Thank you for visiting nature.com. You are using a browser version with limited support for CSS. To obtain the best experience, we recommend you use a more up to date browser (or turn off compatibility mode in Internet Explorer). In the meantime, to ensure continued support, we are displaying the site without styles and JavaScript.
- View all journals
- Explore content
- About the journal
- Publish with us
- Sign up for alerts

Fluid dynamics articles within Scientific Reports
Article 13 August 2024 | Open Access
Unified framework for laser-induced transient bubble dynamics within microchannels
- Nagaraj Nagalingam
- , Vikram Korede
- & Hüseyin Burak Eral
Article 12 August 2024 | Open Access
Effect of liquid level monitor gas injection point size on information source amplitude-frequency characteristics
- , Kailun Quan
- & Zhongzhi Hu
Article 09 August 2024 | Open Access
Evaluating the impact of evolving green and grey urban infrastructure on local particulate pollution around city square parks
- Meng-Yi Jin
- , Kiran A Apsunde
- & John Gallagher
Article 05 August 2024 | Open Access
Spacing effects on flows around two square cylinders in staggered arrangement via LBM
- Ahmed Refaie Ali
- , Waqas Sarwar Abbasi
- & Irshad Ahmad
Article 26 July 2024 | Open Access
Electrical properties determine the liquid flow direction in plasma–liquid interactions
- Calum T. Ryan
- , Anton A. Darhuber
- & Ana Sobota
The effect of including dynamic imaging derived airway wall motion in CFD simulations of respiratory airflow in patients with OSA
- , Chamindu Gunatilaka
- & Alister Bates
Article 24 July 2024 | Open Access
A 3-DOF caudal fin for precise maneuvering of thunniform-inspired unmanned underwater vehicles
- Cecilia Huertas-Cerdeira
- & Morteza Gharib
Article 23 July 2024 | Open Access
Numerical study of cavitation shock wave emission in the thin liquid layer by power ultrasonic vibratory machining
- , Xijing Zhu
- & Yingze Fu
Article 22 July 2024 | Open Access
Drag reduction and degradation by sodium alginate in turbulent flow
- Zhensong Cheng
- , Panpan Zhang
- & Yuan Lu
Article 17 July 2024 | Open Access
Nu–Gr correlation for laminar natural convection heat transfer from a sphere submitted to a constant heat flux surface
- & Hongzhi Wang
Article 15 July 2024 | Open Access
\(\text{Sech}^{2}\) -type solitary waves and the stability analysis for the KdV–mKdV equation
- Zhi-Guo Liu
- , Muhua Liu
- & Jinliang Zhang
Optimization of passive micromixers: effects of pillar configuration and gaps on mixing efficiency
- Ali Kheirkhah Barzoki
Article 12 July 2024 | Open Access
Experimental and CFD analysis of fluid flow through nanofiber filter media
- Mehdi Azimian
- , Matin Naderi
- & Andreas Wiegmann
Article 10 July 2024 | Open Access
Estimation of the UV susceptibility of aerosolized SARS-CoV-2 to 254 nm irradiation using CFD-based room disinfection simulations
- Marc van der Schans
- & Genevieve Martin
Article 09 July 2024 | Open Access
Enhanced carbon dioxide drainage observed in digital rock under intermediate wetting conditions
- Jaione Tirapu Azpiroz
- , Ronaldo Giro
- & Mathias B. Steiner
Article 07 July 2024 | Open Access
Performance analysis of linearization schemes for modelling multi-phase flow in porous media
- Abdul Salam Abd
- & Ahmad Abushaikha
Article 06 July 2024 | Open Access
Modeling of scour hole characteristics under turbulent wall jets using machine learning
- Jnana Ranjan Khuntia
- , Kamalini Devi
- & Mohd Aamir Mumtaz
Article 05 July 2024 | Open Access
Enhancing hydrodynamic forces through miniaturized control of square cylinders using the lattice Boltzmann method
Article 02 July 2024 | Open Access
Physics-informed neural network simulation of thermal cavity flow
- Eric Fowler
- , Christopher J. McDevitt
- & Subrata Roy
Article 27 June 2024 | Open Access
Choice of reaction progress variable under preferential diffusion effects in turbulent syngas combustion based on detailed chemistry direct numerical simulations
- Vinzenz Silvester Wehrmann
- , Nilanjan Chakraborty
- & Josef Hasslberger
Article 25 June 2024 | Open Access
Establishing the distribution of cerebrovascular resistance using computational fluid dynamics and 4D flow MRI
- Axel Vikström
- , Petter Holmlund
- & Anders Eklund
Article 19 June 2024 | Open Access
Research on the influence of pits on the propagation law of explosion shock waves
- & Wang Liangquan
Free convection in a square wavy porous cavity with partly magnetic field: a numerical investigation
- Amirmohammad Mirzaei
- , Bahram Jalili
- & Davood Domiri Ganji
Article 13 June 2024 | Open Access
Numerical simulation and theoretical study on the impact of wind-sand flow of high-speed trains in long tunnel space
- , Sihui Dong
- & Liping Zhou
Article 06 June 2024 | Open Access
Fluid-particle-structure interaction in single shot peening
- Yusuke Mizuno
- , Takashi Misaka
- & Yoshiyuki Furukawa
Article 05 June 2024 | Open Access
Equivalent analytical model for liquid sloshing in a 2-D rectangular container with multiple vertical baffles by subdomain partition approach
- & Ding Zhou
Article 03 June 2024 | Open Access
A computational fluid dynamics study to assess the impact of coughing on cerebrospinal fluid dynamics in Chiari type 1 malformation
- Sarah Vandenbulcke
- , Paul Condron
- & Patrick Segers
Article 31 May 2024 | Open Access
Flow regimes identification of air water counter current flow in vertical annulus using differential pressure signals and machine learning
- , Ruirong Dang
- & Zhimeng Sun
Exergoeconomic analysis and optimization of wind power hybrid energy storage system
- Caifeng Wen
- , Yalin Lyu
- & Hongliang Hao
Article 30 May 2024 | Open Access
Capillary wave tweezer
- Bethany Orme
- , Hamdi Torun
- & Prashant Agrawal
Article 28 May 2024 | Open Access
Experimental and numerical investigations of the water surface profile and wave extrema of supercritical flows in a narrow channel bend
- Subhojit Kadia
- , I. A. Sofia Larsson
- & Elena Pummer
Article 17 May 2024 | Open Access
Uncertainty quantification of the lattice Boltzmann method focussing on studies of human-scale vascular blood flow
- Jon W. S. McCullough
- & Peter V. Coveney
Article 14 May 2024 | Open Access
AI-based predictive approach via FFB propagation in a driven-cavity of Ostwald de-Waele fluid using CFD-ANN and Levenberg–Marquardt
- , Rashid Mahmood
- & Mohamed H. Behiry
Thermal modal analysis of hypersonic composite wing on transient aerodynamic heating
- Kangjie Wang
- , Junli Wang
- & Wenyong Quan
Article 08 May 2024 | Open Access
A non-invasive capacitive sensor to investigate the Leidenfrost phenomenon: a proof of concept study
- Abhishek S. Purandare
- , Jelle Rijs
- & Srinivas Vanapalli
Article 04 May 2024 | Open Access
Turbulence-induced droplet grouping and augmented rain formation in cumulus clouds
- Siddharth Gumber
- , Sudarsan Bera
- & Thara V. Prabhakaran
Predicting the vertical density structure of oceanic gravity current intrusions
- Sévan Rétif
- , Maria Eletta Negretti
- & Achim Wirth
Article 22 April 2024 | Open Access
Experimental investigation of drag loss behavior of dip-lubricated wet clutches for building a data-driven prediction model
- Lukas Pointner-Gabriel
- , Max Menzel
- & Karsten Stahl
Article 20 April 2024 | Open Access
The air conditioning in the nose of mammals depends on their mass and on their maximal running speed
- Clément Rigaut
- , Alice Giaprakis
- & Benoît Haut
Article 16 April 2024 | Open Access
Label-free separation of peripheral blood mononuclear cells from whole blood by gradient acoustic focusing
- Julia Alsved
- , Mahdi Rezayati Charan
- & Per Augustsson
Biogeochemical dynamics in a marine storm demonstrates differences between natural and anthropogenic impacts
- Justin Tiano
- , Rob Witbaard
- & Karline Soetaert
Article 13 April 2024 | Open Access
Study on dynamic characteristics of cavitation in underwater explosion with large charge
- , Xian-pi Zhang
- & Yuan-Qing Xu
Article 09 April 2024 | Open Access
Bubble dynamics and atomization of acoustically levitated diesel and biodiesel droplets using femtosecond laser pulses
- Vishal S. Jagadale
- , Devendra Deshmukh
- & Yogeshwar Nath Mishra
Article 08 April 2024 | Open Access
Evaluating the accuracy of cerebrovascular computational fluid dynamics modeling through time-resolved experimental validation
- Claudio A. Luisi
- , Tom L. Witter
- & Michael Neidlin
Toward the eco-friendly cosmetic cleansing assisted by the micro-bubbly jet
- , Jooyeon Park
- & Hyungmin Park
Article 02 April 2024 | Open Access
Velocity field and turbulence structure of the meandering flow produced by alternating deflectors
- Jie-min Zhan
- , Wing-hong Onyx Wai
- & Ying-ying Luo

The impact of a chemical reaction on the heat and mass transfer mechanisms in a dissipative and radiative nanofluid flow over a nonlinear stretching sheet
- , Ahmed M. Megahed
- & Eman Fares
Article 31 March 2024 | Open Access
Morphogenesis of a chiral liquid crystalline droplet with topological reconnection and Lehmann rotation
- Jun Yoshioka
- & Koji Fukao
Article 30 March 2024 | Open Access
Response spectrum-based analysis of airborne radar random vibration and multi-point control improvement
- , Zezheng Liu
- & Libin Du
Article 29 March 2024 | Open Access
High-efficiency and low-hazard artillery recoil reduction technology based on barrel gas reflection
- , Jinsong Dai
- & Xiaopeng Su
Browse broader subjects
Quick links.
- Explore articles by subject
- Guide to authors
- Editorial policies

Information
- Author Services
Initiatives
You are accessing a machine-readable page. In order to be human-readable, please install an RSS reader.
All articles published by MDPI are made immediately available worldwide under an open access license. No special permission is required to reuse all or part of the article published by MDPI, including figures and tables. For articles published under an open access Creative Common CC BY license, any part of the article may be reused without permission provided that the original article is clearly cited. For more information, please refer to https://www.mdpi.com/openaccess .
Feature papers represent the most advanced research with significant potential for high impact in the field. A Feature Paper should be a substantial original Article that involves several techniques or approaches, provides an outlook for future research directions and describes possible research applications.
Feature papers are submitted upon individual invitation or recommendation by the scientific editors and must receive positive feedback from the reviewers.
Editor’s Choice articles are based on recommendations by the scientific editors of MDPI journals from around the world. Editors select a small number of articles recently published in the journal that they believe will be particularly interesting to readers, or important in the respective research area. The aim is to provide a snapshot of some of the most exciting work published in the various research areas of the journal.
Original Submission Date Received: .
- Active Journals
- Find a Journal
- Proceedings Series
- For Authors
- For Reviewers
- For Editors
- For Librarians
- For Publishers
- For Societies
- For Conference Organizers
- Open Access Policy
- Institutional Open Access Program
- Special Issues Guidelines
- Editorial Process
- Research and Publication Ethics
- Article Processing Charges
- Testimonials
- Preprints.org
- SciProfiles
- Encyclopedia
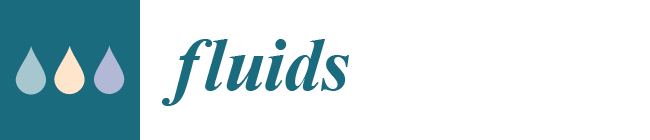
Article Menu
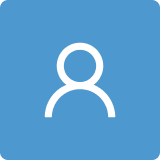
- Subscribe SciFeed
- Recommended Articles
- Google Scholar
- on Google Scholar
- Table of Contents
Find support for a specific problem in the support section of our website.
Please let us know what you think of our products and services.
Visit our dedicated information section to learn more about MDPI.
JSmol Viewer
Current trends in fluid research in the era of artificial intelligence: a review.

1. Introduction
1.1. data science, 1.2. ai/ml in fluid research, 1.3. reviews and perspectives on fluid research and ml, 1.4. aim and objectives, 2. bridging across scales, 3. fluid properties extraction, 4. physics-based cfd, 5. algorithms for fluid flows, 5.1. multiple linear regression, 5.2. ridge regression, 5.3. lasso regression, 5.4. support vector machines, 5.5. gaussian process regression, 5.6. k-nearest neighbors, 5.7. decision trees, 5.8. random forest, 5.9. gradient boosting, 5.10. artificial neural networks, 5.11. symbolic regression, 5.12. performance metrics, 6. comparative investigation, 7. conclusions and future perspectives, author contributions, institutional review board statement, informed consent statement, data availability statement, conflicts of interest, nomenclature.
English Symbols | |
bias term | |
B | number of decision trees in RF method |
D | diffusion coefficient |
external driving force | |
DT function estimation | |
g | ith sample size of data for k-NN regression |
g | the result of query point prediction for k-NN regression |
channel width | |
(x) | function for GBR method |
DT indicator function | |
k | number of neighbors for k-NN regression |
k | Boltzmann constant |
m | particle mass |
MAE | Mean Absolute Error |
MSE | Mean Squared Error |
N | number of particles |
weight for GBR method | |
coefficient of determination | |
distance vector between ith and jth atom | |
T | temperature |
LJ potential of atom i with atom j | |
weight of the variable | |
X’ | number of unknown scenarios in RF method |
input variable | |
predicted variable | |
Y | decision tree in RF method |
mean expected output | |
mean predicted output | |
Greek Symbols | |
penalized residual sum for Ridge regression | |
shrinkage factor | |
Lasso regression estimate | |
ε | energy parameter in the LJ potential |
DT decision path | |
λ | thermal conductivity |
μ | coefficient of shear viscosity |
ρ | fluid density |
σ | length parameter in the LJ potential |
- Agrawal, A.; Choudhary, A. Perspective: Materials Informatics and Big Data: Realization of the “Fourth Paradigm” of Science in Materials Science. APL Mater. 2016 , 4 , 053208. [ Google Scholar ] [ CrossRef ] [ Green Version ]
- Agrawal, A.; Deshpande, P.D.; Cecen, A.; Basavarsu, G.P.; Choudhary, A.N.; Kalidindi, S.R. Exploration of Data Science Techniques to Predict Fatigue Strength of Steel from Composition and Processing Parameters. Integr. Mater. Manuf. Innov. 2014 , 3 , 90–108. [ Google Scholar ] [ CrossRef ] [ Green Version ]
- Wei, J.; Chu, X.; Sun, X.; Xu, K.; Deng, H.; Chen, J.; Wei, Z.; Lei, M. Machine Learning in Materials Science. InfoMat 2019 , 1 , 338–358. [ Google Scholar ] [ CrossRef ]
- Paulson, N.H.; Zomorodpoosh, S.; Roslyakova, I.; Stan, M. Comparison of Statistically-Based Methods for Automated Weighting of Experimental Data in CALPHAD-Type Assessment. Calphad 2020 , 68 , 101728. [ Google Scholar ] [ CrossRef ]
- Frank, M.; Drikakis, D.; Charissis, V. Machine-Learning Methods for Computational Science and Engineering. Computation 2020 , 8 , 15. [ Google Scholar ] [ CrossRef ] [ Green Version ]
- Wang, T.; Zhang, C.; Snoussi, H.; Zhang, G. Machine Learning Approaches for Thermoelectric Materials Research. Adv. Funct. Mater. 2020 , 30 , 1906041. [ Google Scholar ] [ CrossRef ]
- Alexiadis, A. Deep Multiphysics: Coupling Discrete Multiphysics with Machine Learning to Attain Self-Learning in-Silico Models Replicating Human Physiology. Artif. Intell. Med. 2019 , 98 , 27–34. [ Google Scholar ] [ CrossRef ]
- Brenner, M.P.; Eldredge, J.D.; Freund, J.B. Perspective on Machine Learning for Advancing Fluid Mechanics. Phys. Rev. Fluids 2019 , 4 , 100501. [ Google Scholar ] [ CrossRef ]
- Schmid, M.; Altmann, D.; Steinbichler, G. A Simulation-Data-Based Machine Learning Model for Predicting Basic Parameter Settings of the Plasticizing Process in Injection Molding. Polymers 2021 , 13 , 2652. [ Google Scholar ] [ CrossRef ]
- Goh, G.D.; Sing, S.L.; Yeong, W.Y. A Review on Machine Learning in 3D Printing: Applications, Potential, and Challenges. Artif. Intell. Rev. 2021 , 54 , 63–94. [ Google Scholar ] [ CrossRef ]
- Kailkhura, B.; Gallagher, B.; Kim, S.; Hiszpanski, A.; Han, T.Y.-J. Reliable and Explainable Machine-Learning Methods for Accelerated Material Discovery. Npj Comput. Mater. 2019 , 5 , 108. [ Google Scholar ] [ CrossRef ]
- Sofos, F. A Water/Ion Separation Device: Theoretical and Numerical Investigation. Appl. Sci. 2021 , 11 , 8548. [ Google Scholar ] [ CrossRef ]
- Roscher, R.; Bohn, B.; Duarte, M.F.; Garcke, J. Explainable Machine Learning for Scientific Insights and Discoveries. IEEE Access 2020 , 8 , 42200–42216. [ Google Scholar ] [ CrossRef ]
- Vasudevan, R.K.; Choudhary, K.; Mehta, A.; Smith, R.; Kusne, G.; Tavazza, F.; Vlcek, L.; Ziatdinov, M.; Kalinin, S.V.; Hattrick-Simpers, J. Materials Science in the Artificial Intelligence Age: High-Throughput Library Generation, Machine Learning, and a Pathway from Correlations to the Underpinning Physics. MRS Commun. 2019 , 9 , 821–838. [ Google Scholar ] [ CrossRef ] [ Green Version ]
- Craven, G.T.; Lubbers, N.; Barros, K.; Tretiak, S. Machine Learning Approaches for Structural and Thermodynamic Properties of a Lennard-Jones Fluid. J. Chem. Phys. 2020 , 153 , 104502. [ Google Scholar ] [ CrossRef ]
- Sun, W.; Zheng, Y.; Yang, K.; Zhang, Q.; Shah, A.A.; Wu, Z.; Sun, Y.; Feng, L.; Chen, D.; Xiao, Z.; et al. Machine Learning-Assisted Molecular Design and Efficiency Prediction for High-Performance Organic Photovoltaic Materials. Sci. Adv. 2019 , 5 , eaay4275. [ Google Scholar ] [ CrossRef ] [ Green Version ]
- Zhang, W.-W.; Noack, B.R. Artificial Intelligence in Fluid Mechanics. Acta Mech. Sin. 2022 , 1–3. [ Google Scholar ] [ CrossRef ]
- Raissi, M.; Perdikaris, P.; Karniadakis, G.E. Physics-Informed Neural Networks: A Deep Learning Framework for Solving Forward and Inverse Problems Involving Nonlinear Partial Differential Equations. J. Comput. Phys. 2019 , 378 , 686–707. [ Google Scholar ] [ CrossRef ]
- Papastamatiou, K.; Sofos, F.; Karakasidis, T.E. Machine Learning Symbolic Equations for Diffusion with Physics-Based Descriptions. AIP Adv. 2022 , 12 , 025004. [ Google Scholar ] [ CrossRef ]
- Brunton, S.L.; Noack, B.R.; Koumoutsakos, P. Machine Learning for Fluid Mechanics. Annu. Rev. Fluid Mech. 2020 , 52 , 477–508. [ Google Scholar ] [ CrossRef ] [ Green Version ]
- Brunton, S.L. Applying Machine Learning to Study Fluid Mechanics. Acta Mech. Sin. 2022 , 1–9. [ Google Scholar ] [ CrossRef ]
- Jirasek, F.; Hasse, H. Perspective: Machine Learning of Thermophysical Properties. Fluid Phase Equilib. 2021 , 549 , 113206. [ Google Scholar ] [ CrossRef ]
- Arief, H.A.; Wiktorski, T.; Thomas, P.J. A Survey on Distributed Fibre Optic Sensor Data Modelling Techniques and Machine Learning Algorithms for Multiphase Fluid Flow Estimation. Sensors 2021 , 21 , 2801. [ Google Scholar ] [ CrossRef ]
- Pandey, S.; Schumacher, J.; Sreenivasan, K.R. A Perspective on Machine Learning in Turbulent Flows. J. Turbul. 2020 , 21 , 567–584. [ Google Scholar ] [ CrossRef ]
- Botu, V.; Ramprasad, R. Learning Scheme to Predict Atomic Forces and Accelerate Materials Simulations. Phys. Rev. B Condens. Matter Mater. Phys. 2015 , 92 , 094306. [ Google Scholar ] [ CrossRef ] [ Green Version ]
- Behler, J.; Parrinello, M. Generalized Neural-Network Representation of High-Dimensional Potential-Energy Surfaces. Phys. Rev. Lett. 2007 , 98 , 146401. [ Google Scholar ] [ CrossRef ] [ PubMed ]
- Noé, F.; Olsson, S.; Köhler, J.; Wu, H. Boltzmann Generators: Sampling Equilibrium States of Many-Body Systems with Deep Learning. Science 2019 , 365 , eaaw1147. [ Google Scholar ] [ CrossRef ] [ PubMed ] [ Green Version ]
- Sofos, F.; Karakasidis, T.E.; Liakopoulos, A. Fluid Flow at the Nanoscale: How Fluid Properties Deviate from the Bulk. Nanosci. Nanotechnol. Lett. 2013 , 5 , 457–460. [ Google Scholar ] [ CrossRef ]
- Sofos, F.; Karakasidis, T.E.; Giannakopoulos, A.E.; Liakopoulos, A. Molecular Dynamics Simulation on Flows in Nano-Ribbed and Nano-Grooved Channels. Heat Mass Transf./Waerme Stoffuebertragung 2016 , 52 , 153–162. [ Google Scholar ] [ CrossRef ]
- Mueller, T.; Hernandez, A.; Wang, C. Machine Learning for Interatomic Potential Models. J. Chem. Phys. 2020 , 152 , 050902. [ Google Scholar ] [ CrossRef ] [ PubMed ] [ Green Version ]
- Krems, R.V. Bayesian Machine Learning for Quantum Molecular Dynamics. Phys. Chem. Chem. Phys. 2019 , 21 , 13392–13410. [ Google Scholar ] [ CrossRef ] [ Green Version ]
- Mishin, Y. Machine-Learning Interatomic Potentials for Materials Science. Acta Mater. 2021 , 214 , 116980. [ Google Scholar ] [ CrossRef ]
- Veit, M.; Jain, S.K.; Bonakala, S.; Rudra, I.; Hohl, D.; Csányi, G. Equation of State of Fluid Methane from First Principles with Machine Learning Potentials. J. Chem. Theory Comput. 2019 , 15 , 2574–2586. [ Google Scholar ] [ CrossRef ] [ Green Version ]
- Peng, G.C.Y.; Alber, M.; Buganza Tepole, A.; Cannon, W.R.; De, S.; Dura-Bernal, S.; Garikipati, K.; Karniadakis, G.; Lytton, W.W.; Perdikaris, P.; et al. Multiscale Modeling Meets Machine Learning: What Can We Learn? Arch. Comput. Methods Eng. 2021 , 28 , 1017–1037. [ Google Scholar ] [ CrossRef ] [ Green Version ]
- Tchipev, N.; Seckler, S.; Heinen, M.; Vrabec, J.; Gratl, F.; Horsch, M.; Bernreuther, M.; Glass, C.W.; Niethammer, C.; Hammer, N.; et al. TweTriS: Twenty Trillion-Atom Simulation. Int. J. High Perform. Comput. Appl. 2019 , 33 , 838–854. [ Google Scholar ] [ CrossRef ] [ Green Version ]
- Mortazavi, B.; Podryabinkin, E.V.; Roche, S.; Rabczuk, T.; Zhuang, X.; Shapeev, A.V. Machine-Learning Interatomic Potentials Enable First-Principles Multiscale Modeling of Lattice Thermal Conductivity in Graphene/Borophene Heterostructures. Mater. Horiz. 2020 , 7 , 2359–2367. [ Google Scholar ] [ CrossRef ]
- Holland, D.M.; Lockerby, D.A.; Borg, M.K.; Nicholls, W.D.; Reese, J.M. Molecular Dynamics Pre-Simulations for Nanoscale Computational Fluid Dynamics. Microfluid. Nanofluid. 2015 , 18 , 461–474. [ Google Scholar ] [ CrossRef ] [ Green Version ]
- Lin, C.; Li, Z.; Lu, L.; Cai, S.; Maxey, M.; Karniadakis, G.E. Operator Learning for Predicting Multiscale Bubble Growth Dynamics. J. Chem. Phys. 2021 , 154 , 104118. [ Google Scholar ] [ CrossRef ]
- Wang, Y.; Ouyang, J.; Wang, X. Machine Learning of Lubrication Correction Based on GPR for the Coupled DPD–DEM Simulation of Colloidal Suspensions. Soft Matter 2021 , 17 , 5682–5699. [ Google Scholar ] [ CrossRef ]
- Sofos, F.; Chatzoglou, E.; Liakopoulos, A. An Assessment of SPH Simulations of Sudden Expansion/Contraction 3-D Channel Flows. Comput. Part. Mech. 2022 , 9 , 101–115. [ Google Scholar ] [ CrossRef ]
- Albano, A.; Alexiadis, A. A Smoothed Particle Hydrodynamics Study of the Collapse for a Cylindrical Cavity. PLoS ONE 2020 , 15 , e0239830. [ Google Scholar ] [ CrossRef ] [ PubMed ]
- Bai, J.; Zhou, Y.; Rathnayaka, C.M.; Zhan, H.; Sauret, E.; Gu, Y. A Data-Driven Smoothed Particle Hydrodynamics Method for Fluids. Eng. Anal. Bound. Elem. 2021 , 132 , 12–32. [ Google Scholar ] [ CrossRef ]
- Wang, J.; Olsson, S.; Wehmeyer, C.; Pérez, A.; Charron, N.E.; De Fabritiis, G.; Noé, F.; Clementi, C. Machine Learning of Coarse-Grained Molecular Dynamics Force Fields. ACS Cent. Sci. 2019 , 5 , 755–767. [ Google Scholar ] [ CrossRef ] [ Green Version ]
- Wang, W.; Gómez-Bombarelli, R. Coarse-Graining Auto-Encoders for Molecular Dynamics. npj Comput. Mater. 2019 , 5 , 125. [ Google Scholar ] [ CrossRef ] [ Green Version ]
- Scherer, C.; Scheid, R.; Andrienko, D.; Bereau, T. Kernel-Based Machine Learning for Efficient Simulations of Molecular Liquids. J. Chem. Theory Comput. 2020 , 16 , 3194–3204. [ Google Scholar ] [ CrossRef ] [ PubMed ]
- Ye, H.; Xian, W.; Li, Y. Machine Learning of Coarse-Grained Models for Organic Molecules and Polymers: Progress, Opportunities, and Challenges. ACS Omega 2021 , 6 , 1758–1772. [ Google Scholar ] [ CrossRef ] [ PubMed ]
- Moradzadeh, A.; Aluru, N.R. Transfer-Learning-Based Coarse-Graining Method for Simple Fluids: Toward Deep Inverse Liquid-State Theory. J. Phys. Chem. Lett. 2019 , 10 , 1242–1250. [ Google Scholar ] [ CrossRef ] [ PubMed ]
- Noé, F.; Tkatchenko, A.; Müller, K.-R.; Clementi, C. Machine Learning for Molecular Simulation. Annu. Rev. Phys. Chem. 2020 , 71 , 361–390. [ Google Scholar ] [ CrossRef ] [ PubMed ] [ Green Version ]
- Giannakopoulos, A.E.; Sofos, F.; Karakasidis, T.E.; Liakopoulos, A. A Quasi-Continuum Multi-Scale Theory for Self-Diffusion and Fluid Ordering in Nanochannel Flows. Microfluid. Nanofluid. 2014 , 17 , 1011–1023. [ Google Scholar ] [ CrossRef ]
- Allers, J.P.; Garzon, F.H.; Alam, T.M. Artificial Neural Network Prediction of Self-Diffusion in Pure Compounds over Multiple Phase Regimes. Phys. Chem. Chem. Phys. 2021 , 23 , 4615–4623. [ Google Scholar ] [ CrossRef ] [ PubMed ]
- De Pablo, J.J.; Jackson, N.E.; Webb, M.A.; Chen, L.Q.; Moore, J.E.; Morgan, D.; Jacobs, R.; Pollock, T.; Schlom, D.G.; Toberer, E.S.; et al. New Frontiers for the Materials Genome Initiative. npj Comput. Mater. 2019 , 5 , 41. [ Google Scholar ] [ CrossRef ]
- Jakob, J.; Gross, M.; Günther, T. A fluid flow data set for machine learning and its application to neural flow map interpolation. IEEE Trans. Vis. Comput. Graph. 2020 , 27 , 1279–1289. [ Google Scholar ] [ CrossRef ]
- Curtarolo, S.; Setyawan, W.; Hart, G.L.W.; Jahnatek, M.; Chepulskii, R.V.; Taylor, R.H.; Wang, S.; Xue, J.; Yang, K.; Levy, O.; et al. AFLOW: An Automatic Framework for High-Throughput Materials Discovery. Comput. Mater. Sci. 2012 , 58 , 218–226. [ Google Scholar ] [ CrossRef ] [ Green Version ]
- Draxl, C.; Scheffler, M. NOMAD: The FAIR Concept for Big Data-Driven Materials Science. MRS Bull. 2018 , 43 , 676–682. [ Google Scholar ] [ CrossRef ] [ Green Version ]
- Jain, A.; Ong, S.P.; Hautier, G.; Chen, W.; Richards, W.D.; Dacek, S.; Cholia, S.; Gunter, D.; Skinner, D.; Ceder, G.; et al. The Materials Project: A Materials Genome Approach to Accelerating Materials Innovation. APL Mater. 2013 , 1 , 011002. [ Google Scholar ] [ CrossRef ] [ Green Version ]
- Wang, W.; Xu, M.; Xu, X.; Zhou, W.; Shao, Z. Perovskite Oxide Based Electrodes for High-Performance Photoelectrochemical Water Splitting. Angew. Chem. Int. Ed. 2020 , 59 , 136–152. [ Google Scholar ] [ CrossRef ]
- Allen, M.P.; Tildesley, D.J. Computer Simulation of Liquids , 2nd ed.; Oxford University Press: Oxford, UK, 2017; ISBN 9780198803195. [ Google Scholar ]
- Allers, J.P.; Harvey, J.A.; Garzon, F.H.; Alam, T.M. Machine Learning Prediction of Self-Diffusion in Lennard-Jones Fluids. J. Chem. Phys. 2020 , 153 , 034102. [ Google Scholar ] [ CrossRef ]
- Udrescu, S.-M.; Tan, A.; Feng, J.; Neto, O.; Wu, T.; Tegmark, M. AI Feynman 2.0: Pareto-Optimal Symbolic Regression Exploiting Graph Modularity. In Proceedings of the 34th Conference on Neural Information Processing Systems (NeurIPS 2020), Vancouver, BC, Canada, 6–12 December 2020; pp. 1–12. [ Google Scholar ]
- Ye, H.F.; Wang, J.; Zheng, Y.G.; Zhang, H.W.; Chen, Z. Machine Learning for Reparameterization of Four-Site Water Models: TIP4P-BG and TIP4P-BGT. Phys. Chem. Chem. Phys. 2021 , 23 , 10164–10173. [ Google Scholar ] [ CrossRef ]
- Liu, Y.; Hong, W.; Cao, B. Machine Learning for Predicting Thermodynamic Properties of Pure Fluids and Their Mixtures. Energy 2019 , 188 , 116091. [ Google Scholar ] [ CrossRef ]
- Boobier, S.; Hose, D.R.J.; Blacker, A.J.; Nguyen, B.N. Machine Learning with Physicochemical Relationships: Solubility Prediction in Organic Solvents and Water. Nat. Commun. 2020 , 11 , 5753. [ Google Scholar ] [ CrossRef ]
- Wang, K.; Xu, H.; Yang, C.; Qiu, T. Machine Learning-Based Ionic Liquids Design and Process Simulation for CO2 Separation from Flue Gas. Green Energy Environ. 2021 , 6 , 432–443. [ Google Scholar ] [ CrossRef ]
- Saldana, D.A.; Starck, L.; Mougin, P.; Rousseau, B.; Ferrando, N.; Creton, B. Prediction of Density and Viscosity of Biofuel Compounds Using Machine Learning Methods. Energy Fuels 2012 , 26 , 2416–2426. [ Google Scholar ] [ CrossRef ]
- Wu, H.; Lorenson, A.; Anderson, B.; Witteman, L.; Wu, H.; Meredig, B.; Morgan, D. Robust FCC Solute Diffusion Predictions from Ab-Initio Machine Learning Methods. Comput. Mater. Sci. 2017 , 134 , 160–165. [ Google Scholar ] [ CrossRef ] [ Green Version ]
- Amsallem, D.; Farhat, C. Interpolation Method for Adapting Reduced-Order Models and Application to Aeroelasticity. AIAA J. 2008 , 46 , 1803–1813. [ Google Scholar ] [ CrossRef ] [ Green Version ]
- Amsallem, D.; Deolalikar, S.; Gurrola, F.; Farhat, C. Model Predictive Control under Coupled Fluid-Structure Constraints Using a Database of Reduced-Order Models on a Tablet. In Proceedings of the 21st AIAA Computational Fluid Dynamics Conference, Fluid Dynamics and Co-located Conferences, American Institute of Aeronautics and Astronautics, San Diego, CA, USA, 24–27 June 2013. [ Google Scholar ]
- Ooi, C.; Le, Q.T.; Dao, M.H.; Nguyen, V.B.; Nguyen, H.H.; Ba, T. Modeling Transient Fluid Simulations with Proper Orthogonal Decomposition and Machine Learning. Int. J. Numer. Methods Fluids 2021 , 93 , 396–410. [ Google Scholar ] [ CrossRef ]
- Kochkov, D.; Smith, J.A.; Alieva, A.; Wang, Q.; Brenner, M.P.; Hoyer, S. Machine Learning–Accelerated Computational Fluid Dynamics. Proc. Natl. Acad. Sci. USA 2021 , 118 , e2101784118. [ Google Scholar ] [ CrossRef ] [ PubMed ]
- Fukami, K.; Fukagata, K.; Taira, K. Assessment of Supervised Machine Learning Methods for Fluid Flows. Theor. Comput. Fluid Dyn. 2020 , 34 , 497–519. [ Google Scholar ] [ CrossRef ] [ Green Version ]
- Shukla, K.; Jagtap, A.D.; Karniadakis, G.E. Parallel Physics-Informed Neural Networks via Domain Decomposition. J. Comput. Phys. 2021 , 447 , 110683. [ Google Scholar ] [ CrossRef ]
- Zhu, Q.; Liu, Z.; Yan, J. Machine Learning for Metal Additive Manufacturing: Predicting Temperature and Melt Pool Fluid Dynamics Using Physics-Informed Neural Networks. Comput. Mech. 2021 , 67 , 619–635. [ Google Scholar ] [ CrossRef ]
- Rudy, S.H.; Brunton, S.L.; Proctor, J.L.; Kutz, J.N. Data-Driven Discovery of Partial Differential Equations. Sci. Adv. 2017 , 3 , e1602614. [ Google Scholar ] [ CrossRef ] [ PubMed ] [ Green Version ]
- Raissi, M.; Yazdani, A.; Karniadakis, G.E. Hidden Fluid Mechanics: Learning Velocity and Pressure Fields from Flow Visualizations. Science 2020 , 367 , 1026–1030. [ Google Scholar ] [ CrossRef ]
- Wan, Z.Y.; Sapsis, T.P. Machine Learning the Kinematics of Spherical Particles in Fluid Flows. J. Fluid Mech. 2018 , 857 , R2. [ Google Scholar ] [ CrossRef ] [ Green Version ]
- Seong, Y.; Park, C.; Choi, J.; Jang, I. Surrogate Model with a Deep Neural Network to Evaluate Gas–Liquid Flow in a Horizontal Pipe. Energies 2020 , 13 , 968. [ Google Scholar ] [ CrossRef ] [ Green Version ]
- Rastogi, A.; Fan, Y. Machine Learning Augmented Two-Fluid Model for Segregated Flow. Fluids 2022 , 7 , 12. [ Google Scholar ] [ CrossRef ]
- Hanna, B.N.; Dinh, N.T.; Youngblood, R.W.; Bolotnov, I.A. Machine-Learning Based Error Prediction Approach for Coarse-Grid Computational Fluid Dynamics (CG-CFD). Prog. Nucl. Energy 2020 , 118 , 103140. [ Google Scholar ] [ CrossRef ]
- Amini, S.; Mohaghegh, S. Application of Machine Learning and Artificial Intelligence in Proxy Modeling for Fluid Flow in Porous Media. Fluids 2019 , 4 , 126. [ Google Scholar ] [ CrossRef ] [ Green Version ]
- Tian, J.; Qi, C.; Sun, Y.; Yaseen, Z.M.; Pham, B.T. Permeability Prediction of Porous Media Using a Combination of Computational Fluid Dynamics and Hybrid Machine Learning Methods. Eng. Comput. 2021 , 37 , 3455–3471. [ Google Scholar ] [ CrossRef ]
- Kutz, J.N. Deep Learning in Fluid Dynamics. J. Fluid Mech. 2017 , 814 , 1–4. [ Google Scholar ] [ CrossRef ] [ Green Version ]
- Li, B.; Yang, Z.; Zhang, X.; He, G.; Deng, B.-Q.; Shen, L. Using Machine Learning to Detect the Turbulent Region in Flow Past a Circular Cylinder. J. Fluid Mech. 2020 , 905 , A10. [ Google Scholar ] [ CrossRef ]
- Pathak, J.; Mustafa, M.; Kashinath, K.; Motheau, E.; Kurth, T.; Day, M. Using Machine Learning to Augment Coarse-Grid Computational Fluid Dynamics Simulations. arXiv 2020 , arXiv:2010.00072. [ Google Scholar ]
- Dubois, P.; Gomez, T.; Planckaert, L.; Perret, L. Machine Learning for Fluid Flow Reconstruction from Limited Measurements. J. Comput. Phys. 2022 , 448 , 110733. [ Google Scholar ] [ CrossRef ]
- Ghasemi, F.; Mehridehnavi, A.; Pérez-Garrido, A.; Pérez-Sánchez, H. Neural Network and Deep-Learning Algorithms Used in QSAR Studies: Merits and Drawbacks. Drug Discov. Today 2018 , 23 , 1784–1790. [ Google Scholar ] [ CrossRef ] [ PubMed ]
- Vinuesa, R.; Brunton, S.L. The Potential of Machine Learning to Enhance Computational Fluid Dynamics. arXiv 2021 , arXiv:2110.02085. [ Google Scholar ]
- Zhang, J.; Lei, Y.-K.; Zhang, Z.; Chang, J.; Li, M.; Han, X.; Yang, L.; Yang, Y.I.; Gao, Y.Q. A Perspective on Deep Learning for Molecular Modeling and Simulations. J. Phys. Chem. A 2020 , 124 , 6745–6763. [ Google Scholar ] [ CrossRef ] [ PubMed ]
- Hoerl, A.E.; Kannard, R.W.; Baldwin, K.F. Ridge Regression: Some Simulations. Commun. Stat. 1975 , 4 , 105–123. [ Google Scholar ] [ CrossRef ]
- Hoerl, A.E.; Kennard, R.W. Ridge Regression: Biased Estimation for Nonorthogonal Problems. Technometrics 1970 , 12 , 55–67. [ Google Scholar ] [ CrossRef ]
- Gareth, J.D.W.; Hastie, T.; Tibshirani, R. An Introduction to Statistical Learning: With Applications in R ; Springer: New York, NY, USA, 2013. [ Google Scholar ]
- Bibas, K.; Fogel, Y.; Feder, M. A New Look at an Old Problem: A Universal Learning Approach to Linear Regression. In Proceedings of the 2019 IEEE International Symposium on Information Theory (ISIT), Paris, France, 7–12 July 2019; pp. 2304–2308. [ Google Scholar ]
- Zhao, J.; Zhao, C.; Zhang, F.; Wu, G.; Wang, H. Water Quality Prediction in the Waste Water Treatment Process Based on Ridge Regression Echo State Network. IOP Conf. Ser. Mater. Sci. Eng. 2018 , 435 , 012025. [ Google Scholar ] [ CrossRef ] [ Green Version ]
- Wang, T.; Zhang, K.; Thé, J.; Yu, H. Accurate Prediction of Band Gap of Materials Using Stacking Machine Learning Model. Comput. Mater. Sci. 2022 , 201 , 110899. [ Google Scholar ] [ CrossRef ]
- Mansour, R.F. Evolutionary Computing Enriched Ridge Regression Model for Craniofacial Reconstruction. Multimed. Tools Appl. 2020 , 79 , 22065–22082. [ Google Scholar ] [ CrossRef ]
- Tibshirani, R. Regression Shrinkage and Selection via the Lasso. J. R. Stat. Soc. Ser. B (Methodol.) 1996 , 58 , 267–288. [ Google Scholar ] [ CrossRef ]
- Hastie, T.; Tibshirani, R.; Friedman, J. The Elements of Statistical Learning ; Springer Series in Statistics; Springer: New York, NY, USA, 2001. [ Google Scholar ]
- Moon, C.; Mitchell, S.A.; Heath, J.E.; Andrew, M. Statistical Inference Over Persistent Homology Predicts Fluid Flow in Porous Media. Water Resour. Res. 2019 , 55 , 9592–9603. [ Google Scholar ] [ CrossRef ]
- Behesht Abad, A.R.; Tehrani, P.S.; Naveshki, M.; Ghorbani, H.; Mohamadian, N.; Davoodi, S.; Aghdam, S.K.; Moghadasi, J.; Saberi, H. Predicting Oil Flow Rate through Orifice Plate with Robust Machine Learning Algorithms. Flow Meas. Instrum. 2021 , 81 , 102047. [ Google Scholar ] [ CrossRef ]
- Callaham, J.L.; Maeda, K.; Brunton, S.L. Robust Flow Reconstruction from Limited Measurements via Sparse Representation. Phys. Rev. Fluids 2019 , 4 , 103907. [ Google Scholar ] [ CrossRef ] [ Green Version ]
- Li, X.; Zhou, J.; Li, H.; Zhang, S.; Chen, Y. Computational Intelligent Methods for Predicting Complex Ithologies and Multiphase Fluids. Pet. Explor. Dev. 2012 , 39 , 261–267. [ Google Scholar ] [ CrossRef ]
- Chen, H.; Zhang, C.; Jia, N.; Duncan, I.; Yang, S.; Yang, Y. A Machine Learning Model for Predicting the Minimum Miscibility Pressure of CO 2 and Crude Oil System Based on a Support Vector Machine Algorithm Approach. Fuel 2021 , 290 , 120048. [ Google Scholar ] [ CrossRef ]
- Samadianfard, S.; Jarhan, S.; Salwana, E.; Mosavi, A.; Shamshirband, S.; Akib, S. Support Vector Regression Integrated with Fruit Fly Optimization Algorithm for River Flow Forecasting in Lake Urmia Basin. Water 2019 , 11 , 1934. [ Google Scholar ] [ CrossRef ] [ Green Version ]
- Morita, Y.; Rezaeiravesh, S.; Tabatabaei, N.; Vinuesa, R.; Fukagata, K.; Schlatter, P. Applying Bayesian Optimization with Gaussian Process Regression to Computational Fluid Dynamics Problems. J. Comput. Phys. 2022 , 449 , 110788. [ Google Scholar ] [ CrossRef ]
- Deringer, V.L.; Bartók, A.P.; Bernstein, N.; Wilkins, D.M.; Ceriotti, M.; Csányi, G. Gaussian Process Regression for Materials and Molecules. Chem. Rev. 2021 , 121 , 10073–10141. [ Google Scholar ] [ CrossRef ] [ PubMed ]
- Lee, T.R.; Wood, W.T.; Phrampus, B.J. A Machine Learning (KNN) Approach to Predicting Global Seafloor Total Organic Carbon. Glob. Biogeochem. Cycles 2019 , 33 , 37–46. [ Google Scholar ] [ CrossRef ]
- Rahman, J.; Ahmed, K.S.; Khan, N.I.; Islam, K.; Mangalathu, S. Data-Driven Shear Strength Prediction of Steel Fiber Reinforced Concrete Beams Using Machine Learning Approach. Eng. Struct. 2021 , 233 , 111743. [ Google Scholar ] [ CrossRef ]
- Adithiyaa, T.; Chandramohan, D.; Sathish, T. Optimal Prediction of Process Parameters by GWO-KNN in Stirring-Squeeze Casting of AA2219 Reinforced Metal Matrix Composites. Mater. Today Proc. 2020 , 21 , 1000–1007. [ Google Scholar ] [ CrossRef ]
- Khosravi, P.; Vergari, A.; Choi, Y.; Liang, Y.; Van den Broeck, G. Handling Missing Data in Decision Trees: A Probabilistic Approach. In Proceedings of the The Art of Learning with Missing Values Workshop at ICML (Artemiss), Online, 17 July 2020. [ Google Scholar ]
- Winkler, D.; Haltmeier, M.; Kleidorfer, M.; Rauch, W.; Tscheikner-Gratl, F. Pipe Failure Modelling for Water Distribution Networks Using Boosted Decision Trees. Struct. Infrastruct. Eng. 2018 , 14 , 1402–1411. [ Google Scholar ] [ CrossRef ] [ Green Version ]
- Schmidt, J.; Marques, M.R.G.; Botti, S.; Marques, M.A.L. Recent Advances and Applications of Machine Learning in Solid-State Materials Science. npj Comput. Mater. 2019 , 5 , 83. [ Google Scholar ] [ CrossRef ]
- Sofos, F.; Karakasidis, T.E. Nanoscale Slip Length Prediction with Machine Learning Tools. Sci. Rep. 2021 , 11 , 12520. [ Google Scholar ] [ CrossRef ]
- Wei, Z.; Yu, J.; Lu, Y.; Han, J.; Wang, C.; Liu, X. Prediction of Diffusion Coefficients in Fcc, Bcc and Hcp Phases Remained Stable or Metastable by the Machine-Learning Methods. Mater. Des. 2021 , 198 , 109287. [ Google Scholar ] [ CrossRef ]
- Bentéjac, C.; Csörgő, A.; Martínez-Muñoz, G. A Comparative Analysis of Gradient Boosting Algorithms. Artif. Intell. Rev. 2021 , 54 , 1937–1967. [ Google Scholar ] [ CrossRef ]
- Qian, N.; Wang, X.; Fu, Y.; Zhao, Z.; Xu, J.; Chen, J. Predicting Heat Transfer of Oscillating Heat Pipes for Machining Processes Based on Extreme Gradient Boosting Algorithm. Appl. Therm. Eng. 2020 , 164 , 114521. [ Google Scholar ] [ CrossRef ]
- Bikmukhametov, T.; Jäschke, J. Oil Production Monitoring Using Gradient Boosting Machine Learning Algorithm. IFAC-PapersOnLine 2019 , 52 , 514–519. [ Google Scholar ] [ CrossRef ]
- Ma, B.; Meng, F.; Yan, G.; Yan, H.; Chai, B.; Song, F. Diagnostic Classification of Cancers Using Extreme Gradient Boosting Algorithm and Multi-Omics Data. Comput. Biol. Med. 2020 , 121 , 103761. [ Google Scholar ] [ CrossRef ]
- Kim, S.; Lu, P.Y.; Mukherjee, S.; Gilbert, M.; Jing, L.; Ceperic, V.; Soljacic, M. Integration of Neural Network-Based Symbolic Regression in Deep Learning for Scientific Discovery. IEEE Trans. Neural Netw. Learn. Syst. 2020 , 32 , 4166–4177. [ Google Scholar ] [ CrossRef ]
- Udrescu, S.-M.; Tegmark, M. AI Feynman: A Physics-Inspired Method for Symbolic Regression. Sci. Adv. 2020 , 6 , eaay2631. [ Google Scholar ] [ CrossRef ] [ Green Version ]
- Loftis, C.; Yuan, K.; Zhao, Y.; Hu, M.; Hu, J. Lattice Thermal Conductivity Prediction Using Symbolic Regression and Machine Learning. J. Phys. Chem. A 2021 , 125 , 435–450. [ Google Scholar ] [ CrossRef ]
- Wadekar, D.; Villaescusa-Navarro, F.; Ho, S.; Perreault-Levasseur, L. Modeling Assembly Bias with Machine Learning and Symbolic Regression. arXiv 2020 , arXiv:2012.00111. [ Google Scholar ]
- Reinbold, P.A.K.; Kageorge, L.M.; Schatz, M.F.; Grigoriev, R.O. Robust Learning from Noisy, Incomplete, High-Dimensional Experimental Data via Physically Constrained Symbolic Regression. Nat. Commun. 2021 , 12 , 3219. [ Google Scholar ] [ CrossRef ] [ PubMed ]
- Sofos, F.; Karakasidis, T.E.; Liakopoulos, A. Surface Wettability Effects on Flow in Rough Wall Nanochannels. Microfluid. Nanofluid. 2012 , 12 , 25–31. [ Google Scholar ] [ CrossRef ]
- Meier, K.; Laesecke, A.; Kabelac, S. Transport Coefficients of the Lennard-Jones Model Fluid. II Self-Diffusion. J. Chem. Phys. 2004 , 121 , 9526–9535. [ Google Scholar ] [ CrossRef ] [ PubMed ]
- Hess, B. Determining the Shear Viscosity of Model Liquids from Molecular Dynamics Simulations. J. Chem. Phys. 2002 , 116 , 209–217. [ Google Scholar ] [ CrossRef ]
- Bugel, M.; Galliero, G. Thermal Conductivity of the Lennard-Jones Fluid: An Empirical Correlation. Chem. Phys. 2008 , 352 , 249–257. [ Google Scholar ] [ CrossRef ] [ Green Version ]
- Sofos, F.; Karakasidis, T.E. Machine Learning Techniques for Fluid Flows at the Nanoscale. Fluids 2021 , 6 , 96. [ Google Scholar ] [ CrossRef ]
- Zhu, Y.; Lu, X.; Zhou, J.; Wang, Y.; Shi, J. Prediction of Diffusion Coefficients for Gas, Liquid and Supercritical Fluid: Application to Pure Real Fluids and Infinite Dilute Binary Solutions Based on the Simulation of Lennard–Jones Fluid. Fluid Phase Equilib. 2002 , 194 , 1141–1159. [ Google Scholar ] [ CrossRef ]
- Zolotukhin, A.B.; Gayubov, A.T. Machine Learning in Reservoir Permeability Prediction and Modelling of Fluid Flow in Porous Media. IOP Conf. Ser. Mater. Sci. Eng. 2019 , 700 , 012023. [ Google Scholar ] [ CrossRef ]
- Usman, A.; Rafiq, M.; Saeed, M.; Nauman, A.; Almqvist, A.; Liwicki, M. Machine Learning Computational Fluid Dynamics. In Proceedings of the 2021 Swedish Artificial Intelligence Society Workshop (SAIS), Lulea, Sweden, 14–15 June 2021; pp. 1–4. [ Google Scholar ]
- Korteby, Y.; Kristó, K.; Sovány, T.; Regdon, G. Use of Machine Learning Tool to Elucidate and Characterize the Growth Mechanism of an In-Situ Fluid Bed Melt Granulation. Powder Technol. 2018 , 331 , 286–295. [ Google Scholar ] [ CrossRef ] [ Green Version ]
- Ling, J.; Templeton, J. Evaluation of Machine Learning Algorithms for Prediction of Regions of High Reynolds Averaged Navier Stokes Uncertainty. Phys. Fluids 2015 , 27 , 085103. [ Google Scholar ] [ CrossRef ]
- Gul, S. Machine Learning Applications in Drilling Fluid Engineering: A Review. In Proceedings of the ASME 2021 40th International Conference on Ocean, Offshore and Arctic Engineering—OMAE2021, Online, 21 June 2021; Volume 10: Petroleum Technology. [ Google Scholar ]
- Zhu, L.-T.; Tang, J.-X.; Luo, Z.-H. Machine Learning to Assist Filtered Two-Fluid Model Development for Dense Gas–Particle Flows. AlChE J. 2020 , 66 , e16973. [ Google Scholar ] [ CrossRef ]
Click here to enlarge figure
D | D | |||||
---|---|---|---|---|---|---|
R | MAE | MSE | R | MAE | MSE | |
MLR | 0.371 | 1.936 | 9.767 | 0.882 | 0.418 | 0.593 |
Lasso | 0.299 | 1.990 | 10.877 | 0.409 | 1.135 | 2.963 |
Ridge | 0.371 | 1.934 | 9.768 | 0.878 | 0.433 | 0.610 |
SVR-LIN | 0.204 | 1.465 | 12.358 | 0.864 | 0.472 | 0.682 |
SVR-RBF | 0.410 | 1.037 | 9.155 | 0.587 | 0.874 | 2.070 |
SVR-POLY | 0.450 | 1.060 | 8.530 | |||
GP | 0.369 | 1.903 | 9.801 | 0.881 | 0.422 | 0.597 |
k-NN | 0.716 | 0.587 | 4.405 | 0.916 | 0.260 | 0.421 |
DT | 0.971 | 0.284 | 0.446 | 0.564 | 0.766 | 2.185 |
RF | 0.708 | 0.589 | 1.462 | |||
GB | 0.962 | 0.385 | 0.595 | 0.913 | 0.331 | 0.435 |
MLP | 0.878 | 0.395 | 1.901 | 0.943 | 0.284 | 0.287 |
R | MAE | MSE | R | MAE | MSE | |
---|---|---|---|---|---|---|
MLR | 0.697 | 0.661 | 0.578 | 0.111 | 0.236 | 0.075 |
Lasso | 0.327 | 0.849 | 1.285 | −0.368 | 0.283 | 0.116 |
Ridge | 0.698 | 0.659 | 0.577 | 0.126 | 0.233 | 0.074 |
SVR-LIN | 0.626 | 0.505 | 0.714 | 0.013 | 0.250 | 0.083 |
SVR-RBF | 0.958 | 0.132 | 0.080 | 0.067 | 0.164 | 0.079 |
SVR-POLY | 0.983 | 0.115 | 0.032 | −0.726 | 0.278 | 0.146 |
GP | 0.698 | 0.660 | 0.577 | 0.114 | 0.236 | 0.075 |
k-NN | −0.022 | 0.166 | 0.086 | |||
DT | 0.973 | 0.080 | 0.052 | 0.730 | 0.072 | 0.023 |
RF | 0.978 | 0.067 | 0.042 | 0.831 | 0.079 | 0.014 |
GB | 0.984 | 0.109 | 0.031 | |||
MLP | 0.996 | 0.051 | 0.008 | 0.296 | 0.159 | 0.059 |
R | MAE | MSE | R | MAE | MSE | |
---|---|---|---|---|---|---|
MLR | 0.489 | 1.617 | 3.466 | 0.327 | 0.150 | 0.032 |
Lasso | −0.000 | 2.127 | 6.787 | −0.250 | 0.220 | 0.059 |
Ridge | 0.483 | 1.632 | 3.509 | 0.337 | 0.149 | 0.031 |
SVR-LIN | 0.348 | 1.629 | 4.424 | 0.249 | 0.157 | 0.035 |
SVR-RBF | 0.640 | 0.700 | 2.442 | 0.808 | 0.088 | 0.009 |
SVR-POLY | 0.735 | 0.639 | 1.798 | 0.621 | 0.114 | 0.018 |
GP | 0.450 | 1.700 | 3.734 | 0.328 | 0.150 | 0.032 |
k-NN | 0.649 | 0.517 | 2.379 | 0.802 | 0.051 | 0.009 |
DT | 0.960 | 0.332 | 0.271 | |||
RF | 0.980 | 0.135 | 0.960 | 0.024 | 0.002 | |
GB | 0.949 | 0.404 | 0.347 | 0.994 | 0.015 | 0.000 |
MLP | 0.208 | 0.741 | 0.090 | 0.012 |
MDPI stays neutral with regard to jurisdictional claims in published maps and institutional affiliations. |
Share and Cite
Sofos, F.; Stavrogiannis, C.; Exarchou-Kouveli, K.K.; Akabua, D.; Charilas, G.; Karakasidis, T.E. Current Trends in Fluid Research in the Era of Artificial Intelligence: A Review. Fluids 2022 , 7 , 116. https://doi.org/10.3390/fluids7030116
Sofos F, Stavrogiannis C, Exarchou-Kouveli KK, Akabua D, Charilas G, Karakasidis TE. Current Trends in Fluid Research in the Era of Artificial Intelligence: A Review. Fluids . 2022; 7(3):116. https://doi.org/10.3390/fluids7030116
Sofos, Filippos, Christos Stavrogiannis, Kalliopi K. Exarchou-Kouveli, Daniel Akabua, George Charilas, and Theodoros E. Karakasidis. 2022. "Current Trends in Fluid Research in the Era of Artificial Intelligence: A Review" Fluids 7, no. 3: 116. https://doi.org/10.3390/fluids7030116
Article Metrics
Article access statistics, further information, mdpi initiatives, follow mdpi.
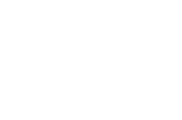
Subscribe to receive issue release notifications and newsletters from MDPI journals
Physical Review Fluids
- Collections
- Editorial Team
Data-driven low-dimensional model of a sedimenting flexible fiber
Andrew j. fox and michael d. graham, phys. rev. fluids 9 , 084101 – published 16 august 2024.
- No Citing Articles
- Supplemental Material
- INTRODUCTION
- FORMULATION
- RESULTS AND DISCUSSION
- CONCLUSIONS
- ACKNOWLEDGMENTS
The dynamics of flexible filaments entrained in flow, important for understanding many biological and industrial processes, are computationally expensive to model with full physics simulations. In this paper, we describe a data-driven technique to create high-fidelity low-dimensional models of flexible fiber dynamics using machine learning; the technique is applied to sedimentation in a quiescent, viscous Newtonian fluid, using results from detailed simulations as the dataset. The approach combines an autoencoder neural network architecture to learn a low-dimensional latent representation of the filament shape, with a neural ordinary differential equation that learns the evolution of the particle in the latent state. The model was designed to model filaments of varying flexibility, characterized by an elastogravitational number B , and was trained on a dataset containing the evolution of fibers beginning at set angles of inclination. For the range of B considered here (100–10 000), the filament shape dynamics can be represented with high accuracy with only four degrees of freedom, in contrast with the 93 present in the original bead-spring model used to generate the dynamic trajectories. We predict the evolution of fibers set at arbitrary angles and demonstrate that our data-driven model can accurately forecast the evolution of a fiber at both trained and untrained elastogravitational numbers.
- Received 16 May 2024
- Accepted 11 July 2024
DOI: https://doi.org/10.1103/PhysRevFluids.9.084101
©2024 American Physical Society
Physics Subject Headings (PhySH)
- Physical Systems
Authors & Affiliations
- Department of Chemical and Biological Engineering, University of Wisconsin-Madison , Madison, Wisconsin 53706, USA
- * Contact author: [email protected]
Article Text (Subscription Required)
Supplemental material (subscription required), references (subscription required).
Vol. 9, Iss. 8 — August 2024
Access Options
- Buy Article »
- Log in with individual APS Journal Account »
- Log in with a username/password provided by your institution »
- Get access through a U.S. public or high school library »
Article part of CHORUS
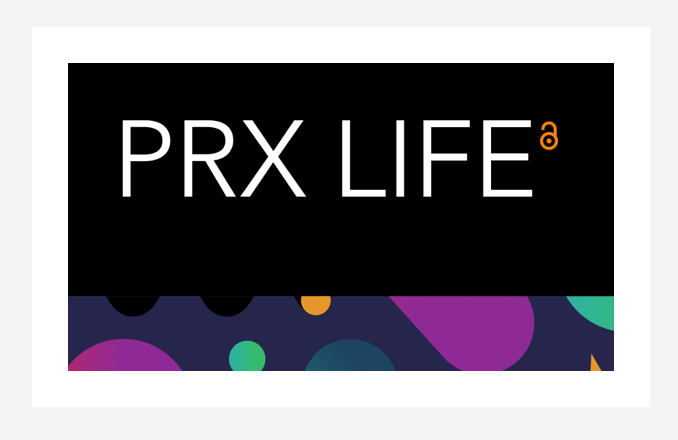
Authorization Required
Other options.
- Buy Article »
- Find an Institution with the Article »
Download & Share
A flexible filament of length L settling under an external force F g in a quiescent Newtonian fluid. The filament begins at an arbitrary initial inclination θ 0 relative to the x axis and evolves until it reaches a terminal shape. The fiber is modeled as a series of N beads of radius a connected by springs, with the center bead, which has position c ( t ) , shown in red.
The evolution of the shape of a filament settling in a quiescent Newtonian fluid from an initial orientation to a common terminal shape at B = 1000 for all initial angles of orientation within the training dataset.
The trajectories c ( t ) of the center bead of a filament settling in a quiescent Newtonian fluid at B = 1000 for all initial angles of inclination within the training dataset. The initial positions are denoted by symbol ▾ , and the terminal positions are denoted by the symbol × .
The evolution of the shape of a filament settling in a quiescent Newtonian fluid from a common initial angle of orientation of π / 4 to a terminal shape for all B within the training dataset.
Block diagram for data-driven model combining the autoencoder and temporal-evolution scheme. The temporal-evolution neural network, expanded in red, can be separated into two distinct neural networks forecasting the evolution of latent representation of the shape h ( t + τ ) and the shape-dependent change in position c ; in practice, these can be forecasted by a single neural network.
(a) Loss over the testing data for each autoencoder architecture as a function of latent dimension. Block diagrams for the autoencoder neural network architectures: (b) No B , (c) Encoder B , (d) Decoder B , and (e) Double B .
Evolution of the shape of a filament settling in quiescent Newtonian fluid given an initial orientation from the best and worst forecasts (red) and the true evolution (black) at a given B . Here, B is within the training dataset, and the initial angles of inclination are not.
Evolution of the shape of a filament settling in quiescent Newtonian fluid given an initial orientation from the best and worst forecasts (red) and the true evolution (black) at a given B . Here, neither B nor the initial angles of inclination are within the training dataset.
Trajectory of the center bead c ( t ) a filament settling in quiescent Newtonian fluid given an initial orientation from the best (solid lines) and worst (dashed lines) forecasts and the true evolution at a given B . The initial positions are denoted by symbol ▾ , and the terminal positions are denoted by the symbol × , with the true and predicted positions in black and red, respectively. In (a), B is within the training dataset but, at initial angles of inclination, not within the training data; in (b), neither B nor the initial angles of inclination are within the training data.
Ensemble-average error vs time for forecasts of the evolution of a filament settling in quiescent Newtonian fluid at each B (gray) and averaged over all B (red). In (a), B is within the training dataset but, at initial angles of inclination, not within the training data; in (b), neither B nor the initial angles of inclination are within the training data.
Sign up to receive regular email alerts from Physical Review Fluids
- Forgot your username/password?
- Create an account
Article Lookup
Paste a citation or doi, enter a citation.

Published 6 issues per year
ISSN Print: 2152-5102
ISSN Online: 2152-5110
Aims and Scope
Most downloaded articles, forthcoming articles, archive articles.
Accessibility Links
- Skip to content
- Skip to search IOPscience
- Skip to Journals list
- Accessibility help
- Accessibility Help
Click here to close this panel.
The Japan Society of Fluid Mechanics (JSFM) originated from a voluntary party of researchers working on fluid mechanics in 1968. The objectives of the society were to discuss about scientific and engineering problems relevant to fluid motion among researchers working in Physics, Engineering and the interdisciplinary fields and to assist in their research activities.
"Fluid Dynamics Research" whose first volume was published in 1986 is the official journal of the JSFM. "Fluid Dynamics Research" is a well-established international journal of Fluid Mechanics, published six times per year by IOPP (Institute of Physics Publishing) on behalf of the JSFM since 2009.
- Author guidelines
What we look for in your article
How to prepare your article, mathematics, supplementary data, length of submissions, what files to submit, naming your files, article text files, figure files.
- Supplementary data files
- How to submit
- Send us your signed Assignment of Copyright form
How your article is assessed
- Revising your article
Comments and Replies
What we do with your article after it is accepted, copyright, reproduction and permissions, transfer of copyright.
- Copying articles
Reproducing published material
We consider for publication in Fluid Dynamics Research articles which:
- are original, novel and add significantly to research already published
- are of interest to the community
- have sound motivation and purpose
- have not been published previously
- are not under consideration for publication in any other journal or book available through a library or by purchase
- present new but trivial and obvious results
- make unsubstantiated conclusions
- bury new results beneath too much scene-setting and recapping of findings already published
- present incremental research
Articles based on theses for higher degrees may be submitted, although authors should take care that such articles are prepared in the format of a research paper, which is more concise than is appropriate for a thesis.
Articles presented at conferences may also be submitted, provided these articles do not appear in substantially the same form in published conference proceedings. Again, authors should take care to ensure the format of a research paper is used. The article length should also be appropriate to the content. In case of doubt, please enquire with the journal team.
Reports that are not available to the general public are not regarded as prior publications. Authors of articles are not restricted to being members of any particular institute, society or association. Please check via the journal homepage that your article is of an accepted article type and within the scope of the journal before submission.
We treat all submitted articles as confidential until they are published and they will only be shared with those referees, Board members and Editors who are directly involved in the peer review of the article. (An exception to this would be if it is felt necessary to share the article with additional external parties in order to investigate a possible breach of the ethical policy.)
Please read these guidelines carefully and familiarize yourself with the style and editorial policies of Fluid Dynamics Research by examining the online version. It is important to check that your research fits well into the scope of Fluid Dynamics Research before you submit it. You are also advised to read the IOP Publishing ethical policy , to which the journal subscribes. If you have any queries, please contact us.
- a title page with title of article, name(s) of author(s) and address(es) of establishment(s)
- where the work was carried out
- an abstract
- a list of references
The following sections give a brief overview of the main elements or structure of an article. Read them first.
Title of article
This should be concise, informative and meaningful to the whole readership of the journal. Please avoid the use of long systemic names and non-standard abbreviations, acronyms or symbols .
Authors and addresses
Author lists should be finalised prior to submission (for more information on this please consult our Ethical Policy for Journals ). For articles with several authors, please list the names of all the authors first, followed by the full postal addresses, using superscript numeric identifiers to link an author with an address, where necessary (see LaTeX guidelines).
If an author's present address is different from the address at which the work was carried out, this should be given as a footnote to the page. The corresponding author is highlighted by a footnote to the page.
Please note that there is also an option to add authors' ORCID numbers when you complete the online submission form, and we encourage you to do so.
Your abstract should give readers concise information about the content of your article. It should be informative and not only indicate the general scope of the article but also state the main results obtained and conclusions drawn. As the abstract is not part of the text it should be complete in itself; no table numbers, figure numbers, references or displayed mathematical expressions should be included. It should be suitable for direct inclusion in abstracting services and should not normally exceed 200 words.
When readers are searching for information online, an abstract of an article is likely to be the first thing they see. Consequently your abstract needs to be concise but convey as much information as possible about the content of your article.
Fluid Dynamics Research publishes Keywords for each article. Authors should choose 3 to 5 keywords for their submission. These will be entered into the online submission form.
Your abstract should give readers concise information about the content of your article. It should be informative , accessible and must indicate the general scope of the article and also state the main results obtained and conclusions drawn. As the abstract is not part of the text it should be complete in itself; no table numbers, figure numbers, references or displayed mathematical expressions should be included. It should be suitable for direct inclusion in abstracting services and should not normally exceed 300 words.
Research papers and review articles can be divided into numbered sections and subsections. A typical article structure is shown below:
Article structure
Introduction.
This should be concise and describe the nature of the problem under investigation and its background. It should also set your work in the context of previous research, citing relevant references. Introductions should expand on the highly specialised terms and abbreviations used in the article to make it accessible for readers.
This section should provide sufficient details of the experiment, simulation, statistical test or analysis carried out to generate the results so that the method can be repeated by another researcher.
The results section should detail the main findings and outcomes of your study. You should use tables only to improve conciseness or where the information cannot be given satisfactorily in other ways such as histograms or graphs.
Tables should be numbered serially and referred to in the text by number (table 1, etc.). Each table should have an explanatory caption which should be as concise as possible.
If your article consists of a very large amount of tabular material such as long lists of crystallographic results, computer programs and spectrographic results we would not normally publish these in full. Instead these may be published online as supplementary data files.
This should discuss the significance of the results and compare them with previous work using relevant references.
This section should be used to highlight the novelty and significance of the work, and any plans for future relevant work.
In terms of general style, writing concisely helps the reader, but clarity is most important. Short sentences and paragraphs make reading the article easier. You should aim for consistency within your article in matters such as hyphenation and spelling.
Do not make a list of nomenclature, instead all acronyms and abbreviations should be clearly explained when they first appear in the text. All units should be consistent throughout the article.
If English is not your first language, you should ask an English speaking colleague to read through your article or at least apply a UK English spellchecker to your article.
Detailed information on the presentation of mathematics, formulae and equations is provided in our LaTeX guidelines.
Acknowledgments
All authors and co-authors are required to disclose any potential conflict of interest when submitting their article (e.g. employment, consulting fees, research contracts, stock ownership, patent licenses, honoraria, advisory affiliations, etc). This information should be included in an acknowledgments section at the end of the manuscript (before the references section). All sources of financial support for the project must also be disclosed in the acknowledgments section.
The name of the funding agency and the grant number should be given, for example:
'This work was partially funded by the National Institutes of Health (NIH) through a National Cancer Institute grant R21CA141833.'
When completing the online submission form, we also ask you to select funders (from the FundRef Registry) and provide grant numbers in order to help you to meet your funder requirements. For more information about FundRef, please see: crossref.org/fundref .
It is vitally important to fully acknowledge all relevant work and we advise that you also consult our ethical policy for general guidance on compiling your reference list.
A complete reference should provide your reader with enough information to locate the article concerned and should consist of: author(s) name(s) and initials, titles of articles in journals, date published, title of journal or book, volume number, editors (if any) and, for books, town of publication and publisher (in parentheses), and finally the first and last page numbers or article number.
Where there are up to ten authors, all authors' names should be given in the reference list. Where there are more than ten authors, only the first name should appear followed by et al.
You should take particular care to ensure that the information is correct so that links to referenced articles can be made successfully. Material which is really a footnote to the text should not be included in the reference list, which should contain only references to bibliographic data.
Copies of cited publications not yet available publicly should be submitted for the benefit of the referees. Unpublished results and lectures should be cited for exceptional reasons only.
Before submitting your article, please ensure you have done a literature search to check for any relevant references you may have missed.
You should use the alphabetical referencing system (Harvard) described below.
For articles prepared in LaTeX, please use the tools provided in your LaTeX class file.
Alphabetical system (Harvard)
In the Harvard alphabetical system the name of the author appears in the text together with the year of publication, e.g. (Smith 2001) or Smith (2001) (as appropriate). Where there are only two authors both names should be given in the text (Smith and Jones 2001) or Smith and Jones (2001); however, if there are more than two authors only the first name should appear followed by et al, (Smith et al 2001) or Smith et al (2001). If you refer to different works by one author or group of authors in the same year they should be differentiated by including a, b, etc after the date (e.g. 2001a). If you refer to different pages of the same article, the page number may be given in the text, e.g. Smith (2001, p 39). The reference list at the end of your article should be in alphabetical order.
Carefully chosen and well-prepared figures, such as diagrams and photos, can greatly enhance your article. We encourage you to prepare figures that are clear, easy to read and of the best possible quality. Characters should appear as they would be set in the main body of the article. We will normally use figures as submitted; it is therefore your responsibility to ensure that they are legible and technically correct.
Note: If you are intending to use previously published figures, you must obtain written permission from the copyright holder before using them in your article.
Please use our permission request form to ensure that you include all relevant details when approaching copyright holders. For further information about permissions please see the copyright and permissions section . Detailed information on common graphic formats and their preparation with examples are provided in our graphics guidelines.
To get the best possible results in print and online, please consider the following points when preparing your figure files:
- Shading and fill patterns should be avoided wherever possible because diagrams containing them have to be printed as half-tones and undesirable interference patterns may be produced on printing.
- Readers of your online article will probably download and print it on a black and white printer which may make coloured lines difficult to distinguish. To avoid this problem, please consider identifying curves by methods other than colour, for example: by letters (upper case Roman), by the symbols used for the data points (e.g.*, ¦ or by the type of line (e.g. --, full curve; - - - , broken curve; - · - · -, chain curve).
- When producing figures using colours, light colours such as yellow, light green, light blue, light grey, etc should be avoided because they generally reproduce poorly during the black and white printing process.
- Wherever possible electronic figures should be tightly cropped to minimize superfluous white space surrounding them. This reduces file sizes and helps the alignment of figures on the printed page.
Detailed information on common graphic formats and their preparation with examples are provided in our graphics guidelines .
Colour figures
The use of colour in figures can enhance the effective presentation of results, and there are no restrictions on the use of colour in the online version of your article. However, because conventional full-colour printing remains an expensive process, we must ask you (or your institution) to pay the additional costs incurred (i.e. the costs over and above the cost of normal black-on-white reproduction) if you also require colour in the printed version of your article. An estimate of the charges for your article can be obtained from the Publishing Administrator by e-mailing [email protected] . There is no charge for colour in the online version of an article.
If you need further information or guidance, please contact the journal .
Figure captions
Your figures should be numbered in the order in which they are referred to in the text. If there is more than one part to a figure (e.g. figure 1(a), figure 1(b) etc), the parts should be identified by a lower-case letter in parentheses close to or within the area of the figure. Captions should be included in the text and not in the graphics files.
Micrographs should include a scale bar of appropriate size, e.g. 1 mm.
FDR allows authors to submit supplementary data attachments to enhance the online versions of published research articles, where relevant to the paper.
All journals encourage authors to submit supplementary data attachments on submission to enhance the online versions of published research articles. Supplementary data typically include multimedia files such as video clips, sound files, animations and additional data such as computer code, large tables, additional figures or appendices. Many options are available, for example, you can use movies and animated GIFs to illustrate the evolution of iterative algorithms or include data sets with your article to allow readers to use the information to test their own work and truly explore the implications of your research. Supplementary data are not included in the PDF of the article or in any print version. The printed journal remains the archival version, and supplementary data items are supplements which enhance a reader's understanding of the article but are not essential to that understanding. Supplementary files are hosted for free with your article on our online journals page and are accessible to the whole readership.
Guidelines for submitting video files
Most standard file formats are suitable: animated GIF, AVI, MPG, etc. The recommended settings are
- frame size: 480 x 360 pixels
- frame rate: 15 frames/s
- data rate: 150 kB/s
- file size: 3 MB, unless more is required to display the science properly.
Regular papers should not exceed 30 journal pages in length and review papers should not exceed 40 journal pages in length.
The length of an article can be calculated by allowing 600 words per page. Diagrams and tables usually occupy the equivalent of 200-300 words each, and you should allow for this in your total.
· A PDF of the complete manuscript for review, containing the names and institutes of authors, and figures and tables embedded within the text. Authors are asked to consider the need for clarity and readability when selecting column type, line spacing, font size and layout when preparing the PDF, to assist the reviewers.
· Any suitable supplementary data (see previous supplementary data section for details about suitable files).
· Any permissions that you have already obtained at this stage.
How to submit a new article
Please submit all new articles via the 'Submit an article' link on the journal homepage . Please ensure that you enter all the required information about your article and all its authors before uploading your files. You are required to select some keywords for your article. Please note that, if your article is accepted for publication, we will display these keywords on the published article. Authors may propose preferred (and non-preferred) referees on submission. The suggested referees should have suitable subject expertise and not have any conflicts of interest (please see the Peer Review policy for further information on conflict of interest). These suggestions will be considered; however, the Editorial Board will make the final decision regarding referee selection.
If you are a new author, you will need to set up an author account before submitting your first article. Using the Author Centre, you will be able to track the progress of your article, respond to the referee reports, and submit your revised version.
When submitting a new article, we only require you to upload a single PDF file and any relevant supplementary data at this stage. The PDF should contain your complete manuscript, including any embedded figures and tables. You may upload your article from the arXiv directly by entering the arXiv e-print number. Please also submit any permissions that you have already obtained at this stage.
If you experience any problems submitting your article online, please contact the journal team for assistance.
How to prepare your revised article
It is common for our referees to request that authors make revisions to their articles. When you submit a revised version of your article in response to the referees' comments, you must accompany it with a detailed list of the changes made (ignoring typographical errors, but mentioning additional paragraphs, changes to figures, etc) suitable for transmission to the referee. Where changes have been made in response to the referees' remarks it is important to mention this and indicate where they can be found. You may also wish to send in a second copy of your article with the changes marked or underlined.
You should go through the referees' comments and for each comment mention whether you followed their suggestion or whether you disagree and wish to respond to the comment. If a referee has misunderstood a point, it is not necessarily their fault and may have been caused by ambiguity or lack of clarity in your article which needs to be corrected. Some authors copy out each of the referees' comments in turn and include their response immediately after. In other cases responses can be made referring back to the reports. Finally, please make sure that you send your revised article and not simply the original version again. This is a common mistake. Electronic revised articles should contain all text and graphics files needed to generate the revised version, and not just those files that have changed.
By observing these guidelines you will be assisting the referees, who give up their time to review manuscripts. If you prepare your article carefully, then this can save valuable time during the publication process.
What files to submit on revision
- A PDF of the complete revised manuscript; containing the names and institutes of authors, and figures and tables embedded within the text.
- The latest set of source files, e.g. TeX/LaTeX files or a single Word file (which includes figure/table captions), individual figure files, and tables. It is also possible to archive or compress large files.
- Any remaining permissions.
How to submit a revised article
Please submit all revised submissions via the link in the e-mail you received informing you of the decision and asking you to make the revisions.
When submitting a revised article, we require you to upload the revised PDF file (deleting the original version) and your latest set of source files used to create the revised PDF . In addition you will need to submit your point-by-point response to the referees. You will subsequently be asked to complete and submit the online assignment of copyright form, if you have not done so already.
Source File Preparation
The guidelines below provide the essential information you need to prepare your article source files (i.e. the files that you used to create your complete PDF).
Please name all your files according to the following guidelines:
- use only characters from the set a to z, A to Z, 0 to 9 and underscore (_);
- do not use spaces in file names;
- include an extension to indicate the file type (e.g., .doc, .txt, .eps, etc);
- do not use any accented characters; for example, à, ê, ñ, ö, ý, etc because these can cause difficulties when processing your files.
In addition to the above points, please give figure files names which indicate the numbers of the figures they contain; for example, figure1.eps, figure2.tif, figure2a.gif etc. If a figure file contains a figure with multiple parts, for example figure 2(a) to 2(e), give it a name such as figure2a_2e.jpg, and so forth.
TeX and LaTeX
Please do not send an amended manuscript at this stage
- ioplatexguidelines.tar
Microsoft Word
We are able to receive articles prepared using Microsoft Word for Windows or Mac.
Fonts used should be restricted to the standard font families (Times, Helvetica, Courier or Symbol). For full details, please refer to our Word guidelines.
If special symbols are needed (e.g. Greek characters, accented characters or mathematical symbols) these should be typed using the appropriate TrueType font. Do not use the Symbol facility on the 'Insert' menu as this often results in font conversion problems.
Equations must be prepared using Microsoft Word Equation Editor or the full commercial MathType package.
For articles prepared using LaTeX2e, please make sure that your figures are all supplied as EPS and linked to your main TeX files using appropriate figure inclusion commands such as \includegraphics. For articles prepared using Microsoft Word, where possible please also supply all figures as separate graphics files (in addition to being embedded in the text). Our preferred graphics format is vector Encapsulated PostScript (EPS). These files can be used directly to give high quality results and file sizes are small in comparison with most bitmap forms. Most graphics software has the facility to save as or export as EPS. For full details, please refer to our graphics guidelines .
Vector formats
The advantage of vector graphics is that they give the best possible quality at all output resolutions.
In order to get the best possible results, please note the following important points:
- Fonts used should be restricted to the standard font families (Times, Helvetica, Courier or Symbol).
- If vector EPS files include bitmap information, the bitmap should conform to the specification given in the section on graphics guidelines .
- Certain proprietary vector graphics formats such as Origin, Kaleidagraph, Cricket Graph and Gnu Plot should not be sent in their native format. If you do use these applications to create your figures, please export them as EPS.
For full details, please refer to our graphics guidelines .
Archive and compress your files
Combine all your files (article text, graphics files and, if applicable, the readme.txt file) into a single compressed archive file for ease of handling and to save you time and space. IOP supports all common compression zip formats including tar+gzip. Please ensure that the archive file has the correct extension for the compression type. To upload this file type, use the upload zipped files field. If you have any difficulty archiving your files, please contact us for assistance ( [email protected] ).
Reviewing of your paper will be handled by the FDR Editorial Office and Editorial Board. For any queries regarding this process please contact the FDR Editorial Office
If you wish to submit a short item as a Comment on a published article or a related scientific issue then you should be aware that these are subject to assessment by FDR Editorial Board. In addition, any criticized author has the right to submit a Reply (and will normally be invited to do so); this will also be assessed on receipt. The expected series of items when published consists of: Article/Comment/Reply. Where possible, a Comment and its related Reply are published together in the same journal issue.
After acceptance your article will be transferred to the IOP Publishing electronic article system. Your article will be edited and processed and a proof will be produced.
We will contact you by e-mail when the PDF proof of your article is ready for you to check. You should check your proof carefully and return corrections using the PDF annotation tools. This is the most efficient way to send them to us.
Please do not send an amended manuscript at this stage.
The ultimate responsibility for ensuring the accuracy of the published article rests with you. If proofs are subject to significant delay without a notification, we may have to publish the article without your corrections.
When checking your proofs you should take particular care checking mathematics, tables and references. Only essential corrections should be made. You should provide new files if figures need correction. We recommend that you check the accuracy of your original figures very carefully before submission: we cannot accept responsibility for any errors in original figures.
On publication, the corresponding author will receive an email with a link to access the PDF of their article for free.
You may purchase physical reprints of your article, for which the minimum order is 25. These may be ordered using a button on the article page IOPscience.
We request that authors transfer (assign) the copyright in their articles to the Japan Society of Fluid Mechanics (JSFM). This ensures that we have the right to work with, reproduce and make your article available to readers. This is the case whether you have chosen to publish on a subscription-only or on a gold open access basis.
Following the submission of your article we will ask you to electronically submit an Assignment of Copyright form via the Author Centre. The assignment of copyright to the JSFM is effective only from the date on which the article is accepted for publication. If you withdraw your article, or if it is not accepted, the transfer does not take effect. The main features of the copyright transfer are that:
- authors transfer the worldwide copyright in their work to JSFM in all formats and media;
- authors assert their moral right to be identified as the authors of the article;
- for subscription-only articles, JSFM grants back to authors certain rights for the future use of their own work , for example self-archiving rights; for full details please see the Copyright FAQ ;
- authors of gold open access articles will have the same rights as all third parties – those described in the relevant Creative Commons licence ; in most cases, this will be the CC BY 3.0 licence;
- provision is made for situations where copyright is owned by an author’s employer as well as for government employees;
- in the case of multi-author articles, only one author needs to sign the form but he or she should have obtained the verbal agreement of all the other authors beforehand.
As well as addressing matters of copyright, the form contains assertions that all authors have received the final version of the article, have agreed to it being submitted to the journal and that the content of the paper is not defamatory, fabricated or an infringement of third-party rights. The copyright assigned to JSFM covers all formats and media (including electronic, microform and paper).
FDR uses a single copyright form. Section 1 applies to authors submitting their work for subscription-only publication and Section 2 to those submitting for gold open access publication.
If you believe that the copyright form is not suitable for your circumstances, please contact [email protected] or select the 'Other' option on the form.
Copying Fluid Dynamics Research articles
Single copying of single published articles is permitted for private study or research, no matter where the copying is done. Multiple copying of journals or parts of journals without permission, however, is in breach of copyright.
Requests for permission should be directed to the FDR Editorial Office . Permission is normally given upon request for figures, tables and short extracts from the text of individual articles published in FDR to be copied, provided that the original source of the material is acknowledged in each case and the permission of the authors is also obtained.
Requests for permission of multiple copying of articles should be directed to the FDR Editorial Office .
Journal links
- Submit an article
- About the journal
- Editorial board
- Publication charges
- News and editorial
- Journal collections
- Pricing and ordering
Academia.edu no longer supports Internet Explorer.
To browse Academia.edu and the wider internet faster and more securely, please take a few seconds to upgrade your browser .
- We're Hiring!
- Help Center
Fluid Dynamics
- Most Cited Papers
- Most Downloaded Papers
- Newest Papers
- Last »
- Computational Fluid Dynamics Follow Following
- Fluid Mechanics Follow Following
- Aerodynamics Follow Following
- Turbomachinery Follow Following
- LES and DNS Follow Following
- Heat Transfer Follow Following
- Biofluids Follow Following
- Combustion Follow Following
- Turbulence Follow Following
- Multiphase flows Follow Following
Enter the email address you signed up with and we'll email you a reset link.
- Academia.edu Journals
- We're Hiring!
- Help Center
- Find new research papers in:
- Health Sciences
- Earth Sciences
- Cognitive Science
- Mathematics
- Computer Science
- Academia ©2024
Hydraulics and Fluid Mechanics, Volume 1
Select Proceedings of HYDRO 2023
- Conference proceedings
- Latest edition
- Manish Pandey 0 ,
- N V Umamahesh 1 ,
- Z. Ahmad 2 ,
- Giuseppe Oliveto 3
Department of Civil Engineering, Indian Institute of Technology Kharagpur, Kharagpur, India
You can also search for this editor in PubMed Google Scholar
Department of Civil Engineering, National Institute of Technology Warangal, Warangal, India
Department of civil engineering, indian institute of technology roorkee, roorkee, india, school of engineering,, university of basilicata, potenza, italy.
- Presents emerging opportunities and challenges in the field of hydraulics and fluid mechanics
- Covers experimental fluid mechanics, sediment dynamics, environmental impact assessment and pollutant transport
- Deals with addresses fundamental concepts and studies in the field of flood forecasting and hydraulic structures
Part of the book series: Lecture Notes in Civil Engineering (LNCE, volume 547)
Buy print copy
About this book.
This book comprises the proceedings of the 28th International Conference on Hydraulics, Water Resources, River and Coastal Engineering (HYDRO 2023) focusing on broad spectrum of emerging opportunities and challenges in the field of hydraulics and fluid mechanics. It covers a range of topics, including, but not limited to, experimental and computational fluid mechanics, sediment dynamics, environmental impact assessment of water resources projects, environmental flows, pollutant transport, etc. Presenting recent advances in the form of illustrations, tables, and text, it offers readers insights for their own research. In addition, the book addresses fundamental concepts and studies in the field of flood forecasting and hydraulic structures, making it a valuable resource for both beginners and researchers wanting to further their understanding of hydraulics, water resources and coastal engineering.
- Environmental Impact Assessment
- Environmental Flows
- Groundwater Contamination
- Geographic Information Systems
- Remote Sensing
- Hydro-Informatics
- Rainfall and Streamflow Prediction
- Optimization of Water Resources Systems
- Soft Computing Techniques
- Urban Flood Modelling
- Dam Hazard Classification
- Flood Forecasting
- Costal Disasters
- Wave-Structure Interaction
- Costal Structures and Oceanography
- Fluvial Geomorphology
- Sediment Dynamics
- Reservoir Sedimentation
- Alluvial River Problems
- Watershed Management
Editors and Affiliations
Manish Pandey
N V Umamahesh
Giuseppe Oliveto
About the editors
Dr Manish Pandey graduated in Civil Engineering from Uttarakhand Technical University, India. He completed his masters and doctorate from Indian Institute of Technology Roorkee, India. Presently, Dr Pandey is Assistant Professor at Indian Institute of Technology Kharagpur, India since 2013. He has more than 6 years teaching and research experience in experimental hydraulics and water resources engineering. He has authored more than 50 peer-reviewed journal papers and 25+ book chapters and conference proceeding papers. He has guided 1 PhD and 10 M.Tech students. Presently he is guiding 4 PhD students. He was also awarded MOST postdoctoral research grant in year 2018. He is a member of the editorial board of Journals: Environmental Development and Sustainability, Journal of Water Management Modeling, Water SA, Journal of Applied Science and Engineering and Journal of Applied Water Engineering and Research. He has also handled guest editorship for Fluid MDPI Journal, SI: Journalof Applied Water Engineering and Research and Frontiers in Environmental Science Journal, SI: The Urban Fluvial and Hydro-Environment System. Dr Pandey is an active reviewer in several reputed peer-reviewed journals.
Prof. N V Umamahesh has more than 35 years of experience as a faculty in the Department of Civil Engineering at NIT, Warangal. His area of specialization is Water Resources Engineering, and his research areas include Hydrological Modelling, Stochastic Hydrology, Hydro-Climatic Extremes, Modelling Impacts of Climate Change, Urban Floods, and Water Resources Systems. He has been well recognized for his analytical and mathematical modelling skills. He guided 13 PhD students and about 100 M.Tech students. He is currently guiding 7 PhD scholars. He has published more than 60 papers in reputed International Journals, and 6 book chapters and presented more than 50 papers in National and International Conferences. He has taken up several sponsored research and consultancy projects. He has held several administrative positions including Chief Warden, Head of the Department of Civil Engineering, Dean (Student Welfare), Dean (Planning and Development), Dean (Academic) and Coordinator of TEQIP-II. He received the Jalamitra Award from the Government of Andhra Pradesh in 2003 for the best implementation of the Watershed Development Program in Warangal District. He received the Best Engineering Faculty Award in 2017. He organized two international conferences and several faculty development programs.
Prof. Giuseppe Oliveto is Associate Professor of Hydraulic Engineering at the School of Engineering of University of Basilicata (Italy). Since 1992 he has been carrying out theoretical and experimental studies encompassing: evolution and patterns of river networks, fluvial hydraulics, sediment transport, bridge hydraulics, and urban drainage hydraulics. He is author of more than 100 papers in scientific journals and conference proceedings. He currently is Associate Editor of: Journal of Irrigation and Drainage Engineering (ASCE); Water Journal (MDPI), and Advances in Civil Engineering (Hindawi). He has been Leading Guest Editor of the Special Collection “Sediment Transport in Water-Resources Engineering” for Journal of Irrigation and Drainage Engineering (ASCE) and the Special Issue “Bridge Hydraulics: Current State of the Knowledge and Perspectives” for Water Journal (MDPI). He was recognized as: "ASCE Outstanding Reviewer" by Journal of Hydraulic Engineering (ASCE) in 2011; "ASCE Outstanding Reviewer" by Journal of Irrigation and Drainage Engineering (ASCE) in 2018 and 2019; "MDPI-Water Outstanding Reviewer" by Water Journal (MDPI) in 2018, 2019, and 2022. He has been awarded the Robert Alfred Carr Prize by the Council of the Institution of Civil Engineers (ICE) - London (UK) for the paper "Temporal scour evolution at non-uniform bridge piers" published in the Proceedings of the Institution of Civil Engineers - Water Management, Volume 170, October 2017.
Bibliographic Information
Book Title : Hydraulics and Fluid Mechanics, Volume 1
Book Subtitle : Select Proceedings of HYDRO 2023
Editors : Manish Pandey, N V Umamahesh, Z. Ahmad, Giuseppe Oliveto
Series Title : Lecture Notes in Civil Engineering
Publisher : Springer Singapore
eBook Packages : Engineering , Engineering (R0)
Copyright Information : The Editor(s) (if applicable) and The Author(s), under exclusive license to Springer Nature Singapore Pte Ltd. 2025
Hardcover ISBN : 978-981-97-8034-1 Due: 12 December 2024
Softcover ISBN : 978-981-97-8037-2 Due: 12 December 2025
eBook ISBN : 978-981-97-8035-8 Due: 12 December 2024
Series ISSN : 2366-2557
Series E-ISSN : 2366-2565
Edition Number : 1
- Publish with us
Policies and ethics
- Find a journal
- Track your research

COMMENTS
Fluid Dynamics Research. originated from a voluntary party of researchers working on fluid mechanics in 1968. The objectives of the society were to discuss about scientific and engineering problems relevant to fluid motion among researchers working in Physics, Engineering and the interdisciplinary fields and to assist in their research activities.
Fluid dynamics articles from across Nature Portfolio. Fluid dynamics is the study of the motion of liquids, gases and plasmas. Flow is dependent on the intrinsic properties of the matter itself ...
Overview. Fluid Dynamics is a peer-reviewed journal that focuses on studies of motion, behavior, and interactions of liquids and gases. Covers on aeromechanics, hydrodynamics, plasma dynamics, underground hydrodynamics, and biomechanics of continuous media. Highlights emerging trends at the forefront of science, such as multi-phase flows ...
Read the latest articles of Fluid Dynamics Research at ScienceDirect.com, Elsevier's leading platform of peer-reviewed scholarly literature.
A computational fluid dynamics study to assess the impact of coughing on cerebrospinal fluid dynamics in Chiari type 1 malformation. Sarah Vandenbulcke. , Paul Condron.
Fluid dynamics is a sub-discipline of fluid mechanics that deals with fluid flow—the natural science of fluids (liquids and gases) in motion. | Explore the latest full-text research PDFs ...
"Fluid Dynamics Research" whose first volume was published in 1986 is the official journal of the JSFM. "Fluid Dynamics Research" is a well-established international journal of Fluid Mechanics, published six times per year by IOPP (Institute of Physics Publishing) on behalf of the JSFM since 2009.
Theoretical and Computational Fluid Dynamics: Addresses scientists, engineers and applied mathematicians working in all fields concerned with fundamental aspects of fluid flow and provides a forum for the cross-fertilization of ideas and techniques across all disciplines in which fluid flow plays a role.
Scope. Fluid Dynamics Research publishes original and creative works in all fields of fluid dynamics. The scope includes theoretical, numerical and experimental studies as well as data-driven approaches that contribute to the fundamental understanding and/or application of fluid flow phenomena, including turbulence, instability, nonlinear waves ...
The literature research in this work has revealed that the majority of fluid dynamics and mechanics applications are currently investing in Deep Neural Network applications on classical CFD problems, from finding solutions to PDEs to analyzing high-fidelity fluid-related images.
A National e-Conference on "Recent Trends in Fluid Dynamics Research (R TFDR-. 21)" was organized by fluid dynamics and heat transfer research groups of the. Department of Chemical ...
Volume 37, Issue 9-10 of International Journal of Computational Fluid Dynamics
The International Journal of Computational Fluid Dynamics publishes innovative CFD research, both fundamental and applied, in a wide variety of fluids and physics fields. The Journal emphasizes accurate predictive tools for 3D flow analysis and design, and those promoting a deeper understanding of the physics of 3D fluid motion.
Fluid dynamics is a research area lying at the crossroads of physics and applied mathematics with an ever-expanding range of applications in natural sciences and engineering. However, despite decades of concerted research efforts, this area abounds with ...
This book presents select proceedings of Conference on Recent Trends in Fluid Dynamics Research (RTFDR-21). It signifies the current research trends in fluid dynamics and convection heat transfer for both laminar and turbulent flow structures. The topics covered include fluid mechanics and applications, microfluidics and nanofluidics, numerical ...
The dynamics of flexible filaments entrained in flow are important for understanding many biological and industrial processes. This work describes a data-driven technique to create high-fidelity low-dimensional models of flexible fiber dynamics using machine learning; the technique is applied to sedimentation in a quiescent, viscous Newtonian fluid, using results from detailed simulations as ...
International Journal of Fluid Mechanics Research publishes original and innovative research on fluid dynamics, rheology, and thermodynamics. Browse the latest articles and sample issues online.
Numerical analysis of the flow separation and adverse pressure gradient in laminar boundary layer over a flat plate due to a rotating cylinder in the vicinity. Farhana Afroz and Muhammad A R Sharif. Open abstract View article PDF. 025502.
Fluid mechanics can be divided into fluid statics, the study of fluids at rest;... | Explore the latest full-text research PDFs, articles, conference papers, preprints and more on FLUID MECHANICS.
Abstract: Computational Fluid Dynamics (CFD) stands at the forefront of modern engineering and scientific simulations, offering powerful insights into the behavior of fluid flows across diverse applications. This research paper provides a comprehensive overview of CFD, covering its fundamental principles, numerical methods, applications in engineering and science, as well as current challenges ...
PDF | This paper will answer a list of questions regarding the computational fluid dynamics (CFD). It will give brief discussion regarding the... | Find, read and cite all the research you need on ...
Author guidelines - Fluid Dynamics Research - IOPscience. Fluid Dynamics Research. The Japan Society of Fluid Mechanics (JSFM) originated from a voluntary party of researchers working on fluid mechanics in 1968. The objectives of the society were to discuss about scientific and engineering problems relevant to fluid motion among researchers ...
The PDE framework Peano applied to fluid dynamics: an efficient implementation of a parallel multiscale fluid dynamics solver on octree-like adaptive Cartesian grids. This paper presents the general purpose framework Peano for the solution of partial differential equations (PDE) on adaptive Cartesian grids.
It covers a range of topics, including, but not limited to, experimental and computational fluid mechanics, sediment dynamics, environmental impact assessment of water resources projects, environmental flows, pollutant transport, etc. Presenting recent advances in the form of illustrations, tables, and text, it offers readers insights for their ...