Have a language expert improve your writing
Run a free plagiarism check in 10 minutes, generate accurate citations for free.
- Knowledge Base
Methodology
- Sampling Methods | Types, Techniques & Examples

Sampling Methods | Types, Techniques & Examples
Published on September 19, 2019 by Shona McCombes . Revised on June 22, 2023.
When you conduct research about a group of people, it’s rarely possible to collect data from every person in that group. Instead, you select a sample . The sample is the group of individuals who will actually participate in the research.
To draw valid conclusions from your results, you have to carefully decide how you will select a sample that is representative of the group as a whole. This is called a sampling method . There are two primary types of sampling methods that you can use in your research:
- Probability sampling involves random selection, allowing you to make strong statistical inferences about the whole group.
- Non-probability sampling involves non-random selection based on convenience or other criteria, allowing you to easily collect data.
You should clearly explain how you selected your sample in the methodology section of your paper or thesis, as well as how you approached minimizing research bias in your work.
Table of contents
Population vs. sample, probability sampling methods, non-probability sampling methods, other interesting articles, frequently asked questions about sampling.
First, you need to understand the difference between a population and a sample , and identify the target population of your research.
- The population is the entire group that you want to draw conclusions about.
- The sample is the specific group of individuals that you will collect data from.
The population can be defined in terms of geographical location, age, income, or many other characteristics.
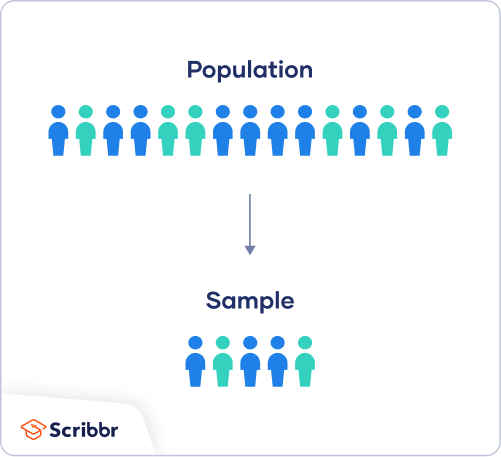
It is important to carefully define your target population according to the purpose and practicalities of your project.
If the population is very large, demographically mixed, and geographically dispersed, it might be difficult to gain access to a representative sample. A lack of a representative sample affects the validity of your results, and can lead to several research biases , particularly sampling bias .
Sampling frame
The sampling frame is the actual list of individuals that the sample will be drawn from. Ideally, it should include the entire target population (and nobody who is not part of that population).
Sample size
The number of individuals you should include in your sample depends on various factors, including the size and variability of the population and your research design. There are different sample size calculators and formulas depending on what you want to achieve with statistical analysis .
Receive feedback on language, structure, and formatting
Professional editors proofread and edit your paper by focusing on:
- Academic style
- Vague sentences
- Style consistency
See an example
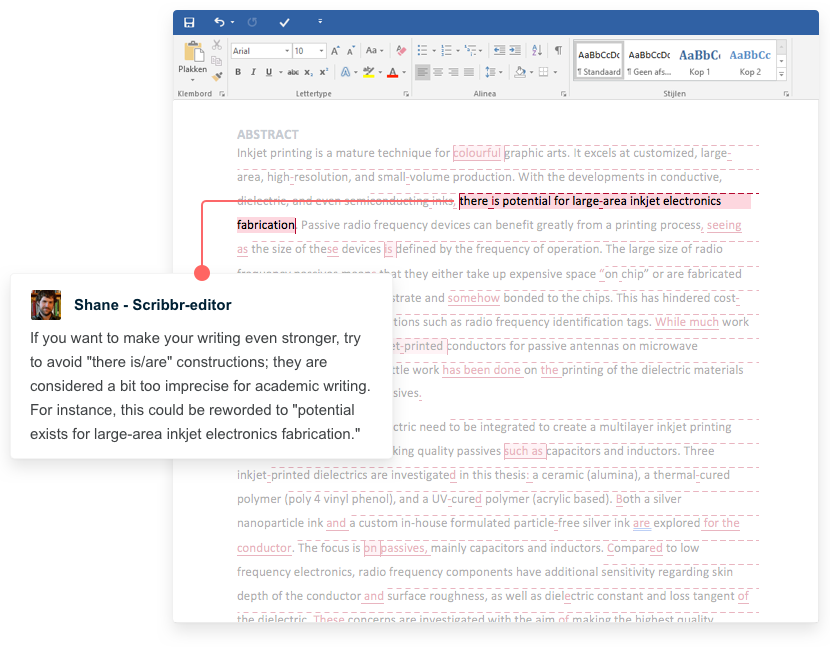
Probability sampling means that every member of the population has a chance of being selected. It is mainly used in quantitative research . If you want to produce results that are representative of the whole population, probability sampling techniques are the most valid choice.
There are four main types of probability sample.
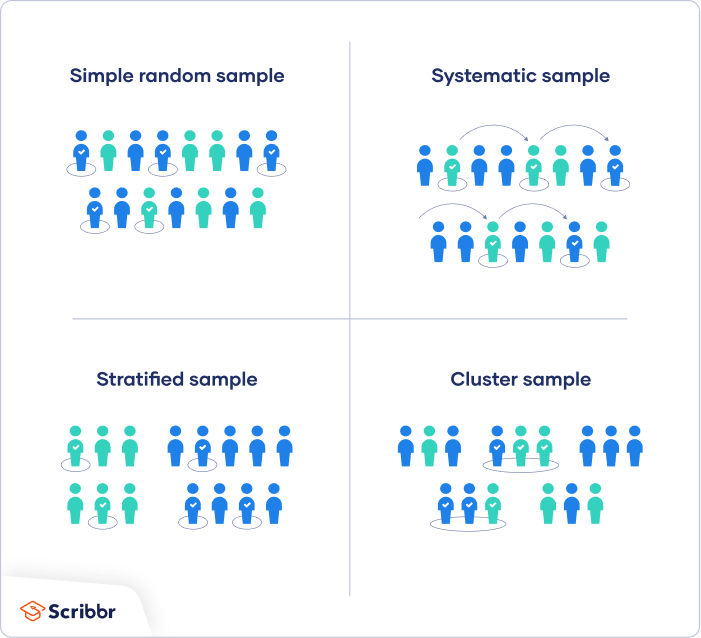
1. Simple random sampling
In a simple random sample, every member of the population has an equal chance of being selected. Your sampling frame should include the whole population.
To conduct this type of sampling, you can use tools like random number generators or other techniques that are based entirely on chance.
2. Systematic sampling
Systematic sampling is similar to simple random sampling, but it is usually slightly easier to conduct. Every member of the population is listed with a number, but instead of randomly generating numbers, individuals are chosen at regular intervals.
If you use this technique, it is important to make sure that there is no hidden pattern in the list that might skew the sample. For example, if the HR database groups employees by team, and team members are listed in order of seniority, there is a risk that your interval might skip over people in junior roles, resulting in a sample that is skewed towards senior employees.
3. Stratified sampling
Stratified sampling involves dividing the population into subpopulations that may differ in important ways. It allows you draw more precise conclusions by ensuring that every subgroup is properly represented in the sample.
To use this sampling method, you divide the population into subgroups (called strata) based on the relevant characteristic (e.g., gender identity, age range, income bracket, job role).
Based on the overall proportions of the population, you calculate how many people should be sampled from each subgroup. Then you use random or systematic sampling to select a sample from each subgroup.
4. Cluster sampling
Cluster sampling also involves dividing the population into subgroups, but each subgroup should have similar characteristics to the whole sample. Instead of sampling individuals from each subgroup, you randomly select entire subgroups.
If it is practically possible, you might include every individual from each sampled cluster. If the clusters themselves are large, you can also sample individuals from within each cluster using one of the techniques above. This is called multistage sampling .
This method is good for dealing with large and dispersed populations, but there is more risk of error in the sample, as there could be substantial differences between clusters. It’s difficult to guarantee that the sampled clusters are really representative of the whole population.
In a non-probability sample, individuals are selected based on non-random criteria, and not every individual has a chance of being included.
This type of sample is easier and cheaper to access, but it has a higher risk of sampling bias . That means the inferences you can make about the population are weaker than with probability samples, and your conclusions may be more limited. If you use a non-probability sample, you should still aim to make it as representative of the population as possible.
Non-probability sampling techniques are often used in exploratory and qualitative research . In these types of research, the aim is not to test a hypothesis about a broad population, but to develop an initial understanding of a small or under-researched population.
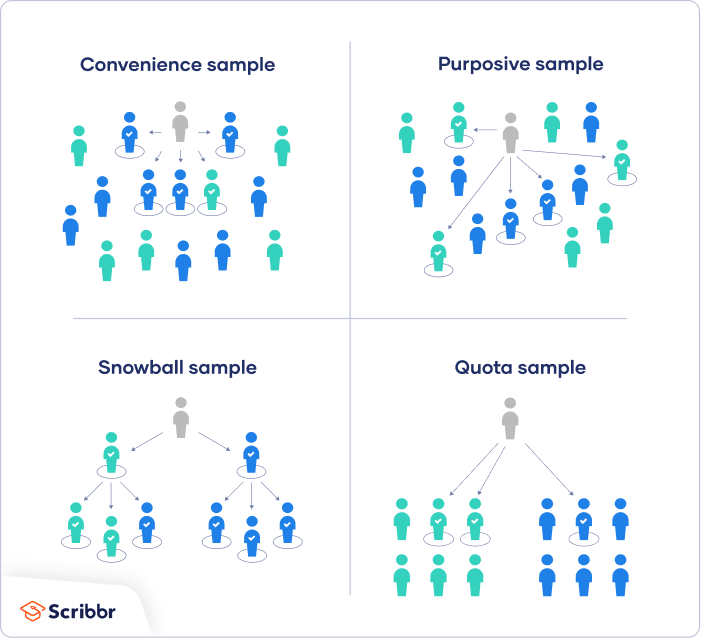
1. Convenience sampling
A convenience sample simply includes the individuals who happen to be most accessible to the researcher.
This is an easy and inexpensive way to gather initial data, but there is no way to tell if the sample is representative of the population, so it can’t produce generalizable results. Convenience samples are at risk for both sampling bias and selection bias .
2. Voluntary response sampling
Similar to a convenience sample, a voluntary response sample is mainly based on ease of access. Instead of the researcher choosing participants and directly contacting them, people volunteer themselves (e.g. by responding to a public online survey).
Voluntary response samples are always at least somewhat biased , as some people will inherently be more likely to volunteer than others, leading to self-selection bias .
3. Purposive sampling
This type of sampling, also known as judgement sampling, involves the researcher using their expertise to select a sample that is most useful to the purposes of the research.
It is often used in qualitative research , where the researcher wants to gain detailed knowledge about a specific phenomenon rather than make statistical inferences, or where the population is very small and specific. An effective purposive sample must have clear criteria and rationale for inclusion. Always make sure to describe your inclusion and exclusion criteria and beware of observer bias affecting your arguments.
4. Snowball sampling
If the population is hard to access, snowball sampling can be used to recruit participants via other participants. The number of people you have access to “snowballs” as you get in contact with more people. The downside here is also representativeness, as you have no way of knowing how representative your sample is due to the reliance on participants recruiting others. This can lead to sampling bias .
5. Quota sampling
Quota sampling relies on the non-random selection of a predetermined number or proportion of units. This is called a quota.
You first divide the population into mutually exclusive subgroups (called strata) and then recruit sample units until you reach your quota. These units share specific characteristics, determined by you prior to forming your strata. The aim of quota sampling is to control what or who makes up your sample.
If you want to know more about statistics , methodology , or research bias , make sure to check out some of our other articles with explanations and examples.
- Student’s t -distribution
- Normal distribution
- Null and Alternative Hypotheses
- Chi square tests
- Confidence interval
- Quartiles & Quantiles
- Cluster sampling
- Stratified sampling
- Data cleansing
- Reproducibility vs Replicability
- Peer review
- Prospective cohort study
Research bias
- Implicit bias
- Cognitive bias
- Placebo effect
- Hawthorne effect
- Hindsight bias
- Affect heuristic
- Social desirability bias
A sample is a subset of individuals from a larger population . Sampling means selecting the group that you will actually collect data from in your research. For example, if you are researching the opinions of students in your university, you could survey a sample of 100 students.
In statistics, sampling allows you to test a hypothesis about the characteristics of a population.
Samples are used to make inferences about populations . Samples are easier to collect data from because they are practical, cost-effective, convenient, and manageable.
Probability sampling means that every member of the target population has a known chance of being included in the sample.
Probability sampling methods include simple random sampling , systematic sampling , stratified sampling , and cluster sampling .
In non-probability sampling , the sample is selected based on non-random criteria, and not every member of the population has a chance of being included.
Common non-probability sampling methods include convenience sampling , voluntary response sampling, purposive sampling , snowball sampling, and quota sampling .
In multistage sampling , or multistage cluster sampling, you draw a sample from a population using smaller and smaller groups at each stage.
This method is often used to collect data from a large, geographically spread group of people in national surveys, for example. You take advantage of hierarchical groupings (e.g., from state to city to neighborhood) to create a sample that’s less expensive and time-consuming to collect data from.
Sampling bias occurs when some members of a population are systematically more likely to be selected in a sample than others.
Cite this Scribbr article
If you want to cite this source, you can copy and paste the citation or click the “Cite this Scribbr article” button to automatically add the citation to our free Citation Generator.
McCombes, S. (2023, June 22). Sampling Methods | Types, Techniques & Examples. Scribbr. Retrieved August 5, 2024, from https://www.scribbr.com/methodology/sampling-methods/
Is this article helpful?
Shona McCombes
Other students also liked, population vs. sample | definitions, differences & examples, simple random sampling | definition, steps & examples, sampling bias and how to avoid it | types & examples, what is your plagiarism score.
Educational resources and simple solutions for your research journey
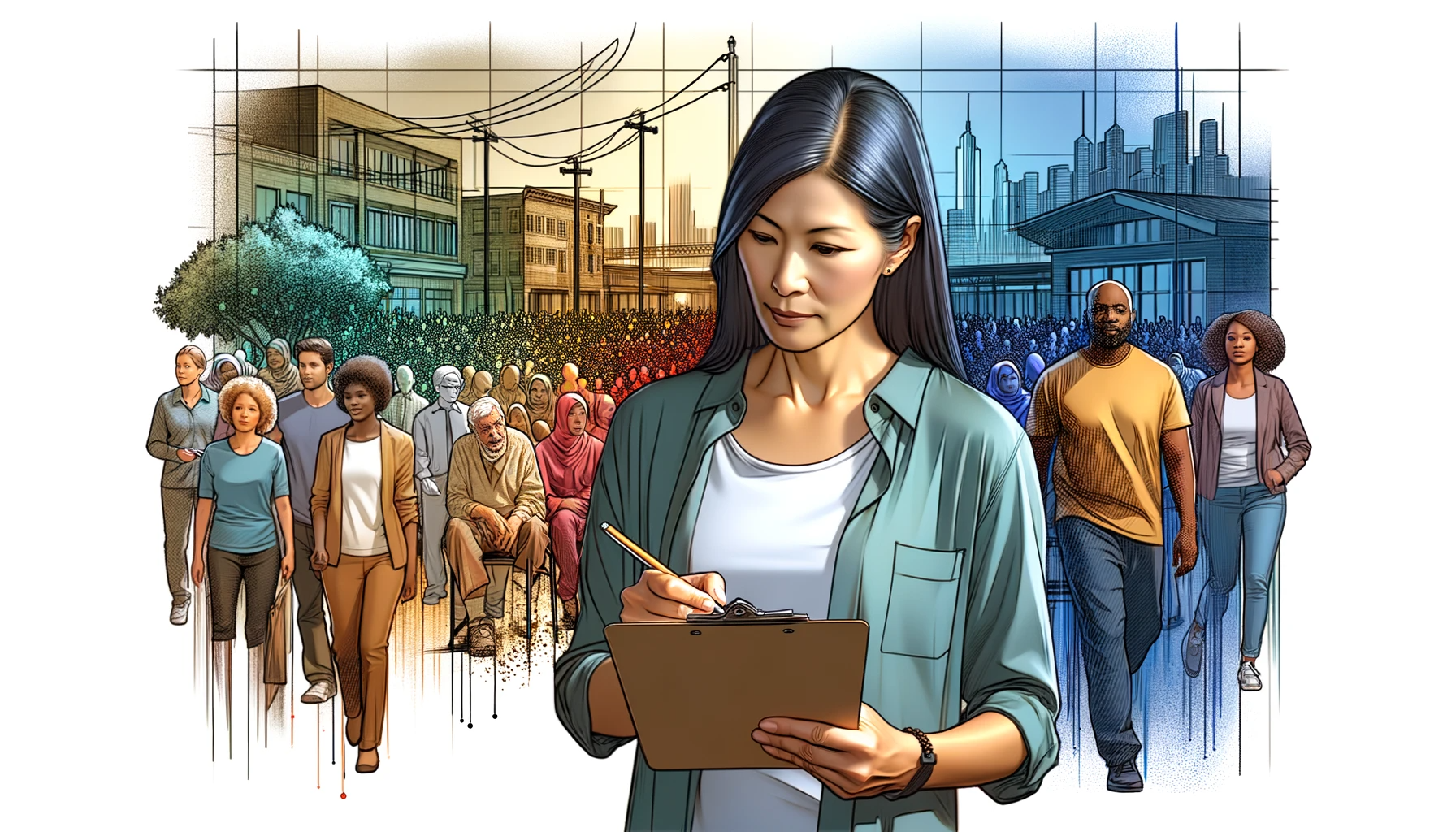
What are Sampling Methods? Techniques, Types, and Examples
Every type of research includes samples from which inferences are drawn. The sample could be biological specimens or a subset of a specific group or population selected for analysis. The goal is often to conclude the entire population based on the characteristics observed in the sample. Now, the question comes to mind: how does one collect the samples? Answer: Using sampling methods. Various sampling strategies are available to researchers to define and collect samples that will form the basis of their research study.
In a study focusing on individuals experiencing anxiety, gathering data from the entire population is practically impossible due to the widespread prevalence of anxiety. Consequently, a sample is carefully selected—a subset of individuals meant to represent (or not in some cases accurately) the demographics of those experiencing anxiety. The study’s outcomes hinge significantly on the chosen sample, emphasizing the critical importance of a thoughtful and precise selection process. The conclusions drawn about the broader population rely heavily on the selected sample’s characteristics and diversity.
Table of Contents
What is sampling?
Sampling involves the strategic selection of individuals or a subset from a population, aiming to derive statistical inferences and predict the characteristics of the entire population. It offers a pragmatic and practical approach to examining the features of the whole population, which would otherwise be difficult to achieve because studying the total population is expensive, time-consuming, and often impossible. Market researchers use various sampling methods to collect samples from a large population to acquire relevant insights. The best sampling strategy for research is determined by criteria such as the purpose of the study, available resources (time and money), and research hypothesis.
For example, if a pet food manufacturer wants to investigate the positive impact of a new cat food on feline growth, studying all the cats in the country is impractical. In such cases, employing an appropriate sampling technique from the extensive dataset allows the researcher to focus on a manageable subset. This enables the researcher to study the growth-promoting effects of the new pet food. This article will delve into the standard sampling methods and explore the situations in which each is most appropriately applied.
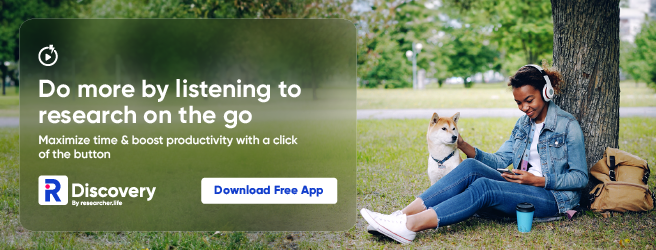
What are sampling methods or sampling techniques?
Sampling methods or sampling techniques in research are statistical methods for selecting a sample representative of the whole population to study the population’s characteristics. Sampling methods serve as invaluable tools for researchers, enabling the collection of meaningful data and facilitating analysis to identify distinctive features of the people. Different sampling strategies can be used based on the characteristics of the population, the study purpose, and the available resources. Now that we understand why sampling methods are essential in research, we review the various sample methods in the following sections.
Types of sampling methods
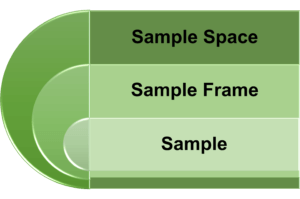
Before we go into the specifics of each sampling method, it’s vital to understand terms like sample, sample frame, and sample space. In probability theory, the sample space comprises all possible outcomes of a random experiment, while the sample frame is the list or source guiding sample selection in statistical research. The sample represents the group of individuals participating in the study, forming the basis for the research findings. Selecting the correct sample is critical to ensuring the validity and reliability of any research; the sample should be representative of the population.
There are two most common sampling methods:
- Probability sampling: A sampling method in which each unit or element in the population has an equal chance of being selected in the final sample. This is called random sampling, emphasizing the random and non-zero probability nature of selecting samples. Such a sampling technique ensures a more representative and unbiased sample, enabling robust inferences about the entire population.
- Non-probability sampling: Another sampling method is non-probability sampling, which involves collecting data conveniently through a non-random selection based on predefined criteria. This offers a straightforward way to gather data, although the resulting sample may or may not accurately represent the entire population.
Irrespective of the research method you opt for, it is essential to explicitly state the chosen sampling technique in the methodology section of your research article. Now, we will explore the different characteristics of both sampling methods, along with various subtypes falling under these categories.
What is probability sampling?
The probability sampling method is based on the probability theory, which means that the sample selection criteria involve some random selection. The probability sampling method provides an equal opportunity for all elements or units within the entire sample space to be chosen. While it can be labor-intensive and expensive, the advantage lies in its ability to offer a more accurate representation of the population, thereby enhancing confidence in the inferences drawn in the research.
Types of probability sampling
Various probability sampling methods exist, such as simple random sampling, systematic sampling, stratified sampling, and clustered sampling. Here, we provide detailed discussions and illustrative examples for each of these sampling methods:
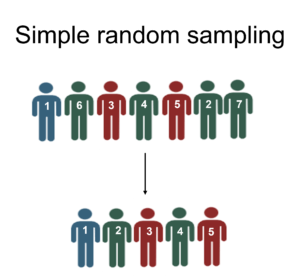
- Simple random sampling: In simple random sampling, each individual has an equal probability of being chosen, and each selection is independent of the others. Because the choice is entirely based on chance, this is also known as the method of chance selection. In the simple random sampling method, the sample frame comprises the entire population.
For example, A fitness sports brand is launching a new protein drink and aims to select 20 individuals from a 200-person fitness center to try it. Employing a simple random sampling approach, each of the 200 people is assigned a unique identifier. Of these, 20 individuals are then chosen by generating random numbers between 1 and 200, either manually or through a computer program. Matching these numbers with the individuals creates a randomly selected group of 20 people. This method minimizes sampling bias and ensures a representative subset of the entire population under study.
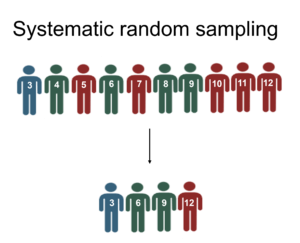
- Systematic sampling: The systematic sampling approach involves selecting units or elements at regular intervals from an ordered list of the population. Because the starting point of this sampling method is chosen at random, it is more convenient than essential random sampling. For a better understanding, consider the following example.
For example, considering the previous model, individuals at the fitness facility are arranged alphabetically. The manufacturer then initiates the process by randomly selecting a starting point from the first ten positions, let’s say 8. Starting from the 8th position, every tenth person on the list is then chosen (e.g., 8, 18, 28, 38, and so forth) until a sample of 20 individuals is obtained.
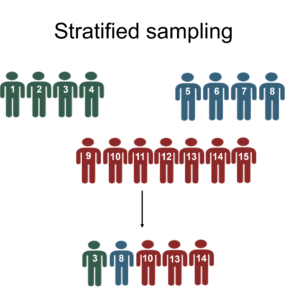
- Stratified sampling: Stratified sampling divides the population into subgroups (strata), and random samples are drawn from each stratum in proportion to its size in the population. Stratified sampling provides improved representation because each subgroup that differs in significant ways is included in the final sample.
For example, Expanding on the previous simple random sampling example, suppose the manufacturer aims for a more comprehensive representation of genders in a sample of 200 people, consisting of 90 males, 80 females, and 30 others. The manufacturer categorizes the population into three gender strata (Male, Female, and Others). Within each group, random sampling is employed to select nine males, eight females, and three individuals from the others category, resulting in a well-rounded and representative sample of 200 individuals.
- Clustered sampling: In this sampling method, the population is divided into clusters, and then a random sample of clusters is included in the final sample. Clustered sampling, distinct from stratified sampling, involves subgroups (clusters) that exhibit characteristics similar to the whole sample. In the case of small clusters, all members can be included in the final sample, whereas for larger clusters, individuals within each cluster may be sampled using the sampling above methods. This approach is referred to as multistage sampling. This sampling method is well-suited for large and widely distributed populations; however, there is a potential risk of sample error because ensuring that the sampled clusters truly represent the entire population can be challenging.
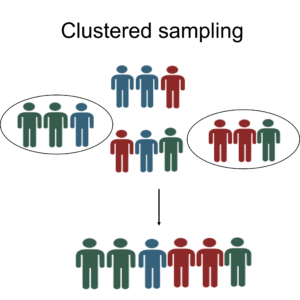
For example, Researchers conducting a nationwide health study can select specific geographic clusters, like cities or regions, instead of trying to survey the entire population individually. Within each chosen cluster, they sample individuals, providing a representative subset without the logistical challenges of attempting a nationwide survey.
Use s of probability sampling
Probability sampling methods find widespread use across diverse research disciplines because of their ability to yield representative and unbiased samples. The advantages of employing probability sampling include the following:
- Representativeness
Probability sampling assures that every element in the population has a non-zero chance of being included in the sample, ensuring representativeness of the entire population and decreasing research bias to minimal to non-existent levels. The researcher can acquire higher-quality data via probability sampling, increasing confidence in the conclusions.
- Statistical inference
Statistical methods, like confidence intervals and hypothesis testing, depend on probability sampling to generalize findings from a sample to the broader population. Probability sampling methods ensure unbiased representation, allowing inferences about the population based on the characteristics of the sample.
- Precision and reliability
The use of probability sampling improves the precision and reliability of study results. Because the probability of selecting any single element/individual is known, the chance variations that may occur in non-probability sampling methods are reduced, resulting in more dependable and precise estimations.
- Generalizability
Probability sampling enables the researcher to generalize study findings to the entire population from which they were derived. The results produced through probability sampling methods are more likely to be applicable to the larger population, laying the foundation for making broad predictions or recommendations.
- Minimization of Selection Bias
By ensuring that each member of the population has an equal chance of being selected in the sample, probability sampling lowers the possibility of selection bias. This reduces the impact of systematic errors that may occur in non-probability sampling methods, where data may be skewed toward a specific demographic due to inadequate representation of each segment of the population.
What is non-probability sampling?
Non-probability sampling methods involve selecting individuals based on non-random criteria, often relying on the researcher’s judgment or predefined criteria. While it is easier and more economical, it tends to introduce sampling bias, resulting in weaker inferences compared to probability sampling techniques in research.
Types of Non-probability Sampling
Non-probability sampling methods are further classified as convenience sampling, consecutive sampling, quota sampling, purposive or judgmental sampling, and snowball sampling. Let’s explore these types of sampling methods in detail.
- Convenience sampling: In convenience sampling, individuals are recruited directly from the population based on the accessibility and proximity to the researcher. It is a simple, inexpensive, and practical method of sample selection, yet convenience sampling suffers from both sampling and selection bias due to a lack of appropriate population representation.
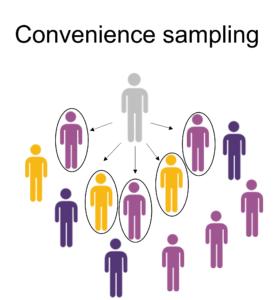
For example, imagine you’re a researcher investigating smartphone usage patterns in your city. The most convenient way to select participants is by approaching people in a shopping mall on a weekday afternoon. However, this convenience sampling method may not be an accurate representation of the city’s overall smartphone usage patterns as the sample is limited to individuals present at the mall during weekdays, excluding those who visit on other days or never visit the mall.
- Consecutive sampling: Participants in consecutive sampling (or sequential sampling) are chosen based on their availability and desire to participate in the study as they become available. This strategy entails sequentially recruiting individuals who fulfill the researcher’s requirements.
For example, In researching the prevalence of stroke in a hospital, instead of randomly selecting patients from the entire population, the researcher can opt to include all eligible patients admitted over three months. Participants are then consecutively recruited upon admission during that timeframe, forming the study sample.
- Quota sampling: The selection of individuals in quota sampling is based on non-random selection criteria in which only participants with certain traits or proportions that are representative of the population are included. Quota sampling involves setting predetermined quotas for specific subgroups based on key demographics or other relevant characteristics. This sampling method employs dividing the population into mutually exclusive subgroups and then selecting sample units until the set quota is reached.
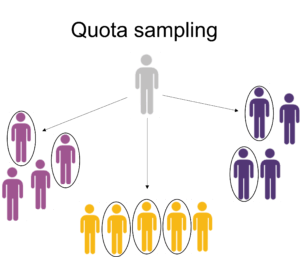
For example, In a survey on a college campus to assess student interest in a new policy, the researcher should establish quotas aligned with the distribution of student majors, ensuring representation from various academic disciplines. If the campus has 20% biology majors, 30% engineering majors, 20% business majors, and 30% liberal arts majors, participants should be recruited to mirror these proportions.
- Purposive or judgmental sampling: In purposive sampling, the researcher leverages expertise to select a sample relevant to the study’s specific questions. This sampling method is commonly applied in qualitative research, mainly when aiming to understand a particular phenomenon, and is suitable for smaller population sizes.
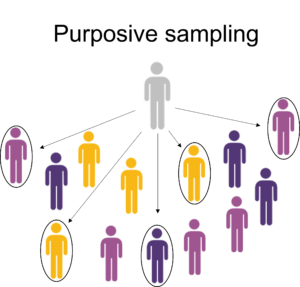
For example, imagine a researcher who wants to study public policy issues for a focus group. The researcher might purposely select participants with expertise in economics, law, and public administration to take advantage of their knowledge and ensure a depth of understanding.
- Snowball sampling: This sampling method is used when accessing the population is challenging. It involves collecting the sample through a chain-referral process, where each recruited candidate aids in finding others. These candidates share common traits, representing the targeted population. This method is often used in qualitative research, particularly when studying phenomena related to stigmatized or hidden populations.

For example, In a study focusing on understanding the experiences and challenges of individuals in hidden or stigmatized communities (e.g., LGBTQ+ individuals in specific cultural contexts), the snowball sampling technique can be employed. The researcher initiates contact with one community member, who then assists in identifying additional candidates until the desired sample size is achieved.
Uses of non-probability sampling
Non-probability sampling approaches are employed in qualitative or exploratory research where the goal is to investigate underlying population traits rather than generalizability. Non-probability sampling methods are also helpful for the following purposes:
- Generating a hypothesis
In the initial stages of exploratory research, non-probability methods such as purposive or convenience allow researchers to quickly gather information and generate hypothesis that helps build a future research plan.
- Qualitative research
Qualitative research is usually focused on understanding the depth and complexity of human experiences, behaviors, and perspectives. Non-probability methods like purposive or snowball sampling are commonly used to select participants with specific traits that are relevant to the research question.
- Convenience and pragmatism
Non-probability sampling methods are valuable when resource and time are limited or when preliminary data is required to test the pilot study. For example, conducting a survey at a local shopping mall to gather opinions on a consumer product due to the ease of access to potential participants.
Probability vs Non-probability Sampling Methods
Selection of participants | Random selection of participants from the population using randomization methods | Non-random selection of participants from the population based on convenience or criteria |
Representativeness | Likely to yield a representative sample of the whole population allowing for generalizations | May not yield a representative sample of the whole population; poor generalizability |
Precision and accuracy | Provides more precise and accurate estimates of population characteristics | May have less precision and accuracy due to non-random selection |
Bias | Minimizes selection bias | May introduce selection bias if criteria are subjective and not well-defined |
Statistical inference | Suited for statistical inference and hypothesis testing and for making generalization to the population | Less suited for statistical inference and hypothesis testing on the population |
Application | Useful for quantitative research where generalizability is crucial | Commonly used in qualitative and exploratory research where in-depth insights are the goal |
Frequently asked questions
- What is multistage sampling ? Multistage sampling is a form of probability sampling approach that involves the progressive selection of samples in stages, going from larger clusters to a small number of participants, making it suited for large-scale research with enormous population lists.
- What are the methods of probability sampling? Probability sampling methods are simple random sampling, stratified random sampling, systematic sampling, cluster sampling, and multistage sampling.
- How to decide which type of sampling method to use? Choose a sampling method based on the goals, population, and resources. Probability for statistics and non-probability for efficiency or qualitative insights can be considered . Also, consider the population characteristics, size, and alignment with study objectives.
- What are the methods of non-probability sampling? Non-probability sampling methods are convenience sampling, consecutive sampling, purposive sampling, snowball sampling, and quota sampling.
- Why are sampling methods used in research? Sampling methods in research are employed to efficiently gather representative data from a subset of a larger population, enabling valid conclusions and generalizations while minimizing costs and time.
R Discovery is a literature search and research reading platform that accelerates your research discovery journey by keeping you updated on the latest, most relevant scholarly content. With 250M+ research articles sourced from trusted aggregators like CrossRef, Unpaywall, PubMed, PubMed Central, Open Alex and top publishing houses like Springer Nature, JAMA, IOP, Taylor & Francis, NEJM, BMJ, Karger, SAGE, Emerald Publishing and more, R Discovery puts a world of research at your fingertips.
Try R Discovery Prime FREE for 1 week or upgrade at just US$72 a year to access premium features that let you listen to research on the go, read in your language, collaborate with peers, auto sync with reference managers, and much more. Choose a simpler, smarter way to find and read research – Download the app and start your free 7-day trial today !
Related Posts

What is Cluster Sampling? Definition, Method, and Examples

What is Thematic Analysis and How to Do It (with Examples)
Sampling Methods In Reseach: Types, Techniques, & Examples
Saul Mcleod, PhD
Editor-in-Chief for Simply Psychology
BSc (Hons) Psychology, MRes, PhD, University of Manchester
Saul Mcleod, PhD., is a qualified psychology teacher with over 18 years of experience in further and higher education. He has been published in peer-reviewed journals, including the Journal of Clinical Psychology.
Learn about our Editorial Process
Olivia Guy-Evans, MSc
Associate Editor for Simply Psychology
BSc (Hons) Psychology, MSc Psychology of Education
Olivia Guy-Evans is a writer and associate editor for Simply Psychology. She has previously worked in healthcare and educational sectors.
On This Page:
Sampling methods in psychology refer to strategies used to select a subset of individuals (a sample) from a larger population, to study and draw inferences about the entire population. Common methods include random sampling, stratified sampling, cluster sampling, and convenience sampling. Proper sampling ensures representative, generalizable, and valid research results.
- Sampling : the process of selecting a representative group from the population under study.
- Target population : the total group of individuals from which the sample might be drawn.
- Sample: a subset of individuals selected from a larger population for study or investigation. Those included in the sample are termed “participants.”
- Generalizability : the ability to apply research findings from a sample to the broader target population, contingent on the sample being representative of that population.
For instance, if the advert for volunteers is published in the New York Times, this limits how much the study’s findings can be generalized to the whole population, because NYT readers may not represent the entire population in certain respects (e.g., politically, socio-economically).
The Purpose of Sampling
We are interested in learning about large groups of people with something in common in psychological research. We call the group interested in studying our “target population.”
In some types of research, the target population might be as broad as all humans. Still, in other types of research, the target population might be a smaller group, such as teenagers, preschool children, or people who misuse drugs.
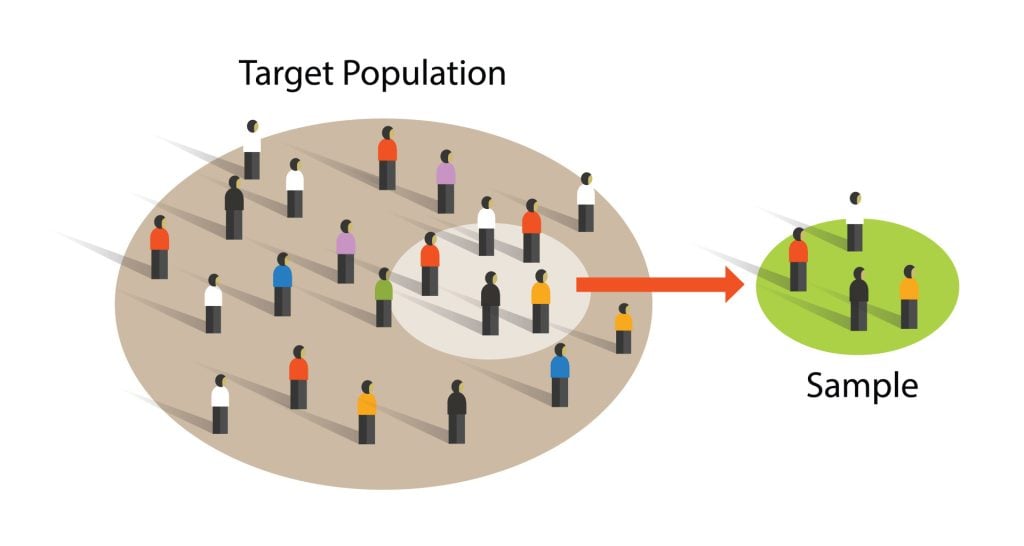
Studying every person in a target population is more or less impossible. Hence, psychologists select a sample or sub-group of the population that is likely to be representative of the target population we are interested in.
This is important because we want to generalize from the sample to the target population. The more representative the sample, the more confident the researcher can be that the results can be generalized to the target population.
One of the problems that can occur when selecting a sample from a target population is sampling bias. Sampling bias refers to situations where the sample does not reflect the characteristics of the target population.
Many psychology studies have a biased sample because they have used an opportunity sample that comprises university students as their participants (e.g., Asch ).
OK, so you’ve thought up this brilliant psychological study and designed it perfectly. But who will you try it out on, and how will you select your participants?
There are various sampling methods. The one chosen will depend on a number of factors (such as time, money, etc.).
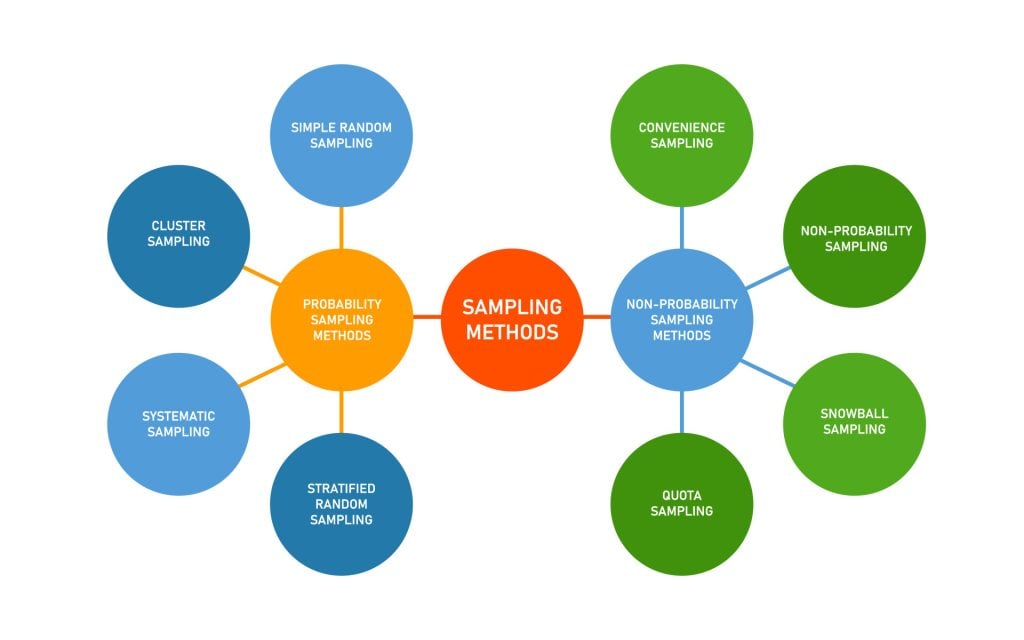
Random Sampling
Random sampling is a type of probability sampling where everyone in the entire target population has an equal chance of being selected.
This is similar to the national lottery. If the “population” is everyone who bought a lottery ticket, then everyone has an equal chance of winning the lottery (assuming they all have one ticket each).
Random samples require naming or numbering the target population and then using some raffle method to choose those to make up the sample. Random samples are the best method of selecting your sample from the population of interest.
- The advantages are that your sample should represent the target population and eliminate sampling bias.
- The disadvantage is that it is very difficult to achieve (i.e., time, effort, and money).
Stratified Sampling
During stratified sampling , the researcher identifies the different types of people that make up the target population and works out the proportions needed for the sample to be representative.
A list is made of each variable (e.g., IQ, gender, etc.) that might have an effect on the research. For example, if we are interested in the money spent on books by undergraduates, then the main subject studied may be an important variable.
For example, students studying English Literature may spend more money on books than engineering students, so if we use a large percentage of English students or engineering students, our results will not be accurate.
We have to determine the relative percentage of each group at a university, e.g., Engineering 10%, Social Sciences 15%, English 20%, Sciences 25%, Languages 10%, Law 5%, and Medicine 15%. The sample must then contain all these groups in the same proportion as the target population (university students).
- The disadvantage of stratified sampling is that gathering such a sample would be extremely time-consuming and difficult to do. This method is rarely used in Psychology.
- However, the advantage is that the sample should be highly representative of the target population, and therefore we can generalize from the results obtained.
Opportunity Sampling
Opportunity sampling is a method in which participants are chosen based on their ease of availability and proximity to the researcher, rather than using random or systematic criteria. It’s a type of convenience sampling .
An opportunity sample is obtained by asking members of the population of interest if they would participate in your research. An example would be selecting a sample of students from those coming out of the library.
- This is a quick and easy way of choosing participants (advantage)
- It may not provide a representative sample and could be biased (disadvantage).
Systematic Sampling
Systematic sampling is a method where every nth individual is selected from a list or sequence to form a sample, ensuring even and regular intervals between chosen subjects.
Participants are systematically selected (i.e., orderly/logical) from the target population, like every nth participant on a list of names.
To take a systematic sample, you list all the population members and then decide upon a sample you would like. By dividing the number of people in the population by the number of people you want in your sample, you get a number we will call n.
If you take every nth name, you will get a systematic sample of the correct size. If, for example, you wanted to sample 150 children from a school of 1,500, you would take every 10th name.
- The advantage of this method is that it should provide a representative sample.
Sample size
The sample size is a critical factor in determining the reliability and validity of a study’s findings. While increasing the sample size can enhance the generalizability of results, it’s also essential to balance practical considerations, such as resource constraints and diminishing returns from ever-larger samples.
Reliability and Validity
Reliability refers to the consistency and reproducibility of research findings across different occasions, researchers, or instruments. A small sample size may lead to inconsistent results due to increased susceptibility to random error or the influence of outliers. In contrast, a larger sample minimizes these errors, promoting more reliable results.
Validity pertains to the accuracy and truthfulness of research findings. For a study to be valid, it should accurately measure what it intends to do. A small, unrepresentative sample can compromise external validity, meaning the results don’t generalize well to the larger population. A larger sample captures more variability, ensuring that specific subgroups or anomalies don’t overly influence results.
Practical Considerations
Resource Constraints : Larger samples demand more time, money, and resources. Data collection becomes more extensive, data analysis more complex, and logistics more challenging.
Diminishing Returns : While increasing the sample size generally leads to improved accuracy and precision, there’s a point where adding more participants yields only marginal benefits. For instance, going from 50 to 500 participants might significantly boost a study’s robustness, but jumping from 10,000 to 10,500 might not offer a comparable advantage, especially considering the added costs.

- Privacy Policy

Home » Sampling Methods – Types, Techniques and Examples
Sampling Methods – Types, Techniques and Examples
Table of Contents

Sampling refers to the process of selecting a subset of data from a larger population or dataset in order to analyze or make inferences about the whole population.
In other words, sampling involves taking a representative sample of data from a larger group or dataset in order to gain insights or draw conclusions about the entire group.
Sampling Methods
Sampling methods refer to the techniques used to select a subset of individuals or units from a larger population for the purpose of conducting statistical analysis or research.
Sampling is an essential part of the Research because it allows researchers to draw conclusions about a population without having to collect data from every member of that population, which can be time-consuming, expensive, or even impossible.
Types of Sampling Methods
Sampling can be broadly categorized into two main categories:
Probability Sampling
This type of sampling is based on the principles of random selection, and it involves selecting samples in a way that every member of the population has an equal chance of being included in the sample.. Probability sampling is commonly used in scientific research and statistical analysis, as it provides a representative sample that can be generalized to the larger population.
Type of Probability Sampling :
- Simple Random Sampling: In this method, every member of the population has an equal chance of being selected for the sample. This can be done using a random number generator or by drawing names out of a hat, for example.
- Systematic Sampling: In this method, the population is first divided into a list or sequence, and then every nth member is selected for the sample. For example, if every 10th person is selected from a list of 100 people, the sample would include 10 people.
- Stratified Sampling: In this method, the population is divided into subgroups or strata based on certain characteristics, and then a random sample is taken from each stratum. This is often used to ensure that the sample is representative of the population as a whole.
- Cluster Sampling: In this method, the population is divided into clusters or groups, and then a random sample of clusters is selected. Then, all members of the selected clusters are included in the sample.
- Multi-Stage Sampling : This method combines two or more sampling techniques. For example, a researcher may use stratified sampling to select clusters, and then use simple random sampling to select members within each cluster.
Non-probability Sampling
This type of sampling does not rely on random selection, and it involves selecting samples in a way that does not give every member of the population an equal chance of being included in the sample. Non-probability sampling is often used in qualitative research, where the aim is not to generalize findings to a larger population, but to gain an in-depth understanding of a particular phenomenon or group. Non-probability sampling methods can be quicker and more cost-effective than probability sampling methods, but they may also be subject to bias and may not be representative of the larger population.
Types of Non-probability Sampling :
- Convenience Sampling: In this method, participants are chosen based on their availability or willingness to participate. This method is easy and convenient but may not be representative of the population.
- Purposive Sampling: In this method, participants are selected based on specific criteria, such as their expertise or knowledge on a particular topic. This method is often used in qualitative research, but may not be representative of the population.
- Snowball Sampling: In this method, participants are recruited through referrals from other participants. This method is often used when the population is hard to reach, but may not be representative of the population.
- Quota Sampling: In this method, a predetermined number of participants are selected based on specific criteria, such as age or gender. This method is often used in market research, but may not be representative of the population.
- Volunteer Sampling: In this method, participants volunteer to participate in the study. This method is often used in research where participants are motivated by personal interest or altruism, but may not be representative of the population.
Applications of Sampling Methods
Applications of Sampling Methods from different fields:
- Psychology : Sampling methods are used in psychology research to study various aspects of human behavior and mental processes. For example, researchers may use stratified sampling to select a sample of participants that is representative of the population based on factors such as age, gender, and ethnicity. Random sampling may also be used to select participants for experimental studies.
- Sociology : Sampling methods are commonly used in sociological research to study social phenomena and relationships between individuals and groups. For example, researchers may use cluster sampling to select a sample of neighborhoods to study the effects of economic inequality on health outcomes. Stratified sampling may also be used to select a sample of participants that is representative of the population based on factors such as income, education, and occupation.
- Social sciences: Sampling methods are commonly used in social sciences to study human behavior and attitudes. For example, researchers may use stratified sampling to select a sample of participants that is representative of the population based on factors such as age, gender, and income.
- Marketing : Sampling methods are used in marketing research to collect data on consumer preferences, behavior, and attitudes. For example, researchers may use random sampling to select a sample of consumers to participate in a survey about a new product.
- Healthcare : Sampling methods are used in healthcare research to study the prevalence of diseases and risk factors, and to evaluate interventions. For example, researchers may use cluster sampling to select a sample of health clinics to participate in a study of the effectiveness of a new treatment.
- Environmental science: Sampling methods are used in environmental science to collect data on environmental variables such as water quality, air pollution, and soil composition. For example, researchers may use systematic sampling to collect soil samples at regular intervals across a field.
- Education : Sampling methods are used in education research to study student learning and achievement. For example, researchers may use stratified sampling to select a sample of schools that is representative of the population based on factors such as demographics and academic performance.
Examples of Sampling Methods
Probability Sampling Methods Examples:
- Simple random sampling Example : A researcher randomly selects participants from the population using a random number generator or drawing names from a hat.
- Stratified random sampling Example : A researcher divides the population into subgroups (strata) based on a characteristic of interest (e.g. age or income) and then randomly selects participants from each subgroup.
- Systematic sampling Example : A researcher selects participants at regular intervals from a list of the population.
Non-probability Sampling Methods Examples:
- Convenience sampling Example: A researcher selects participants who are conveniently available, such as students in a particular class or visitors to a shopping mall.
- Purposive sampling Example : A researcher selects participants who meet specific criteria, such as individuals who have been diagnosed with a particular medical condition.
- Snowball sampling Example : A researcher selects participants who are referred to them by other participants, such as friends or acquaintances.
How to Conduct Sampling Methods
some general steps to conduct sampling methods:
- Define the population: Identify the population of interest and clearly define its boundaries.
- Choose the sampling method: Select an appropriate sampling method based on the research question, characteristics of the population, and available resources.
- Determine the sample size: Determine the desired sample size based on statistical considerations such as margin of error, confidence level, or power analysis.
- Create a sampling frame: Develop a list of all individuals or elements in the population from which the sample will be drawn. The sampling frame should be comprehensive, accurate, and up-to-date.
- Select the sample: Use the chosen sampling method to select the sample from the sampling frame. The sample should be selected randomly, or if using a non-random method, every effort should be made to minimize bias and ensure that the sample is representative of the population.
- Collect data: Once the sample has been selected, collect data from each member of the sample using appropriate research methods (e.g., surveys, interviews, observations).
- Analyze the data: Analyze the data collected from the sample to draw conclusions about the population of interest.
When to use Sampling Methods
Sampling methods are used in research when it is not feasible or practical to study the entire population of interest. Sampling allows researchers to study a smaller group of individuals, known as a sample, and use the findings from the sample to make inferences about the larger population.
Sampling methods are particularly useful when:
- The population of interest is too large to study in its entirety.
- The cost and time required to study the entire population are prohibitive.
- The population is geographically dispersed or difficult to access.
- The research question requires specialized or hard-to-find individuals.
- The data collected is quantitative and statistical analyses are used to draw conclusions.
Purpose of Sampling Methods
The main purpose of sampling methods in research is to obtain a representative sample of individuals or elements from a larger population of interest, in order to make inferences about the population as a whole. By studying a smaller group of individuals, known as a sample, researchers can gather information about the population that would be difficult or impossible to obtain from studying the entire population.
Sampling methods allow researchers to:
- Study a smaller, more manageable group of individuals, which is typically less time-consuming and less expensive than studying the entire population.
- Reduce the potential for data collection errors and improve the accuracy of the results by minimizing sampling bias.
- Make inferences about the larger population with a certain degree of confidence, using statistical analyses of the data collected from the sample.
- Improve the generalizability and external validity of the findings by ensuring that the sample is representative of the population of interest.
Characteristics of Sampling Methods
Here are some characteristics of sampling methods:
- Randomness : Probability sampling methods are based on random selection, meaning that every member of the population has an equal chance of being selected. This helps to minimize bias and ensure that the sample is representative of the population.
- Representativeness : The goal of sampling is to obtain a sample that is representative of the larger population of interest. This means that the sample should reflect the characteristics of the population in terms of key demographic, behavioral, or other relevant variables.
- Size : The size of the sample should be large enough to provide sufficient statistical power for the research question at hand. The sample size should also be appropriate for the chosen sampling method and the level of precision desired.
- Efficiency : Sampling methods should be efficient in terms of time, cost, and resources required. The method chosen should be feasible given the available resources and time constraints.
- Bias : Sampling methods should aim to minimize bias and ensure that the sample is representative of the population of interest. Bias can be introduced through non-random selection or non-response, and can affect the validity and generalizability of the findings.
- Precision : Sampling methods should be precise in terms of providing estimates of the population parameters of interest. Precision is influenced by sample size, sampling method, and level of variability in the population.
- Validity : The validity of the sampling method is important for ensuring that the results obtained from the sample are accurate and can be generalized to the population of interest. Validity can be affected by sampling method, sample size, and the representativeness of the sample.
Advantages of Sampling Methods
Sampling methods have several advantages, including:
- Cost-Effective : Sampling methods are often much cheaper and less time-consuming than studying an entire population. By studying only a small subset of the population, researchers can gather valuable data without incurring the costs associated with studying the entire population.
- Convenience : Sampling methods are often more convenient than studying an entire population. For example, if a researcher wants to study the eating habits of people in a city, it would be very difficult and time-consuming to study every single person in the city. By using sampling methods, the researcher can obtain data from a smaller subset of people, making the study more feasible.
- Accuracy: When done correctly, sampling methods can be very accurate. By using appropriate sampling techniques, researchers can obtain a sample that is representative of the entire population. This allows them to make accurate generalizations about the population as a whole based on the data collected from the sample.
- Time-Saving: Sampling methods can save a lot of time compared to studying the entire population. By studying a smaller sample, researchers can collect data much more quickly than they could if they studied every single person in the population.
- Less Bias : Sampling methods can reduce bias in a study. If a researcher were to study the entire population, it would be very difficult to eliminate all sources of bias. However, by using appropriate sampling techniques, researchers can reduce bias and obtain a sample that is more representative of the entire population.
Limitations of Sampling Methods
- Sampling Error : Sampling error is the difference between the sample statistic and the population parameter. It is the result of selecting a sample rather than the entire population. The larger the sample, the lower the sampling error. However, no matter how large the sample size, there will always be some degree of sampling error.
- Selection Bias: Selection bias occurs when the sample is not representative of the population. This can happen if the sample is not selected randomly or if some groups are underrepresented in the sample. Selection bias can lead to inaccurate conclusions about the population.
- Non-response Bias : Non-response bias occurs when some members of the sample do not respond to the survey or study. This can result in a biased sample if the non-respondents differ from the respondents in important ways.
- Time and Cost : While sampling can be cost-effective, it can still be expensive and time-consuming to select a sample that is representative of the population. Depending on the sampling method used, it may take a long time to obtain a sample that is large enough and representative enough to be useful.
- Limited Information : Sampling can only provide information about the variables that are measured. It may not provide information about other variables that are relevant to the research question but were not measured.
- Generalization : The extent to which the findings from a sample can be generalized to the population depends on the representativeness of the sample. If the sample is not representative of the population, it may not be possible to generalize the findings to the population as a whole.
About the author
Muhammad Hassan
Researcher, Academic Writer, Web developer
You may also like

Convenience Sampling – Method, Types and Examples

Purposive Sampling – Methods, Types and Examples

Stratified Random Sampling – Definition, Method...

Volunteer Sampling – Definition, Methods and...

Snowball Sampling – Method, Types and Examples

Cluster Sampling – Types, Method and Examples
If you're seeing this message, it means we're having trouble loading external resources on our website.
If you're behind a web filter, please make sure that the domains *.kastatic.org and *.kasandbox.org are unblocked.
To log in and use all the features of Khan Academy, please enable JavaScript in your browser.
Statistics and probability
Course: statistics and probability > unit 6.
- Picking fairly
- Using probability to make fair decisions
- Techniques for generating a simple random sample
- Simple random samples
- Techniques for random sampling and avoiding bias
- Sampling methods
Sampling methods review
- Samples and surveys

What are sampling methods?
Bad ways to sample.
- (Choice A) Convenience sampling A Convenience sampling
- (Choice B) Voluntary response sampling B Voluntary response sampling
Good ways to sample
- (Choice A) Simple random sampling A Simple random sampling
- (Choice B) Stratified random sampling B Stratified random sampling
- (Choice C) Cluster random sampling C Cluster random sampling
- (Choice D) Systematic random sampling D Systematic random sampling
Want to join the conversation?
- Upvote Button navigates to signup page
- Downvote Button navigates to signup page
- Flag Button navigates to signup page

Have a language expert improve your writing
Run a free plagiarism check in 10 minutes, automatically generate references for free.
- Knowledge Base
- Methodology
- Sampling Methods | Types, Techniques, & Examples
Sampling Methods | Types, Techniques, & Examples
Published on 3 May 2022 by Shona McCombes . Revised on 10 October 2022.
When you conduct research about a group of people, it’s rarely possible to collect data from every person in that group. Instead, you select a sample. The sample is the group of individuals who will actually participate in the research.
To draw valid conclusions from your results, you have to carefully decide how you will select a sample that is representative of the group as a whole. There are two types of sampling methods:
- Probability sampling involves random selection, allowing you to make strong statistical inferences about the whole group. It minimises the risk of selection bias .
- Non-probability sampling involves non-random selection based on convenience or other criteria, allowing you to easily collect data.
You should clearly explain how you selected your sample in the methodology section of your paper or thesis.
Table of contents
Population vs sample, probability sampling methods, non-probability sampling methods, frequently asked questions about sampling.
First, you need to understand the difference between a population and a sample , and identify the target population of your research.
- The population is the entire group that you want to draw conclusions about.
- The sample is the specific group of individuals that you will collect data from.
The population can be defined in terms of geographical location, age, income, and many other characteristics.
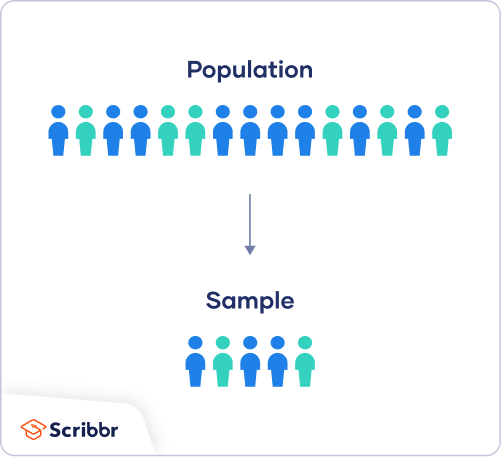
It is important to carefully define your target population according to the purpose and practicalities of your project.
If the population is very large, demographically mixed, and geographically dispersed, it might be difficult to gain access to a representative sample.
Sampling frame
The sampling frame is the actual list of individuals that the sample will be drawn from. Ideally, it should include the entire target population (and nobody who is not part of that population).
You are doing research on working conditions at Company X. Your population is all 1,000 employees of the company. Your sampling frame is the company’s HR database, which lists the names and contact details of every employee.
Sample size
The number of individuals you should include in your sample depends on various factors, including the size and variability of the population and your research design. There are different sample size calculators and formulas depending on what you want to achieve with statistical analysis .
Prevent plagiarism, run a free check.
Probability sampling means that every member of the population has a chance of being selected. It is mainly used in quantitative research . If you want to produce results that are representative of the whole population, probability sampling techniques are the most valid choice.
There are four main types of probability sample.
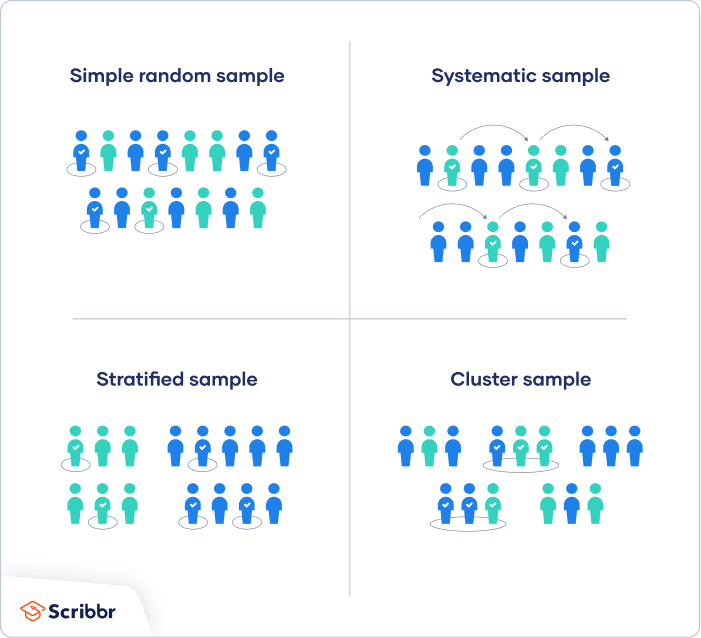
1. Simple random sampling
In a simple random sample , every member of the population has an equal chance of being selected. Your sampling frame should include the whole population.
To conduct this type of sampling, you can use tools like random number generators or other techniques that are based entirely on chance.
You want to select a simple random sample of 100 employees of Company X. You assign a number to every employee in the company database from 1 to 1000, and use a random number generator to select 100 numbers.
2. Systematic sampling
Systematic sampling is similar to simple random sampling, but it is usually slightly easier to conduct. Every member of the population is listed with a number, but instead of randomly generating numbers, individuals are chosen at regular intervals.
All employees of the company are listed in alphabetical order. From the first 10 numbers, you randomly select a starting point: number 6. From number 6 onwards, every 10th person on the list is selected (6, 16, 26, 36, and so on), and you end up with a sample of 100 people.
If you use this technique, it is important to make sure that there is no hidden pattern in the list that might skew the sample. For example, if the HR database groups employees by team, and team members are listed in order of seniority, there is a risk that your interval might skip over people in junior roles, resulting in a sample that is skewed towards senior employees.
3. Stratified sampling
Stratified sampling involves dividing the population into subpopulations that may differ in important ways. It allows you draw more precise conclusions by ensuring that every subgroup is properly represented in the sample.
To use this sampling method, you divide the population into subgroups (called strata) based on the relevant characteristic (e.g., gender, age range, income bracket, job role).
Based on the overall proportions of the population, you calculate how many people should be sampled from each subgroup. Then you use random or systematic sampling to select a sample from each subgroup.
The company has 800 female employees and 200 male employees. You want to ensure that the sample reflects the gender balance of the company, so you sort the population into two strata based on gender. Then you use random sampling on each group, selecting 80 women and 20 men, which gives you a representative sample of 100 people.
4. Cluster sampling
Cluster sampling also involves dividing the population into subgroups, but each subgroup should have similar characteristics to the whole sample. Instead of sampling individuals from each subgroup, you randomly select entire subgroups.
If it is practically possible, you might include every individual from each sampled cluster. If the clusters themselves are large, you can also sample individuals from within each cluster using one of the techniques above. This is called multistage sampling .
This method is good for dealing with large and dispersed populations, but there is more risk of error in the sample, as there could be substantial differences between clusters. It’s difficult to guarantee that the sampled clusters are really representative of the whole population.
The company has offices in 10 cities across the country (all with roughly the same number of employees in similar roles). You don’t have the capacity to travel to every office to collect your data, so you use random sampling to select 3 offices – these are your clusters.
In a non-probability sample , individuals are selected based on non-random criteria, and not every individual has a chance of being included.
This type of sample is easier and cheaper to access, but it has a higher risk of sampling bias . That means the inferences you can make about the population are weaker than with probability samples, and your conclusions may be more limited. If you use a non-probability sample, you should still aim to make it as representative of the population as possible.
Non-probability sampling techniques are often used in exploratory and qualitative research . In these types of research, the aim is not to test a hypothesis about a broad population, but to develop an initial understanding of a small or under-researched population.
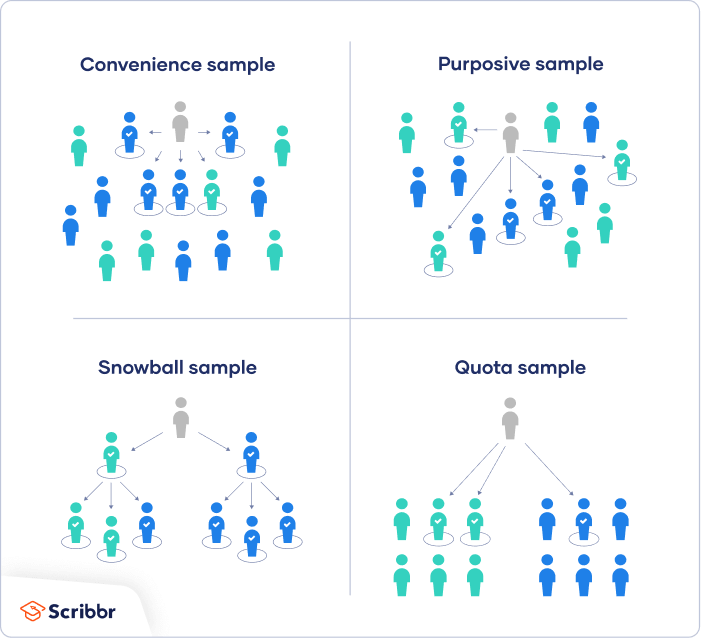
1. Convenience sampling
A convenience sample simply includes the individuals who happen to be most accessible to the researcher.
This is an easy and inexpensive way to gather initial data, but there is no way to tell if the sample is representative of the population, so it can’t produce generalisable results.
You are researching opinions about student support services in your university, so after each of your classes, you ask your fellow students to complete a survey on the topic. This is a convenient way to gather data, but as you only surveyed students taking the same classes as you at the same level, the sample is not representative of all the students at your university.
2. Voluntary response sampling
Similar to a convenience sample, a voluntary response sample is mainly based on ease of access. Instead of the researcher choosing participants and directly contacting them, people volunteer themselves (e.g., by responding to a public online survey).
Voluntary response samples are always at least somewhat biased, as some people will inherently be more likely to volunteer than others.
You send out the survey to all students at your university and many students decide to complete it. This can certainly give you some insight into the topic, but the people who responded are more likely to be those who have strong opinions about the student support services, so you can’t be sure that their opinions are representative of all students.
3. Purposive sampling
Purposive sampling , also known as judgement sampling, involves the researcher using their expertise to select a sample that is most useful to the purposes of the research.
It is often used in qualitative research , where the researcher wants to gain detailed knowledge about a specific phenomenon rather than make statistical inferences, or where the population is very small and specific. An effective purposive sample must have clear criteria and rationale for inclusion.
You want to know more about the opinions and experiences of students with a disability at your university, so you purposely select a number of students with different support needs in order to gather a varied range of data on their experiences with student services.
4. Snowball sampling
If the population is hard to access, snowball sampling can be used to recruit participants via other participants. The number of people you have access to ‘snowballs’ as you get in contact with more people.
You are researching experiences of homelessness in your city. Since there is no list of all homeless people in the city, probability sampling isn’t possible. You meet one person who agrees to participate in the research, and she puts you in contact with other homeless people she knows in the area.
A sample is a subset of individuals from a larger population. Sampling means selecting the group that you will actually collect data from in your research.
For example, if you are researching the opinions of students in your university, you could survey a sample of 100 students.
Statistical sampling allows you to test a hypothesis about the characteristics of a population. There are various sampling methods you can use to ensure that your sample is representative of the population as a whole.
Samples are used to make inferences about populations . Samples are easier to collect data from because they are practical, cost-effective, convenient, and manageable.
Probability sampling means that every member of the target population has a known chance of being included in the sample.
Probability sampling methods include simple random sampling , systematic sampling , stratified sampling , and cluster sampling .
In non-probability sampling , the sample is selected based on non-random criteria, and not every member of the population has a chance of being included.
Common non-probability sampling methods include convenience sampling , voluntary response sampling, purposive sampling , snowball sampling , and quota sampling .
Sampling bias occurs when some members of a population are systematically more likely to be selected in a sample than others.
Cite this Scribbr article
If you want to cite this source, you can copy and paste the citation or click the ‘Cite this Scribbr article’ button to automatically add the citation to our free Reference Generator.
McCombes, S. (2022, October 10). Sampling Methods | Types, Techniques, & Examples. Scribbr. Retrieved 5 August 2024, from https://www.scribbr.co.uk/research-methods/sampling/
Is this article helpful?
Shona McCombes
Other students also liked, what is quantitative research | definition & methods, a quick guide to experimental design | 5 steps & examples, controlled experiments | methods & examples of control.

Sampling Methods & Strategies 101
Everything you need to know (including examples)
By: Derek Jansen (MBA) | Expert Reviewed By: Kerryn Warren (PhD) | January 2023
If you’re new to research, sooner or later you’re bound to wander into the intimidating world of sampling methods and strategies. If you find yourself on this page, chances are you’re feeling a little overwhelmed or confused. Fear not – in this post we’ll unpack sampling in straightforward language , along with loads of examples .
Overview: Sampling Methods & Strategies
- What is sampling in a research context?
- The two overarching approaches
Simple random sampling
Stratified random sampling, cluster sampling, systematic sampling, purposive sampling, convenience sampling, snowball sampling.
- How to choose the right sampling method
What (exactly) is sampling?
At the simplest level, sampling (within a research context) is the process of selecting a subset of participants from a larger group . For example, if your research involved assessing US consumers’ perceptions about a particular brand of laundry detergent, you wouldn’t be able to collect data from every single person that uses laundry detergent (good luck with that!) – but you could potentially collect data from a smaller subset of this group.
In technical terms, the larger group is referred to as the population , and the subset (the group you’ll actually engage with in your research) is called the sample . Put another way, you can look at the population as a full cake and the sample as a single slice of that cake. In an ideal world, you’d want your sample to be perfectly representative of the population, as that would allow you to generalise your findings to the entire population. In other words, you’d want to cut a perfect cross-sectional slice of cake, such that the slice reflects every layer of the cake in perfect proportion.
Achieving a truly representative sample is, unfortunately, a little trickier than slicing a cake, as there are many practical challenges and obstacles to achieving this in a real-world setting. Thankfully though, you don’t always need to have a perfectly representative sample – it all depends on the specific research aims of each study – so don’t stress yourself out about that just yet!
With the concept of sampling broadly defined, let’s look at the different approaches to sampling to get a better understanding of what it all looks like in practice.
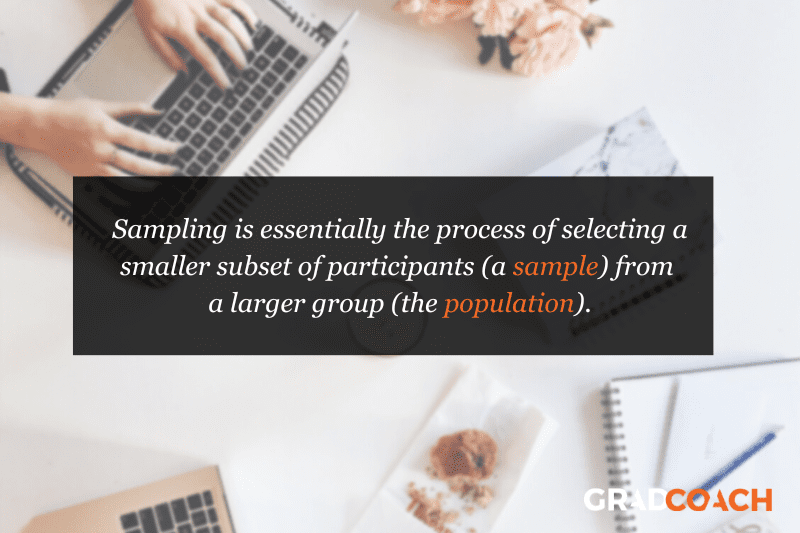
The two overarching sampling approaches
At the highest level, there are two approaches to sampling: probability sampling and non-probability sampling . Within each of these, there are a variety of sampling methods , which we’ll explore a little later.
Probability sampling involves selecting participants (or any unit of interest) on a statistically random basis , which is why it’s also called “random sampling”. In other words, the selection of each individual participant is based on a pre-determined process (not the discretion of the researcher). As a result, this approach achieves a random sample.
Probability-based sampling methods are most commonly used in quantitative research , especially when it’s important to achieve a representative sample that allows the researcher to generalise their findings.
Non-probability sampling , on the other hand, refers to sampling methods in which the selection of participants is not statistically random . In other words, the selection of individual participants is based on the discretion and judgment of the researcher, rather than on a pre-determined process.
Non-probability sampling methods are commonly used in qualitative research , where the richness and depth of the data are more important than the generalisability of the findings.
If that all sounds a little too conceptual and fluffy, don’t worry. Let’s take a look at some actual sampling methods to make it more tangible.
Need a helping hand?
Probability-based sampling methods
First, we’ll look at four common probability-based (random) sampling methods:
Importantly, this is not a comprehensive list of all the probability sampling methods – these are just four of the most common ones. So, if you’re interested in adopting a probability-based sampling approach, be sure to explore all the options.
Simple random sampling involves selecting participants in a completely random fashion , where each participant has an equal chance of being selected. Basically, this sampling method is the equivalent of pulling names out of a hat , except that you can do it digitally. For example, if you had a list of 500 people, you could use a random number generator to draw a list of 50 numbers (each number, reflecting a participant) and then use that dataset as your sample.
Thanks to its simplicity, simple random sampling is easy to implement , and as a consequence, is typically quite cheap and efficient . Given that the selection process is completely random, the results can be generalised fairly reliably. However, this also means it can hide the impact of large subgroups within the data, which can result in minority subgroups having little representation in the results – if any at all. To address this, one needs to take a slightly different approach, which we’ll look at next.
Stratified random sampling is similar to simple random sampling, but it kicks things up a notch. As the name suggests, stratified sampling involves selecting participants randomly , but from within certain pre-defined subgroups (i.e., strata) that share a common trait . For example, you might divide the population into strata based on gender, ethnicity, age range or level of education, and then select randomly from each group.
The benefit of this sampling method is that it gives you more control over the impact of large subgroups (strata) within the population. For example, if a population comprises 80% males and 20% females, you may want to “balance” this skew out by selecting a random sample from an equal number of males and females. This would, of course, reduce the representativeness of the sample, but it would allow you to identify differences between subgroups. So, depending on your research aims, the stratified approach could work well.
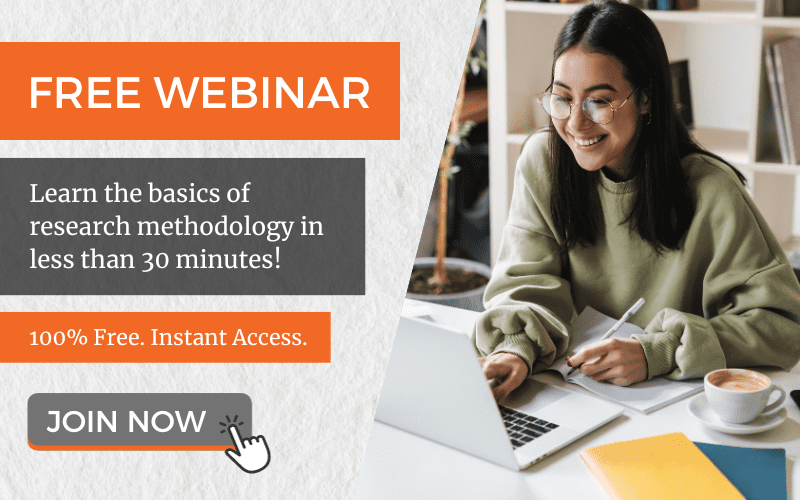
Next on the list is cluster sampling. As the name suggests, this sampling method involves sampling from naturally occurring, mutually exclusive clusters within a population – for example, area codes within a city or cities within a country. Once the clusters are defined, a set of clusters are randomly selected and then a set of participants are randomly selected from each cluster.
Now, you’re probably wondering, “how is cluster sampling different from stratified random sampling?”. Well, let’s look at the previous example where each cluster reflects an area code in a given city.
With cluster sampling, you would collect data from clusters of participants in a handful of area codes (let’s say 5 neighbourhoods). Conversely, with stratified random sampling, you would need to collect data from all over the city (i.e., many more neighbourhoods). You’d still achieve the same sample size either way (let’s say 200 people, for example), but with stratified sampling, you’d need to do a lot more running around, as participants would be scattered across a vast geographic area. As a result, cluster sampling is often the more practical and economical option.
If that all sounds a little mind-bending, you can use the following general rule of thumb. If a population is relatively homogeneous , cluster sampling will often be adequate. Conversely, if a population is quite heterogeneous (i.e., diverse), stratified sampling will generally be more appropriate.
The last probability sampling method we’ll look at is systematic sampling. This method simply involves selecting participants at a set interval , starting from a random point .
For example, if you have a list of students that reflects the population of a university, you could systematically sample that population by selecting participants at an interval of 8 . In other words, you would randomly select a starting point – let’s say student number 40 – followed by student 48, 56, 64, etc.
What’s important with systematic sampling is that the population list you select from needs to be randomly ordered . If there are underlying patterns in the list (for example, if the list is ordered by gender, IQ, age, etc.), this will result in a non-random sample, which would defeat the purpose of adopting this sampling method. Of course, you could safeguard against this by “shuffling” your population list using a random number generator or similar tool.
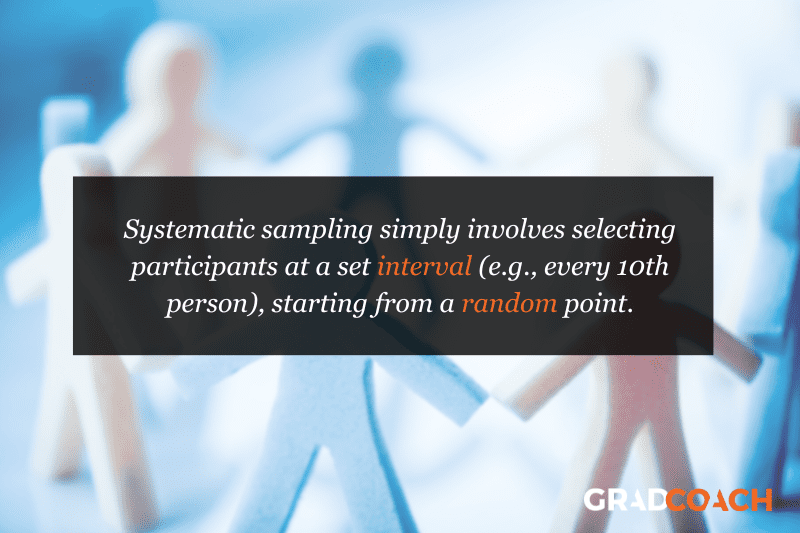
Non-probability-based sampling methods
Right, now that we’ve looked at a few probability-based sampling methods, let’s look at three non-probability methods :
Again, this is not an exhaustive list of all possible sampling methods, so be sure to explore further if you’re interested in adopting a non-probability sampling approach.
First up, we’ve got purposive sampling – also known as judgment , selective or subjective sampling. Again, the name provides some clues, as this method involves the researcher selecting participants using his or her own judgement , based on the purpose of the study (i.e., the research aims).
For example, suppose your research aims were to understand the perceptions of hyper-loyal customers of a particular retail store. In that case, you could use your judgement to engage with frequent shoppers, as well as rare or occasional shoppers, to understand what judgements drive the two behavioural extremes .
Purposive sampling is often used in studies where the aim is to gather information from a small population (especially rare or hard-to-find populations), as it allows the researcher to target specific individuals who have unique knowledge or experience . Naturally, this sampling method is quite prone to researcher bias and judgement error, and it’s unlikely to produce generalisable results, so it’s best suited to studies where the aim is to go deep rather than broad .
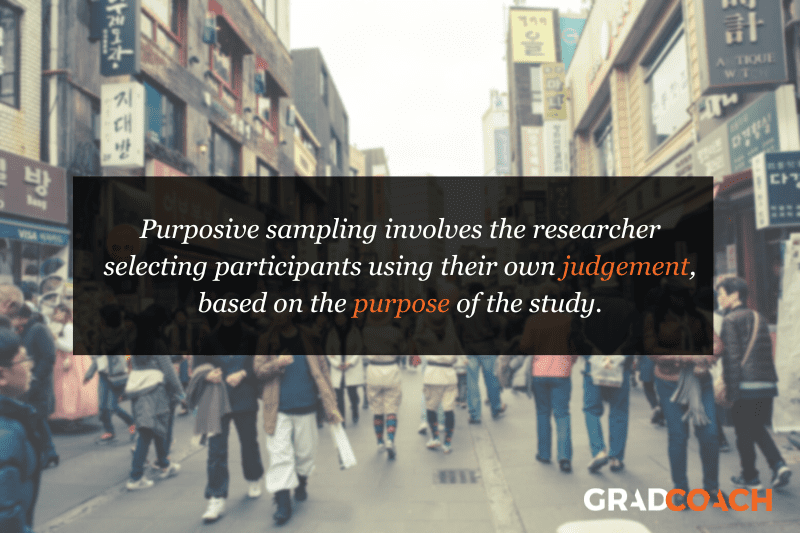
Next up, we have convenience sampling. As the name suggests, with this method, participants are selected based on their availability or accessibility . In other words, the sample is selected based on how convenient it is for the researcher to access it, as opposed to using a defined and objective process.
Naturally, convenience sampling provides a quick and easy way to gather data, as the sample is selected based on the individuals who are readily available or willing to participate. This makes it an attractive option if you’re particularly tight on resources and/or time. However, as you’d expect, this sampling method is unlikely to produce a representative sample and will of course be vulnerable to researcher bias , so it’s important to approach it with caution.
Last but not least, we have the snowball sampling method. This method relies on referrals from initial participants to recruit additional participants. In other words, the initial subjects form the first (small) snowball and each additional subject recruited through referral is added to the snowball, making it larger as it rolls along .
Snowball sampling is often used in research contexts where it’s difficult to identify and access a particular population. For example, people with a rare medical condition or members of an exclusive group. It can also be useful in cases where the research topic is sensitive or taboo and people are unlikely to open up unless they’re referred by someone they trust.
Simply put, snowball sampling is ideal for research that involves reaching hard-to-access populations . But, keep in mind that, once again, it’s a sampling method that’s highly prone to researcher bias and is unlikely to produce a representative sample. So, make sure that it aligns with your research aims and questions before adopting this method.
How to choose a sampling method
Now that we’ve looked at a few popular sampling methods (both probability and non-probability based), the obvious question is, “ how do I choose the right sampling method for my study?”. When selecting a sampling method for your research project, you’ll need to consider two important factors: your research aims and your resources .
As with all research design and methodology choices, your sampling approach needs to be guided by and aligned with your research aims, objectives and research questions – in other words, your golden thread. Specifically, you need to consider whether your research aims are primarily concerned with producing generalisable findings (in which case, you’ll likely opt for a probability-based sampling method) or with achieving rich , deep insights (in which case, a non-probability-based approach could be more practical). Typically, quantitative studies lean toward the former, while qualitative studies aim for the latter, so be sure to consider your broader methodology as well.
The second factor you need to consider is your resources and, more generally, the practical constraints at play. If, for example, you have easy, free access to a large sample at your workplace or university and a healthy budget to help you attract participants, that will open up multiple options in terms of sampling methods. Conversely, if you’re cash-strapped, short on time and don’t have unfettered access to your population of interest, you may be restricted to convenience or referral-based methods.
In short, be ready for trade-offs – you won’t always be able to utilise the “perfect” sampling method for your study, and that’s okay. Much like all the other methodological choices you’ll make as part of your study, you’ll often need to compromise and accept practical trade-offs when it comes to sampling. Don’t let this get you down though – as long as your sampling choice is well explained and justified, and the limitations of your approach are clearly articulated, you’ll be on the right track.
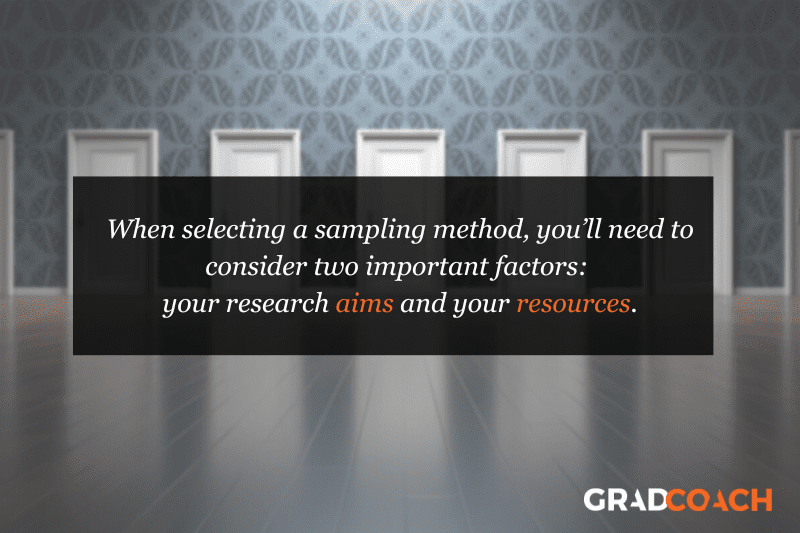
Let’s recap…
In this post, we’ve covered the basics of sampling within the context of a typical research project.
- Sampling refers to the process of defining a subgroup (sample) from the larger group of interest (population).
- The two overarching approaches to sampling are probability sampling (random) and non-probability sampling .
- Common probability-based sampling methods include simple random sampling, stratified random sampling, cluster sampling and systematic sampling.
- Common non-probability-based sampling methods include purposive sampling, convenience sampling and snowball sampling.
- When choosing a sampling method, you need to consider your research aims , objectives and questions, as well as your resources and other practical constraints .
If you’d like to see an example of a sampling strategy in action, be sure to check out our research methodology chapter sample .
Last but not least, if you need hands-on help with your sampling (or any other aspect of your research), take a look at our 1-on-1 coaching service , where we guide you through each step of the research process, at your own pace.
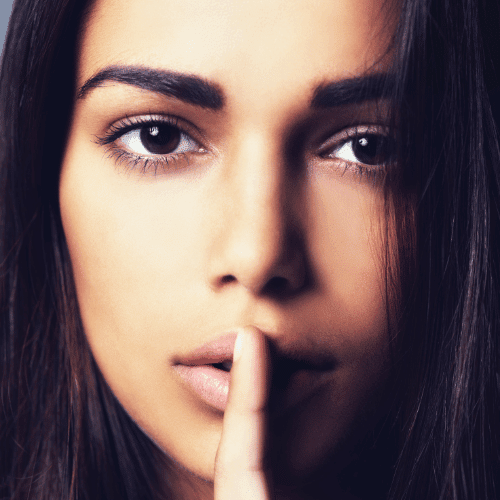
Psst... there’s more!
This post was based on one of our popular Research Bootcamps . If you're working on a research project, you'll definitely want to check this out ...
Excellent and helpful. Best site to get a full understanding of Research methodology. I’m nolonger as “clueless “..😉
Excellent and helpful for junior researcher!
Grad Coach tutorials are excellent – I recommend them to everyone doing research. I will be working with a sample of imprisoned women and now have a much clearer idea concerning sampling. Thank you to all at Grad Coach for generously sharing your expertise with students.
Submit a Comment Cancel reply
Your email address will not be published. Required fields are marked *
Save my name, email, and website in this browser for the next time I comment.
- Print Friendly
- Skip to secondary menu
- Skip to main content
- Skip to primary sidebar
Statistics By Jim
Making statistics intuitive
Sampling Methods: Different Types in Research
By Jim Frost 3 Comments
What Are Sampling Methods?
Sampling methods are the processes by which you draw a sample from a population . When performing research, you’re typically interested in the results for an entire population. Unfortunately, they are almost always too large to study fully. Consequently, researchers use samples to draw conclusions about a population—the process of making statistical inferences.

A population is the complete set of individuals that you’re studying. A sample is the subset of the population that you actually measure, test, or evaluate and base your results. Sampling methods are how you obtain your sample.
Before beginning your study, carefully define the population because your results apply to the target population. You can define your population as narrowly as necessary to meet the needs of your study—for example, adult Swedish women who are otherwise healthy but have osteoporosis. Then choose your sampling method.
Learn more about populations and samples , inferential vs. descriptive statistics and populations and parameters .
In research and inferential statistics , sampling methods are a vital issue. How you draw your sample affects how much you can trust the results! If your sample doesn’t reflect the population, your results might not be valid. It’s a crucial part of experimental design .
In this post, learn more about sampling methods, which ones produce representative samples, and the pros and cons of each procedure.
Probability vs Non-Probability Sampling Methods
Sampling methods have the following two broad categories:
- Probability sampling : Entails random selection and typically, but not always, requires a list of the entire population.
- Non-probability sampling : Does not use random selection but some other process, such as convenience. Usually does not sample from the whole population.
Probability sampling is typically more difficult and costly to implement, but, in exchange, these processes tend to increase validity by producing representative samples. In short, you can make valid conclusions about the population. A statistical inference is when you use a sample to learn about a population. Learn more about Making Statistical Inferences .
On the other hand, non-probability sampling methods are often easier and less expensive, but the trade-off is that the validity of your conclusions is questionable. You might not be able to trust the results. Sampling bias is more likely to occur.
Learn more about Validity in Research and Psychology: Types & Examples and Internal and External Validity .
Probability Sampling Methods
Given the benefits of using representative samples, you’ll typically want to use a probability sampling method whenever possible. Let’s go over the standard methods. They each have pros and cons. Click the links to learn more about each sampling method and see examples. Learn more about representative samples .
To use a probability method, you’ll first need to develop a sampling frame, which lists all members of your target population. Then you can use one of the following methods.
Learn more about Sampling Frames: Definition, Examples & Uses .
Simple Random Sampling (SRS)
In simple random sampling (SRS), researchers take a complete list of the population and randomly select participants from it. All population members have an equal likelihood of being selected. Out of all sampling methods, statisticians consider this one to be the gold standard for producing representative samples. It’s entirely random, leaving little room for accidentally biasing the results.
However, this sampling method has some drawbacks.
First and foremost, this method can be pretty unwieldy and require abundant resources. For one thing, it requires a list of all population members, which can be a tremendous hurdle by itself. Attempting to perform SRS with an incomplete population list causes undercoverage bias and a nonrepresentative sample.
Furthermore, while random selection is beneficial, it also ensures that the subjects are maximally dispersed, making them harder to contact.
SRS can exclude smaller but crucial subpopulations purely by chance. Additionally, this approach produces less precise estimates for subgroups and the differences between subgroups than some other probability sampling methods.
Learn more about Simple Random Sampling and Undercoverage Bias: Definition & Examples
Systematic Sampling
Systematic sampling is similar to SRS but attempts to ease some of the difficulties for researchers. There are several versions of this method.
One form uses a complete list of the population. The researchers randomly select the first subject and then move down the list choosing every X th subject rather than using a randomized technique.
The other form does not use a complete list of the population. This sampling method is suitable for populations that are tough to document, such as the homeless, because a comprehensive list won’t exist. The essential requirement for this sampling method is knowing how to locate them. While it’s not perfect, it’s a feasible option when you can’t obtain the full list.
Suppose you want to survey theater patrons but lack a complete list. Instead, you can use systematic sampling and recruit every 20th person who exits the theater. This approach works because they leave randomly.
This sampling method has some disadvantages. The form that uses a complete list of the population can closely mirror the results of simple random sampling. However, the non-randomness increases the potential for manipulation, even if accidentally. Additionally, patterns in the list can unintentionally create a non-representative sample.
The form that doesn’t use a list has more potential problems. Namely, it increases the potential for missing subgroups and acquiring a non-representative sample. This sampling method increases the knowledge you must have about the population and their habits. Without that knowledge, you won’t be able to find subjects that reflect the whole population.
Learn more about Systematic Sampling .
Stratified Sampling
In stratified sampling, researchers divide a population into similar subpopulations (strata). Then they randomly sample from the strata.
This sampling method can guarantee the presence of small but vital subpopulations in the sample. Relative to SRS, this method can increase the precision of subgroup estimates and the differences between subgroups. In short, it helps researchers gain a better understanding of the subgroups. Dividing the whole population into smaller, more similar subsets can also reduce costs and simplify data collection.
The drawbacks are that this sampling method requires additional upfront knowledge and planning. The researchers must know enough about the subgroups to devise an effective strata scheme. Then they must have sufficient information about all population members to assign them to the correct strata.
Learn more about Stratified Sampling .
Cluster Sampling
Like stratified sampling, the cluster sampling method divides the whole population into smaller groups. However, unlike strata, each cluster mirrors the full diversity present in the population. Then the researchers randomly sample from some of these clusters.
The primary benefit of this sampling method is that it reduces the costs of studying large, geographically dispersed populations. Using this method, researchers don’t need to sample the entire geographic region but only certain areas because they know individual clusters are similar to the population. Additionally, they don’t need to develop a list of potential subjects for clusters from which they’re not sampling. These considerations can significantly reduce planning, administrative, and travel costs.
When researchers can’t create a list of the entire population, cluster sampling can be an excellent choice.
On the downside, cluster sampling increases the design complexity. Researchers must understand how well each cluster approximates the whole population. If the clusters don’t fully represent the population, results can be biased. In real-world studies, clusters tend to be naturally occurring groups that don’t mirror the population, which reduces the ability to draw valid conclusions.
Learn more about Cluster Sampling .
Non-Probability Sampling Methods
Non-probability sampling methods don’t use random selection, and they typically don’t use a complete population list. While these methods are simpler and less expensive, your results are more likely to be biased, reducing your ability to make sound conclusions.
Researchers often use non-probability sampling methods for exploratory research, pilot studies, and qualitative research . These sampling methods provide quick and rough assessments, help work kinks out of measurement instruments and procedures, and help refine the design for a more rigorous study in the future.
Below are several standard non-probability sampling methods:
- Convenience sampling : The main criteria for recruiting subjects are those who are easy to contact and willing to participate. There are no inclusion requirements. Online polls are a type of convenience sampling. Learn more about Convenience Sampling .
- Quota Sampling : Non-random selection of subjects from population subgroups that the researchers define. Learn more about Quota Sampling .
- Purposive sampling : Investigators use subject-area knowledge to handpick a sample they think will help their study. Learn more about Purposive Sampling .
- Snowball sampling : Researchers use subjects to find and recruit other subjects. This method is helpful when a population is hard to contact. When recruits help you find more recruits, and those help find even more, and so on, the total number snowballs. Learn more about Snowball Sampling .
As you can see, there are many sampling methods. Each one has benefits and disadvantages. When designing a study, evaluate the nature of your target population, your research goals, and the available time and resources to choose your sampling method. After deciding between the sampling methods, calculate your sample size using a power analysis .
Sampling in Developmental Science: Situations, Shortcomings, Solutions, and Standards (nih.gov)
Share this:

Reader Interactions
July 24, 2024 at 8:56 am
Hello Mr. Frost,
I would like to know whether people with mild Parkinson’s Disease symptoms are less likely to have kidney stones. Do PwP (People with Parkinson’s) have significantly less incidences of kidney stones than in the general population (~ 10%). So far, I have asked 12 people I know who has been diagnosed with Parkinson’s and 0% had kidney stones. I would like to increase my sampling size by randomly sampling members of a forum for PwP I belong to. Should I get a list of all forum subscribers and randomly select around 40 forum members to pose the question, “If you have been officially diagnosed with Parkinson’s, have also had a kidney stone?”. What would you suggest? I had posed the question in the forum before, but only PwP folks that had a Kidney stone responded.
Thanks, Mike
May 17, 2022 at 12:38 am
I think stratified sampling will work __ mke two groups as stratas _ then use SRS to obtain a complete sample .
May 15, 2022 at 7:37 pm
hi.what sampling technique will i use if my respondents are 1st yr college students awardees vs non awardees of different courses?
Comments and Questions Cancel reply
Root out friction in every digital experience, super-charge conversion rates, and optimize digital self-service
Uncover insights from any interaction, deliver AI-powered agent coaching, and reduce cost to serve
Increase revenue and loyalty with real-time insights and recommendations delivered to teams on the ground
Know how your people feel and empower managers to improve employee engagement, productivity, and retention
Take action in the moments that matter most along the employee journey and drive bottom line growth
Whatever they’re are saying, wherever they’re saying it, know exactly what’s going on with your people
Get faster, richer insights with qual and quant tools that make powerful market research available to everyone
Run concept tests, pricing studies, prototyping + more with fast, powerful studies designed by UX research experts
Track your brand performance 24/7 and act quickly to respond to opportunities and challenges in your market
Explore the platform powering Experience Management
- Free Account
- Product Demos
- For Digital
- For Customer Care
- For Human Resources
- For Researchers
- Financial Services
- All Industries
Popular Use Cases
- Customer Experience
- Employee Experience
- Net Promoter Score
- Voice of Customer
- Customer Success Hub
- Product Documentation
- Training & Certification
- XM Institute
- Popular Resources
- Customer Stories
- Artificial Intelligence
- Market Research
- Partnerships
- Marketplace
The annual gathering of the experience leaders at the world’s iconic brands building breakthrough business results, live in Salt Lake City.
- English/AU & NZ
- Español/Europa
- Español/América Latina
- Português Brasileiro
- REQUEST DEMO
- Experience Management
- Sampling Methods
Try Qualtrics for free
Sampling methods, types & techniques.
15 min read Your comprehensive guide to the different sampling methods available to researchers – and how to know which is right for your research.
Author: Will Webster
What is sampling?
In survey research, sampling is the process of using a subset of a population to represent the whole population. To help illustrate this further, let’s look at data sampling methods with examples below.
Let’s say you wanted to do some research on everyone in North America. To ask every person would be almost impossible. Even if everyone said “yes”, carrying out a survey across different states, in different languages and timezones, and then collecting and processing all the results , would take a long time and be very costly.
Sampling allows large-scale research to be carried out with a more realistic cost and time-frame because it uses a smaller number of individuals in the population with representative characteristics to stand in for the whole.
However, when you decide to sample, you take on a new task. You have to decide who is part of your sample list and how to choose the people who will best represent the whole population. How you go about that is what the practice of sampling is all about.
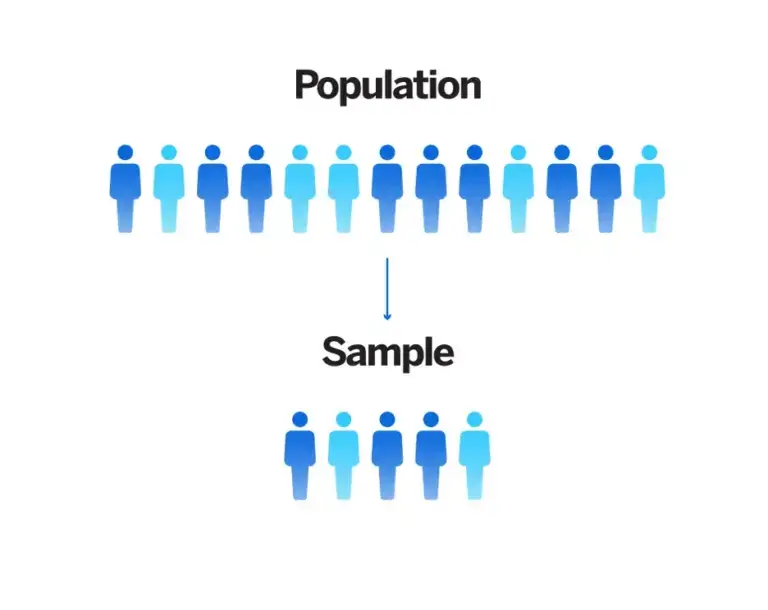
Sampling definitions
- Population: The total number of people or things you are interested in
- Sample: A smaller number within your population that will represent the whole
- Sampling: The process and method of selecting your sample
Free eBook: 2024 Market Research Trends
Why is sampling important?
Although the idea of sampling is easiest to understand when you think about a very large population, it makes sense to use sampling methods in research studies of all types and sizes. After all, if you can reduce the effort and cost of doing a study, why wouldn’t you? And because sampling allows you to research larger target populations using the same resources as you would smaller ones, it dramatically opens up the possibilities for research.
Sampling is a little like having gears on a car or bicycle. Instead of always turning a set of wheels of a specific size and being constrained by their physical properties, it allows you to translate your effort to the wheels via the different gears, so you’re effectively choosing bigger or smaller wheels depending on the terrain you’re on and how much work you’re able to do.
Sampling allows you to “gear” your research so you’re less limited by the constraints of cost, time, and complexity that come with different population sizes.
It allows us to do things like carrying out exit polls during elections, map the spread and effects rates of epidemics across geographical areas, and carry out nationwide census research that provides a snapshot of society and culture.
Types of sampling
Sampling strategies in research vary widely across different disciplines and research areas, and from study to study.
There are two major types of sampling methods: probability and non-probability sampling.
- Probability sampling , also known as random sampling , is a kind of sample selection where randomization is used instead of deliberate choice. Each member of the population has a known, non-zero chance of being selected.
- Non-probability sampling techniques are where the researcher deliberately picks items or individuals for the sample based on non-random factors such as convenience, geographic availability, or costs.
As we delve into these categories, it’s essential to understand the nuances and applications of each method to ensure that the chosen sampling strategy aligns with the research goals.
Probability sampling methods
There’s a wide range of probability sampling methods to explore and consider. Here are some of the best-known options.
1. Simple random sampling
With simple random sampling , every element in the population has an equal chance of being selected as part of the sample. It’s something like picking a name out of a hat. Simple random sampling can be done by anonymizing the population – e.g. by assigning each item or person in the population a number and then picking numbers at random.
Pros: Simple random sampling is easy to do and cheap. Designed to ensure that every member of the population has an equal chance of being selected, it reduces the risk of bias compared to non-random sampling.
Cons: It offers no control for the researcher and may lead to unrepresentative groupings being picked by chance.
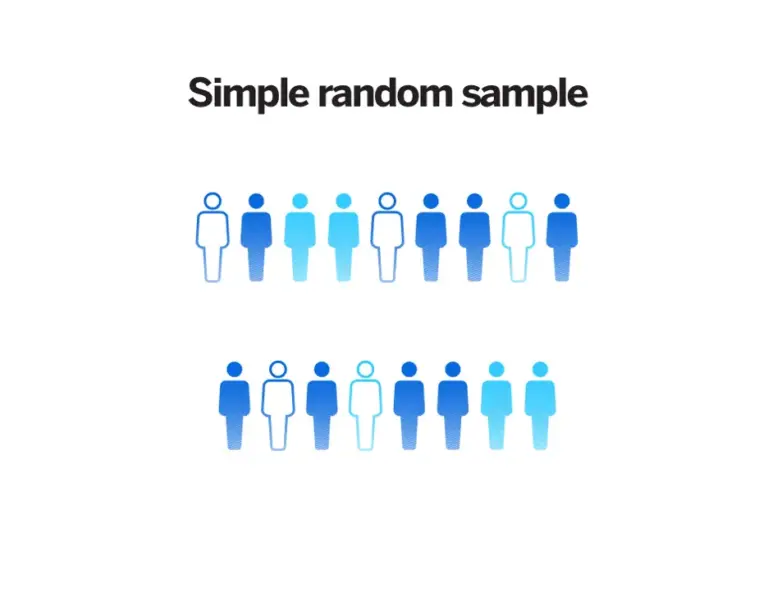
2. Systematic sampling
With systematic sampling the random selection only applies to the first item chosen. A rule then applies so that every nth item or person after that is picked.
Best practice is to sort your list in a random way to ensure that selections won’t be accidentally clustered together. This is commonly achieved using a random number generator. If that’s not available you might order your list alphabetically by first name and then pick every fifth name to eliminate bias, for example.
Next, you need to decide your sampling interval – for example, if your sample will be 10% of your full list, your sampling interval is one in 10 – and pick a random start between one and 10 – for example three. This means you would start with person number three on your list and pick every tenth person.
Pros: Systematic sampling is efficient and straightforward, especially when dealing with populations that have a clear order. It ensures a uniform selection across the population.
Cons: There’s a potential risk of introducing bias if there’s an unrecognized pattern in the population that aligns with the sampling interval.
3. Stratified sampling
Stratified sampling involves random selection within predefined groups. It’s a useful method for researchers wanting to determine what aspects of a sample are highly correlated with what’s being measured. They can then decide how to subdivide (stratify) it in a way that makes sense for the research.
For example, you want to measure the height of students at a college where 80% of students are female and 20% are male. We know that gender is highly correlated with height, and if we took a simple random sample of 200 students (out of the 2,000 who attend the college), we could by chance get 200 females and not one male. This would bias our results and we would underestimate the height of students overall. Instead, we could stratify by gender and make sure that 20% of our sample (40 students) are male and 80% (160 students) are female.
Pros: Stratified sampling enhances the representation of all identified subgroups within a population, leading to more accurate results in heterogeneous populations.
Cons: This method requires accurate knowledge about the population’s stratification, and its design and execution can be more intricate than other methods.
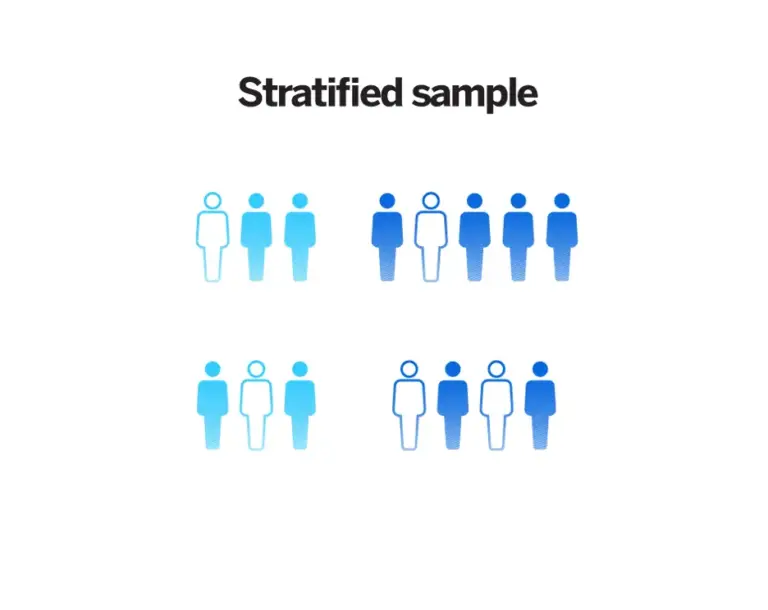
4. Cluster sampling
With cluster sampling, groups rather than individual units of the target population are selected at random for the sample. These might be pre-existing groups, such as people in certain zip codes or students belonging to an academic year.
Cluster sampling can be done by selecting the entire cluster, or in the case of two-stage cluster sampling, by randomly selecting the cluster itself, then selecting at random again within the cluster.
Pros: Cluster sampling is economically beneficial and logistically easier when dealing with vast and geographically dispersed populations.
Cons: Due to potential similarities within clusters, this method can introduce a greater sampling error compared to other methods.
Non-probability sampling methods
The non-probability sampling methodology doesn’t offer the same bias-removal benefits as probability sampling, but there are times when these types of sampling are chosen for expediency or simplicity. Here are some forms of non-probability sampling and how they work.
1. Convenience sampling
People or elements in a sample are selected on the basis of their accessibility and availability. If you are doing a research survey and you work at a university, for example, a convenience sample might consist of students or co-workers who happen to be on campus with open schedules who are willing to take your questionnaire .
This kind of sample can have value, especially if it’s done as an early or preliminary step, but significant bias will be introduced.
Pros: Convenience sampling is the most straightforward method, requiring minimal planning, making it quick to implement.
Cons: Due to its non-random nature, the method is highly susceptible to biases, and the results are often lacking in their application to the real world.
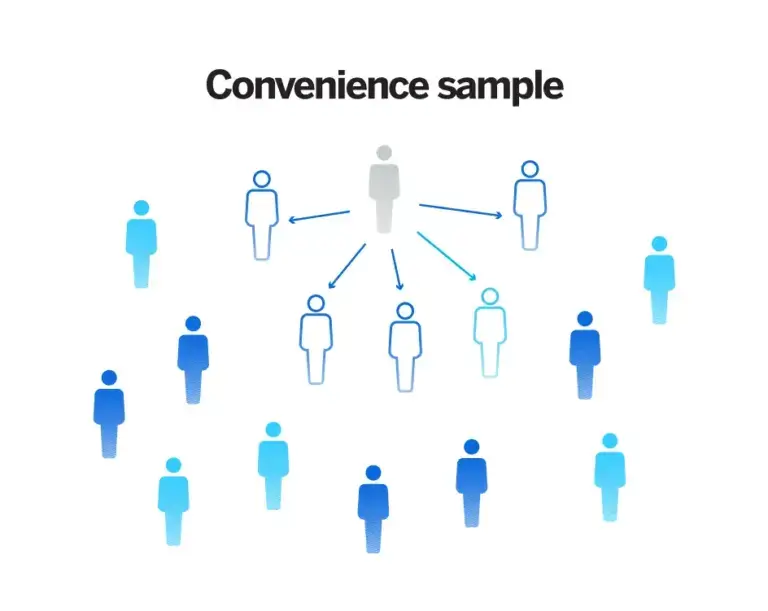
2. Quota sampling
Like the probability-based stratified sampling method, this approach aims to achieve a spread across the target population by specifying who should be recruited for a survey according to certain groups or criteria.
For example, your quota might include a certain number of males and a certain number of females. Alternatively, you might want your samples to be at a specific income level or in certain age brackets or ethnic groups.
Pros: Quota sampling ensures certain subgroups are adequately represented, making it great for when random sampling isn’t feasible but representation is necessary.
Cons: The selection within each quota is non-random and researchers’ discretion can influence the representation, which both strongly increase the risk of bias.
3. Purposive sampling
Participants for the sample are chosen consciously by researchers based on their knowledge and understanding of the research question at hand or their goals.
Also known as judgment sampling, this technique is unlikely to result in a representative sample , but it is a quick and fairly easy way to get a range of results or responses.
Pros: Purposive sampling targets specific criteria or characteristics, making it ideal for studies that require specialized participants or specific conditions.
Cons: It’s highly subjective and based on researchers’ judgment, which can introduce biases and limit the study’s real-world application.

4. Snowball or referral sampling
With this approach, people recruited to be part of a sample are asked to invite those they know to take part, who are then asked to invite their friends and family and so on. The participation radiates through a community of connected individuals like a snowball rolling downhill.
Pros: Especially useful for hard-to-reach or secretive populations, snowball sampling is effective for certain niche studies.
Cons: The method can introduce bias due to the reliance on participant referrals, and the choice of initial seeds can significantly influence the final sample.
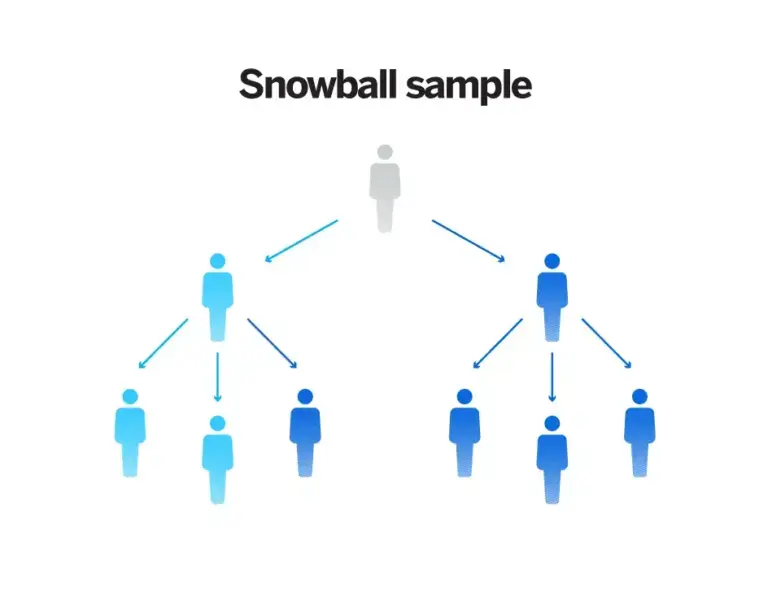
What type of sampling should I use?
Choosing the right sampling method is a pivotal aspect of any research process, but it can be a stumbling block for many.
Here’s a structured approach to guide your decision.
1) Define your research goals
If you aim to get a general sense of a larger group, simple random or stratified sampling could be your best bet. For focused insights or studying unique communities, snowball or purposive sampling might be more suitable.
2) Assess the nature of your population
The nature of the group you’re studying can guide your method. For a diverse group with different categories, stratified sampling can ensure all segments are covered. If they’re widely spread geographically , cluster sampling becomes useful. If they’re arranged in a certain sequence or order, systematic sampling might be effective.
3) Consider your constraints
Your available time, budget and ease of accessing participants matter. Convenience or quota sampling can be practical for quicker studies, but they come with some trade-offs. If reaching everyone in your desired group is challenging, snowball or purposive sampling can be more feasible.
4) Determine the reach of your findings
Decide if you want your findings to represent a much broader group. For a wider representation, methods that include everyone fairly (like probability sampling ) are a good option. For specialized insights into specific groups, non-probability sampling methods can be more suitable.
5) Get feedback
Before fully committing, discuss your chosen method with others in your field and consider a test run.
Avoid or reduce sampling errors and bias
Using a sample is a kind of short-cut. If you could ask every single person in a population to take part in your study and have each of them reply, you’d have a highly accurate (and very labor-intensive) project on your hands.
But since that’s not realistic, sampling offers a “good-enough” solution that sacrifices some accuracy for the sake of practicality and ease. How much accuracy you lose out on depends on how well you control for sampling error, non-sampling error, and bias in your survey design . Our blog post helps you to steer clear of some of these issues.
How to choose the correct sample size
Finding the best sample size for your target population is something you’ll need to do again and again, as it’s different for every study.
To make life easier, we’ve provided a sample size calculator . To use it, you need to know your:
- Population size
- Confidence level
- Margin of error (confidence interval)
If any of those terms are unfamiliar, have a look at our blog post on determining sample size for details of what they mean and how to find them.
Unlock the insights of yesterday to shape tomorrow
In the ever-evolving business landscape, relying on the most recent market research is paramount. Reflecting on 2022, brands and businesses can harness crucial insights to outmaneuver challenges and seize opportunities.
Equip yourself with this knowledge by exploring Qualtrics’ ‘2022 Market Research Global Trends’ report.
Delve into this comprehensive study to unearth:
- How businesses made sense of tricky situations in 2022
- Tips that really helped improve research results
- Steps to take your findings and put them into action
Related resources
How to determine sample size 12 min read, selection bias 11 min read, systematic random sampling 15 min read, convenience sampling 18 min read, probability sampling 8 min read, non-probability sampling 17 min read, stratified random sampling 12 min read, request demo.
Ready to learn more about Qualtrics?
An overview of sampling methods
Last updated
27 February 2023
Reviewed by
Cathy Heath
When researching perceptions or attributes of a product, service, or people, you have two options:
Survey every person in your chosen group (the target market, or population), collate your responses, and reach your conclusions.
Select a smaller group from within your target market and use their answers to represent everyone. This option is sampling .
Sampling saves you time and money. When you use the sampling method, the whole population being studied is called the sampling frame .
The sample you choose should represent your target market, or the sampling frame, well enough to do one of the following:
Generalize your findings across the sampling frame and use them as though you had surveyed everyone
Use the findings to decide on your next step, which might involve more in-depth sampling
Make research less tedious
Dovetail streamlines research to help you uncover and share actionable insights
How was sampling developed?
Valery Glivenko and Francesco Cantelli, two mathematicians studying probability theory in the early 1900s, devised the sampling method. Their research showed that a properly chosen sample of people would reflect the larger group’s status, opinions, decisions, and decision-making steps.
They proved you don't need to survey the entire target market, thereby saving the rest of us a lot of time and money.
- Why is sampling important?
We’ve already touched on the fact that sampling saves you time and money. When you get reliable results quickly, you can act on them sooner. And the money you save can pay for something else.
It’s often easier to survey a sample than a whole population. Sample inferences can be more reliable than those you get from a very large group because you can choose your samples carefully and scientifically.
Sampling is also useful because it is often impossible to survey the entire population. You probably have no choice but to collect only a sample in the first place.
Because you’re working with fewer people, you can collect richer data, which makes your research more accurate. You can:
Ask more questions
Go into more detail
Seek opinions instead of just collecting facts
Observe user behaviors
Double-check your findings if you need to
In short, sampling works! Let's take a look at the most common sampling methods.
- Types of sampling methods
There are two main sampling methods: probability sampling and non-probability sampling. These can be further refined, which we'll cover shortly. You can then decide which approach best suits your research project.
Probability sampling method
Probability sampling is used in quantitative research , so it provides data on the survey topic in terms of numbers. Probability relates to mathematics, hence the name ‘quantitative research’. Subjects are asked questions like:
How many boxes of candy do you buy at one time?
How often do you shop for candy?
How much would you pay for a box of candy?
This method is also called random sampling because everyone in the target market has an equal chance of being chosen for the survey. It is designed to reduce sampling error for the most important variables. You should, therefore, get results that fairly reflect the larger population.
Non-probability sampling method
In this method, not everyone has an equal chance of being part of the sample. It's usually easier (and cheaper) to select people for the sample group. You choose people who are more likely to be involved in or know more about the topic you’re researching.
Non-probability sampling is used for qualitative research. Qualitative data is generated by questions like:
Where do you usually shop for candy (supermarket, gas station, etc.?)
Which candy brand do you usually buy?
Why do you like that brand?
- Probability sampling methods
Here are five ways of doing probability sampling:
Simple random sampling (basic probability sampling)
Systematic sampling
Stratified sampling.
Cluster sampling
Multi-stage sampling
Simple random sampling.
There are three basic steps to simple random sampling:
Choose your sampling frame.
Decide on your sample size. Make sure it is large enough to give you reliable data.
Randomly choose your sample participants.
You could put all their names in a hat, shake the hat to mix the names, and pull out however many names you want in your sample (without looking!)
You could be more scientific by giving each participant a number and then using a random number generator program to choose the numbers.
Instead of choosing names or numbers, you decide beforehand on a selection method. For example, collect all the names in your sampling frame and start at, for example, the fifth person on the list, then choose every fourth name or every tenth name. Alternatively, you could choose everyone whose last name begins with randomly-selected initials, such as A, G, or W.
Choose your system of selecting names, and away you go.
This is a more sophisticated way to choose your sample. You break the sampling frame down into important subgroups or strata . Then, decide how many you want in your sample, and choose an equal number (or a proportionate number) from each subgroup.
For example, you want to survey how many people in a geographic area buy candy, so you compile a list of everyone in that area. You then break that list down into, for example, males and females, then into pre-teens, teenagers, young adults, senior citizens, etc. who are male or female.
So, if there are 1,000 young male adults and 2,000 young female adults in the whole sampling frame, you may want to choose 100 males and 200 females to keep the proportions balanced. You then choose the individual survey participants through the systematic sampling method.
Clustered sampling
This method is used when you want to subdivide a sample into smaller groups or clusters that are geographically or organizationally related.
Let’s say you’re doing quantitative research into candy sales. You could choose your sample participants from urban, suburban, or rural populations. This would give you three geographic clusters from which to select your participants.
This is a more refined way of doing cluster sampling. Let’s say you have your urban cluster, which is your primary sampling unit. You can subdivide this into a secondary sampling unit, say, participants who typically buy their candy in supermarkets. You could then further subdivide this group into your ultimate sampling unit. Finally, you select the actual survey participants from this unit.
- Uses of probability sampling
Probability sampling has three main advantages:
It helps minimizes the likelihood of sampling bias. How you choose your sample determines the quality of your results. Probability sampling gives you an unbiased, randomly selected sample of your target market.
It allows you to create representative samples and subgroups within a sample out of a large or diverse target market.
It lets you use sophisticated statistical methods to select as close to perfect samples as possible.
- Non-probability sampling methods
To recap, with non-probability sampling, you choose people for your sample in a non-random way, so not everyone in your sampling frame has an equal chance of being chosen. Your research findings, therefore, may not be as representative overall as probability sampling, but you may not want them to be.
Sampling bias is not a concern if all potential survey participants share similar traits. For example, you may want to specifically focus on young male adults who spend more than others on candy. In addition, it is usually a cheaper and quicker method because you don't have to work out a complex selection system that represents the entire population in that community.
Researchers do need to be mindful of carefully considering the strengths and limitations of each method before selecting a sampling technique.
Non-probability sampling is best for exploratory research , such as at the beginning of a research project.
There are five main types of non-probability sampling methods:
Convenience sampling
Purposive sampling, voluntary response sampling, snowball sampling, quota sampling.
The strategy of convenience sampling is to choose your sample quickly and efficiently, using the least effort, usually to save money.
Let's say you want to survey the opinions of 100 millennials about a particular topic. You could send out a questionnaire over the social media platforms millennials use. Ask respondents to confirm their birth year at the top of their response sheet and, when you have your 100 responses, begin your analysis. Or you could visit restaurants and bars where millennials spend their evenings and sign people up.
A drawback of convenience sampling is that it may not yield results that apply to a broader population.
This method relies on your judgment to choose the most likely sample to deliver the most useful results. You must know enough about the survey goals and the sampling frame to choose the most appropriate sample respondents.
Your knowledge and experience save you time because you know your ideal sample candidates, so you should get high-quality results.
This method is similar to convenience sampling, but it is based on potential sample members volunteering rather than you looking for people.
You make it known you want to do a survey on a particular topic for a particular reason and wait until enough people volunteer. Then you give them the questionnaire or arrange interviews to ask your questions directly.
Snowball sampling involves asking selected participants to refer others who may qualify for the survey. This method is best used when there is no sampling frame available. It is also useful when the researcher doesn’t know much about the target population.
Let's say you want to research a niche topic that involves people who may be difficult to locate. For our candy example, this could be young males who buy a lot of candy, go rock climbing during the day, and watch adventure movies at night. You ask each participant to name others they know who do the same things, so you can contact them. As you make contact with more people, your sample 'snowballs' until you have all the names you need.
This sampling method involves collecting the specific number of units (quotas) from your predetermined subpopulations. Quota sampling is a way of ensuring that your sample accurately represents the sampling frame.
- Uses of non-probability sampling
You can use non-probability sampling when you:
Want to do a quick test to see if a more detailed and sophisticated survey may be worthwhile
Want to explore an idea to see if it 'has legs'
Launch a pilot study
Do some initial qualitative research
Have little time or money available (half a loaf is better than no bread at all)
Want to see if the initial results will help you justify a longer, more detailed, and more expensive research project
- The main types of sampling bias, and how to avoid them
Sampling bias can fog or limit your research results. This will have an impact when you generalize your results across the whole target market. The two main causes of sampling bias are faulty research design and poor data collection or recording. They can affect probability and non-probability sampling.
Faulty research
If a surveyor chooses participants inappropriately, the results will not reflect the population as a whole.
A famous example is the 1948 presidential race. A telephone survey was conducted to see which candidate had more support. The problem with the research design was that, in 1948, most people with telephones were wealthy, and their opinions were very different from voters as a whole. The research implied Dewey would win, but it was Truman who became president.
Poor data collection or recording
This problem speaks for itself. The survey may be well structured, the sample groups appropriate, the questions clear and easy to understand, and the cluster sizes appropriate. But if surveyors check the wrong boxes when they get an answer or if the entire subgroup results are lost, the survey results will be biased.
How do you minimize bias in sampling?
To get results you can rely on, you must:
Know enough about your target market
Choose one or more sample surveys to cover the whole target market properly
Choose enough people in each sample so your results mirror your target market
Have content validity . This means the content of your questions must be direct and efficiently worded. If it isn’t, the viability of your survey could be questioned. That would also be a waste of time and money, so make the wording of your questions your top focus.
If using probability sampling, make sure your sampling frame includes everyone it should and that your random sampling selection process includes the right proportion of the subgroups
If using non-probability sampling, focus on fairness, equality, and completeness in identifying your samples and subgroups. Then balance those criteria against simple convenience or other relevant factors.
What are the five types of sampling bias?
Self-selection bias. If you mass-mail questionnaires to everyone in the sample, you’re more likely to get results from people with extrovert or activist personalities and not from introverts or pragmatists. So if your convenience sampling focuses on getting your quota responses quickly, it may be skewed.
Non-response bias. Unhappy customers, stressed-out employees, or other sub-groups may not want to cooperate or they may pull out early.
Undercoverage bias. If your survey is done, say, via email or social media platforms, it will miss people without internet access, such as those living in rural areas, the elderly, or lower-income groups.
Survivorship bias. Unsuccessful people are less likely to take part. Another example may be a researcher excluding results that don’t support the overall goal. If the CEO wants to tell the shareholders about a successful product or project at the AGM, some less positive survey results may go “missing” (to take an extreme example.) The result is that your data will reflect an overly optimistic representation of the truth.
Pre-screening bias. If the researcher, whose experience and knowledge are being used to pre-select respondents in a judgmental sampling, focuses more on convenience than judgment, the results may be compromised.
How do you minimize sampling bias?
Focus on the bullet points in the next section and:
Make survey questionnaires as direct, easy, short, and available as possible, so participants are more likely to complete them accurately and send them back
Follow up with the people who have been selected but have not returned their responses
Ignore any pressure that may produce bias
- How do you decide on the type of sampling to use?
Use the ideas you've gleaned from this article to give yourself a platform, then choose the best method to meet your goals while staying within your time and cost limits.
If it isn't obvious which method you should choose, use this strategy:
Clarify your research goals
Clarify how accurate your research results must be to reach your goals
Evaluate your goals against time and budget
List the two or three most obvious sampling methods that will work for you
Confirm the availability of your resources (researchers, computer time, etc.)
Compare each of the possible methods with your goals, accuracy, precision, resource, time, and cost constraints
Make your decision
- The takeaway
Effective market research is the basis of successful marketing, advertising, and future productivity. By selecting the most appropriate sampling methods, you will collect the most useful market data and make the most effective decisions.
Should you be using a customer insights hub?
Do you want to discover previous research faster?
Do you share your research findings with others?
Do you analyze research data?
Start for free today, add your research, and get to key insights faster
Editor’s picks
Last updated: 18 April 2023
Last updated: 27 February 2023
Last updated: 6 February 2023
Last updated: 6 October 2023
Last updated: 5 February 2023
Last updated: 16 April 2023
Last updated: 9 March 2023
Last updated: 12 December 2023
Last updated: 11 March 2024
Last updated: 4 July 2024
Last updated: 6 March 2024
Last updated: 5 March 2024
Last updated: 13 May 2024
Latest articles
Related topics, .css-je19u9{-webkit-align-items:flex-end;-webkit-box-align:flex-end;-ms-flex-align:flex-end;align-items:flex-end;display:-webkit-box;display:-webkit-flex;display:-ms-flexbox;display:flex;-webkit-flex-direction:row;-ms-flex-direction:row;flex-direction:row;-webkit-box-flex-wrap:wrap;-webkit-flex-wrap:wrap;-ms-flex-wrap:wrap;flex-wrap:wrap;-webkit-box-pack:center;-ms-flex-pack:center;-webkit-justify-content:center;justify-content:center;row-gap:0;text-align:center;max-width:671px;}@media (max-width: 1079px){.css-je19u9{max-width:400px;}.css-je19u9>span{white-space:pre;}}@media (max-width: 799px){.css-je19u9{max-width:400px;}.css-je19u9>span{white-space:pre;}} decide what to .css-1kiodld{max-height:56px;display:-webkit-box;display:-webkit-flex;display:-ms-flexbox;display:flex;-webkit-align-items:center;-webkit-box-align:center;-ms-flex-align:center;align-items:center;}@media (max-width: 1079px){.css-1kiodld{display:none;}} build next, decide what to build next, log in or sign up.
Get started for free
- Medical Technology
- snowball sampling
Types of sampling in research
- January 2019
- Journal of the Practice of Cardiovascular Sciences 5(3):157
- CC BY-NC-SA

- All India Institute of Medical Sciences
Abstract and Figures

Discover the world's research
- 25+ million members
- 160+ million publication pages
- 2.3+ billion citations
- Johnson L. Gudaga

- Mlondi Vilakazi

- D J Hlalele
- J Coast Conservat

- Aluwani Nthangeni
- Marcia Lebambo
- Susan Ansibey

- Junfang Huang
- Tianqing Wang
- Siti Sarina Sulaiman

- Evid Base Nurs

- Pamela M Ling
- Y.S. Lincoln
- P Guidelines
- M S Choices
- Joseph F Hair
- J Black Barry
- E Babin Rolph
- M N Saunders
- A Thornhill
- Recruit researchers
- Join for free
- Login Email Tip: Most researchers use their institutional email address as their ResearchGate login Password Forgot password? Keep me logged in Log in or Continue with Google Welcome back! Please log in. Email · Hint Tip: Most researchers use their institutional email address as their ResearchGate login Password Forgot password? Keep me logged in Log in or Continue with Google No account? Sign up

Types of Sampling Methods (With Examples)
Researchers are often interested in answering questions about populations like:
- What is the average height of a certain species of plant?
- What is the average weight of a certain species of bird?
- What percentage of citizens in a certain city support a certain law?
One way to answer these questions is to go around and collect data on every single individual in the population of interest.
However, this is typically too costly and time-consuming which is why researchers instead take a sample of the population and use the data from the sample to draw conclusions about the population as a whole.

There are many different methods researchers can potentially use to obtain individuals to be in a sample. These are known as sampling methods .
In this post we share the most commonly used sampling methods in statistics, including the benefits and drawbacks of the various methods.
Probability Sampling Methods
The first class of sampling methods is known as probability sampling methods because every member in a population has an equal probability of being selected to be in the sample.
Simple random sample

Definition: Every member of a population has an equal chance of being selected to be in the sample. Randomly select members through the use of a random number generator or some means of random selection.
Example: We put the names of every student in a class into a hat and randomly draw out names to get a sample of students.
Benefit: Simple random samples are usually representative of the population we’re interested in since every member has an equal chance of being included in the sample.
Stratified random sample

Definition: Split a population into groups. Randomly select some members from each group to be in the sample.
Example: Split up all students in a school according to their grade – freshman, sophomores, juniors, and seniors. Ask 50 students from each grade to complete a survey about the school lunches.
Benefit: Stratified random samples ensure that members from each group in the population are included in the survey.
Cluster random sample

Definition: Split a population into clusters. Randomly select some of the clusters and include all members from those clusters in the sample.
Example: A company that gives whale watching tours wants to survey its customers. Out of ten tours they give one day, they randomly select four tours and ask every customer about their experience.
Benefit: Cluster random samples get every member from some of the groups, which is useful when each group is reflective of the population as a whole.
Systematic random sample

Definition: Put every member of a population into some order. Choosing a random starting point and select every n th member to be in the sample.
Example: A teacher puts students in alphabetical order according to their last name, randomly chooses a starting point, and picks every 5th student to be in the sample.
Benefit: Systematic random samples are usually representative of the population we’re interested in since every member has an equal chance of being included in the sample.
Non-probability Sampling Methods
Another class of sampling methods is known as non-probability sampling methods because not every member in a population has an equal probability of being selected to be in the sample.
This type of sampling method is sometimes used because it’s much cheaper and more convenient compared to probability sampling methods. It’s often used during exploratory analysis when researchers simply want to gain an initial understanding of a population.
However, the samples that result from these sampling methods cannot be used to draw inferences about the populations they came from because they typically aren’t representative samples.
Convenience sample
Definition: Choose members of a population that are readily available to be included in the sample.
Example: A researcher stands in front of a library during the day and polls people that happen to walk by.
Drawback: Location and time of day will affect the results. More than likely, the sample will suffer from undercoverage bias since certain people (e.g. those who work during the day) will not be represented as much in the sample.
Voluntary response sample
Definition: A researcher puts out a request for volunteers to be included in a study and members of a population voluntarily decide to be included in the sample or not.
Example: A radio host asks listeners to go online and take a survey on his website.
Drawback: People who voluntarily respond will likely have stronger opinions (positive or negative) than the rest of the population, which makes them an unrepresentative sample. Using this sampling method, the sample is likely to suffer from nonresponse bias – certain groups of people are simply less likely to provide responses.
Snowball sample
Definition: Researchers recruit initial subjects to be in a study and then ask those initial subjects to recruit additional subjects to be in the study. Using this approach, the sample size “snowballs” bigger and bigger as each additional subject recruits more subjects.
Example: Researchers are conducting a study of individuals with rare diseases, but it’s difficult to find individuals who actually have the disease. However, if they can find just a few initial individuals to be in the study then they can ask them to recruit further individuals they may know through a private support group or through some other means.
Drawback: Sampling bias is likely to occur. Because initial subjects recruit additional subjects, it’s likely that many of the subjects will share similar traits or characteristics that might be unrepresentative of the larger population under study. Thus, findings from the sample can’t be extrapolated to the population.
Read more about snowballing sampling here .
Purposive sample
Definition: Researchers recruit individuals based on who they think will be most useful based on the purpose of their study.
Example: Researchers want to know about the opinions that individuals in a city have about a potential new rock climbing gym being placed in the city square so they purposely seek out individuals that hang out at other rock climbing gyms around the city.
Drawback: The individuals in the sample are unlikely to be representative of the overall population. Thus, findings from the sample can’t be extrapolated to the population.
Featured Posts

Hey there. My name is Zach Bobbitt. I have a Masters of Science degree in Applied Statistics and I’ve worked on machine learning algorithms for professional businesses in both healthcare and retail. I’m passionate about statistics, machine learning, and data visualization and I created Statology to be a resource for both students and teachers alike. My goal with this site is to help you learn statistics through using simple terms, plenty of real-world examples, and helpful illustrations.
9 Replies to “Types of Sampling Methods (With Examples)”
Thank You for explaining the sampling types with simple easy to understand examples. According to me this feedback falls under voluntary type as you ask to fill forms online which not compulsory ……. hope I understand the concept.
Insightful. Thanks for sharing these notes.
Wow this is amazing it’s really understandable
I am so glad I found your site
Nice topic before reading this I have no idea about the topic but reading it makes me understand the whole concept of it thanks to you for making this topic simple. The words you use and the examples you gave were like very simple to understand for a person. The topic may be explained in another way in difficult manner but you choosed simple words to make understand the difficult topic. Thank you sir for this information.
I really enjoyed the whole course. A great course about sampling.
Simple rendom sampling Stratified random sample Cluster random sample Systematic random sample Non-probability Sampling Methods Convenience sample Voluntary response sample Snowball sample Purposive sample
All the topic explained in a very simple way really amazing Thanks 👍
Compelling statistical work. Good job and congratulations.
Thank you for your support and feedback Ruth! We greatly appreciate it!
irrevocably perfect !
Leave a Reply Cancel reply
Your email address will not be published. Required fields are marked *
Join the Statology Community
Sign up to receive Statology's exclusive study resource: 100 practice problems with step-by-step solutions. Plus, get our latest insights, tutorials, and data analysis tips straight to your inbox!
By subscribing you accept Statology's Privacy Policy.
- Skip to main content
- Skip to primary sidebar
- Skip to footer
- QuestionPro

- Solutions Industries Gaming Automotive Sports and events Education Government Travel & Hospitality Financial Services Healthcare Cannabis Technology Use Case NPS+ Communities Audience Contactless surveys Mobile LivePolls Member Experience GDPR Positive People Science 360 Feedback Surveys
- Resources Blog eBooks Survey Templates Case Studies Training Help center

Home Market Research
Sampling Methods: Guide To All Types with Examples
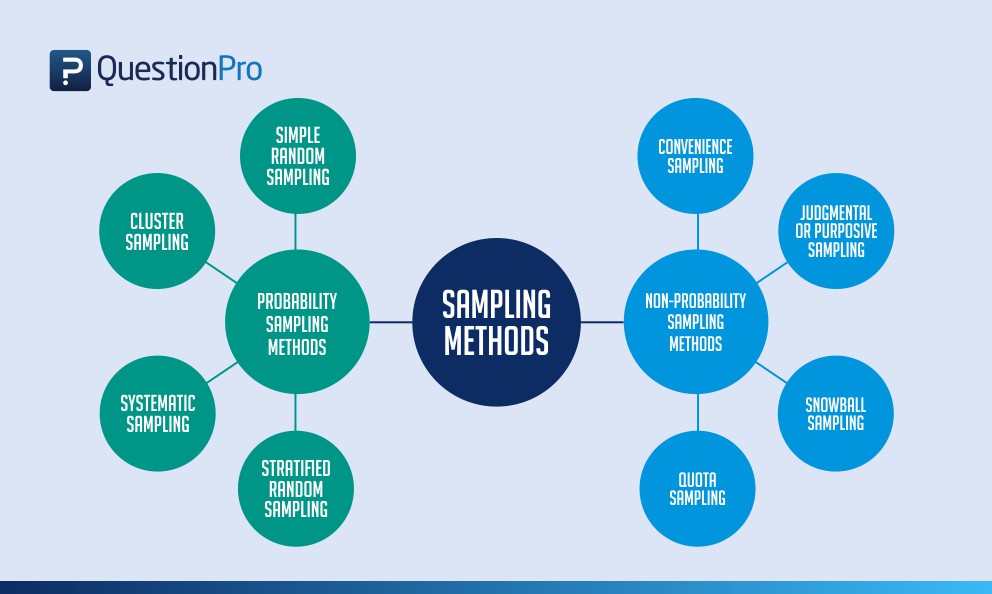
Sampling is an essential part of any research project. The right sampling method can make or break the validity of your research, and it’s essential to choose the right method for your specific question. In this article, we’ll take a closer look at some of the most popular sampling methods and provide real-world examples of how they can be used to gather accurate and reliable data.
LEARN ABOUT: Research Process Steps
From simple random sampling to complex stratified sampling , we’ll explore each method’s pros, cons, and best practices. So, whether you’re a seasoned researcher or just starting your journey, this article is a must-read for anyone looking to master sampling methods. Let’s get started!
Content Index
What is sampling?
Types of sampling: sampling methods, types of probability sampling with examples:, uses of probability sampling, types of non-probability sampling with examples, uses of non-probability sampling, how do you decide on the type of sampling to use, difference between probability sampling and non-probability sampling methods.
Sampling is a technique of selecting individual members or a subset of the population to make statistical inferences from them and estimate the characteristics of the whole population. Different sampling methods are widely used by researchers in market research so that they do not need to research the entire population to collect actionable insights.
It is also a time-convenient and cost-effective method and hence forms the basis of any research design . Sampling techniques can be used in research survey software for optimum derivation.
For example, suppose a drug manufacturer would like to research the adverse side effects of a drug on the country’s population. In that case, it is almost impossible to conduct a research study that involves everyone. In this case, the researcher decides on a sample of people from each demographic and then researches them, giving him/her indicative feedback on the drug’s behavior.
Learn more about Audience by QuestionPro
Sampling in market action research is of two types – probability sampling and non-probability sampling. Let’s take a closer look at these two methods of sampling.
- Probability sampling: Probability sampling is a sampling technique where a researcher selects a few criteria and chooses members of a population randomly. All the members have an equal opportunity to participate in the sample with this selection parameter.
- Non-probability sampling: In non-probability sampling, the researcher randomly chooses members for research. This sampling method is not a fixed or predefined selection process. This makes it difficult for all population elements to have equal opportunities to be included in a sample.
This blog discusses the various probability and non-probability sampling methods you can implement in any market research study.
LEARN ABOUT: Survey Sampling
Probability sampling is a technique in which researchers choose samples from a larger population based on the theory of probability. This sampling method considers every member of the population and forms samples based on a fixed process.
For example, in a population of 1000 members, every member will have a 1/1000 chance of being selected to be a part of a sample. Probability sampling eliminates sampling bias in the population and allows all members to be included in the sample.
There are four types of probability sampling techniques:
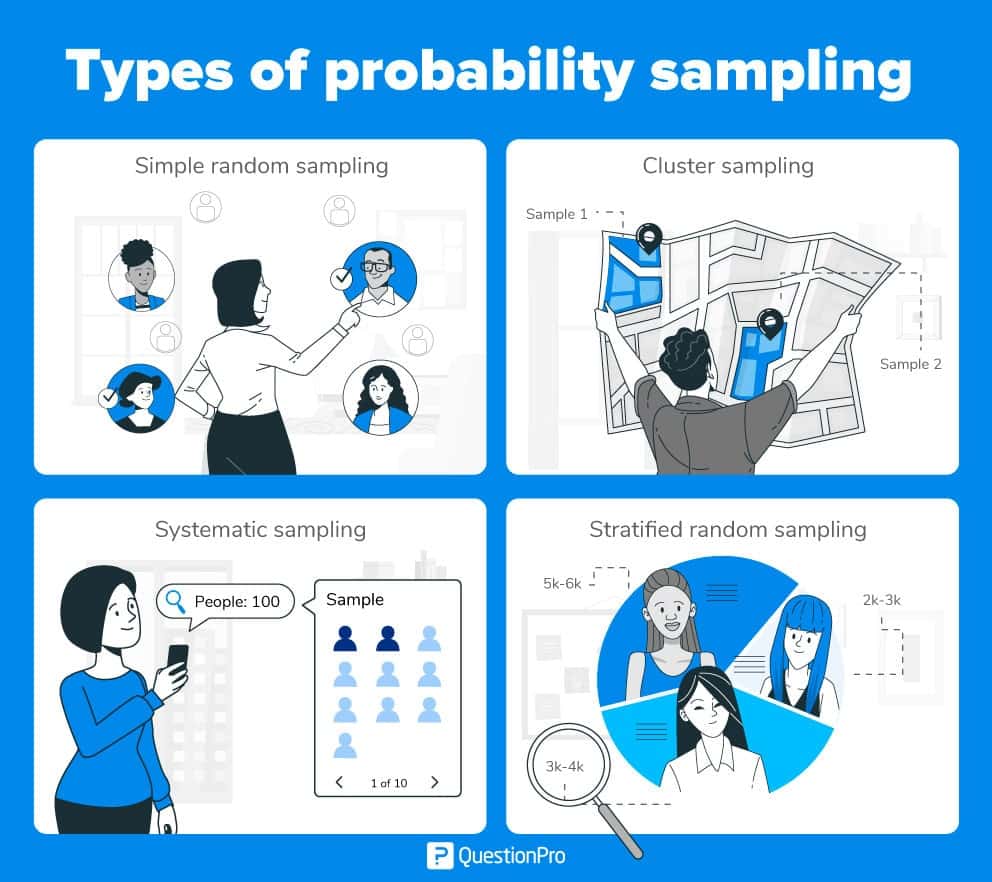
- Simple random sampling: One of the best probability sampling techniques that helps in saving time and resources is the Simple Random Sampling method. It is a reliable method of obtaining information where every single member of a population is chosen randomly, merely by chance. Each individual has the same probability of being chosen to be a part of a sample. For example, in an organization of 500 employees, if the HR team decides on conducting team-building activities, they would likely prefer picking chits out of a bowl. In this case, each of the 500 employees has an equal opportunity of being selected.
- Cluster sampling: Cluster sampling is a method where the researchers divide the entire population into sections or clusters representing a population. Clusters are identified and included in a sample based on demographic parameters like age, sex, location, etc. This makes it very simple for a survey creator to derive effective inferences from the feedback. For example, suppose the United States government wishes to evaluate the number of immigrants living in the Mainland US. In that case, they can divide it into clusters based on states such as California, Texas, Florida, Massachusetts, Colorado, Hawaii, etc. This way of conducting a survey will be more effective as the results will be organized into states and provide insightful immigration data.
- Systematic sampling: Researchers use the systematic sampling method to choose the sample members of a population at regular intervals. It requires selecting a starting point for the sample and sample size determination that can be repeated at regular intervals. This type of sampling method has a predefined range; hence, this sampling technique is the least time-consuming. For example, a researcher intends to collect a systematic sample of 500 people in a population of 5000. He/she numbers each element of the population from 1-5000 and will choose every 10th individual to be a part of the sample (Total population/ Sample Size = 5000/500 = 10).
- Stratified random sampling: Stratified random sampling is a method in which the researcher divides the population into smaller groups that don’t overlap but represent the entire population. While sampling, these groups can be organized, and then draw a sample from each group separately. For example, a researcher looking to analyze the characteristics of people belonging to different annual income divisions will create strata (groups) according to the annual family income. Eg – less than $20,000, $21,000 – $30,000, $31,000 to $40,000, $41,000 to $50,000, etc. By doing this, the researcher concludes the characteristics of people belonging to different income groups. Marketers can analyze which income groups to target and which ones to eliminate to create a roadmap that would bear fruitful results.
LEARN ABOUT: Purposive Sampling
There are multiple uses of probability sampling:
- Reduce Sample Bias: Using the probability sampling method, the research bias in the sample derived from a population is negligible to non-existent. The sample selection mainly depicts the researcher’s understanding and inference. Probability sampling leads to higher-quality data collection as the sample appropriately represents the population.
- Diverse Population: When the population is vast and diverse, it is essential to have adequate representation so that the data is not skewed toward one demographic . For example, suppose Square would like to understand the people that could make their point-of-sale devices. In that case, a survey conducted from a sample of people across the US from different industries and socio-economic backgrounds helps.
- Create an Accurate Sample: Probability sampling helps the researchers plan and create an accurate sample. This helps to obtain well-defined data.
Learn More: Data Collection Methods: Types & Examples
The non-probability method is a sampling method that involves a collection of feedback based on a researcher or statistician’s sample selection capabilities and not on a fixed selection process. In most situations, the output of a survey conducted with a non-probable sample leads to skewed results, which may not represent the desired target population. But, there are situations, such as the preliminary stages of research or cost constraints for conducting research, where non-probability sampling will be much more useful than the other type.
Four types of non-probability sampling explain the purpose of this sampling method in a better manner:
- Convenience sampling: This method depends on the ease of access to subjects such as surveying customers at a mall or passers-by on a busy street. It is usually termed as convenience sampling because of the researcher’s ease of carrying it out and getting in touch with the subjects. Researchers have nearly no authority to select the sample elements, and it’s purely done based on proximity and not representativeness. This non-probability sampling method is used when there are time and cost limitations in collecting feedback. In situations with resource limitations, such as the initial stages of research, convenience sampling is used. For example, startups and NGOs usually conduct convenience sampling at a mall to distribute leaflets of upcoming events or promotion of a cause – they do that by standing at the mall entrance and giving out pamphlets randomly.
- Judgmental or purposive sampling: Judgmental or purposive samples are formed at the researcher’s discretion. Researchers purely consider the purpose of the study, along with the understanding of the target audience. For instance, when researchers want to understand the thought process of people interested in studying for their master’s degree. The selection criteria will be: “Are you interested in doing your masters in …?” and those who respond with a “No” are excluded from the sample.
- Snowball sampling: Snowball sampling is a sampling method that researchers apply when the subjects are difficult to trace. For example, surveying shelterless people or illegal immigrants will be extremely challenging. In such cases, using the snowball theory, researchers can track a few categories to interview and derive results. Researchers also implement this sampling method when the topic is highly sensitive and not openly discussed—for example, surveys to gather information about HIV Aids. Not many victims will readily respond to the questions. Still, researchers can contact people they might know or volunteers associated with the cause to get in touch with the victims and collect information.
- Quota sampling: In Quota sampling , members in this sampling technique selection happens based on a pre-set standard. In this case, as a sample is formed based on specific attributes, the created sample will have the same qualities found in the total population. It is a rapid method of collecting samples.
Non-probability sampling is used for the following:
- Create a hypothesis: Researchers use the non-probability sampling method to create an assumption when limited to no prior information is available. This method helps with the immediate return of data and builds a base for further research.
- Exploratory research: Researchers use this sampling technique widely when conducting qualitative research, pilot studies, or exploratory research .
- Budget and time constraints: The non-probability method when there are budget and time constraints, and some preliminary data must be collected. Since the survey design is not rigid, it is easier to pick respondents randomly and have them take the survey or questionnaire .
For any research, it is essential to choose a sampling method accurately to meet the goals of your study. The effectiveness of your sampling relies on various factors. Here are some steps expert researchers follow to decide the best sampling method.
- Jot down the research goals. Generally, it must be a combination of cost, precision, or accuracy.
- Identify the effective sampling techniques that might potentially achieve the research goals.
- Test each of these methods and examine whether they help achieve your goal.
- Select the method that works best for the research.
Unlock the power of accurate sampling!
We have looked at the different types of sampling methods above and their subtypes. To encapsulate the whole discussion, though, the significant differences between probability sampling methods and non-probability sampling methods are as below:
Probability Sampling is a sampling technique in which samples from a larger population are chosen using a method based on the theory of probability. | Non-probability sampling is a sampling technique in which the researcher selects samples based on the researcher’s subjective judgment rather than random selection. | |
Random sampling method. | Non-random sampling method | |
The population is selected randomly. | The population is selected arbitrarily. | |
The research is conclusive. | The research is exploratory. | |
Since there is a method for deciding the sample, the population demographics are conclusively represented. | Since the sampling method is arbitrary, the population demographics representation is almost always skewed. | |
Takes longer to conduct since the research design defines the selection parameters before the market research study begins. | This type of sampling method is quick since neither the sample nor the selection criteria of the sample are undefined. | |
This type of sampling is entirely unbiased; hence, the results are also conclusive. | This type of sampling is entirely biased, and hence the results are biased, too, rendering the research speculative. | |
In probability sampling, there is an underlying hypothesis before the study begins, and this method aims to prove the hypothesis. | In non-probability sampling, the hypothesis is derived after conducting the research study. |
Now that we have learned how different sampling methods work and are widely used by researchers in market research so that they don’t need to research the entire population to collect actionable insights, let’s go over a tool that can help you manage these insights.
LEARN ABOUT: 12 Best Tools for Researchers
QuestionPro understands the need for an accurate, timely, and cost-effective method to select the proper sample; that’s why we bring QuestionPro Software, a set of tools that allow you to efficiently select your target audience , manage your insights in an organized, customizable repository and community management for post-survey feedback.
Don’t miss the chance to elevate the value of research.
LEARN MORE FREE TRIAL
MORE LIKE THIS
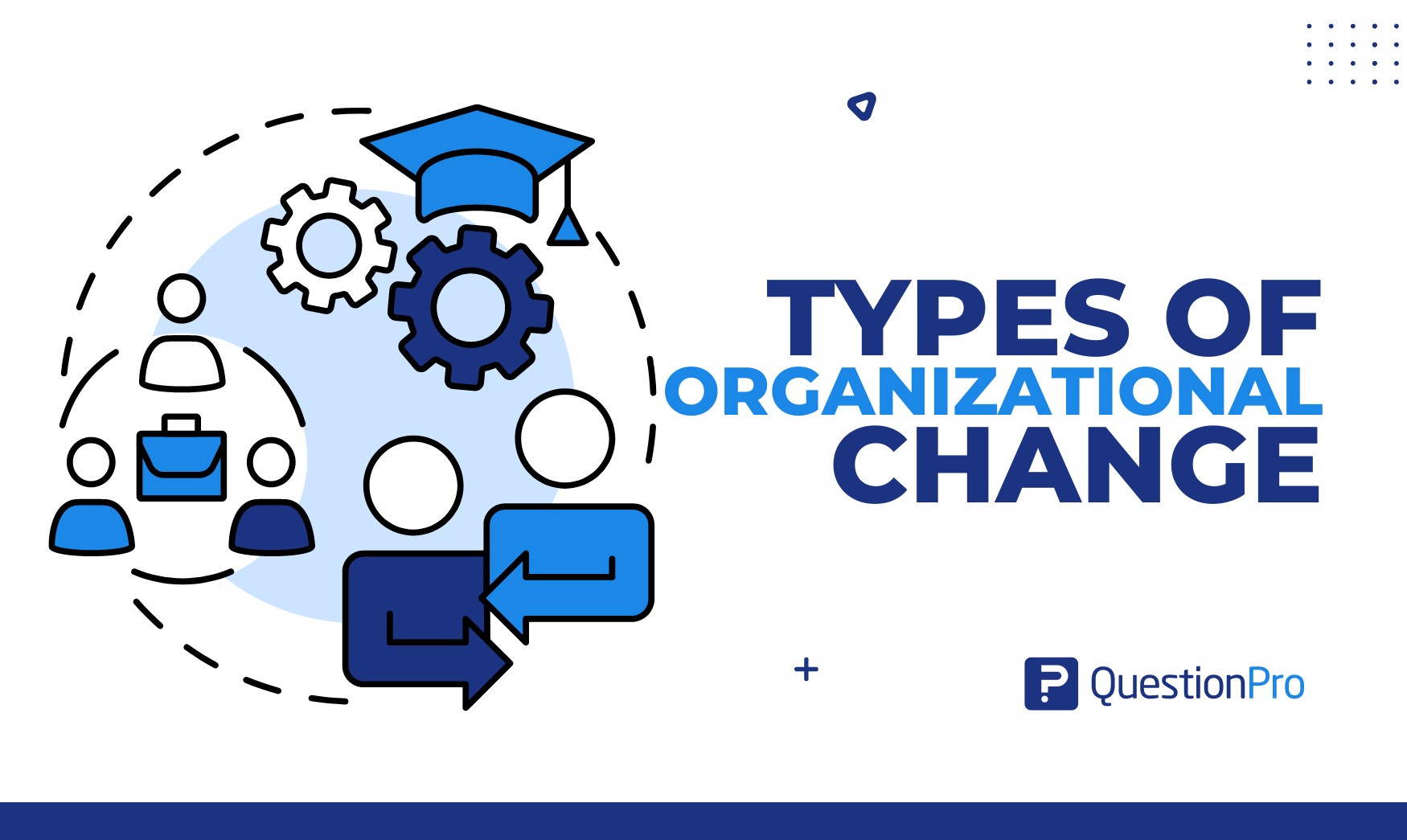
Types of Organizational Change & Strategies for Business
Aug 2, 2024
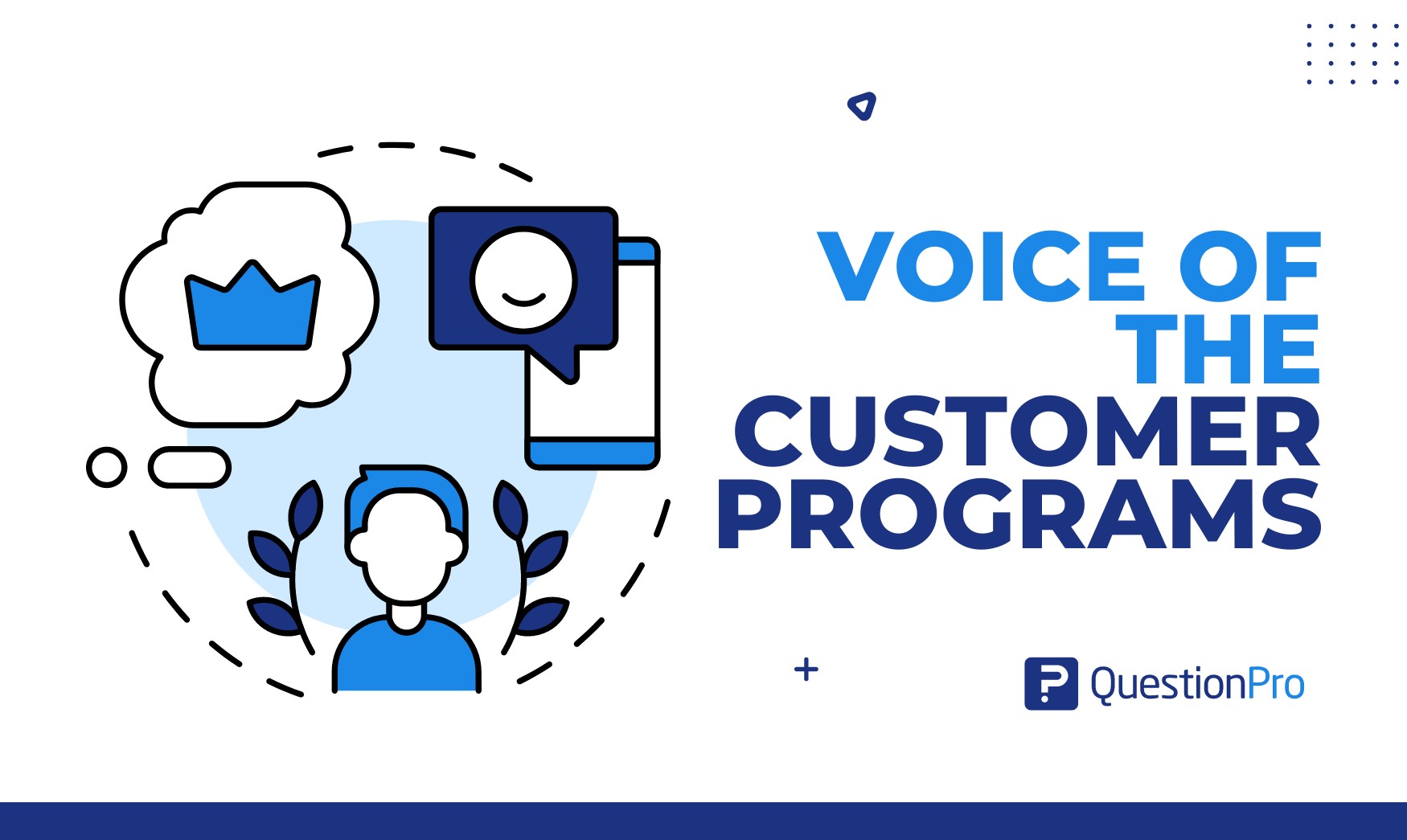
Voice of the Customer Programs: What It Is, Implementations
Aug 1, 2024
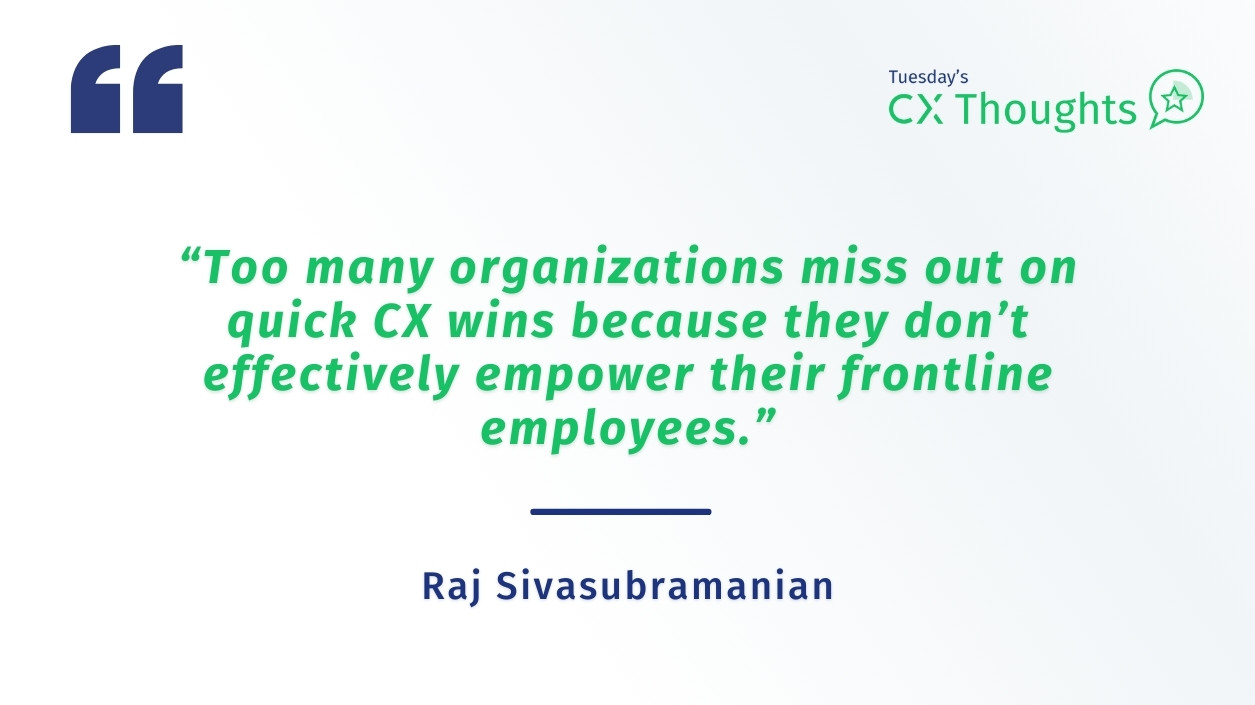
A Case for Empowerment and Being Bold — Tuesday CX Thoughts
Jul 30, 2024
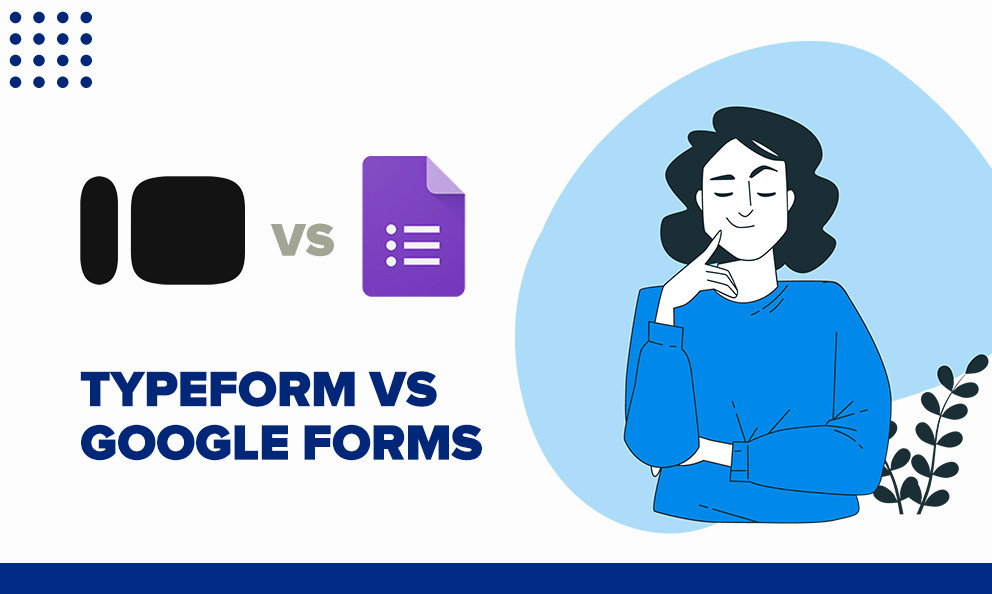
Typeform vs. Google Forms: Which one is best for my needs?
Other categories.
- Academic Research
- Artificial Intelligence
- Assessments
- Brand Awareness
- Case Studies
- Communities
- Consumer Insights
- Customer effort score
- Customer Engagement
- Customer Experience
- Customer Loyalty
- Customer Research
- Customer Satisfaction
- Employee Benefits
- Employee Engagement
- Employee Retention
- Friday Five
- General Data Protection Regulation
- Insights Hub
- Life@QuestionPro
- Market Research
- Mobile diaries
- Mobile Surveys
- New Features
- Online Communities
- Question Types
- Questionnaire
- QuestionPro Products
- Release Notes
- Research Tools and Apps
- Revenue at Risk
- Survey Templates
- Training Tips
- Tuesday CX Thoughts (TCXT)
- Uncategorized
- What’s Coming Up
- Workforce Intelligence
- Technical Support
- Technical Papers
- Knowledge Base
- Question Library
Call our friendly, no-pressure support team.
Sampling Methods in Survey Research: Definition, Types, Methods, and More
Table of Contents
What Is Sampling? Why Is Sampling Important?
Sampling is the backbone for primary data collection and analysis in marketing research and the social sciences. It involves selecting a subset of individuals from a larger population to participate in a study, with the aim of making valid inferences about the entire population without needing to survey every individual within that population. Sampling makes research both practical (cost and time effective) and feasible.
The key to effective sampling lies in the concept of a representative sample —a subset that fairly mirrors the diverse characteristics of the population it is drawn from. Achieving this ensures the data collection and data analysis phases yield accurate and meaningful insights, minimizing the risk of biased (systematically wrong) or irrelevant findings.
Get Started with Your Survey Research Today!
Ready for your next research study? Get access to our free survey research tool. In just a few minutes, you can create powerful surveys with our easy-to-use interface.
Start Survey Research for Free or Request a Product Tour
“Dewey Beats Truman” USA Presidential Election 1948 Example
The classic example showing how poor sampling procedures can lead to erroneous conclusions about the population is had in the oft-cited “Dewey Beats Truman” false headline newspaper article on November 3, 1948, the day after Truman had actually beaten Dewey to win the presidency of the United States of America.
How did the newspaper get it wrong? Conventional wisdom, based on various polls with large sample sizes, predicted that Dewey would win. Facing the printing deadline and anxious to be able to report the winner of the election the next morning, they decided to print the expected results prior to the election results being finalized. “Dewey Defeats Truman” the headline trumpeted; but they had gotten it wrong.
(President Harry Truman gleefully showing the erroneous headline) Image Credit: Byron Rollins, The Washington Post
There were multiple reasons that the polls didn’t get it right. Prominent among those was that telephone polls in 1948 led to a biased sample of respondents who tended to favor Dewey, because telephones were something more commonly found in well-to-do households. Well-to-do households were more likely to vote for Dewey. The sample used to project that Dewey would beat Truman, though substantial, was nonetheless biased.
Key Sampling Definitions: Population vs. Sample
Understanding the distinction between a population and sample is crucial in sampling:
- Sampling : The process of selecting a sample from a population.
- Population : The entire group of individuals or entities relevant to a particular research study, from which a sample is drawn.
- Sample : A subset of the population selected for participation in the research study.
- Sample Frame : A list or database from which the sample is drawn, ideally encompassing the entire population.
These foundational concepts lay the groundwork for understanding the various sampling methods and their applications in research, guiding the choice of method based on the research objectives and constraints.
Types of Sampling Methods in Research
Sampling methods are broadly categorized into probability and non-probability sampling , each with distinct approaches and implications for research accuracy and validity.
Probability Sampling Method
Probability sampling , the first of two primary sampling methods, ensures every member of the population has a known and non-zero chance of being selected. This method fosters objectivity and minimizes sampling bias, enhancing the representativeness of the sample.
1. Simple Random Sampling (SRS) Method
Simple random sampling (SRS) epitomizes equal chance selection, where each population member has an identical probability of being chosen. Its advantages include simplicity and reduced bias, while disadvantages may involve logistical challenges in large populations. Theory-based classroom examples involve drawing balls of different colors out of a cylinder where the balls have been thoroughly mixed. A real-world application is selecting respondents for a customer satisfaction survey from a database of all customers.
2. Systematic Sampling Method
Systemic sampling selection is made at regular intervals from an ordered list, combining efficiency with a reduced risk of selection bias. However, its systematic nature can introduce bias if the list has an underlying pattern. An application example is selecting every 100th theme park visitor coming through the turnstiles to provide feedback.
3. Stratified Sampling Method
Stratified sampling involves dividing the population into subgroups based on identifiable characteristics and randomly sampling from each. It enhances representation but is more complex to implement. A practical use case is conducting a health survey across different age groups to ensure all are adequately represented.
Stratified sampling reduces the likelihood of obtaining an unlucky sample that is not representative. For example, if we sampled 30 respondents, it’s possible you’ll obtain only a very few male respondents. If we divided the sample frame into males and females and drew 15 females and 15 males, we could ensure good representation with respect to gender. In practice, stratified sampling typically involves consideration of more than one demographic (or otherwise known) characteristic of the sample elements.
4. Cluster Sampling Method
Cluster sampling selection occurs by dividing the population into clusters and randomly selecting entire clusters. This approach is cost-effective, especially for geographically dispersed populations, but can increase sampling error. It's sometimes used in field surveys where researchers select specific neighborhoods or schools to study.
Non-Probability Sampling Method
Non-probability sampling, the second primary sampling method, does not provide every individual with a known and non-zero chance of selection, often used when probability sampling is impractical or unnecessary.
1. Convenience Sampling Method
Convenience sampling selection is based on ease of access, favoring quick and easy data collection despite inherent bias risks. Examples related to survey research involve placing a link to take a survey on social media, or using Mechanical Turk (Amazon’s crowdsourcing marketplace) where people go to do a variety of tasks, such as completing surveys, to make money.
Although we would like to think otherwise, many sample sources we rely on for marketing and social survey research purposes, including online panel samples, are in reality convenience samples.
2. Quota Sampling Method
Quota sampling involves selecting individuals to fill a "quota" such as 500 smokers in a non-random manner, such as through convenience sampling approaches.
3. Judgement Sampling Method
In judgement sampling, researchers select participants based on specific criteria and judgment, useful for targeted studies but susceptible to subjective bias. For example, selecting experts in a field for in-depth interviews.
4. Snowball Sampling Method
For hard-to-reach populations, snowball sampling relies on referrals from initial subjects to recruit further participants. While it can be effective for niche studies, it can lead to bias. A case in point is researching a rare medical condition where patients might know and might be able to refer other patients. (Snowball sampling is rarely used in practice.)
Each sampling method has its context of application, influenced by the study's objectives, the population's nature, and logistical considerations. Choosing the appropriate method is important for research validity and reliability.
Get Started with Market Research Today!
Ready for your next market research study? Get access to our free survey research tool. In just a few minutes, you can create powerful surveys with our easy-to-use interface.
Start Market Research for Free or Request a Product Tour
Differences Between Probability and Non-Probability Sampling Methods
Each sampling method has its own set of advantages and limitations, influencing the accuracy, applicability, and generalizability of research findings. Here's a summary exploration of the differences:
|
|
|
| Random selection, every member has a known and non-zero chance of being selected. | Non-random selection, not all members have a known and non-zero chance of being selected. |
| Simple random sampling, systematic sampling, stratified sampling, cluster sampling. | Convenience sampling, quota sampling, judgement sampling, snowball sampling. |
| Generally results in a representative sample. | Usually does not produce a representative sample. |
| Appropriate for inferential statistics and studies requiring generalization. | Used in exploratory research, qualitative studies, or specific subgroup focuses. |
| Reduces sampling bias, allows calculation of sampling error, more reliable. | Easier and less expensive to implement, useful for inaccessible populations. |
| More time-consuming and costly, requires comprehensive population list. | May introduce bias, limits generalization of findings to the entire population. |
Choosing the Right Sampling Method in Research
Selecting the appropriate sampling method can significantly affect the validity of your research findings. This choice should be guided by a structured decision-making process, taking into account several key considerations:
- Research Goals : Begin by clarifying whether your study seeks to gain general insights applicable to the broader population or if it focuses on specific segments or behaviors. This determination will influence whether a probability or non-probability sampling method is more appropriate.
- Nature of the Population : Consider the diversity, geographic distribution, and accessibility of your target population. Probability sampling methods are preferable for a diverse and widespread population to ensure representativeness, while non-probability methods might suffice for more homogeneous or accessible groups.
- Constraints : Practical constraints such as time, budget, and resources available for your study can significantly affect your sampling strategy. Non-probability methods often require less time and resources, making them suitable for studies with tight constraints.
- Reach of Findings : Decide on the importance of the study’s findings being representative of the broader population. Studies aiming for broad applicability should lean towards probability methods to ensure a representative sample.
- Feedback : Engaging with peers or experts in your field can provide valuable insights and feedback on your chosen sampling method. Pilot tests or preliminary studies can also help validate your approach before full-scale implementation.
When interviewing human subjects for market or social survey research, we can do our best to try to follow sound sampling procedures, but non-response bias (when the people who don’t complete the survey systematically differ from those that do) can still be a significant problem, leading to incorrect inferences about the population.
Sampling Methods Theory vs. Practice
This article mainly focuses on the theory of sampling. The theory and science of sampling leads to well-established formulas that lead to probability-based inferences we can make about the population (such as a mean with its accompanying confidence interval). But, in the practice of marketing research and social sciences, we’re dealing with humans, rather than colored balls being drawn from buckets or samples of widgets being pulled off the line at a factory.
Humans self-select themselves out of the sample, by refusing to complete surveys or by having filters on their email servers that block our emailed invitations to complete a survey. The formulas we rely on typically assume simple random sampling (SRS), whereas we rarely ever achieve SRS when conducting survey research with human respondents. To the degree that our samples are biased, the measurements and confidence intervals we obtain in surveys are also systematically incorrect. No amount of sample size (short of interviewing every human in the population) can erase the negative effects of biased sampling procedures.
Avoiding Sampling Errors and Bias
The integrity of research findings hinges on the ability to control and minimize sampling errors and biases . Sampling error refers to the natural variation that occurs by chance because a sample, rather than the entire population, is surveyed. Non-sampling error, on the other hand, encompasses all other errors in the research process, from data collection to analysis. Bias, a systematic error, can significantly alter the results (e.g., too high, too low, not variable enough, or too variable), leading to inaccurate conclusions.
Sampling Error and Bias Mitigation Strategies
- Careful Design and Planning : Developing a robust sampling plan that considers the objectives and scope of the study can help in selecting the most suitable sampling method to minimize errors and bias.
- Randomization : Employing random selection methods where possible to ensure each population member has an equal chance of being included, thereby reducing selection bias.
- Stratification : Using stratified sampling to ensure that important subgroups within the population are adequately represented can minimize sampling bias related to specific characteristics.
By proactively addressing these areas, researchers can enhance the reliability and validity of their study outcomes, ensuring that conclusions drawn are reflective of the true population characteristics.
A Note on Online Panel Providers
Whereas phone and snail mail surveys were popular in the 1940s through the 1980s for sampling from the general population, online panel providers are the most commonly used approach today. Although panel providers may report that their samples are balanced to represent the demographic characteristics of the population as a whole, the samples are not unbiased. The kinds of people who participate in panels and self-select to take surveys may be systematically different on the characteristics that are the subject of your study than those who do not participate in your survey.
Need Sample for Your Research?
Let us connect you with your ideal audience! Reach out to us to request sample for your survey research.
Request Sample
Figuring Out Sample Size
Figuring out (determining) the appropriate sample size is crucial for ensuring that your study results are statistically significant and representative of the population. An inadequately small sample size may lead to unreliable results, while an unnecessarily large sample can waste resources and time.
Factors to consider when determining sample size include:
- Population Size : Larger populations may require a larger sample to accurately reflect the population's characteristics, although the relationship is not linear.
- Confidence Level : This reflects how sure you can be that the population’s true mean falls within a certain range. 95% confidence is a common threshold. A higher confidence level requires a larger sample size.
- Margin of Error : Also known as the confidence interval, it indicates the range within which the true value is likely to lie (given a specified degree of confidence, such as 95%). A smaller margin of error requires a larger sample size.
- Power: The ability to detect true differences or relationships in statistics/variables if they in actuality exist.
Combining the elements of confidence level and margin of error, it’s common to read that a poll found that 30% of respondents said they would vote for candidate A with a margin of error of +/- 3% at the 95% confidence level. (If it isn’t explicitly stated, it is generally assumed that the research organization has assumed a 95% confidence level when calculating the margin of error.)
Several sample size calculators and formulas are available to assist researchers in selecting the sample size for determining margins of error at given confidence levels. Consulting with statistical experts or utilizing specialized software can provide additional accuracy and confidence in these calculations, especially regarding power .
False Positives (Type 1 error) vs. False Negatives (Type 2 error)
Most common sample size calculators used for survey research focus on achieving reasonably good precision, meaning tight confidence intervals (such as the common +/-3% confidence interval for proportions at 95% confidence).
Most researchers applying tests for significance (of effects or parameters) want a likelihood of 5% or lower of signaling that a difference or impact (effect) is non-zero when in reality it is not. This is called a False Positive, or a Type 1 error, and 5% “alpha” threshold for Type 1 errors is typical.
Most researchers don’t think about the danger of False Negatives (Type 2 error) when calculating needed sample size. Setting the likelihood of Type 1 errors at 5% means that you are only 50% likely to find a significant effect that actually exists for hypothesis testing purposes. To increase the power that you will be able to detect significant effects if they exist requires additional sample size. Our consultants at Sawtooth Analytics can help you with decisions regarding precision and power related to selecting an appropriate sample size.
Understanding and correctly applying sampling methods are pivotal for the success of market and survey research. The choice of sampling method directly impacts the accuracy, reliability, and applicability of the research findings. By carefully considering the research objectives, population characteristics, practical constraints, and by diligently working to avoid errors and biases, researchers can select the most appropriate sampling strategy for their studies.
Furthermore, determining the right sample size is essential for achieving statistically significant results without overextending resources. Through thoughtful planning and execution, researchers can ensure their studies contribute valuable insights that lead to good policy, decision-making, and inferences about the population.
Sawtooth Software
3210 N Canyon Rd Ste 202
Provo UT 84604-6508
United States of America
Support: [email protected]
Consulting: [email protected]
Sales: [email protected]
Products & Services
Support & Resources
About The Author

Silvia Valcheva
Silvia Valcheva is a digital marketer with over a decade of experience creating content for the tech industry. She has a strong passion for writing about emerging software and technologies such as big data, AI (Artificial Intelligence), IoT (Internet of Things), process automation, etc.
thank you.. helped me a lot..

Glad to help!
Thankyou so much for this info. It helped me a lot
Leave a Reply Cancel Reply
This site uses Akismet to reduce spam. Learn how your comment data is processed .
The Largest T. Rex Was Probably Much Bigger Than We Ever Realized
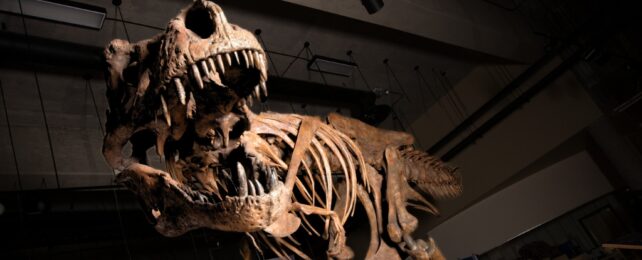
If you select a random person out of a crowd, odds are they won't be the tallest human that ever lived. So why should we expect the same for dinosaurs ?
Of all the Tyrannosaurus rex fossils we've found, it's statistically unlikely that any of them are the largest of their species to have lived. It's even difficult to gauge where they sit on the T. rex height chart.
A new study estimates that, statistically, the largest T. rex could have been 70 percent more massive than the largest specimen we have on record. That's a whopping weight of around 15,000 kg (30,000 pounds), compared to our largest fossil T. rex, Scotty , who is estimated to have tipped the scales at 8,800 kg when he died.
Paleobiologist Jordan Mallon from the Canadian Museum of Nature and paleontologist David Hone from Queen Mary University of London interrogate the issue that many life scientists face when trying to estimate the size range of a species based on a small sample of data.
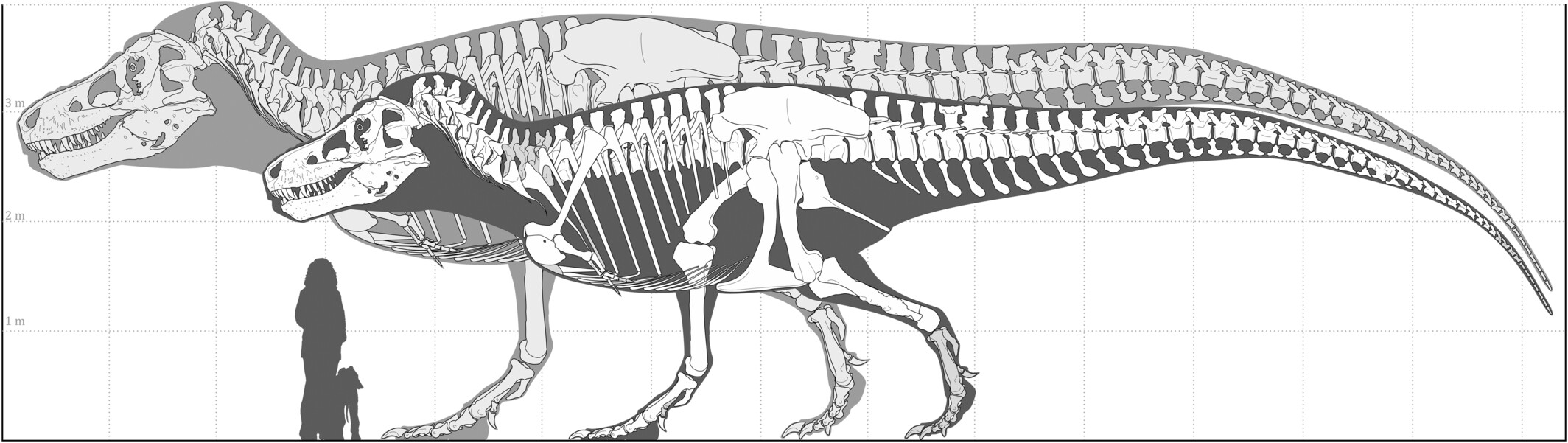
As the team writes , "unless population sampling is both intensive and spatiotemporally exhaustive, it can be difficult to establish the upper limits of body size even for extant species."
Approximately 2.5 billion T. rexes are thought to have graced our planet in the species' short 2.4 million years, and yet so far we have only 84 reasonably complete skeletons to go off.
"Some isolated bones and pieces certainly hint at still larger individuals than for which we currently have skeletons," says Hone.
To fill in the gaps, Mallon and Hone generated 140 million virtual T. rex characters using a computer model that assigns body mass to each individual based on all the variables they could muster, including population size, growth rate, lifespan, and gaps in the fossil record.
This allowed them to estimate where the known fossil specimens would have stood on school photo day, if dinosaurs had those.
They found that we've likely already sampled a T. rex that is larger than 99 percent of all others that lived on Earth. The jury is still out on just how steep the curve is in that final 1 percent.
T. rex was chosen because it's a well-known species, for which many of those variables have been well-estimated.
But we don't know much about the king lizard's body size variation at adulthood, so the researchers turned to one of the dinosaur 's closest living relatives, the American alligator ( Alligator mississippiensis ), as a reference point to account for the sizes of different sexes.
This may not be an ideal approximation. While that's the model in which a dinosaur 70 percent larger than Scotty outshines him, the authors note that the 15,000 kg maximum body mass estimate is more of a statistical fancy than a size we can be sure any T. rex actually reached.
Further research into the sexual dimorphism of T. rex and the biomechanical and ecological constraints on their body size may offer a clearer picture.
When it comes to the largest dinosaur contest, Mallon and Hone's research reminds us of the importance of comparing stats rather than skeletons.
"It's important to stress that this isn't really about T. rex , which is the basis of our study, but this issue would apply to all dinosaurs, and lots of other fossil species," says Hone.
"Arguing about 'which is the biggest?' based on a handful of skeletons really isn't very meaningful."
This research is published in Ecology and Evolution .
- MyServiceNSW
- Manage account
- Logout of MyServiceNSW
Understanding our customers: the role of research in the OneCX Program
How do we create exceptional user experiences (UX) that meet customers’ needs? Through research, we uncover who our customers are and how best to serve them.
The OneCX Program vision is to create a more connected, accessible and inclusive future for all of NSW. To achieve this, we use research to understand our customers. We aim to create exceptional user experiences (UX) that meet customers' needs.
The essence of great UX design lies in solving the right problem for the right customer with the right solution. Just as Henry Ford famously noted, if he had asked people what they wanted, they would have said a faster horse. Instead, he understood their underlying need. They wanted something faster and more convenient. This led to the invention of the motor vehicle. This highlights the importance of knowing who your customers are and what they need.
The power of research
The OneCX Program team works with agencies to identify who their customers are. Together we conduct research to better serve their needs. Research involves gathering information and data systematically to answer questions or solve problems. It's the key to understanding and empathising with our customers. We use quantitative and qualitative research methods to gather comprehensive and accurate insights.
Quantitative and qualitative research
Quantitative research involves collecting numerical data and analysing it using statistical methods. This includes experiments, data analyses and closed-ended surveys. It provides objective, generalisable results but may lack depth and context.
Qualitative research focuses on exploring experiences, thoughts, feelings, and behaviours. This is often through speaking with people. It includes interviews, focus groups and open-ended surveys. Qualitative research offers deep, detailed insights. Yet, on their own, these can be subjective and harder to generalise.
Rather than choosing one method over the other, we combine both. This helps to gather diverse findings that are both deep and detailed.
Sampling is the process of selecting a small group of individuals from a larger population. This helps to draw conclusions about that population’s needs. For instance, if we want to understand what information and services preschool mothers use. Asking every mother would lead to accurate findings. However, it would be very expensive and time-consuming. Instead, we can ask a small, representative group to gain sufficiently accurate insights efficiently and affordably.
However, it's crucial to be mindful of the margin of error. This is the degree of uncertainty that what our sample says is true for the population. Tools like sample size calculators can help calculate this margin.
Our research methods
- Persona creation : develop detailed profiles of our key customer segments. Our research archetypes are citizens, businesses, visitors and public servants. Within these broad archetypes we have created a set of personas to represent a range of typical customers.
- Customer journey mapping : visualise the steps our customers take when interacting with our services.
- User interviews : conduct online interviews with people from across NSW to gain in-depth insights.
- Usability testing : assess how easily people can use our digital products.
- Card sorting : understand how people categorise and label information.
- Google Analytics data analysis : identify trends in how people use nsw.gov.au.
- Surveys : collect a range of insights from our customers.
- Heatmapping and session replays : observe how users interact with our digital platforms.
- Culturally and linguistically diverse (CALD) audiences : we also consider the needs for CALD audiences . Where relevant, we use in-language focus groups. Our 4 key languages are Mandarin, Cantonese, Vietnamese and Arabic.
Analyse the data to make sound design decisions
Once research is complete, we analyse the data and summarise the findings. These findings become the basis of our design decisions.
We save all the research into a central repository. Labelled by topic, this helps other teams draw on existing knowledge. Aside from cost and resource savings, this helps to reduce duplication.
We work with agencies to identify their customers and how to best service their needs. We design content based on the customer, and not the structure of government. Conducting research and learning about our customers, helps deliver on this focus.
Want to learn more? Check out our previous blog on how our design and research teams work and learn together .
Stay in touch with the OneCX program
Sign up to our newsletter for regular updates on the program rollout.

Site-resolved energetic information from HX/MS experiments
- Find this author on Google Scholar
- Find this author on PubMed
- Search for this author on this site
- ORCID record for Chenlin Lu
- ORCID record for Malcolm L. Wells
- ORCID record for Andrew Reckers
- ORCID record for Anum Glasgow
- For correspondence: [email protected]
- Info/History
- Supplementary material
- Preview PDF
While bioinformatics reveals patterns in protein sequences and structural biology methods elucidate atomic details of protein structures, it is difficult to attain equally high-resolution energetic information about protein conformational ensembles. We present PIGEON-FEATHER, a method for calculating free energies of opening (∆Gop) at single- or near-single-amino acid resolution for protein ensembles of all sizes from hydrogen exchange/mass spectrometry (HX/MS) data. PIGEON-FEATHER disambiguates and reconstructs all experimentally measured isotopic mass envelopes using a Bayesian Monte Carlo sampling approach. We applied PIGEON-FEATHER to reveal how E. coli and human dihydrofolate reductase orthologs (ecDHFR, hDHFR) have evolved distinct ensembles tuned to their catalytic cycles, and how two competitive inhibitors of ecDHFR arrest its ensemble in different ways. Extending the method to a large protein-DNA complex, we mapped ligand-induced ensemble reweighting in the E. coli lac repressor to understand the functional switching mechanism crucial for transcriptional regulation.
Competing Interest Statement
The authors have declared no competing interest.
https://github.com/glasgowlab/PIGEON-FEATHER/tree/main
View the discussion thread.
Supplementary Material
Thank you for your interest in spreading the word about bioRxiv.
NOTE: Your email address is requested solely to identify you as the sender of this article.

Citation Manager Formats
- EndNote (tagged)
- EndNote 8 (xml)
- RefWorks Tagged
- Ref Manager
- Tweet Widget
- Facebook Like
- Google Plus One
Subject Area
- Animal Behavior and Cognition (5501)
- Biochemistry (12485)
- Bioengineering (9352)
- Bioinformatics (30658)
- Biophysics (15772)
- Cancer Biology (12832)
- Cell Biology (18401)
- Clinical Trials (138)
- Developmental Biology (9945)
- Ecology (14883)
- Epidemiology (2067)
- Evolutionary Biology (19078)
- Genetics (12684)
- Genomics (17447)
- Immunology (12590)
- Microbiology (29578)
- Molecular Biology (12301)
- Neuroscience (64383)
- Paleontology (478)
- Pathology (1984)
- Pharmacology and Toxicology (3435)
- Physiology (5289)
- Plant Biology (11024)
- Scientific Communication and Education (1725)
- Synthetic Biology (3050)
- Systems Biology (7650)
- Zoology (1724)
Log in using your username and password
- Search More Search for this keyword Advanced search
- Latest content
- Supplements
- BMJ Journals
You are here
- Volume 8, Issue 7
- War-related sexual and gender-based violence in Tigray, Northern Ethiopia: a community-based study
- Article Text
- Article info
- Citation Tools
- Rapid Responses
- Article metrics

- Girmatsion Fisseha 1 ,
- Tesfay Gebregzabher Gebrehiwot 2 ,
- Mengistu Welday Gebremichael 3 ,
- Shishay Wahdey 1 ,
- http://orcid.org/0000-0001-6563-0001 Gebrekiros Gebremichael Meles 1 ,
- http://orcid.org/0000-0002-5874-3304 Kebede Embaye Gezae 1 ,
- Awol Yemane Legesse 4 ,
- Akeza Awealom Asgedom 1 ,
- Mache Tsadik 1 ,
- Abraha Woldemichael 1 ,
- Aregawi Gebreyesus 1 ,
- Haftom Temesgen Abebe 1 ,
- Yibrah Alemayehu Haile 5 ,
- Selome Gezahegn 6 , 7 ,
- Maru Aregawi 8 ,
- http://orcid.org/0000-0003-2303-8493 Kiros T Berhane 9 ,
- Hagos Godefay 5 ,
- Afework Mulugeta 1
- 1 School of Public Health , Mekelle University College of Health Sciences , Mekelle , Tigray , Ethiopia
- 2 Epidemiology , Mekelle University College of Health Sciences , Mekelle , Tigray , Ethiopia
- 3 Department of Midwifery , Mekelle University College of Health Sciences , Mekelle , Tigray , Ethiopia
- 4 School of Medicine , Mekelle University College of Health Sciences , Mekelle , Tigray , Ethiopia
- 5 Tigray Health Bureau , Mekelle , Tigray , Ethiopia
- 6 Hennepin Healthcare , Minneapolis , Minnesota , USA
- 7 University of Minnesota Medical School , Minneapolis , Minnesota , USA
- 8 Global Malaria Program , World Health Organization , Geneve , Switzerland
- 9 Biostatistics , Columbia University , New York , New York , USA
- Correspondence to Professor Kiros T Berhane; kiros.berhane{at}columbia.edu
Introduction Sexual and gender-based violence (SGBV) during armed conflicts has serious ramifications with women and girls disproportionally affected. The impact of the conflict that erupted in November 2020 in Tigray on SGBV is not well documented. This study is aimed at assessing war-related SGBV in war-affected Tigray, Ethiopia.
Methods A community-based survey was conducted in 52 (out of 84) districts of Tigray, excluding its western zone and some districts bordering Eritrea due to security reasons. Using a two-stage multistage cluster sampling technique, a total of 5171 women of reproductive age (15–49 years) were randomly selected and included in the study. Analysis used weighted descriptive statistics, regression modelling and tests of associations.
Results Overall, 43.3% (2241/5171) of women experienced at least one type of gender-based violence. The incidents of sexual, physical and psychological violence, and rape among women of reproductive age were found to be 9.7% (500/5171), 28.6% (1480/5171), 40.4% (2090/5171) and 7.9% (411/5171), respectively. Of the sexual violence survivors, rape accounted for 82.2% (411/500) cases, of which 68.4% (247) reported being gang raped. Young women (aged 15–24 years) were the most affected by sexual violence, 29.2% (146/500). Commonly reported SGBV-related issues were physical trauma, 23.8% (533/2241), sexually transmitted infections, 16.5% (68/411), HIV infection, 2.7% (11/411), unwanted pregnancy, 9.5% (39/411) and depression 19.2% (431/2241). Most survivors (89.7%) did not receive any postviolence medical or psychological support.
Conclusions Systemic war-related SGBV was prevalent in Tigray, with gang-rape as the most common form of sexual violence. Immediate medical and psychological care, and long-term rehabilitation and community support for survivors are urgently needed and recommended.
- health policy
- public health
- community-based survey
Data availability statement
Data are available on reasonable request.
This is an open access article distributed in accordance with the Creative Commons Attribution Non Commercial (CC BY-NC 4.0) license, which permits others to distribute, remix, adapt, build upon this work non-commercially, and license their derivative works on different terms, provided the original work is properly cited, appropriate credit is given, any changes made indicated, and the use is non-commercial. See: http://creativecommons.org/licenses/by-nc/4.0/ .
https://doi.org/10.1136/bmjgh-2022-010270
Statistics from Altmetric.com
Request permissions.
If you wish to reuse any or all of this article please use the link below which will take you to the Copyright Clearance Center’s RightsLink service. You will be able to get a quick price and instant permission to reuse the content in many different ways.
WHAT IS ALREADY KNOWN ON THIS TOPIC
Conflict-based sexual and gender-based violence (SGBV) is known to have serious immediate and long-term adverse societal impact with women and girls affected the most.
WHAT THIS STUDY ADDS
This study provides first-of-its-kind objectively and carefully collected primary data on the scale and level of SGBV in the Tigray region, Northern Ethiopia, as a result of the conflict that erupted in November 2020.
HOW THIS STUDY MIGHT AFFECT RESEARCH, PRACTICE OR POLICY
By providing carefully collected evidence on the level and impact of SGBV in the Tigray region, the study findings will help policy makers develop models of working with women who have experienced SGBV in the context of war, on establishing accountability for the atrocities committed and in planning for the unprecedented medical, psychological and rehabilitation needs of SGBV survivors.
Introduction
Sexual and gender-based violence (SGBV) is a worldwide phenomenon without any geographical, cultural, social, economic, ethnic or other boundaries. It is a form of violence that is inflicted on the basis of gender differences. 1 War-related SGBV has significant and severe adverse social impact, both during and the postconflict period. SGBV against women is often committed on a massive scale during wars and conflicts. That is, women and girls are disproportionately targeted in conflicts, systematically raped, intimidated, sexually and physically abused, forced into unwanted pregnancies and/or killed. 2–6
War-related sexual and human right abuses are still prevalent at the global scale. They mostly occur during conflicts in low-income (especially in Africa) and some high-income countries in general. 7–15 Independent studies in many countries including in Africa have reported prevalence of sexual violence ranging from 2.6% in the current war crisis in Ukraine (2.6%) to 21.3% in South Sudan during the civil war between 2005 and 2011. 8–15 However, sexual violence in most conflicts has not been well assessed for several reasons including prolonged periods of conflict, as well as sociocultural and other complex issues, especially in low-income countries. 7
Most sexually abused women suffer emotional breakdowns, especially those from the rural communities where the moral codes are strict. Raped women do not routinely report the incidents for fear of family alienation and stigmatisation by their communities. In low-income countries, raped daughters are often disclaimed by their parents, and raped wives are rejected by their husbands. 16
Many impregnated women, after rape, undergo ‘back-street’ abortions that put their lives at risk. Some cannot even look at their babies. Still others give them away. 5 6 During a conflict, men and women often lose their lives from various causes and are likely to be tortured and abused in various ways for biological, psychological or socio-economic reasons. While relatively more men are killed during wars, women often experience violence, forced pregnancy, abduction, sexual abuse and slavery. The harm, silence and shame women experience because of war is pervasive; with their redress, almost non-existent. The situation of women in armed conflicts has been systematically neglected 5 17 in taking concrete actions, although the United Nation has designated SGBV as war crimes in Article 8 of the Rome Statute of the International Criminal Court. 18
On 4 November 2020, war erupted in the Tigray region of Ethiopia following years of growing tensions between the federal government of Ethiopia and the regional government of Tigray in Ethiopia. The causes and development of the war in Tigray were highly complex and multidimensional including the involvement of both internal and external parties’ interests. 19 A complete account and analysis of the cause of the conflict is beyond the scope of this manuscript. During the war, several parties were involved with the Tigray regional special forces on one side and allied forces such as the Ethiopian National Defence Forces Amhara regional special armed forces and Amhara militias, and the Eritrean Defence Forces on the other side. 20 21
The war in Tigray that erupted in the beginning of November 2020 has resulted in a massive humanitarian crisis. Preliminary reports have shown that Tigrayan women and girls have experienced deliberate and organised widespread war-related SGBV, in which some were subjected to severe violence including gang-raping, and the insertion of foreign objects to their reproductive organs. 20 According to the report of the Human Rights Watch (HRW), 2204 survivors sought services for sexual violence at health facilities across Tigray from November 2020 through June 2021. 21 This figure is more likely to be under-reported owing to the fact that many of the victims had poor healthcare access and some of them are less likely to seek healthcare for fear of stigmatisation. Besides, many of the health facilities were non-functional because the war has eroded the more than two decades previous investment and progress in the health systems and resulted in 70% of the health institutions either destroyed or their status could not be ascertained. 20 22
Furthermore, a recent survey report revealed that only 17.5% of the health centres were functional after 6 months of the war. 22 Most of the available evidence on SGBV in Tigray during the conflict period was based on reports from the limited functioning health facilities and hence it is likely to be unrepresentative. The scale and burden of the war-related violence at community level in Tigray is also not comprehensively known. 21 Thus, the purpose of this study was to determine the extent, and distribution of the SGBV and its impact on survivors using community-level survey during the first round of the active war period of the war in Tigray. The findings of this study are anticipated to be used as baseline data on the burden, severity and factors of the SGBV during the war period in Tigray, Ethiopia. Besides, the findings contribute valuable data to humanitarian agencies, as well as national and local authorities in providing a comprehensive medical and psychological support to survivors, and in reducing the burden of SGBV against women and girls during wars and conflicts in Tigray and elsewhere. The findings will also provide guidance on the needs for, and/or availability of, health services for survivors of SGBV, including further intervention for establishing medical and psychological services, continuous follow-up and support for survivors.
A community-based survey was conducted in six zones of Tigray, after the Eritrean, Ethiopian and Amhara forces left Mekelle, the capital of Tigray, Ethiopia. By 28 June 2021, the Regional Government of Tigray restored its administrative control of most parts of Tigray. After the withdrawal of the allied forces, there was a relatively reduced active conflict in the parts of Tigray under the control of the Regional forces of the Government of Tigray. Thus, the survey was conducted during 4–20 August 2021 immediately after the withdrawal of the allied forces from most parts of Tigray. The western zone of Tigray and the districts bordering Eritrea were not included due to security reasons.
Women of reproductive age (ie, 15–49 years) recruited from the study communities were included as primary respondents in this survey. Information on girls under 15 years and women above 50 years of age were also collected from the primary respondents and is separately presented in this study. The period of the SGBV incidents covered from 4 November 2020 to 28 June 2021.
Multistage cluster sampling was used to select women of reproductive age from selected households (HHs). Tabiya/Kebelle (smallest administration unit) was considered as a cluster. A total of 52 districts out of 84 districts in the 6 zones of Tigray were randomly included in the study. The 52 districts included in this study accounted for 64.4% of the Tigray population. From each of these 52 districts, 4 Tabiyas/clusters were randomly selected and from each cluster, 20 HHs were randomly selected making a total sample size of 4160 HHs. If a selected HH had multiple women of reproductive age, only one woman was randomly selected for the interview.
Taking the prevailing situation on the ground into consideration, two sampling approaches (random and purposive) were designed with their own sample sizes. In the random approach, the list of all the Tabiyas/clusters in the district was used as a sampling frame and four Tabiyas/clusters were selected randomly. However, in the purposive approach, the Tabiyas/clusters were grouped into moderately and severely war-affected ones. The list of the severely affected Tabiyas/clusters was used as a sampling frame and four severely affected Tabiyas/clusters were randomly selected ( figure 1 ). ‘Random group’ and ‘purposive group’ was defined based on the information obtained from the local administrative authorities at the field level related to war situation of the context. The ‘purposive group’ was composed of those clusters which sustained repeated fighting in their communities, longer duration of stay of the combatants and harassment among the community members in the context. However, the ‘random group’ involved randomly selected clusters irrespective of the active war, and other characteristics of the prevailing situation. At the beginning of sampling, we planned to select four distinct Tabiyas/clusters per district from each group. However, due to the availability of only few Tabiyas/clusters in some districts, some Tabiyas/clusters were selected in both groups. Due to this situation, HHs data from a subset of Tabiyas/cluster were shared by both groups. A total of 3693 HHs were included from the purposive group and of these, 1489 HHs were unique to the purposive group. From the random group, 3682 HHs were randomly selected of which 1478 HHs were unique for random group. Thus, 2202 HHs were included in both groups. During the preliminary stage of the analysis, it was observed that there were no significant differences in sexual violence/rape between the two groups ( online supplemental table 1 ). Thus, the findings reported in this study are the results generated from the merged data of 5171 HHs. Additionally, the study included SGBV incidents on other members of the HH (including underage girls, men and old age women) based on the report of the index woman in each HH interviewed. Then, detailed interviews were conducted with each reported HH member about the types of violence and consequences. Generally, the dataset for the final analysis included a total of 5171 women of reproductive age group (15–49 years), 1196 men, 53 girls aged <15 years and 227 women aged 50 years and older.
Supplemental material
- Download figure
- Open in new tab
- Download powerpoint
The sampling framework for the study. HHs, Households.
The outcome variables of this study were types of SGBV, and consequence of SGBV. For modelling the SGBV outcome variables like age, residence, religion, occupation, education, reproductive health characteristics, family member violence and health-facility utilisation were used as explanatory variables. For the consequence of SGBV, types of SGBV, age and education were used as explanatory variables.
Data collection, management and analysis
The data collection and field coordination process was challenging due to the ongoing war. Furthermore, all services including telephone network, electricity and transportation were not available in Tigray because of the war and siege. 21 For these reasons and other security-related issues, the application of electronic data collection tools including the use of mobile applications was impossible. Thus, we used a standard and validated interviewer-administered paper-based questionnaire to collect data following adoption of the tool from those used in WHO multicountry study 23 ( online supplemental file 1 ). The questionnaire was translated from English to Tigrigna (the local language) and then back translated to English by another translator to ensure consistency of the tool in data collection. The questionnaire consisted of various sections that enable the collection of data related to sociodemographic, reproductive health characteristics, SGBV, consequences, coping mechanism, self and family member violence and health-facility utilisation by victims.
We recruited two supervisors per district with educational level of MSc and above from the College of Health Sciences of Mekelle University, Tigray Health Research Institute and Tigray Regional Health Bureau. They were trained on the objectives of the study and the administration of the tool for 5 days in Mekelle, capital of Tigray. Transportation was arranged for field work 1 week ahead of the data collection period to facilitate the recruitment of competent female health extension workers (HEWs) for the data collection, to develop a map of each selected cluster and to prepare list of the HHs in the selected clusters. Two female HEWs were used as interviewers (data collectors) at each selected Tabiya/cluster; and a total of 416 HEWs participated in the 52 districts. HEWs were assigned one per group for both the ‘purposive group’ and the ‘random group’. The team of supervisors provided training to the data collectors for 3 days at each district. A representative of the health office at each selected district participated in the orientation of the HEWs to support the process of data collection. Then, the HEWs were allowed a 1 day exercise and pretest of the tool in the field in the same community but in HHs that were not sampled for the study. The tool was further adapted and adjusted to maximise the validity and reliability of the collected data. The supervisors used the closest health facility for accommodation and the district health office as a meeting place. Besides, they checked the completed questionnaires daily for any unclear or incomplete information or wrong coding. Errors were checked and addressed in the field, if any. The entire data collection process was coordinated and supervised by six teams of investigators (one team per zone), and any challenges during the data collection were addressed in a timely manner.
The collected data were entered into EpiData V.3.1. Quality of the data was further ascertained during data-entry and cleaning through visualisation and thorough correction of any errors and outliers. Besides, the rejected responses were re-evaluated. All these processes were done together with senior biostatisticians. Descriptive statistical analyses, with sampling weighting as necessary, were used for tabulation, cross-tabulation and computation of frequencies and percentages on selected variables. Sensitivity analysis was conducted by considering weighting for each selected district (Woreda) in the descriptive analysis. The χ² test was used to test for any associations of SGBV types with the consequences of the SGBV, with attention to potential corrections for multiple comparisons. 24 A logistic regression model for binary data was also used to assess the factors associated with war-related rape in the study area. All analyses were performed using the statistical package STATA V.15.1 (StataCorp, College Station, Texas, USA) and we considered the association to be statistically significant at a value of p<0.05.
Measurements
SGBV was measured according to WHO standard guidelines. 23
Sexual violence
Includes, at least, rape/attempted rape, sexual abuse and sexual exploitation. Sexual violence is any sexual act, attempt to obtain a sexual act, unwanted sexual comments or advances or acts to traffic a person’s sexuality, using coercion, threats of harm or physical force, by any person regardless of relationship to the victim, in any setting, including but not limited to home and work. Sexual violence can take many forms, including rape, sexual slavery and/or trafficking, forced pregnancy, sexual harassment, sexual exploitation and/or abuse and forced abortion.
Rape was defined to occur if a woman experienced any act of non-consensual sexual intercourse. This can include the invasion of any part of the body with a sexual organ and/or the invasion of the genital or anal opening with any object or body part. Rape and attempted rape involve the use of force, threat of force and/or coercion. Any penetration without consent is considered rape. Efforts to rape someone which do not result in penetration are considered attempted rape.
Physical violence
An act of physical violence that is not sexual in nature. It was measured as physical violence, if a woman had experienced at least one of the following: hitting, slapping, choking, cutting, shoving, burning, shooting or use of any weapons, acid attacks or any other act that results in pain, discomfort or injury. This incident type does not include female genital mutilation or cutting.
Psychological violence
Infliction of mental or emotional pain or injury. It was measured as psychological violence, if a woman had experienced at least one of the following: threats of physical or sexual violence, intimidation, humiliation, forced isolation, stalking, harassment, unwanted attention, remarks, gestures or written words of a sexual and/or menacing nature, destruction of cherished things, etc.
‘Purposive group’
Tabiyas/clusters selected randomly from the severely war-affected Tabiyas/clusters. The Tabiyas/clusters were considered severely affected if they are exposed to sustained active war and/or intermittent war, long duration of stay of the combatants in the area and harassment among the community members in context.
‘Random group’
Tabiyas/clusters selected randomly from a given district using the list of all Tabiyas/clusters (moderately and severely affected) as a sampling frame. Regardless of active war and other characters of the situation, random selections of Tabiyas/clusters were conducted.
Calculation of weights
Lists of districts/Tabiyas/clusters included in ‘random group’ and ‘purposive group’ based on the population of women aged 15–49 years were grouped and taken from the Tigray regional Health Bureau during 2020/21. Three columns were then created (list of districts, total sample taken, total population of women in the selected clusters per district) for each group within Excel. To calculate proper weights, we summed up the total samples and total population in each group separately. Then, weights were calculated as follows:
where w1 (district in purposive group) =total population in each district in purposive group/total population in purposive group; w1 (district in random group) =total population in each district in random group/total population in random group; w2 (district in purposive group) =total sample in each district in purposive group/total sample in purposive group and w2 (district in random group) =total sample in random group/total sample in random group.
Then, weighting data were generated in STATA. Based on this mechanism, the weight assigned to each selected district is found in online supplemental table 4 .
Patient and public involvement
The development of the research topic was directly motivated by the unprecedented experience of the people of Tigray due to the devastating conflict and the need to document the experienced conflict related to SGBV. The participation of study subjects was via their willingness to respond to the survey instruments. Findings from the study are disseminated to the local communities and appropriate authorities in order to inform policy decisions in restoring health services and providing much-needed medical and psychological treatment for SGBV victims.
Ethical considerations
As the interview was conducted by trained female HEWs, the probability of harm or discomfort in this assessment is anticipated to be minimal. All filled-questionnaires were anonymised via subject-level numerical identifiers and kept confidential.
Sociodemographic characteristics of women of reproductive age
In this study, information about 5171 women of reproductive age was included from six zones. Women included in this study were mainly from central (32.3%), eastern (28.2%) and north-west (15.2%) zones of Tigray. The median age of women was 32 years (IQR 26–38). Roughly, half of the study participants were from rural residences (2602, 50.3%). More than a third of the women interviewed were unable to read or write (1879, 36.3%); and 70% of the women were married while 34.5% of them reported their occupation as housewives and 29.9% as farmers ( online supplemental table 2 ). To enable contextual comparisons, the current population profile of Tigray, Northern Ethiopia is included in online supplemental table 3 .
Reproductive and obstetric characteristics of women aged 15–49 years
Of the 5171 surveyed women, 3476 (67.2 %) had at least one child under 5 years of age; and 422 (8%) were pregnant at the time of the survey. Of these pregnant women, 75 (17.8%) of the pregnancies were unwanted. A total of 137 (2.7%) women had an abortion during the study period (first 8 months of the war) ( online supplemental table 5 ).
Magnitude of sexual and gender-based violence
The incidents of sexual violence, physical violence and psychological violence were found to be 9.7%, 28.6% and 40.4%, respectively. Detailed disaggregated data on types of violence is provided in online supplemental table 6 . Overall, 43.3% (2241/5171) women experienced at least one type of gender-based violence (psychological, physical or sexual violence). Most women experienced various, and at times, multiple forms of violence. Nearly 7.4% of the women who experienced sexual violence had at the same time experienced physical and psychological violence ( figure 2 ).
War time prevalence of sexual and gender-based violence (SGBV) among women of reproductive age in 52 districts of Tigray (n=5171), 4 November 2020 to 28 June 2021.
Sexual and gender-based violence in relation to some sociodemographic characteristics
The level of SGBV varied among zones as well as by age, residence, education and marital status. Sexual, physical and psychological violence were highest in central zone followed by eastern zone of Tigray. Of the 500 women that reported experiencing sexual violence, 202 (40.4%) women were from the central zone followed by 144 (28.8%) women in the eastern zone. Similarly, 146 (29.2%) young women aged 15–24 years reported as being highly sexually abused, whereas women aged 35–39 years were more physically (307/1480; 20.7%) or psychologically (459/2091; 21.9%) abused. Women in reproductive age living in urban residence were more sexually abused (243, 48.6%), whereas rural residents were more physically (660; 44.6%) or psychologically (1011; 48.3%) abused. The women who did not join formal education (both those ‘unable to read and write’ and those ‘able to read and write’) were the most sexually abused groups (249; 43.8%). Of the 692 pregnant women who responded to the violence related to questions during the war time, 62 (12.6%) pregnant women reported of being sexually abused ( online supplemental table 7 ).
About 9.7% (500/5171) of the women of reproductive age had suffered from sexual violence; and 7.9% (411/5,171) women were raped during the study period. Of the 411 women that experienced sexual violence, 411/500 (82.2%) reported of being raped ( online supplemental table 7 ). Repeated cases of sexual violence were reported by women. Of those 411 women that reported being raped, 271 (65.9%) were abused once, 62 (15.1%) twice and 78 (19.0%) three times or more ( figure 3 ). Women of reproductive age were sexually abused on average by three soldiers to a maximum of nine (median=3, IQR 2–6). Most raped women reported being gang-raped, 68.4% (247/361). The remaining 50 raped women did not respond to this question. Most (45.9%) of the sexual violence on women of reproductive age had taken place during the first 150 days of the war, while 26.3% of the sexual violence occurred during the subsequent 86 days—a period that corresponded to the second-round of a large-scale military operation by the allied forces against Tigray forces.
Frequency and type of rape during Tigray war, 4 November 2020 to 28 June 2021.
Consequences of SGBV
The consequences of sexual, psychological and physical violence during the conflict period ranged from behavioural problems and injury to potentially lifelong health and physical complications. In this study, physical trauma was the most common consequence of SGBV (28.3%; 533/2241) including incidents of dislocations (cases=84), fracture or broken bone (cases=37), perforated eardrum or eye injuries (cases=20) and broken teeth (cases=8). Moreover, severe consequences of sexual violence among those who visited a health facility included HIV infection (11 cases), sexually transmitted infections excluding HIV infection (68 cases), unwanted pregnancy (39 cases) and others. The emotional and behavioural consequences of physical and sexual violence were also common, including depression (19.2%; 431/2241), social isolation (3.8%, 85/2241), suicidal ideation and attempt (2.6%, 58/2241) and others. As a result of the SGBV, majority of women reported an emotional change such as stress (84.1%; 1438/1709), anxiety (11.5%; 197/1709) as well as instances of flashbacks of the incidence, nightmare or sleeping disturbance. Although many women of reproductive age experienced various forms of violence, nearly 90% of the women (1629/1817) did not receive any medical care at a health facility (424 women did not provide a response about healthcare use after violence). The main reported reasons for not receiving healthcare were destruction of the health facilities (52.9%; 618/1169), victims’ disappointments (leading to hopelessness) (27.0%; 316/1169) and other reasons including physical disability and lack of transport ( table 1 ).
- View inline
Consequences of SGBV in women of reproductive age from Tigray, 4 November 2020 to 28 June 2021
The findings showed that women who were physically abused had experienced statistically significant physical trauma, depression, suicidal ideation or attempt and social isolation (p<0.001). Similarly, sexually abused women experienced physical trauma, excessive worry, depression, suicidal ideation and attempt (p<0.001) ( table 2 ). These findings were still statistically significant after Bonferroni-type corrections were made for multiple comparisons within a given type of violence (ie, physical or sexual).
Consequences of SGBV in relation to the types of violence and sociodemography of women, Tigray, 2021
SGBV to any household members other than women of reproductive age
During the first 8 months of armed conflict, gender-based violence was not confined to women of reproductive age only. Other HH members (children, men/boys or elderly women) were also victims of SGBV as reported by the women of reproductive age interviewed in the study. Based on the responses obtained from the primarily interviewed respondents, more than a quarter (27.8%; 1417/5171) of other members (children, men/boys or elderly women) of the HH were reported to be victims of some form of psychological, physical or sexual violence. A total of 57.4% (686/1196) men/boys were reported to have experienced physical violence. Moreover, about 17.0% (9/53) of girls under 15 years of age and 8.4% (19/227) of older women above the age of 49 years were experienced sexual violence. Deaths of 242 HH members perpetrated by the allied forces were also reported in this study ( table 3 ).
SGBV among children, elderly women household members and women of reproductive age in Tigray war, 4 November 2020 to 28 June 2021
Factor associated with war-related rape
After entering significant variables with p value <0.05 in the bivariate and multivariate logistic regression models, women in the age group 20 and 24 years, with no formal education, being urban residence, student and unemployed, living in the temporary shelter within the community and rental house and having knowledge about raped incidence in the community were associated with war-related rape ( table 4 ).
Factors associated with war-related rape in Tigray, Ethiopia (n=411)
SGBV was one of the most serious and life-threatening occurrences that have affected women and children during the first 8 months of the war in Tigray. The findings from women of reproductive age showed that 1 in 10 women and girls experienced sexual violence, mostly rape, physical violence and psychological violence. Many women experienced severe forms of sexual violence such as gang-rape, sexual salivary, insertion of foreign objects into the vagina and harassment. Gang-rape was the most common form of sexual violence reported among those who were raped. Young girls under 15 years and elderly women above 49 years were also victims of sexual violence, mostly rape. Living in a temporary shelter within the community, age 20–24 years, being urban residence, no formal education, being student and unemployed by occupation were the risk factors associated with war-related rape in this study.
The findings from this study indicate higher incidence (nearly 10%) of rape than those reported in other studies during conflicts such as in Northern Uganda (4.2%), 13 Seirra Leone (8%) 15 and Ukriane (2.6%). 8 Similarly, the physical violence (28.6%) observed in this study was higher than the findings for East Timor, Indonesia where 22.7% of the women were physically assaulted. 25 However, the finding for the current study is lower than other studies for the Kurdistan region of Iraq (16.6%) 10 and South Sudan (21.3%). 11 The difference in prevalence with the Iraq study might be due to the fact that the study was at camp and dealing with a vulnerable group, and the South Sudan study covering a long duration of civil war from 2005 to 2011. In contrast, the current study was conducted at community level for only 8-month duration of war. Overall, the sexual violence observed in the current study showed consistently and systematically higher levels of incidence in the central and eastern zones of Tigray, where the invading allied forces of Ethiopia and Eritrea had more control. The higher incidence of rape and physical violence in this study compared with other studies may be due to (i) ill-intentions of the perpetrators to use systemic raping as weapon of war to dehumanise the population and (ii) the relatively long duration of the war (8 months) inside Tigray at the hands of the invaders, thereby exposing victims to more violence.
Most of the sexual violence reported in the current study was due to gang-rape, mainly perpetrated by soldiers and other armed groups affiliated with the governments of Ethiopia and Eritrea. This is a severe form of sexual violence and is likely to cause severe forms of psychological, mental, social and sexual disorders among the survivors. Similar findings during a conflict period were reported in eastern Democratic Republic of the Congo (DRC), Rwanda, Bosnia and Herzegovina. 14 26 27 Moreover, in the current study, many women and girls experienced sexual violence by three to nine soldieries. This is generally in-line with the report of the joint United Nations Office of the Human Rights Commission and Ethiopian Human Rights Commission, where women and girls in Tigray were sexually abused by maximum of six armed men during the war period. 20 Most sexual and physical violence was committed by Eritrean defence forces, consistent with the report of the HRW, where most brutal sexual violence was reported as committed by the Eritrean defence forces in the Tigray war. 21
Severe forms of sexual violence such as insertion of foreign objects to vagina and anus, and high rate of HIV transmission were reported in the current study, again consistent with the report from HRW report in February 2021, where insertion of foreign objects into the vagina and transmission of HIV by different armed forces were reported in the Tigray war. 20 21 28 The finding on the severity of sexual violence in the Tigray war in this study is reminiscent and in conformity with similar observations elsewhere. 3 16 17 The risk of HIV infection during conflicts is reported to be relatively higher in Africa due to multiple perpetrators of sexual violence. 29
Sexual violence of underage girls and elderly women reported in this study, ascertained indirectly via the interviewed index participants, are also consistent with the reports of different humanitarian agencies on the Tigray war during the same period. 20 This indicates that the extent and impact of sexual violence, mostly via rapes, were highly prevalent among all age groups of girls and women. The mental and psychological problems experienced by underage girls could have severe, lifelong and generational impact on sexual, behavioural, productivity and well-being of the victims and their families.
The incidence of sexual violence, mostly rape, was typically high in urban centres during the first 8 months of the war period (12% (urban) vs 8.3% (rural); online supplemental table 5 ). This is consistent with the other reports on the Tigray war, where most survivors who sought health services for postsexual violence (rape) were mainly from big towns. 20 Moreover, women from lower socio-economic status are less likely to disclose sexual violence as also reported in a study from eastern DRC. 16 The SGBV figures reported in this study are likely to be the tip of the iceberg of the problem as most of the women, particularly in the rural areas of Tigray are highly traditional and religious; have less sexual literacy and less access to healthcare during the early days of the war as most of the communities, trapped by the war, were hiding in hard-to-reach areas away from their houses and villages. Moreover, potential gross underestimation of cases could result from the strict moral codes and fear of stigma by traditional community and family hostility. 16 All survivors from rural or urban areas appear to share the same level of mental, psychological and health consequences as a result of sexual violence.
In this study, 90% of the sexually abused women were unable to receive medical and psychological support after experiencing sexual violence. This implies the likelihood of the majority of women and girls suffering from incidence of SGBV violence to suffer from its complications such as medical, behavioural and emotional disorders. This is consistent with the study on DRC conflict where only <5% women of SGBV victims sought medical care and the remaining women had to wait a year or longer prior to accessing SGBV services. 30 According to a recent survey, >70% of the health facilities in Tigray were non-operational in the first 6 months of the war (4 November 2020 to April 2021) 22 and only 17.5% of the health centres were functional, most of which were within the vicinities of Mekelle and other big cities such as Shire, Axum, Adwa, Adigrat and others on the main roads. 20 Therefore, the reason for the poor health seeking by survivors could be because most health facilities were destroyed, closed; lack of medications; absence of trained health providers; absences of transportation to health facility; traditional and religious barriers of discussing on sexual matters and fear for physical safety. The mental, psychological, economical and behavioural impact of SGBV on survivors and their families are thus severe and lifelong. Therefore, provisions of immediate medical and psychological support for all survivors are long overdue.
During the prewar period, the accessibility of health service in Tigray was >90%. Health centres could typically provide service for survivors of SGBV, including administered Pruritic papular eruption (PPE) in case of HIV exposure, test for pregnancy and other health services. However, during the period of war, 70% of the health facilities were non-functional 22 either due to complete destruction or absence of medications and/or trained health providers. Most functional health facilities were located at big towns where survivors were usually using the service during the war period. During the war period, the interim office of Tigray health bureau was reporting the numbers of raped survivors who sought health facility every month. 20 There were also mobile clinics given to increase accessibility of healthcare during the war period by the interim Tigray health bureau and different non-governmental organisations (NGOs). However, the availability of health services to respond to cases of SGBV during the war was very low. Few NGOs such as Médecins Sans Frontières-Spain, International Committee of the Red Cross (ICRC), local NGO, Mothers and Children Multisectoral Development Organization (MCMDO) and mobile clinics were participating to support survivors. Overall, it was very difficult to assure whether survivors were using health services appropriately given that 70% of the health facilities were non-functional during the war period. 22 In this study, we did not assess the situation of the catchment of health facilities on whether functional or not. We simply asked the survivors about their use of health services after the violence and, where appropriate, the reasons for not getting health service.
Survivors of sexual violence are likely to be at high risk of severe and long-lasting health problems, including death from injuries or suicide. 31 The deaths from sexual violence were not captured in this study, as the study was solely based on responses from women of reproductive age that were alive at the time of the study. Health consequences such as unwanted pregnancy, unsafe or self-induced abortion and sexually transmitted infections (STIs), including HIV infections were reported by survivors. Moreover, physical, mental and psychological traumas and emotional breakdown, stigma and discrimination were reported as consequences of the sexual and physical violence. These consequences have lifelong impact. 20 21 Thus, treatment of STIs, HIV, abortion care for late pregnancy and care for the child born from rapist, emotional and psychological support from local and international healthcare and humanitarian stakeholders is urgently recommended. Involving family members in counselling with survivors is also an important strategy to reduce conflict, stigma, disagreement and divorce between family and couples. Comprehensive approach in caring for survivors including provision of medical, psychological, social and economic support is critically needed. Therefore, establishing rehabilitation centres to care for survivors at the affected sites and devising local integration strategies are important.
The association of rape with young age and living in the temporary shelter within the community during the war period was evident in this study. The higher the incidence of rape in those groups may be explained due to family breakdown and absence of legal and social protection during crisis. Living in shelter without protection for women is difficult. The higher incidence of rape in urban area can be explained by the higher number of combatants who stayed in urban towns and distribution sites where most wars were taking place. In this study, most of the women in lower status were abused higher (girls/women with no formal education, student and/or unemployed women). This can be explained due to poor awareness of girls/women about combatants and may be disproportionally affected due to low status and poor protection given by family.
The findings of this study should be interpreted in the context of its strengths and limitations. The study has several notable strengths. It is the first population-based study of its kind to be conducted in Tigray following the eruption of the war on SGBV related, while still under severe siege. The study was carefully designed using sampling techniques that ensured adequate sample size randomly selected to represent all parts of Tigray region (except western zone and some northeastern districts occupied by the invaders) to systematically assess critical cross-cutting issues at the community level. Since the survey was conducted immediately after the invading forces left most parts of Tigray and not far from the time of the violence, the likelihood of recall biases is minimal. In the analysis, we considered weighted analysis in the summary statistics to minimise error in estimation of prevalence as result of the sampling procedure used. However, there are some limitations to be considered in using weighting in this study where the population figures are only likely to provide a partial picture during the conflict period as there is so much potential for forced mobility. Thus, calculating weighting by considering the fact that a district/Tabiya/cluster-level population may not show the actual population during the conflict period due to high mobility, even though it should still be valid in relative terms. The fact that the main findings do not change after the use of weighting is a strength and further evidence about the robustness of the findings reported in this study.
The interpretation of the findings should also consider some limitations of the study. Although the use of female local data collectors (ie, HEWs) may have had a positive outcome in creating an environment of safety and comfort for the respondents to disclose information, the use of the HEWs residing in the same place with the victims might also have negatively led to hiding or distortion of information for fear of shame or leak of information. Moreover, the HEWs are government employees in the specific area they may overestimate/underestimate finding. However, adequate orientation, and training was given with close supervision during data collection period. The majority of the western zone of Tigray and some pockets of areas bordering Eritrea were not included since they are currently under control of the Ethiopian and Eritrean forces and still active war areas. Note that the level of SGBV is expected to be more extensively prevalent in these areas where the conflict has been more severe and still ongoing. 20 21 Thus, the findings of this study are likely to be an underestimation of the true extent of SGBV in Tigray as a result of the war. Some data were missing due to reluctance to respond owing to the sensitive nature of the variables. Moreover, internally displaced women living at camps were not included, even though displaced women within the community or living in their own new HHs were included. In general, the number or proportions of recorded sexual violence might be underestimated due to under-reporting of sexual violence from surviving victims and/or lack of information from victims who were murdered or perished due to injuries during or after the assault.
Survivors/Victims generally do not speak of the incident for many reasons, including self-blame, fear of reprisals, mistrust of data collectors, risk/fear of re-victimisation, fear of shaming and blaming, social stigma and often rejection by the survivor/victim’s family and community. However, the findings in this study still indicate levels of SGBV that are substantially and alarmingly high—similar or even higher than reported levels from other conflict areas around the world. 3 16
Conclusions
SGBV was highly prevalent during the first 8 months of the Tigray war. Almost 10% of the girls and women of reproductive age interviewed were sexually abused, mostly by rape. Gang-rape was the most common and frequent form of sexual violence. Physical and psychological forms of violence were common too. Underage girls, elder women and men were also victims of sexual and physical violence. Physical traumas, depression, suicidal attempts, emotional change, unwanted pregnancy, STIs and HIV infection were the most common consequences of the SGBV reported. Ninety per cent of the survivors of sexual violence have not received medical care or psychological care because most health facilities were destroyed and looted. Urgent survivor centre approach with medical and psychological service; and sustained community support are recommended to reduce lifelong impact on behavioural, emotional, sexual, social and economic fortunes of SGBV victims. Of significant importance, there will be a need for further investigation of SGBV among girls and women in rural and urban communities of Tigray including those excluded from this study due to the occupation. Where and when possible, a complete inventory and investigation of every girl and woman of reproductive age in each Tabias/Kebeles is recommended in order to provide a full picture and extent of the SGBV as a result of the war throughout Tigray in order to reach out comprehensively to all victims to ensure they receive short-term and long-term rehabilitation, access to post-trauma services and socio-economic support.
Region-wide tracing of survivors is needed for further medical and psychological support. Because of the complete collapse of the health system in Tigray, the challenges in dealing with the health and psychosocial ramifications from this unprecedented crisis from SGBV in Tigray will require urgent and multisectoral effort by local and international partners.
Ethics statements
Patient consent for publication.
Not applicable.
Ethics approval
Ethical clearance was obtained from the Institutional Review Board (IRB) of the College of Health Sciences at Mekelle University (reference no: MU-IRB1905/2011) and support letters were secured from the Tigray Health Bureau and District Health Offices before the start of the actual data collection. Written consent (for adult women) or assents (for underage girls) were obtained prior to data collection.
- UN general assembly
- Muluneh MD ,
- Francis L , et al
- García-Moreno C ,
- Rashida M ,
- ↵ Guidelines on reporting on sexual violence in conflict . n.d. Available : https://www.coveringcrsv.org/wpcontent/uploads/2021/05/CRSV_Downloadable_UK_FULL.pdf
- Capasso A ,
- Skipalska H ,
- Guttmacher S , et al
- Goessmann K ,
- Ibrahim H ,
- Ellsberg M ,
- Murphy M , et al
- Gingerich T ,
- Kinyanda E ,
- Biryabarema C , et al
- Bartels SA ,
- Mukwege D , et al
- Amowitz LL ,
- Lyons KH , et al
- Betancourt TS ,
- Verhoeven H ,
- Woldemariam M
- EHRC and OHCHR
- Human Rights Watch
- Gesesew H ,
- Berhane K ,
- Siraj ES , et al
- Schraiber LB ,
- Latorre M do RDO ,
- França I , et al
- Kleinbaum D ,
- Nizam A , et al
- Robertson K ,
- Ward J , et al
- Jovanović N , et al
- Elisabeth R ,
- Hossain M , et al
- Steiner B ,
- Benner MT ,
- Sondorp E , et al
- Bastick M ,
Supplementary materials
Supplementary data.
This web only file has been produced by the BMJ Publishing Group from an electronic file supplied by the author(s) and has not been edited for content.
- Data supplement 1
- Data supplement 2
Handling editor Seye Abimbola
Contributors All authors have contributed significantly to the conduct of the study, analysis of the data, writing and interpretation of the manuscript and revision of this submission. GF is the guarator with full responsibility for the work and/or the conduct of the study, had access to the data, and controlled the decision to publish.
Funding The authors have not declared a specific grant for this research from any funding agency in the public, commercial or not-for-profit sectors.
Competing interests None declared.
Patient and public involvement Patients and/or the public were involved in the design, or conduct, or reporting, or dissemination plans of this research. Refer to the 'Methods' section for further details.
Provenance and peer review Not commissioned; externally peer reviewed.
Supplemental material This content has been supplied by the author(s). It has not been vetted by BMJ Publishing Group Limited (BMJ) and may not have been peer-reviewed. Any opinions or recommendations discussed are solely those of the author(s) and are not endorsed by BMJ. BMJ disclaims all liability and responsibility arising from any reliance placed on the content. Where the content includes any translated material, BMJ does not warrant the accuracy and reliability of the translations (including but not limited to local regulations, clinical guidelines, terminology, drug names and drug dosages), and is not responsible for any error and/or omissions arising from translation and adaptation or otherwise.
Read the full text or download the PDF:

IMAGES
VIDEO
COMMENTS
Sampling methods are crucial for conducting reliable research. In this article, you will learn about the types, techniques and examples of sampling methods, and how to choose the best one for your study. Scribbr also offers free tools and guides for other aspects of academic writing, such as citation, bibliography, and fallacy.
Understand sampling methods in research, from simple random sampling to stratified, systematic, and cluster sampling. Learn how these sampling techniques boost data accuracy and representation, ensuring robust, reliable results. Check this article to learn about the different sampling method techniques, types and examples.
Sampling methods in psychology refer to strategies used to select a subset of individuals (a sample) from a larger population, to study and draw inferences about the entire population. Common methods include random sampling, stratified sampling, cluster sampling, and convenience sampling. Proper sampling ensures representative, generalizable, and valid research results.
Sampling methods refer to the techniques used to select a subset of individuals or units from a larger population for the purpose of conducting statistical analysis or research. Sampling is an essential part of the Research because it allows researchers to draw conclusions about a population without having to collect data from every member of ...
There are many types of sampling methods because different research questions and study designs require different approaches to ensure representative and unbiased samples. Using appropriate sampling techniques helps researchers generalize their findings to the broader population and reduces the risk of introducing biases that could invalidate ...
1. Simple random sampling. In a simple random sample, every member of the population has an equal chance of being selected. Your sampling frame should include the whole population. To conduct this type of sampling, you can use tools like random number generators or other techniques that are based entirely on chance.
First, we'll look at four common probability-based (random) sampling methods: Simple random sampling. Stratified random sampling. Cluster sampling. Systematic sampling. Importantly, this is not a comprehensive list of all the probability sampling methods - these are just four of the most common ones.
Researchers often use non-probability sampling methods for exploratory research, pilot studies, and qualitative research. These sampling methods provide quick and rough assessments, help work kinks out of measurement instruments and procedures, and help refine the design for a more rigorous study in the future. Below are several standard non ...
There are two major types of sampling methods: probability and non-probability sampling. Probability sampling, also known as random sampling, is a kind of sample selection where randomization is used instead of deliberate choice. Each member of the population has a known, non-zero chance of being selected.
Abstract. Knowledge of sampling methods is essential to design quality research. Critical questions are provided to help researchers choose a sampling method. This article reviews probability and non-probability sampling methods, lists and defines specific sampling techniques, and provides pros and cons for consideration.
Evaluate your goals against time and budget. List the two or three most obvious sampling methods that will work for you. Confirm the availability of your resources (researchers, computer time, etc.) Compare each of the possible methods with your goals, accuracy, precision, resource, time, and cost constraints.
Sampling Methods Explained: 10 Types of Sampling Methods. When researchers want to gain insight into a large number of people, they use different sampling methods to offer a snapshot of the entire population. When properly planned, these sampling techniques can offer representative samples that can then be extrapolated to a much larger group of ...
in research including Probability sampling techniques, which include simple random sampling, systematic random sampling and strati ed. random sampling and Non-probability sampling, which include ...
Stratified random sample. Definition: Split a population into groups. Randomly select some members from each group to be in the sample. Example: Split up all students in a school according to their grade - freshman, sophomores, juniors, and seniors. Ask 50 students from each grade to complete a survey about the school lunches.
Sampling in market action research is of two types - probability sampling and non-probability sampling. Let's take a closer look at these two methods of sampling. Probability sampling:Probability sampling is a sampling technique where a researcher selects a few criteria and chooses members of a population randomly.
All types of sampling fall into one of these two fundamental categories: Probability sampling: In probability sampling, researchers can calculate the probability of any single person in the population being selected for the study. These studies provide greater mathematical precision and analysis. Nonprobability sampling: In nonprobability ...
Call our friendly, no-pressure support team. +1 801 477 4700. Sampling Methods in Survey Research: Definition, Types, Methods, and More. Differences Between Probability and Non-Probability Sampling. and analysis in marketing research and the social sciences. It involves selecting a subset of individuals from a larger population to participate ...
The main goal of any marketing or statistical research is to provide quality results that are a reliable basis for decision-making. That is why the different types of sampling methods and techniques have a crucial role in research methodology and statistics. Your sample is one of the key factors that determine if your findings are accurate.
As the team writes, "unless population sampling is both intensive and spatiotemporally exhaustive, it can be difficult to establish the upper limits of body size even for extant species." Approximately 2.5 billion T. rexes are thought to have graced our planet in the species' short 2.4 million years, and yet so far we have only 84 reasonably ...
Sampling is the process of selecting a small group of individuals from a larger population. This helps to draw conclusions about that population's needs. ... Our research methods. Persona creation: develop detailed profiles of our key customer segments. Our research archetypes are citizens, businesses, visitors and public servants. Within ...
While bioinformatics reveals patterns in protein sequences and structural biology methods elucidate atomic details of protein structures, it is difficult to attain equally high-resolution energetic information about protein conformational ensembles. We present PIGEON-FEATHER, a method for calculating free energies of opening (∆Gop) at single- or near-single-amino acid resolution for protein ...
Methods A community-based survey was conducted in 52 (out of 84) districts of Tigray, excluding its western zone and some districts bordering Eritrea due to security reasons. Using a two-stage multistage cluster sampling technique, a total of 5171 women of reproductive age (15-49 years) were randomly selected and included in the study.