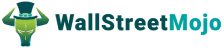
Trending Courses
Course Categories
Certification Programs
Free Courses
Investment Banking Resources
- Career Guides
- Interview Prep Guides
- Free Practice Tests
- Excel Cheatsheets
By Dheeraj Vaidya, CFA, FRM
(ex. J.P. Morgan & CLSA Equity Analyst with 20+ years of training experience)
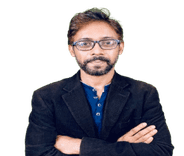

Investment Banking Case Studies
Last Updated :
21 Aug, 2024
Blog Author :
Wallstreetmojo Team
Edited by :
Ashish Kumar Srivastav
Reviewed by :
Dheeraj Vaidya, CFA, FRM
Table Of Contents
What Are Investment Banking Case Studies?
Investment banking case studies are a part of the hiring process of the investment banking sector. This is the round of interview where organizations try to assess the ability of an individual to work efficiently on a particular real-life scenario. Interviewers provide candidates a theoretical situation and seek answers to questions that follow.
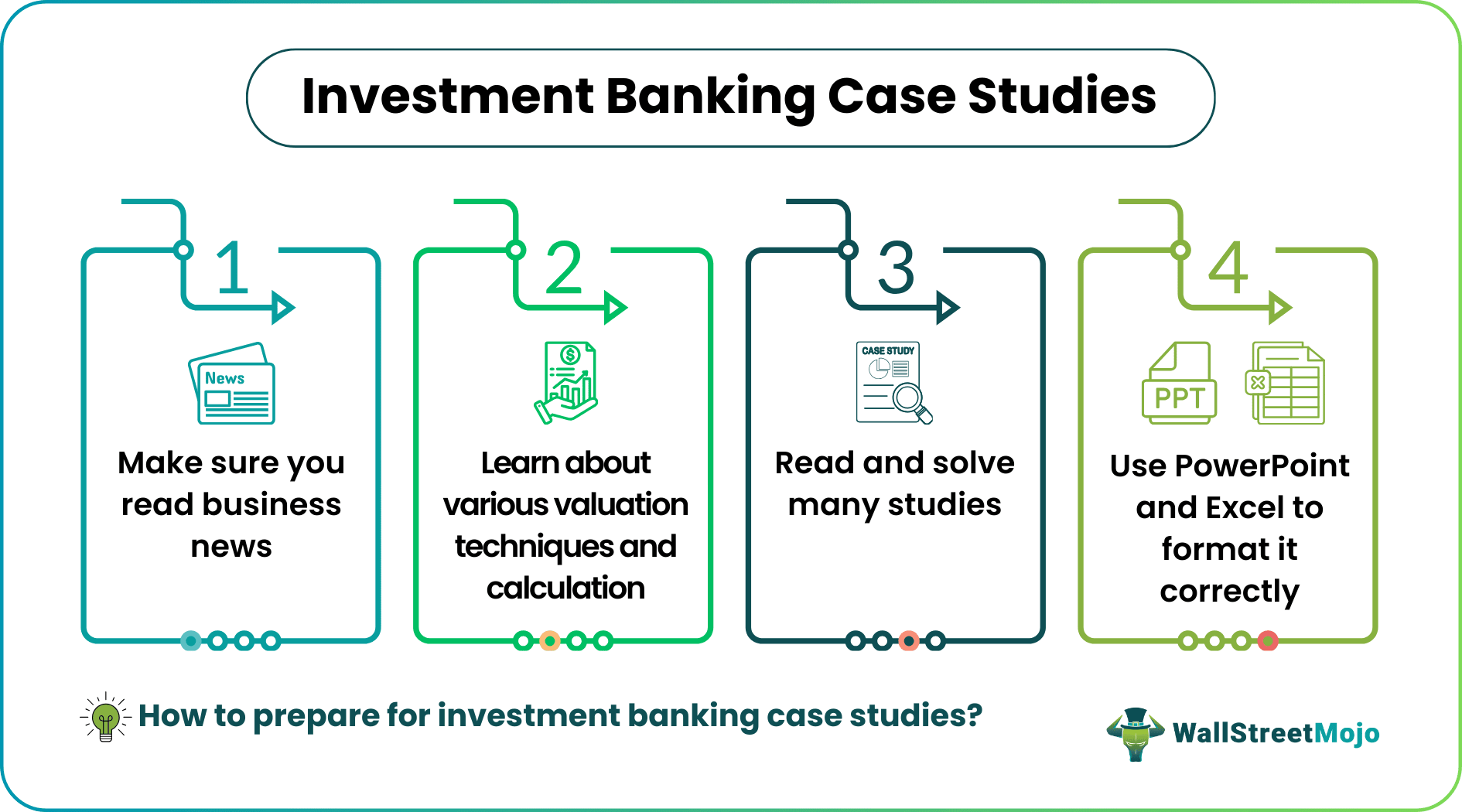
It is the most crucial part of the interview process and the toughest to crack. Hence, proper preparation is expected from the ones applying for the investment banking jobs . Not clearing this round might lead to the rejection of the candidate no matter how well they perform in the other stages of the investment banking interview.
Table of contents
Investment banking case studies explained, why investment banking case studies, how to prepare, tips for performing well, frequently asked questions (faqs), recommended articles.
- Investment banking case studies are a practice followed by nearly every bulge bracket Investment Bank (IB) hiring process.
- Typically, investment banking case studies have the features such as hypothetical situations. However, it resembles an existing corporation condition, recreates strategic decisions and advisors, and financial details that may or may not be provided depending on the analysis's relevance.
- The investment banking job requirements differ from those of general finance jobs. Therefore, bankers must use non-traditional candidates to recognize investment banking capabilities.
- Decision-making case studies and modeling investment banking case studies are the two case studies.
Investment banking case studies play a great role in assessing the abilities of a candidate and figure out their presence of mind and effective utilization of their skills and qualifications. Though it is an important part of the assessment, case studies are not for all. It is meant for the ones applying for a higher position in the investment banking sector.
Investment banking case studies are incorporated in the interviews of senior professionals, including senior analysts and associates. For someone who has attended business school, solving case studies are common. However, as part of the hiring process, the case studies given here are business-based and candidates are expected to provide detailed recommendations based on the given scenario.
Candidates receive all the required information and enough time to study general case studies. It is typically a business problem that asks for their opinion. Hence, they need to:
- Make necessary assumptions
- Analyze the given situation, which could usually be a client’s business
- Advise solution on the present problem with supportive reasons
While the case study round would happen most of the time on the day of your interview, some recruiters also provide the material beforehand for the candidates to prepare well at home. Candidates are then expected to discuss the case study during the interview.
A typical case study would have the following features: –
- It would be a hypothetical situation, although it could resemble an existing condition of a corporation.
- It attempts to recreate its strategic decisions and its advisors.
- Financial information may or may not be provided depending on the relevance of the analysis that needs to be done.
- The suggestions must be original work of the candidates or their team.
Investment banking case studies are the best ways of analyzing the practical skills and abilities of a candidate and this is what make it an important part of the interview process in any investment banking unit. Through this process, the bankers try to judge a candidate in real-world situations. They want to test the three most important skills required in a banker, analytical, communication, and people skills. Due to these reasons, the case study weighs much more than the other ways of judging candidates in the investment banking recruitment process.
The investment banking job requirements differ from those of general finance jobs. Hence, the bankers want to use non-traditional candidates to identify the IB potential. Case studies give banking recruiters an indicator of how one would perform on the job. Hence, considered a better measure to judge candidates.
- One does not have to worry if an answer is correct or not. Instead, the interviewers are eyeing the candidate’s thought process and analytical skills to find a solution to the given problem creatively.
- Investment banking case studies are designed in such a manner that it enables the candidates to think on their own and brainstorm.
- One of the primary skills required in candidates for such jobs is solving problems. Therefore, recruiters want a basic insight into tackling challenging situations and apply their intelligence, education, and work experience to handle them successfully.
- Case studies appraise how one processes information and react to new and surprising situations.
- Many times, the case studies are to be solved with a group. Hence, the interviewer here tests how they work within a team.
Broadly, candidates can expect two types of case studies at the IB assessment center: the decision-making case studies and financial modeling case studies.
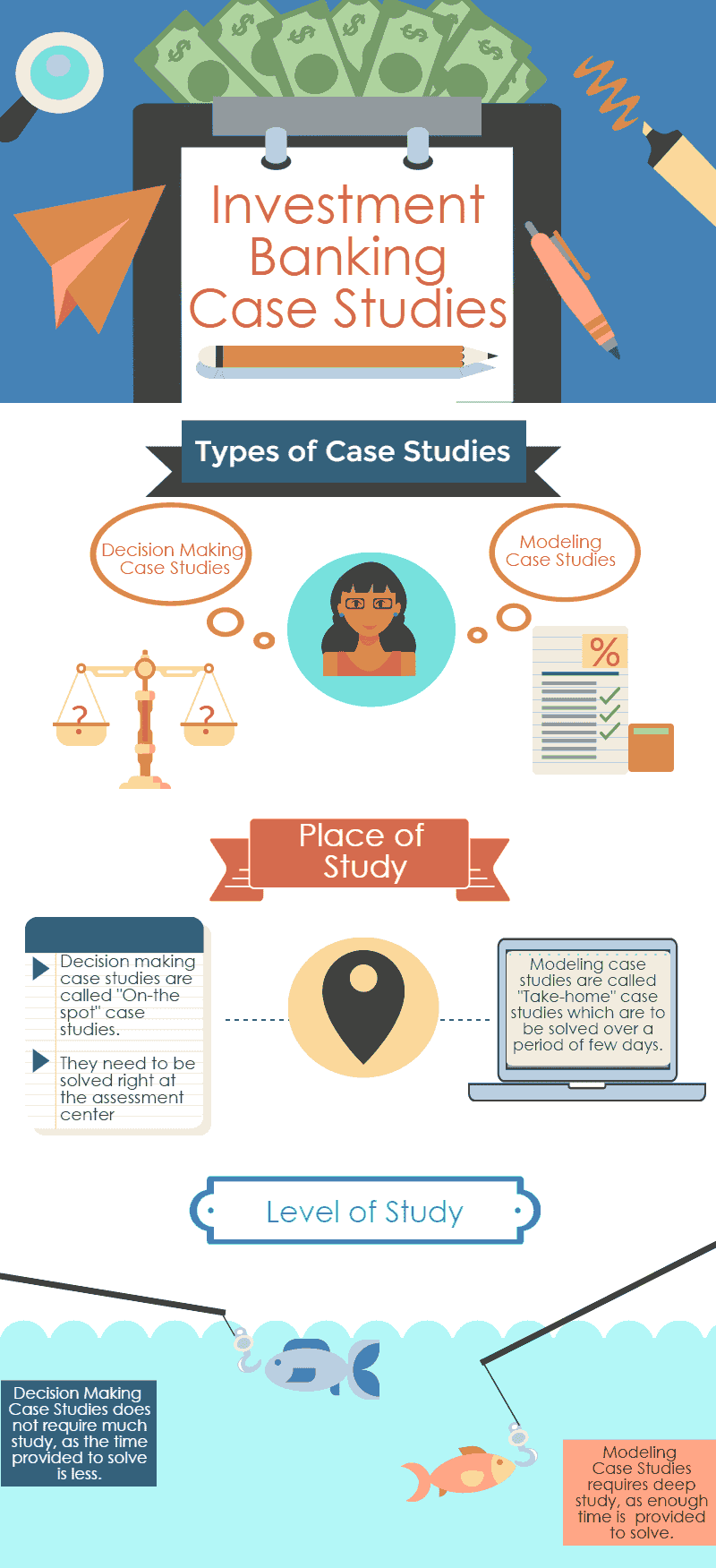
Decision-Making Case Studies
They are more commonly asked as compared to the modeling-type case study. In this case study, candidates must make decisions for clients and advise them in certain situations.
The client case studies could be based on finding sources through which one should raise capital, whether they should undertake the proposed merger , and why.
One should expect these questions to be made available on the spot., They must solve and present the case within the given time frame. For this entire process, they would be given around 45-60 minutes for preparation and a 10 minutes presentation followed by a round of questions and answers. On-the-spot case studies would not involve a deep analysis of the case as the time required to do the same is not sufficient and would be more about presentation and teamwork skills put to the test.
Let’s say one of the clients is a global corporation that manufactures and distributes a wide range of perfumes. They are contemplating ways to expand their business. There are two ways to either introduce a new range of fragrances with the current distribution channels or start a completely new company with different stores.
The candidates are supposed to find out which would be a better solution for the business. To solve this, they must compare the returns of the investments and decide on the solution with supportive reasons.
Modeling Case Studies
These are the take-home case studies where the candidates are expected to do financial modeling and simple valuation. So, it is more like a modeling test than a case study.
The case study would perform FCFF valuation on a company or prepare a simple merger or leveraged buyout model .
One would be expected to analyze the corporations’ valuation multiples and decide whether they are undervalued or overvalued. Here, they are given a few days to complete the analysis. Then, the candidates need to showcase their recommendations to the bankers on the interview day over a 30–45-minutes presentation. Compared to the client case studies, the analysis would be much more profound as the candidates get enough time to work on them.
A pharmaceutical company has decided to make an acquisition . It has identified the company and has approached candidates to advise how much they should pay. In addition, they are provided with the necessary financial information , metrics and multiples, and the buyer and seller company overview.
To solve this, first, one needs to find out if the acquisition is possible. Then, how would the deal structure and synergies be if the buyer has sources to finance the deal? After this, they must use multiple valuation metrics to decide the price range of the agreement.
The preparation of the investment banking case studies involves an organized approach. Let us have a look at the most common ways of preparing for this part of the hiring process:
- Make sure to read business news often and focus on discussing how and what business transactions are concerned.
- Learn about various valuation techniques, their calculation, and how they are interpreted.
- Especially for modeling and valuation-based case studies, one must be prepared to format it consistently using PowerPoint and Excel.
- Yes, that is all one needs to do. Read and solve as many case studies as possible so that they get the knack for understanding business scenarios and solving them.
- The candidates may not find real case study questions that banks use for interviews. But since they have to practice, try asking a friend or colleague they know who has been through such case study rounds for the kind of questions they received.
- If even that is possible, create a case study . Yes, candidates are allowed to do that by taking up a company, building up a hypothetical situation, and asking themselves questions like whether they should merge with ABC Co.? What kind of capital structure should the company have?
Preparing for this round of interview for an investment banking job opportunity is tough. Hence. Here are a few tips that one may utilize to ensure their preparation remains on track and they efficiently crack this round:
While working on the Investment Banking Case Studies
- Make a concrete decision and base recommendations on logical reasons.
- Use a structured approach to tackle the problem.
- Focus on the most important issues prevalent in the case.
- Understand the case and questions carefully before interpreting and think twice before finalizing the problem’s decision.
- Do not panic if the solution to the case is not obvious.
- For modeling case studies, format the Excel and PowerPoint professionally.
- Prepare the type of questions that may be asked during the presentation.
- Assess all the relevant factors and possible problems, but keep in mind the resources.
- The solutions provided should be realistic and be aware of the implications of the organizations under study.
- Have strong logical reasons behind every statement made and cater to the case’s critical issues at the beginning.
- Having specific knowledge regarding the industry under study is unnecessary, but it would be an added advantage.
- When preparing, focus on reading deal news and practice as many scenarios as possible.
While presenting the Investment Banking Case study
- Practice public speaking.
- Speak slowly and clearly.
- The presentation needs to be structured logically.
- While working in groups, interact with everyone. The interviewer usually eyes leadership skills and teamwork.
- Do not just try to show the ability to talk finance and business knowledge in an applied sense.
- Remember not to exceed the time limit allotted to present the slides.
- Rehearse well before so that it goes through smoothly.
- If it is a group presentation, ensure everyone gets the chance to speak and express their views. The assessors would also mark the behavior while working in a team.
While Answering the Questions
- Do not hurry to provide the answer. Instead, always organize thoughts and then answer.
- Be attentive throughout the process.
- Expect the interviewer to ask additional questions to test how to deal with the unexpected.
- Be creative and think out of the box to get the banker’s attention.
- The next important step is collecting thoughts and bringing them across the main points. Do not beat around the bush, as the time given is limited. Hence, be precise while speaking.
- There is nothing right and wrong, but arguments (which surely happen if there are group discussions) strongly mention why one did not opt for those possibilities.
Let us understand investment banking case studies through an example discussed below: –
Investment Banking Case Study - Situation:
Simons Ltd., a software company, wants to maximize its shareholder value. It has three options to look forward to selling the company, i.e., making small acquisitions or growing organically. Argus Ltd. requests advice from the bank on the right course of action.
What to do:
- They would provide an overview of the business, its competitors, probable acquisition candidates, financial statements, and future projections to review the company.
- Read through the provided information and understand the industry.
- Try to gauge the worth of the company compared to its peers .
- Conduct a valuation analysis using DCF and relative valuation techniques.
- Compare the three options with the valuation and the impact of acquisitions.
- Prepare a presentation giving our recommendation on the best method to maximize the shareholder value in this situation.
The solution to the Investment Banking Case Study
The answer to this case study is somewhat subjective. They could take a stand and support it with reasons. However, here, we assume to sell the company for understanding purposes. Let us now see how one could sell as the suggested option.
We recommend selling because of the following reasons: -
- The industry is growing slowly (less than 5% a year).
- The companies are overvalued.
- The acquisitions would not increase the revenue or profit significantly.
Presentation of Investment Banking Case Study
- Please keep it straightforward with the reasons for recommendation to sell.
- A brief overview of the industry, its growth characteristic, and its position.
- How would the company grow organically in the next 5-10 years?
- Briefly explain the acquisition candidates and opportunities with them.
- Justify why both the organ growth options and the acquisitions do not work out.
- Explain the DCF analysis performed and show how selling is the best feasible option.
Yes, there are various resources available to practice investment banking case studies. For example, investment banking textbooks, online financial modeling courses, and case study interview preparation guides can provide valuable insights and practice materials. Additionally, some investment banking firms and university career service departments may offer sample case studies or practice resources.
Case studies are commonly used in these interviews to assess a candidate's ability to apply financial and analytical skills to real-world situations. In addition, they test the candidate's critical thinking, problem-solving, and presentation abilities, essential skills for investment banking analysts and associates.
The banking case study examples are the Light Bank, Shine Bank, ITTI Digital Back-Office, Banking Super app, CashMetrics, MasterCard, FinTarget Trading, VR / AR Banking, Bank of Jordan, and Islamic Bank in Qatar.
This has been a guide to what are Investment Banking Case Studies. We explain its examples, why are they required, types, sample, and tips for preparation. You may also look into the articles below to explore investment banking sector a bit more.
- Hedge Fund Manager vs Investment Banking
- Investment Management vs Investment Banking
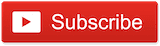

- SUGGESTED TOPICS
- The Magazine
- Newsletters
- Managing Yourself
- Managing Teams
- Work-life Balance
- The Big Idea
- Data & Visuals
- Case Selections
- HBR Learning
- Topic Feeds
- Account Settings
- Email Preferences
Case Study: Will a Bank’s New Technology Help or Hurt Morale?
- Leonard A. Schlesinger

A CEO weighs the growth benefits of AI against the downsides of impersonal decision making.
Beth Daniels, the CEO of Michigan’s Vanir Bancorp, sat silent as her chief human resources officer and chief financial officer traded jabs. The trio had founded their community bank three years earlier with the mission of serving small-business owners, particularly those on the lower end of the credit spectrum. After getting a start-up off the ground in a mature, heavily regulated industry, they were a tight-knit, battle-tested team. But the current meeting was turning into a civil war.

- Leonard A. Schlesinger is the Baker Foundation Professor at Harvard Business School, where he serves as chair of its practice-based faculty.
Partner Center
- Digital Marketing
- Facebook Marketing
- Instagram Marketing
- Ecommerce Marketing
- Content Marketing
- Data Science Certification
- Machine Learning
- Artificial Intelligence
- Data Analytics
- Graphic Design
- Adobe Illustrator
- Web Designing
- UX UI Design
- Interior Design
- Front End Development
- Back End Development Courses
- Business Analytics
- Entrepreneurship
- Supply Chain
- Financial Modeling
- Corporate Finance
- Project Finance
- Harvard University
- Stanford University
- Yale University
- Princeton University
- Duke University
- UC Berkeley
- Harvard University Executive Programs
- MIT Executive Programs
- Stanford University Executive Programs
- Oxford University Executive Programs
- Cambridge University Executive Programs
- Yale University Executive Programs
- Kellog Executive Programs
- CMU Executive Programs
- 45000+ Free Courses
- Free Certification Courses
- Free DigitalDefynd Certificate
- Free Harvard University Courses
- Free MIT Courses
- Free Excel Courses
- Free Google Courses
- Free Finance Courses
- Free Coding Courses
- Free Digital Marketing Courses
AI in Banking [20 Case Studies] [2024]
In the rapidly altering finance landscape, AI has emerged as a pivotal significance, extending banks’ abilities and reshaping traditional financial patterns. From enhancing customer experiences to mitigating financial risks, AI’s role in banking is pivotal and transformative. This exploration delves into 20 distinct case studies where leading banks have successfully implemented AI to address complex challenges in the industry. These examples showcase AI’s innovative applications and highlight its potential to revolutionize banking operations, improve customer service, and bolster financial security. As we navigate through these case studies, we gain insights into the strategic advantages and practical impacts of AI in the banking sector, underscoring its importance in shaping the future of finance.
Related: High-Paying Banking Jobs & Career Paths
Case Study 1: JP Morgan Chase: Streamlining Loan Approvals
The traditional loan approval process is notoriously cumbersome and slow, heavily reliant on manual data handling. This results in prolonged wait times, leading to significant customer dissatisfaction and increasing operational costs due to the extensive need for human oversight and intervention.
To address these inefficiencies, JP Morgan Chase has implemented an advanced AI system that automates key aspects of the loan approval process. This system utilizes machine learning to swiftly and accurately analyze various data points, including applicants’ credit history, recent transaction data, and current financial behaviors. Doing so enhances the speed and accuracy of creditworthiness assessments, reduces reliance on manual processes, and improves overall customer experience by expediting loan approvals.
Overall Impact:
- Increased Speed: Loan processing times have dramatically reduced from days to minutes and hours.
- Enhanced Customer Satisfaction: Faster loan approvals increase customer satisfaction and loyalty.
- Cost Efficiency: Reduced reliance on manual processes decreases operation expenses and improves profitability.
- Scalable Operations: The bank can handle more loan applications without significantly increasing staff or resources.
Key Learnings:
- Process Efficiency: AI drastically cuts down the time required for loan approvals.
- Operational Cost Reduction: Automation reduces the labor-intensive elements of loan processing.
- Enhanced Risk Management: AI provides a more accurate and comprehensive loan risk assessment.
- Customer Retention: Improved process speeds and accuracy improve customer retention rates.
Future Prospects:
AI algorithms could be enhanced for faster processing, achieving near-instant approval times. Future iterations may further integrate broader economic indicators to refine credit risk assessments, enhancing personalized lending strategies.
Case Study 2: Bank of America: Erica, the AI-Powered Financial Assistant
As digital banking gains traction, customer expectations are also evolving. Users now demand personalized services on-demand and easily accessible through their digital devices. This shift has pushed banks to find innovative solutions to meet these new customer demands without compromising service quality.
Bank of America responded to this digital shift by launching Erica, an AI-driven virtual assistant designed to enhance the mobile banking experience. Accessible via mobile apps, Erica offers a wide range of functionalities that cater to the modern banking customer’s needs. These include handling transaction queries, updating credit reports, and providing proactive financial advice. Erica’s capabilities are powered by sophisticated algorithms that analyze user behavior and large datasets, enabling customized and efficient service that meets the high expectations of today’s bank customers.
- Personalized Customer Interaction: Erica offers tailored banking advice, enhancing user engagement.
- Increased Accessibility: Round-the-clock availability allows customers to receive instant assistance without waiting for human help.
- Data-Driven Insights: Erica provides insights based on a deep analysis of user transactions and behaviors, helping customers manage their finances better.
- Operational Efficiency: The AI assistant handles regular inquiries, leaving humans to deal with more complex issues.
- Enhanced User Experience: AI-driven tools like Erica improve customer experience by providing quick, personalized service.
- Operational Scalability: AI can manage increasing volumes of consumer interactions without additional human resources.
- Proactive Service: AI enables proactive engagement, offering financial advice and alerts that can prevent issues before they arise.
- Customer Data Utilization: Using AI to analyze customer data effectively can lead to more accurate and useful financial advice.
Erica could develop more sophisticated natural language processing capabilities to manage increasingly complex inquiries and transactions. Integration with IoT devices and other platforms may offer holistic financial management solutions, extending personalized services beyond traditional banking.
Case Study 3: HSBC: Enhancing Anti-Money Laundering Efforts
Money laundering remains a formidable challenge for financial institutions worldwide. Traditional systems designed to detect such activities often struggle under modern financial transactions’ heavy volume and complex nature. These systems can be overwhelmed, resulting in undetected fraudulent activities and significant regulatory penalties for banks.
In response, HSBC has integrated an AI-driven system to bolster its anti-money laundering (AML) efforts. This advanced system employs sophisticated machine learning algorithms to analyze many real-time transactions. By detecting unusual patterns and potential illegal activities, the system can far more effectively differentiate between normal and suspicious activities than traditional methods. This AI-enhanced approach allows HSBC to address the complexities of modern financial crime while improving compliance and reducing the risk of oversight.
- Improved Detection Rates: The AI system has significantly increased the detection of suspicious transactions, reducing the risk of financial crimes.
- Reduced False Positives: Enhanced accuracy in distinguishing legitimate from suspicious activities, minimizing disruptions to innocent customers.
- Compliance Efficiency: AI assists in maintaining compliance with evolving regulatory requirements, adapting more quickly to new rules.
- Cost Reduction: Automating surveillance reduces the need for extensive manual review teams, lowering operational costs.
- Accuracy in Surveillance: AI technologies improve the accuracy and efficiency of financial monitoring systems.
- Adaptive Compliance: AI can adapt quickly to new regulatory changes, aiding compliance efforts.
- Resource Optimization: Implementing AI reduces the need for large human oversight teams, optimizing resource use.
Future developments may incorporate predictive analytics to detect and predict laundering schemes before they are fully enacted. Integration with international finance monitoring systems could enhance global compliance and tracking capabilities.
Case Study 4: Citibank: Optimizing Customer Service with AI Chatbots
In the fast-paced banking world, high demand for customer service can lead to long wait times and inconsistent service experiences. Such delays and variability often detract from customer satisfaction and can negatively impact customer retention rates. As digital interactions become the norm, banks face the challenge of maintaining high service standards while managing large volumes of customer inquiries efficiently.
Citibank has implemented AI-powered chatbots across its digital platforms to address this challenge. These chatbots are arranged to address a spectrum of consumer inquiries, offer real-time support, and efficiently settle typical issues. By deploying these AI chatbots, Citibank ensures a uniform and agile consumer service experience. The chatbots are equipped to understand and process user queries quickly, offering solutions and guidance instantaneously. This technology reduces the burden on human customer service representatives and enhances overall customer satisfaction by providing timely and reliable support.
- Enhanced Customer Service: Immediate response to inquiries improves customer satisfaction.
- 24/7 Availability: Customers receive help anytime without needing human agent availability.
- Consistent Experience: AI ensures that every customer interaction is handled uniformly, enhancing service reliability.
- Operational Savings: The chatbots handle routine inquiries, decreasing the workload on human client service agents and decreasing operational costs.
- Service Accessibility: AI tools can provide constant and consistent consumer service.
- Cost Efficiency: Automating routine interactions can significantly reduce customer service costs.
- Customer Engagement: Real-time interactions facilitated by AI can boost customer engagement and loyalty.
AI chatbots could evolve to handle more sophisticated negotiations and problem-solving tasks, further reducing the need for human intervention. Future versions might seamlessly integrate into omnichannel customer service strategies, providing a unified interface across all banking platforms.
Case Study 5: Santander: Predictive Analytics for Loan Default Prevention
Loan defaults pose a great financial risk to banks, affecting their profits and stability. Traditional risk assessment models often fall short in accurately predicting defaults before they occur, primarily because they may not account for dynamic changes in customers’ financial situations or broader economic trends. This limitation leads to unexpected financial losses and inefficient allocation of resources for risk management.
Santander has adopted a proactive approach to this challenge by integrating predictive analytics models powered by AI into its risk management strategy. These models use a combination of historical data analysis and real-time monitoring of account behaviors to detect early warning signs of potential loan defaults. By identifying at-risk customers before defaults occur, Santander can engage with them to offer tailored financial advice, restructuring options, or other support measures. This early intervention helps mitigate risks associated with loan defaults and improves the bank’s and its customers’ overall financial health.
- Reduced Default Rates: Early identification and intervention have led to a decrease in loan defaults.
- Enhanced Customer Support: At-risk customers receive tailored advice and restructuring options, improving financial outcomes.
- Operational Efficiency: The bank optimizes resource allocation by focusing efforts where they are needed the most.
- Improved Risk Management: Better predictive capabilities allow for more accurate risk pricing and reserve allocation.
- Proactive Risk Management: Early detection of potential defaults enables more effective mitigation strategies.
- Customer Retention: Proactive engagement helps maintain customer relationships and loyalty.
- Financial Health: Improved risk assessment contributes to the bank’s overall financial health and stability.
- Resource Allocation: AI enables more targeted and efficient use of resources in risk management activities.
Integrating wider socio-economic data could improve predictive models, offering even more precise forecasts of potential defaults. These enhancements allow customized intervention strategies tailored to individual customer profiles and economic conditions.
Related: Is Banking a stressful job?
Case Study 6: Wells Fargo: Fraud Detection Enhancement
Real-time fraud detection in financial transactions presents a major challenge, as traditional methods often lag behind fraudsters’ sophisticated techniques. Wells Fargo faced significant challenges in effectively identifying and preventing fraudulent activities. Their traditional systems struggled to keep up without mistakenly flagging legitimate transactions as fraudulent, leading to customer dissatisfaction and operational inefficiencies.
To address this issue, Wells Fargo implemented an AI-based fraud detection system employing deep learning algorithms to scrutinize real-time transaction patterns. This advanced system is designed to compare each transaction against an extensive database of known fraudulent behaviors, enhancing its ability to make accurate assessments instantly. By doing so, the system significantly improves fraud detection accuracy, minimizing false positives and ensuring that legitimate customer transactions are not disrupted. This method boosts security and enhances the overall customer experience by minimizing delays and errors in transaction processing.
- Improved Fraud Detection: The AI system has a higher accuracy rate in identifying fraudulent transactions, reducing the incidence of fraud.
- Minimized Customer Disruption: Accurate fraud detection means fewer legitimate transactions are flagged incorrectly, ensuring smoother customer experiences.
- Enhanced Security: The system enhances overall transaction security, giving customers greater confidence in using Wells Fargo’s services.
- Cost Efficiency: Decreased fraud incidence reduces financial losses and related costs for the bank.
- Real-Time Processing: AI can process and analyze real-time transactions, offering immediate fraud alerts.
- Data Utilization: Leveraging large datasets enhances the system’s ability to identify and learn from emerging fraud patterns.
- Customer Trust: Improved security measures boost customer trust and satisfaction.
Wells Fargo plans to integrate further enhancements into the AI system, such as adaptive learning capabilities that can evolve with changing fraud tactics. This will allow for even more dynamic and robust fraud prevention mechanisms.
Case Study 7: Barclays: Streamlining Wealth Management
Barclays faced challenges in meeting the high expectations of its high net-worth clients who demand personalized, efficient wealth management services. Traditional methods were slow and often ineffective in providing the customization and rapid service these clients expected, leading to dissatisfaction and operational inefficiencies.
Barclays introduced an AI-driven platform to transform its wealth management services. This platform uses advanced analytics to deeply understand individual client preferences and performance, enabling tailored investment advice and automated portfolio adjustments. This automation enhances service speed and accuracy, improving client satisfaction and streamlining operations.
- Personalized Service: Clients receive highly customized investment advice, improving satisfaction and engagement.
- Increased Efficiency: The AI platform automates routine portfolio management tasks, freeing up advisors to focus on client relationships.
- Better Investment Performance: AI-enhanced analytics provide deeper insights into market trends, aiding better investment decisions.
- Scalability: The platform can efficiently manage many portfolios, scaling as the client base grows.
- Enhanced Customization: AI enables a high degree of personalization in delivering services. This technology tailors interactions to meet individual user needs effectively.
- Advisor Efficiency: Automating routine tasks allows wealth managers to focus more on strategic client interaction.
- Data-Driven Decisions: Utilizing AI for data analysis improves the accuracy and timeliness of investment decisions.
Barclays intends to refine its AI capabilities further, incorporating more comprehensive data sources, including global economic indicators and social trends, to enhance investment strategy recommendations.
Case Study 8: Deutsche Bank: Optimizing Credit Card Fraud Detection
Credit card fraud poses a major problem for banks, resulting in annual losses amounting to millions and eroding customer trust. This persistent issue challenges financial institutions to enhance their security measures and maintain client confidence. Deutsche Bank faced the challenge of rapidly identifying and mitigating fraudulent credit card activities without affecting genuine transactions.
Deutsche Bank implemented an AI-based solution specifically designed to improve credit card fraud detection. This solution uses advanced machine learning models to monitor and analyze real-time credit card transactions. The system can quickly identify anomalies that suggest fraudulent activity by learning from historical transaction data and continuously adapting to new fraud patterns.
- Increased Detection Accuracy: The AI system significantly enhances the ability to spot fraudulent transactions, reducing financial losses.
- Enhanced Customer Trust: Customers feel more secure using their credit cards, knowing that advanced measures are in place to protect them.
- Operational Efficiency: The automated system allows for faster response times and reduces the workload on manual review teams.
- Reduced False Positives: The system effectively minimizes disruptions to innocent customers by accurately distinguishing between legitimate and fraudulent activities.
- Adaptive Learning: Machine learning models adapting to new data and evolving fraud tactics are more effective than static models.
- Customer Experience: Maintaining a balance between aggressive fraud detection and customer convenience is crucial for customer satisfaction.
- Security as a Priority: Investing in advanced security measures like AI protects the bank’s assets and builds customer loyalty.
Deutsche Bank plans to integrate more granular behavioral analytics to refine the system’s accuracy further. Additionally, collaborating with global financial networks to share fraud intelligence could enhance the system’s predictive capabilities, setting a new standard for fraud prevention in the banking industry.

Case Study 9: Credit Suisse: Enhancing Mortgage Underwriting with AI
Credit Suisse encountered significant challenges in its mortgage underwriting process, which relied heavily on manual input, making it both time-consuming and prone to creating backlogs of applications. This inefficient process delayed loan disbursals and negatively impacted customer satisfaction, as clients experienced lengthy wait times and unpredictable service levels. Streamlining this process was crucial to improving operational efficiency and maintaining customer trust and loyalty.
Credit Suisse adopted an AI-driven approach to transform its mortgage underwriting process. The AI system uses machine learning to assess applicant data such as income, credit score, employment history, market trends, and property evaluations more quickly and accurately than manual methods. This automation allows for faster decision-making and more precise risk assessment.
- Faster Processing Times: The time taken to approve mortgages has been significantly reduced, enhancing customer satisfaction.
- Increased Accuracy: AI provides more accurate assessments of applicant risk profiles, reducing the likelihood of loan defaults.
- Operational Efficiency: Automating routine tasks allows human underwriters to concentrate on handling more complex cases. This shift frees up valuable resources for more critical and detailed work.
- Scalable Underwriting Capacity: The system can handle more applications without additional staff.
- Automation in Risk Assessment: The use of AI for processing and analyzing complex applicant data streamlines risk assessment.
- Improved Customer Experience: Reducing wait times for loan approvals directly impacts customer satisfaction positively.
- Enhanced Decision Making: AI tools provide a deeper insight into potential risks and applicant credibility, aiding better decision-making.
Credit Suisse plans to further enhance the capabilities of its AI system by integrating it with real-time economic indicators and more detailed applicant lifestyle data to predict future financial stability more accurately. This advancement aims to streamline the process and tailor mortgage products more specifically to individual needs, setting a new standard in personalized banking services.
Case Study 10: Standard Chartered: Streamlining Trade Finance Operations
Standard Chartered faced complexities in managing trade finance operations, which involve extensive documentation and verification processes that are traditionally manual and error-prone. These challenges resulted in slow transaction times and higher operational costs, affecting client satisfaction and competitiveness in the global market.
Standard Chartered introduced an AI-driven platform designed to automate and enhance the efficiency of its trade finance operations. Utilizing sophisticated machine learning algorithms, the platform efficiently verifies documents, authenticates data, and streamlines the entire approval process for trade transactions. This integration of advanced technology ensures faster, more accurate handling of the complex documentation and regulatory requirements inherent in trade finance, improving overall transaction speed and reliability. By automating these key steps, the bank has significantly reduced manual errors and sped up the processing of trade finance operations.
- Reduced Processing Time: Transaction times for trade finance operations have been drastically reduced, increasing client satisfaction and transaction volumes.
- Decreased Operational Costs: Automation has minimized the need for extensive manual intervention, significantly cutting operational costs.
- Enhanced Accuracy: The AI system provides a higher level of precision in document verification and data authentication, decreasing the risk of fraud and errors.
- Improved Compliance: The system ensures better adherence to international trade regulations through accurate and automated compliance checks.
- Efficiency through Automation: Automating complex, repetitive tasks can significantly enhance efficiency and accuracy in high-stakes financial operations.
- Client Satisfaction: Quicker processing times and fewer errors directly enhance client relationships and contribute to business expansion.
- Regulatory Compliance: AI tools are vital in ensuring compliance with the continuously changing international trade laws. They help organizations adapt quickly to regulatory updates, maintaining legal integrity across global operations.
Standard Chartered is looking to expand its AI capabilities to include predictive analytics for assessing the potential risks and opportunities in trade finance. Further integration with blockchain technology could enhance security and transparency in international trade transactions, setting new industry standards for efficiency and trust.
Related: Will Banking jobs be Automated?
Case Study 11: Goldman Sachs: Advanced Algorithmic Trading
The volatility of financial markets and the sheer volume of data they generate present significant challenges for traders and investors, especially in executing trades swiftly and efficiently to capitalize on market opportunities. Goldman Sachs needed to enhance its trading strategies and execution speed to remain competitive and maximize client returns.
Goldman Sachs deployed an AI-driven algorithmic trading system to process and analyze vast market data in real-time. This system utilizes advanced machine learning models to forecast market trends, pinpoint profitable trading opportunities, and execute trades at the most favorable prices. By automating these tasks, the system enhances the speed and efficiency of trading operations while reducing human error, enabling more strategic and informed investment decisions.
- Increased Trading Efficiency: The system facilitates quicker and more precise execution of trades.
- Enhanced Profitability: Algorithmic strategies maximize returns by capitalizing on short-lived market opportunities.
- Reduced Operational Risk: Minimizes errors associated with human trading.
- Scalability: Enables handling of a larger volume of trades without proportional increases in overhead.
- Leverage of Real-time Data: Leveraging real-time data can greatly improve decision-making processes.
- Algorithmic Precision: Advanced algorithms improve precision in trading, leading to better market positioning.
- Risk Management: Improved ability to respond to market volatility and manage risks more effectively.
Goldman Sachs plans to incorporate deeper learning algorithms and broader data sets, including global economic indicators, to refine its trading algorithms further. Enhancements in AI models may also include adaptive learning capabilities to better respond to market changes, offering more robust and dynamic trading strategies.
Case Study 12: BNP Paribas: Revolutionizing Risk Assessment with AI
Risk assessment processes in banks are critical for maintaining financial stability and regulatory compliance but are often cumbersome, slow, and prone to errors. BNP Paribas faced challenges in accurately assessing and managing the myriad risks associated with its global banking operations, from credit to operational risks.
BNP Paribas implemented an AI-powered risk assessment platform that utilizes machine learning to enhance the accuracy and speed of risk evaluations. This platform analyzes various risk factors by ingesting and processing large datasets to predict potential defaults and financial instabilities. It also monitors ongoing operations to identify deviations or anomalies indicating heightened risk.
- Improved Risk Prediction: AI algorithms offer early alerts to potential risks, enabling prompt intervention.
- Operational Efficiency: Decreases the time and labor required for risk assessments.
- Comprehensive Risk Coverage: Provides a comprehensive perspective on risks across various departments and regions.
- Dynamic Risk Management: Enables immediate modifications to risk strategies using the most recent data analytics.
- Predictive Analytics: AI can accurately forecast potential risks, allowing for proactive risk management.
- Data Integration: Integrating data from several sources provides a more comprehensive risk analysis.
- Continuous Learning: AI systems can evolve and adjust to new risk indicators continuously.
BNP Paribas is exploring the integration of AI with blockchain technology to secure further and streamline risk management processes. Future developments may also include using AI to simulate various risk scenarios, enhancing predictive capabilities, and strategic planning for potential crises.
Case Study 13: ING: Personalizing Customer Experience with AI
In the competitive digital banking landscape, ING faced the challenge of delivering a personalized and engaging customer experience. Traditional banking interactions lacked the personal touch and responsiveness that modern customers expect, leading to a gap in customer engagement and satisfaction.
ING introduced a personalized recommendation engine powered by AI to enhance its digital banking services. This engine analyzes customer data, such as transaction histories, browsing behaviors, and previous interactions, to tailor banking offers and advice. ING seeks to boost customer engagement and satisfaction by delivering personalized content and recommendations.
- Enhanced Personalization: Customers receive offers and advice relevant to their financial needs and behaviors.
- Increased Engagement: Personalized interactions have increased customer activity and engagement on digital platforms.
- Customer Satisfaction: The tailored experience boosts overall customer satisfaction and loyalty.
- Operational Efficiency: AI-driven personalization reduces the need for manual customer segmentation and campaign management.
- Data Utilization: Strategic utilization of customer data can greatly improve personalization.
- Customer Loyalty: Personalized experiences contribute to increased customer loyalty and retention.
- Scalability: AI solutions can expand personalized services without proportional increases in resource allocation.
ING plans to refine its AI models further to offer even more customized financial guidance and product recommendations. The bank also aims to integrate its AI systems with emerging technologies like IoT to provide real-time financial advice based on customers’ geographic locations and activities.
Case Study 14: Scotiabank: Streamlining Regulatory Compliance
Regulatory compliance is a major area of focus for financial institutions, requiring significant resources to ensure adherence to laws and regulations. Scotiabank needed to enhance its compliance measures, particularly in detecting and preventing breaches that could lead to legal penalties and damage to reputation.
Scotiabank deployed an AI-driven compliance monitoring system to streamline its regulatory processes. This system employs natural language processing (NLP) to examine and interpret communications and transactions throughout the bank. By automating the detection of non-compliant behaviors and potential breaches, the system enhances the effectiveness and efficiency of the bank’s regulatory compliance measures.
- Improved Compliance Monitoring: The system allows real-time compliance monitoring across all bank operations.
- Reduced Risk of Breaches: Early detection of potential breaches minimizes legal risks and penalties.
- Operational Efficiency: Automates the labor-intensive compliance monitoring process, reducing operational costs.
- Enhanced Regulatory Reporting: AI-driven analytics facilitate more precise and prompt reporting to regulatory authorities.
- Proactive Compliance: AI enables a more proactive approach to compliance, catching issues before they escalate.
- Cost Reduction: Automating compliance processes significantly reduces manual monitoring and reporting costs.
- Regulatory Adaptation: AI systems can be quickly updated to reflect changes in regulatory requirements, ensuring ongoing compliance.
Scotiabank is exploring the potential for AI to integrate further with blockchain technologies to enhance transparency and security in compliance reporting. Additionally, the bank is considering expanding AI applications to other areas of regulatory compliance, such as anti-corruption and financial conduct, to bolster its compliance framework further.
Case Study 15: Morgan Stanley: Enhancing Investment Advisory Services
Navigating investment decisions in a highly volatile and complex global market is challenging for clients and advisors. Morgan Stanley sought to enhance its advisory services to provide clients with more precise and strategic investment advice, ensuring better portfolio performance and client satisfaction.
Morgan Stanley integrated AI-powered analytics into its advisory services. This platform utilizes developed machine learning algorithms to analyze global market trends, financial news, and investment patterns. It delivers personalized investment insights and recommendations customized to align with each client’s financial goals and risk tolerance. This tool assists advisors in crafting optimized investment strategies and making data-backed decisions swiftly.
- Enhanced Decision-Making: Advisors are equipped with tools that provide deeper insights, improving investment decision quality.
- Personalized Client Services: Clients receive investment advice customized to their financial goals.
- Improved Portfolio Performance: The precision of AI analytics helps improve overall portfolio returns and client wealth growth.
- Increased Client Trust: More informed advice and consistent portfolio success help to build and maintain client trust.
- Precision in Personalization: Leveraging AI to tailor services to individual needs increases client satisfaction.
- Empowered Advisors: AI tools support advisors by providing them with a comprehensive analytical base for their recommendations.
- Market Adaptability: AI-driven tools adapt quickly to market changes, providing real-time insights for better responsiveness.
Morgan Stanley plans to develop its AI capabilities further to include predictive analytics for future market conditions, enhancing the proactive management of client portfolios. Natural language processing enhancement could also improve the system’s ability to extract actionable insights from global financial news.
Related: Banking Cybersecurity Case Studies
Case Study 16: Lloyds Banking Group: Optimizing Operational Efficiency
Maintaining operational efficiency in managing customer inquiries and processing transactions is essential for preserving customer satisfaction and minimizing overhead costs. Lloyds Banking Group faced challenges related to operational delays, human error, and the high cost of maintaining quality customer service.
Lloyds implemented an AI-driven process automation system across its customer service and transaction processing units. This system uses robotics process automation (RPA) and AI to handle jobs such as data entry, transaction processing, and customer inquiry responses. AI integration helps identify patterns to optimize workflows and predict peak periods for deploying additional resources.
- Streamlined Operations: AI automation significantly reduces the time required for processing transactions and responding to customer inquiries.
- Cost Efficiency: Decreasing the reliance on manual labor for routine tasks reduces operational costs.
- Enhanced Customer Satisfaction: Quiker processing times and fewer errors in customer service improve overall satisfaction.
- Scalability: The system can readily expand to manage higher loads during peak periods without incurring extra resource costs.
- Automation in Service Delivery: Applying AI in routine tasks frees up human resources for more complicated and valuable activities.
- Adaptive Resource Management: AI’s ability to predict demand helps optimize resource allocation.
- Continuous Improvement: AI systems provide ongoing insights into operational processes, which can be used to refine and improve workflows continuously.
Lloyds plans to expand the use of AI in customer service, risk management, and fraud detection, leveraging AI’s predictive capabilities to enhance security and efficiency. Further integration with IoT devices could also offer real-time data for better operational management across its physical branches.
Case Study 17: American Express: Improving Customer Retention with AI
Customer retention is vital for financial institutions, requiring sophisticated strategies to understand and predict customer behaviors and needs. American Express faced challenges in retaining customers considering other competitive offerings due to a lack of personalized engagement and timely offers.
American Express implemented an AI-driven analytics tool that utilizes customer data to forecast churn and pinpoint factors leading to customer dissatisfaction. The AI system analyzes spending patterns, customer service interactions, and feedback to offer personalized rewards and services that align with individual preferences and requirements, thus enhancing customer loyalty and retention.
- Reduced Customer Churn: Personalized engagement strategies have effectively reduced the rate of customer churn.
- Increased Customer Loyalty: Targeted rewards and services improve customer satisfaction and loyalty.
- Enhanced Personalization: The AI system allows for high customization in customer interactions.
- Data-Driven Decision Making: Insights from AI analytics help shape strategic decisions regarding customer relationship management.
- Predictive Analytics: Understanding and predicting customer behavior can preemptively address potential issues, enhancing retention.
- Customer Insights: Deep learning about customer preferences enables more effective targeted marketing and loyalty programs.
- Strategic Personalization: AI facilitates a more strategic approach to personalization, increasing its effectiveness.
American Express plans to enhance its AI models to integrate real-time data processing, allowing even faster and more accurate personalization of services. Expanding AI applications to include voice and image recognition could offer more intuitive and interactive ways for customers to engage with their services.
Case Study 18: UniCredit: AI-Driven Debt Collection
Debt collection is a critical function for banks but often involves sensitive negotiations that can impact customer relations. UniCredit faced challenges in managing collections efficiently while maintaining positive customer relationships, especially with an increasing volume of delinquent accounts.
UniCredit implemented an AI system designed to optimize debt collection processes. The system segments customers based on their payment history and behavioral patterns to tailor collection strategies most likely to result in successful repayment. It also utilizes natural language processing to automate and personalize customer communication, making interactions more respectful and customer-friendly.
- Improved Recovery Rates: Tailored strategies have led to higher success rates in debt recovery.
- Customer-Centric Collections: Personalized communication helps maintain positive relationships with customers during collections.
- Operational Efficiency: AI automation reduces the labor-intensive aspects of debt collection.
- Enhanced Compliance: The system guarantees compliance with regulatory standards in all collection efforts.
- Behavioral Segmentation: Grasping customer behavior is essential for crafting effective collection strategies.
- Communication Automation: Automated, personalized communication can improve the customer experience, even in collections.
- Efficiency and Compliance: AI tools can enhance operational efficiency and ensure compliance in sensitive operational areas like debt collection.
UniCredit is looking to further refine its AI capabilities by integrating more advanced predictive analytics to anticipate late payments before they occur. Additional enhancements may include sentiment analysis to understand customer emotions during communications better and adjust strategies accordingly.
Case Study 19: TD Bank: Enhancing Branch Experience with AI
TD Bank aimed to revolutionize the traditional branch visit experience to meet customer expectations for efficiency and better personalization. With many consumers preferring digital interactions but still valuing face-to-face consultations, the bank faced the challenge of integrating these preferences seamlessly within its branches.
TD Bank implemented AI-driven kiosks within its branches. These kiosks use facial recognition and machine learning to identify returning customers, predict their banking needs based on past interactions, and offer personalized service options. The system is designed to streamline routine transactions and guide customers to the appropriate service areas or personnel, enhancing the overall efficiency and personalization of the branch experience.
- Reduced Wait Times: Automated kiosks speed up service delivery, shortening customer wait times.
- Increased Customer Satisfaction: Personalized interactions at the branch level improve the overall customer experience.
- Enhanced Operational Efficiency: The kiosks manage routine inquiries and transactions, allowing staff to address more complex customer needs.
- Improved Personalization: AI enables a more tailored approach to each customer visit, anticipating needs and preferences.
- Integration of Digital and Physical: Bridging digital technology with physical branch services can enhance customer engagement.
- Customer Data Utilization: Strategic utilization of customer data can substantially enhance service personalization and efficiency.
- Staff Optimization: AI tools can free staff to focus on high-value interactions, improving job satisfaction and customer service.
TD Bank plans to expand the capabilities of its AI kiosks, including multilingual support and more complex problem-solving abilities, to cater to a broader customer base and enhance the depth of service personalization.
Case Study 20: BBVA: AI in Loan Risk Management
BBVA needed to improve its loan risk management to minimize defaults and optimize loan pricing. The traditional risk assessment models were not sufficient to accurately predict the risk associated with new loan applications, especially in an economically volatile environment.
BBVA deployed an AI-powered risk management system that leverages deep learning to analyze both traditional and non-traditional data sources, including real-time economic trends, social media sentiment, and transactional behavior. This comprehensive approach enables for a more nuanced and accurate loan risk assessment, leading to better-informed lending decisions.
- Enhanced Risk Assessment: The AI system offers a more comprehensive and precise assessment of borrower risk.
- Reduced Default Rates: Improved risk assessment capabilities lead to lower rates of loan defaults.
- Dynamic Pricing: AI enables more dynamic, individualized loan pricing based on calculated risk, improving profit margins.
- Regulatory Compliance: The system ensures compliance with international lending regulations by providing detailed audit trails.
- Comprehensive Data Analysis: Utilizing a wider array of data sources enhances the accuracy of risk predictions.
- Dynamic Adaptability: AI systems can adjust to fluctuating market conditions, delivering insights in real-time.
- Precision Lending: More precise risk assessments allow for better-targeted lending policies.
BBVA is considering further integration of AI to continuously monitor loan performance and borrower health, enabling proactive adjustments to loan terms and conditions. The bank also plans to enhance AI interaction with global economic models to predict better future financial scenarios impacting loan performance.
Related: AI in Finance Case Studies
The integration of AI in banking, as demonstrated through these 20 case studies, marks a significant leap toward a more efficient, secure, and customer-centric future in finance. Banks like JP Morgan Chase, Bank of America, HSBC, Citibank, and Santander are at the forefront, harnessing AI to enhance decision-making, streamline operations, and enrich customer interactions. These cases vividly illustrate how AI can effectively address traditional banking challenges, driving significant service delivery and risk management improvements. As the banking industry continues to evolve, the strategic deployment of AI will not only be a competitive advantage but a necessity, paving the way for innovative solutions that meet the complex demands of modern finance.
- What is Sentient AI [Pros & Cons][Deep Analysis] [2024]
- AI in Product Development [5 Case Studies] [2024]
Team DigitalDefynd
We help you find the best courses, certifications, and tutorials online. Hundreds of experts come together to handpick these recommendations based on decades of collective experience. So far we have served 4 Million+ satisfied learners and counting.
Will Banking jobs be Automated or replaced by AI? [2024]

How can Leaders make use of Artificial Intelligence [2024]

20 AI Programming Languages [2024]

How Can AI Be Used in Sustainability? [5 Case Studies][2024]

10 Ways AI is Revolutionizing Ticketing [2024]

How is AI being used in Predictive Policing? [2024]
Minna Bank: Japan's first digital bank
Japan’s digital native consumers don't need a brick-and-mortar banking experience, so Minna Bank built a different bank for them—in the cloud.
5-MINUTE READ
Call for change
First came the digital natives. Then, the financial technology companies flexed their muscles. Next, we saw a variety of non-banking companies entering the banking field. With all of these rule changes and paradigm shifts affecting banking on a global level, Bank of Fukuoka, the core bank of the Fukuoka Financial Group (FFG), based in Kyushu, Japan, knew they needed to transform. “The number of customers visiting traditional branches of the FFG decreased by 40% over the past 10 years, while the number of customers using internet banking increased by 2.4 times over the same period,” said Koji Yokota, President, Minna Bank. To create a bank for everyone—including digital natives—FFG would have to change. But how?
FFG began by establishing iBank Marketing Corporation, a platform company to explore potential business models for the bank of the future by connecting the financial and non-financial sectors with local communities. Kenichi Nagayoshi, the founder of iBank Marketing and Director and Vice President of Minna Bank, explained:
"Our mission was to create innovative financial services, which is why we launched iBank Marketing to develop simple financial functions and digital marketing, with data and analytics at its core. Our core product app, Wallet+, has been downloaded more than 1.6 million times. We thought it was time to create a new platform for financial services now that the game is changing."

We chose Accenture as our partner largely because of their global digital expertise in technology, in design, and in data analytics. This, combined with their ability to execute, enabled us to launch our service on time, even in the midst of the COVID-19 pandemic. Accenture is an excellent company and our best partner.
Koji Yokota / President, Minna Bank
Minna Bank has won the "Brand of the Year" award in the brand category of the Red Dot Design Award 2021, one of the world's three major design awards. They are the first Japanese company to win this award, and the first financial institution in the world to win it. The company also won "Best of the Best" (the highest award of the year) in the Communication Design category (Applications) and "Red Dot" in the Communication Design category (Brand Design & Identity), winning three awards simultaneously.
When tech meets human ingenuity
Under these circumstances, FFG is implementing a "two-way approach" in digital transformation. While FFG, which has a traditional bank, is steadily implementing digital transformation, the approach is to establish Japan's first digital bank, Minna Bank, as an organization to implement digital transformation in a single step without the constraints of the existing business. This bank was the first bank in the world to build a full cloud banking system, and the system was built in the midst of a pandemic, with overwhelming speed.
Minna Bank was designed as a digital technology company that provides financial services to digital native customers. “We looked all over the world for a suitable platform for a digital bank, but there was no banking system built in the public cloud. So we decided to create a full cloud bank ourselves,“ said Nagayoshi.
Accenture is providing support in the adoption of Agile development and in multiple areas such as automation, strategy and talent development. Its Banking, Strategy & Consulting, Technology, and Interactive teams have come together from Fukuoka, Osaka, Tokyo, Aizu, Hokkaido and two overseas locations, transcending national and organizational boundaries to partner with Minna Bank.
In addition to its own resources, Accenture has drawn on its vast ecosystem of technology partners—in this case, industry leaders such as Google, Microsoft, AWS, Salesforce and Oracle—to take advantage of their solutions and best practices.
Specifically, in the "Zero Bank Core Solution" jointly developed by Minna Bank and Accenture, the core system will be implemented on Google Cloud using Accenture's Digital Experience and cloud-first approach, connected technology and cloud-native core solution. For contact center operations, Amazon Web Services (AWS), Amazon Connect and Salesforce's Service Cloud have been combined. Microsoft's Azure is being used for the virtual desktop infrastructure for employee and system operations, and Oracle Cloud is being used for the accounting system. Collaboration with these solution providers has allowed Minna Bank to build its foundation as a cloud-first business with the latest technology available worldwide.
In 2020, in the midst of the COVID-19 pandemic, the Minna Bank project team continued to press forward. It took no more than 18 months to invent and launch a transformational bank in a country with strict regulations governing financial institutions—an unprecedented achievement.
"If it wasn't for cloud, we would have been six months late in opening. Cloud's scalability, speed of deployment and efficiency in fixing bugs are the reasons for the agility of our banking services," said Yokota.

A valuable difference
Minna Bank differs from traditional banks not only by virtue of its operating model, but also its marketing and promotion. Instead of using mass media, it actively employs social media and develops promotions by observing mentions among users. This approach is made possible by a user interface and experience that perfectly matches the preferences of the bank’s target market. To target digital natives, Accenture's team of designers pursued a simple and appealing graphical presentation with minimal descriptive information.
The planning and design process started with a thorough understanding of the thinking and behavior of digital natives, and a commitment to develop services from the customer's perspective: when and how do they want to use financial services? This approach enabled Minna Bank to become a frictionless app that people want to use every day. It is also a portal for non-financial services, providing great value to customers by turning data-based marketing into a service. "We are the first bank in Japan to truly integrate financial and non-financial data into a single service," said Nagayoshi.
Minna Bank has three core business concepts:
- Give shape to everyone's voice—provide new financial services in line with changes in customer behavior.
- Deliver the best for everyone—become a comprehensive financial concierge based on an understanding of customers.
- Integrate into people's daily lives—realize the concept of a BaaS (Banking as a Service) business.
BaaS is a new banking system offering based on the Accenture Cloud Native Core Solution. It helps business partners to create new value in the banking industry.
Minna Bank, a unique digital entity, is a bank for the age of a data-driven society. It will continue to be a bank that explores the potential of hyper-personalization and makes customers say "Wow!”
"As Japan's first digital bank, Minna Bank will be the epicenter of innovation in the Japanese financial industry. Accenture is committed to continuing to be an engine of innovation for Minna Bank," said Masashi Nakano, Senior Managing Director, Financial Services, Accenture.
Japan's first digital bank
Launched the business in 18 months
of employees are engineers
As Japan's first digital bank, Minna Bank will be the epicenter of innovation in the Japanese financial industry. Accenture is committed to continuing to be an engine of innovation for Minna Bank
Masashi Nakano / Senior Managing Director, Financial Services, Accenture Japan Ltd
Built for change podcast
Listen to our award-winning podcast, Built for Change: Adweek Podcast of the Year Award Winner for Best Thought Leadership Podcast.
EPISODE 11: Preparing for the Society of the Future
A select group of companies recognize that emerging consumer and investor lifestyle shifts will have a tremendous impact on business in the future. Learn how these “forerunners” are charting a course to growth by prioritizing ethical usage of technology, environmental sustainability, human care and more.
Related capabilities
- Cloud services
- Banking cloud services
MEET THE TEAM
Masashi Nakano
Senior Managing Director – Financial Services, Accenture Japan Ltd
Koji Miyara
Managing Director – Banking Lead, Financial Services, Accenture Japan Ltd
Kentaro Mori
Managing Director – Strategy, Banking Lead, Accenture Strategy & Consulting, Accenture Japan Ltd
Keisuke Yamane
Senior Managing Director – Accenture Technology Cloud Infrastructure Engineering Lead, Accenture Technology Intelligent Software Engineering Services Co-Lead, Japan
Ryota Mochizuki
Managing Director – Accenture Interactive, Accenture Japan Ltd
