
Current Topics in Soil Science
An Environmental Approach
- © 2022
- Swapna Mukherjee 0
Formerly at Geological Survey of India, Kolkata, India
You can also search for this author in PubMed Google Scholar
- Addresses current environmental issues and its remedy with soil
- Discusses the increasing concentration of carbon dioxide in our atmosphere
- Suggests possible remedies that can be done to mitigate the issues that our planet is facing
16k Accesses
9 Citations
This is a preview of subscription content, log in via an institution to check access.
Access this book
Subscribe and save.
- Get 10 units per month
- Download Article/Chapter or eBook
- 1 Unit = 1 Article or 1 Chapter
- Cancel anytime
- Available as EPUB and PDF
- Read on any device
- Instant download
- Own it forever
- Durable hardcover edition
- Dispatched in 3 to 5 business days
- Free shipping worldwide - see info
Tax calculation will be finalised at checkout
Other ways to access
Licence this eBook for your library
Institutional subscriptions
About this book
Soil is an important but often neglected element of the climate system. It is the second largest carbon store, or ‘sink’, after the oceans. Despite being a fundamental resource that supports all kinds of life on Earth, concerns related to soil are often not included as an important environmental issue. Climate changes put soil under pressure. The increasing concentration of carbon dioxide in our atmosphere may cause the microbes in the soil to work faster to break down organic matter, potentially releasing even more carbon dioxide. The soil moisture content is being constantly affected by rising temperatures and changes in precipitation patterns and future projections show that this may continue.
This book presents current environmental issues and their remedies for soil which are mainly based on soil degradation, soil pollution and the effect of climate change on the soil. Adding xenobiotic chemicals or other alterations in the natural soil environment for agricultural, industrial or urban purposes result in a decline in the soil quality due to improper use or poor management, which is a serious environmental problem. The book is divided into five parts - soil science, soil physics, soil chemistry, soil biology and soil environment. The first part “Soil Science” serves as the introduction to the book and discusses some common topics such as soil formation, mineralogy, taxonomy, quality and analytical techniques. The second part “Soil Physics” is mainly concerned with the physical properties and processes of soil and their association with effects on air, water and temperature. Soil Chemistry, the third part, discusses the chemical reactions and processes between inorganic and organic components. The fourth part “Soil Biology” explains the biological properties and processes of the soil, with special concern to microbial diversity and its effect on the ecology. Lastly, the fifth part “Soil Environment” discusses the current environmental problems such as climate change and soil pollution, including processes to mitigate these issues through carbon sequestration, nutrient management and land management.
Similar content being viewed by others
The Soil. Physical, Chemical and Biological Properties
Soil Quality: Concepts, Importance, Indicators, and Measurement
Urban Soil Functions
- soil science
- soil biology
- soil chemistry
- soil classification
- soil physics
- Environment
Table of contents (25 chapters)
Front matter, soil science, soil formation.
Swapna Mukherjee
Soil Minerology
Soil taxonomy and classification, soil quality, soil analytical techniques, soil physics, physical properties of soils, soil structure, soil tillage, soil air and temperature, soil erosion, soil chemistry, concepts of soil chemistry, chemical composition of soil, ion exchange, ph, salinity and sodicity, chemistry of soil nutrient, authors and affiliations, about the author.
A doctorate in Physics, Swapna Mukherjee started her career with the Geological Survey of India and spent the next 30 years handling various issues both from an applied and academic perspective. Her post doctoral studies sponsored by International Atomic Energy Agency (IAEA), allowed her to concentrate in various analytical techniques in geological studies and subsequently her research interest included other fields of geology and social science. Her research publications have been recognized internationally and cited at various symposium and in international journals. This book is the comprehensive presentation of her vast expertise in different aspects of social science.
Bibliographic Information
Book Title : Current Topics in Soil Science
Book Subtitle : An Environmental Approach
Authors : Swapna Mukherjee
DOI : https://doi.org/10.1007/978-3-030-92669-4
Publisher : Springer Cham
eBook Packages : Earth and Environmental Science , Earth and Environmental Science (R0)
Copyright Information : Capital Publishing Company, New Delhi, India 2022
Hardcover ISBN : 978-3-030-92668-7 Published: 30 April 2022
eBook ISBN : 978-3-030-92669-4 Published: 29 April 2022
Edition Number : 1
Number of Pages : XXIII, 268
Number of Illustrations : 1 b/w illustrations
Additional Information : Jointly published with Capital Publishing Company, New Delhi, India
Topics : Soil Science & Conservation , Systems Biology , Applied Microbiology , Environmental Management , Climate, general , Environmental Physics
- Publish with us
Policies and ethics
- Find a journal
- Track your research
Thank you for visiting nature.com. You are using a browser version with limited support for CSS. To obtain the best experience, we recommend you use a more up to date browser (or turn off compatibility mode in Internet Explorer). In the meantime, to ensure continued support, we are displaying the site without styles and JavaScript.
- View all journals
- Explore content
- About the journal
- Publish with us
- Sign up for alerts
- Perspective
- Published: 25 August 2020
The concept and future prospects of soil health
- Johannes Lehmann ORCID: orcid.org/0000-0002-4701-2936 1 , 2 , 3 ,
- Deborah A. Bossio ORCID: orcid.org/0000-0002-2296-9125 4 ,
- Ingrid Kögel-Knabner 3 , 5 &
- Matthias C. Rillig ORCID: orcid.org/0000-0003-3541-7853 6 , 7
Nature Reviews Earth & Environment volume 1 , pages 544–553 ( 2020 ) Cite this article
13k Accesses
572 Citations
157 Altmetric
Metrics details
- Biogeochemistry
- Environmental sciences
- Sustainability
Soil health is the continued capacity of soil to function as a vital living ecosystem that sustains plants, animals and humans, and connects agricultural and soil science to policy, stakeholder needs and sustainable supply-chain management. Historically, soil assessments focused on crop production, but, today, soil health also includes the role of soil in water quality, climate change and human health. However, quantifying soil health is still dominated by chemical indicators, despite growing appreciation of the importance of soil biodiversity, owing to limited functional knowledge and lack of effective methods. In this Perspective, the definition and history of soil health are described and compared with other soil concepts. We outline ecosystem services provided by soils, the indicators used to measure soil functionality and their integration into informative soil-health indices. Scientists should embrace soil health as an overarching principle that contributes to sustainability goals, rather than only a property to measure.
This is a preview of subscription content, access via your institution
Access options
Access Nature and 54 other Nature Portfolio journals
Get Nature+, our best-value online-access subscription
$29.99 / 30 days
cancel any time
Subscribe to this journal
Receive 12 digital issues and online access to articles
$99.00 per year
only $8.25 per issue
Buy this article
- Purchase on SpringerLink
- Instant access to full article PDF
Prices may be subject to local taxes which are calculated during checkout
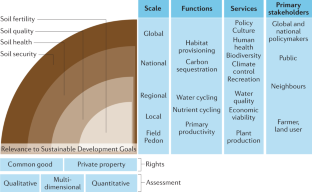
Similar content being viewed by others
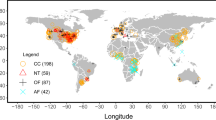
A database for global soil health assessment
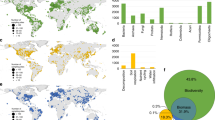
Blind spots in global soil biodiversity and ecosystem function research
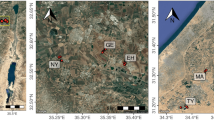
A sensitive soil biological indicator to changes in land-use in regions with Mediterranean climate
Ladyman, J., Lambert, J. & Wiesner, K. What is a complex system? Eur. J. Philos. Sci. 3 , 33–67 (2013).
Google Scholar
Brevik, E. C. et al. The interdisciplinary nature of SOIL. Soil 1 , 117–129 (2015).
Blum, W. E. Functions of soil for society and the environment. Rev. Environ. Sci. Biotechnol. 4 , 75–79 (2005).
Baveye, P. C., Baveye, J. & Gowdy, J. Soil “ecosystem” services and natural capital: critical appraisal of research on uncertain ground. Front. Environ. Sci. 4 , 41 (2016).
Keith, A. M., Schmidt, O. & McMahon, B. J. Soil stewardship as a nexus between ecosystem services and one health. Ecosyst. Serv. 17 , 40–42 (2016).
Bünemann, E. K. et al. Soil quality–a critical review. Soil Biol. Biochem. 120 , 105–125 (2018).
Patzel, N., Sticher, H. & Karlen, D. L. Soil fertility - phenomenon and concept. J. Plant Nutr. Soil Sci. 163 , 129–142 (2000).
Doran, J. W. & Parkin, T. B. in Defining Soil Quality for a Sustainable Environment Vol. 32 (eds Doran, J. W., Coleman, D. C., Bezdicek, D. F. & Stewart, B. A.) 1–21 (Soil Science Society of America, 1994).
Pankhurst, C. E., Doube, B. M. & Gupta, V. V. S. R. in Biological Indicators of Soil Health (eds Pankhurst, C., Doube, B. & Gupta, V.) 419–435 (CAB International, 1997).
McBratney, A., Field, D. J. & Koch, A. The dimensions of soil security. Geoderma 213 , 203–213 (2014).
Koch, A. et al. Soil security: solving the global soil crisis. Glob. Policy 4 , 434–441 (2013).
Stankovics, P., Tóth, G. & Tóth, Z. Identifying gaps between the legislative tools of soil protection in the EU member states for a common European soil protection legislation. Sustainability 10 , 2886 (2018).
Montanarella, L. Agricultural policy: govern our soils. Nature 528 , 32–33 (2015).
Jian, J., Du, X. & Stewart, R. D. A database for global soil health assessment. Sci. Data 7 , 16 (2020).
Karlen, D. L., Veum, K. S., Sudduth, K. A., Obrycki, J. F. & Nunes, M. R. Soil health assessment: past accomplishments, current activities, and future opportunities. Soil Tillage Res. 195 , 104365 (2019).
Norris, C. E. & Congreves, K. A. Alternative management practices improve soil health indices in intensive vegetable cropping systems: a review. Front. Environ. Sci. 6 , 50 (2018).
O’Dell, R. E. & Claassen, V. P. Vertical distribution of organic amendment influences the rooting depth of revegetation species on barren, subgrade serpentine substrate. Plant Soil 285 , 19–29 (2006).
Congreves, K. A., Hayes, A., Verhallen, E. A. & Van Eerd, L. L. Long-term impact of tillage and crop rotation on soil health at four temperate agroecosystems. Soil Tillage Res. 152 , 17–28 (2015).
Hamza, M. A. & Anderson, W. K. Soil compaction in cropping systems: a review of the nature, causes and possible solutions. Soil Tillage Res. 82 , 121–145 (2005).
Jenkinson, D. S. The Rothamsted long-term experiments: Are they still of use? Agron. J. 83 , 2–10 (1991).
Berendsen, R. L., Pieterse, C. M. & Bakker, P. A. The rhizosphere microbiome and plant health. Trends Plant Sci. 17 , 478–486 (2012).
Chaparro, J. M., Sheflin, A. M., Manter, D. K. & Vivanco, J. M. Manipulating the soil microbiome to increase soil health and plant fertility. Biol. Fertil. Soils 48 , 489–499 (2012).
Bonanomi, G., Lorito, M., Vinale, F. & Woo, S. L. Organic amendments, beneficial microbes, and soil microbiota: toward a unified framework for disease suppression. Annu. Rev. Phytopathol. 56 , 1–20 (2018).
Chen, X. D. et al. Soil biodiversity and biogeochemical function in managed ecosystems. Soil Res. 58 , 1–20 (2020).
Pittelkow, C. M. et al. Productivity limits and potentials of the principles of conservation agriculture. Nature 517 , 365–368 (2015).
Ogle, S. M., Swan, A. & Paustian, K. No-till management impacts on crop productivity, carbon input and soil carbon sequestration. Agric. Ecosyst. Environ. 149 , 37–49 (2012).
Zimnicki, T. et al. On quantifying water quality benefits of healthy soils. BioScience 70 , 343–352 (2020).
Evans, A. E., Mateo-Sagasta, J., Qadir, M., Boelee, E. & Ippolito, A. Agricultural water pollution: key knowledge gaps and research needs. Curr. Opin. Environ. Sustain. 36 , 20–27 (2019).
Carpenter, S. R. et al. Nonpoint pollution of surface waters with phosphorus and nitrogen. Ecol. Appl. 8 , 559–568 (1998).
Lamichhane, S., Krishna, K. B. & Sarukkalige, R. Polycyclic aromatic hydrocarbons (PAHs) removal by sorption: a review. Chemosphere 148 , 336–353 (2016).
Tournebize, J., Chaumont, C. & Mander, Ü. Implications for constructed wetlands to mitigate nitrate and pesticide pollution in agricultural drained watersheds. Ecol. Eng. 103 , 415–425 (2017).
Hanson, J. R., Macalady, J. L., Harris, D. & Scow, K. M. Linking toluene degradation with specific microbial populations in soil. Appl. Environ. Microbiol. 65 , 5403–5408 (1999).
Li, G., Sun, G. X., Ren, Y., Luo, X. S. & Zhu, Y. G. Urban soil and human health: a review. Eur. J. Soil Sci. 69 , 196–215 (2018).
Laurenson, G., Laurenson, S., Bolan, N., Beecham, S. & Clark, I. The role of bioretention systems in the treatment of stormwater. Adv. Agron. 120 , 223–274 (2013).
Wagg, C., Bender, S. F., Widmer, F. & van der Heijden, M. G. Soil biodiversity and soil community composition determine ecosystem multifunctionality. Proc. Natl Acad. Sci. USA 111 , 5266–5270 (2014).
Kadam, A. M., Oza, G. H., Nemade, P. D. & Shankar, H. S. Pathogen removal from municipal wastewater in constructed soil filter. Ecol. Eng. 33 , 37–44 (2008).
Welch, R. M. & Graham, R. D. Breeding for micronutrients in staple food crops from a human nutrition perspective. J. Exp. Bot. 55 , 353–364 (2004).
Barrett, C. B. & Bevis, L. E. The self-reinforcing feedback between low soil fertility and chronic poverty. Nat. Geosci. 8 , 907–912 (2015).
Wood, S. A., Tirfessa, D. & Baudron, F. Soil organic matter underlies crop nutritional quality and productivity in smallholder agriculture. Agric. Ecosyst. Environ. 266 , 100–108 (2018).
Wall, D. H., Nielsen, U. N. & Six, J. Soil biodiversity and human health. Nature 528 , 69–76 (2015).
Jacoby, R., Peukert, M., Succurro, A., Koprivova, A. & Kopriva, S. The role of soil microorganisms in plant mineral nutrition—current knowledge and future directions. Front. Plant Sci. 8 , 1617 (2017).
Schlatter, D., Kinkel, L., Thomashow, L., Weller, D. & Paulitz, T. Disease suppressive soils: new insights from the soil microbiome. Phytopathology 107 , 1284–1297 (2017).
Rillig, M. C., Lehmann, A., Lehmann, J., Camenzind, T. & Rauh, C. Soil biodiversity effects from field to fork. Trends Plant Sci. 23 , 17–24 (2018).
Oliver, M. A. & Gregory, P. J. Soil, food security and human health: a review. Eur. J. Soil Sci. 66 , 257–276 (2015).
Hussein, H. S. & Brasel, J. M. Toxicity, metabolism, and impact of mycotoxins on humans and animals. Toxicology 167 , 101–134 (2001).
Bethony, J. et al. Soil-transmitted helminth infections: ascariasis, trichuriasis, and hookworm. Lancet 367 , 1521–1532 (2006).
Schatz, A., Bugle, E. & Waksman, S. A. Streptomycin, a substance exhibiting antibiotic activity against gram-positive and gram-negative bacteria. Proc. Soc. Exp. Biol. Med. 55 , 66–69 (1944).
Ling, L. L. et al. A new antibiotic kills pathogens without detectable resistance. Nature 517 , 455–459 (2015).
Veresoglou, S. D., Halley, J. M. & Rillig, M. C. Extinction risk of soil biota. Nat. Commun. 6 , 8862 (2015).
Lal, R. Soil carbon sequestration impacts on global climate change and food security. Science 304 , 1623–1627 (2004).
Paustian, K. et al. Climate-smart soils. Nature 532 , 49–57 (2016).
Denef, K. & Six, J. Clay mineralogy determines the importance of biological versus abiotic processes for macroaggregate formation and stabilization. Eur. J. Soil Sci. 56 , 469–479 (2005).
Rinot, O., Levy, G. J., Steinberger, Y., Svoray, T. & Eshel, G. Soil health assessment: A critical review of current methodologies and a proposed new approach. Sci. Total. Environ. 648 , 1484–1491 (2019).
Van Wesemael, B. et al. An indicator for organic matter dynamics in temperate agricultural soils. Agric. Ecosyst. Environ. 274 , 62–75 (2019).
Bouma, J. et al. in Global Soil Security (eds Field, D. J., Morgan, C. L. S. & McBratney, A. B.) 27–44 (Springer, 2017).
Schoenholtz, S. H., Van Miegroet, H. & Burger, J. A. A review of chemical and physical properties as indicators of forest soil quality: challenges and opportunities. For. Ecol. Manag. 138 , 335–356 (2000).
Andrews, S. S. & Carroll, C. R. Designing a soil quality assessment tool for sustainable agroecosystem management. Ecol. Appl. 11 , 1573–1585 (2001).
Lilburne, L. R., Hewitt, A. E., Sparling, G. P. & Selvarajah, N. Soil quality in New Zealand: policy and the science response. J. Environ. Qual. 31 , 1768–1773 (2002).
Idowu, O. J. et al. Use of an integrative soil health test for evaluation of soil management impacts. Renew. Agric. Food Syst. 24 , 214–224 (2009).
Cherubin, M. R. et al. A Soil Management Assessment Framework (SMAF) evaluation of Brazilian sugarcane expansion on soil quality. Soil Sci. Soc. Am. J. 80 , 215–226 (2016).
E.U. Mission Board Soil Health and Food. Caring for Soil is Caring for Life . The Publications Office of the European Union https://op.europa.eu/en/web/eu-law-and-publications/publication-detail/-/publication/32d5d312-b689-11ea-bb7a-01aa75ed71a1 (European Commission, 2020).
Nunes, M. R., Karlen, D. L., Veum, K. S., Moorman, T. B. & Cambardella, C. A. Biological soil health indicators respond to tillage intensity: a US meta-analysis. Geoderma 369 , 114335 (2020).
Kaiser, E. A. et al. Nitrous oxide release from arable soil: importance of N-fertilization, crops and temporal variation. Soil Biol. Biochem. 30 , 1553–1563 (1998).
Baldock, J. A., Beare, M. H., Curtin, D. & Hawke, B. Stocks, composition and vulnerability to loss of soil organic carbon predicted using mid-infrared spectroscopy. Soil Res. 56 , 468–480 (2018).
Rossel, R. V. et al. Continental-scale soil carbon composition and vulnerability modulated by regional environmental controls. Nat. Geosci. 12 , 547–552 (2019).
Six, J., Bossuyt, H., Degryze, S. & Denef, K. A history of research on the link between (micro)aggregates, soil biota, and soil organic matter dynamics. Soil Tillage Res. 79 , 7–31 (2004).
Pietrelli, A., Bavasso, I., Lovecchio, N., Ferrara, V. & Allard, B. in 8th IEEE International Workshop on Advances in Sensors and Interfaces (IWASI) 302–306 (IEEE, 2019).
Shaikh, F. K. & Zeadally, S. Energy harvesting in wireless sensor networks: a comprehensive review. Renew. Sustain. Energy Rev. 55 , 1041–1054 (2016).
Tan, X., Sun, Z., Wang, P. & Sun, Y. Environment-aware localization for wireless sensor networks using magnetic induction. Ad Hoc Netw. 98 , 102030 (2020).
Lehmann, J. et al. Persistence of soil organic carbon caused by functional complexity. Nat. Geosci. 13 , 529–534 (2020).
Tedersoo, L. et al. Global diversity and geography of soil fungi. Science 346 , 1078 (2014).
Van Den Hoogen, J. et al. Soil nematode abundance and functional group composition at a global scale. Nature 572 , 194–198 (2019).
Rillig, M. C., Bonneval, K. & Lehmann, J. Sounds of soil: a new world of interactions under our feet? Soil Syst. 3 , 45 (2019).
Smolka, M. et al. A mobile lab-on-a-chip device for on-site soil nutrient analysis. Precision Agric. 18 , 152–168 (2017).
Rossel, R. A. V. & Bouma, J. Soil sensing: a new paradigm for agriculture. Agric. Syst. 148 , 71–74 (2016).
Ali, M. A., Dong, L., Dhau, J., Khosla, A. & Kaushik, A. Perspective — electrochemical sensors for soil quality assessment. J. Electrochem. Soc. 167 , 037550 (2020).
Enell, A. et al. Combining leaching and passive sampling to measure the mobility and distribution between porewater, DOC, and colloids of native oxy-PAHs, N-PACs, and PAHs in historically contaminated soil. Environ. Sci. Technol. 50 , 11797–11805 (2016).
Sismaet, H. J. & Goluch, E. D. Electrochemical probes of microbial community behaviour. Annu. Rev. Anal. Chem. 11 , 441–461 (2018).
Chabrillat, S. et al. Imaging spectroscopy for soil mapping and monitoring. Surv. Geophys. 40 , 361–399 (2019).
Mohanty, B. P., Cosh, M. H., Lakshmi, V. & Montzka, C. Soil moisture remote sensing: state-of-the-science. Vadose Zone J. 16 , 1–9 (2017).
Paustian, K. et al. Quantifying carbon for agricultural soil management: from the current status toward a global soil information system. Carbon Manage. 10 , 567–587 (2019).
Duckett, T. et al. Agricultural robotics: the future of robotic agriculture. Preprint arXiv https://arxiv.org/abs/1806.06762 (2018).
Hussain, I., Olson, K. R., Wander, M. M. & Karlen, D. L. Adaptation of soil quality indices and application to three tillage systems in southern Illinois. Soil Tillage Res. 50 , 237–249 (1999).
Fine, A. K., van Es, H. M. & Schindelbeck, R. R. Statistics, scoring functions, and regional analysis of a comprehensive soil health database. Soil Sci. Soc. Am. J. 81 , 589–601 (2017).
Svoray, T., Hassid, I., Atkinson, P. M., Moebius-Clune, B. N. & van Es, H. M. Mapping soil health over large agriculturally important areas. Soil Sci. Soc. Am. J. 79 , 1420–1434 (2015).
Moebius-Clune, B. N. et al. Comprehensive Assessment of Soil Health – The Cornell Framework, Edition 3.1 (Cornell Univ. Press, 2016).
Wiesmeier, M. et al. Soil organic carbon storage as a key function of soils-a review of drivers and indicators at various scales. Geoderma 333 , 149–162 (2019).
Lima, A. C. R., Brussaard, L., Totola, M. R., Hoogmoed, W. B. & de Goede, R. G. M. A functional evaluation of three indicator sets for assessing soil quality. Appl. Soil Ecol. 64 , 194–200 (2013).
Verheijen, F. G., Bellamy, P. H., Kibblewhite, M. G. & Gaunt, J. L. Organic carbon ranges in arable soils of England and Wales. Soil Use Manage. 21 , 2–9 (2005).
Bucka, F. B., Kölbl, A., Uteau, D., Peth, S. & Kögel-Knabner, I. Organic matter input determines structure development and aggregate formation in artificial soils. Geoderma 354 , 113881 (2019).
Kaiser, K. et al. Driving forces of soil bacterial community structure, diversity, and function in temperate grasslands and forests. Sci. Rep. 6 , 33696 (2016).
Jordan-Meille, L. et al. An overview of fertilizer-P recommendations in Europe: soil testing, calibration and fertilizer recommendations. Soil Use Manage. 28 , 419–435 (2012).
McLellan, E. L. et al. The nitrogen balancing act: tracking the environmental performance of food production. Bioscience 68 , 194–203 (2018).
Brevik, E. C. & Sauer, T. J. The past, present, and future of soils and human health studies. Soil 1 , 35–46 (2015).
Pereira, P., Bogunovic, I., Muñoz-Rojas, M. & Brevik, E. C. Soil ecosystem services, sustainability, valuation and management. Curr. Opin. Environ. Sci. Health 5 , 7–13 (2018).
Bampa, F. et al. Harvesting European knowledge on soil functions and land management using multi-criteria decision analysis. Soil Use Manage. 35 , 6–20 (2019).
Schulte, R. P. et al. Demands on land: mapping competing societal expectations for the functionality of agricultural soils in Europe. Environ. Sci. Policy 100 , 113–125 (2019).
Ward, M. O., Grinstein, G. & Keim, D. Interactive Data Visualization: Foundations, Techniques, and Applications (AK Peters/CRC Press, 2015).
Villamil, M. B., Miguez, F. E. & Bollero, G. A. Multivariate analysis and visualization of soil quality data for no-till systems. J. Environ. Qual. 37 , 2063–2069 (2008).
Börner, K., Bueckle, A. & Ginda, M. Data visualization literacy: definitions, conceptual frameworks, exercises, and assessments. Proc. Natl Acad. Sci. USA 116 , 1857–1864 (2019).
Reichstein, M. et al. Deep learning and process understanding for data-driven Earth system science. Nature 566 , 195–204 (2019).
Tian, R. & Wu, J. Groundwater quality appraisal by improved set pair analysis with game theory weightage and health risk estimation of contaminants for Xuecha drinking water source in a loess area in Northwest China. Hum. Ecol. Risk Assess. Int. J. 25 , 132–157 (2019).
Finger, R., Swinton, S. M., Benni, N. E. & Walter, A. Precision farming at the nexus of agricultural production and the environment. Annu. Rev. Resour. Econ. 11 , 313–335 (2019).
van Joolingen, W. R., de Jong, T., Lazonder, A. W., Savelsbergh, E. R. & Manlove, S. Co-Lab: research and development of an online learning environment for collaborative scientific discovery learning. Comput. Hum. Behav. 21 , 671–688 (2005).
Stott, D. E. Recommended Soil Health Indicators and Associated Laboratory Procedures. Soil Health Technical Note No. 450-03 (U.S. Department of Agriculture, Natural Resources Conservation Service, 2019).
Haberern, J. A soil health index. J. Soil Water Conserv. 47 , 6 (1992).
Pankhurst, C. E. et al. Evaluation of soil biological properties as potential bioindicators of soil health. Austr. J. Exp. Agric. 35 , 1015–1028 (1995).
Doran, J. W. & Zeiss, M. R. Soil health and sustainability: managing the biotic component of soil quality. Appl. Soil Ecol. 15 , 3–11 (2000).
Winiwarter, V. & Blum, W. E. in Footprints in the Soil. People and Ideas in Soil History (ed. Warkentin, B.) 107–122 (Elsevier, 2006).
Capra, G. F., Ganga, A. & Moore, A. F. Songs for our soils. How soil themes have been represented in popular song. Soil Sci. Plant Nutr. 63 , 517–525 (2017).
Jenny, H. in Study Week on Organic Matter and Soil Fertility. Pontificiae Academiae Scientarium Scripta, Varia 32. 947–979 (North Holland Publ. Co and Wiley Interscience Division, 1968).
Feller, C., Landa, E. R., Toland, A. & Wessolek, G. Case studies of soil in art. Soil 1 , 543–559 (2015).
Brevik, E. C. & Hartemink, A. E. Early soil knowledge and the birth and development of soil science. Catena 83 , 23–33 (2010).
Carson, R. Silent Spring (Houghton Mifflin, 1962).
Lovelock, J. E. Gaia, a New Look at Life on Earth (Oxford Univ. Press, 1979).
Keesstra, S. D. et al. The significance of soils and soil science towards realization of the United Nations Sustainable Development Goals. Soil 2 , 111–128 (2016).
Mausel, P. W. Soil quality in Illinois — an example of a soils geography resource analysis. Prof. Geogr. 23 , 127–136 (1971).
Sojka, R. E. & Upchurch, D. R. Reservations regarding the soil quality concept. Soil Sci. Soc. Am. J. 63 , 1039–1054 (1999).
Rumpel, C. et al. Put more carbon in soils to meet Paris climate pledges. Nature 564 , 32–34 (2018).
Freidberg, S. Assembled but unrehearsed: corporate food power and the ‘dance’ of supply chain sustainability. J. Peasant Stud. 47 , 383–400 (2020).
Chabbi, A. et al. Aligning agriculture and climate policy. Nat. Clim. Change 7 , 307–309 (2017).
Puig de la Bellacasa, M. Re-animating soils: transforming human–soil affections through science, culture and community. Sociol. Rev. 67 , 391–407 (2019).
Download references
Acknowledgements
J.L. acknowledges the Hans Fischer Senior Fellowship of the Institute for Advanced Study (Technical University Munich) and a TNC-ACSF project (Cornell University), D.A.B. the support by the Craig and Susan McCaw Foundation, I.K.-K. the support by the German Federal Ministry of Education and Research (BMBF) in the framework of the funding measure ‘Soil as a Sustainable Resource for the Bioeconomy’ (BonaRes project), BonaRes Centre for Soil Research (FKZ 031B0516A; BonaRes, Module A) and M.C.R. an ERC Advanced Grant (694368) and the BMBF for the project ‘Bridging in Biodiversity Science (BIBS)’ (01LC1501A). Sincere thanks to Else Bünemann-König for sharing raw data.
Author information
Authors and affiliations.
School of Integrative Plant Science, Cornell University, Ithaca, NY, USA
Johannes Lehmann
Cornell Atkinson Center for Sustainability, Cornell University, Ithaca, NY, USA
Institute for Advanced Study, Technical University of Munich, Garching, Germany
Johannes Lehmann & Ingrid Kögel-Knabner
The Nature Conservancy, Arlington, VA, USA
Deborah A. Bossio
Chair of Soil Science, Technical University of Munich, Freising, Germany
Ingrid Kögel-Knabner
Institut für Biologie, Freie Universität Berlin, Berlin, Germany
Matthias C. Rillig
Berlin-Brandenburg Institute of Advanced Biodiversity Research (BBIB), Berlin, Germany
You can also search for this author in PubMed Google Scholar
Contributions
All authors contributed substantial discussion of content, edited the manuscript and its revisions.
Corresponding author
Correspondence to Johannes Lehmann .
Ethics declarations
Competing interests.
The authors declare no competing interests.
Additional information
Peer review information.
Nature Reviews Earth & Environment thanks Hailin Zhang, Nanthi Bolan and the other, anonymous, reviewer(s) for their contribution to the peer review of this work.
Publisher’s note
Springer Nature remains neutral with regard to jurisdictional claims in published maps and institutional affiliations.
Rights and permissions
Reprints and permissions
About this article
Cite this article.
Lehmann, J., Bossio, D.A., Kögel-Knabner, I. et al. The concept and future prospects of soil health. Nat Rev Earth Environ 1 , 544–553 (2020). https://doi.org/10.1038/s43017-020-0080-8
Download citation
Accepted : 06 July 2020
Published : 25 August 2020
Issue Date : October 2020
DOI : https://doi.org/10.1038/s43017-020-0080-8
Share this article
Anyone you share the following link with will be able to read this content:
Sorry, a shareable link is not currently available for this article.
Provided by the Springer Nature SharedIt content-sharing initiative
This article is cited by
Artificially regulated humification in creating humic-like biostimulators.
npj Clean Water (2024)
Patterns and determinants of microbial- and plant-derived carbon contributions to soil organic carbon in tea plantation chronosequence
- Xiaozhi Wang
Plant and Soil (2024)
Integrating farmers’ and experts’ perspectives for soil health-informed decision-making in conservation agriculture systems
- Akhtar Veisi
- Korous Khoshbakht
- Reza Haghparast Tanha
Environment Systems and Decisions (2024)
An Assessment of Subjective Well-Being of Inter-Provincial Migrant Workers in Balochistan, Pakistan
International Journal of Community Well-Being (2024)
No farm is an island: constrained choice, landscape thinking, and ecological insect management among Wisconsin farmers
- Benjamin Iuliano
Agriculture and Human Values (2024)
Quick links
- Explore articles by subject
- Guide to authors
- Editorial policies
Sign up for the Nature Briefing: Microbiology newsletter — what matters in microbiology research, free to your inbox weekly.

Information
- Author Services
Initiatives
You are accessing a machine-readable page. In order to be human-readable, please install an RSS reader.
All articles published by MDPI are made immediately available worldwide under an open access license. No special permission is required to reuse all or part of the article published by MDPI, including figures and tables. For articles published under an open access Creative Common CC BY license, any part of the article may be reused without permission provided that the original article is clearly cited. For more information, please refer to https://www.mdpi.com/openaccess .
Feature papers represent the most advanced research with significant potential for high impact in the field. A Feature Paper should be a substantial original Article that involves several techniques or approaches, provides an outlook for future research directions and describes possible research applications.
Feature papers are submitted upon individual invitation or recommendation by the scientific editors and must receive positive feedback from the reviewers.
Editor’s Choice articles are based on recommendations by the scientific editors of MDPI journals from around the world. Editors select a small number of articles recently published in the journal that they believe will be particularly interesting to readers, or important in the respective research area. The aim is to provide a snapshot of some of the most exciting work published in the various research areas of the journal.
Original Submission Date Received: .
- Active Journals
- Find a Journal
- Proceedings Series
- For Authors
- For Reviewers
- For Editors
- For Librarians
- For Publishers
- For Societies
- For Conference Organizers
- Open Access Policy
- Institutional Open Access Program
- Special Issues Guidelines
- Editorial Process
- Research and Publication Ethics
- Article Processing Charges
- Testimonials
- Preprints.org
- SciProfiles
- Encyclopedia
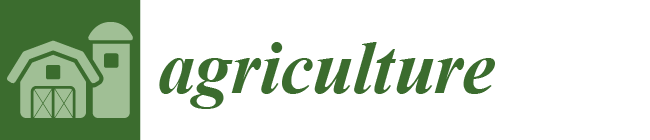
Article Menu
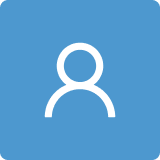
- Subscribe SciFeed
- Recommended Articles
- Author Biographies
- Google Scholar
- on Google Scholar
- Table of Contents
Find support for a specific problem in the support section of our website.
Please let us know what you think of our products and services.
Visit our dedicated information section to learn more about MDPI.
JSmol Viewer
Trends in soil science over the past three decades (1992–2022) based on the scientometric analysis of 39 soil science journals.
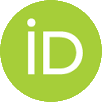
1. Introduction
2. materials and methods, 3. results and discussion, 3.1. general information of the 39 soil science journals, 3.2. publication volume and citation metrics, 3.3. journal impact factor and citescore trends, 3.4. bibliometric analysis, 3.4.1. countries and organizations, 3.4.2. co-authorship and most influential publications, 3.4.3. co-authorship and most influential publications, 3.5. emerging issues on soil science publications, 3.5.1. research performance assessment in countries and organizations, 3.5.2. equity, diversity, and inclusion, 3.5.3. artificial intelligence-based research in soil science, 4. conclusions and perspectives, author contributions, institutional review board statement, data availability statement, acknowledgments, conflicts of interest.
- Abrahams, P.W. Soils: Their implications to human health. Sci. Total Environ. 2002 , 291 , 1–32. [ Google Scholar ] [ CrossRef ] [ PubMed ]
- Totsche, K.U.; Amelung, W.; Gerzabek, M.H.; Guggenberger, G.; Klumpp, E.; Knief, C.; Lehndorff, E.; Mikutta, R.; Peth, S.; Prechtel, A.; et al. Microaggregates in soils. J. Plant Nutr. Soil Sci. 2018 , 181 , 104–136. [ Google Scholar ] [ CrossRef ]
- Young, I.M.; Crawford, J.W. Interactions and Self-Organization in the Soil-Microbe Complex. Science 2004 , 304 , 1634–1637. [ Google Scholar ] [ CrossRef ]
- Pronk, G.J.; Heister, K.; Vogel, C.; Babin, D.; Bachmann, J.; Ding, G.-C.; Ditterich, F.; Gerzabek, M.H.; Giebler, J.; Hemkemeyer, M.; et al. Interaction of minerals, organic matter, and microorganisms during biogeochemical interface formation as shown by a series of artificial soil experiments. Biol. Fertil. Soils 2017 , 53 , 9–22. [ Google Scholar ] [ CrossRef ]
- Andrea, F.; Bini, C.; Amaducci, S. Soil and ecosystem services: Current knowledge and evidences from Italian case studies. Appl. Soil Ecol. 2018 , 123 , 693–698. [ Google Scholar ] [ CrossRef ]
- Doula, M.K.; Sarris, A. Chapter 4—Soil Environment. In Environment and Development ; Poulopoulos, S.G., Inglezakis, V.J., Eds.; Elsevier: Amsterdam, The Netherlands, 2016; pp. 213–286. [ Google Scholar ]
- Wilding, L.P.; Lin, H. Advancing the frontiers of soil science towards a geoscience. Geoderma 2006 , 131 , 257–274. [ Google Scholar ] [ CrossRef ]
- van Baren, H.; Hartemink, A.E.; Tinker, P.B. 75 years The International Society of Soil Science. Geoderma 2000 , 96 , 1–18. [ Google Scholar ] [ CrossRef ]
- Hartemink, A.E.; McBratney, A.B.; Cattle, J.A. Developments and trends in soil science: 100 volumes of Geoderma (1967–2001). Geoderma 2001 , 100 , 217–268. [ Google Scholar ] [ CrossRef ]
- Tinker, P.B. Soil science in a changing world. J. Soil Sci. 1985 , 36 , 1–8. [ Google Scholar ] [ CrossRef ]
- Brevik, E.C.; Hartemink, A.E. Early soil knowledge and the birth and development of soil science. Catena 2010 , 83 , 23–33. [ Google Scholar ] [ CrossRef ]
- Rojas, R.V.; Caon, L. The international year of soils revisited: Promoting sustainable soil management beyond 2015. Environ. Earth Sci. 2016 , 75 , 1184. [ Google Scholar ] [ CrossRef ]
- Brevik, E.C.; Calzolari, C.; Miller, B.A.; Pereira, P.; Kabala, C.; Baumgarten, A.; Jordán, A. Soil mapping, classification, and pedologic modeling: History and future directions. Geoderma 2016 , 264 , 256–274. [ Google Scholar ] [ CrossRef ]
- Landa, E.R.; Brevik, E.C. Soil science and its interface with the history of geology community. Earth Sci. Hist. 2015 , 34 , 296–309. [ Google Scholar ] [ CrossRef ]
- Hartemink, A.E.; McBratney, A. A soil science renaissance. Geoderma 2008 , 148 , 123–129. [ Google Scholar ] [ CrossRef ]
- Minasny, B.; Hartemink, A.E.; McBratney, A. Individual, country, and journal self-citation in soil science. Geoderma 2010 , 155 , 434–438. [ Google Scholar ] [ CrossRef ]
- Hartemink, A.E. Open access publishing and soil science—Trends and developments. Geoderma Reg. 2019 , 18 , e00231. [ Google Scholar ] [ CrossRef ]
- Liu, Y.; Wu, K.; Zhao, R. Bibliometric analysis of research on soil health from 1999 to 2018. J. Soils Sediments 2020 , 20 , 1513–1525. [ Google Scholar ] [ CrossRef ]
- Zhang, D.; Dyck, M.; Filipović, L.; Filipović, V.; Lv, J.; He, H. Hyperaccumulators for Potentially Toxic Elements: A Scientometric Analysis. Agronomy 2021 , 11 , 1729. [ Google Scholar ] [ CrossRef ]
- Pan, X.Y.; Lv, J.L.; Dyck, M.; He, H.L. Bibliometric Analysis of Soil Nutrient Research between 1992 and 2020. Agriculture 2021 , 11 , 223. [ Google Scholar ] [ CrossRef ]
- He, D.; Bristow, K.; Filipović, V.; Lv, J.; He, H. Microplastics in Terrestrial Ecosystems: A Scientometric Analysis. Sustainability 2020 , 12 , 8739. [ Google Scholar ] [ CrossRef ]
- Oliveira, J.D.; Pereira, M.G. Global soil science research on drylands: An analysis of research evolution, collaboration, and trends. J. Soils Sediments 2021 , 21 , 3856–3867. [ Google Scholar ] [ CrossRef ]
- Feng, S.N.; Zhang, H.; Lv, J.L.; Dyck, M.; Wu, Q.B.; He, H.L. A Scientometric Review of Research Status on Unfrozen Soil Water. Water 2021 , 13 , 708. [ Google Scholar ] [ CrossRef ]
- Feng, S.; Chen, J.; Jones, S.B.; Flerchinger, G.; Dyck, M.; Filipovic, V.; Hu, Y.; Si, B.; Lv, J.; Wu, Q.; et al. Miscellaneous methods for determination of unfrozen water content in frozen soils. J. Hydrol. 2024 , 631 , 130802. [ Google Scholar ] [ CrossRef ]
- Bezak, N.; Mikos, M.; Borrelli, P.; Alewell, C.; Alvarez, P.; Anache, J.A.A.; Baartman, J.; Ballabio, C.; Biddoccu, M.; Cerda, A.; et al. Soil erosion modelling: A bibliometric analysis. Environ. Res. 2021 , 197 , 111087. [ Google Scholar ] [ CrossRef ] [ PubMed ]
- Zhang, H.; He, H.; Gao, Y.; Mady, A.; Filipović, V.; Dyck, M.; Lv, J.; Liu, Y. Applications of Computed Tomography (CT) in environmental soil and plant sciences. Soil Tillage Res. 2023 , 226 , 105574. [ Google Scholar ] [ CrossRef ]
- He, H.; Dyck, M.F.; Horton, R.; Ren, T.; Bristow, K.L.; Lv, J.; Si, B. Development and Application of the Heat Pulse Method for Soil Physical Measurements. Rev. Geophys. 2018 , 56 , 567–620. [ Google Scholar ] [ CrossRef ]
- He, H.; Dyck, M.F.; Horton, R.; Li, M.; Jin, H.; Si, B. Chapter Five—Distributed Temperature Sensing for Soil Physical Measurements and Its Similarity to Heat Pulse Method. In Advances in Agronomy ; Sparks, D.L., Ed.; Academic Press: Cambridge, MA, USA, 2018; Volume 148, pp. 173–230. [ Google Scholar ]
- He, H.; Dyck, M.; Lv, J. The Heat Pulse Method for Soil Physical Measurements: A Bibliometric Analysis. Appl. Sci. 2020 , 10 , 6171. [ Google Scholar ] [ CrossRef ]
- Li, K.; Horton, R.; He, H. Application of machine learning algorithms to model soil thermal diffusivity. Int. Commun. Heat Mass Transf. 2023 , 149 , 107092. [ Google Scholar ] [ CrossRef ]
- Wang, X.W.; Yang, Y.Z.; Lv, J.L.; He, H.L. Past, present and future of the applications of machine learning in soil science and hydrology. Soil Water Res. 2023 , 18 , 67–80. [ Google Scholar ] [ CrossRef ]
- Zhao, T.; Liu, S.; Xu, J.; He, H.; Wang, D.; Horton, R.; Liu, G. Comparative analysis of seven machine learning algorithms and five empirical models to estimate soil thermal conductivity. Agric. For. Meteorol. 2022 , 323 , 109080. [ Google Scholar ] [ CrossRef ]
- Agyeman, P.C.; Ahado, S.K.; Boruvka, L.; Biney, J.K.M.; Sarkodie, V.Y.O.; Kebonye, N.M.; Kingsley, J. Trend analysis of global usage of digital soil mapping models in the prediction of potentially toxic elements in soil/sediments: A bibliometric review. Environ. Geochem. Health 2021 , 43 , 1715–1739. [ Google Scholar ] [ CrossRef ] [ PubMed ]
- Yang, J.H.; Wu, G.L.; Jiao, J.Y.; Dyck, M.; He, H.L. Freeze-thaw induced landslides on grasslands in cold regions. Catena 2022 , 219 , 106650. [ Google Scholar ] [ CrossRef ]
- Aria, M.; Cuccurullo, C. bibliometrix: An R-tool for comprehensive science mapping analysis. J. Informetr. 2017 , 11 , 959–975. [ Google Scholar ] [ CrossRef ]
- van Eck, N.J.; Waltman, L. Software survey: VOSviewer, a computer program for bibliometric mapping. Scientometrics 2010 , 84 , 523–538. [ Google Scholar ] [ CrossRef ] [ PubMed ]
- Chen, C.M. CiteSpace II: Detecting and visualizing emerging trends and transient patterns in scientific literature. J. Am. Soc. Inf. Sci. Technol. 2006 , 57 , 359–377. [ Google Scholar ] [ CrossRef ]
- Rodríguez-Soler, R.; Uribe-Toril, J.; De Pablo Valenciano, J. Worldwide trends in the scientific production on rural depopulation, a bibliometric analysis using bibliometrix R-tool. Land Use Policy 2020 , 97 , 104787. [ Google Scholar ] [ CrossRef ]
- Durieux, V.; Gevenois, P.A. Bibliometric Indicators: Quality Measurements of Scientific Publication. Radiology 2010 , 255 , 342–351. [ Google Scholar ] [ CrossRef ]
- He, Z.; Gong, K.; Zhang, Z.; Dong, W.; Feng, H.; Yu, Q.; He, J. What is the past, present, and future of scientific research on the Yellow River Basin?—A bibliometric analysis. Agric. Water Manag. 2022 , 262 , 107404. [ Google Scholar ] [ CrossRef ]
- Chen, C.; Ibekwe-SanJuan, F.; Hou, J. The structure and dynamics of cocitation clusters: A multiple-perspective cocitation analysis. J. Am. Soc. Inf. Sci. Technol. 2010 , 61 , 1386–1409. [ Google Scholar ] [ CrossRef ]
- Nguyen, V.M.; Haddaway, N.R.; Gutowsky, L.F.G.; Wilson, A.D.M.; Gallagher, A.J.; Donaldson, M.R.; Hammerschlag, N.; Cooke, S.J. How Long Is Too Long in Contemporary Peer Review? Perspectives from Authors Publishing in Conservation Biology Journals. PLoS ONE 2015 , 10 , e0132557. [ Google Scholar ] [ CrossRef ]
- Kovanis, M.; Porcher, R.; Ravaud, P.; Trinquart, L. The Global Burden of Journal Peer Review in the Biomedical Literature: Strong Imbalance in the Collective Enterprise. PLoS ONE 2016 , 11 , e0166387. [ Google Scholar ] [ CrossRef ]
- Cicchetti, D.V. The reliability of peer review for manuscript and grant submissions: A cross-disciplinary investigation. Behav. Brain Sci. 1991 , 14 , 119–135. [ Google Scholar ] [ CrossRef ]
- Fang, H. A modification of citation-based journal indexes. Scientometrics 2023 , 128 , 1119–1132. [ Google Scholar ] [ CrossRef ]
- Zhang, X. Effect of reviewer’s origin on peer review: China vs. non-China. Learn. Publ. 2012 , 25 , 265–270. [ Google Scholar ] [ CrossRef ]
- Qeios. Qeios Publishing Policy. Available online: https://www.qeios.com/publishing-policy#how-reviewing-process-works (accessed on 29 May 2023).
- Mulligan, A.; Hall, L.; Raphael, E. Peer review in a changing world: An international study measuring the attitudes of researchers. J. Am. Soc. Inf. Sci. Technol. 2013 , 64 , 132–161. [ Google Scholar ] [ CrossRef ]
- Gruda, D. Dear journals: Stop hoarding our papers. Nature Careers Community , 10 October 2023. [ Google Scholar ] [ CrossRef ]
- Donahue, M.Z. An Avid New Audience for Preprints—Extremists: Preprints can speed scientific communication but offer fuel for nefarious agendas. BioScience 2021 , 71 , 1004–1010. [ Google Scholar ] [ CrossRef ]
- Flury, M.; Lakshmi, V.; Lu, N.; Vanderborght, J. Editorial: Open Access on the move. Vadose Zone J. 2023 , 22 , e20237. [ Google Scholar ] [ CrossRef ]
- European Science Foundation. Plan S: Making Full and Immediate Open Access a Reality. Available online: https://www.coalition-s.org (accessed on 29 May 2023).
- The White House. Breakthroughs for All: Delivering Equitable Access to America’s Research [Office of Science and Technology Policy Blog]. Available online: https://www.whitehouse.gov/ostp/news-updates/2022/08/25/breakthroughs-for-alldelivering-equitable-access-to-americas-research (accessed on 29 May 2023).
- Schimmer, R.; Geschuhn, K.K.; Vogler, A. Disrupting the Subscription Journals’ Business Model for the Necessary Large-Scale Transformation to Open Access ; ScienceOpen Research: Burlington, MA, USA, 2015; pp. 1–7. [ Google Scholar ] [ CrossRef ]
- Siler, K. Demarcating spectrums of predatory publishing: Economic and institutional sources of academic legitimacy. J. Assoc. Inf. Sci. Technol. 2020 , 71 , 1386–1401. [ Google Scholar ] [ CrossRef ]
- Roldan-Valadez, E.; Salazar-Ruiz, S.Y.; Ibarra-Contreras, R.; Rios, C. Current concepts on bibliometrics: A brief review about impact factor, Eigenfactor score, CiteScore, SCImago Journal Rank, Source-Normalised Impact per Paper, H-index, and alternative metrics. Ir. J. Med. Sci. 2019 , 188 , 939–951. [ Google Scholar ] [ CrossRef ] [ PubMed ]
- Van Noorden, R. Controversial impact factor gets a heavyweight rival. Nature 2016 , 540 , 325–326. [ Google Scholar ] [ CrossRef ]
- Atayero, A.A.; Popoola, S.I.; Egeonu, J.; Oludayo, O. Citation analytics: Data exploration and comparative analyses of CiteScores of Open Access and Subscription-Based publications indexed in Scopus (2014–2016). Data Brief 2018 , 19 , 198–213. [ Google Scholar ] [ CrossRef ] [ PubMed ]
- Fang, H. Analysis of the new scopus CiteScore. Scientometrics 2021 , 126 , 5321–5331. [ Google Scholar ] [ CrossRef ]
- Okagbue, H.I.; Teixeira da Silva, J.A. Correlation between the CiteScore and Journal Impact Factor of top-ranked library and information science journals. Scientometrics 2020 , 124 , 797–801. [ Google Scholar ] [ CrossRef ]
- Quaderi, N. The Road to Journal Citation Reports 2021: New Content and a New Metric. Available online: https://clarivate.com/blog/the-road-to-journal-citation-reports-2021-new-content-and-a-new-metric/ (accessed on 29 May 2023).
- Clark, M.P.; Hanson, R.B. The citation impact of hydrology journals. Water Resour. Res. 2017 , 53 , 4533–4541. [ Google Scholar ] [ CrossRef ]
- Vinkler, P. Correlation between the structure of scientific research, scientometric indicators and GDP in EU and non-EU countries. Scientometrics 2008 , 74 , 237–254. [ Google Scholar ] [ CrossRef ]
- Lal, R. Soil carbon sequestration impacts on global climate change and food security. Science 2004 , 304 , 1623–1627. [ Google Scholar ] [ CrossRef ] [ PubMed ]
- Minasny, B.; Malone, B.P.; McBratney, A.B.; Angers, D.A.; Arrouays, D.; Chambers, A.; Chaplot, V.; Chen, Z.S.; Cheng, K.; Das, B.S.; et al. Soil carbon 4 per mille. Geoderma 2017 , 292 , 59–86. [ Google Scholar ] [ CrossRef ]
- Six, J.; Conant, R.T.; Paul, E.A.; Paustian, K. Stabilization mechanisms of soil organic matter: Implications for C-saturation of soils. Plant Soil 2002 , 241 , 155–176. [ Google Scholar ] [ CrossRef ]
- Shen, Q.R.; Ran, W.; Cao, Z.H. Mechanisms of nitrite accumulation occurring in soilnitrification. Chemosphere 2003 , 50 , 747–753. [ Google Scholar ] [ CrossRef ]
- Whalen, J.K.; Chang, C.; Clayton, G.W.; Carefoot, J.P. Cattle manure amendments can increase the pH of acid soils. Soil Sci. Soc. Am. J. 2000 , 64 , 962–966. [ Google Scholar ] [ CrossRef ]
- Liu, M.Q.; Hu, F.; Chen, X.Y.; Huang, Q.R.; Jiao, J.G.; Zhang, B.; Li, H.X. Organic amendments with reduced chemical fertilizer promote soil microbial development and nutrient availability in a subtropical paddy field: The influence of quantity, type and application time of organic amendments. Appl. Soil Ecol. 2009 , 42 , 166–175. [ Google Scholar ] [ CrossRef ]
- Marschner, P.; Yang, C.H.; Lieberei, R.; Crowley, D.E. Soil and plant specific effects on bacterial community composition in the rhizosphere. Soil Biol. Biochem. 2001 , 33 , 1437–1445. [ Google Scholar ] [ CrossRef ]
- Lambers, H.; Raven, J.A.; Shaver, G.R.; Smith, S.E. Plant nutrient-acquisition strategies change with soil age. Trends Ecol. Evol. 2008 , 23 , 95–103. [ Google Scholar ] [ CrossRef ]
- Rengel, Z. Availability of Mn, Zn and Fe in the rhizosphere. J. Soil Sci. Plant Nutr. 2015 , 15 , 397–409. [ Google Scholar ] [ CrossRef ]
- Kuzyakov, Y.; Friedel, J.K.; Stahr, K. Review of mechanisms and quantification of priming effects. Soil Biol. Biochem. 2000 , 32 , 1485–1498. [ Google Scholar ] [ CrossRef ]
- He, J.; Shen, J.; Zhang, L.; Zhu, Y.G.; Zheng, Y.; Xu, M.; Di, H.J. Quantitative analyses of the abundance and composition of ammonia-oxidizing bacteria and ammonia-oxidizing archaea of a Chinese upland red soil under long-term fertilization practices. Environ. Microbiol. 2007 , 9 , 2364–2374. [ Google Scholar ] [ CrossRef ] [ PubMed ]
- Frouz, J. Effects of soil macro- and mesofauna on litter decomposition and soil organic matter stabilization. Geoderma 2018 , 332 , 161–172. [ Google Scholar ] [ CrossRef ]
- Ge, N.N.; Wei, X.R.; Wang, X.; Liu, X.T.; Shao, M.A.; Jia, X.X.; Li, X.Z.; Zhang, Q.Y. Soil texture determines the distribution of aggregate-associated carbon, nitrogen and phosphorous under two contrasting land use types in the Loess Plateau. Catena 2019 , 172 , 148–157. [ Google Scholar ] [ CrossRef ]
- Lu, S.; Ren, T.S.; Gong, Y.S.; Horton, R. An improved model for predicting soil thermal conductivity from water content at room temperature. Soil Sci. Soc. Am. J. 2007 , 71 , 8–14. [ Google Scholar ] [ CrossRef ]
- Ochsner, T.E.; Horton, R.; Ren, T.H. A new perspective on soil thermal properties. Soil Sci. Soc. Am. J. 2001 , 65 , 1641–1647. [ Google Scholar ] [ CrossRef ]
- Ren, T.S.; Ochsner, T.E.; Horton, R. Development of Thermo-Time Domain Reflectometry for Vadose Zone Measurements. Vadose Zone J. 2003 , 2 , 544–551. [ Google Scholar ] [ CrossRef ]
- Horn, R.; Peng, X.H.; Fleige, H.; Dorner, J. Pore rigidity in structured soils-only a theoretical boundary condition for hydraulic properties? Soil Sci. Plant Nutr. 2014 , 60 , 3–14. [ Google Scholar ] [ CrossRef ]
- Vereecken, H.; Huisman, J.A.; Bogena, H.; Vanderborght, J.; Vrugt, J.A.; Hopmans, J.W. On the value of soil moisture measurements in vadose zone hydrology: A review. Water Resour. Res. 2008 , 44 , W00D06. [ Google Scholar ] [ CrossRef ]
- Vanderborght, J.; Vereecken, H. Review of dispersivities for transport modeling in soils. Vadose Zone J. 2007 , 6 , 29–52. [ Google Scholar ] [ CrossRef ]
- Jones, D.L.; Nguyen, C.; Finlay, R.D. Carbon flow in the rhizosphere: Carbon trading at the soil-root interface. Plant Soil 2009 , 321 , 5–33. [ Google Scholar ] [ CrossRef ]
- Zhu, Z.K.; Zeng, G.J.; Ge, T.D.; Hu, Y.J.; Tong, C.L.; Shibistova, O.; He, X.H.; Wang, J.; Guggenberger, G.; Wu, J.S. Fate of rice shoot and root residues, rhizodeposits, and microbe-assimilated carbon in paddy soil—Part 1: Decomposition and priming effect. Biogeosciences 2016 , 13 , 4481–4489. [ Google Scholar ] [ CrossRef ]
- Yuan, H.Z.; Zhu, Z.K.; Liu, S.L.; Ge, T.D.; Jing, H.Z.; Li, B.Z.; Liu, Q.; Lynn, T.M.; Wu, J.S.; Kuzyakov, Y. Microbial utilization of rice root exudates: C-13 labeling and PLFA composition. Biol. Fertil. Soils 2016 , 52 , 615–627. [ Google Scholar ] [ CrossRef ]
- Lehmann, J.; Rillig, M.C.; Thies, J.; Masiello, C.A.; Hockaday, W.C.; Crowley, D. Biochar effects on soil biota—A review. Soil Biol. Biochem. 2011 , 43 , 1812–1836. [ Google Scholar ] [ CrossRef ]
- Six, J.; Bossuyt, H.; Degryze, S.; Denef, K. A history of research on the link between (micro)aggregates, soil biota, and soil organic matter dynamics. Soil Tillage Res. 2004 , 79 , 7–31. [ Google Scholar ] [ CrossRef ]
- von Lutzow, M.; Kogel-Knabner, I.; Ekschmitt, K.; Matzner, E.; Guggenberger, G.; Marschner, B.; Flessa, H. Stabilization of organic matter in temperate soils: Mechanisms and their relevance under different soil conditions—A review. Eur. J. Soil Sci. 2006 , 57 , 426–445. [ Google Scholar ] [ CrossRef ]
- Cambardella, C.A.; Elliott, E.T. Particulate soil organic-matter changes across a grassland cultivation sequence. Soil Sci. Soc. Am. J. 1992 , 56 , 777–783. [ Google Scholar ] [ CrossRef ]
- Kalbitz, K.; Solinger, S.; Park, J.H.; Michalzik, B.; Matzner, E. Controls on the dynamics of dissolved organic matter in soils: A review. Soil Sci. 2000 , 165 , 277–304. [ Google Scholar ] [ CrossRef ]
- Kogel-Knabner, I. The macromolecular organic composition of plant and microbial residues as inputs to soil organic matter. Soil Biol. Biochem. 2002 , 34 , 139–162. [ Google Scholar ] [ CrossRef ]
- Kuzyakov, Y. Priming effects: Interactions between living and dead organic matter. Soil Biol. Biochem. 2010 , 42 , 1363–1371. [ Google Scholar ] [ CrossRef ]
- Six, J.; Elliott, E.T.; Paustian, K.; Doran, J.W. Aggregation and soil organic matter accumulation in cultivated and native grassland soils. Soil Sci. Soc. Am. J. 1998 , 62 , 1367–1377. [ Google Scholar ] [ CrossRef ]
- Fontaine, S.; Mariotti, A.; Abbadie, L. The priming effect of organic matter: A question of microbial competition? Soil Biol. Biochem. 2003 , 35 , 837–843. [ Google Scholar ] [ CrossRef ]
- Sollins, P.; Homann, P.; Caldwell, B.A. Stabilization and destabilization of soil organic matter: Mechanisms and controls. Geoderma 1996 , 74 , 65–105. [ Google Scholar ] [ CrossRef ]
- Bronick, C.J.; Lal, R. Soil structure and management: A review. Geoderma 2005 , 124 , 3–22. [ Google Scholar ] [ CrossRef ]
- Hamza, M.A.; Anderson, W.K. Soil compaction in cropping systems—A review of the nature, causes and possible solutions. Soil Tillage Res. 2005 , 82 , 121–145. [ Google Scholar ] [ CrossRef ]
- Cambardella, C.A.; Moorman, T.B.; Novak, J.M.; Parkin, T.B.; Karlen, D.L.; Turco, R.F.; Konopka, A.E. Field-scale variability of soil properties in central iowa soils. Soil Sci. Soc. Am. J. 1994 , 58 , 1501–1511. [ Google Scholar ] [ CrossRef ]
- Glaser, B.; Lehmann, J.; Zech, W. Ameliorating physical and chemical properties of highly weathered soils in the tropics with charcoal—A review. Biol. Fertil. Soils 2002 , 35 , 219–230. [ Google Scholar ] [ CrossRef ]
- Saxton, K.E.; Rawls, W.J. Soil water characteristic estimates by texture and organic matter for hydrologic solutions. Soil Sci. Soc. Am. J. 2006 , 70 , 1569–1578. [ Google Scholar ] [ CrossRef ]
- Rossel, R.A.V.; Walvoort, D.J.J.; McBratney, A.B.; Janik, L.J.; Skjemstad, J.O. Visible, near infrared, mid infrared or combined diffuse reflectance spectroscopy for simultaneous assessment of various soil properties. Geoderma 2006 , 131 , 59–75. [ Google Scholar ] [ CrossRef ]
- Liang, B.; Lehmann, J.; Solomon, D.; Kinyangi, J.; Grossman, J.; O’Neill, B.; Skjemstad, J.O.; Thies, J.; Luizao, F.J.; Petersen, J.; et al. Black Carbon increases cation exchange capacity in soils. Soil Sci. Soc. Am. J. 2006 , 70 , 1719–1730. [ Google Scholar ] [ CrossRef ]
- Chang, C.W.; Laird, D.A.; Mausbach, M.J.; Hurburgh, C.R. Near-infrared reflectance spectroscopy-principal components regression analyses of soil properties. Soil Sci. Soc. Am. J. 2001 , 65 , 480–490. [ Google Scholar ] [ CrossRef ]
- Lehmann, J.; da Silva, J.P.; Steiner, C.; Nehls, T.; Zech, W.; Glaser, B. Nutrient availability and leaching in an archaeological Anthrosol and a Ferralsol of the Central Amazon basin: Fertilizer, manure and charcoal amendments. Plant Soil 2003 , 249 , 343–357. [ Google Scholar ] [ CrossRef ]
- Vonuexkull, H.R.; Mutert, E. Global extent, development and economic impact of acid soils. Plant Soil 1995 , 171 , 1–15. [ Google Scholar ] [ CrossRef ]
- Batjes, N.H. Total carbon and nitrogen in the soils of the world. Eur. J. Soil Sci. 2014 , 65 , 10–21. [ Google Scholar ] [ CrossRef ]
- Kirschbaum, M.U.F. The temperature dependence of soil organic matter decomposition, and the effect of global warming on soil organic C storage. Soil Biol. Biochem. 1995 , 27 , 753–760. [ Google Scholar ] [ CrossRef ]
- Wrage, N.; Velthof, G.L.; van Beusichem, M.L.; Oenema, O. Role of nitrifier denitrification in the production of nitrous oxide. Soil Biol. Biochem. 2001 , 33 , 1723–1732. [ Google Scholar ] [ CrossRef ]
- Lal, R. Soil carbon sequestration to mitigate climate change. Geoderma 2004 , 123 , 1–22. [ Google Scholar ] [ CrossRef ]
- Six, J.; Elliott, E.T.; Paustian, K. Soil macroaggregate turnover and microaggregate formation: A mechanism for C sequestration under no-tillage agriculture. Soil Biol. Biochem. 2000 , 32 , 2099–2103. [ Google Scholar ] [ CrossRef ]
- West, T.O.; Post, W.M. Soil organic carbon sequestration rates by tillage and crop rotation: A global data analysis. Soil Sci. Soc. Am. J. 2002 , 66 , 1930–1946. [ Google Scholar ] [ CrossRef ]
- Six, J.; Frey, S.D.; Thiet, R.K.; Batten, K.M. Bacterial and fungal contributions to carbon sequestration in agroecosystems. Soil Sci. Soc. Am. J. 2006 , 70 , 555–569. [ Google Scholar ] [ CrossRef ]
- Rasse, D.P.; Rumpel, C.; Dignac, M.F. Is soil carbon mostly root carbon? Mechanisms for a specific stabilisation. Plant Soil 2005 , 269 , 341–356. [ Google Scholar ] [ CrossRef ]
- McBratney, A.B.; Santos, M.L.M.; Minasny, B. On digital soil mapping. Geoderma 2003 , 117 , 3–52. [ Google Scholar ] [ CrossRef ]
- Frostegard, A.; Baath, E. The use of phospholipid fatty acid analysis to estimate bacterial and fungal biomass in soil. Biol. Fertil. Soils 1996 , 22 , 59–65. [ Google Scholar ] [ CrossRef ]
- Zelles, L. Fatty acid patterns of phospholipids and lipopolysaccharides in the characterisation of microbial communities in soil: A review. Biol. Fertil. Soils 1999 , 29 , 111–129. [ Google Scholar ] [ CrossRef ]
- Giller, K.E.; Witter, E.; McGrath, S.P. Toxicity of heavy metals to microorganisms and microbial processes in agricultural soils: A review. Soil Biol. Biochem. 1998 , 30 , 1389–1414. [ Google Scholar ] [ CrossRef ]
- Nannipieri, P.; Ascher, J.; Ceccherini, M.T.; Landi, L.; Pietramellara, G.; Renella, G. Microbial diversity and soil functions. Eur. J. Soil Sci. 2003 , 54 , 655–670. [ Google Scholar ] [ CrossRef ]
- Fierer, N.; Schimel, J.P.; Holden, P.A. Variations in microbial community composition through two soil depth profiles. Soil Biol. Biochem. 2003 , 35 , 167–176. [ Google Scholar ] [ CrossRef ]
- Lauber, C.L.; Strickland, M.S.; Bradford, M.A.; Fierer, N. The influence of soil properties on the structure of bacterial and fungal communities across land-use types. Soil Biol. Biochem. 2008 , 40 , 2407–2415. [ Google Scholar ] [ CrossRef ]
- Compant, S.; Clement, C.; Sessitsch, A. Plant growth-promoting bacteria in the rhizo- and endosphere of plants: Their role, colonization, mechanisms involved and prospects for utilization. Soil Biol. Biochem. 2010 , 42 , 669–678. [ Google Scholar ] [ CrossRef ]
- Frostegard, A.; Baath, E.; Tunlid, A. Shifts in the structure of soil microbial communities in limed forests as revealed by phospholipid fatty-acid analysis. Soil Biol. Biochem. 1993 , 25 , 723–730. [ Google Scholar ] [ CrossRef ]
- Burns, R.G.; DeForest, J.L.; Marxsen, J.; Sinsabaugh, R.L.; Stromberger, M.E.; Wallenstein, M.D.; Weintraub, M.N.; Zoppini, A. Soil enzymes in a changing environment: Current knowledge and future directions. Soil Biol. Biochem. 2013 , 58 , 216–234. [ Google Scholar ] [ CrossRef ]
- Saiya-Cork, K.R.; Sinsabaugh, R.L.; Zak, D.R. The effects of long term nitrogen deposition on extracellular enzyme activity in an Acer saccharum forest soil. Soil Biol. Biochem. 2002 , 34 , 1309–1315. [ Google Scholar ] [ CrossRef ]
- Jones, D.L. Organic acids in the rhizosphere—A critical review. Plant Soil 1998 , 205 , 25–44. [ Google Scholar ] [ CrossRef ]
- Vessey, J.K. Plant growth promoting rhizobacteria as biofertilizers. Plant Soil 2003 , 255 , 571–586. [ Google Scholar ] [ CrossRef ]
- Hinsinger, P. Bioavailability of soil inorganic P in the rhizosphere as affected by root-induced chemical changes: A review. Plant Soil 2001 , 237 , 173–195. [ Google Scholar ] [ CrossRef ]
- Atkinson, C.J.; Fitzgerald, J.D.; Hipps, N.A. Potential mechanisms for achieving agricultural benefits from biochar application to temperate soils: A review. Plant Soil 2010 , 337 , 1–18. [ Google Scholar ] [ CrossRef ]
- Van Zwieten, L.; Kimber, S.; Morris, S.; Chan, K.Y.; Downie, A.; Rust, J.; Joseph, S.; Cowie, A. Effects of biochar from slow pyrolysis of papermill waste on agronomic performance and soil fertility. Plant Soil 2010 , 327 , 235–246. [ Google Scholar ] [ CrossRef ]
- Chan, K.Y.; Van Zwieten, L.; Meszaros, I.; Downie, A.; Joseph, S. Agronomic values of greenwaste biochar as a soil amendment. Aust. J. Soil Res. 2007 , 45 , 629–634. [ Google Scholar ] [ CrossRef ]
- Le Mer, J.; Roger, P. Production, oxidation, emission and consumption of methane by soils: A review. Eur. J. Soil Biol. 2001 , 37 , 25–50. [ Google Scholar ] [ CrossRef ]
- Cakmak, I. Enrichment of cereal grains with zinc: Agronomic or genetic biofortification? Plant Soil 2008 , 302 , 1–17. [ Google Scholar ] [ CrossRef ]
- Bossio, D.A.; Cook-Patton, S.C.; Ellis, P.W.; Fargione, J.; Sanderman, J.; Smith, P.; Wood, S.; Zomer, R.J.; von Unger, M.; Emmer, I.M.; et al. The role of soil carbon in natural climate solutions. Nat. Sustain. 2020 , 3 , 391–398. [ Google Scholar ] [ CrossRef ]
- Westoby, M. A leaf-height-seed (LHS) plant ecology strategy scheme. Plant Soil 1998 , 199 , 213–227. [ Google Scholar ] [ CrossRef ]
- Mingers, J.; Yang, L. Evaluating journal quality: A review of journal citation indicators and ranking in business and management. Eur. J. Oper. Res. 2017 , 257 , 323–337. [ Google Scholar ] [ CrossRef ]
- Mingers, J.; Leydesdorff, L. A review of theory and practice in scientometrics. Eur. J. Oper. Res. 2015 , 246 , 1–19. [ Google Scholar ] [ CrossRef ]
- Teixeira da Silva, J.A.; Memon, A.R. CiteScore: A cite for sore eyes, or a valuable, transparent metric? Scientometrics 2017 , 111 , 553–556. [ Google Scholar ] [ CrossRef ]
- Engqvist, L.; Frommen, J.G. The h -index and self-citations. Trends Ecol. Evol. 2008 , 23 , 250–252. [ Google Scholar ] [ CrossRef ]
- Falagas, M.E.; Kouranos, V.D.; Arencibia-Jorge, R.; Karageorgopoulos, D.E. Comparison of SCImago journal rank indicator with journal impact factor. FASEB J. 2008 , 22 , 2623–2628. [ Google Scholar ] [ CrossRef ]
- Leydesdorff, L.; Opthof, T. Scopus’s source normalized impact per paper (SNIP) versus a journal impact factor based on fractional counting of citations. J. Am. Soc. Inf. Sci. Technol. 2010 , 61 , 2365–2369. [ Google Scholar ] [ CrossRef ]
- Fersht, A. The most influential journals: Impact Factor and Eigenfactor. Proc. Natl. Acad. Sci. USA 2009 , 106 , 6883–6884. [ Google Scholar ] [ CrossRef ]
- Brown, M. Is Almetrics an Acceptable Replacement for Citation Counts and the Impact Factor? Ser. Libr. 2014 , 67 , 27–30. [ Google Scholar ] [ CrossRef ]
- Gagliardi, A.R.; Chen, R.H.C.; Boury, H.; Albert, M.; Chow, J.; DaCosta, R.S.; Hoffman, M.; Keshavarz, B.; Kontos, P.; Liu, J.; et al. DORA-compliant measures of research quality and impact to assess the performance of researchers in biomedical institutions: Review of published research, international best practice and Delphi survey. PLoS ONE 2023 , 18 , e0270616. [ Google Scholar ] [ CrossRef ]
- Boufarss, M.; Laakso, M. Open Sesame? Open access priorities, incentives, and policies among higher education institutions in the United Arab Emirates. Scientometrics 2020 , 124 , 1553–1577. [ Google Scholar ] [ CrossRef ]
- Grunwald, S.; Daroub, S. A 360° perspective of women in soil science focused on the U.S. Front. Soil Sci. 2023 , 3 , 1072758. [ Google Scholar ] [ CrossRef ]
- Dawson, L.; Brevik, E.C.; Reyes-Sánchez, L.B. International gender equity in soil science. Eur. J. Soil Sci. 2021 , 72 , 1929–1939. [ Google Scholar ] [ CrossRef ]
- Carter, T.L.; Jennings, L.L.; Pressler, Y.; Gallo, A.C.; Berhe, A.A.; Marín-Spiotta, E.; Shepard, C.; Ghezzehei, T.; Vaughan, K.L. Towards diverse representation and inclusion in soil science in the United States. Soil Sci. Soc. Am. J. 2021 , 85 , 963–974. [ Google Scholar ] [ CrossRef ]
- Berhe, A.A.; Ghezzehei, T.A. Race and racism in soil science. Eur. J. Soil Sci. 2021 , 72 , 1292–1297. [ Google Scholar ] [ CrossRef ]
- Kurt, S. Why do authors publish in predatory journals? Learn. Publ. 2018 , 31 , 141–147. [ Google Scholar ] [ CrossRef ]
- Klebel, T.; Ross-Hellauer, T. The APC-barrier and its effect on stratification in open access publishing. Quant. Sci. Stud. 2023 , 4 , 22–43. [ Google Scholar ] [ CrossRef ]
- Maqsood, A.; Akbar Sial, T.; Iqbal, Q. Researchers’ Accessibility to the Library Resources: A Comparative Study of Developed and Developing Countries. J. Inf. Manag. Pract. JIMP 2023 , 3 , 39–58. [ Google Scholar ] [ CrossRef ]
- Ali, P.A.; Watson, R. Peer review and the publication process. Nurs. Open 2016 , 3 , 193–202. [ Google Scholar ] [ CrossRef ] [ PubMed ]
- Resnik, D.B.; Elmore, S.A. Ensuring the Quality, Fairness, and Integrity of Journal Peer Review: A Possible Role of Editors. Sci. Eng. Ethics 2016 , 22 , 169–188. [ Google Scholar ] [ CrossRef ] [ PubMed ]
- Golden, M.; Schultz, D.M. Quantifying the Volunteer Effort of Scientific Peer Reviewing. Bull. Am. Meteorol. Soc. 2012 , 93 , 337–345. [ Google Scholar ] [ CrossRef ]
- Wolbring, G.; Nguyen, A. Equity/Equality, Diversity and Inclusion, and Other EDI Phrases and EDI Policy Frameworks: A Scoping Review. Trends High. Educ. 2023 , 2 , 168–237. [ Google Scholar ] [ CrossRef ]
- Ceci, S.J.; Williams, W.M. Understanding current causes of women’s underrepresentation in science. Proc. Natl. Acad. Sci. USA 2011 , 108 , 3157–3162. [ Google Scholar ] [ CrossRef ]
- Vaughan, K.; Van Miegroet, H.; Pennino, A.; Pressler, Y.; Duball, C.; Brevik, E.C.; Berhe, A.A.; Olson, C. Women in Soil Science: Growing Participation, Emerging Gaps, and the Opportunities for Advancement in the USA. Soil Sci. Soc. Am. J. 2019 , 83 , 1278–1289. [ Google Scholar ] [ CrossRef ]
- Keesstra, S.D.; Bouma, J.; Wallinga, J.; Tittonell, P.; Smith, P.; Cerdà, A.; Montanarella, L.; Quinton, J.N.; Pachepsky, Y.; van der Putten, W.H.; et al. The significance of soils and soil science towards realization of the United Nations Sustainable Development Goals. SOIL 2016 , 2 , 111–128. [ Google Scholar ] [ CrossRef ]
- Cioffi, R.; Travaglioni, M.; Piscitelli, G.; Petrillo, A.; De Felice, F. Artificial Intelligence and Machine Learning Applications in Smart Production: Progress, Trends, and Directions. Sustainability 2020 , 12 , 492. [ Google Scholar ] [ CrossRef ]
- Liakos, K.G.; Busato, P.; Moshou, D.; Pearson, S.; Bochtis, D. Machine Learning in Agriculture: A Review. Sensors 2018 , 18 , 2674. [ Google Scholar ] [ CrossRef ]
- Nabiollahi, K.; Taghizadeh-Mehrjardi, R.; Shahabi, A.; Heung, B.; Amirian-Chakan, A.; Davari, M.; Scholten, T. Assessing agricultural salt-affected land using digital soil mapping and hybridized random forests. Geoderma 2021 , 385 , 114858. [ Google Scholar ] [ CrossRef ]
- Fathizad, H.; Ardakani, M.A.H.; Heung, B.; Sodaiezadeh, H.; Rahmani, A.; Fathabadi, A.; Scholten, T.; Taghizadeh-Mehrjardi, R. Spatio-temporal dynamic of soil quality in the central Iranian desert modeled with machine learning and digital soil assessment techniques. Ecol. Indic. 2020 , 118 , 106736. [ Google Scholar ] [ CrossRef ]
- Padarian, J.; Minasny, B.; McBratney, A.B. Machine learning and soil sciences: A review aided by machine learning tools. SOIL 2020 , 6 , 35–52. [ Google Scholar ] [ CrossRef ]
- Strobl, C.; Ailhaud, E.; Benetos, K.; Devitt, A.; Kruse, O.; Proske, A.; Rapp, C. Digital support for academic writing: A review of technologies and pedagogies. Comput. Educ. 2019 , 131 , 33–48. [ Google Scholar ] [ CrossRef ]
- Dong, Y.; Yu, X.; Alharbi, A.; Ahmad, S. AI-based production and application of English multimode online reading using multi-criteria decision support system. Soft Comput. 2022 , 26 , 10927–10937. [ Google Scholar ] [ CrossRef ]
- Teubner, T.; Flath, C.M.; Weinhardt, C.; van der Aalst, W.; Hinz, O. Welcome to the Era of ChatGPT et al. Bus. Inf. Syst. Eng. 2023 , 65 , 95–101. [ Google Scholar ] [ CrossRef ]
- Editorials, N. Tools such as ChatGPT threaten transparent science; here are our ground rules for their use. Nature 2023 , 613 , 10–38. [ Google Scholar ]
Click here to enlarge figure
JCR Quartile | Journal Name (Full and Abbrev.) | Region | Inaugural Issue Year | Process Duration (Weeks) | Acceptance Rate | Publisher | Model | Cost per Open-Access Paper (USD) | Website | |||
---|---|---|---|---|---|---|---|---|---|---|---|---|
First Decision | Review Time | Publication Time | ||||||||||
Q1 | Soil Biology and Biochemistry | SBB | England | 1969 | First decision: 4.6 wk (week); Review time: 6.9 wk; Publication time: 0.9 wk | N/A | Elsevier (Amsterdam, The Netherlands) | Hybrid | USD 4520 + taxes | |||
Geoderma | GeoD | The Netherlands | 1967 | First decision: 5.4 wk; Review time: 8.3 wk; Publication time: 1.8 wk | 16% | Elsevier (Amsterdam, The Netherlands) | Open access (since 2023) | USD 2800 + taxes | ||||
Catena | Catena | Germany | 1973 | First decision: 4.6 wk; Review time: 7.7 wk; Publication time: 1.8 wk | N/A | Elsevier (Amsterdam, The Netherlands) | Hybrid | USD 3580 + taxes | ||||
Soil and Tillage Research | STR | The Netherlands | 1980 | First decision: 7 wk; Review time: 10.1 wk; Publication time: 1.7 wk | N/A | Elsevier (Amsterdam, The Netherlands) | Hybrid | USD 3970 + taxes | ||||
Biology and Fertility of Soils | BFS | Germany | 1985 | Submission to first decision (median): 0.7 wk | N/A | Springer (Berlin, Germany) | Hybrid | USD 4390 + taxes | ||||
Pedosphere | PedoS | China mainland | 1991 | Publication time: 0.3 wk | N/A | Elsevier (Amsterdam, The Netherlands)/Science Press (Beijing, China) | Subscription | N/A | ||||
Soil | Soil | Germany | 2015 | Submission to initial decision: 0.3 wk Public discussion: 8.1 wk; Author’s revision: 13.6 wk Re-evaluation: 19.4 wk; Acceptance to publication: 23.4 wk | N/A | Copernicus (Göttingen, Germany) | Open access (since 2015) | Price per journal page: EUR 77/93 net | ||||
International Soil and Water Conservation Research | ISWCR | China Mainland | 2013 | Time to first decision: 4.1 weeks; Review time: 7 wk; Publication time: 2.6 wk | N/A | KeAi Publishing (Beijing, China) | Open access (since 2013) | USD 1200 + taxes | ||||
Biochar | BioC | China mainland | 2019 | Submission to first decision (median): 2.1 wk | N/A | Springer (Berlin, Germany) | Open access (since 2022) | USD 2490 + taxes | ||||
Q2 | Plant and Soil | P & S | The Netherlands | 1948 | Submission to first decision (median): 5 wk | N/A | Springer (Berlin, Germany) | Hybrid | USD 4390 + taxes | |||
Applied Soil Ecology | ASE | The Netherlands | 1994 | First decision: 5.8 wk; Review time: 9.2 wk; Publication time: 1.6 wk | N/A | Elsevier (Amsterdam, The Netherlands) | Hybrid | USD 3830 + taxes | ||||
Journal of Soils and Sediments | JSS | Germany | 2001 | Submission to first decision (median): 1.3 wk | N/A | Springer (Berlin, Germany) | Hybrid | USD 3690 + taxes | ||||
Land Degradation & Development | LDD | England | 1989 | N/A | N/A | Wiley (Hoboken, NJ, USA) | Hybrid | USD 4740 + taxes | ||||
European Journal of Soil Science (was Journal for Soil Science) | EJSS | England | 1949 | First decision (median): 3.6 wk; Review duration (median): 9.3 wk; Publication time (median): 2.0 wk | N/A | Wiley (Hoboken, NJ, USA) | Hybrid | USD 4400 + taxes | ||||
Nutrient Cycling in Agroecosystems (was Fertilizer research) | NCA | The Netherlands | 1980 | Submission to first decision (median): 1.4 wk | N/A | Springer (Berlin, Germany) | Hybrid | USD 3490 + taxes | ||||
Soil Use and Management | SUM | England | 1985 | N/A | N/A | Wiley (Hoboken, NJ, USA) | Hybrid | USD 3850 + taxes | ||||
Journal of Soil Science and Plant Nutrition | JSSPN | Chile | 2001 | Submission to first decision (median): 1.9 wk | N/A | Springer (Berlin, Germany) | Hybrid | USD 2990 + taxes | ||||
Rhizosphere | Rhiz | The Netherlands | 2016 | First decision: 2.7 wk; Review time: 4.2 wk; Publication time: 1.1 wk | N/A | Elsevier (Amsterdam, The Netherlands) | Hybrid | USD 2880 + taxes | ||||
Geoderma Regional | GR | The Netherlands | 2014 | First decision: 8.4 wk; Review time: 10.9 wk; Publication time: 0.7 wk | N/A | Elsevier (Amsterdam, The Netherlands) | Hybrid | USD 2640 + taxes | ||||
Q3 | Soil Science Society of America Journal | SSSAJ | USA | 1921 | Submission to first decision: 7 wk | 35% | Wiley (Hoboken, NJ, USA) | Hybrid | USD 1350 + taxes | |||
Journal of Plant Nutrition and Soil Science (was Zeitschrift fur Pflanzenernahrung und Bodenkunde) | JPNSS | Germany | 1922 | N/A | N/A | Wiley (Hoboken, NJ, USA) | Hybrid | USD 3660 + taxes | ||||
Vadose Zone Journal | VZJ | USA | 2002 | Submission to first decision (median): 7.6 wk; Final decision: 10.9 wk; Acceptance to publication (median): <5.7 wk | 65% | Wiley (Hoboken, NJ, USA) | Open access (since 2018) | USD 2450 + taxes | ||||
Journal of Soil and Water Conservation | JSWC | USA | 1946 | Initial manuscript review decision within 10 wk; Accepted manuscripts are typically published within 26.1 to 39.1 wk from the date final files are submitted | N/A | Soil and Water Conservation Society (Ankeny, IA, USA) | Hybrid | Additional USD 750 | ||||
Clays and Clay Minerals | CCM | USA | 1952 | Submission to first decision (median): 4.3 wk | N/A | Springer (Berlin, Germany) | Hybrid | USD 2890 + taxes | ||||
Archives of Agronomy and Soil Science | AASS | England | 1956 | 0 wk avg. from submission to first decision; 12.3 wk avg. from submission to first post-review decision; 1.4 wk avg. from acceptance to online publication | 13% | Taylor & Francis (Oxford, UK) | Open access (since 2022) | USD 600–USD 4800 Use open access cost finder to view | ||||
European Journal of Soil Biology | EJSB | France | 1964 | Time to first decision: 2.2 wk; Review time: 3.8 wk; Publication time: 1.6 wk | N/A | Elsevier (Amsterdam, The Netherlands) | Hybrid | USD 3140 + taxes | ||||
Pedobiologia | PedoB | Germany | 1961 | Review time: 9.2 wk; Publication time: 0.6 wk | N/A | Elsevier (Amsterdam, The Netherlands) | Hybrid | USD 2780 + taxes | ||||
Arid Land Research and Management (was Arid Soil Research and Rehabilitation) | ALRM | USA | 1987 | 1 wk avg. from submission to first decision; 11 wk avg. from submission to first post-review decision; 2.7 wk avg. from acceptance to online publication | 17% | Taylor & Francis (Oxford, UK) | Open access | USD 600–USD 4800 Use open access cost finder to view | ||||
Soil and Water Research | SWR | Czech Republic | 2006 | N/A | N/A | Czech Academy of Agricultural Sciences (Prague, Czechia) | Open access (since 2006) | 565 EUR/article or 14,000 CZK | ||||
Q4 | Communications in Soil Science and Plant Analysis | CSSPA | USA | 1970 | 10 wk avg. from submission to first decision; 20.1 wk avg. from submission to first post-review decision; 1.3 wk avg. from acceptance to online publication | 25% | Taylor & Francis (Oxford, UK) | Open access | USD 600–USD 4800 Use open access cost finder to view | |||
Eurasian Soil Science | ESS | Russia | 1899 | N/A | N/A | Pleiades Publishing (New York, NY, USA) | Hybrid | Contact the Open Research Support Team: | ||||
Soil Science and Plant Nutrition | SSPN | Japan | 1955 | 5.6 wk avg. from submission to first decision; 7.7 wk avg. from submission to first post-review decision; 1.3 wk avg. from acceptance to online publication | 27% | Taylor & Francis (Oxford, UK) | Open access | USD 600–USD 4800 Use open access cost finder to view | ||||
Revista Brasileira De Ciencia Do Solo | RBCS | Brazil | 1977 | N/A | N/A | Sociedade Brasileira de Ciencia do Solo (Viçosa, Brazil) | Open access (since 2003) | USD 70/100 per page | ||||
Soil Research (was Australian Journal of Soil Research) | SR | Australia | 1963 | 5.6 wk from manuscript submission to first decision; 8.4 wk from manuscript acceptance to publication | 28% | CSIRO publishing (Clayton, Australia) | Hybrid | USD 2700 + taxes | ||||
Soil Science | SS | USA | 1916 | N/A | N/A | Lippincott Williams & Wilkins (Philadelphia, PA, USA) | Subscription | N/A | ||||
Canadian Journal of Soil Science | CJSS | Canada | 1921 | 6.4 wk avg. from submission to first decision | 47% | Canadian Science Publishing (Ottawa, ON, Canada) | Hybrid | USD 1000 + taxes | ||||
Acta Agriculturae Scandinavica Section B-Soil and Plant Science | AASSB | Norway | 1950 | 0.1 wk avg. from submission to first decision; 5.7 wk avg. from submission to first post-review decision; 3 wk avg. from acceptance to online publication | 14% | Taylor & Francis (Oxford, UK) | Open access (since 2022) | USD 2070 + taxes | ||||
Agrochimica | AgroC | Italy | 1956 | Reviewed within 8.7 wk from the submission date; Published within 13 wk from the final acceptance | N/A | Pisa University Press (Pisa, Italy) | Subscription | N/A | ||||
Compost Science & Utilization | CSU | USA | 1993 | 28.9 wk avg. from submission to first decision; 55.1 wk avg. from submission to first post-review decision | 7% | Taylor & Francis (Oxford, UK) | Open access | USD 600–USD 4800 Use open access cost finder to view |
JCR Quartile | NO. | Journal Name | Abbrev. | N | TC | LC | TC/N | LC/N | Journal H-Index |
---|---|---|---|---|---|---|---|---|---|
Q1 | 1 | Soil Biology and Biochemistry | SBB | 8627 | 504,821 | 219,855 | 58.52 | 25.48 | 241 |
2 | Geoderma | GeoD | 7712 | 303,420 | 112,315 | 39.34 | 14.56 | 183 | |
3 | Catena | Catena | 6144 | 175,432 | 42,026 | 28.55 | 6.84 | 142 | |
4 | Soil and Tillage Research | STR | 4454 | 176,957 | 74,552 | 39.73 | 16.74 | 151 | |
5 | Biology and Fertility of Soils | BFS | 3394 | 137,836 | 58,816 | 40.61 | 17.33 | 137 | |
6 | Pedosphere | PedoS | 1673 | 36,985 | 8703 | 22.11 | 5.20 | 72 | |
7 | Soil | Soil | 297 | 6970 | 2163 | 23.47 | 7.28 | 39 | |
8 | International Soil and Water Conservation Research | ISWCR | 268 | 4282 | 1139 | 15.98 | 4.25 | 34 | |
9 | Biochar | BioC | 187 | 3133 | 479 | 16.75 | 2.56 | 25 | |
Q2 | 1 | Plant and Soil | P & S | 10,963 | 452,766 | 131,225 | 41.30 | 11.97 | 206 |
2 | Applied Soil Ecology | ASE | 4251 | 131,221 | 36,256 | 30.87 | 8.53 | 129 | |
3 | Journal of Soils and Sediments | JSS | 3383 | 58,352 | 12,826 | 17.25 | 3.79 | 80 | |
4 | Land Degradation & Development | LDD | 2947 | 65,258 | 16,867 | 22.14 | 5.72 | 93 | |
5 | European Journal of Soil Science | EJSS | 2515 | 103,670 | 37,821 | 41.22 | 15.04 | 125 | |
6 | Nutrient Cycling in Agroecosystems | NCA | 2465 | 74,701 | 17,128 | 30.30 | 6.95 | 104 | |
7 | Soil Use and Management | SUM | 1778 | 45,608 | 15,385 | 25.65 | 8.65 | 84 | |
8 | Journal of Soil Science and Plant Nutrition | JSSPN | 1695 | 18,891 | 4441 | 11.15 | 2.62 | 49 | |
9 | Rhizosphere | Rhiz | 556 | 3637 | 769 | 6.54 | 1.38 | 23 | |
10 | Geoderma Regional | GR | 476 | 3775 | 1762 | 7.93 | 3.70 | 25 | |
Q3 | 1 | Soil Science Society of America Journal | SSSAJ | 6595 | 295,425 | 186,463 | 44.80 | 28.27 | 191 |
2 | Journal of Plant Nutrition and Soil Science | JPNSS | 2771 | 65,776 | 17,043 | 23.74 | 6.15 | 90 | |
3 | Vadose Zone Journal | VZJ | 2081 | 55,573 | 12,634 | 26.70 | 6.07 | 87 | |
4 | Journal of Soil and Water Conservation | JSWC | 2067 | 43,118 | 14,364 | 20.86 | 6.95 | 87 | |
5 | Clays and Clay Minerals | CCM | 1927 | 61,800 | 15,646 | 32.07 | 8.12 | 101 | |
6 | Archives of Agronomy and Soil Science | AASS | 1760 | 16,569 | 4233 | 9.41 | 2.41 | 43 | |
7 | European Journal of Soil Biology | EJSB | 1553 | 40,950 | 10,729 | 26.37 | 6.91 | 77 | |
8 | Pedobiologia | PedoB | 1524 | 36,282 | 16,485 | 23.81 | 10.82 | 75 | |
9 | Arid Land Research and Management | ALRM | 926 | 11,341 | 1722 | 12.25 | 1.86 | 33 | |
10 | Soil and Water Research | SWR | 339 | 2976 | 682 | 8.78 | 2.01 | 24 | |
Q4 | 1 | Communications in Soil Science and Plant Analysis | CSSPA | 7086 | 74,665 | 32,437 | 10.54 | 4.58 | 83 |
2 | Eurasian Soil Science | ESS | 5061 | 22,165 | 11,257 | 4.38 | 2.22 | 36 | |
3 | Soil Science and Plant Nutrition | SSPN | 2783 | 43,129 | 16,169 | 15.50 | 5.81 | 68 | |
4 | Revista Brasileira De Ciencia Do Solo | RBCS | 2662 | 35,306 | 17,643 | 13.26 | 6.63 | 56 | |
5 | Soil Research | SR | 2507 | 64,335 | 35,807 | 25.66 | 14.28 | 41 | |
6 | Soil Science | SS | 2073 | 57,274 | 54,539 | 27.63 | 26.31 | 97 | |
7 | Canadian Journal of Soil Science | CJSS | 1903 | 41,127 | 27,528 | 21.61 | 14.47 | 77 | |
8 | Acta Agriculturae Scandinavica Section B-Soil and Plant Science | AASSB | 1801 | 17,167 | 2842 | 9.53 | 1.58 | 43 | |
9 | Agrochimica | AgroC | 920 | 3304 | 1417 | 3.59 | 1.54 | 20 | |
10 | Compost Science & Utilization | CSU | 787 | 13,391 | 2930 | 17.02 | 3.72 | 52 | |
Sum | 112,911 | 3,309,388 | 1,277,098 | 29.31 | 11.31 | / |
Publication | Journal | DOI | TC | TC/Y | LC | LC/Y |
---|---|---|---|---|---|---|
Lehmann et al. (2011) [ ] | SBB | 10.1016/j.soilbio.2011.04.022 | 2765 | 230.42 | 655 | 54.58 |
Six et al. (2002) [ ] | P & S | 10.1023/A:1016125726789 | 2590 | 123.33 | 1191 | 56.71 |
Bronick and Lal (2005) [ ] | GeoD | 10.1016/j.geoderma.2004.03.005 | 2354 | 130.78 | 1078 | 59.89 |
Batjes (2014) [ ] | EJSS | 10.1111/ejss.12114_2 | 2290 | 254.44 | 1 | 0.11 |
Six et al. (2004) [ ] | STR | 10.1016/j.still.2004.03.008 | 2287 | 120.37 | 1198 | 63.05 |
Cambardella et al. (1994) [ ] | SSSAJ | 10.2136/sssaj1994.03615995005800050033x | 2160 | 74.48 | 607 | 20.93 |
McBratney et al. (2003) [ ] | GeoD | 10.1016/S0016-7061(03)00223-4 | 1964 | 98.20 | 879 | 43.95 |
Lal [ ] | GeoD | 10.1016/j.geoderma.2004.01.032 | 1925 | 101.32 | 601 | 31.63 |
Six et al. (2000) [ ] | SBB | 10.1016/S0038-0717(00)00179-6 | 1864 | 81.04 | 1006 | 43.74 |
Kuzyakov et al. (2000) [ ] | SBB | 10.1016/S0038-0717(00)00084-5 | 1843 | 80.13 | 823 | 35.78 |
Frostegard and Baath (1996) [ ] | BFS | 10.1007/BF00384433 | 1834 | 67.93 | 819 | 30.33 |
von Lutzow et al. (2006) [ ] | EJSS | 10.1111/j.1365-2389.2006.00809.x | 1781 | 104.76 | 787 | 46.29 |
Jones (1998) [ ] | P & S | 10.1023/A:1004356007312 | 1757 | 70.28 | 484 | 19.36 |
Cambardella and Elliott (1992) [ ] | SSSAJ | 10.2136/sssaj1992.03615995005600030017x | 1689 | 54.48 | 955 | 30.81 |
Glaser et al. (2002) [ ] | BFS | 10.1007/s00374-002-0466-4 | 1673 | 79.67 | 446 | 21.24 |
Kalbitz et al. (2000) [ ] | SS | 10.1097/00010694-200004000-00001 | 1644 | 71.48 | 506 | 22.00 |
Vessey (2003) [ ] | P & S | 10.1023/A:1026037216893 | 1569 | 78.45 | 184 | 9.20 |
Hinsinger (2001) [ ] | P & S | 10.1023/A:1013351617532 | 1511 | 68.68 | 491 | 22.32 |
West and Post (2002) [ ] | SSSAJ | 10.2136/sssaj2002.1930 | 1495 | 71.19 | 553 | 26.33 |
Zelles (1999) [ ] | BFS | 10.1007/s003740050533 | 1489 | 62.04 | 633 | 26.38 |
Saxton and Rawls (2006) [ ] | SSSAJ | 10.2136/sssaj2005.0117 | 1441 | 84.76 | 191 | 11.24 |
Kirschbaum (1995) [ ] | SBB | 10.1016/0038-0717(94)00242-S | 1407 | 50.25 | 385 | 13.75 |
Giller et al. (1998) [ ] | SBB | 10.1016/S0038-0717(97)00270-8 | 1386 | 55.44 | 262 | 10.48 |
Atkinson et al. (2010) [ ] | P & S | 10.1007/s11104-010-0464-5 | 1333 | 102.54 | 334 | 25.69 |
Rossel et al. (2006) [ ] | GeoD | 10.1016/j.geoderma.2005.03.007 | 1332 | 78.35 | 517 | 30.41 |
Nannipieri et al. (2003) [ ] | EJSS | 10.1046/j.1351-0754.2003.0556.x | 1331 | 66.55 | 456 | 22.80 |
Liang et al. (2006) [ ] | SSSAJ | 10.2136/sssaj2005.0383 | 1330 | 78.24 | 318 | 18.71 |
Wrage et al. (2001) [ ] | SBB | 10.1016/S0038-0717(01)00096-7 | 1282 | 58.27 | 316 | 14.36 |
Chang et al. (2001) [ ] | SSSAJ | 10.2136/sssaj2001.652480x | 1281 | 58.23 | 426 | 19.36 |
Le Mer and Roger (2001) [ ] | EJSB | 10.1016/S1164-5563(01)01067-6 | 1265 | 57.50 | 230 | 10.45 |
Westoby (1998) [ ] | P & S | 10.1023/A:1004327224729 | 1248 | 49.92 | 19 | 0.76 |
Kogel-Knabner (2002) [ ] | SBB | 10.1016/S0038-0717(01)00158-4 | 1228 | 58.48 | 470 | 22.38 |
Lehmann et al. (2003) [ ] | P & S | 10.1023/A:1022833116184 | 1217 | 60.85 | 319 | 15.95 |
Kuzyakov (2010) [ ] | SBB | 10.1016/j.soilbio.2010.04.003 | 1178 | 90.62 | 482 | 37.08 |
Fierer et al. (2003) [ ] | SBB | 10.1016/S0038-0717(02)00251-1 | 1170 | 58.50 | 471 | 23.55 |
Cakmak (2008) [ ] | P & S | 10.1007/s11104-007-9466-3 | 1150 | 76.67 | 185 | 12.33 |
Rasse et al. (2005) [ ] | P & S | 10.1007/s11104-004-0907-y | 1150 | 63.89 | 442 | 24.56 |
Six et al. (2006) [ ] | SSSAJ | 10.2136/sssaj2004.0347 | 1147 | 67.47 | 509 | 29.94 |
Chan et al. (2007) [ ] | SR | 10.1071/SR07109 | 1139 | 71.19 | 265 | 16.56 |
Van Zwieten et al. (2010) [ ] | P & S | 10.1007/s11104-009-0050-x | 1139 | 87.62 | 266 | 20.46 |
Six et al. (1998) [ ] | SSSAJ | 10.2136/sssaj1998.03615995006200050032x | 1126 | 45.04 | 642 | 25.68 |
Lauber et al. (2008) [ ] | SBB | 10.1016/j.soilbio.2008.05.021 | 1123 | 74.87 | 324 | 21.60 |
Burns et al. (2013) [ ] | SBB | 10.1016/j.soilbio.2012.11.009 | 1117 | 111.70 | 438 | 43.80 |
Hamza and Anderson (2005) [ ] | STR | 10.1016/j.still.2004.08.009 | 1104 | 61.33 | 404 | 22.44 |
Saiya-Cork et al. (2002) [ ] | SBB | 10.1016/S0038-0717(02)00074-3 | 1087 | 51.76 | 434 | 20.67 |
Compant et al. (2010) [ ] | SBB | 10.1016/j.soilbio.2009.11.024 | 1079 | 83.00 | 112 | 8.62 |
Fontaine et al. (2003) [ ] | SBB | 10.1016/S0038-0717(03)00123-8 | 1074 | 53.70 | 507 | 25.35 |
Frostegard et al. (1993b) [ ] | SBB | 10.1016/0038-0717(93)90113-P | 1072 | 35.73 | 540 | 18.00 |
Vonuexkull and Mutert (1995) [ ] | P & S | 10.1007/BF00009558 | 1051 | 37.54 | 190 | 6.79 |
Sollins et al. (1996) [ ] | GeoD | 10.1016/S0016-7061(96)00036-5 | 1047 | 38.78 | 463 | 17.15 |
Keywords | Year | Strength | Begin | End | 1992–2022 |
---|---|---|---|---|---|
aluminum | 1992 | 263.34 | 1992 | 2005 | ▃▃▃▃▃▃▃▃▃▃▃▃▃▃▂▂▂▂▂▂▂▂▂▂▂▂▂▂▂▂▂ |
soil | 1992 | 223.71 | 1992 | 2000 | ▃▃▃▃▃▃▃▃▃▂▂▂▂▂▂▂▂▂▂▂▂▂▂▂▂▂▂▂▂▂▂ |
nitrogen fixation | 1992 | 214.72 | 1992 | 2003 | ▃▃▃▃▃▃▃▃▃▃▃▃▂▂▂▂▂▂▂▂▂▂▂▂▂▂▂▂▂▂▂ |
extraction | 1992 | 258.23 | 1992 | 2009 | ▃▃▃▃▃▃▃▃▃▃▃▃▃▃▃▃▃▃▂▂▂▂▂▂▂▂▂▂▂▂▂ |
nitrification | 1992 | 200.12 | 1992 | 2006 | ▃▃▃▃▃▃▃▃▃▃▃▃▃▃▃▂▂▂▂▂▂▂▂▂▂▂▂▂▂▂▂ |
denitrification | 1992 | 149.81 | 1992 | 2009 | ▃▃▃▃▃▃▃▃▃▃▃▃▃▃▃▃▃▃▂▂▂▂▂▂▂▂▂▂▂▂▂ |
nitrate | 1992 | 139.23 | 1992 | 2006 | ▃▃▃▃▃▃▃▃▃▃▃▃▃▃▃▂▂▂▂▂▂▂▂▂▂▂▂▂▂▂▂ |
pH | 1992 | 114.67 | 1992 | 2005 | ▃▃▃▃▃▃▃▃▃▃▃▃▃▃▂▂▂▂▂▂▂▂▂▂▂▂▂▂▂▂▂ |
ammonium | 1992 | 111.07 | 1992 | 2000 | ▃▃▃▃▃▃▃▃▃▂▂▂▂▂▂▂▂▂▂▂▂▂▂▂▂▂▂▂▂▂▂ |
clay | 1992 | 90.99 | 1992 | 1997 | ▃▃▃▃▃▃▂▂▂▂▂▂▂▂▂▂▂▂▂▂▂▂▂▂▂▂▂▂▂▂▂ |
seedling | 1992 | 87.39 | 1992 | 1999 | ▃▃▃▃▃▃▃▃▂▂▂▂▂▂▂▂▂▂▂▂▂▂▂▂▂▂▂▂▂▂▂ |
N 15 | 1992 | 81.14 | 1992 | 1997 | ▃▃▃▃▃▃▂▂▂▂▂▂▂▂▂▂▂▂▂▂▂▂▂▂▂▂▂▂▂▂▂ |
bacteria | 1992 | 80.3 | 1992 | 2002 | ▃▃▃▃▃▃▃▃▃▃▃▂▂▂▂▂▂▂▂▂▂▂▂▂▂▂▂▂▂▂▂ |
adsorption | 1992 | 61.62 | 1992 | 2003 | ▃▃▃▃▃▃▃▃▃▃▃▃▂▂▂▂▂▂▂▂▂▂▂▂▂▂▂▂▂▂▂ |
barley | 1992 | 52.24 | 1992 | 1997 | ▃▃▃▃▃▃▂▂▂▂▂▂▂▂▂▂▂▂▂▂▂▂▂▂▂▂▂▂▂▂▂ |
calcium | 1992 | 42.78 | 1992 | 1996 | ▃▃▃▃▃▂▂▂▂▂▂▂▂▂▂▂▂▂▂▂▂▂▂▂▂▂▂▂▂▂▂ |
nodulation | 1992 | 42.56 | 1992 | 1995 | ▃▃▃▃▂▂▂▂▂▂▂▂▂▂▂▂▂▂▂▂▂▂▂▂▂▂▂▂▂▂▂ |
infection | 1992 | 41.75 | 1992 | 1996 | ▃▃▃▃▃▂▂▂▂▂▂▂▂▂▂▂▂▂▂▂▂▂▂▂▂▂▂▂▂▂▂ |
fungi | 1992 | 39.97 | 1992 | 1996 | ▃▃▃▃▃▂▂▂▂▂▂▂▂▂▂▂▂▂▂▂▂▂▂▂▂▂▂▂▂▂▂ |
nutrition | 1992 | 39.08 | 1992 | 1995 | ▃▃▃▃▂▂▂▂▂▂▂▂▂▂▂▂▂▂▂▂▂▂▂▂▂▂▂▂▂▂▂ |
fixation | 1992 | 37.52 | 1992 | 1994 | ▃▃▃▂▂▂▂▂▂▂▂▂▂▂▂▂▂▂▂▂▂▂▂▂▂▂▂▂▂▂▂ |
wheat | 1992 | 29.91 | 1992 | 1995 | ▃▃▃▃▂▂▂▂▂▂▂▂▂▂▂▂▂▂▂▂▂▂▂▂▂▂▂▂▂▂▂ |
litter | 1993 | 101.11 | 1993 | 2001 | ▂▃▃▃▃▃▃▃▃▃▂▂▂▂▂▂▂▂▂▂▂▂▂▂▂▂▂▂▂▂▂ |
winter wheat | 1993 | 43.78 | 1993 | 1998 | ▂▃▃▃▃▃▃▂▂▂▂▂▂▂▂▂▂▂▂▂▂▂▂▂▂▂▂▂▂▂▂ |
movement | 1993 | 37.08 | 1993 | 1995 | ▂▃▃▃▂▂▂▂▂▂▂▂▂▂▂▂▂▂▂▂▂▂▂▂▂▂▂▂▂▂▂ |
acid soil | 1993 | 26.22 | 1993 | 1995 | ▂▃▃▃▂▂▂▂▂▂▂▂▂▂▂▂▂▂▂▂▂▂▂▂▂▂▂▂▂▂▂ |
manganese | 1993 | 22.74 | 1993 | 1994 | ▂▃▃▂▂▂▂▂▂▂▂▂▂▂▂▂▂▂▂▂▂▂▂▂▂▂▂▂▂▂▂ |
nitrogen mineralization | 1992 | 216.23 | 1994 | 2011 | ▂▂▃▃▃▃▃▃▃▃▃▃▃▃▃▃▃▃▃▃▂▂▂▂▂▂▂▂▂▂▂ |
oxide | 1994 | 26.07 | 1994 | 1996 | ▂▂▃▃▃▂▂▂▂▂▂▂▂▂▂▂▂▂▂▂▂▂▂▂▂▂▂▂▂▂▂ |
systems | 1994 | 27.24 | 1994 | 2013 | ▃▃▃▃▃▃▃▃▃▃▃▃▃▃▃▃▃▃▃▃▂▂▂▂▂▂▂▂▂▂▂ |
population | 1992 | 217.24 | 1995 | 2010 | ▂▂▂▃▃▃▃▃▃▃▃▃▃▃▃▃▃▃▃▂▂▂▂▂▂▂▂▂▂▂▂ |
zinc | 1992 | 151.25 | 1995 | 2010 | ▂▂▂▃▃▃▃▃▃▃▃▃▃▃▃▃▃▃▃▂▂▂▂▂▂▂▂▂▂▂▂ |
rotation | 1995 | 44.19 | 1995 | 1999 | ▂▂▂▃▃▃▃▃▂▂▂▂▂▂▂▂▂▂▂▂▂▂▂▂▂▂▂▂▂▂▂ |
chemistry | 1995 | 26.8 | 1995 | 2000 | ▂▂▂▃▃▃▃▃▃▂▂▂▂▂▂▂▂▂▂▂▂▂▂▂▂▂▂▂▂▂▂ |
dissolution | 1995 | 25.91 | 1995 | 1996 | ▂▂▂▃▃▂▂▂▂▂▂▂▂▂▂▂▂▂▂▂▂▂▂▂▂▂▂▂▂▂▂ |
pasture | 1996 | 48.73 | 1996 | 2000 | ▂▂▂▂▃▃▃▃▃▂▂▂▂▂▂▂▂▂▂▂▂▂▂▂▂▂▂▂▂▂▂ |
transformation | 1992 | 150.94 | 1997 | 2007 | ▂▂▂▂▂▃▃▃▃▃▃▃▃▃▃▃▂▂▂▂▂▂▂▂▂▂▂▂▂▂▂ |
extraction method | 1997 | 47.29 | 1997 | 2001 | ▂▂▂▂▂▃▃▃▃▃▂▂▂▂▂▂▂▂▂▂▂▂▂▂▂▂▂▂▂▂▂ |
oxidation | 1997 | 29.07 | 1997 | 1998 | ▂▂▂▂▂▃▃▂▂▂▂▂▂▂▂▂▂▂▂▂▂▂▂▂▂▂▂▂▂▂▂ |
field | 1992 | 25.8 | 1998 | 2000 | ▂▂▂▂▂▂▃▃▃▂▂▂▂▂▂▂▂▂▂▂▂▂▂▂▂▂▂▂▂▂▂ |
sorption | 1992 | 100.27 | 1999 | 2008 | ▂▂▂▂▂▂▂▃▃▃▃▃▃▃▃▃▃▂▂▂▂▂▂▂▂▂▂▂▂▂▂ |
humic substance | 1993 | 36.25 | 1999 | 2000 | ▂▂▂▂▂▂▂▃▃▂▂▂▂▂▂▂▂▂▂▂▂▂▂▂▂▂▂▂▂▂▂ |
loess | 2000 | 73 | 2000 | 2003 | ▂▂▂▂▂▂▂▂▃▃▃▃▂▂▂▂▂▂▂▂▂▂▂▂▂▂▂▂▂▂▂ |
surface | 1992 | 34.42 | 2000 | 2001 | ▂▂▂▂▂▂▂▂▃▃▂▂▂▂▂▂▂▂▂▂▂▂▂▂▂▂▂▂▂▂▂ |
iron | 1992 | 78.04 | 2001 | 2005 | ▂▂▂▂▂▂▂▂▂▃▃▃▃▃▂▂▂▂▂▂▂▂▂▂▂▂▂▂▂▂▂ |
rate | 1992 | 65.23 | 2001 | 2004 | ▂▂▂▂▂▂▂▂▂▃▃▃▃▂▂▂▂▂▂▂▂▂▂▂▂▂▂▂▂▂▂ |
kinetics | 1992 | 31.24 | 2001 | 2002 | ▂▂▂▂▂▂▂▂▂▃▃▂▂▂▂▂▂▂▂▂▂▂▂▂▂▂▂▂▂▂▂ |
copper | 1992 | 67.71 | 2002 | 2005 | ▂▂▂▂▂▂▂▂▂▂▃▃▃▃▂▂▂▂▂▂▂▂▂▂▂▂▂▂▂▂▂ |
toxicity | 1992 | 100.52 | 2003 | 2007 | ▂▂▂▂▂▂▂▂▂▂▂▃▃▃▃▃▂▂▂▂▂▂▂▂▂▂▂▂▂▂▂ |
release | 1993 | 34.44 | 2003 | 2004 | ▂▂▂▂▂▂▂▂▂▂▂▃▃▂▂▂▂▂▂▂▂▂▂▂▂▂▂▂▂▂▂ |
cultivation | 1992 | 28.61 | 2003 | 2004 | ▂▂▂▂▂▂▂▂▂▂▂▃▃▂▂▂▂▂▂▂▂▂▂▂▂▂▂▂▂▂▂ |
grassland | 2004 | 24.37 | 2004 | 2013 | ▂▂▂▂▂▂▂▂▂▂▂▃▃▃▃▃▃▃▃▃▃▂▂▂▂▂▂▂▂▂▂ |
infiltration | 1992 | 107.19 | 2004 | 2011 | ▂▂▂▂▂▂▂▂▂▂▂▂▃▃▃▃▃▃▃▃▂▂▂▂▂▂▂▂▂▂▂ |
turnover | 1992 | 66.38 | 2005 | 2007 | ▂▂▂▂▂▂▂▂▂▂▂▂▂▃▃▃▂▂▂▂▂▂▂▂▂▂▂▂▂▂▂ |
corn | 1992 | 50.36 | 2005 | 2007 | ▂▂▂▂▂▂▂▂▂▂▂▂▂▃▃▃▂▂▂▂▂▂▂▂▂▂▂▂▂▂▂ |
carbon dioxide | 2001 | 108.17 | 2006 | 2010 | ▂▂▂▂▂▂▂▂▂▂▂▂▂▂▃▃▃▃▃▂▂▂▂▂▂▂▂▂▂▂▂ |
residue | 1996 | 58.19 | 2006 | 2007 | ▂▂▂▂▂▂▂▂▂▂▂▂▂▂▃▃▂▂▂▂▂▂▂▂▂▂▂▂▂▂▂ |
sewage sludge | 1992 | 44.82 | 2006 | 2007 | ▂▂▂▂▂▂▂▂▂▂▂▂▂▂▃▃▂▂▂▂▂▂▂▂▂▂▂▂▂▂▂ |
manure | 2004 | 128.74 | 2008 | 2017 | ▂▂▂▂▂▂▂▂▂▂▂▂▂▂▂▂▃▃▃▃▃▃▃▃▃▃▂▂▂▂▂ |
acid | 1992 | 48.05 | 2008 | 2009 | ▂▂▂▂▂▂▂▂▂▂▂▂▂▂▂▂▃▃▂▂▂▂▂▂▂▂▂▂▂▂▂ |
spatial variability | 2003 | 72.7 | 2009 | 2011 | ▂▂▂▂▂▂▂▂▂▂▂▂▂▂▂▂▂▃▃▃▂▂▂▂▂▂▂▂▂▂▂ |
water content | 2000 | 52.45 | 2009 | 2010 | ▂▂▂▂▂▂▂▂▂▂▂▂▂▂▂▂▂▃▃▂▂▂▂▂▂▂▂▂▂▂▂ |
cadmium | 1996 | 40.61 | 2010 | 2011 | ▂▂▂▂▂▂▂▂▂▂▂▂▂▂▂▂▂▂▃▃▂▂▂▂▂▂▂▂▂▂▂ |
no tillage | 2007 | 148.31 | 2011 | 2016 | ▂▂▂▂▂▂▂▂▂▂▂▂▂▂▂▂▂▂▂▃▃▃▃▃▃▂▂▂▂▂▂ |
carbon sequestration | 2009 | 67.83 | 2011 | 2022 | ▂▂▂▂▂▂▂▂▂▂▂▂▂▂▂▂▂▂▂▃▃▃▃▃▃▃▃▃▃▃▃ |
flow | 1992 | 41.29 | 2011 | 2012 | ▂▂▂▂▂▂▂▂▂▂▂▂▂▂▂▂▂▂▂▃▃▂▂▂▂▂▂▂▂▂▂ |
hydraulic conductivity | 1992 | 23.87 | 2011 | 2013 | ▂▂▂▂▂▂▂▂▂▂▂▂▂▂▂▂▂▂▂▃▃▃▂▂▂▂▂▂▂▂▂ |
nitrous oxide | 1996 | 42.59 | 2012 | 2015 | ▂▂▂▂▂▂▂▂▂▂▂▂▂▂▂▂▂▂▂▂▃▃▃▃▂▂▂▂▂▂▂ |
plant growth | 2010 | 95.38 | 2014 | 2022 | ▂▂▂▂▂▂▂▂▂▂▂▂▂▂▂▂▂▂▂▂▂▂▃▃▃▃▃▃▃▃▃ |
emission | 1997 | 63.31 | 2014 | 2015 | ▂▂▂▂▂▂▂▂▂▂▂▂▂▂▂▂▂▂▂▂▂▂▃▃▂▂▂▂▂▂▂ |
productivity | 2010 | 95.65 | 2015 | 2022 | ▂▂▂▂▂▂▂▂▂▂▂▂▂▂▂▂▂▂▂▂▂▂▂▃▃▃▃▃▃▃▃ |
moisture | 1996 | 93.37 | 2015 | 2020 | ▂▂▂▂▂▂▂▂▂▂▂▂▂▂▂▂▂▂▂▂▂▂▂▃▃▃▃▃▃▂▂ |
arbuscular mycorrhizal fungi | 2005 | 81.7 | 2015 | 2017 | ▂▂▂▂▂▂▂▂▂▂▂▂▂▂▂▂▂▂▂▂▂▂▂▃▃▃▂▂▂▂▂ |
climate change | 2010 | 242.51 | 2016 | 2022 | ▂▂▂▂▂▂▂▂▂▂▂▂▂▂▂▂▂▂▂▂▂▂▂▂▃▃▃▃▃▃▃ |
microbial community | 2005 | 184.58 | 2016 | 2022 | ▂▂▂▂▂▂▂▂▂▂▂▂▂▂▂▂▂▂▂▂▂▂▂▂▃▃▃▃▃▃▃ |
biodiversity | 2008 | 110.87 | 2016 | 2022 | ▂▂▂▂▂▂▂▂▂▂▂▂▂▂▂▂▂▂▂▂▂▂▂▂▃▃▃▃▃▃▃ |
land use change | 2016 | 77.07 | 2016 | 2017 | ▂▂▂▂▂▂▂▂▂▂▂▂▂▂▂▂▂▂▂▂▂▂▂▂▃▃▂▂▂▂▂ |
crop | 1992 | 39.27 | 2016 | 2019 | ▂▂▂▂▂▂▂▂▂▂▂▂▂▂▂▂▂▂▂▂▂▂▂▂▃▃▃▃▂▂▂ |
soil erosion | 1998 | 26.08 | 2016 | 2018 | ▂▂▂▂▂▂▂▂▂▂▂▂▂▂▂▂▂▂▂▂▂▂▂▂▃▃▃▂▂▂▂ |
loess plateau | 2017 | 265.78 | 2017 | 2022 | ▂▂▂▂▂▂▂▂▂▂▂▂▂▂▂▂▂▂▂▂▂▂▂▂▂▃▃▃▃▃▃ |
bacterial community | 2017 | 229.87 | 2017 | 2022 | ▂▂▂▂▂▂▂▂▂▂▂▂▂▂▂▂▂▂▂▂▂▂▂▂▂▃▃▃▃▃▃ |
amendment | 2015 | 118.07 | 2017 | 2019 | ▂▂▂▂▂▂▂▂▂▂▂▂▂▂▂▂▂▂▂▂▂▂▂▂▂▃▃▃▂▂▂ |
china | 2017 | 103.43 | 2017 | 2018 | ▂▂▂▂▂▂▂▂▂▂▂▂▂▂▂▂▂▂▂▂▂▂▂▂▂▃▃▂▂▂▂ |
climate | 2013 | 180.11 | 2018 | 2022 | ▂▂▂▂▂▂▂▂▂▂▂▂▂▂▂▂▂▂▂▂▂▂▂▂▂▂▃▃▃▃▃ |
prediction | 2014 | 152.55 | 2018 | 2022 | ▂▂▂▂▂▂▂▂▂▂▂▂▂▂▂▂▂▂▂▂▂▂▂▂▂▂▃▃▃▃▃ |
organic carbon | 1995 | 145.27 | 2018 | 2022 | ▂▂▂▂▂▂▂▂▂▂▂▂▂▂▂▂▂▂▂▂▂▂▂▂▂▂▃▃▃▃▃ |
community structure | 2008 | 76.06 | 2018 | 2022 | ▂▂▂▂▂▂▂▂▂▂▂▂▂▂▂▂▂▂▂▂▂▂▂▂▂▂▃▃▃▃▃ |
storage | 2008 | 69.09 | 2018 | 2019 | ▂▂▂▂▂▂▂▂▂▂▂▂▂▂▂▂▂▂▂▂▂▂▂▂▂▂▃▃▂▂▂ |
aggregate stability | 2001 | 56.62 | 2018 | 2019 | ▂▂▂▂▂▂▂▂▂▂▂▂▂▂▂▂▂▂▂▂▂▂▂▂▂▂▃▃▂▂▂ |
maize | 1993 | 29.46 | 2018 | 2019 | ▂▂▂▂▂▂▂▂▂▂▂▂▂▂▂▂▂▂▂▂▂▂▂▂▂▂▃▃▂▂▂ |
use efficiency | 2019 | 184.81 | 2019 | 2022 | ▂▂▂▂▂▂▂▂▂▂▂▂▂▂▂▂▂▂▂▂▂▂▂▂▂▂▂▃▃▃▃ |
impact | 2002 | 136.36 | 2019 | 2022 | ▂▂▂▂▂▂▂▂▂▂▂▂▂▂▂▂▂▂▂▂▂▂▂▂▂▂▂▃▃▃▃ |
stabilization | 2014 | 81.88 | 2019 | 2020 | ▂▂▂▂▂▂▂▂▂▂▂▂▂▂▂▂▂▂▂▂▂▂▂▂▂▂▂▃▃▂▂ |
response | 1998 | 71.46 | 2019 | 2022 | ▂▂▂▂▂▂▂▂▂▂▂▂▂▂▂▂▂▂▂▂▂▂▂▂▂▂▂▃▃▃▃ |
abundance | 2017 | 139.99 | 2019 | 2022 | ▂▂▂▂▂▂▂▂▂▂▂▂▂▂▂▂▂▂▂▂▂▂▂▂▂▂▂▃▃▃▃ |
enzyme activity | 1999 | 49.54 | 2019 | 2022 | ▂▂▂▂▂▂▂▂▂▂▂▂▂▂▂▂▂▂▂▂▂▂▂▂▂▂▂▃▃▃▃ |
bacterial | 2020 | 176.55 | 2020 | 2022 | ▂▂▂▂▂▂▂▂▂▂▂▂▂▂▂▂▂▂▂▂▂▂▂▂▂▂▂▂▃▃▃ |
diversity | 1999 | 96.31 | 2020 | 2022 | ▂▂▂▂▂▂▂▂▂▂▂▂▂▂▂▂▂▂▂▂▂▂▂▂▂▂▂▂▃▃▃ |
N O emission | 2020 | 93.46 | 2020 | 2022 | ▂▂▂▂▂▂▂▂▂▂▂▂▂▂▂▂▂▂▂▂▂▂▂▂▂▂▂▂▃▃▃ |
soil property | 2009 | 89.86 | 2020 | 2022 | ▂▂▂▂▂▂▂▂▂▂▂▂▂▂▂▂▂▂▂▂▂▂▂▂▂▂▂▂▃▃▃ |
agricultural soil | 2008 | 67.93 | 2020 | 2022 | ▂▂▂▂▂▂▂▂▂▂▂▂▂▂▂▂▂▂▂▂▂▂▂▂▂▂▂▂▃▃▃ |
Journal Name | Abbrev. | Gender Diversity of Editors | Editorial Board by Country/Region |
---|---|---|---|
Soil Biology and Biochemistry | SBB | 50% men; 50% women Data represent responses from 67% of 18 editors | 95 members in 21 countries/regions |
Geoderma | GeoD | 63% men; 38% women Data represent responses from 83% of 29 editors | 122 members in 25 countries/regions |
Catena | Catena | 67% men; 28% women; 6% prefer not to disclose Data represent responses from 82% of 22 editors | 58 members in 20 countries/regions |
Soil and Tillage Research | STR | 86% men; 14% women Data represent responses from 70% of 10 editors | 49 members in 21 countries/regions |
Applied Soil Ecology | ASE | 50% men; 50% women Data represent responses from 75% of 8 editors | 65 members in 23 countries/regions |
Rhizosphere | Rhiz | N/A | 40 members in 20 countries/regions |
Geoderma Regional | GR | N/A | 54 members in 26 countries/regions |
European Journal of Soil Biology | EJSB | N/A | 65 members in 21 countries/regions |
Pedobiologia | PedoB | N/A | 52 members in 18 countries/regions |
The statements, opinions and data contained in all publications are solely those of the individual author(s) and contributor(s) and not of MDPI and/or the editor(s). MDPI and/or the editor(s) disclaim responsibility for any injury to people or property resulting from any ideas, methods, instructions or products referred to in the content. |
Share and Cite
Jia, L.; Wang, W.; Zvomuya, F.; He, H. Trends in Soil Science over the Past Three Decades (1992–2022) Based on the Scientometric Analysis of 39 Soil Science Journals. Agriculture 2024 , 14 , 445. https://doi.org/10.3390/agriculture14030445
Jia L, Wang W, Zvomuya F, He H. Trends in Soil Science over the Past Three Decades (1992–2022) Based on the Scientometric Analysis of 39 Soil Science Journals. Agriculture . 2024; 14(3):445. https://doi.org/10.3390/agriculture14030445
Jia, Lang, Wenjuan Wang, Francis Zvomuya, and Hailong He. 2024. "Trends in Soil Science over the Past Three Decades (1992–2022) Based on the Scientometric Analysis of 39 Soil Science Journals" Agriculture 14, no. 3: 445. https://doi.org/10.3390/agriculture14030445
Article Metrics
Article access statistics, further information, mdpi initiatives, follow mdpi.
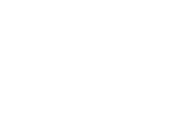
Subscribe to receive issue release notifications and newsletters from MDPI journals
Article
- Volume 6, issue 1
- SOIL, 6, 35–52, 2020
- Peer review
- Related articles
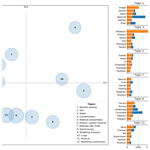
Machine learning and soil sciences: a review aided by machine learning tools
José padarian, budiman minasny, alex b. mcbratney.
The application of machine learning (ML) techniques in various fields of science has increased rapidly, especially in the last 10 years. The increasing availability of soil data that can be efficiently acquired remotely and proximally, and freely available open-source algorithms, have led to an accelerated adoption of ML techniques to analyse soil data. Given the large number of publications, it is an impossible task to manually review all papers on the application of ML in soil science without narrowing down a narrative of ML application in a specific research question. This paper aims to provide a comprehensive review of the application of ML techniques in soil science aided by a ML algorithm (latent Dirichlet allocation) to find patterns in a large collection of text corpora. The objective is to gain insight into publications of ML applications in soil science and to discuss the research gaps in this topic. We found that (a) there is an increasing usage of ML methods in soil sciences, mostly concentrated in developed countries, (b) the reviewed publications can be grouped into 12 topics, namely remote sensing, soil organic carbon, water, contamination, methods (ensembles), erosion and parent material, methods (NN, neural networks, SVM, support vector machines), spectroscopy, modelling (classes), crops, physical, and modelling (continuous), and (c) advanced ML methods usually perform better than simpler approaches thanks to their capability to capture non-linear relationships. From these findings, we found research gaps, in particular, about the precautions that should be taken (parsimony) to avoid overfitting, and that the interpretability of the ML models is an important aspect to consider when applying advanced ML methods in order to improve our knowledge and understanding of soil. We foresee that a large number of studies will focus on the latter topic.
- Article (PDF, 2353 KB)
- Article (2353 KB)
- Full-text XML

Padarian, J., Minasny, B., and McBratney, A. B.: Machine learning and soil sciences: a review aided by machine learning tools, SOIL, 6, 35–52, https://doi.org/10.5194/soil-6-35-2020, 2020.
The application of machine learning (ML) techniques in various fields of science has increased rapidly, especially in the last 10 years. Soil science research, in particular, pedometrics, has used statistical models to “learn” or understand from data how soil is distributed in space and time ( McBratney et al. , 2019 ) . The increasing availability of soil data that can be efficiently acquired remotely and proximally, and freely available open-source algorithms, have led to an accelerated adoption of ML techniques to analyse soil data. Several well-known ML applications in soils science include the prediction of soil types and properties via digital soil mapping (DSM) or pedotransfer functions and analysis of infrared spectral data to infer soil properties. Machine learning analysis of soil data is also used to draw conclusions on the controls of the distribution of the soil.
The definition of what constitutes ML is still contentious or sometimes mistaken. In this work, instead of adding a new argument to differentiate ML from statistical science, we will focus on the view of Jordan and Mitchell ( 2015 ) , where ML is “lying at the intersection of computer science and statistics”. With respect to artificial intelligence (AI), sometimes we have seen the terms ML and AI used interchangeably. This is understandable confusion since ML is a subset of AI, but not everything related to AI falls in the ML category (e.g. expert systems).
There are concerns that ML application ignores soil science knowledge ( Rossiter , 2018 ) and that the results could be misleading and wrong. Nevertheless, many would find that ML methods can help in the scientific process ( Mjolsness and DeCoste , 2001 ; Rudin and Wagstaff , 2014 ) : observations, empirical and theory-based model development, and simulations of soil processes ( Rossiter , 2018 ) . For example, exploration of high-dimensional infrared spectral data helps in understanding the horizonation designation in a soil profile ( Fajardo et al. , 2016 ) . The process of modelling and validation can be used to formulate a model to explain soil distribution ( Brungard et al. , 2015 ) . Modelling via ML can also be used to improve our understanding of the causes of soil variation. Results from ML models can inform on which environmental variables control soil distribution. New relationships revealed by ML analysis can help to stimulate ideas, generate hypotheses, and formulate future questions for research ( Ma et al. , 2019 ) .
This paper aims to provide a comprehensive review of the application of ML techniques in soil science. A quick Google Scholar search of “soil” and “machine learning” resulted in more than 70 000 items, with 16 000 items published in 2018. While we can narrow down a narrative of ML application in a specific research question, such as the application of ML in yield prediction in precision agriculture ( Chlingaryan et al. , 2018 ) or DSM, it is an impossible task to manually review all papers on the application of ML in soil science. One ML technique that has not been applied in soil science is topic modelling, a type of quantitative text mining method. Similar to what ML does to numerical data, topic modelling finds patterns in a large collection of text corpora ( Blei et al. , 2003 ; Blei , 2012 ) , and it has been used to study the evolution of various disciplines and topics ( Zhou et al. , 2006 ; Sugimoto et al. , 2011 ; Wu et al. , 2014 ) .
This paper uses topic modelling to analyse the trend in ML application in soil science. The objective is to gain insight into publications of ML applications in soil science; in particular, we will try to answer the following questions.
Who is using ML, and is the application of ML as ubiquitous as we think?
Which ML methods are commonly used and how often have they been used?
In which areas of soil sciences do we use ML, and how are they clustered and related?
Do advanced ML methods perform significantly better than linear or non-linear statistical approaches?
Can ML methods simulate soil processes in space and time?
Can we use ML methods to improve our knowledge and understanding of soil?
Throughout this review, we will refer to models as “simple” or “complex/advanced”, trusting in the readers' criteria. To illustrate that gradient between simple and complex, we considered a linear model (LM) with 2 variables to be simple compared to a LM with 100 variables; a classification and regression tree (CART) with 2 branches to be simple compared to a CART with 100 branches; and finally, a CART with 2 branches to be simple compared with a LM with 100 variables. We also hope that is clear for the reader that a model such as a deep convolutional neural network (CNN) has many parameters; hence, it is more complex than a CART model.
2.1 Article selection
In order to identify the primary group of articles, we used the term “soil `machine learning”' to perform a full-text search in databases from different publishers. We selected the publishers based on (a) our institution having access to full-text articles and (b) that they provide text-mining permission. We limited our search to the English-only literature, without fixing a specific time frame, and completing the search on 1 February 2019. After performing a screening for the relevance of the initial 3044 matches, we decided to narrow down the selection to the articles containing the word “soil” in their title, yielding a total of 322 articles. The final journal names and number of articles are shown in Table A1 in the Appendix.
2.2 Topic modelling
Topic modelling is a probabilistic ML method that aims to discover and annotate large archives of documents with thematic information Blei ( 2012 ) . By analysing the words contained in a set of documents, these topic modelling algorithms are capable of identifying common themes. These methods allow processing of an arbitrarily large number of articles, which can help to reduce part of the bias introduced by only selecting a manageable subset of documents or by manually assigning documents to topics.
In order to determine in which areas of soil sciences we use ML, we selected an algorithm commonly used in topic modelling called latent Dirichlet allocation (LDA) ( Blei et al. , 2003 ) to perform the task of allocating the articles to topics. LDA is a probabilistic model that assumes that a number of topics exist in a document collection and each topic is represented by a distribution of words. Each document is represented by a distribution over topics, and each word is a sample over each topic's vocabulary (Fig. 1 ). For more details about the LDA, we refer the reader to Blei ( 2012 ) .
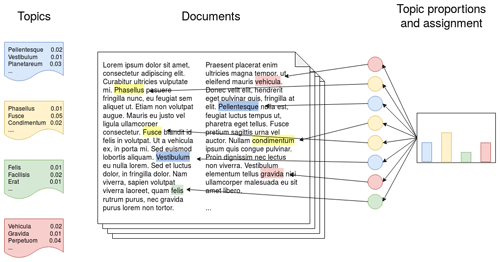
Figure 1 Collection of topics with distribution of words (left), document distribution over topics (histogram, right), and words sampled from the topics' vocabularies (circles). The topics, words, and assignment are for illustrative purposes. Adapted from Blei ( 2012 ) .
Before modelling the topics, we pre-processed the documents in order to reduce the noise of the unstructured texts. We (a) removed stop words (common words such as “from” and “are”), (b) generated bi- and tri-grams, which are groups of two or three words which commonly appear together in the text (e.g. “remote sensing”, “particle size distribution”), and (c) removed extremely uncommon (that appear in less than five documents) and common words (that appear in more than 50 % of the documents), which do not help to differentiate between topics.
The LDA algorithm is capable of learning different topics to which each document is assigned given the words that constitute it. The first challenge is to find the optimal number of topics, which has to be general enough to capture similarities between articles but with some degree of specificity in order to have a manageable and sensible group of topics. That balance between generality and specificity is key to generating topics that are semantically interpretable by a human ( Stevens et al. , 2012 ) . One of the measures that is highly correlated with human interpretation of the topics is topic coherence ( Stevens et al. , 2012 ) . We estimated a coherence measure proposed by Röder et al. ( 2015 ) (referenced as C V in their paper) for different models trained with an increasing number of topics, from 2 to 30. C V is an aggregated measure which combines a normalised point-wise mutual information coherence measure, cosine vector similarity, and a Boolean sliding window of size 110. It ranges from 0 to 1, 1 being the highest coherence. Other parameters of the LDA algorithm, such as the threshold of the probability above which a topic is considered, and the number of training iterations, were set to 0.2 and 1000, respectively, after performing a parameter grid search.
2.3 Text extraction
In order to identify the information required to answer our questions, we used a combination of named-entity recognition and rule-based matching. To extract the MODEL entities, we used a list of modelling methods from the Outline_of_machine_learning Wikipedia article in addition to other algorithms that are commonly used in soil sciences and that were not present in the list (e.g. Cubist). After extracting the MODEL entities, we proceeded to extract the abbreviations used to reference those models. In order to extract the abbreviations, we relied on the commonly seen pattern of writing model names followed by their corresponding abbreviation (e.g. “we used a random forest model (RF)”). By extracting the abbreviations we expected to discriminate between (a) models used to generate the results reported in the articles and (b) models mentioned to give context to the studies. Extracting abbreviations also allowed us to capture variations of models not present in our original list (e.g. BART for bagged regression trees in Fig. 2 ).
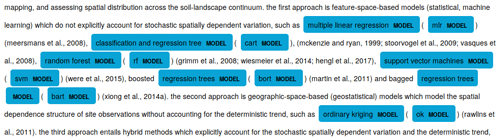
Figure 2 Excerpt from one of the reviewed articles showing named entities recognised as models. Note that the word “bagged” is not recognised, but the abbreviation “bart” is.
2.4 Implementation
We performed all our analysis in Python, using the libraries gensim v3.6.0 ( Řehůřek and Sojka , 2010 ) and the MALLET package ( McCallum , 2002 ) for the topic modelling and spacy v2.1.0a6 ( Matthew and Honnibal , 2017 ) for the named entity recognition.
3.1 Who is using machine learning methods?
The first questions related to the current status of the ML literature in soil sciences can be answered after correctly organising all the articles' metadata. Regarding the general usage of ML methods, in our review, we observed an expected increment in time in the number of publications using ML to model different aspects of soils (Fig. 3 ). This increment is most likely due to a combination of increasing computational power and accessibility to high-performance computers, increasing availability of data (e.g. remote sensing) ( Jordan and Mitchell , 2015 ) , and the increasing interest in “data science”. It is also confounded with the overall increase in the number of publications, which was estimated in 2015 at nearly 2.5 million new publications per year ( Ware and Mabe , 2015 ) .
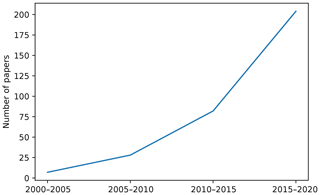
Figure 3 Distribution in time of the articles used in this review.
Besides the temporal trend in publishing, we were also interested in how ubiquitous the application of ML methods is. Fig. 4 shows the number of institutions per country (log 10 ) that appeared listed as affiliations in the analysed articles. ML techniques in the context of soil sciences are used in many countries around the world, but are mostly concentrated in developed countries. This is due to the inseparable relationship between science, technology, and development ( Sagasti , 1973 ) , which is also related to what is usually called the “digital divide” ( Rossiter , 2018 ) . Inter-institutional collaboration could be an important aspect of closing this gap ( Sonnenwald , 2007 ) . Similarly to what is happening in many disciplines ( Sonnenwald , 2007 ) , we observed an increase in the number of co-authors per article (Fig. 5 ), which might be a good sign if we avoid bad practices like “helicopter science” ( Minasny and Flantis , 2018 ) .
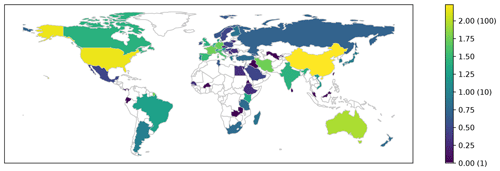
Figure 4 Total number (log 10 ) of institutions per country that participated in articles included in this review. Numbers between brackets are the real number of publications. Outlined countries have zero occurrences.
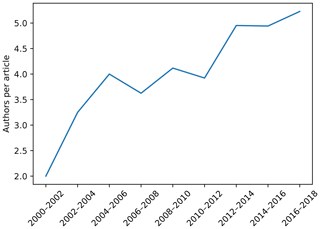
Figure 5 Evolution of the number of authors per publication. Mean values per time period.
The advance of a discipline is not only measured by the number of publications. Dissemination of knowledge is a key component of research and open access (OA) has been recognised as an optimal solution since it is in the best interests of all stakeholders involved in the process ( Björk , 2017 ) . In the application of ML in soil sciences, the proportion of OA publications is very low (Fig. 6 ). This number is in line with the overall OA presence in science ( Björk , 2017 ) but on the opposite side of the general trend in ML, where scientists prefer AO ( Hutson , 2018 ) .
3.2 Most used methods
From the huge variety of ML models available, we found over 100 different variants that have been applied in the soil sciences. From those, most have been applied experimentally in one or two papers, and just a handful are consistently used. Fig. 7 depicts the evolution of some selected models. There is an overall increase in the usage of all the models but, proportionally, it is possible to see a decrease in the usage of some models such as support vector machines (SVM), multivariate adaptive regression spline (MARS), and CART, giving way to more advanced alternatives such as random forest (RF). The adoption of the latter has an accelerated growth, and it has been used in a diversity of topics, including mapping and spectroscopy. The appearance of deep learning is also noticeable, which at the moment has only been used in a few publications related to mapping and spectroscopy.
3.3 Main topics
As we mentioned in Sect. 2.2 , in order to find the optimal number of topics present in the corpora, we trained models with an increasing number of topics (from 2 to 30) and we plotted the evolution of the C V coherence (Fig. 8 ). From this curve it is possible to select the number of topics that yield the highest coherence, which in this case is 12.
These 12 topics correspond to main soil areas detected by the LDA algorithm where ML is applied. We extracted the most relevant words for each of the 12 topics and we examined the titles of the more relevant papers to identify suitable “topic names”. The 12 identified areas were the following.
Articles heavily based on remote sensing ( Grunwald et al. , 2015 ; Xu et al. , 2017 ; Zhang et al. , 2018 b ) . Articles related to salinity were also assigned to this group since most of them use remote sensing techniques ( Khadim et al. , 2019 ; Zhang et al. , 2019 ) .
Articles related to soil organic carbon (SOC) cycles and dynamics and its relationship with the environment. Carbon stocks in different ecosystems, with particular emphasis on grasslands and topsoil ( Rial et al. , 2017 ; Liu et al. , 2018 ; Song et al. , 2018 ; Wang et al. , 2018 a ) .
Articles mostly focused on soil water content and its changes over time ( Ahmad et al. , 2010 ; Coopersmith et al. , 2014 ; Greifeneder et al. , 2018 ; Han et al. , 2018 ) . Other articles in this category are related to soil temperature and CO 2 fluxes ( Xing et al. , 2018 ; Oh et al. , 2019 ; Warner et al. , 2019 ; Zeynoddin et al. , 2019 ) . All these articles comprise measurements made by “stations”.
Articles addressing problems related to heavy metals, soil pollution, and bio-availability ( Costa et al. , 2017 ; Reeves et al. , 2018 ; Wu et al. , 2013 ) .
Articles with a focus on model ensembles such as RF ( Blanco et al. , 2018 ; Tziachris et al. , 2019 ) .
Articles focused on soil formation processes, specifically additions and losses by deposition and erosion, respectively ( Geissen et al. , 2007 ; Märker et al. , 2011 ; Martinez et al. , 2017 ) . Since soil formation depends on the parent material, articles aiming to characterise it were also included in this category ( Kheir et al. , 2008 ; Lacoste et al. , 2011 ) .
Articles with a focus on methods such as neural networks (NN) and SVM ( Kovačević et al. , 2010 ; Farfani et al. , 2015 ; Hanna et al. , 2007 ) .
This topic is related to proximal soil sensing covering different light wavelength sections, from microwave to infrared to gamma ( Heggemann et al. , 2017 ; Butler et al. , 2018 ; Xie and Li , 2018 ) .
Articles focused on the modelling, especially mapping, of categorical soil properties based on their relationship with environmental covariates ( Mansuy et al. , 2014 ; Camera et al. , 2017 ; Dharumarajan et al. , 2017 ; Massawe et al. , 2018 ) . In this category it is also possible to find articles related to the use of conventional soil maps, especially spatial disaggregation of polygons ( Subburayalu et al. , 2014 ; Vincent et al. , 2018 ; Flynn et al. , 2019 ) .
This group of articles focused not merely on soil, but also on its interaction within the soil–plant continuum. Water and nutrient availability in order to ensure crop yields is a key component of this topic ( Karandish and Šimŭnek , 2016 ; Ivushkin et al. , 2018 ; Khanal et al. , 2018 ; Leenaars et al. , 2018 ) .
Articles related to the physical properties of soils, including texture and bulk density ( Bondi et al. , 2018 ; Naderi-Boldaji et al. , 2019 ) , and how they affect aspects of soil such as water retention and flow ( Koestel and Jorda , 2014 ; Gao et al. , 2018 ) .
Articles focused on the modelling, especially mapping, of continuous soil properties based on their relationship with environmental covariates, from regional to continental scales ( Henderson et al. , 2005 ; Dai et al. , 2014 ; Poggio et al. , 2016 ; Padarian et al. , 2019 a ; Caubet et al. , 2019 ) . In this category it is also possible to find articles related to pedotransfer functions ( Dobarco et al. , 2019 ) .
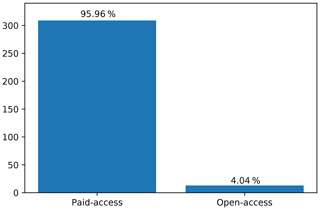
Figure 6 Number of articles published under paid and open access.
These topics are not completely independent and they share some commonalities. For instance, Fig. 9 shows an overlap between Topic 12 (Modelling continuous properties) and 9 (Modelling classes) since both are related to mapping using environmental covariables. Both topics are also related to Topic 3 (Water) since its articles usually have a spatial component. Something similar occurs between Topics 8 (Spectroscopy) and 1 (Remote sensing) since both are related to spectral data.
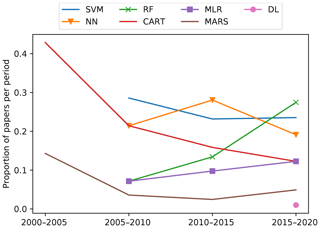
Figure 7 Evolution of model usage in time. SVM: support vector machines; NN: neural networks; RF: random forest; CART: classification and regression trees; MLR: multiple linear regression; MARS: multivariate adaptive regression spline; DL: deep learning
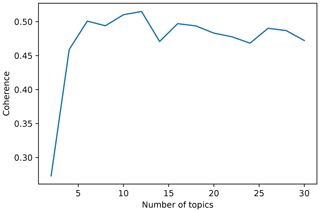
Figure 8 Coherence by number of topics used to train a LDA model.
Besides the shared features between topics, given that LDA is a probabilistic model, articles also contain features related to more than one topic; i.e. they talk about more than one topic (Fig. 10 ). For instance, many of the articles related to SOC are also related to soil modelling and mapping ( Deng et al. , 2018 ; Wang et al. , 2018 b ; Gomes et al. , 2019 ; Keskin et al. , 2019 ) .
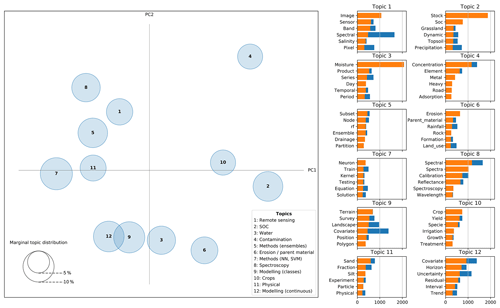
Figure 9 Inter-topic distance map. Dimension reduction via Jensen–Shannon divergence ( Lin , 1991 ) and principal coordinate analysis. Top-6 more relevant words per topic. Complete bars (blue + orange) correspond to overall term frequency and shaded bars (orange) correspond to term frequency within the selected topic.
3.4 Performance of machine learning models
Our review shows that more advanced modelling techniques usually yield better results compared with simpler approaches. In one of the more extensive comparisons, Sirsat et al. ( 2018 ) compared 76 different algorithms, where ensembles of extremely randomised regression trees ranked first when predicting soil fertility indices. Other comparative studies also showed a consistent higher performance of ML methods (NN, SVM, RF) over simpler approaches (principal component regression, partial least squares regression (PLSR), multiple linear regression (MLR), k -nearest neighbours) in applications such as spectroscopy ( Viscarra-Rossel and Behrens , 2010 ; Morellos et al. , 2016 ) and DSM ( Brungard et al. , 2015 ; Taghizadeh-Mehrjardi et al. , 2015 ; Camera et al. , 2017 ; Jeong et al. , 2017 ) . Most studies mention that the superiority of these algorithms is given by their capability to deal with complex non-linearities present in the data. Moreover, the better performance of more advanced ML methods is reported in studies related to the prediction of continuous properties and classes.
Regarding the connection between performance and model usage (Sect. 3.2 ), we observed that some simpler methods such as MLR, despite their lower performance compared to more advanced models, are very popular. This is expected for statistical models since they have a long tradition in science. On the other hand, we also observed a natural tendency of leaving some models behind despite being used for a long time. For instance, PLSR is very popular and has been used since the 1980–1990s but, when used in the studies included in this review (mostly published post the 2000s), very few studies use it as their main algorithm and, instead, it is used in comparative studies where it is outperformed by more advanced models.
It is worth noting that the final performance is not solely dependent on the selected modelling method. Advanced methods like NN have a big number of parameters to fit, especially in the context of deep learning. In order to correctly fit those parameters, from a computational and statistical point of view, the size of the dataset is an essential factor ( Jordan and Mitchell , 2015 ) . Padarian et al. ( 2019 b ) show that a deep CNN trained using a large dataset (around 20 000 soil samples) outperformed methods such as PLS and Cubist when predicting soil properties from spectral data. Using the same method but training on a significantly smaller dataset (390 soil samples), the CNN yielded the worst results.
There is no clear rule on how big a dataset should be, especially because it certainly depends on the complexity of the underlying problem, but the relationship between dataset size and performance has been shown in many studies, using what is usually known as “learning curves” ( Catlett , 1991 ; Shavlik et al. , 1991 ; Cortes et al. , 1994 ; Perlich et al. , 2003 ; Somarathna et al. , 2017 ) . During our review, we observed that the dataset size varied greatly depending on the ML methods (Fig. 11 ).
Considering that ML models could generate a similar solution to a linear model (e.g. a single-rule tree), it should not be a problem to use any method for any dataset size. However, the main difficulty is that training a complex ML model is not a trivial task, especially to avoid overfitting and to obtain a good generalisation, which becomes challenging in the presence of small datasets (i.e. training and test datasets). Even if a researcher can overcome the training process, it is probable that a simpler model can yield similar results.
3.5 Space–time modelling
Compared with the spatial component of soil variation, which is prominent in the topics found using the LDA algorithm (Sect. 3.3 ), the number of studies that address the spatio-temporal dynamics of soil properties using ML methods is still limited. Our findings agree with the review by Grunwald ( 2009 ) , who characterised studies covering the years 2007 and 2008. A big proportion of studies that deal with the temporal dynamics of soil properties are related to soil–water interactions, as shown in Topic 3 of our topic detection analysis (Fig. 9 ).
We found three main approaches to deal with the temporal variation of soil.
The studies generate models for a specific time step, including one or more predictors that vary in time to then apply that fitted model to another time step (e.g. Grinand et al. , 2017 ).
The studies model two or more time steps independently followed by a change analysis. For instance, Schillaci et al. ( 2017 a ) and Zhang et al. ( 2018 b ) subtracted the maps of the modelled properties from 2 different years to compute the change in SOC concentration and pH, respectively.
Studies that model the actual dynamics of a soil property based on some mechanistic or semi-mechanistic method. Stumpf et al. ( 2018 ) created yearly land-use covers for 8500 km 2 in Switzerland using a combination of Landsat 5–7–8 and field land-use observations in order to model the SOC dynamics based on the conversion regimes from their land-use sequence patterns ( Watson et al. , 2014 ) .
Despite there being ML algorithms that have the capacity to capture 4-D structures (e.g. convolutional recurrent neural networks), we did not find studies using ML to continuously model space and time simultaneously. We think the main reason is that soil observations are usually sparse in space–time ( Grunwald , 2016 ) and that it is not possible to fulfill the dataset size requirements of such models. That is the reason why we mostly find studies that use a mechanistic or semi-mechanistic approach.
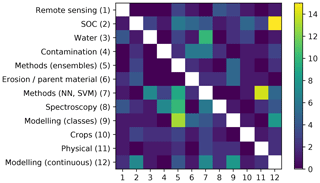
Figure 10 Co-occurrence between the two most likely topics per document. Values correspond to the number of papers.
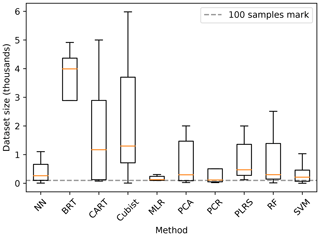
Figure 11 Boxplot of reported dataset sizes grouped by method. Outliers were removed.
3.6 Uncertainty assessment
Uncertainty assessment is an important requirement for any model, especially if the predictions are going to be used to guide decision-making. In this review, 24 % of the studies, among most topics, present uncertainty assessment or mention the importance of considering it (Fig. 12 ).
In this review, a major contributor to the promotion of uncertainty assessment in soil modelling is the GlobalSoilMap project ( Arrouays et al. , 2014 ) which, through specification developed by the DSM scientific community, recommends an uncertainty assessment of all their products. This is evident from Fig. 12 , where topics related to DSM show a relatively high proportion of articles mentioning or reporting uncertainty. In the GlobalSoilMap specifications, the proposed uncertainty assessment method is the use of bootstrapping when training the model ( Stine , 1985 ) , effectively making predictions with many models trained with subsets of the original data to then estimate the 90 % prediction interval (e.g. Castro-Franco et al. , 2017 ; Ma et al. , 2017 ). Another approach is the use of quantile regression ( Koenker and Bassett Jr. , 1978 ) to estimate the complete conditional distribution of the prediction. This method has been recently applied in some DSM studies ( Vaysse and Lagacherie , 2017 ; Sirsat et al. , 2018 ; Cao et al. , 2019 ) . Less common approaches are the use of the fuzzy k -means with the extragrades ( Tranter et al. , 2010 ) algorithm, which defines areas within the covariate space, with different levels of uncertainty, where a new observation (to be predicted) can be placed, and the use of Bayesian optimisation approaches ( Snoek et al. , 2015 ; Gal and Ghahramani , 2016 ) .
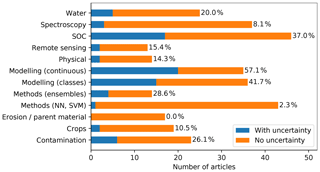
Figure 12 Number of articles per topic that report or mention “uncertainty”. Percentage represents the proportion of total articles per topic.
4.1 Interpretability
Based on our findings, it is possible to state that, in general, ML methods have shown superior performance over more traditional methods in terms of predictive power. We now address the last questions from the aims of this paper — does an advanced model provide new insights that improve our knowledge and understanding of soils?
In order for a human to understand the decisions made by the model, the model has to be interpretable. The motivations for interpretability are varied, including trust, causality, transferability, informativeness, and fairness (in ethical terms) ( Lipton , 2016 ) . In our review, researches usually associate advanced ML models with low interpretability. For instance, Brungard et al. ( 2015 ) assigned multiple models to different groups according to their complexity, with NN and SVM categorised as difficult to interpret compared to MLR or CART. Beguin et al. ( 2017 ) also mention the lower interpretability of ML models compared with an explicit geostatistical model. Because how to measure interpretability is usually not well defined, there are also contradictory opinions. For instance, RF is mostly considered in the category of low interpretability ( Brungard et al. , 2015 ; Were et al. , 2015 ; Taghizadeh-Mehrjardi et al. , 2016 ; Deng et al. , 2018 ) , but its use is also sometimes justified due to its ease of interpretability via the use of variables of importance ( Jeong et al. , 2017 ) .
It is important to clearly define the goal of a modelling exercise. If we want to obtain the model with the greatest accuracy in order to solve a specific problem, maybe interpretability should not be an important factor. If we consider a) that nature is a complex combination of non-linear phenomena and b) the limited capacity of humans to understand non-linear relationships ( Doherty and Balzer , 1988 ) , by requiring our model to have complete transparency we are limiting its capability. However, it is important to corroborate that the model is a valid generalisation of the studied phenomenon. If our goal is to obtain new insights, it is important to consider that interpretability goes hand in hand with prior knowledge and biases and that we could be optimising an algorithm to present misleading but plausible explanations ( Lipton , 2016 ) .
4.1.1 How can we increase interpretability?
A common conclusion reported by authors of the reviewed papers is that the selection of the most informative or relevant predictors before training the model can increase interpretability ( Xiong et al. , 2014 ; Prasad et al. , 2018 ; Wang et al. , 2018 a ; Keskin et al. , 2019 ) , although some authors do not recommend selection of predictors based on the researchers' knowledge since it could lead to biased and suboptimal model performance ( Brungard et al. , 2015 ; Keskin et al. , 2019 ) . This discordance leads to a large range in the number of the predictors used, with some extreme cases using more than 200 ( Xiong et al. , 2014 ; Keskin et al. , 2019 ) .
NN are some of the most performing models but, given the complexity of their operation, they are usually labelled as “black-box” models. In consequence, many authors have focused on trying to provide frameworks to interpret the knowledge extracted by these models. For instance, Bau et al. ( 2017 ) dissected a CNN to understand how different layers work and which features they favour by visualising their (neurons) activation map. Rauber et al. ( 2017 ) used the activation maps projected into a 2-D space in order to visualise and identify confusion zones, outliers, and clusters in the internal representations learned by the model.
In soil sciences, one of the reported methods to interpret ML models is to assess the importance of the variables used, usually derived from the number of times they have been used in the rules generated by tree-like models ( Henderson et al. , 2005 ; Martin et al. , 2014 ; Schillaci et al. , 2017 b ; Khanal et al. , 2018 ) . Another method to assess the relative influence of predictors in tree-like models is to estimate the average reduction of the error at each split of the tree, for all the predictors ( Friedman , 2001 ) . Another alternative, in the context of soil mapping, is to map the rules generated by the model to identify their spatial context or to map where important predictors were used ( Bui et al. , 2006 ) . For CNN, by feeding simulated data to a trained model, Ng et al. ( 2019 ) explored the most important wavelengths used when predicting multiple soil properties from soil spectral data using a sensitivity analysis. The logic behind their analysis is that modifying unimportant wavelengths should not affect the prediction. By plotting the variance for the predictions by wavelengths it is possible to unveil the most important areas of the spectrum (Fig. 13 ).
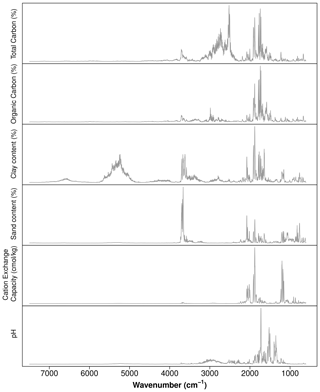
Figure 13 Sensitivity analysis of CNN model prediction as a function of wavelength. Average variance of predictions by wavelength. This analysis allows us to explore the most important wavelengths in a CNN model. Adapted from Ng et al. ( 2019 ) .
Interpretability is an important concept that should be revisited, since it is neither absolute nor static; hence, a specific model cannot be simply labelled as interpretable or not. Linear models can quickly become unintelligible as we add more variables ( Lou et al. , 2012 ) , and methods to better understand complex models such as NN are constantly being developed ( Bau et al. , 2017 ; Montavon et al. , 2018 ; Zhang et al. , 2018 a ) .
4.2 New good practices
Thanks to the effort of some groups to rescue soil legacy data ( Arrouays et al. , 2017 ) and cheaper and faster methods to analyse soil samples, there are more soil data available than ever before. This data availability not only allows us to use new ML algorithms, which usually require more observations, but also opens the door to new ways to train those models. An important part of model development is validation. The literature traditionally recommends that an independent, unseen (by the model) dataset should be used as validation ( Kohavi , 1995 ) . In practice, the data is usually partitioned into training and validation datasets. A more stable solution is the use of k cross-validation where the dataset is partitioned into k groups, where k −1 groups are used for training and 1 groups for validation, repeating the training k times, each with a different validation group. When data availability is a limitation, researchers resort to techniques such as n cross-validation or “leave-one-out” validation to make the most of the available data ( Stevens et al. , 2008 ; Pasini , 2015 ) .
A new generation of models based on NN has been introduced in the later years, which has revolutionised many fields. Deep learning (DL) models, consisting of multiple hidden layers of neurons, have many parameters (from hundreds to millions) which need to be fitted in the training process. This is the reason why they usually need access to large sample sizes. A second characteristic of these models is that they have a considerable number of hyper-parameters. Hyper-parameters are parameters that are not learned from the data during the training phase and include things like the number of iterations during the training, learning rate, layer parameters, or number of layers. A common practice when training DL models is to split the original dataset into three sub-datasets: training, validation, and test. The training dataset is used to learn the parameters, the validation dataset to compare models fitted with different hyper-parameters in order to find the optimal combination, and the test dataset as the independent, unseen data.
In soil sciences, ML algorithms are usually trained using the traditional train–validation split or cross-validation ( Keskin et al. , 2019 ; Liang et al. , 2019 ) , or even no validation ( Feng et al. , 2019 ) , except for some studies based on DL or with an engineering background (e.g. Reale et al. , 2018 ), including some of our publications on the use of DL for DSM ( Padarian et al. , 2019 c ) or soil spectroscopy ( Padarian et al. , 2019 b , a ) , which use a train–validation–test split. Considering the increasing size of datasets, we think soil scientists should transition towards the implementation of some DL practices such as dataset split and hyper-parameter optimisation ( Bergstra and Bengio , 2012 ; Snoek et al. , 2012 ) , not only for NN, but also for any algorithm that has hyper-parameters. Some potential candidates are random forest, Cubist, classification and regression trees, and support vector machines. Most of the implementations of these algorithms have sensible default hyper-parameters, but some studies report an important impact of them in their results ( Mutanga et al. , 2012 ; Lu et al. , 2018 ) . For general hyper-parameter tuning strategies, we refer the reader to Bergstra and Bengio ( 2012 ) for simple strategies such as grid or random search. For an in-depth report of hyper-parameter tuning and its effects in the context of random forest, we refer the reader to Probst et al. ( 2019 ) .
4.3 Commercial ML applications
This work explores the use of ML in soil sciences by exploring the current scientific literature, but use of ML extends beyond research and companies are very welcoming to this technology, especially in applications such as computer vision, speech recognition, natural language processing, and robot control ( Jordan and Mitchell , 2015 ) . It is not hard to imagine a commercial application of approaches such as soil properties prediction using vis-NIR spectroscopy, either in the laboratory or the field. While in research there are some transparency requirements, including describing the methods and data used, companies are usually very secretive about their methods since they are a trade secret that gives them a competitive advantage. Considering that lack of transparency, how can we be sure that the predictions of their models are good? There is not a unique answer, but it should include at least some uncertainty assessment (as discussed in Sect. 3.6 ) and information about the range of soils used during training.
In terms of reporting soil types coverage, different approaches can be applied. A simple, perhaps overconfident method can be to report the geographical extent from where the soil samples used during training were collected (e.g. Tomasella et al. , 2000 ; Børgesen and Schaap , 2005 ) or a broad soil classification based on the soil characteristics such as “sandy soils” (e.g Schaap and Bouten , 1996 and Shaw et al. , 2000 ). A better approach, based on the covariate space of the samples used during training, is fuzzy k -means with extragrades, which has the benefit of describing both coverage and uncertainty levels.
Even if uncertainty levels and coverage are reported, another factor to consider is how much we should trust in companies and their reports. Especially for applications involving public funding, but generally as a consumer protection measure, these type of products should be certifiable in the same way many soil laboratories are. A usual approach is the use of reference materials ( Dybczyński et al. , 1979 ; Pueyo et al. , 2001 ; Ahmed et al. , 2017 ) , which should be consistent with the model coverage reported. The properties measured in the reference materials should fall within the prediction interval produced by the model, with a confidence defined for each application.
Aided by a topic modelling approach, we were able to review the status of ML in soil sciences. We observed a general increase in the adoption of ML methods in time, mostly concentrated in developed countries. This gap is probably due to the link between science, technology, and development. We believe that proper inter-institutional collaboration plans should be put in place in order to close this gap.
By using topic modelling, we identified 12 categories of studies where ML is commonly used, namely remote sensing, soil organic carbon, water, contamination, methods (ensembles), erosion and parent material, methods (NN, SVM), spectroscopy, modelling (classes), crops, physical, and modelling (continuous). The final topic model successfully captured relationships between topics such as modelling of continuous and categorical soil properties, and water, given that all these topics share a spatial component.
We also found that advanced ML methods usually perform better than simpler approaches thanks to their capability to capture non-linear relationships. However, it is important to note that more advanced methods usually require more data and that some precautions should be taken in order to avoid obtaining misleading results. Considering parsimony is always advised, if only a small, simple dataset is available, we recommend using a simple model. This also applies to the number of predictors. In consequence, according to many authors of the reviewed articles, it is better to use meaningful predictors instead of relying on the model capabilities to “select the best variables” in order to improve interpretability.
Interpretability is an important aspect to consider when applying advanced ML methods in order to improve our knowledge and understanding of soil. Simpler methods (e.g. linear models) have been used for a long time and the way of interpreting them is well defined. More advanced methods (e.g. neural networks) are usually considered “black box” models, but that is just a reflection of the current research state and not because it is impossible to interpret them. During our review, we found studies that proposed some solutions to improve their interpretability, and we foresee that a large number of studies will focus on this topic.
Table A1 List of journals by publisher and number of articles that matched the term “soil `machine learning”' in a full-text search.
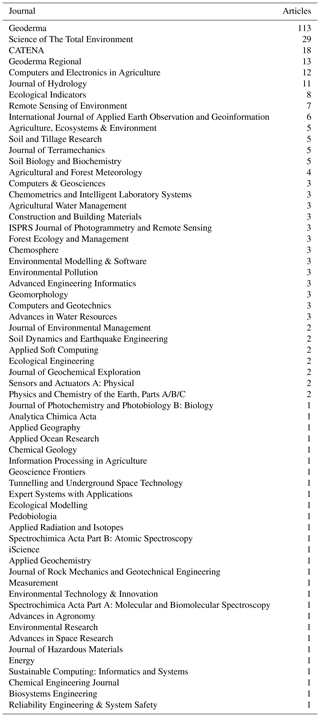
Download Print Version | Download XLSX
Acronyms | |
---|---|
AI | artificial intelligence |
CART | classification and regression tree |
DSM | digital soil mapping |
LDA | latent Dirichlet allocation |
LM | linear model |
MARS | multivariate adaptive regression spline |
ML | machine learning |
MLR | multiple linear regression |
NN | neural network |
PLSR | partial least squares regression |
RF | random forest |
SOC | soil organic carbon |
SVM | support vector machines |
The data are not publicly available due to publisher restriction. A complete list of the publication DOIs used is available from the corresponding author on reasonable request.
JP conceived of and designed the study, performed the modelling and analysis, and wrote the first draft. All the authors contributed to generating and reviewing the subsequent versions of the manuscript.
The authors declare that they have no conflict of interest.
The authors acknowledge the University of Sydney HPC service at the University of Sydney for providing HPC resources that have contributed to the research results reported within this paper.
This paper was edited by Olivier Evrard and reviewed by two anonymous referees.
Ahmad, S., Kalra, A., and Stephen, H.: Estimating soil moisture using remote sensing data: A machine learning approach, Adv. Water Resour., 33, 69–80, 2010. a
Ahmed, O., Habbani, F. I., Mustafa, A., Mohamed, E., Salih, A., and Seedig, F.: Quality assessment statistic evaluation of X-ray fluorescence via NIST and IAEA standard reference materials, World Journal of Nuclear Science and Technology, 7, 121–128, 2017. a
Arrouays, D., Grundy, M. G., Hartemink, A. E., Hempel, J. W., Heuvelink, G. B., Hong, S. Y., Lagacherie, P., Lelyk, G., McBratney, A. B., McKenzie, N., Mendonca-Santos, M. d. L., Minasny, B., Montanarella, L., Odeh, I., Sanchez, P., Thompson, J., and Zhang, G.: GlobalSoilMap: Toward a fine-resolution global grid of soil properties, in: Advances in agronomy, Elsevier, 125, 93–134, 2014. a
Arrouays, D., Leenaars, J., Richer-de-Forges, A., Adhikari, K., Ballabio, C., Greve, M., Grundy, M., Guerrero, E., Hempel, J., Hengl, T., Heuvelink, G., Batjes, N., Carvalho, E., Hartemink, A., Hewitt, A., Hong, S., Krasilnikov, P., Lagacherie, P., Lelyk, G., Libohova, Z., Lilly, A., McBratney, A., McKenzie, N., Vasquez, G., Mulder, V., Minasny, B., Montanarella, L., Odeh, I., Padarian, J., Poggio, L., Roudier, P., Saby, N., Savin, I., Searle, R., Solbovoy, V., Thompson, J., Smith, S., Sulaeman, Y., Vintila, R., Rossel, R., Wilson, P., Zhang, G., Swerts, M., Oorts, K., Karklins, A., Feng, L., Navarro, A., Levin, A., Laktionova, T., Dell'Acqua, M., Suvannang, N., Ruam, W., Prasad, J., Patil, N., Husnjak, S., Pásztor, L., Okx, J., Hallett, S., Keay, C., Farewell, T., Lilja, H., Juilleret, J., Marx, S., Takata, Y., Kazuyuki, Y., Mansuy, N., Panagos, P., Liedekerke, M., Skalsky, R., Sobocka, J., Kobza, J., Eftekhari, K., Alavipanah, S., Moussadek, R., Badraoui, M., Silva, M., Paterson, G., da Gonçalves, M., Theocharopoulos, S., Yemefack, M., Tedou, S., Vrscaj, B., Grob, U., Kozák, J., Boruvka, L., Dobos, E., Taboada, M., Moretti, L., and Rodriguez, D.: Soil legacy data rescue via GlobalSoilMap and other international and national initiatives, Geo. Res. J., 14, 1–19, 2017. a
Bau, D., Zhou, B., Khosla, A., Oliva, A., and Torralba, A.: Network dissection: Quantifying interpretability of deep visual representations, in: Proceedings of the IEEE Conference on Computer Vision and Pattern Recognition, 6541–6549, 2017. a , b
Beguin, J., Fuglstad, G.-A., Mansuy, N., and Paré, D.: Predicting soil properties in the Canadian boreal forest with limited data: Comparison of spatial and non-spatial statistical approaches, Geoderma, 306, 195–205, 2017. a
Bergstra, J. and Bengio, Y.: Random search for hyper-parameter optimization, J. Mach. Learn. Res., 13, 281–305, 2012. a , b
Björk, B.-C.: Open access to scientific articles: a review of benefits and challenges, Int. Emerg. Med., 21, 247–253, 2017. a , b
Blanco, C. M. G., Gomez, V. M. B., Crespo, P., and Ließ, M.: Spatial prediction of soil water retention in a Páramo landscape: Methodological insight into machine learning using random forest, Geoderma, 316, 100–114, 2018. a
Blei, D. M.: Probabilistic topic models, Commun. ACM, 55, 77–84, 2012. a , b , c , d
Blei, D. M., Ng, A. Y., and Jordan, M. I.: Latent dirichlet allocation, J. Mach. Learn. Res., 3, 993–1022, 2003. a , b
Bondi, G., Creamer, R., Ferrari, A., Fenton, O., and Wall, D.: Using machine learning to predict soil bulk density on the basis of visual parameters: Tools for in-field and post-field evaluation, Geoderma, 318, 137–147, 2018. a
Børgesen, C. D. and Schaap, M. G.: Point and parameter pedotransfer functions for water retention predictions for Danish soils, Geoderma, 127, 154–167, 2005. a
Brungard, C. W., Boettinger, J. L., Duniway, M. C., Wills, S. A., and Edwards Jr., T. C.: Machine learning for predicting soil classes in three semi-arid landscapes, Geoderma, 239, 68–83, 2015. a , b , c , d , e
Bui, E. N., Henderson, B. L., and Viergever, K.: Knowledge discovery from models of soil properties developed through data mining, Ecol. Modell., 191, 431–446, 2006. a
Butler, B. M., O'Rourke, S. M., and Hillier, S.: Using rule-based regression models to predict and interpret soil properties from X-ray powder diffraction data, Geoderma, 329, 43–53, https://doi.org/10.1016/j.geoderma.2018.04.005 , 2018. a
Camera, C., Zomeni, Z., Noller, J. S., Zissimos, A. M., Christoforou, I. C., and Bruggeman, A.: A high resolution map of soil types and physical properties for Cyprus: A digital soil mapping optimization, Geoderma, 285, 35–49, 2017. a , b
Cao, B., Domke, G. M., Russell, M. B., and Walters, B. F.: Spatial modeling of litter and soil carbon stocks on forest land in the conterminous United States, Sci. Total Environ., 654, 94–106, 2019. a
Castro-Franco, M., Domenech, M. B., Borda, M. R., and Costa, J.: Spatial dataset of topsoil texture for the southern Argentine Pampas, Geoderma Regional, 12, 18–27, 2017. a
Catlett, J.: Mega induction: A test flight, in: Machine Learning Proceedings 1991, Proceedings of the Eighth International Conference, Evanston, Elsevier, Illinois, 596–599, 1991. a
Caubet, M., Dobarco, M. R., Arrouays, D., Minasny, B., and Saby, N. P.: Merging country, continental and global predictions of soil texture: Lessons from ensemble modelling in France, Geoderma, 337, 99–110, 2019. a
Chlingaryan, A., Sukkarieh, S., and Whelan, B.: Machine learning approaches for crop yield prediction and nitrogen status estimation in precision agriculture: A review, Comput. Electron. Agr., 151, 61–69, 2018. a
Coopersmith, E. J., Minsker, B. S., Wenzel, C. E., and Gilmore, B. J.: Machine learning assessments of soil drying for agricultural planning, Comput. Electron. Agr., 104, 93–104, 2014. a
Cortes, C., Jackel, L. D., Solla, S. A., Vapnik, V., and Denker, J. S.: Learning curves: Asymptotic values and rate of convergence, in: Advances in Neural Information Processing Systems, 327–334, 1994. a
Costa, J. G., Reigosa, M., Matías, J., and Covelo, E.: Soil Cd, Cr, Cu, Ni, Pb and Zn sorption and retention models using SVM: variable selection and competitive model, Sci. Total Environ., 593, 508–522, 2017. a
Dai, F., Zhou, Q., Lv, Z., Wang, X., and Liu, G.: Spatial prediction of soil organic matter content integrating artificial neural network and ordinary kriging in Tibetan Plateau, Ecol. Indic., 45, 184–194, 2014. a
Deng, X., Chen, X., Ma, W., Ren, Z., Zhang, M., Grieneisen, M. L., Long, W., Ni, Z., Zhan, Y., and Lv, X.: Baseline map of organic carbon stock in farmland topsoil in East China, Agr. Ecosyst. Environ., 254, 213–223, 2018. a , b
Dharumarajan, S., Hegde, R., and Singh, S.: Spatial prediction of major soil properties using Random Forest techniques-A case study in semi-arid tropics of South India, Geoderma Regional, 10, 154–162, 2017. a
Dobarco, M. R., Cousin, I., Le Bas, C., and Martin, M. P.: Pedotransfer functions for predicting available water capacity in French soils, their applicability domain and associated uncertainty, Geoderma, 336, 81–95, 2019. a
Doherty, M. E. and Balzer, W. K.: Cognitive feedback, in: Advances in psychology, Elsevier, 54, 163–197, 1988. a
Dybczyński, R., Tugsavul, A., and Suschny, O.: Soil-5, a new IAEA certified reference material for trace element determinations, Geostandard. Newslett., 3, 61–87, 1979. a
Fajardo, M., McBratney, A., and Whelan, B.: Fuzzy clustering of Vis–NIR spectra for the objective recognition of soil morphological horizons in soil profiles, Geoderma, 263, 244–253, 2016. a
Farfani, H. A., Behnamfar, F., and Fathollahi, A.: Dynamic analysis of soil-structure interaction using the neural networks and the support vector machines, Expert Syst. Appl., 42, 8971–8981, 2015. a
Feng, Y., Cui, N., Hao, W., Gao, L., and Gong, D.: Estimation of soil temperature from meteorological data using different machine learning models, Geoderma, 338, 67–77, 2019. a
Flynn, T., Rozanov, A., de Clercq, W., Warr, B., and Clarke, C.: Semi-automatic disaggregation of a national resource inventory into a farm-scale soil depth class map, Geoderma, 337, 1136–1145, 2019. a
Friedman, J. H.: Greedy function approximation: a gradient boosting machine, Ann. Stat., 29, 1189–1232, 2001. a
Gal, Y. and Ghahramani, Z.: Dropout as a bayesian approximation: Representing model uncertainty in deep learning, in: International conference on machine learning, 1050–1059, 2016. a
Gao, M., Li, H.-Y., Liu, D., Tang, J., Chen, X., Chen, X., Blöschl, G., and Leung, L. R.: Identifying the dominant controls on macropore flow velocity in soils: A meta-analysis, J. Hydrol., 567, 590–604, 2018. a
Geissen, V., Kampichler, C., López-de Llergo-Juárez, J., and Galindo-Acántara, A.: Superficial and subterranean soil erosion in Tabasco, tropical Mexico: development of a decision tree modeling approach, Geoderma, 139, 277–287, 2007. a
Gomes, L. C., Faria, R. M., de Souza, E., Veloso, G. V., Schaefer, C. E. G., and Fernandes Filho, E. I.: Modelling and mapping soil organic carbon stocks in Brazil, Geoderma, 340, 337–350, 2019. a
Greifeneder, F., Khamala, E., Sendabo, D., Wagner, W., Zebisch, M., Farah, H., and Notarnicola, C.: Detection of soil moisture anomalies based on Sentinel-1, Phys. Chem. Earth, Pt. A/B/C, 112, 75–82, 2018. a
Grinand, C., Le Maire, G., Vieilledent, G., Razakamanarivo, H., Razafimbelo, T., and Bernoux, M.: Estimating temporal changes in soil carbon stocks at ecoregional scale in Madagascar using remote-sensing, Int. J. Appl. Earth Obs., 54, 1–14, 2017. a
Grunwald, S.: Multi-criteria characterization of recent digital soil mapping and modeling approaches, Geoderma, 152, 195–207, 2009. a
Grunwald, S.: What do we really know about the space–time continuum of soil-landscapes?, in: Environmental Soil-Landscape Modeling, CRC Press, 16–49, 2016. a
Grunwald, S., Vasques, G. M., and Rivero, R. G.: Fusion of soil and remote sensing data to model soil properties, Adv. Agron., Elsevier, 131, 1–109, 2015. a
Han, J., Mao, K., Xu, T., Guo, J., Zuo, Z., and Gao, C.: A soil moisture estimation framework based on the cart algorithm and its application in china, J. Hydrol., 563, 65–75, 2018. a
Hanna, A. M., Ural, D., and Saygili, G.: Neural network model for liquefaction potential in soil deposits using Turkey and Taiwan earthquake data, Soil Dyn. Earthq. Eng., 27, 521–540, 2007. a
Heggemann, T., Welp, G., Amelung, W., Angst, G., Franz, S. O., Koszinski, S., Schmidt, K., and Pätzold, S.: Proximal gamma-ray spectrometry for site-independent in situ prediction of soil texture on ten heterogeneous fields in Germany using support vector machines, Soil Till. Res., 168, 99–109, https://doi.org/10.1016/j.still.2016.10.008 , 2017. a
Henderson, B. L., Bui, E. N., Moran, C. J., and Simon, D.: Australia-wide predictions of soil properties using decision trees, Geoderma, 124, 383–398, 2005. a , b
Hutson, M.: Boycott highlights AI's publishing rebellion, Science, 360, p. 699, 2018. a
Ivushkin, K., Bartholomeus, H., Bregt, A. K., Pulatov, A., Bui, E. N., and Wilford, J.: Soil salinity assessment through satellite thermography for different irrigated and rainfed crops, Int. J. Appl. Earth Obs., 68, 230–237, 2018. a
Jeong, G., Oeverdieck, H., Park, S. J., Huwe, B., and Ließ, M.: Spatial soil nutrients prediction using three supervised learning methods for assessment of land potentials in complex terrain, Catena, 154, 73–84, 2017. a , b
Jordan, M. I. and Mitchell, T. M.: Machine learning: Trends, perspectives, and prospects, Science, 349, 255–260, 2015. a , b , c , d
Karandish, F. and Šimŭnek, J.: A field-modeling study for assessing temporal variations of soil-water-crop interactions under water-saving irrigation strategies, Agr. Water Manage., 178, 291–303, 2016. a
Keskin, H., Grunwald, S., and Harris, W. G.: Digital mapping of soil carbon fractions with machine learning, Geoderma, 339, 40–58, 2019. a , b , c , d , e
Khadim, F. K., Su, H., Xu, L., and Tian, J.: Soil salinity mapping in Everglades National Park using remote sensing techniques and vegetation salt tolerance, Phys. Chem. Earth, Pt. A/B/C, 110, 31–50, 2019. a
Khanal, S., Fulton, J., Klopfenstein, A., Douridas, N., and Shearer, S.: Integration of high resolution remotely sensed data and machine learning techniques for spatial prediction of soil properties and corn yield, Comput. Electron. Agr., 153, 213–225, 2018. a , b
Kheir, R. B., Chorowicz, J., Abdallah, C., and Dhont, D.: Soil and bedrock distribution estimated from gully form and frequency: A GIS-based decision-tree model for Lebanon, Geomorphology, 93, 482–492, https://doi.org/10.1016/j.geomorph.2007.03.010 , 2008. a
Koenker, R. and Bassett Jr., G.: Regression quantiles, Econometrica: journal of the Econometric Society, 33–50, 1978. a
Koestel, J. and Jorda, H.: What determines the strength of preferential transport in undisturbed soil under steady-state flow?, Geoderma, 217, 144–160, 2014. a
Kohavi, R.: A study of cross-validation and bootstrap for accuracy estimation and model selection, in: Ijcai, Montreal, Canada, 14, 1137–1145, 1995. a
Kovačević, M., Bajat, B., and Gajić, B.: Soil type classification and estimation of soil properties using support vector machines, Geoderma, 154, 340–347, 2010. a
Lacoste, M., Lemercier, B., and Walter, C.: Regional mapping of soil parent material by machine learning based on point data, Geomorphology, 133, 90–99, https://doi.org/10.1016/j.geomorph.2011.06.026 , 2011. a
Leenaars, J. G., Claessens, L., Heuvelink, G. B., Hengl, T., González, M. R., van Bussel, L. G., Guilpart, N., Yang, H., and Cassman, K. G.: Mapping rootable depth and root zone plant-available water holding capacity of the soil of sub-Saharan Africa, Geoderma, 324, 18–36, 2018. a
Liang, Z., Chen, S., Yang, Y., Zhao, R., Shi, Z., and Rossel, R. A. V.: National digital soil map of organic matter in topsoil and its associated uncertainty in 1980's China, Geoderma, 335, 47–56, 2019. a
Lin, J.: Divergence measures based on the Shannon entropy, IEEE T. Inform. Theory, 37, 145–151, 1991. a
Lipton, Z. C.: The mythos of model interpretability, arXiv preprint arXiv:1606.03490, 2016. a , b
Liu, S., Yang, Y., Shen, H., Hu, H., Zhao, X., Li, H., Liu, T., and Fang, J.: No significant changes in topsoil carbon in the grasslands of northern China between the 1980s and 2000s, Sci. Total Environ., 624, 1478–1487, 2018. a
Lou, Y., Caruana, R., and Gehrke, J.: Intelligible models for classification and regression, in: Proceedings of the 18th ACM SIGKDD international conference on Knowledge discovery and data mining, ACM, 150–158, 2012. a
Lu, W., Lu, D., Wang, G., Wu, J., Huang, J., and Li, G.: Examining soil organic carbon distribution and dynamic change in a hickory plantation region with Landsat and ancillary data, Catena, 165, 576–589, 2018. a
Ma, Y., Minasny, B., and Wu, C.: Mapping key soil properties to support agricultural production in Eastern China, Geoderma Regional, 10, 144–153, 2017. a
Ma, Y., Minasny, B., Malone, B. P., and Mcbratney, A. B.: Pedology and digital soil mapping (DSM), Europ. J. Soil Sci., 70, 216–235, 2019. a
Mansuy, N., Thiffault, E., Paré, D., Bernier, P., Guindon, L., Villemaire, P., Poirier, V., and Beaudoin, A.: Digital mapping of soil properties in Canadian managed forests at 250 m of resolution using the k-nearest neighbor method, Geoderma, 235, 59–73, 2014. a
Märker, M., Pelacani, S., and Schröder, B.: A functional entity approach to predict soil erosion processes in a small Plio-Pleistocene Mediterranean catchment in Northern Chianti, Italy, Geomorphology, 125, 530–540, 2011. a
Martin, M., Orton, T., Lacarce, E., Meersmans, J., Saby, N., Paroissien, J., Jolivet, C., Boulonne, L., and Arrouays, D.: Evaluation of modelling approaches for predicting the spatial distribution of soil organic carbon stocks at the national scale, Geoderma, 223, 97–107, 2014. a
Martinez, G., Weltz, M., Pierson, F. B., Spaeth, K. E., and Pachepsky, Y.: Scale effects on runoff and soil erosion in rangelands: Observations and estimations with predictors of different availability, Catena, 151, 161–173, 2017. a
Massawe, B. H., Subburayalu, S. K., Kaaya, A. K., Winowiecki, L., and Slater, B. K.: Mapping numerically classified soil taxa in Kilombero Valley, Tanzania using machine learning, Geoderma, 311, 143–148, 2018. a
Matthew and Honnibal, M. I.: spaCy 2: Natural language understanding with Bloom embeddings, convolutional neural networks and incremental parsing, https://github.com/explosion/spaCy/ (last access: 5 February 2020), 2017. a
McBratney, A., de Gruijter, J., and Bryce, A.: Pedometrics timeline, Geoderma, 338, 568–575, 2019. a
McCallum, A. K.: MALLET: A Machine Learning for Language Toolkit, http://mallet.cs.umass.edu (last access: 5 February 2020), 2002. a
Minasny, B. and Flantis, D.: “Helicopter research”: who benefits from international studies in Indonesia?, https://theconversation.com/helicopter-research-who-benefits-from-international-studies-in-indonesia-102165 (last access: 29 April 2019), 2018. a
Mjolsness, E. and DeCoste, D.: Machine learning for science: state of the art and future prospects, Science, 293, 2051–2055, 2001. a
Montavon, G., Samek, W., and Müller, K.-R.: Methods for interpreting and understanding deep neural networks, Digit. Signal Process., 73, 1–15, 2018. a
Morellos, A., Pantazi, X.-E., Moshou, D., Alexandridis, T., Whetton, R., Tziotzios, G., Wiebensohn, J., Bill, R., and Mouazen, A. M.: Machine learning based prediction of soil total nitrogen, organic carbon and moisture content by using VIS-NIR spectroscopy, Biosyst. Eng., 152, 104–116, 2016. a
Mutanga, O., Adam, E., and Cho, M. A.: High density biomass estimation for wetland vegetation using WorldView-2 imagery and random forest regression algorithm, Int. J. Appl. Earth Obs., 18, 399–406, 2012. a
Naderi-Boldaji, M., Tekeste, M. Z., Nordstorm, R. A., Barnard, D. J., and Birrel, S. J.: A mechanical-dielectric-high frequency acoustic sensor fusion for soil physical characterization, Comput. Electron. Agr., 156, 10–23, 2019. a
Ng, W., Minasny, B., Montazerolghaem, M., Padarian, J., Ferguson, R., Bailey, S., and McBratney, A. B.: Convolutional neural network for simultaneous prediction of several soil properties using visible/near-infrared, mid-infrared, and their combined spectra, Geoderma, 352, 251-267, 2019. a , b
Oh, Y.-Y., Yun, S.-T., Yu, S., Kim, H.-J., and Jun, S.-C.: A novel wavelet-based approach to characterize dynamic environmental factors controlling short-term soil surface CO 2 flux: Application to a controlled CO2 release test site (EIT) in South Korea, Geoderma, 337, 76–90, 2019. a
Padarian, J., Minasny, B., and McBratney, A.: Transfer learning to localise a continental soil vis-NIR calibration model, Geoderma, 340, 279–288, 2019a. a , b
Padarian, J., Minasny, B., and McBratney, A.: Using deep learning to predict soil properties from regional spectral data, Geoderma Regional, 16, e00198, https://doi.org/10.1016/j.geodrs.2018.e00198 , 2019b. a , b
Padarian, J., Minasny, B., and McBratney, A. B.: Using deep learning for digital soil mapping, Soil, 5, 79–89, 2019c. a
Pasini, A.: Artificial neural networks for small dataset analysis, J. Thoracic Dis., 7, 953–960, 2015. a
Perlich, C., Provost, F., and Simonoff, J. S.: Tree induction vs. logistic regression: A learning-curve analysis, J. Mach. Learn. Res., 4, 211–255, 2003. a
Poggio, L., Gimona, A., Spezia, L., and Brewer, M. J.: Bayesian spatial modelling of soil properties and their uncertainty: The example of soil organic matter in Scotland using R-INLA, Geoderma, 277, 69–82, 2016. a
Prasad, R., Deo, R. C., Li, Y., and Maraseni, T.: Ensemble committee-based data intelligent approach for generating soil moisture forecasts with multivariate hydro-meteorological predictors, Soil Till. Res., 181, 63–81, 2018. a
Probst, P., Wright, M. N., and Boulesteix, A.-L.: Hyperparameters and tuning strategies for random forest, WIRES Data Min. Knowl., 9, e1301, https://doi.org/10.1002/widm.1301 , 2019. a
Pueyo, M., Rauret, G., Bacon, J., Gomez, A., Muntau, H., Quevauviller, P., and López-Sánchez, J.: A new organic-rich soil reference material certified for its EDTA-and acetic acid-extractable contents of Cd, Cr, Cu, Ni, Pb and Zn, following collaboratively tested and harmonised procedures, J. Environ. Monit., 3, 238–242, 2001. a
Rauber, P. E., Fadel, S. G., Falcao, A. X., and Telea, A. C.: Visualizing the hidden activity of artificial neural networks, IEEE T. Vis. Comput. Gr., 23, 101–110, 2017. a
Reale, C., Gavin, K., Librić, L., and Jurić-Kaćunić, D.: Automatic classification of fine-grained soils using CPT measurements and Artificial Neural Networks, Adv. Eng. Inform., 36, 207–215, 2018. a
Reeves, M. K., Perdue, M., Munk, L. A., and Hagedorn, B.: Predicting risk of trace element pollution from municipal roads using site-specific soil samples and remotely sensed data, Sci. Total Environ., 630, 578–586, 2018. a
Rial, M., Cortizas, A. M., Taboada, T., and Rodríguez-Lado, L.: Soil organic carbon stocks in Santa Cruz Island, Galapagos, under different climate change scenarios, Catena, 156, 74–81, 2017. a
Röder, M., Both, A., and Hinneburg, A.: Exploring the space of topic coherence measures, in: Proceedings of the eighth ACM international conference on Web search and data mining, ACM, 399–408, 2015. a
Rossiter, D. G.: Past, present & future of information technology in pedometrics, Geoderma, 324, 131–137, 2018. a , b , c
Rudin, C. and Wagstaff, K. L.: Machine learning for science and society, Mach. Learn., 95, 1–9, 2014. a
Sagasti, F. R.: Underdevelopment, science and technology: the point of view of the underdeveloped countries, Sci. Stud., 3, 47–59, 1973. a
Schaap, M. G. and Bouten, W.: Modeling water retention curves of sandy soils using neural networks, Water Resour. Res., 32, 3033–3040, 1996. a
Schillaci, C., Acutis, M., Lombardo, L., Lipani, A., Fantappie, M., Märker, M., and Saia, S.: Spatio-temporal topsoil organic carbon mapping of a semi-arid Mediterranean region: The role of land use, soil texture, topographic indices and the influence of remote sensing data to modelling, Sci. Total Environ., 601, 821–832, 2017a. a
Schillaci, C., Lombardo, L., Saia, S., Fantappiè, M., Märker, M., and Acutis, M.: Modelling the topsoil carbon stock of agricultural lands with the Stochastic Gradient Treeboost in a semi-arid Mediterranean region, Geoderma, 286, 35–45, 2017b. a
Shavlik, J. W., Mooney, R. J., and Towell, G. G.: Symbolic and neural learning algorithms: An experimental comparison, Mach. Learn., 6, 111–143, 1991. a
Shaw, J., West, L., Radcliffe, D., and Bosch, D.: Preferential flow and pedotransfer functions for transport properties in sandy Kandiudults, Soil Sci. Soc. Am. J., 64, 670–678, 2000. a
Sirsat, M., Cernadas, E., Fernández-Delgado, M., and Barro, S.: Automatic prediction of village-wise soil fertility for several nutrients in India using a wide range of regression methods, Comput. Electron. Agr., 154, 120–133, 2018. a , b
Snoek, J., Larochelle, H., and Adams, R. P.: Practical bayesian optimization of machine learning algorithms, Adv. Neur. In., 25, 2951–2959, 2012. a
Snoek, J., Rippel, O., Swersky, K., Kiros, R., Satish, N., Sundaram, N., Patwary, M., Prabhat, M., and Adams, R.: Scalable bayesian optimization using deep neural networks, Proceedings of the 32nd International Conference on Machine Learning, Int. Conf. Mach. Learn., 2171–2180, 2015. a
Somarathna, P., Minasny, B., and Malone, B. P.: More data or a better model? Figuring out what matters most for the spatial prediction of soil carbon, Soil Sci. Soc. Am. J., 81, 1413–1426, 2017. a
Song, X.-D., Yang, F., Ju, B., Li, D.-C., Zhao, Y.-G., Yang, J.-L., and Zhang, G.-L.: The influence of the conversion of grassland to cropland on changes in soil organic carbon and total nitrogen stocks in the Songnen Plain of Northeast China, Catena, 171, 588–601, 2018. a
Sonnenwald, D. H.: Scientific collaboration, Ann. Rev. Inf. Sci. Technol., 41, 643–681, 2007. a , b
Stevens, A., van Wesemael, B., Bartholomeus, H., Rosillon, D., Tychon, B., and Ben-Dor, E.: Laboratory, field and airborne spectroscopy for monitoring organic carbon content in agricultural soils, Geoderma, 144, 395–404, 2008. a
Stevens, K., Kegelmeyer, P., Andrzejewski, D., and Buttler, D.: Exploring topic coherence over many models and many topics, in: Proceedings of the 2012 Joint Conference on Empirical Methods in Natural Language Processing and Computational Natural Language Learning, Association for Computational Linguistics, 952–961, 2012. a , b
Stine, R. A.: Bootstrap prediction intervals for regression, J. Am. Stat. Assoc., 80, 1026–1031, 1985. a
Stumpf, F., Keller, A., Schmidt, K., Mayr, A., Gubler, A., and Schaepman, M.: Spatio-temporal land use d ynamics and soil organic carbon in Swiss agroecosystems, Agr. Ecosyst. Environ., 258, 129–142, 2018. a
Subburayalu, S., Jenhani, I., and Slater, B.: Disaggregation of component soil series on an Ohio County soil survey map using possibilistic decision trees, Geoderma, 213, 334–345, 2014. a
Sugimoto, C. R., Li, D., Russell, T. G., Finlay, S. C., and Ding, Y.: The shifting sands of disciplinary development: Analyzing North American Library and Information Science dissertations using latent Dirichlet allocation, J. Am. Soc. Inf. Sci. Tech., 62, 185–204, 2011. a
Taghizadeh-Mehrjardi, R., Nabiollahi, K., Minasny, B., and Triantafilis, J.: Comparing data mining classifiers to predict spatial distribution of USDA-family soil groups in Baneh region, Iran, Geoderma, 253, 67–77, 2015. a
Taghizadeh-Mehrjardi, R., Nabiollahi, K., and Kerry, R.: Digital mapping of soil organic carbon at multiple depths using different data mining techniques in Baneh region, Iran, Geoderma, 266, 98–110, 2016. a
Tomasella, J., Hodnett, M. G., and Rossato, L.: Pedotransfer Functions for the Estimation of Soil Water Retention in Brazilian Soils, Soil Sci. Soc. Am. J., 64, 327–338, 2000. a
Tranter, G., Minasny, B., and McBratney, A.: Estimating Pedotransfer Function Prediction Limits Using Fuzzy k-Means with Extragrades, Soil Sci. Soc. Am. J., 74, 1967–1975, 2010. a
Tziachris, P., Aschonitis, V., Chatzistathis, T., and Papadopoulou, M.: Assessment of spatial hybrid methods for predicting soil organic matter using DEM derivatives and soil parameters, Catena, 174, 206–216, 2019. a
Vaysse, K. and Lagacherie, P.: Using quantile regression forest to estimate uncertainty of digital soil mapping products, Geoderma, 291, 55–64, 2017. a
Vincent, S., Lemercier, B., Berthier, L., and Walter, C.: Spatial disaggregation of complex Soil Map Units at the regional scale based on soil-landscape relationships, Geoderma, 311, 130–142, 2018. a
Viscarra-Rossel, R. and Behrens, T.: Using data mining to model and interpret soil diffuse reflectance spectra, Geoderma, 158, 46–54, 2010. a
Řehůřek, R. and Sojka, P.: Software Framework for Topic Modelling with Large Corpora, in: Proceedings of the LREC 2010 Workshop on New Challenges for NLP Frameworks, ELRA, Valletta, Malta, 45–50, http://is.muni.cz/publication/884893/en (last access: 5 February 2020), 2010. a
Wang, B., Waters, C., Orgill, S., Cowie, A., Clark, A., Li Liu, D., Simpson, M., McGowen, I., and Sides, T.: Estimating soil organic carbon stocks using different modelling techniques in the semi-arid rangelands of eastern Australia, Ecol. Indic., 88, 425–438, 2018a. a , b
Wang, B., Waters, C., Orgill, S., Gray, J., Cowie, A., Clark, A., and Li Liu, D.: High resolution mapping of soil organic carbon stocks using remote sensing variables in the semi-arid rangelands of eastern Australia, Sci. Total Environ., 630, 367–378, 2018b. a
Ware, M. and Mabe, M.: The STM report: An overview of scientific and scholarly journal publishing, available at: https://www.stm-assoc.org/2015_02_20_STM_Report_2015.pdf (last access: 5 February 2020), 2015. a
Warner, D. L., Guevara, M., Inamdar, S., and Vargas, R.: Upscaling soil-atmosphere CO 2 and CH 4 fluxes across a topographically complex forested landscape, Agr. Forest Meteorol., 264, 80–91, 2019. a
Watson, S. J., Luck, G. W., Spooner, P. G., and Watson, D. M.: Land-use change: incorporating the frequency, sequence, time span, and magnitude of changes into ecological research, Front. Ecol. Environ., 12, 241–249, 2014. a
Were, K., Bui, D. T., Dick, Ø. B., and Singh, B. R.: A comparative assessment of support vector regression, artificial neural networks, and random forests for predicting and mapping soil organic carbon stocks across an Afromontane landscape, Ecol. Ind., 52, 394–403, 2015. a
Wu, G., Kechavarzi, C., Li, X., Wu, S., Pollard, S. J., Sui, H., and Coulon, F.: Machine learning models for predicting PAHs bioavailability in compost amended soils, Chem. Engin. J., 223, 747–754, 2013. a
Wu, Q., Zhang, C., Hong, Q., and Chen, L.: Topic evolution based on LDA and HMM and its application in stem cell research, J. Inf. Sci., 40, 611–620, 2014. a
Xie, X.-L. and Li, A.-B.: Identification of soil profile classes using depth-weighted visible-near-infrared spectral reflectance, Geoderma, 325, 90–101, https://doi.org/10.1016/j.geoderma.2018.03.029 , 2018. a
Xing, L., Li, L., Gong, J., Ren, C., Liu, J., and Chen, H.: Daily soil temperatures predictions for various climates in United States using data-driven model, Energy, 160, 430–440, 2018. a
Xiong, X., Grunwald, S., Myers, D. B., Kim, J., Harris, W. G., and Comerford, N. B.: Holistic environmental soil-landscape modeling of soil organic carbon, Environ. Modell. Softw., 57, 202–215, 2014. a , b
Xu, Y., Smith, S. E., Grunwald, S., Abd-Elrahman, A., and Wani, S. P.: Incorporation of satellite remote sensing pan-sharpened imagery into digital soil prediction and mapping models to characterize soil property variability in small agricultural fields, ISPRS J Photogramm., 123, 1–19, 2017. a
Zeynoddin, M., Bonakdari, H., Ebtehaj, I., Esmaeilbeiki, F., Gharabaghi, B., and Haghi, D. Z.: A reliable linear stochastic daily soil temperature forecast model, Soil Till. Res., 189, 73–87, 2019. a
Zhang, C., Mishra, D. R., and Pennings, S. C.: Mapping salt marsh soil properties using imaging spectroscopy, ISPRS J. Photogramm., 148, 221–234, 2019. a
Zhang, Q., Nian Wu, Y., and Zhu, S.-C.: Interpretable convolutional neural networks, in: Proceedings of the IEEE Conference on Computer Vision and Pattern Recognition, 8827–8836, 2018a. a
Zhang, Y., Sui, B., Shen, H., and Wang, Z.: Estimating temporal changes in soil pH in the black soil region of Northeast China using remote sensing, Comput. Electron. Agr., 154, 204–212, 2018b. a , b
Zhou, D., Ji, X., Zha, H., and Giles, C. L.: Topic evolution and social interactions: how authors effect research, in: Proceedings of the 15th ACM international conference on Information and knowledge management, ACM, 248–257, 2006. a
- Introduction
- Results and discussion
- General discussion
- Conclusions and recommendations
- Data availability
- Author contributions
- Competing interests
- Acknowledgements
- Review statement

An official website of the United States government
The .gov means it’s official. Federal government websites often end in .gov or .mil. Before sharing sensitive information, make sure you’re on a federal government site.
The site is secure. The https:// ensures that you are connecting to the official website and that any information you provide is encrypted and transmitted securely.
- Publications
- Account settings
Preview improvements coming to the PMC website in October 2024. Learn More or Try it out now .
- Advanced Search
- Journal List
- Elsevier - PMC COVID-19 Collection

Sustainable soil use and management: An interdisciplinary and systematic approach
a School of Environment, Tsinghua University, Beijing 100084, China

Nanthi S. Bolan
b Global Centre for Environmental Remediation, The University of Newcastle, Callaghan, NSW 2308, Australia
Daniel C.W. Tsang
c Department of Civil and Environmental Engineering, The Hong Kong Polytechnic University, Hung Hom, Kowloon, Hong Kong, China
Mary B. Kirkham
d Department of Agronomy, Throckmorton Plant Sciences Center, Kansas State University, Manhattan, KS, United States
David O'Connor
Soil is a key component of Earth's critical zone. It provides essential services for agricultural production, plant growth, animal habitation, biodiversity, carbon sequestration and environmental quality, which are crucial for achieving the United Nations' Sustainable Development Goals (SDGs). However, soil degradation has occurred in many places throughout the world due to factors such as soil pollution, erosion, salinization, and acidification. In order to achieve the SDGs by the target date of 2030, soils may need to be used and managed in a manner that is more sustainable than is currently practiced. Here we show that research in the field of sustainable soil use and management should prioritize the multifunctional value of soil health and address interdisciplinary linkages with major issues such as biodiversity and climate change. As soil is the largest terrestrial carbon pool, as well as a significant contributor of greenhouse gases, much progress can be made toward curtailing the climate crisis by sustainable soil management practices. One identified option is to increase soil organic carbon levels, especially with recalcitrant forms of carbon (e.g., biochar application). In general, soil health is primarily determined by the actions of the farming community. Therefore, information management and knowledge sharing are necessary to improve the sustainable behavior of practitioners and end-users. Scientists and policy makers are important actors in this social learning process, not only to disseminate evidence-based scientific knowledge, but also in generating new knowledge in close collaboration with farmers. While governmental funding for soil data collection has been generally decreasing, newly available 5G telecommunications, big data and machine learning based data collection and analytical tools are maturing. Interdisciplinary studies that incorporate such advances may lead to the formation of innovative sustainable soil use and management strategies that are aimed toward optimizing soil health and achieving the SDGs.
Graphical abstract

- • Soil degradation impedes achieving the United Nations' Sustainable Development Goals.
- • Soil plays a fundamental role for biodiversity conservation.
- • Soil researchers ought to prioritize the multifunctional value of soil health.
- • A framework for interdisciplinary research in soil sustainability is presented.
- • Information management and knowledge sharing may drive sustainable behavior change.
1. Introduction
Soil, commonly viewed as a non-renewable resource due to the extremely slow pace of its regeneration, is under serious threat from modern society ( Amundson et al., 2015 ). Soil degradation occurs due to factors such as water erosion, wind erosion, salinization, and deforestation ( Carlson et al., 2012 ; Celentano et al., 2017 ; Rojas et al., 2016 ). Activities that introduce polluting substances, such as heavy metals, pesticides, polycyclic aromatic hydrocarbons (PAHs), are further causing wide-spread soil degradation. Globally, it is estimated that ~24 billion metric tons of soil are lost through factors such as erosion each year ( UNCCD, 2017 ) and that ~30% of the world's soils are now in a degraded state ( FAO, 2011 ). In China, ~19% of agricultural soil and ~ 16% of all soils exceed national soil quality standards ( MEP, 2014 ). Soil degradation threatens the realization of the United Nations Sustainable Development Goals (SDGs) ( Bouma, 2019 ). To help address soil degradation, the United Nations Food and Agriculture Organization declared 2015–2024 as the International Decade of Soils, aiming to raise public awareness of soil protection. Since then, there has been a burgeoning trend of scientific literature and public debate on soil.
Soil is primarily viewed as a critical component of agricultural production in traditional wisdom. In more recent years, the scientific community has increasingly recognized that soil is also an essential component for environmental protection ( Obrist et al., 2017 ), climate change mitigation ( Le Quere et al., 2018 ), ecosystem services ( Bahram et al., 2018 ), as well as land use and planning ( Gossner et al., 2016 ). There is also a growing recognition that soil health relates not only to the classical biogeophysical processes that are traditionally studied by soil scientists, but also information management, knowledge sharing, and human behavior ( Bampa et al., 2019 ; Bouma et al., 2019 ). Interdisciplinary studies (see Section 2.3 ) are required to understand better the coupling of complex human-nature systems linked to soil management ( Bouma and Montanarella, 2016 ). However, current knowledge on soil processes is scattered across various disciplines, lacking comprehensive views on the sustainable management of soil resources ( Vogel et al., 2018 ).
In 2015, the United Nations General Assembly established 17 goals to be achieved by 2030, which are named the Sustainable Development Goals (SDGs). These include, among others, no poverty, zero hunger, good health and wellbeing, clean water and sanitation and climate action ( UN, 2015 ). The SDGs have become a central theme of global development and international collaboration. Considerable progress has been made in recent years toward reaching the SDGs. For example, the proportion of the global population with access to safe drinking water and the percentage of children receiving vaccinations have both risen considerably. However, many challenges still exist, such as: 821 million people remain undernourished, representing a 5% increase between 2015 and 2017; investment in agriculture from governmental sources and foreign aid has dropped; and, atmospheric concentrations of CO 2 and other greenhouse gases (GHGs) continue to rise ( UN, 2019 ), exacerbating the current climate crisis. Governments from local to national levels need to develop integrated programs addressing these sustainability challenges ( Bryan et al., 2018 ).
In the ongoing actions toward reaching the United Nations SDGs, the soil science community has somewhat underplayed the potential role it could play, partly due to the scattered nature of soil knowledge mentioned above. If researchers from wider disciplines were to collaborate more with soil scientists, it may help progress approaches to achieving the SDGs in a manner more effective than acting alone. Therefore, the profile of the soil science discipline may need to be raised, especially the interdisciplinary components that support food security, climate change mitigation, biodiversity, and public health, in order to better design comprehensive strategies toward realizing the SDGs.
In the present paper, we do not reiterate the importance of the interaction between soil science and agronomy covering crop productivity, which has been discussed in other existing publications ( Sanchez, 2002 ; Tisdale et al., 1985 ). Instead, we focus on the interdisciplinary nature of soil and sustainable soil use and management and linkages with soil science with social science, climate science, ecological science, and environmental science.
2. The interdisciplinary nature of sustainable soil use and management
2.1. sustainable development goals (sdgs).
Soil plays a pivotal role in the United Nations SDGs, most notably SDGs 2, 3, 6, 12, 13, and 15 ( Bouma and Montanarella, 2016 ; Keesstra et al., 2016 ). Most people in poverty live in rural areas where crop production is a vital source of income. In these areas, soil health is a decisive factor for productivity and income levels. Among other roles, soil provides the basis for food production and ecosystem services ( Bender et al., 2016 ; Oliver and Gregory, 2015 ). Moreover, as soil biodiversity is related to lower crop diseases and pests, the ecological services offered by healthy soil systems are important in reducing poverty and ending hunger. Soil also affects water quality, GHG emissions, and other important environmental considerations in regard to the SDGs ( Bharati et al., 2002 ; Franzluebbers, 2005 ). An overview of the identified relationships between soil and the relevant SDGs are illustrated in Fig. 1 .

The relevance of soil to the United Nations' Sustainable Development Goals (SDGs).
It is imperative to disseminate soil science knowledge to policy makers and practitioners who design and implement SDG programs (see Section 3 ). Effective action needs to be taken by the soil science community to help develop suitable indicators that are not only scientifically sound, but also practical for small hold farmers and other stakeholders. Scientific research needs to be specifically directed toward realizing the SDGs, rather than to just understand soil science. The influence of human behavior must be factored into this complex human-nature system. It is also necessary to include the impacts of socio-economic activity on soil health when carrying out sustainability assessments, thus allowing more informed decision making ( Vogel et al., 2018 ).
2.2. The soil health concept
Soils have a wide range of physical, chemical, and biological properties that are attributable to the parent material (e.g., geologic origin and depositional processes), environmental factors (e.g., climate conditions, topography) as well as anthropogenic influences (e.g. farming practice, surface disturbance, pollutant emissions). Because soil plays such a critical role in multiple natural and anthropogenic systems, such soil properties will affect ecosystem services, environmental quality, agricultural sustainability, climate change, and human health. This multi-functional aspect makes traditional soil quality evaluation systems, which have tended to focus on soil fertility and agricultural production ( Doran and Parkin, 1994 ), no longer fully appropriate. Most recently, the “soil health” concept has been the subject of increasing research attention (see Fig. 2 ). This holistic approach accounts for non-linear mechanistic relationships between various physical, chemical, and biological properties. Moreover, the soil health holistic concept is advantageous over traditional soil quality assessments because it considers ecosystem services as well as agricultural production, i.e., both nature and human driven objectives ( Kibblewhite et al., 2008 ).

Number of research articles listed in the Web of Science database ( www.webofknowledge.com ) when soil AND sustainability and “soil health” were searched as topics (searched on 3rd March 2020).
Doran and Zeiss (2000) defined soil health as “the capacity of soil to function as a vital living system, within ecosystem and land-use boundaries, to sustain plant and animal productivity, maintain or enhance water and air quality, and promote plant and animal health” Their definition has been well received by the scientific community, as evidenced by the article being cited ~1500 times according to Google Scholar. The authors argued that soil health is a holistic concept which portrays soil as a living system (i.e., the capacity of soil to function as a living system), while soil quality describes a soil's capacity for a specific use (i.e., fitness for different uses). The outcomes of soil use and management decisions are reflected in soil health ( Doran and Safley, 1997 ).
Assessing soil health involves the selection of indicators, quantification or qualitative scoring, and providing a final index with appropriate weighting and integration ( Rinot et al., 2019 ). Biophysical indicators are particularly relevant for assessing soil health. This is because healthy soil is manifested through a variety of soil functions that are reliant upon biological processes, e.g. carbon transformation, nutrient cycling, maintaining soil structure, and regulating pests and disease ( Kibblewhite et al., 2008 ). Scientists have explored the use of soil microorganisms ( Nielsen et al., 2002 ; Van Bruggen and Semenov, 2000 ), enzyme activities ( Ananbeh et al., 2019 ; Janvier et al., 2007 ), earthworms and nematodes ( Neher, 2001 ), as well as other biological indicators to assess soil health. Similarly, soil structure, compaction and moisture retention have been used as physical indicators of soil health.
2.3. Interdisciplinary research
The sustainability of soil systems is affected by their bio-physico-chemical properties, and the soil use and management decisions made by farmers ( Doran and Zeiss, 2000 ). These two aspects can be broadly categorized into natural and anthropogenic processes. Complex dynamics are involved in the coupled human-nature systems, rendering many challenges for the study of soil systems from any single disciplinary lens. We must develop an interdisciplinary approach to address these challenges ( Totsche et al., 2010 ). It should be noted that interdisciplinary approaches differ from multidisciplinary approaches, in that they integrate insights on a common problem (e.g. climate change) from different disciplines (e.g. soil science and climate science) to construct a comprehensive understanding of the issue. In comparison, multidisciplinary approaches involve gaining separate insights on a common problem from the perspectives of different disciplines ( Repko and Szostak, 2020 ).
As many of the problems surrounding soil sustainability are complex and broad, they cannot be sufficiently addressed by one single discipline, thus interdisciplinary studies are needed ( Klein and Newell, 1997 ). Based on a published framework that interconnected disciplinary lines for another topic ( Hammond and Dubé, 2012 ), here we propose a general framework for developing an interdisciplinary perspective on sustainable soil use and management ( Fig. 3 ). We propose that five broad issues have a root in soil science and are linked to at least one other discipline. The issues themselves are also interconnected. Take management and behavior as an example, which is directly linked to soil science and social science. At the same time, soil fertility and soil pollution are also involved, which are directly linked to agronomy and environmental science, respectively. Another example is soil carbon (or soil organic matter) which is directly linked to both soil science and climate science while also affecting soil biodiversity linked to ecology, and soil fertility linked to agronomy. In a sense, the network shown on Fig. 3 forms a complex six-disciplinary system, which can be used for studying soil sustainability.

A framework for interdisciplinary research in soil sustainability linking soil science with social science, environmental science, ecology, climate science, and agronomy.
3. Soil and social science
3.1. knowledge transfer.
A myriad of scientific knowledge exists regarding best practice for soil management. However, there has been a general lack of adoption by farmers ( Bouma, 2019 ). This can be attributed to obstacles that hinder the distribution of relevant scientific information. Scientific evidence from in-depth studies is often scattered within various disciplines that use technical jargon that is little understood by the social scientists or journalists who are engaged in information transmittal and knowledge sharing. Modern electronic information sharing techniques, including social media tools (e.g., Twitter and Facebook), make mass information distribution easier ( Mills et al., 2019 ), but they can also make it difficult for lay people to distinguish between evidence-based reliable information and inaccurate or even misleading information. A parallel example occurred during the novel coronavirus disease (COVID-19) outbreak, during which large amounts of misinformation were transmitted across social media. Scientists felt the need to publish a joint statement to denounce such rumors ( Calisher et al., 2020 ).
Information management and knowledge sharing may help to fill the gap between knowledge generation and its useful application. This is particularly important for the application of soil science. A variety of soil information management and knowledge sharing mechanisms exist, including training workshops (online or offline), websites, social media, advisory services. In Australia, the New South Wales local government uses webinars to disseminate soil science information to a geographically disperse community of practice (CoP) ( Jenkins et al., 2019 ). Grain advisors, however, were reported to be guiding farmers to historically established “rules of thumb” for calculating nitrogen fertilizer needs, rather than the latest evidence-based science on soil water and nitrogen management ( Schwenke et al., 2019 ). Another Australian local government decided to share soil information and knowledge using a website coupled with training workshops. The type of information shared may include soil properties and landscape characteristics obtained from field assessment studies. Such initiatives show that centralized knowledge sharing can bring significant tangible benefits ( Imhof et al., 2019 ). However, a 10-year follow-up survey showed that while training workshops could be effective in the short term, behavioral change was not sustained in the long term. It was suggested that continuing professional development to upskill farm advisors and the CoP may render a more persistent uptake of knowledge at the farm level ( Andersson and Orgill, 2019 ).
In Europe, both private and public sector advisors, operating on national, provincial or local levels offer science communication to farmers ( Ingram and Mills, 2019 ). In Switzerland, sustainable soil management knowledge was successfully shared among farmers via social learning in a video format ( Fry and Thieme, 2019 ). A study in the English East Midlands suggested that soil advisors ought to incorporate hands-on practical knowledge ( Stoate et al., 2019 ). This concurs with another study in Australia, which showed that establishing a network of senior ex-governmental soil scientists and farmers enabled effective soil knowledge transfer ( Packer et al., 2019 ).
As precision agriculture incentivizes the use of sensing technologies to collect soil data, it becomes increasingly important to form public-private partnerships to collect, store, and use the huge amounts of geographically referenced soil data generated ( Robinson et al., 2019 ). The emerging fifth generation of wireless technology for digital cellular networks (5G), big data, and machine learning offer data collection and analysis techniques that may enable a new generation of soil information sharing tools. Within the 5G system, an internet of things (IoT) can be established with low latency, enabling real time soil measurement and response. For instance, unmanned aerial vehicle (UAV) based remote sensing can be coupled with soil amendment delivery in precision agricultural practice ( Kota and Giambene, 2019 ; Morais et al., 2019 ). Big data applications with machine learning also provide predictive power, facilitating smart farming to save energy, water, and cost, while increasing crop yields ( Wolfert et al., 2017 ).
3.2. Farmer behavior
The sustainability of soil use and management is ultimately reliant on the real-world behavior by practitioners, most particularly farmers. Therefore, there is a growing interest to integrate social components and farmer behavior with the ecological component of soil management ( Amin et al., 2019 ). In modern society, with the fast-growing use of various types of information technology, farmer behavior can be influenced by different network-based approaches. For instance, a study in Europe found that farmers formed a learning network by sharing information and soil knowledge on the microblogging and social networking service, Twitter. This platform has a limited length for each message (280 characters for non-Asian languages), making it easy for time-constrained farmers to follow ( Mills et al., 2019 ). In the US, an integrated network-based approach enabled a quarter of respondents to adopt cover crops for weed control, and respondents also increased their follow-up usage from information shared on Twitter (22%), YouTube (23%), and web sites (21%) ( Wick et al., 2019 ).
Farmer behavior and farming practice is also directly affected by professional advisors. In Australia, farmers apply the recommendations of professional crop advisors to select suitable fertilizer dosages. However, attitudes concerning financial risk, soil heterogeneity, and local climate conditions can affect their perception and adoption of such advice ( Schwenke et al., 2019 ). In Europe, a knowledge gap regarding sustainable soil management was identified as a major issue among both farmers and soil advisors. As the current trend of privatization and decentralization of advisory services continues, there is an increasing need to educate those who provide advisory services, thus enabling effective empowerment of farmers ( Ingram and Mills, 2019 ). Governments ought also to provide workshops that encourage farmers to adopt greater soil testing, so that they can then make informed soil management decisions ( Lobry de Bruyn, 2019 ).
Lack of education and awareness creates an obstacle for sustainable soil use and management, especially in developing countries. For example, it was found that farmer perception strongly correlates to adoption rates for conservation agriculture (r = 0.81; p < 0.05) ( Mugandani and Mafongoya, 2019 ). It has been reported that concerns over soil type, weed control, and weather conditions were the main inhibiting factors when English farmers consider reduced tillage practice. The authors suggested that enhanced adoption of sustainable soil management practice will require improved communication between the soil research community and farmers ( Alskaf et al., 2020 ).
3.3. Stakeholders
The creation, dissemination and usage of soil sustainability knowledge involves a wide range of stakeholders, such as scientists, farmers, land managers, advisory services, commercial product suppliers, regulators, funding agencies, educators, students, as well as the general public ( Knox et al., 2019 ; Tulau et al., 2019 ). Different stakeholders will have different concerns. Farmers and crop advisors are primarily concerned about local soil knowledge, while regulators and scientists are more concerned about policy, scientific solutions and the wider environment ( Bampa et al., 2019 ). There is also a dynamic interaction and potential gap between awareness and perception, i.e., what can be done and what is worth doing ( Krzywoszynska, 2019 ). Based on an analysis in England, Krzywoszynska (2019) argued that interactions between soil researchers and end users are multifaceted and that these actors must work together on both knowledge generation and knowledge sharing to enhance sustainable behavior.
Scientists and governments are pivotal stakeholders in promoting sustainable soil use and management practices. Their action can enhance the robustness of scientific knowledge creation and broaden its applicability by incorporating evidence into policy instruments. In Scotland, soil risk maps are created by scientists, policy makers and industrial representatives working in close collaboration ( Baggaley et al., 2020 ). Similarly, in Australia, soil constraints maps have been produced for site-specific management ( van Gool, 2016 ). Such tools can help mitigate constraints to achieving climate-driven genetic yield potential of agricultural crops. Models that incorporate learnings from stakeholder engagement can also render strong predictive power ( Inam et al., 2017 ). Traditionally, the main channel of soil knowledge generation has been government funded. However, there has been a general decreasing trend in the provision of government funds for soil data collection in many developed countries, while privately funded collection of soil information has increased dramatically ( Robinson et al., 2019 ). Under this situation, it is even more important to bring in additional stakeholders to create and share soil knowledge. The Soil Knowledge Network (SKN) in Australia demonstrated that ex-governmental soil scientists can exert long-lasting positive impacts by coaching new generations of early career soil scientists ( McInnes-Clarke et al., 2019 ).
4. Soil and climate science
4.1. soil organic carbon.
Soil organic carbon (SOC) has been recognized as a critical indicator of soil health, because it reflects the level of soil functionality associated with soil structure, hydraulic properties, and microbial activity, thereby integrating physical, chemical and biological health of soil ( Vogel et al., 2018 ). Recently, increasing attention has been placed on SOC beyond the traditional sphere of soil science. This is because it is a key component of Earth's carbon cycle, thus having huge implications for the current climate crisis ( Kell, 2012 ) and SDG13: Climate action. Soil is the largest terrestrial carbon pool, holding an estimated 1500–2400 GtC and permafrost (i.e. frozen soil) storing 1700 GtC ( Le Quere et al., 2018 ). A global initiative known as ‘4 per 1000’, which aims to increase soil organic carbon by 0.4% per year, would result in an additional carbon storage of 1.2 GtC per year if successful ( Paustian et al., 2016 ; Rumpel et al., 2018 ). In Australia, surface soils provide a significant reservoir of carbon, holding ~19 billion metric tons. However, most of these soils (~75%) contain <1% SOC, suggesting huge additional capacity for carbon sequestration. An annual 0.8% increase in carbon storage across all Australian surface soils would fully offset the nation's GHG emissions ( Baldock et al., 2010 )
Soil properties and vegetation are affected by the climatic condition ( Bond-Lamberty et al., 2018 ). For example, global warming may accelerate soil erosion due to its impact on microorganisms and plant and animal species ( Garcia-Pichel et al., 2013 ). Moreover, different soil types and land use systems are unevenly sensitive to temperature changes. Soil carbon that is normally recalcitrant in semi-arid regions is vulnerable to rising temperature ( Maia et al., 2019 ). Therefore, soil management practice in these areas may have a tremendous effect on carbon cycling.
Organic fertilizer applications can improve soil functionality and significantly increase SOC levels. Thus, applying organic amendments, including biosolids and composts, to agricultural land can increase carbon storage and contribute significantly to offsetting GHG emissions. Studies have shown that manure can potentially increase crop yields and soil organic contents in comparison with mineral fertilizers ( Jing et al., 2019 ). A 37-year field study showed that organic fertilization increased soil carbon input by 25% to 80%, although levels of carbon retention ranged from only 1.6% for green manure to 13.7% for fresh cattle manure ( Maltas et al., 2018 ). Similarly, Bolan et al. (2013) demonstrated that biosolid applications likely result in higher levels of carbon sequestration compared to other management strategies including fertilizer application and conservation tillage. This was attributed to an increased microbial biomass, and Fe and Al oxide-induced immobilization of carbon ( Bolan et al., 2013 ). In comparison with open-air systems, the use of organic fertilizers for indoor greenhouse soils may have a greater positive influence on soil functionality due to its effect on porosity and pore connectivity ( Xu et al., 2019 ). It should be noted that organic fertilizers may not increase crops yields to the levels achievable with inorganic fertilizers. This issue can be overcome by supplementing organic fertilizers with inorganic ones ( Maltas et al., 2018 ).
A variety of conservation farming practices can increase SOC levels, while also increasing crop productivity and decreasing water demand ( Kumar et al., 2019 ; Mehra et al., 2018 ). Crop residue return to surface soils can have a positive effect on soil carbon sequestration ( Chowdhury et al., 2015 ; Li et al., 2019b ). For example, chopping and returning wheat straw and corn stover can increase SOC levels by 14.5% in a double-cropping system ( Zhao et al., 2019 ). Reduced tillage and non-tillage practices can also increase soil SOC levels ( Chatskikh et al., 2008 ; Lafond et al., 2011 ). For example, a 22-year study showed that with no tillage, mulch treatment had a significantly positive effect on carbon retention ( Kahlon et al., 2013 ). Integrated methods have the potential to achieve even more significant increases in SOC levels. For example, SOC data collected over 35 years in a semi-arid region of China showed that carbon levels were enhanced (by 453% to 757%) using a combination of best practice cultivation, mulching, and planting methods ( Guoju et al., 2020 ). Different land uses also affect SOC, not only in terms of concentration, but also the fractions of SOC that are vulnerable to mineralization ( Ramesh et al., 2019 ). For example, labile and humified SOC fractions have been reported to be more prone to mineralization in arable lands than in grasslands ( Ukalska-Jaruga et al., 2019 ).
Accurate quantification of SOC remains a challenge because of high spatial heterogeneity in soils. For instance, features such as hedgerows and fences can influence SOC due to their impact on soil moisture and bulk density ( Ford et al., 2019 ). Soil compaction by agricultural machinery reduces macropores and creates water ponding ( Mossadeghi-Björklund et al., 2019 ), which can affect SOC. There are also discrepancies between SOC estimates using regional versus local parameters, particularly for in woodland soils containing large amounts of decaying organic matter (e.g., Histosols) and low-input high-diversity ecosystems ( Ottoy et al., 2019 ).
4.2. Biochar as a mitigation
Biochar is a carbon rich product that is produced by the burning of biomass with a limited supply of oxygen (i.e., pyrolysis) ( Lehmann and Joseph, 2009 ; Wang et al., 2020c ). It typically possess a stable fixed carbon structure with high porosity, a high specific surface area and a high alkalinity. These characteristics enable biochar to enhance soil moisture content, sorb polluting substances and increase soil pH ( Andrés et al., 2019 ). Moreover, biochar is considered carbon negative because the carbon within its structure, which is captured from the atmosphere during biomass formation, is more recalcitrant in the natural environment than carbon in biomass that has not been pyrolized. Because of its carbon negativity and beneficial properties for soil management, biochar has been proposed as a possible technology to help mitigate climate change ( Woolf et al., 2010 ). Numerous studies have explored the usage of biochar in croplands ( Laird et al., 2010b ), while recent studies have also examined its application in other systems, such as alpine grassland ( Rafiq et al., 2019 ).
At the current carbon price, applying biochar to soil is not commercially viable unless there is an additional benefit to farmers. Therefore, researchers have conducted extensive research on the benefits biochar for agricultural and environmental purposes. One of the most researched areas is the use of biochar to increase crop yields. A recent meta-analysis found that in comparison with inorganic fertilizer alone, biochar can increase crop yields by 11% to 19% (95% confidence intervals) ( Ye et al., 2020 ). Biochar has also been put forward as a sustainable technique for remediating soils degraded by contaminants, especially heavy metals ( Hou, 2020 ; O'Connor et al., 2018c ; Song et al., 2019 ). The sustainability of biochar is increased if the biomass feedstock is a biological waste that would otherwise be burned or discarded at landfill, thus avoiding air pollution or the consumption of landfill space. However, while a myriad of studies have shown biochar applications have positive effects on soil, it should be noted that such effects may diminish after 3– 5 years ( Dong et al., 2019 ). Biochar effectiveness and longevity may be enhanced by the invention of engineered biochars ( O'Connor et al., 2018b ).
4.3. Soil greenhouse gases
Soils act as significant sources of various greenhouse gases (GHGs), including CO 2 , CH 4 , and N 2 O. Reducing the emission of such GHGs is one of the greatest challenges for sustainable farming ( de Araújo Santos et al., 2019 ) and the achievement of SDG13: Climate action. Soil CO 2 emissions are affected by agricultural practice (e.g. tillage and fertilizer application), as well as the soil properties (e.g. soil texture). For sandy soils, greater macroporosity tends to be associated with higher CO 2 emissions, while microporosity is associated with lower emissions, which likely related to their respective tortuosity levels ( Farhate et al., 2019 ; Tavares et al., 2015 ). The use of lime to treat low pH soils may also relate to CO 2 emissions. Therefore, sustainable management of low pH grasslands may involve the use of low liming dosage rates, which provide almost the same result as higher rates ( Bolan et al., 2003 ; Kunhikrishnan et al., 2016 ; Lochon et al., 2019 ). A study in Denmark showed that reduced tillage practice can decrease net GHG emissions by 0.56 Mg CO 2 -eq. ha −1 per year; moreover, the use of disc coulters that minimally disturb soil can reduce net GHG emissions by 1.84 Mg CO 2 -eq. ha −1 per year ( Chatskikh et al., 2008 ).
Atmospheric N 2 O accounts for ~6% of radiative forcing caused by anthropogenic activity, which largely stems from soil systems ( Davidson, 2009 ). Therefore, emission of N 2 O from agricultural soil is particularly concerning. Davidson (2009) estimated that 2% of nitrogen in manures and 2.5% of nitrogen in fertilizers used by farmers over the period of 1860–2005 was converted to atmospheric N 2 O. In China, emissions derived from synthetic nitrogen fertilizers account for ~7% of the nation's annual GHG budget. By implementing new technology and best management practices that minimize nitrogen use in soil management, it is feasible to reduce GHG emissions by 102–357 Tg CO 2 -equivalent in China alone ( Zhang et al., 2013 ). Soil amendment with more sustainable alternatives to synthetic nitrogen (e.g., biochar) may help reduce N 2 O emissions from soil ( Senbayram et al., 2019 ).
Methane emissions from soil represent another major factor for climate change. An early study found that the application of rice straw to paddy fields increased CH 4 emissions by a factor of 1.8 to 3.5 ( Yagi and Minami, 1990 ). Recently, methane emissions from permafrost (permanently frozen soil) has drawn attention from the climate science community, owing to its critical role in carbon cycling ( Schuur et al., 2015 ). As climate change occurs, rising temperature in the polar regions causes permafrost to thaw and microbial activity to increase ( Hollesen et al., 2015 ). This leads to increased methane and CO 2 emissions from organic-rich Arctic soils ( Schuur et al., 2013 ). As these gases are associated with increased global warming potential, their emission increases the levels of permafrost thaw, thus forming a positive feedback loop. It is imperative to understand these processes in a quantitative way. As the climate change crisis worsens, it may be necessary to take mitigating measures involving soil management in areas associated with high methane fluxes.
5. Soil biodiversity and ecology
5.1. soil biodiversity.
Sustainable soil management practice can improve or conserve soil biodiversity, which represent a significant proportion of Earth's total biodiversity ( Bahram et al., 2018 ) and is pertinent to the achievement of the United Nations' SDGs (e.g., SDG15: Life on land). Among other factors, soil microbial communities are affected by the availability of nutrients corresponding to the type of soil management practice ( Bolan et al., 1996 ; Lauber et al., 2009 ; Leff et al., 2015 ). For example, the use of soluble fertilizers (e.g., monocalcium phosphate), less soluble organic fertilizer (e.g., sugarcane filter cake) or nearly insoluble rock phosphate ( Arruda et al., 2019 ) have different impacts on soil microbial communities. Soil management practices also affect soil hydraulics, which affects plant and microbial biodiversity and ecosystem resilience ( Alley et al., 2002 ; Anderegg et al., 2018 ). A study in India reported that integrating crop residue return with green manure application and no-tillage in a rice-wheat double cropping system increased SOC levels by 13%, the microbial biomass by 38%, the basal soil respiration rate by 33%, and the microbial quotient by 30% ( Saikia et al., 2020 ). Certain soil amendments are associated with increased soil biodiversity. For example, biochar amendment of a Mediterranean vineyard soil decreased the mineralization of both SOC and microbial biomass, while the functional microbial diversity and biodiversity of soil micro-arthropods were maintained ( Andrés et al., 2019 ). Soil properties and biodiversity are also affected by plant root systems within the rhizosphere ( Dey et al., 2012 ).
Larger species in soil are also an important aspect of soil biodiversity as well as being influential on soil properties ( Bardgett and van der Putten, 2014 ; Wu et al., 2011 ). Earthworms (Oligochaeta) are a particularly important soil species due to their creation of soil macro-pores (>0.3 mm) and channels (burrows) that increase water and gas infiltration rates ( Bartz et al., 2013 ; Bhadauria and Saxena, 2010 ). Thus earthworm activity can render soil environments that are more amenable to microbial activity and diversity ( Eriksen-Hamel et al., 2009 ). Conservation tillage practices that involve crop residue return to surface soils can increase earthworm numbers by hundreds of thousands per hectare ( Barthod et al., 2018 ; Giannitsopoulos et al., 2020 )
5.2. Ecosystem services
Soils provide vital ecosystem services, rendering both economic and societal benefits ( Adhikari and Hartemink, 2016 ; Dominati et al., 2010 ; Pavan and Ometto, 2018 ; Su et al., 2018 ). Monetary valuation methods have been put forward to account for the natural capital of this resource ( Robinson et al., 2014 ). In this way, a national-scale study in the UK suggested that an additional £18 billion GBP of ecosystem services could be achieved under an optimal policy scenario. This value takes into account major ecosystem services, such as agricultural production, carbon sequestration, recreational usage, and wildlife diversity ( Bateman et al., 2013 ). However, some scholars have argued that systematic monetarization is unnecessary. For example, Bayesian Belief Networks (BBNs) and Multi-Criteria Decision Analysis (MCDA) methods can provide decision makers with semi-quantitative information that takes into account the multifunctionality of soil ecosystem services ( Baveye et al., 2016 ).
Living organisms in soil have a direct impact on agricultural productivity and ecosystem services. For instance, the microbial community is essential for the natural decontamination of polluted soils. Therefore, monitoring biological indicators is necessary for managing soil ecosystems effectively. Some of the most important soil biota indicators include microsymbionts, decomposers, elemental transformers, soil ecosystem engineers, soil-borne pests and diseases, and microregulators ( Barrios, 2007 ). Soil invertebrates also play a significant role in soil ecosystem services ( Lavelle et al., 2006 ).
In Europe, a large number of monitoring programs and field studies have been conducted since the 1990s, to gain data for optimizing ecosystem services ( Pulleman et al., 2012 ). The data shows that spatial heterogeneity within soil systems translates into the uneven distribution of ecosystem services ( Aitkenhead and Coull, 2019 ). Governments may intervene to restore or improve ecological services in limited soil systems. In China, for example, the government has made subsidies available to farmers to protect natural woodlands and convert steep agricultural cropland into other land uses, such as grassland or woodland ( Liu et al., 2008 ). If farmland is degraded to an extent that it is abandoned, soil treatments may help bring about natural revegetation and the recovery of ecosystem services ( Li et al., 2019a ). For example, the recovery of severely degraded land can be facilitated by the use of soil amendments such as biochar ( O'Connor et al., 2018c ).
6. Soil and environmental science
6.1. soil pollution.
Contaminants are an issue for many agricultural sites ( Bolan et al., 2014 ; Khan, 2016 ; O'Connor et al., 2019b ; Wilcke, 2000 ), which hinders efforts toward the achievement of the United Nations' SDGs (e.g., SDG3: Good health and well-being). Soil contaminants include heavy metals, such as cadmium (Cd), copper (Cu), lead (Pb), mercury (Hg) and zinc (Zn), and organic pollutants, such as pesticides and polycyclic aromatic hydrocarbons (PAHs). As an emerging contaminant, microplastics in the soil environment have also drawn attention in recent years ( Bradney et al., 2019 ; Jia et al., 2020 ; O'Connor et al., 2020 ; Wang et al., 2020a ). Assessment of their fate and transport is critical for understanding the environmental risk ( Corradini et al., 2019 ; Wang et al., 2019a ).
A global map of soil pollution is urgently needed to understand better the situation globally, but few countries are investing in national-scale investigations ( Hou and Ok, 2019 ). Elevated levels of soil pollutants can result from a wide variety of anthropogenic activities, ranging from metal mining to fossil fuel burning ( Zhang et al., 2020b ). The spatial redistribution of these pollutants involves inter-phase transfer such as dissolution from soil to water, volatilization from soil to air, and deposition from air to soil ( O'Connor et al., 2019a ; Zhang et al., 2019 ). Anthropogenic soil pollution in under-developed regions where industrial activities are less intensive can also occur due to traffic and mining related emissions, etc. For instance, a recent study in a suburban area of Central Asia showed that Pb, Zn, and Cu can accumulate to high levels in soils because of road traffic up to 200 m away ( Ma et al., 2019 ).
The remediation of contaminated soil is an important research field interlinking soil science and environmental science. Traditionally, remediation practitioners focused on either physical cleanup methods, such as soil excavation and disposal at landfill ( Qi et al., 2020 ), or chemical treatment methods, such as in situ chemical oxidation ( O'Connor et al., 2018a ). In recent years, nature-based solutions, such as phytoremediation and green stabilization, have gained attention among the scientific research community ( Wang et al., 2019b ; Wang et al., 2020b ; Zhang et al., 2020a ). For example, microbial strains from unique natural environments are being harvested, cultured, and exploited to render economic and environmentally friendly solutions for soil decontamination ( Atashgahi et al., 2018 ; Bunge et al., 2003 ).
6.2. Soil erosion
Soil erosion, a major land degradation process, is caused by the weathering effects of water and wind ( Lal, 2003 ). For land covered by native vegetation, natural erosion rates will tend to balance with soil production rates. However, typical agricultural tillage practice can disrupt this balance, causing levels of soil erosion to be one to two orders of magnitude higher than that of soil formation ( Montgomery, 2007b ). Soil systems that experience net soil erosion can suffer the loss of fertile surface soils, removal of soil organic carbon, and reduced agricultural productivity, thus rendering a high environmental and economic cost globally ( Montgomery, 2007a ; Pimentel et al., 1995 ). Because heavy metals tend to bind strongly to eroded soil particles, the widespread distribution of soil pollutants is also often associated with soil erosion ( Xiao et al., 2019 ).
Soil erosion not only causes damage to the land where it occurs, but also jeopardizes local aquatic systems due to excessive sediment loading ( Boardman et al., 2019 ). Soil erosion models have been developed to predict impacts of water quality on a catchment-scale ( Fu et al., 2019 ). It can also cause damage to nearby housing due to increased surface runoff and landslides. Because of such impacts, many governments are taking largescale mitigating action, such as revegetation with native species and woodland restoration ( Teng et al., 2019 ).
6.3. Soil leaching
During heavy rainfall, irrigation, or recharge events, large volumes of water may come into contact with various substances as soil pore spaces fill ( O'Connor and Hou, 2019 ). In this process, there are complex interactions between gaseous, liquid, and solid phases for soil nutrients, potentially toxic elements, and organic pollutants. If soil nutrients or contaminants are leached from surface soils, they can transport into the subsurface via the vertical migration of infiltration water. This can lead to large scale groundwater pollution involving substances such as ammonia ( Jia et al., 2019 ). Leached nutrients in surface runoff may also enter nearby surface water bodies, causing eutrophication ( Maguire and Sims, 2002 ). Soil leaching may be particularly prominent in the autumn-winter season due to reduced plant activity ( Welten et al., 2019 ).
Soil leaching potential is exacerbated by common physical farming practices, including the installation of deep drainage ( Nachimuthu et al., 2019 ). The potential for soil leaching is also affected by soil management practices that alter the chemical composition of soil. For instance, liming is a common farming method to increase soil pH and reduce flocculation. However, recent studies have suggested that soil particle surfaces become more negatively charged as soil pH increases. Therefore, liming activity may lead to soil-bound harmful substances, such as perfluorooctane sulfonic acid (PFOS) and perfluorooctanoic acid (PFOA), leaching from soil and entering groundwater systems ( Oliver et al., 2019 ). In New Zealand, intensified agricultural production on steep landscapes, which is encouraged by the government's policy to significantly increase agricultural exports, has involved the replacement of perennial pastures with winter forage crops. This has increased the use agrochemicals, including glyphosate and diazinon, which not only pose an environmental risk in themselves, but also facilitate the leaching of organic carbon and nitrogen ( Chibuike et al., 2019 ). The reporting of such unintended consequences reinforces the importance of comprehensive assessments for sustainable soil use and management. It should be noted that certain soil amendments, such as biochar, have been shown to reduce soil nutrient leaching potential ( Laird et al., 2010a ).
Soil leaching can increase the spatial heterogeneity of soil nutrients, which makes soil management more difficult. For instance, intensively farmed cropland tends to be subject to high nitrogen input levels. However, plant-animal-soil systems are not efficient in utilizing large amounts of nitrogen, with only 15–35% being embedded in agricultural products. A large percentage of the surplus nitrogen is returned to localized spots via animal urinary excretions, resulting in elevated nitrogen hotspots.
7. Summary, challenges and future directions
The international community's commitment to achieving the United Nations' Sustainable Development Goals (SDGs) hinge on soil health. However, neither the scientific community nor policy makers have paid sufficient attention to soil in their SDG efforts. Soil scientists have not been adequately involved in the discussion on SDG targets and indicators ( Bouma et al., 2019 ). Consequently, while there are four SDG targets that specifically mention soil, and others that indirectly relate to soil, only one explicit soil indicator has been established ( Bouma et al., 2019 ). The lack of involvement by soil scientists may be due to their strong focus on pure soil science, rather than conducting cross-disciplinary and elaborate discussions on big picture soil related issues with other stakeholders. To help provide effective SDG solutions, it is imperative to encourage interdisciplinary soil research among soil scientists and researchers in fields relating to social science, climate science, ecology, and environmental science. When national and local governments form policies according to the United Nations SDGs, soil scientists need to be encouraged to play a more active role, and their advice needs to be sought by decision makers. For instance, by nominating soil scientists to key steering committees.
A big challenge for sustainable soil use and management is the inherent spatial heterogeneity of soil properties, from the micro to the global scale. This makes it difficult to predict non-linear relationships among various soil processes and system behaviors ( Manzoni and Porporato, 2009 ). For example, regional estimates of soil organic carbon stocks have differed by as much as 60% on different scales due to this heterogeneity ( Illiger et al., 2019 ). There is little known about the vertical distribution of organic carbon in the subsurface ( Balesdent et al., 2018 ). As large amounts of carbon are stored in deep soils ( Yu et al., 2019 ), it is essential to understand the status, as well as the mechanisms, of soil carbon cycling across the full extent of the lithosphere.
Spatial heterogeneity also exists in socioeconomic systems. Consider for example the size of typical farm holdings among different countries. In rural China, most farms are smallholdings of <0.5 ha. In Hungary, most farms are also relatively small, with 79% being <2 ha. In contrast, Danish farms tend to quite large, with 55% being larger than 20 ha ( Ingram and Mills, 2019 ). Such differences create challenges for knowledge transfer between countries. For instance, farm size may act as a barrier to the adoption of sustainable farming technology because of financial or technical constraints ( Alskaf et al., 2020 ).
It is important to describe long-term temporal trends in soil system behavior because many prominent issues, such as the climate crisis, require perceptive solutions based on long-term evidence. However, many existing studies, especially studies on emerging issues, are based on short-term findings. For instance, a recent pasture-system study suggested that various species could be planted to control nitrogen leaching associated with cow urine ( Welten et al., 2019 ). This promising finding, however, was based on less than one year of data. Longer-term studies are necessary to verify the effectiveness of such strategies. Greater efforts should be paid on the research and development of accelerated aging techniques ( Shen et al., 2019 )
Progress in sustainable soil use and management relies upon the development of suitable and holistic indicators for soil health that reflect the diverse processes involved, in a concise, quantifiable, reliable and meaningful way. To achieve this goal, soil health needs to be evaluated under site-specific conditions that account for the different processes of different geological, climatic, and societal conditions ( Vogel et al., 2018 ). This would be particularly valuable for aiding farmers with decision making and translating soil science into practical sustainable soil use and management practice. Moreover, to support policy making processes, it is necessary to map soil properties on a regional scale, or even on national and global scales. High resolution mapping and clustering of soil properties would enable targeted recommendations for sustainable soil management ( Donoghue et al., 2019 ). It should also be noted that while many existing soil sustainability studies have focused on the impacts of socioeconomic activities (i.e. soil management) on soil systems (i.e. soil health), studies regarding the impacts of soil systems on socioeconomic systems are less common ( Vogel et al., 2018 ).
Information management and knowledge sharing are critical for building collaborative governance and delivering sustainable solutions ( Bodin, 2017 ). In this new era of information, massive amounts of valuable information (and misinformation) are produced. This poses a challenge to both the knowledge creators, who struggle to make it visible in an ocean of information, and the knowledge users, who struggle to distinguish whether information is valuable or not. Emerging and advanced technologies, such as 5G, big data and machine learning present great opportunities for addressing these challenges. Interdisciplinary studies initiated by, or in collaboration with, communication engineers and computer scientists hold much potential in advancing our capability in sustainable use and management of soil resources.
Declaration of competing interest
The authors declare that they have no known competing financial interests or personal relationships that could have appeared to influence the work reported in this paper.
Acknowledgements
This work was supported by the National Key Research and Development Program of China (Grant No. 2018YFC1801300).
Editor: Jay Gan
- Adhikari K., Hartemink A.E. Linking soils to ecosystem services—a global review. Geoderma. 2016; 262 :101–111. [ Google Scholar ]
- Aitkenhead M.J., Coull M.C. Digital mapping of soil ecosystem services in Scotland using neural networks and relationship modelling. Part 2: mapping of soil ecosystem services. Soil Use Manag. 2019; 35 :217–231. [ Google Scholar ]
- Alley W.M., Healy R.W., LaBaugh J.W., Reilly T.E. Hydrology - flow and storage in groundwater systems. Science. 2002; 296 :1985–1990. [ PubMed ] [ Google Scholar ]
- Alskaf K., Sparkes D.L., Mooney S.J., Sjögersten S., Wilson P. The uptake of different tillage practices in England. Soil Use Manag. 2020; 36 :27–44. [ Google Scholar ]
- Amin M.N., Hossain M.S., Lobry de Bruyn L., Wilson B. A systematic review of soil carbon management in Australia and the need for a social-ecological systems framework. Sci. Total Environ. 2019:135182. [ PubMed ] [ Google Scholar ]
- Amundson R., Berhe A.A., Hopmans J.W., Olson C., Sztein A.E., Sparks D.L. Soil and human security in the 21st century. Science. 2015; 348 [ PubMed ] [ Google Scholar ]
- Ananbeh H., Stojanović M., Pompeiano A., Voběrková S., Trasar-Cepeda C. Use of soil enzyme activities to assess the recovery of soil functions in abandoned coppice forest systems. Sci. Total Environ. 2019; 694 [ PubMed ] [ Google Scholar ]
- Anderegg W.R.L., Konings A.G., Trugman A.T., Yu K., Bowling D.R., Gabbitas R. Hydraulic diversity of forests regulates ecosystem resilience during drought. Nature. 2018; 561 :538. [ PubMed ] [ Google Scholar ]
- Andersson K.O., Orgill S.E. Soil extension needs to be a continuum of learning; soil workshop reflections 10 years on. Soil Use Manag. 2019; 35 :117–127. [ Google Scholar ]
- Andrés P., Rosell-Melé A., Colomer-Ventura F., Denef K., Cotrufo M.F., Riba M. Belowground biota responses to maize biochar addition to the soil of a Mediterranean vineyard. Sci. Total Environ. 2019; 660 :1522–1532. [ PubMed ] [ Google Scholar ]
- de Araújo Santos G.A., Moitinho M.R., de Oliveira Silva B., Xavier C.V., Teixeira D.D.B., Corá J.E. Effects of long-term no-tillage systems with different succession cropping strategies on the variation of soil CO2 emission. Sci. Total Environ. 2019; 686 :413–424. [ PubMed ] [ Google Scholar ]
- Arruda B., Rodrigues M., Gumiere T., Richardson A.E., Andreote F.D., Soltangheisi A. The impact of sugarcane filter cake on the availability of P in the rhizosphere and associated microbial community structure. Soil Use Manag. 2019; 35 :334–345. [ Google Scholar ]
- Atashgahi S., Sanchez-Andrea I., Heipieper H.J., van der Meer J.R., Stams A.J.M., Smidt H. Prospects for harnessing biocide resistance for bioremediation and detoxification. Science. 2018; 360 :743–746. [ PubMed ] [ Google Scholar ]
- Baggaley N., Lilly A., Blackstock K., Dobbie K., Carson A., Leith F. Soil risk maps–interpreting soils data for policy makers, agencies and industry. Soil Use Manag. 2020; 36 :19–26. [ Google Scholar ]
- Bahram M., Hildebrand F., Forslund S.K., Anderson J.L., Soudzilovskaia N.A., Bodegom P.M. Structure and function of the global topsoil microbiome. Nature. 2018; 560 :233. [ PubMed ] [ Google Scholar ]
- Baldock J., Sanderman J., Farquharson R. Proceedings of the 19th World Congress of Soil Science. 2010. Capturing carbon in Australian soils: Potential and realities; pp. 1–6. [ Google Scholar ]
- Balesdent J., Basile-Doelsch I., Chadoeuf J., Cornu S., Derrien D., Fekiacova Z. Atmosphere-soil carbon transfer as a function of soil depth. Nature. 2018; 559 :599. [ PubMed ] [ Google Scholar ]
- Bampa F., O’Sullivan L., Madena K., Sandén T., Spiegel H., Henriksen C.B. Harvesting European knowledge on soil functions and land management using multi-criteria decision analysis. Soil Use Manag. 2019; 35 :6–20. [ Google Scholar ]
- Bardgett R.D., van der Putten W.H. Belowground biodiversity and ecosystem functioning. Nature. 2014; 515 :505–511. [ PubMed ] [ Google Scholar ]
- Barrios E. Soil biota, ecosystem services and land productivity. Ecol. Econ. 2007; 64 :269–285. [ Google Scholar ]
- Barthod J., Rumpel C., Calabi-Floody M., Mora M.-L., Bolan N., Dignac M.-F. Adding worms during composting of organic waste with red mud and fly ash reduces CO2 emissions and increases plant available nutrient contents. J. Environ. Manag. 2018; 222 :207–215. [ PubMed ] [ Google Scholar ]
- Bartz M.L.C., Pasini A., Brown G.G. Earthworms as soil quality indicators in Brazilian no-tillage systems. Appl. Soil Ecol. 2013; 69 :39–48. [ Google Scholar ]
- Bateman I.J., Harwood A.R., Mace G.M., Watson R.T., Abson D.J., Andrews B. Bringing ecosystem services into economic decision-making: land use in the United Kingdom. Science. 2013; 341 :45–50. [ PubMed ] [ Google Scholar ]
- Baveye P.C., Baveye J., Gowdy J. Soil “ecosystem” services and natural capital: critical appraisal of research on uncertain ground. Frontiers in Environmental Science. 2016; 4 :41. [ Google Scholar ]
- Bender S.F., Wagg C., van der Heijden M.G. An underground revolution: biodiversity and soil ecological engineering for agricultural sustainability. Trends Ecol. Evol. 2016; 31 :440–452. [ PubMed ] [ Google Scholar ]
- Bhadauria T., Saxena K.G. Role of earthworms in soil fertility maintenance through the production of biogenic structures. Applied and environmental soil science. 2010; 2010 [ Google Scholar ]
- Bharati L., Lee K.-H., Isenhart T., Schultz R. Soil-water infiltration under crops, pasture, and established riparian buffer in Midwestern USA. Agrofor. Syst. 2002; 56 :249–257. [ Google Scholar ]
- Boardman J., Vandaele K., Evans R., Foster I.D. Off-site impacts of soil erosion and runoff: why connectivity is more important than erosion rates. Soil Use Manag. 2019; 35 :245–256. [ Google Scholar ]
- Bodin O. Collaborative environmental governance: achieving collective action in social-ecological systems. Science. 2017; 357 :659–+. [ PubMed ] [ Google Scholar ]
- Bolan N., Currie L., Baskaran S. Assessment of the influence of phosphate fertilizers on the microbial activity of pasture soils. Biol. Fertil. Soils. 1996; 21 :284–292. [ Google Scholar ]
- Bolan N.S., Adriano D.C., Curtin D. Soil acidification and liming interactions with nutrient and heavy metal transformation and bioavailability. Adv. Agron. 2003; 78 :5–272. [ Google Scholar ]
- Bolan N.S., Kunhikrishnan A., Naidu R. Carbon storage in a heavy clay soil landfill site after biosolid application. Sci. Total Environ. 2013; 465 :216–225. [ PubMed ] [ Google Scholar ]
- Bolan N., Kunhikrishnan A., Thangarajan R., Kumpiene J., Park J., Makino T. Remediation of heavy metal (loid) s contaminated soils–to mobilize or to immobilize? J. Hazard. Mater. 2014; 266 :141–166. [ PubMed ] [ Google Scholar ]
- Bond-Lamberty B., Bailey V.L., Chen M., Gough C.M., Vargas R. Globally rising soil heterotrophic respiration over recent decades. Nature. 2018; 560 :80. [ PubMed ] [ Google Scholar ]
- Bouma J. How to communicate soil expertise more effectively in the information age when aiming at the UN Sustainable Development Goals. Soil Use Manag. 2019; 35 :32–38. [ Google Scholar ]
- Bouma J., Montanarella L. Facing policy challenges with inter-and transdisciplinary soil research focused on the UN Sustainable Development Goals. Soil. 2016; 2 :135. [ Google Scholar ]
- Bouma J., Montanarella L., Evanylo G. The challenge for the soil science community to contribute to the implementation of the UN Sustainable Development Goals. Soil Use Manag. 2019; 35 :538–546. [ Google Scholar ]
- Bradney L., Wijesekara H., Palansooriya K.N., Obadamudalige N., Bolan N.S., Ok Y.S. Particulate plastics as a vector for toxic trace-element uptake by aquatic and terrestrial organisms and human health risk. Environ. Int. 2019; 131 [ PubMed ] [ Google Scholar ]
- Bryan B.A., Gao L., Ye Y., Sun X., Connor J.D., Crossman N.D. China’s response to a national land-system sustainability emergency. Nature. 2018; 559 :193–204. [ PubMed ] [ Google Scholar ]
- Bunge M., Adrian L., Kraus A., Opel M., Lorenz W.G., Andreesen J.R. Reductive dehalogenation of chlorinated dioxins by an anaerobic bacterium. Nature. 2003; 421 :357–360. [ PubMed ] [ Google Scholar ]
- Calisher C., Carroll D., Colwell R., Corley R.B., Daszak P., Drosten C. Statement in support of the scientists, public health professionals, and medical professionals of China combatting COVID-19. Lancet. 2020; 395 (10226):PE42–E43. [ PMC free article ] [ PubMed ] [ Google Scholar ]
- Carlson K.M., Curran L.M., Ratnasari D., Pittman A.M., Soares-Filho B.S., Asner G.P. Committed carbon emissions, deforestation, and community land conversion from oil palm plantation expansion in West Kalimantan, Indonesia. Proc. Natl. Acad. Sci. U. S. A. 2012; 109 :7559–7564. [ PMC free article ] [ PubMed ] [ Google Scholar ]
- Celentano D., Rousseau G.X., Engel V.L., Zelarayán M., Oliveira E.C., Araujo A.C.M. Degradation of riparian forest affects soil properties and ecosystem services provision in eastern Amazon of Brazil. Land Degrad. Dev. 2017; 28 :482–493. [ Google Scholar ]
- Chatskikh D., Olesen J.E., Hansen E.M., Elsgaard L., Petersen B.M. Effects of reduced tillage on net greenhouse gas fluxes from loamy sand soil under winter crops in Denmark. Agric. Ecosyst. Environ. 2008; 128 :117–126. [ Google Scholar ]
- Chibuike G., Burkitt L., Camps-Arbestain M., Bishop P., Bretherton M., Singh R. Effect of forage crop establishment on dissolved organic carbon dynamics and leaching in a hill country soil. Soil Use Manag. 2019; 35 :453–465. [ Google Scholar ]
- Chowdhury S., Farrell M., Butler G., Bolan N. Assessing the effect of crop residue removal on soil organic carbon storage and microbial activity in a no-till cropping system. Soil Use Manag. 2015; 31 :450–460. [ Google Scholar ]
- Corradini F., Meza P., Eguiluz R., Casado F., Huerta-Lwanga E., Geissen V. Evidence of microplastic accumulation in agricultural soils from sewage sludge disposal. Sci. Total Environ. 2019; 671 :411–420. [ PubMed ] [ Google Scholar ]
- Davidson E.A. The contribution of manure and fertilizer nitrogen to atmospheric nitrous oxide since 1860. Nat. Geosci. 2009; 2 :659. [ Google Scholar ]
- Dey R., Pal K.K., Tilak K.V.B.R. Influence of soil and plant types on diversity of rhizobacteria. Proceedings of the National Academy of Sciences India Section B-Biological Sciences. 2012; 82 :341–352. [ Google Scholar ]
- Dominati E., Patterson M., Mackay A. A framework for classifying and quantifying the natural capital and ecosystem services of soils. Ecol. Econ. 2010; 69 :1858–1868. [ Google Scholar ]
- Dong X., Singh B.P., Li G., Lin Q., Zhao X. Biochar has little effect on soil dissolved organic carbon pool 5 years after biochar application under field condition. Soil Use Manag. 2019; 35 :466–477. [ Google Scholar ]
- Donoghue S., Furley P.A., Stuart N., Haggis R., Trevaskis A., Lopez G. The nature and spatial variability of lowland savanna soils: improving the resolution of soil properties to support land management policy. Soil Use Manag. 2019; 35 :547–560. [ Google Scholar ]
- Doran J.W., Parkin T.B. Defining and assessing soil quality. Defining soil quality for a sustainable environment. 1994; 35 :1–21. [ Google Scholar ]
- Doran J., Safley M. CAB International; New York: 1997. Defining and Assessing Soil Health and Sustainable Productivity. Biological Indicators of Soil Health. [ Google Scholar ]
- Doran J.W., Zeiss M.R. Soil health and sustainability: managing the biotic component of soil quality. Appl. Soil Ecol. 2000; 15 :3–11. [ Google Scholar ]
- Eriksen-Hamel N.S., Speratti A.B., Whalen J.K., Légère A., Madramootoo C.A. Earthworm populations and growth rates related to long-term crop residue and tillage management. Soil Tillage Res. 2009; 104 :311–316. [ Google Scholar ]
- FAO . Earthscan; 2011. The State of the World’s Land and Water Resources for Food and Agriculture: Managing Systems at Risk. [ Google Scholar ]
- Farhate C.V., de Souza Z.M., La Scala N., Jr., de Sousa A.C.M., Santos A.P.G., Carvalho J.L.N. Soil tillage and cover crop on soil CO2 emissions from sugarcane fields. Soil Use Manag. 2019; 35 :273–282. [ Google Scholar ]
- Ford H., Healey J.R., Webb B., Pagella T.F., Smith A.R. How do hedgerows influence soil organic carbon stock in livestock-grazed pasture? Soil Use Manag. 2019; 35 :576–584. [ Google Scholar ]
- Franzluebbers A.J. Soil organic carbon sequestration and agricultural greenhouse gas emissions in the southeastern USA. Soil Tillage Res. 2005; 83 :120–147. [ Google Scholar ]
- Fry P., Thieme S. A social learning video method: identifying and sharing successful transformation knowledge for sustainable soil management in Switzerland. Soil Use Manag. 2019; 35 :185–194. [ Google Scholar ]
- Fu B., Merritt W.S., Croke B.F., Weber T.R., Jakeman A.J. A review of catchment-scale water quality and erosion models and a synthesis of future prospects. Environ. Model Softw. 2019; 114 :75–97. [ Google Scholar ]
- Garcia-Pichel F., Loza V., Marusenko Y., Mateo P., Potrafka R.M. Temperature drives the continental-scale distribution of key microbes in topsoil communities. Science. 2013; 340 :1574–1577. [ PubMed ] [ Google Scholar ]
- Giannitsopoulos M.L., Burgess P.J., Rickson R.J. Effects of conservation tillage drills on soil quality indicators in a wheat–oilseed rape rotation: organic carbon, earthworms and water-stable aggregates. Soil Use Manag. 2020; 36 :139–152. [ Google Scholar ]
- van Gool D. 2016. Identifying Soil Constraints That Limit Wheat Yield in the South-west of Western Australia. [ Google Scholar ]
- Gossner M.M., Lewinsohn T.M., Kahl T., Grassein F., Boch S., Prati D. Land-use intensification causes multitrophic homogenization of grassland communities. Nature. 2016; 540 :266–+. [ PubMed ] [ Google Scholar ]
- Guoju X., Yanbin H., Qiang Z., Jing W., Ming L. Impact of cultivation on soil organic carbon and carbon sequestration potential in semiarid regions of China. Soil Use Manag. 2020; 36 :83–92. [ Google Scholar ]
- Hammond R.A., Dubé L. A systems science perspective and transdisciplinary models for food and nutrition security. Proc. Natl. Acad. Sci. 2012; 109 :12356–12363. [ PMC free article ] [ PubMed ] [ Google Scholar ]
- Hollesen J., Matthiesen H., Møller A.B., Elberling B. Permafrost thawing in organic Arctic soils accelerated by ground heat production. Nat. Clim. Chang. 2015; 5 :574–578. [ Google Scholar ]
- Hou D. Elsevier Inc; 2020. Sustainable Remediation of Contaminated Soil and Groundwater: Materials, Processes, and Assessment. [ Google Scholar ]
- Hou D., Ok Y.S. Speed up mapping of soil pollution. Nature. 2019; 566 :455. [ PubMed ] [ Google Scholar ]
- Illiger P., Schmidt G., Walde I., Hese S., Kudrjavzev A.E., Kurepina N. Estimation of regional soil organic carbon stocks merging classified land-use information with detailed soil data. Sci. Total Environ. 2019; 695 [ PubMed ] [ Google Scholar ]
- Imhof M.P., Heemskerk G.E., Cox M.T. Soil information management and knowledge sharing in Victoria, Australia—user perspectives. Soil Use Manag. 2019; 35 :39–51. [ Google Scholar ]
- Inam A., Adamowski J., Prasher S., Halbe J., Malard J., Albano R. Coupling of a distributed stakeholder-built system dynamics socio-economic model with SAHYSMOD for sustainable soil salinity management–part 1: model development. J. Hydrol. 2017; 551 :596–618. [ Google Scholar ]
- Ingram J., Mills J. Are advisory services “fit for purpose” to support sustainable soil management? An assessment of advice in Europe. Soil Use Manag. 2019; 35 :21–31. [ Google Scholar ]
- Janvier C., Villeneuve F., Alabouvette C., Edel-Hermann V., Mateille T., Steinberg C. Soil health through soil disease suppression: which strategy from descriptors to indicators? Soil Biol. Biochem. 2007; 39 :1–23. [ Google Scholar ]
- Jenkins A., Beange L., Morris S. Using webinars to extend the reach of soil learning in New South Wales: a first look. Soil Use Manag. 2019; 35 :52–62. [ Google Scholar ]
- Jia X., O’Connor D., Hou D., Jin Y., Li G., Zheng C. Groundwater depletion and contamination: spatial distribution of groundwater resources sustainability in China. Sci. Total Environ. 2019; 672 :551–562. [ PubMed ] [ Google Scholar ]
- Jia H., Hou D., O’Connor D., Pan S., Zhu J., Bolan N.S. Exogenous phosphorus treatment facilitates chelation-mediated cadmium detoxification in perennial ryegrass (Lolium perenne L.) J. Hazard. Mater. 2020; 389 [ PubMed ] [ Google Scholar ]
- Jing J., Christensen J.T., Sørensen P., Christensen B.T., Rubæk G.H. Long-term effects of animal manure and mineral fertilizers on phosphorus availability and silage maize growth. Soil Use Manag. 2019; 35 :323–333. [ Google Scholar ]
- Kahlon M.S., Lal R., Ann-Varughese M. Twenty two years of tillage and mulching impacts on soil physical characteristics and carbon sequestration in Central Ohio. Soil Tillage Res. 2013; 126 :151–158. [ Google Scholar ]
- Keesstra S.D., Bouma J., Wallinga J., Tittonell P., Smith P., Cerdà A. The significance of soils and soil science towards realization of the United Nations Sustainable Development Goals. Soil. 2016; 2 :111–128. doi: 10.5194/soil-2-111-2016. [ CrossRef ] [ Google Scholar ]
- Kell D.B. Large-scale sequestration of atmospheric carbon via plant roots in natural and agricultural ecosystems: why and how. Philosophical Transactions of the Royal Society B: Biological Sciences. 2012; 367 :1589–1597. [ PMC free article ] [ PubMed ] [ Google Scholar ]
- Khan S.U. Elsevier; 2016. Pesticides in the Soil Environment. [ Google Scholar ]
- Kibblewhite M., Ritz K., Swift M. Soil health in agricultural systems. Philosophical Transactions of the Royal Society B: Biological Sciences. 2008; 363 :685–701. [ PMC free article ] [ PubMed ] [ Google Scholar ]
- Klein J.T., Newell W.H. Handbook of the Undergraduate Curriculum: A Comprehensive Guide to Purposes, Structures, Practices, and Change. 1997. Advancing interdisciplinary studies; pp. 393–415. [ Google Scholar ]
- Knox O.G., Osanai Y., Polain K., Pereg L., Nachimuthu G., Wilson B. Lessons from extension activity related to cotton rotation impacts on soil—a scientist’s perspective. Soil Use Manag. 2019; 35 :141–149. [ Google Scholar ]
- Kota S., Giambene G. Proceedings of the Eleventh International Conference on Advances in Satellite and Space Communications-SPACOMM. 2019. Satellite 5G: IoT use case for rural areas applications; pp. 24–28. [ Google Scholar ]
- Krzywoszynska A. Making knowledge and meaning in communities of practice: what role may science play? The case of sustainable soil management in England. Soil Use Manag. 2019; 35 :160–168. [ Google Scholar ]
- Kumar V., Gathala M.K., Saharawat Y.S., Parihar C.M., Kumar R., Kumar R. Impact of tillage and crop establishment methods on crop yields, profitability and soil physical properties in rice–wheat system of indo-Gangetic Plains of India. Soil Use Manag. 2019; 35 :303–313. [ Google Scholar ]
- Kunhikrishnan A., Thangarajan R., Bolan N., Xu Y., Mandal S., Gleeson D. Functional relationships of soil acidification, liming, and greenhouse gas flux. Adv. Agron. 2016; 139 :1–71. Elsevier. [ Google Scholar ]
- Lafond G.P., Walley F., May W., Holzapfel C. Long term impact of no-till on soil properties and crop productivity on the Canadian prairies. Soil Tillage Res. 2011; 117 :110–123. [ Google Scholar ]
- Laird D., Fleming P., Wang B., Horton R., Karlen D. Biochar impact on nutrient leaching from a Midwestern agricultural soil. Geoderma. 2010; 158 :436–442. [ Google Scholar ]
- Laird D.A., Fleming P., Davis D.D., Horton R., Wang B., Karlen D.L. Impact of biochar amendments on the quality of a typical Midwestern agricultural soil. Geoderma. 2010; 158 :443–449. [ Google Scholar ]
- Lal R. Soil erosion and the global carbon budget. Environ. Int. 2003; 29 :437–450. [ PubMed ] [ Google Scholar ]
- Lauber C.L., Hamady M., Knight R., Fierer N. Pyrosequencing-based assessment of soil pH as a predictor of soil bacterial community structure at the continental scale. Appl. Environ. Microbiol. 2009; 75 :5111–5120. [ PMC free article ] [ PubMed ] [ Google Scholar ]
- Lavelle P., Decaëns T., Aubert M., Sb Barot, Blouin M., Bureau F. Soil invertebrates and ecosystem services. Eur. J. Soil Biol. 2006; 42 :S3–S15. [ Google Scholar ]
- Le Quere C., Andrew R.M., Friedlingstein P., Sitch S., Hauck J., Pongratz J. Global carbon budget 2018. Earth System Science Data. 2018; 10 :2141–2194. [ Google Scholar ]
- Leff J.W., Jones S.E., Prober S.M., Barberan A., Borer E.T., Firn J.L. Consistent responses of soil microbial communities to elevated nutrient inputs in grasslands across the globe. Proc. Natl. Acad. Sci. U. S. A. 2015; 112 :10967–10972. [ PMC free article ] [ PubMed ] [ Google Scholar ]
- Lehmann J., Joseph S. Earthscan London; 2009. Biochar for Environmental Management. [ Google Scholar ]
- Li J., Liu Y., Hai X., Shangguan Z., Deng L. Dynamics of soil microbial C:N:P stoichiometry and its driving mechanisms following natural vegetation restoration after farmland abandonment. Sci. Total Environ. 2019; 693 [ PubMed ] [ Google Scholar ]
- Li X., Han H., Ning T., Shen Y., Lal R. Variations of SOC and MBC observed in an incubated brown loam soil managed under different tillage systems for 12 years. Soil Use Manag. 2019; 35 :585–594. [ Google Scholar ]
- Liu J., Li S., Ouyang Z., Tam C., Chen X. Ecological and socioeconomic effects of China’s policies for ecosystem services. Proc. Natl. Acad. Sci. U. S. A. 2008; 105 :9477–9482. [ PMC free article ] [ PubMed ] [ Google Scholar ]
- Lobry de Bruyn L.A. Learning opportunities: understanding farmers’ soil testing practice through workshop activities to improve extension support for soil health management. Soil Use Manag. 2019; 35 :128–140. [ Google Scholar ]
- Lochon I., Carrère P., Yvin J.-C., Houdusse-Lemenager D., Bloor J.M.G. Impacts of low-level liming on soil respiration and forage production in a fertilized upland grassland in Central France. Sci. Total Environ. 2019; 697 [ PubMed ] [ Google Scholar ]
- Ma L., Abuduwaili J., Li Y., Liu W. Anthropogenically disturbed potentially toxic elements in roadside topsoils of a suburban region of Bishkek, Central Asia. Soil Use Manag. 2019; 35 :283–292. [ Google Scholar ]
- Maguire R.O., Sims J.T. Soil testing to predict phosphorus leaching. J. Environ. Qual. 2002; 31 :1601–1609. [ PubMed ] [ Google Scholar ]
- Maia S.M.F., Gonzaga G.B.M., Silva LkdS, Lyra G.B., Gomes TcdA. Soil organic carbon temperature sensitivity of different soil types and land use systems in the Brazilian semi-arid region. Soil Use Manag. 2019; 35 :433–442. [ Google Scholar ]
- Maltas A., Kebli H., Oberholzer H.R., Weisskopf P., Sinaj S. The effects of organic and mineral fertilizers on carbon sequestration, soil properties, and crop yields from a long-term field experiment under a Swiss conventional farming system. Land Degrad. Dev. 2018; 29 :926–938. [ Google Scholar ]
- Manzoni S., Porporato A. Soil carbon and nitrogen mineralization: theory and models across scales. Soil Biol. Biochem. 2009; 41 :1355–1379. [ Google Scholar ]
- McInnes-Clarke S.K., Jenkins B.R., Rawson A., Murphy B.W. Sharing soil knowledge and evaluating progress in the New South Wales Soil Knowledge Network. Soil Use Manag. 2019; 35 :105–116. [ Google Scholar ]
- Mehra P., Baker J., Sojka R.E., Bolan N., Desbiolles J., Kirkham M.B. A review of tillage practices and their potential to impact the soil carbon dynamics. Adv. Agron. 2018; 150 :185–230. Elsevier. [ Google Scholar ]
- MEP . Ministry of Environmental Protection; Beijing, China: 2014. National Soil Contamination Survey Report. [ Google Scholar ]
- Mills J., Reed M., Skaalsveen K., Ingram J. The use of Twitter for knowledge exchange on sustainable soil management. Soil Use Manag. 2019; 35 :195–203. [ Google Scholar ]
- Montgomery D.R. Soil erosion and agricultural sustainability. Proc. Natl. Acad. Sci. U. S. A. 2007; 104 :13268–13272. [ PMC free article ] [ PubMed ] [ Google Scholar ]
- Montgomery D.R. Soil erosion and agricultural sustainability. Proc. Natl. Acad. Sci. 2007; 104 :13268–13272. [ PMC free article ] [ PubMed ] [ Google Scholar ]
- Morais R., Silva N., Mendes J., Adão T., Pádua L., López-Riquelme J. Mysense: a comprehensive data management environment to improve precision agriculture practices. Comput. Electron. Agric. 2019; 162 :882–894. [ Google Scholar ]
- Mossadeghi-Björklund M., Jarvis N., Larsbo M., Forkman J., Keller T. Effects of compaction on soil hydraulic properties, penetration resistance and water flow patterns at the soil profile scale. Soil Use Manag. 2019; 35 :367–377. [ Google Scholar ]
- Mugandani R., Mafongoya P. Behaviour of smallholder farmers towards adoption of conservation agriculture in Zimbabwe. Soil Use Manag. 2019; 35 (4):561–575. [ Google Scholar ]
- Nachimuthu G., Watkins M.D., Hulugalle N.R., Weaver T.B., Finlay L.A., McCorkell B.E. Leaching of dissolved organic carbon and nitrogen under cotton farming systems in a Vertisol. Soil Use Manag. 2019; 35 :443–452. [ Google Scholar ]
- Neher D.A. Role of nematodes in soil health and their use as indicators. J. Nematol. 2001; 33 :161. [ PMC free article ] [ PubMed ] [ Google Scholar ]
- Nielsen M.N., Winding A., Binnerup S. 2002. Microorganisms as Indicators of Soil Health. [ Google Scholar ]
- Obrist D., Agnan Y., Jiskra M., Olson C.L., Colegrove D.P., Hueber J. Tundra uptake of atmospheric elemental mercury drives Arctic mercury pollution. Nature. 2017; 547 :201. [ PubMed ] [ Google Scholar ]
- O’Connor D., Hou D. More haste, less speed in replenishing China’s groundwater. Nature. 2019; 569 :487. [ PubMed ] [ Google Scholar ]
- O’Connor D., Hou D., Ok Y.S., Song Y., Sarmah A., Li X. Sustainable in situ remediation of recalcitrant organic pollutants in groundwater with controlled release materials: a review. J. Control. Release. 2018; 283 :200–213. [ PubMed ] [ Google Scholar ]
- O’Connor D., Peng T., Li G., Wang S., Duan L., Mulder J. Sulfur-modified rice husk biochar: a green method for the remediation of mercury contaminated soil. Sci. Total Environ. 2018; 621 :819–826. [ PubMed ] [ Google Scholar ]
- O’Connor D., Peng T., Zhang J., Tsang D.C., Alessi D.S., Shen Z. Biochar application for the remediation of heavy metal polluted land: a review of in situ field trials. Sci. Total Environ. 2018; 619 :815–826. [ PubMed ] [ Google Scholar ]
- O’Connor D., Hou D., Ok Y.S., Mulder J., Duan L., Wu Q. Mercury speciation, transformation, and transportation in soils, atmospheric flux, and implications for risk management: a critical review. Environ. Int. 2019; 126 :747–761. [ PubMed ] [ Google Scholar ]
- O’Connor D., Pan S., Shen Z., Song Y., Jin Y., Wu W.-M. Microplastics undergo accelerated vertical migration in sand soil due to small size and wet-dry cycles. Environ. Pollut. 2019; 249 :527–534. [ PubMed ] [ Google Scholar ]
- O’Connor D., Hou D., Ok Y.S., Lanphear B.P. The effects of iniquitous lead exposure on health. Nature Sustainability. 2020; 3 :77–79. [ Google Scholar ]
- Oliver M.A., Gregory P. Soil, food security and human health: a review. Eur. J. Soil Sci. 2015; 66 :257–276. [ Google Scholar ]
- Oliver D.P., Li Y., Orr R., Nelson P., Barnes M., McLaughlin M.J. The role of surface charge and pH changes in tropical soils on sorption behaviour of per- and polyfluoroalkyl substances (PFASs) Sci. Total Environ. 2019; 673 :197–206. [ PubMed ] [ Google Scholar ]
- Ottoy S., Vanierschot L., Dondeyne S., Vancampenhout K., Hermy M., Van Orshoven J. The devil is in the detail: discrepancy between soil organic carbon stocks estimated from regional and local data sources in Flanders, Belgium. Soil Use Manag. 2019; 35 :421–432. [ Google Scholar ]
- Packer I.J., Chapman G.A., Lawrie J.W. On-ground extension of soil information to improve land management. Soil Use Manag. 2019; 35 :75–84. [ Google Scholar ]
- Paustian K., Lehmann J., Ogle S., Reay D., Robertson G.P., Smith P. Climate-smart soils. Nature. 2016; 532 :49–57. [ PubMed ] [ Google Scholar ]
- Pavan A.L.R., Ometto A.R. Ecosystem Services in Life Cycle Assessment: a novel conceptual framework for soil. Sci. Total Environ. 2018; 643 :1337–1347. [ PubMed ] [ Google Scholar ]
- Pimentel D., Harvey C., Resosudarmo P., Sinclair K., Kurz D., McNair M. Environmental and economic costs of soil erosion and conservation benefits. Science. 1995; 267 :1117–1123. [ PubMed ] [ Google Scholar ]
- Pulleman M., Creamer R., Hamer U., Helder J., Pelosi C., Peres G. Soil biodiversity, biological indicators and soil ecosystem services—an overview of European approaches. Curr. Opin. Environ. Sustain. 2012; 4 :529–538. [ Google Scholar ]
- Qi S., Luo J., O’Connor D., Wang Y., Hou D. A numerical model to optimize LNAPL remediation by multi-phase extraction. Sci. Total Environ. 2020; 718 [ PubMed ] [ Google Scholar ]
- Rafiq M.K., Bai Y., Aziz R., Rafiq M.T., Mašek O., Bachmann R.T. Biochar amendment improves alpine meadows growth and soil health in Tibetan plateau over a three year period. Sci. Total Environ. 2019:135296. [ PubMed ] [ Google Scholar ]
- Ramesh T., Bolan N.S., Kirkham M.B., Wijesekara H., Kanchikerimath M., Rao C.S. Soil organic carbon dynamics: impact of land use changes and management practices: a review. Adv. Agron. 2019; 156 :1–107. [ Google Scholar ]
- Repko A.F., Szostak R. SAGE Publications, Incorporated; 2020. Interdisciplinary Research: Process and Theory. [ Google Scholar ]
- Rinot O., Levy G.J., Steinberger Y., Svoray T., Eshel G. Soil health assessment: a critical review of current methodologies and a proposed new approach. Sci. Total Environ. 2019; 648 :1484–1491. [ PubMed ] [ Google Scholar ]
- Robinson D., Fraser I., Dominati E., Davíðsdóttir B., Jónsson J., Jones L. On the value of soil resources in the context of natural capital and ecosystem service delivery. Soil Sci. Soc. Am. J. 2014; 78 :685–700. [ Google Scholar ]
- Robinson N.J., Dahlhaus P.G., Wong M., MacLeod A., Jones D., Nicholson C. Testing the public–private soil data and information sharing model for sustainable soil management outcomes. Soil Use Manag. 2019; 35 :94–104. [ Google Scholar ]
- Rojas R.V., Achouri M., Maroulis J., Caon L. Healthy soils: a prerequisite for sustainable food security. Environ. Earth Sci. 2016; 75 :180. [ Google Scholar ]
- Rumpel C., Amiraslani F., Koutika L.-S., Smith P., Whitehead D., Wollenberg E. Put more carbon in soils to meet Paris climate pledges. Nature. 2018; 564 :32–34. [ PubMed ] [ Google Scholar ]
- Saikia R., Sharma S., Thind H.S., Singh Y. Tillage and residue management practices affect soil biological indicators in a rice-wheat cropping system in north-western India. Soil Use Manag. 2020; 36 :157–172. [ Google Scholar ]
- Sanchez P.A. Soil fertility and hunger in Africa. Science. 2002; 295 :2019–2020. [ PubMed ] [ Google Scholar ]
- Schuur E.A., Abbott B., Bowden W., Brovkin V., Camill P., Canadell J. Expert assessment of vulnerability of permafrost carbon to climate change. Clim. Chang. 2013; 119 :359–374. [ Google Scholar ]
- Schuur E.A., McGuire A.D., Schädel C., Grosse G., Harden J., Hayes D.J. Climate change and the permafrost carbon feedback. Nature. 2015; 520 :171–179. [ PubMed ] [ Google Scholar ]
- Schwenke G., Beange L., Cameron J., Bell M., Harden S. What soil information do crop advisors use to develop nitrogen fertilizer recommendations for grain growers in New South Wales, Australia? Soil Use Manag. 2019; 35 :85–93. [ Google Scholar ]
- Senbayram M., Saygan E.P., Chen R., Aydemir S., Kaya C., Wu D. Effect of biochar origin and soil type on the greenhouse gas emission and the bacterial community structure in N fertilised acidic sandy and alkaline clay soil. Sci. Total Environ. 2019; 660 :69–79. [ PubMed ] [ Google Scholar ]
- Shen Z., Jin F., O’Connor D., Hou D. Solidification/stabilization for soil remediation: an old technology with new vitality. Environmental Science & Technology. 2019; 53 :11615–11617. [ PubMed ] [ Google Scholar ]
- Song Y., Kirkwood N., Maksimovic C., Zhen X., O’Connor D., Jin Y. Nature based solutions for contaminated land remediation and brownfield redevelopment in cities: a review. Sci. Total Environ. 2019; 663 :568–579. [ PubMed ] [ Google Scholar ]
- Stoate C., Jones S., Crotty F., Morris C., Seymour S. Participatory research approaches to integrating scientific and farmer knowledge of soil to meet multiple objectives in the English east midlands. Soil Use Manag. 2019; 35 :150–159. [ Google Scholar ]
- Su C., Liu H., Wang S. A process-based framework for soil ecosystem services study and management. Sci. Total Environ. 2018; 627 :282–289. [ PubMed ] [ Google Scholar ]
- Tavares R.L.M., de Souza Z.M., Siqueira D.S., La Scala Júnior N., Panosso A.R., Campos M.C.C. Soil CO2 emission in sugarcane management systems. Acta Agriculturae Scandinavica, Section B—Soil & Plant Science. 2015; 65 :755–762. [ Google Scholar ]
- Teng M., Huang C., Wang P., Zeng L., Zhou Z., Xiao W. Impacts of forest restoration on soil erosion in the Three Gorges Reservoir area, China. Sci. Total Environ. 2019; 697 [ PubMed ] [ Google Scholar ]
- Tisdale S.L., Nelson W.L., Beaton J.D. Collier Macmillan Publishers; 1985. Soil Fertility and Fertilizers. [ Google Scholar ]
- Totsche K.U., Rennert T., Gerzabek M.H., Kögel-Knabner I., Smalla K., Spiteller M. Biogeochemical interfaces in soil: the interdisciplinary challenge for soil science. J. Plant Nutr. Soil Sci. 2010; 173 :88–99. [ Google Scholar ]
- Tulau M.J., McInnes-Clarke S.K., Yang X., McAlpine R.A., Karunaratne S.B., Zhu Q. The Warrumbungle post-fire recovery project—raising the profile of soils. Soil Use Manag. 2019; 35 :63–74. [ Google Scholar ]
- Ukalska-Jaruga A., Klimkowicz-Pawlas A., Smreczak B. Characterization of organic matter fractions in the top layer of soils under different land uses in Central-Eastern Europe. Soil Use Manag. 2019; 35 :595–606. [ Google Scholar ]
- UN . 2015. Transforming our World: The 2030 Agenda for Sustainable Development A/RES/70/1. [ Google Scholar ]
- UN . 2019. The Sustainable Development Goals Report, New York. [ Google Scholar ]
- UNCCD . 2017. Global Land Outlook. [ Google Scholar ]
- Van Bruggen A.H., Semenov A.M. In search of biological indicators for soil health and disease suppression. Appl. Soil Ecol. 2000; 15 :13–24. [ Google Scholar ]
- Vogel H.-J., Bartke S., Daedlow K., Helming K., Kögel-Knabner I., Lang B. A systemic approach for modeling soil functions. Soil. 2018; 4 :83. [ Google Scholar ]
- Wang J., Liu X., Li Y., Powell T., Wang X., Wang G. Microplastics as contaminants in the soil environment: a mini-review. Sci. Total Environ. 2019; 691 :848–857. [ PubMed ] [ Google Scholar ]
- Wang L., Hou D., Shen Z., Zhu J., Jia X., Ok Y.S. Field trials of phytomining and phytoremediation: a critical review of influencing factors and effects of additives. Crit. Rev. Environ. Sci. Technol. 2019:1–51. [ Google Scholar ]
- Wang L., Hou D., Cao Y., Ok Y.S., Tack F.M., Rinklebe J. Remediation of mercury contaminated soil, water, and air: a review of emerging materials and innovative technologies. Environ. Int. 2020; 134 [ PubMed ] [ Google Scholar ]
- Wang L., Li X., Tsang D.C., Jin F., Hou D. Green remediation of Cd and Hg contaminated soil using humic acid modified montmorillonite: immobilization performance under accelerated ageing conditions. J. Hazard. Mater. 2020:122005. [ PubMed ] [ Google Scholar ]
- Wang L., Ok Y.S., Tsang D.C., Alessi D.S., Rinklebe J., Wang H. New trends in biochar pyrolysis and modification strategies: feedstock, pyrolysis conditions, sustainability concerns and implications for soil amendment. Soil Use Manag. 2020 doi: 10.1111/sum.12592. (In Press) [ CrossRef ] [ Google Scholar ]
- Welten B.G., Ledgard S.F., Judge A.A., Sprosen M.S., McGowan A.W., Dexter M.M. Efficacy of different temperate pasture species to reduce nitrogen leaching from cattle urine applied in different seasons: a soil lysimeter study. Soil Use Manag. 2019; 35 :653–663. [ Google Scholar ]
- Wick A.F., Haley J., Gasch C., Wehlander T., Briese L., Samson-Liebig S. Network-based approaches for soil health research and extension programming in North Dakota, USA. Soil Use Manag. 2019; 35 :177–184. [ Google Scholar ]
- Wilcke W. Synopsis polycyclic aromatic hydrocarbons (PAHs) in soil—a review. J. Plant Nutr. Soil Sci. 2000; 163 :229–248. [ Google Scholar ]
- Wolfert S., Ge L., Verdouw C., Bogaardt M.-J. Big data in smart farming–a review. Agric. Syst. 2017; 153 :69–80. [ Google Scholar ]
- Woolf D., Amonette J.E., Street-Perrott F.A., Lehmann J., Joseph S. Sustainable biochar to mitigate global climate change. Nat. Commun. 2010; 1 :56. [ PMC free article ] [ PubMed ] [ Google Scholar ]
- Wu T., Ayres E., Bardgett R.D., Wall D.H., Garey J.R. Molecular study of worldwide distribution and diversity of soil animals. Proc. Natl. Acad. Sci. 2011; 108 :17720–17725. [ PMC free article ] [ PubMed ] [ Google Scholar ]
- Xiao J., Wang L., Deng L., Jin Z. Characteristics, sources, water quality and health risk assessment of trace elements in river water and well water in the Chinese Loess Plateau. Sci. Total Environ. 2019; 650 :2004–2012. [ PubMed ] [ Google Scholar ]
- Xu L., Wang M., Tian Y., Shi X., Shi Y., Yu Q. Changes in soil macropores: superposition of the roles of organic nutrient amendments and the greenhouse pattern in vegetable plantations. Soil Use Manag. 2019; 35 :412–420. [ Google Scholar ]
- Yagi K., Minami K. Effect of organic matter application on methane emission from some Japanese paddy fields. Soil science and plant nutrition. 1990; 36 :599–610. [ Google Scholar ]
- Ye L., Camps-Arbestain M., Shen Q., Lehmann J., Singh B., Sabir M. Biochar effects on crop yields with and without fertilizer: a meta-analysis of field studies using separate controls. Soil Use Manag. 2020; 36 :2–18. [ Google Scholar ]
- Yu H., Zha T., Zhang X., Ma L. Vertical distribution and influencing factors of soil organic carbon in the Loess Plateau, China. Sci. Total Environ. 2019; 693 [ PubMed ] [ Google Scholar ]
- Zhang W.-F., Z-x Dou, He P., Ju X.-T., Powlson D., Chadwick D. New technologies reduce greenhouse gas emissions from nitrogenous fertilizer in China. Proc. Natl. Acad. Sci. U. S. A. 2013; 110 :8375–8380. [ PMC free article ] [ PubMed ] [ Google Scholar ]
- Zhang Y., Hou D., O’Connor D., Shen Z., Shi P., Ok Y.S. Lead contamination in Chinese surface soils: source identification, spatial-temporal distribution and associated health risks. Crit. Rev. Environ. Sci. Technol. 2019; 49 (15) [ Google Scholar ]
- Zhang J., Hou D., Shen Z., Jin F., O’Connor D., Pan S. Effects of excessive impregnation, magnesium content, and pyrolysis temperature on MgO-coated watermelon rind biochar and its lead removal capacity. Environ. Res. 2020; 183 :109152. [ PubMed ] [ Google Scholar ]
- Zhang Y., O’Connor D., Xu W., Hou D. Blood lead levels among Chinese children: the shifting influence of industry, traffic, and e-waste over three decades. Environ. Int. 2020; 135 [ PubMed ] [ Google Scholar ]
- Zhao H., Ning P., Chen Y., Liu J., Ghaffar S.A., Xiaohong T. Effect of straw amendment modes on soil organic carbon, nitrogen sequestration and crop yield on the North-Central Plain of China. Soil Use Manag. 2019; 35 :511–525. [ Google Scholar ]
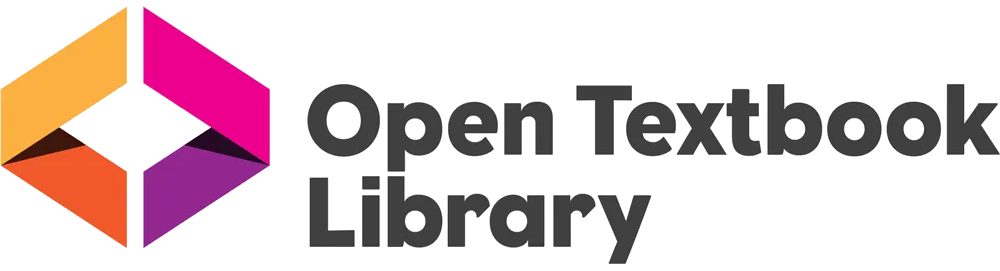
Introduction to Soil Science
(0 reviews)
Amber Anderson, Iowa State University
Copyright Year: 2023
Publisher: Iowa State University Digital Press
Language: English
Formats Available
Conditions of use.

Table of Contents
- Introduction
- Getting started
- Soil physical properties
- Soil erosion
- Soil chemistry
- Soil management
- Soil Fertility
- Case studies
- Soil Geography
Ancillary Material
About the book.
This textbook introduces readers to the basics of soil science, including the physical, chemical, and biological properties of soils; soil formation, classification, and global distribution; soil health, soils and humanity, and sustainable land management.
About the Contributors
Dr. Amber Anderson , Iowa State University
Contribute to this Page
Business Management Ideas

Term Paper on Soil: Introduction, Properties & Formation | Soil Science
ADVERTISEMENTS:
Here is a term paper on ‘Soil’. Find paragraphs, long and short term papers on ‘Soil’ especially written for school and college students.
Term Paper on Soil
Term paper # 1. introduction to soil :.
Soil has been defined differently by workers in different fields. Thus, for an agriculturist, the soil is simply the upper layer of ground which is useful for supporting life. Geologists define it as a surface layer of rock waste in which the physical and chemical processes of rock weathering cooperate intimately with organic processes.
In a technical way, however, the term soil may be defined as “the upper layer of the ground made of unconsolidated material produced due to weathering agencies from the rocks and generally modified subsequently by a variety of mechanical, chemical and organic processes all operating constantly in a complex manner.”
The branch of science dealing with soils is called pedology. It embraces the study of formation and development of soils as also soil mapping.
Constitution :
The essential constituents of soil are:
(i) The solid matter
(ii) Air and water
(i) The Solid Matter:
It consists chiefly of inorganic particles derived from rocks and minerals. These particles are of different size and composition and reflect the type of parent rocks from which the soils are derived. Organic matter is also present in many soils in subordinate proportion. It is derived from roots and remains of life forms that live on or within the soils such as rodents, worms, insects and bacteria.
(ii) Air and Water:
The solid particles in a lump of soil are seldom closely packed. In fact, soils are generally characterised by a porous texture and the pores between the solid particles may be filled completely or partially by air (various gases) or water or by both. The soil water is in fact a complex chemical solution having many essential nutrients and other components which are very important in the biochemical and organic processes taking place in the soils. Similarly, many engineering properties of soil are due to the presence and proportion of air and water in the soils.
Term Paper # 2. Physical Properties of Soil :
It is an important property that has been commonly used by farmers and scientists alike for a broad classification of soils e.g. red soils, grey soils, and black soils and so on. Colour of the soil depends upon its composition, drainage condition and also on its age. Thus, presence of humus (decayed organic material) in good proportion will impart a black or deep brown colour to the soil.
Similarly, finely dispersed iron oxide is responsible for the red colour and iron hydroxide for the yellow colour of the soils. Absence of these components may result in colourless or grey soils. Further, effective drainage may result in dilution of the original colours whereas time may make a soil more mature and concentrate the original colour if there is not much drainage.
2. Texture:
In soils, texture is understood to define their composition and make up in terms of particle size of the solid components. (Fig. 21.1).

We’re fighting to restore access to 500,000+ books in court this week. Join us!
Internet Archive Audio
- This Just In
- Grateful Dead
- Old Time Radio
- 78 RPMs and Cylinder Recordings
- Audio Books & Poetry
- Computers, Technology and Science
- Music, Arts & Culture
- News & Public Affairs
- Spirituality & Religion
- Radio News Archive
- Flickr Commons
- Occupy Wall Street Flickr
- NASA Images
- Solar System Collection
- Ames Research Center
- All Software
- Old School Emulation
- MS-DOS Games
- Historical Software
- Classic PC Games
- Software Library
- Kodi Archive and Support File
- Vintage Software
- CD-ROM Software
- CD-ROM Software Library
- Software Sites
- Tucows Software Library
- Shareware CD-ROMs
- Software Capsules Compilation
- CD-ROM Images
- ZX Spectrum
- DOOM Level CD

- Smithsonian Libraries
- FEDLINK (US)
- Lincoln Collection
- American Libraries
- Canadian Libraries
- Universal Library
- Project Gutenberg
- Children's Library
- Biodiversity Heritage Library
- Books by Language
- Additional Collections
- Prelinger Archives
- Democracy Now!
- Occupy Wall Street
- TV NSA Clip Library
- Animation & Cartoons
- Arts & Music
- Computers & Technology
- Cultural & Academic Films
- Ephemeral Films
- Sports Videos
- Videogame Videos
- Youth Media
Search the history of over 866 billion web pages on the Internet.
Mobile Apps
- Wayback Machine (iOS)
- Wayback Machine (Android)
Browser Extensions
Archive-it subscription.
- Explore the Collections
- Build Collections
Save Page Now
Capture a web page as it appears now for use as a trusted citation in the future.
Please enter a valid web address
- Donate Donate icon An illustration of a heart shape
Essential soil science : a clear and concise introduction to soil science
Bookreader item preview, share or embed this item, flag this item for.
- Graphic Violence
- Explicit Sexual Content
- Hate Speech
- Misinformation/Disinformation
- Marketing/Phishing/Advertising
- Misleading/Inaccurate/Missing Metadata
![[WorldCat (this item)] [WorldCat (this item)]](https://archive.org/images/worldcat-small.png)
plus-circle Add Review comment Reviews
5 Favorites
Better World Books
DOWNLOAD OPTIONS
No suitable files to display here.
IN COLLECTIONS
Uploaded by station22.cebu on January 14, 2022
SIMILAR ITEMS (based on metadata)

Glossary of Soil Science Terms
Term of the week.
hydraulic head : Refer to soil water and total head.
This Is What Happens To Trees When They Take In Excess CO2
Oak trees accumulate more wood when there is more carbon dioxide (CO₂) in the atmosphere.
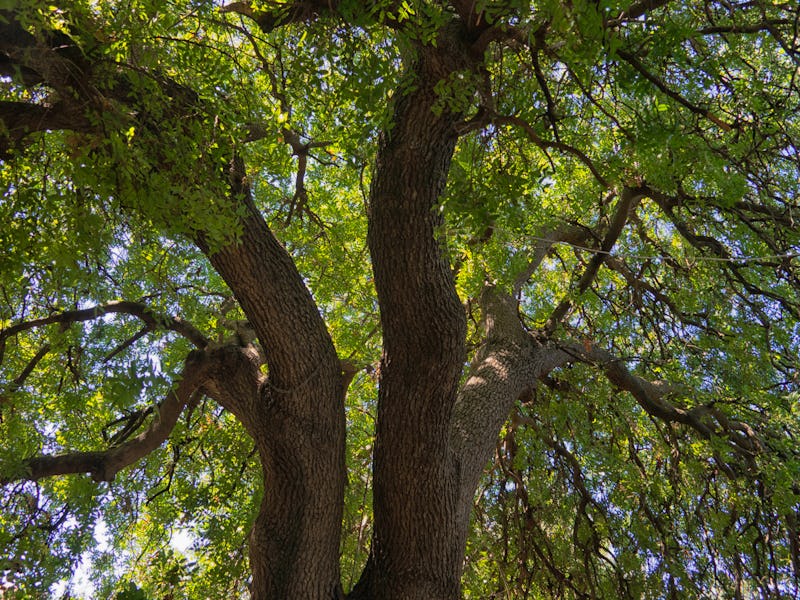
Oak trees accumulate more wood when there is more carbon dioxide (CO₂) in the atmosphere. That’s the key finding from our new study , carried out in a long-established forest in Staffordshire, England, that we have turned into a huge field experiment by injecting with extra CO₂. After we increased CO₂ levels to what will be the planetary level in the 2050s, trees took more of it from the atmosphere and their wood production increased by 10 percent.
In some ways, this result is reassuring. We know that more CO₂ in the atmosphere can often help plants grow bigger and faster since photosynthesis captures the carbon from which plants are largely made. However, until now, the only comparable study on an older, mature forest (an Australian eucalyptus forest ) found no link between extra CO₂ and tree growth. Our work shows the link really does exist — at least in some common broadleaf forests.
However, woodier trees do not offer a silver bullet to solve climate change. While carbon is certainly better off in trees than in the atmosphere, where it causes global warming, it’s not a long-term solution. Over decades or centuries, wood rots away, and carbon dioxide is released back into the atmosphere. So, as a store of carbon, trees are not remotely equivalent to it being locked away in coal seams and oil reservoirs deep underground.
To study what even more CO₂ in the atmosphere will do to trees in future, we used a technique called Free-Air CO2 Enrichment (Face) . This involves installing eight-story high pipes on the upwind side of various patches of forest, and then gently releasing air infused with extra CO₂. We then monitor the trees to see if the CO₂ has had any effect.
Our new study uses patches of 180-year-old English or “pedunculate” oak ( Quercus robur ). These trees are much older than those studied previously to see how they will behave in the atmosphere of the future.
Some forests can’t make use of extra carbon
Why did our research on oak trees generate different results from the research on Australian eucalyptus, which found no link between CO₂ and wood growth?
The difference almost certainly lies in the nutritional condition of the two forests. Trees in forests need a balance of nitrogen (N) and phosphorus (P) and a host of “micro-nutrients” if they are to grow well. The Australian forest grows in ancient, “highly weathered” soil where any extra phosphorus is in very short supply so that, even when given an extra supply of carbon “for free,” the trees are unable to use it to lay down more plant material.
Such intense nutrient shortage does not exist in our oak forest, so the experiment more directly tests whether the trees can make use of the new “free” carbon resource. And it seems they can, especially to form wood.
Although every forest is unique in one way or another, our results are likely to be relevant to many of the world’s “temperate deciduous broadleaf” forests — those that are neither exceptionally hot nor cold and which drop their leaves each year.
The Australian results perhaps point to how trees will respond in other phosphorus-limited environments, such as tropical rainforests. Scientists in Brazil are beginning a similar experiment at the Amazon Face facility, which comes on-stream later this year. That should add another crucial piece to the global forest carbon jigsaw, though other Face facilities elsewhere are also needed .
We did look for changes in other tissues — leaves, fine roots and seeds — in trees with elevated CO₂, but any extra growth was minimal and not distinguishable from measurement uncertainties. In any case, these other tissues turn back into atmospheric CO₂ more quickly than woody tissue. So it is lucky that the extra carbon is being stored in “woodier trees” since the world’s hopes for avoiding disastrous climate change are pinned on forests offsetting essential fossil-fuel use.
This article was originally published on The Conversation by Rob MacKenzie at the University of Birmingham and Richard Norby at the University of Tennessee Knoxville and University of Birmingham. Read the original article here .
- Environment
- Climate Crisis

South Dakota researchers lead NASA-funded soil moisture mapping project

This interview originally aired on "In the Moment" on SDPB Radio.
Agricultural producers who need to know vital information about their farmland can look to the skies.
South Dakota researchers are using satellite data to more accurately map soil moisture. The NASA-funded project is seeking to create new algorithms that uses AI to create larger, more accurate maps.
Hankui Zhang, Ph.D., is an assistant professor at South Dakota State University. He's the project's primary science investigator and joins us to discuss the potential impacts of this project for South Dakota's farmers.

- Share full article

Opinion Guest Essay
The Soul of Soil
Credit... Sarah Meadows
Supported by
By Ferris Jabr
Mr. Jabr is the author of “ Becoming Earth: How Our Planet Came to Life .”
- Aug. 4, 2024
When my partner and I bought our home in Portland, Ore., four years ago, we immediately began designing our dream garden, intending to replace a derelict grass lawn with ample beds of lush, long-blooming perennials. We soon discovered, however, that our soil was unyielding, clay-heavy and strewed with rubble. In previous, much tinier gardens, I’d circumvented such difficulties with a few bags of high-quality soil from the nursery. Replacing this vastly greater quantity of dirt was neither practical nor financially feasible. Instead, I resolved to remediate what we already had.
Learning how to do so transformed much more than our yard — it completely changed the way I think about soil, and about our planet as a whole. I now see soil not simply as a medium for life, but as a living entity in its own right — one that is rapidly going extinct.
In some parts of the world, intensive farming, overgrazing and deforestation are destroying soil up to 1,000 times as fast as the base line rate of erosion. If current trends continue, 90 percent of the planet’s habitable land areas could be substantially degraded by 2050, causing crop yields to drop by an average of 10 percent — and up to 50 percent in some areas — and most likely forcing up to hundreds of millions of people to migrate.
The eradication of soil could culminate in the collapse of complex terrestrial life — unless we rethink our relationship to the world beneath our feet.
Soil is the result of eons of planetary evolution — billions of years of the elements weathering rock and more than 425 million years of interactions with complex life. A single inch of fertile topsoil requires centuries to develop.
Microbes, fungi, plants and animals create and maintain soil through myriad processes: by breaking apart rock with roots and secreted acids; enriching fragmented rock with their own remains and byproducts; and circulating air, water and nutrients via crawling, slithering and burrowing.
We are having trouble retrieving the article content.
Please enable JavaScript in your browser settings.
Thank you for your patience while we verify access. If you are in Reader mode please exit and log into your Times account, or subscribe for all of The Times.
Thank you for your patience while we verify access.
Already a subscriber? Log in .
Want all of The Times? Subscribe .
Advertisement
- You are here:
- American Chemical Society
- Discover Chemistry
- Science Releases
Rising mercury pollution in soil could be related to climate change, study says
FOR IMMEDIATE RELEASE
“Warming-Induced Vegetation Greening May Aggravate Soil Mercury Levels Worldwide” Environmental Science & Technology
In 2017, the Minamata Convention on Mercury went into effect, designed to help curb mercury emissions and limit exposure across the globe. However, a new study of mercury levels in soil suggests that the treaty’s provisions might not be enough. The study published in ACS’ Environmental Science & Technology estimates that soil stores substantially more mercury than previously thought, and it predicts that increases in plant growth due to climate change may add even more.

Mercury is a persistent environmental pollutant, moving through air, water and soil, and accumulating within plants and animals. Soil is the primary reservoir for mercury, storing three times the amount found in the oceans and 150 times the amount found in the atmosphere. Typically, the heavy metal naturally moves through these reservoirs, but humans have altered this cycling. Human-caused climate change increases carbon dioxide levels, promoting vegetation growth and most likely depositing more mercury in the soil when the vegetation decomposes. Previous studies on soil mercury levels have mostly focused on small, regional scales. But Xuejun Wang, Maodian Liu and colleagues wanted to develop a more accurate, worldwide model of soil mercury levels that could take into account the effects of a continuously warming climate.
The team began by compiling nearly 19,000 previously published soil mercury measurements, producing one of the largest databases of its kind. The dataset was fed into a machine learning algorithm to estimate the global distribution of mercury in both topsoil and subsoil. They found that the total amount of mercury stored in the first 40 inches (around 1 meter) of soil is approximately 4.7 million tons. This value is double what some previous estimates concluded, though some of those studies accounted for a shallower depth of soil. The team’s model identified the highest levels of mercury in plant-dense areas such as low latitudes of the tropics, but also in permafrost and areas with high human density. Conversely, bare land such as shrubland or grassland had relatively low levels of soil mercury.
To understand how climate warming could affect mercury soil levels, the researchers combined their initial model with datasets of environmental factors representing future climate scenarios. Their model predicts that as temperatures increase around the globe, vegetation growth will be promoted as well, which could raise soil mercury levels in turn. This symbiotic effect would outweigh the reduction efforts proposed by current worldwide control schemes, like those in the Minamata Convention. Though additional research and observations are needed, the researchers say that this work emphasizes the need for stricter, long-term and simultaneous control of mercury and carbon dioxide emissions.
The authors acknowledge funding from the National Natural Science Foundation of China; the High-Performance Computing Platform of Peking University; the Beijing Natural Science Foundation; the China Postdoctoral Science Foundation; and the Fundamental Research Funds for the Central Universities, Peking University.
The American Chemical Society (ACS) is a nonprofit organization chartered by the U.S. Congress. ACS’ mission is to advance the broader chemistry enterprise and its practitioners for the benefit of Earth and all its people. The Society is a global leader in promoting excellence in science education and providing access to chemistry-related information and research through its multiple research solutions, peer-reviewed journals, scientific conferences, eBooks and weekly news periodical Chemical & Engineering News . ACS journals are among the most cited, most trusted and most read within the scientific literature; however, ACS itself does not conduct chemical research. As a leader in scientific information solutions, its CAS division partners with global innovators to accelerate breakthroughs by curating, connecting and analyzing the world’s scientific knowledge. ACS’ main offices are in Washington, D.C., and Columbus, Ohio.
To automatically receive press releases from the American Chemical Society, contact newsroom@acs.org .
Note: ACS does not conduct research, but publishes and publicizes peer-reviewed scientific studies.
Media Contact
ACS Newsroom newsroom@acs.org
Related Content

More From This Series

Accept & Close The ACS takes your privacy seriously as it relates to cookies. We use cookies to remember users, better understand ways to serve them, improve our value proposition, and optimize their experience. Learn more about managing your cookies at Cookies Policy .
1155 Sixteenth Street, NW, Washington, DC 20036, USA | service@acs.org | 1-800-333-9511 (US and Canada) | 614-447-3776 (outside North America)
- Terms of Use
- Accessibility
Copyright © 2024 American Chemical Society

COMMENTS
1435-0661. 0361-5995. Soil Science Society of America Journal publishes basic and applied soil research covering all areas of soil science in agricultural, forest, wetlands, and urban settings. Soil Science Society of America Journal is an international, comprehensive venue for interdisciplinary soil scientists, biogeochemists, and agronomists ...
A myriad of challenges faces soil science at the beginning of the 2020s. The main aim of this overview is to assess past achievements and current challenges regarding soil threats such as erosion and soil contamination related to different United Nations sustainable development goals (SDGs) including (1) sustainable food production, (2) ensure ...
The book is divided into five parts - soil science, soil physics, soil chemistry, soil biology and soil environment. The first part "Soil Science" serves as the introduction to the book and discusses some common topics such as soil formation, mineralogy, taxonomy, quality and analytical techniques. The second part "Soil Physics" is ...
This editorial was written by early career scientists in soil science and gives an overview of the articles published in the special issue "Soil Science Challenges—An Interdisciplinary Overview of Current and Future Topics" on the occasion of the 100th anniversary of Journal of Plant Nutrition and Soil Science.A broad range of articles addressing soil carbon dynamics from the microscale ...
Soil science has arguably shifted from a discipline largely concerned with the fundamental mechanics of soil systems (soil physics, soil biology, soil chemistry, soil hydrology, etc.), to one more focused on confronting contemporary environmental challenges (Hartemink & McBratney, 2008). The importance of, and need to understand, the components ...
Abstract. Soil health is the continued capacity of soil to function as a vital living ecosystem that sustains plants, animals and humans, and connects agricultural and soil science to policy ...
Soil structure and aggregate characteristics are core features determining soil health and functionality. Employing a soil column experimental system, Liu et al.'s study revealed that polymer γ-PGA amendment would increase the mean weight diameter (MWD) and the portion of large aggregates (particle size larger than 0.25 mm) of both sandy and loam soils subjected to wetting-drying (WD) cycles.
Soil degradation negatively affects the well-being of over 3 billion people, costing more than 10% of annual global GDP via the loss of ecosystem services, and reducing the productivity of 23% of the global terrestrial area. The sustainable management of soil ecosystems is therefore fundamental to global food, water and energy security ...
Erosion is defined by the Soil Science Society of America (SSSA 2008, 19) as: "(i) The wearing away of the land surface by rain or irrigation water, wind, ice, or other natural or anthropogenic agents that abrade, detach, and remove geologic parent material or soil from one point on the earth's surface and deposit it elsewhere, including such ...
As one of the basic disciplines of agricultural, natural resource, and environmental science, soil science has played a critical role in global food security and socio-economic and ecological sustainability. The number of soil science journals and publications has increased remarkably with the development of soil science. However, there is a lack of systematic and comprehensive studies on the ...
A soil incubation study was carried out in the Department of Soil Science and Agricultural Chemistry, to assess dynamics of micronutrient release. Soil pots were filled with 5 kg air-dried, 2 mm ...
ABSTRACT: Transdisciplinary approaches that provide holistic views are essential to properly understand soil processes and the importance. of soil to society and will be crucial in the future to ...
For instance, three soil types of different initial soil pH, namely, Wodjil sandy loam with pH(CaCl 2) 3.87, Bodallin sandy loam soil with pH 4.54, and Lancelin sandy soil with pH 5.06, were incubated with residues of chickpea, lucerne, medic, high-N wheat, and low-N wheat. Thereafter, the pH increased by about 3.3 units with lucerne in the ...
Within the last decade, considerable efforts have been made to define an agenda for soil science research (35; 59) by proposing institutional, professional, and educational changes that can enhance the relevance and recognition of soil science in contemporary society (8; 51). Through these efforts, themes and future directions for soil science ...
Soil science is one of the land-related disciplines and has an essential linked with several SDGs (Keesstra et al., 2016). One of the land degradation issues related to the soil that has attract different stakeholder attention is soil erosion. Soil erosion is a global problem and rise as one of the major issues in many countries.
This paper is based on an extensive review of soil fertility in the literature of soil science, agronomy and ethnic studies. The spectrum of scientific opinions on soil fertility was visualized in ...
The application of machine learning (ML) techniques in various fields of science has increased rapidly, especially in the last 10 years. Soil science research, in particular, pedometrics, has used statistical models to "learn" or understand from data how soil is distributed in space and time (McBratney et al., 2019).The increasing availability of soil data that can be efficiently acquired ...
It is imperative to disseminate soil science knowledge to policy makers and practitioners who design and implement SDG programs (see Section 3).Effective action needs to be taken by the soil science community to help develop suitable indicators that are not only scientifically sound, but also practical for small hold farmers and other stakeholders.
About the Book. This textbook introduces readers to the basics of soil science, including the physical, chemical, and biological properties of soils; soil formation, classification, and global distribution; soil health, soils and humanity, and sustainable land management.
Term Paper # 2. Physical Properties of Soil: 1. Colour: ADVERTISEMENTS: It is an important property that has been commonly used by farmers and scientists alike for a broad classification of soils e.g. red soils, grey soils, and black soils and so on. Colour of the soil depends upon its composition, drainage condition and also on its age.
Access-restricted-item true Addeddate 2022-01-31 05:09:02 Associated-names Puri, Geeta, 1967- Boxid
TERM OF THE WEEK. [soil taxonomy] A surface layer of mineral soil that has been modified by human activity and has the same requirements as the mollic epipedon with respect to color, thickness, organic carbon content, consistence, and base saturation but that has>110 mg P kg -1 soluble in 0.05 M citric acid, or is dry>300 days (cumulative ...
1 INTRODUCTION. The United Nations declared 2021 to 2030 the Decade on Ecosystem Restoration (Fischer et al., 2021; Robinson et al., 2022), which reflects the urgency with which we must restore degraded ecosystems.Soil is foundational to most terrestrial ecosystems (Thies & Grossman, 2023) and is being degraded at an unprecedented rate, largely due to unsustainable human activities (e.g ...
Gypseous (particle-size substitute class).—A family class term used instead of a particle-size class for soils with a high content of the mineral gypsum. The soil contains 40 percent or more, by weight, gypsum in the part consisting of particles less than 20 mm in diameter (e.g., medium gravel and finer).
Mercury, a neurotoxic substance, circulates globally, significantly stored in soils through atmospheric deposition and plant decay. Despite being deposited, mercury can be remobilized and released into the atmosphere and water, enhancing its global cycle. Recent research suggests that climate warming may amplify the remobilization of soil mercury, facilitating its incorporation into food webs ...
Under conditions of low soil Cd concentration (Cd ≤ 1 mg·kg −1), the Cd content in rice seeds from the group subjected to the combined treatment remained below the national standard (Cd ≤ 0.2 mg·kg −1). Furthermore, the combined treatment modulated the uptake of Fe and Zn by rice, while simultaneously suppressing the expression of ...
The Australian forest grows in ancient, "highly weathered" soil where any extra phosphorus is in very short supply so that, even when given an extra supply of carbon "for free," the trees ...
South Dakota researchers are using satellite data to more accurately map soil moisture. The NASA-funded project is seeking to create new algorithms that uses AI to create larger, more accurate maps. Hankui Zhang, Ph.D., is an assistant professor at South Dakota State University. He's the project's primary science investigator and joins us to ...
Even the Soil Science Society of America and the U.S.D.A. have characterized soil as alive. Recognizing soil as a form of life reframes our perception of another interconnected, self-regulating ...
The study published in ACS' Environmental Science & Technology estimates that soil stores substantially more mercury than previously thought, and it predicts that increases in plant growth due to climate change may add even more. Tropical jungles, such as this one in Southeast Asia, currently contain some of the highest levels of mercury in ...