enterprises has been evolving over the last. years. More often than not, students, professors ...
Introduction. Statistical analysis is necessary for any research project seeking to make quantitative conclusions. The following is a primer for research-based statistical analysis. It is intended to be a high-level overview of appropriate statistical testing, while not diving too deep into any specific methodology.
Statistical methods involved in carrying out a study include planning, designing, collecting data, analysing, drawing meaningful interpretation and reporting of the research findings. The statistical analysis gives meaning to the meaningless numbers, thereby breathing life into a lifeless data. The results and inferences are precise only if ...
Overview. Statistical Papers is a forum for presentation and critical assessment of statistical methods encouraging the discussion of methodological foundations and potential applications. The Journal stresses statistical methods that have broad applications, giving special attention to those relevant to the economic and social sciences.
STATISTICAL ANALYSIS WITH SPSS FOR RESEARCH. January 2017. Edition: First Edition. Publisher: ECRTD Publication. Editor: European Center for Research Training and Development. ISBN: Hardcover 978 ...
Statistical analysis involves gathering and testing quantitative data to make inferences about the world. ... In the methods section of an APA research paper, you report in detail the participants, measures, and procedure of your study. 266. APA format for academic papers and essays
In many fields, a statistical analysis forms the heart of both the methods and results sections of a manuscript. Learn how to report statistical analyses, and what other context is important for publication success and future reproducibility. A matter of principle. First and foremost, the statistical methods employed in research must always be:
JOURNAL METRICS >. Statistical Analysis and Data Mining: The ASA Data Science Journal addresses the broad area of data analysis, including data mining algorithms, statistical approaches, and practical applications. Topics include problems involving massive and complex datasets, solutions utilizing innovative data mining algorithms and/or novel ...
Provides well-organized coverage of statistical analysis and applications in biology, kinesiology, and physical anthropology with comprehensive insights into the techniques and interpretations of R, SPSS®, Excel®, and Numbers® output An Introduction to Statistical Analysis in Research: With Applications in the Biological and Life Sciences develops a conceptual foundation in statistical ...
A bstract. The choice of statistical test used for analysis of data from a research study is crucial in interpreting the results of the study. This article gives an overview of the various factors that determine the selection of a statistical test and lists some statistical testsused in common practice. How to cite this article: Ranganathan P.
There are 3 main types of descriptive statistics: The distribution concerns the frequency of each value. The central tendency concerns the averages of the values. The variability or dispersion concerns how spread out the values are. You can apply these to assess only one variable at a time, in univariate analysis, or to compare two or more, in ...
Background: The selection of statistical analysis methods in research is a critical and nuanced task that requires a scientific and rational approach. Aligning the chosen method with the specifics of the research design and hypothesis is paramount, as it can significantly impact the reliability and quality of the research outcomes.
Statistical analysis of the effect of socio-political factors on individual life satisfaction ... A paper in Physical Review X presents a method for numerically generating data sequences that are ...
A Simplified Definition. Statistical analysis uses quantitative data to investigate patterns, relationships, and patterns to understand real-life and simulated phenomena. The approach is a key analytical tool in various fields, including academia, business, government, and science in general. This statistical analysis in research definition ...
Study Design and Statistical Analysis A Practical Guide for Clinicians This book takes the reader through the entire research process: choosing a question, designing a study, collecting the data, using univariate, bivariate and multivariable analysis, and publishing the results. It does so by using plain language rather than complex
Abstract. The purpose of this paper is to elaborate on the importance of the Statistical Package for the Social Sciences, widely known as SPSS in the field of social sciences as an effective tool ...
Read the latest Research articles in Statistics from Scientific Reports. ... Statistical analysis of the effect of socio-political factors on individual life satisfaction. ... Calls for Papers
Results. Although biostatistical inputs are critical for the entire research study (online supplemental table 2), biostatistical consultations were mostly used for statistical analyses only 15.Even though the conduct of statistical analysis mismatched with the study objective and DGP was identified as the major problem in articles submitted to high-impact medical journals. 16 In addition ...
Statistical analysis is the process of collecting and analyzing data in order to discern patterns and trends. It is a method for removing bias from evaluating data by employing numerical analysis. This technique is useful for collecting the interpretations of research, developing statistical models, and planning surveys and studies.
important writing you will do for the paper. IMHO your reader will either be interested and continuing on with your paper, or... A scholarly introduction is respectful of the literature. In my experience, the introduction is part of a paper that I will outline relatively early in the process, but will nish and repeatedly edit at the end of the ...
A research hypothesis is your proposed answer to your research question. The research hypothesis usually includes an explanation ("x affects y because …"). A statistical hypothesis, on the other hand, is a mathematical statement about a population parameter. Statistical hypotheses always come in pairs: the null and alternative hypotheses.
Zero-inflated and hurdle models are widely applied to count data possessing excess zeros, where they can simultaneously model the process from how the zeros were generated and potentially help mitigate the eff... Yixuan Zou, Jan Hannig and Derek S. Young. Journal of Statistical Distributions and Applications 2021 8:5.
Education and financial development are crucial factors in achieving better health, including the reduction of infant mortality. This article investigates the causal relationship between education, financial development and infant mortality rate using annual data for 81 countries for the period 1980-2015 and employing the Emirmahmutoglu and Rose (2011) Granger causality test procedure for ...
Table 1. Number of people receiving Social Security, Supplemental Security Income (SSI), or both, July 2024 (in thousands)Type of beneficiary Total Social Security only SSI only Both Social Security and SSI; All beneficiaries
Statistical analysis revealed that 69 countries/regions have published a substantial number of research papers related to SRFs/CRFs. Figure 4A presents the top 10 countries/regions in SRF/CRF research. China and the USA are the predominant contributors, with 521 and 278 papers published, respectively.
ASA and SD conceptualised and drafted the research proposal. ASA, SD, and JL performed the statistical analysis, assisted with interpretation of the results, and co-drafted the manuscript. All authors contributed to the discussion of the paper, read, and approved the final manuscript. Corresponding author