You are using an outdated browser. Please upgrade your browser to improve your experience.

Constraints on cortical and thalamic projections: the no-strong-loops hypothesis
The many distinct cortical areas of the macaque monkey visual system can be arranged hierarchically, but not in a unique way. We suggest that the connections between these cortical areas never form strong, directed loops. For connections between the visual cortex and particular thalamic nuclei, we predict that certain types of connections will not be found. If strong, directed loops were to exist, we suggest that the cortex would go into uncontrolled oscillations.
Additional Information
Additional details.

An official website of the United States government
The .gov means it’s official. Federal government websites often end in .gov or .mil. Before sharing sensitive information, make sure you’re on a federal government site.
The site is secure. The https:// ensures that you are connecting to the official website and that any information you provide is encrypted and transmitted securely.
- Publications
- Account settings
- My Bibliography
- Collections
- Citation manager
Save citation to file
Email citation, add to collections.
- Create a new collection
- Add to an existing collection
Add to My Bibliography
Your saved search, create a file for external citation management software, your rss feed.
- Search in PubMed
- Search in NLM Catalog
- Add to Search
Constraints on cortical and thalamic projections: the no-strong-loops hypothesis
Affiliation.
- 1 The Salk Institute, La Jolla, California 92037, USA.
- PMID: 9440687
- DOI: 10.1038/34584
The many distinct cortical areas of the macaque monkey visual system can be arranged hierarchically, but not in a unique way. We suggest that the connections between these cortical areas never form strong, directed loops. For connections between the visual cortex and particular thalamic nuclei, we predict that certain types of connections will not be found. If strong, directed loops were to exist, we suggest that the cortex would go into uncontrolled oscillations.
PubMed Disclaimer
Similar articles
- Hierarchical organization of macaque and cat cortical sensory systems explored with a novel network processor. Hilgetag CC, O'Neill MA, Young MP. Hilgetag CC, et al. Philos Trans R Soc Lond B Biol Sci. 2000 Jan 29;355(1393):71-89. doi: 10.1098/rstb.2000.0550. Philos Trans R Soc Lond B Biol Sci. 2000. PMID: 10703045 Free PMC article.
- Visual thalamocortical projections in the flying fox: parallel pathways to striate and extrastriate areas. Manger PR, Rosa MG. Manger PR, et al. Neuroscience. 2005;130(2):497-511. doi: 10.1016/j.neuroscience.2004.09.047. Neuroscience. 2005. PMID: 15664706
- Thalamic connections of the dorsomedial visual area in primates. Beck PD, Kaas JH. Beck PD, et al. J Comp Neurol. 1998 Jul 6;396(3):381-98. J Comp Neurol. 1998. PMID: 9624591
- The functional logic of cortico-pulvinar connections. Shipp S. Shipp S. Philos Trans R Soc Lond B Biol Sci. 2003 Oct 29;358(1438):1605-24. doi: 10.1098/rstb.2002.1213. Philos Trans R Soc Lond B Biol Sci. 2003. PMID: 14561322 Free PMC article. Review.
- Visual cortex: structure and connections. Jones EG. Jones EG. Invest Ophthalmol. 1972 May;11(5):333-7. Invest Ophthalmol. 1972. PMID: 4623890 Review. No abstract available.
- Prior probability cues bias sensory encoding with increasing task exposure. Walsh K, McGovern DP, Dully J, Kelly SP, O'Connell RG. Walsh K, et al. Elife. 2024 Apr 2;12:RP91135. doi: 10.7554/eLife.91135. Elife. 2024. PMID: 38564237 Free PMC article.
- The dorsal lateral geniculate nucleus and the pulvinar as essential partners for visual cortical functions. Casanova C, Chalupa LM. Casanova C, et al. Front Neurosci. 2023 Aug 30;17:1258393. doi: 10.3389/fnins.2023.1258393. eCollection 2023. Front Neurosci. 2023. PMID: 37712093 Free PMC article. Review.
- Canonical circuit computations for computer vision. Schmid D, Jarvers C, Neumann H. Schmid D, et al. Biol Cybern. 2023 Oct;117(4-5):299-329. doi: 10.1007/s00422-023-00966-9. Epub 2023 Jun 12. Biol Cybern. 2023. PMID: 37306782 Free PMC article. Review.
- External drivers of BOLD signal's non-stationarity. Ashourvan A, Pequito S, Bertolero M, Kim JZ, Bassett DS, Litt B. Ashourvan A, et al. PLoS One. 2022 Sep 19;17(9):e0257580. doi: 10.1371/journal.pone.0257580. eCollection 2022. PLoS One. 2022. PMID: 36121808 Free PMC article.
- Conserved patterns of functional organization between cortex and thalamus in mice. Miller-Hansen AJ, Sherman SM. Miller-Hansen AJ, et al. Proc Natl Acad Sci U S A. 2022 May 24;119(21):e2201481119. doi: 10.1073/pnas.2201481119. Epub 2022 May 19. Proc Natl Acad Sci U S A. 2022. PMID: 35588455 Free PMC article.
Publication types
- Search in MeSH
Related information
- Cited in Books
LinkOut - more resources
Full text sources.
- Nature Publishing Group
Other Literature Sources
- The Lens - Patent Citations

- Citation Manager
NCBI Literature Resources
MeSH PMC Bookshelf Disclaimer
The PubMed wordmark and PubMed logo are registered trademarks of the U.S. Department of Health and Human Services (HHS). Unauthorized use of these marks is strictly prohibited.
Europe PMC requires Javascript to function effectively.
Either your web browser doesn't support Javascript or it is currently turned off. In the latter case, please turn on Javascript support in your web browser and reload this page.
Recurrent Long-Range Interactions in Early Vision
- First Online: 01 January 2001
Cite this chapter
- Thorsten Hansen 4 ,
- Wolfgang Sepp 4 &
- Heiko Neumann 4
Part of the book series: Lecture Notes in Computer Science ((LNAI,volume 2036))
A general principle of cortical architecture is the bidirec- tional flow of information along feedforward and feedback connections. In the feedforward path, converging connections mainly define the fea- ture detection characteristics of cells. The computational role of feedback connections, on the contrary, is largely unknown. Based on empirical find- ings we suggest that top-down feedback projections modulate activity of target cells in a context dependent manner. The context is represented by the spatial extension and direction of long-range connections. In this scheme, bottom-up activity which is consistent in a more global context is enhanced, inconsistent activity is suppressed. We present two instantia- tions of this general scheme having complementary functionality, namely a model of cortico-cortical V1-V2 interactions and a model of recurrent intracortical V1 interactions. The models both have long-range interac- tions for the representation of contour shapes and modulating feedback in common. They differ in their response properties to illusory contours and corners, and in the details of computing the bipole filter which models the long-range connections. We demonstrate that the models are capable of basic processing tasks in vision, such as, e.g., contour enhancement, noise suppression and corner detection. Also, a variety of perceptual phe- such as grouping of fragmented shape outline and interpolation nomena of illusory contours can be explained.
This is a preview of subscription content, log in via an institution to check access.
Access this chapter
Subscribe and save.
- Get 10 units per month
- Download Article/Chapter or eBook
- 1 Unit = 1 Article or 1 Chapter
- Cancel anytime
- Available as PDF
- Read on any device
- Instant download
- Own it forever
- Compact, lightweight edition
- Dispatched in 3 to 5 business days
- Free shipping worldwide - see info
Tax calculation will be finalised at checkout
Purchases are for personal use only
Institutional subscriptions
Unable to display preview. Download preview PDF.
Similar content being viewed by others
A recurrent neural model for proto-object based contour integration and figure-ground segregation.
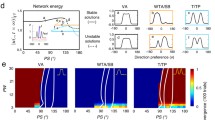
Recurrent network dynamics reconciles visual motion segmentation and integration
A Geometric Model for the Functional Circuits of the Visual Front-End
I. Biederman. Human image understanding: Recent research and a theory. CVGIP , 32(1):29–73, 1985.
Google Scholar
J. Bolz, C.D. Gilbert, and T. Wiesel. Pharmacological analysis of cortical circuitry. TINS , 12(8):292–296, 1989.
J. Bullier, M.E. McCourt, and G.H. Henry. Physiological studies on the feedback connection to the striate cortex from cortical areas 18 and 19 of the cat. Exp. Brain Res. , 70:90–98, 1988.
F. Crick and C. Koch. Why is the a hierarchy of visual cortical and thalamic areas: The no-strong loops hypothesis. Nature , 391:245–250, 1998.
Article Google Scholar
A. Das and C. Gilbert. Topography of contextual modulations mediated by short-range interactions in primary visual cortex. Nature , 399:655–661, 1999.
D.J. Field, A. Hayes, and R.F. Hess. Contour integration by the human visual system: Evidence for local “association field”. Vision Res. , 33(2):173–193, 1993.
L.H. Finkel and G.M. Edelman. Integration of distributed cortical systems by reentry: A computer simulation of interactive functionally segregated visual areas. J. Neurosci. , 9(9):3188–3208, 1989.
L.H. Finkel and P. Sajda. Object discrimination based on depth-from-occlusion. Neural Comput. , 4:901–921, 1992.
C. Gilbert. Horizontal integration and cortical dynamics. Neuron , 9:1–13, 1992.
C.D. Gilbert. Circuitry, architecture, and functional dynamics of visual cortex. Cereb. Cortex , 3(5):373–386, Sep/Oct 1993.
C.D. Gilbert and T.N. Wiesel. Clustered intrinsic connections in cat visual cortex. J. Neurosci. , 3:1116–1133, 1983.
C.D. Gilbert and T.N. Wiesel. Columnar specificity of intrinsic horizontal and corticocortical connections in cat visual cortex. J. Neurosci. , 9(7):2432–2442, 1989.
A. Gove, S. Grossberg, and E. Mingolla. Brightness perception, illusory contours and corticogeniculate feedback. In Proc. World Conference on Neural Networks (WCNN-93) , Vol. I-IV , pages (I) 25–28, Portland (Oreg./USA), July 11-15 1993.
S. Grossberg. How does a brain build a cognitive code? Psych. Rev. , 87:1–51, 1980.
S. Grossberg and E. Mingolla. Neural dynamics of perceptual grouping: Textures, boundaries, and emergent segmentation. Percept. Psychophys. , 38:141–171, 1985.
S. Grossberg, E. Mingolla, and W.D. Ross. Visual brain and visual perception: how does the cortex do perceptual grouping? TINS , 20(3):106–111, 1997.
T. Hansen and H. Neumann. A model of V1 visual contrast processing utilizing long-range connections and recurrent interactions. In Proc. ICANN , pages 61–66, Edinburgh, UK, Sept. 7-10 1999.
F. Heitger, R. von der Heydt, E. Peterhans, L. Rosenthaler, and O. Kübler. Simulation of neural contour mechanisms: Representing anomalous contours. Image Vis. Comp. , 16:407–421, 1998.
J.A. Hirsch and C.D. Gilbert. Synaptic physiology of horizontal connections in the cat’s visual cortex. J. Neurosci. , 11(6):1800–1809, June 1991.
J.M. Hupé, A.C. James, B.R. Payne, S.G. Lomber, P. Girard, and J. Bullier. Cortical feedback improves discrimination between figure and background by V1, V2 and V3 neurons. Nature , 394:784–787, Aug. 1998.
G. Kanizsa. Percezione attuale, esperienza passata l‘“esperimento impossible”. In G. Kanizsa and G. Vicario, editors, Ricerche sperimentali sulla percezione. , pages 9–47. Universitá degli studi, Triente, 1968.
G. Kanizsa. Subjective contours. Sci. Am. , 234(4):48–52, 1976.
M.K. Kapadia, M. Ito, C.D. Gilbert, and G. Westheimer. Improvement in visual sensitivity by changes in local context: Parallel studies in human observers and in V1 of alert monkeys. Neuron , 15:843–856, Oct. 1995.
J.J. Knierim and D.C. Van Essen. Neuronal responses to static texture patterns in area V1 of the alert macaque monkey. J. Neurophys. , 67(4):961–980, 1992.
G.W. Lesher and E. Mingolla. The role of edges and line-ends in illusory contour formation. Vision Res. , 33(16):2253–2270, 1993.
Z. Li. Pre-attentive segmentation in the primary visual cortex. M.I.T. A.I. Lab., Memo No. 1640, 1998.
M. Livingstone and D. Hubel. Anatomy and physiology of a color system in the primate visual cortex. J. Neurosci. , 4(1):309–356, 1984.
D. Mumford. On the computational architecture of the neocortex II: The role of cortico-cortical loops. Biol. Cybern. , 65:241–251, 1991.
H. Neumann and W. Sepp. Recurrent V1-V2 interaction in early visual boundary processing. Biol. Cybern ., 81:425–444, 1999.
E. Peterhans and R. von der Heydt. Mechanisms of contour perception in monkey visual cortex. II. Contours bridging gaps. J. Neurosci. , 9(5):1749–1763, 1989.
E. Peterhans and R. von der Heydt. Subjective contours-bridging the gap between psychophysics and physiology. TINS , 14(3):112–119, 1991.
U. Polat and D. Sagi. The arcitecture of perceptual spatial interactions. Vision Res. , 34:73–78, 1994.
K. Prazdny. Illusory contours are not caused by simultaneous brightness contrast. Percept. Psychophys. , 34(4):403–404, 1983.
K. Rockland and A. Virga. Terminal arbors of individual “feedback” axons projecting from area V2 to V1 in the macaque monkey: A study using immunohistochemistry of anterogradely transported Phaseolus vulgaris-lencoagglunitin. J. Comp. Neurol. , 285:54–72, 1989.
P.-A. Salin and J. Bullier. Corticocortical connections in the visual system: Structure and function. Physiol. Rev. , 75(1):107–154, 1995.
J. Sandell and P. Schiller. Effect of cooling area 18 on striate cortex cells in the squirrel monkey. J. Neurophys. , 48(1):38–48, 1982.
K. Schmidt, R. Goebel, S. Löwel, and W. Singer. The perceptual grouping criterion of colinearity is reflected by anisotropies of connections in the primary visual cortex. Europ. J. Neurosci. , 9:1083–1089, 1997.
T.F. Shipley and P.J. Kellman. The role of discontinuities in the perception of subjective figures. Percept. Psychophys. , 48(3):259–270, 1990.
T.F. Shipley and P.J. Kellman. Strength of visual interpolation depends on the ratio of physically specified to total edge length. Percept. Psychophys. , 52(1):97–106, 1992.
S. Ullman. Sequence of seeking and counter streams: A computational model for bidirectional information flow in the visual cortex. Cereb. Cortex , 2:310–335, 1995.
R. von der Heydt, F. Heitger, and E. Peterhans. Perception of occluding contours: Neural mechanisms and a computational model. Biomed. Res. , 14:1–6, 1993.
R. von der Heydt and E. Peterhans. Mechanisms of contour perception in monkey visual cortex. I. Lines of pattern discontinuity. J. Neurosci. , 9(5):1731–1748, 1989.
R. von der Heydt, E. Peterhans, and G. Baumgartner. Illusory contours and cortical neuron responses. Science , 224:1260–1262, 1984.
Download references
Author information
Authors and affiliations.
Abt. Neuroinformatik, Universität Ulm, D-89069, Ulm, Germany
Thorsten Hansen, Wolfgang Sepp & Heiko Neumann
You can also search for this author in PubMed Google Scholar
Editor information
Editors and affiliations.
Centre of Informatics, SCET, University of Sunderland, St PetersWay, Sunderland SR6 0DD, UK
Stefan Wermter
Department of Computer Science, University of York, York, Y010 5DD, UK
Institute for Adaptive and Neural Computation, University of Edinburgh, 5 Forrest Hill, Edinburgh, UK
David Willshaw
Rights and permissions
Reprints and permissions
Copyright information
© 2001 Springer-Verlag Berlin Heidelberg
About this chapter
Hansen, T., Sepp, W., Neumann, H. (2001). Recurrent Long-Range Interactions in Early Vision. In: Wermter, S., Austin, J., Willshaw, D. (eds) Emergent Neural Computational Architectures Based on Neuroscience. Lecture Notes in Computer Science(), vol 2036. Springer, Berlin, Heidelberg. https://doi.org/10.1007/3-540-44597-8_9
Download citation
DOI : https://doi.org/10.1007/3-540-44597-8_9
Published : 24 July 2001
Publisher Name : Springer, Berlin, Heidelberg
Print ISBN : 978-3-540-42363-8
Online ISBN : 978-3-540-44597-5
eBook Packages : Springer Book Archive
Share this chapter
Anyone you share the following link with will be able to read this content:
Sorry, a shareable link is not currently available for this article.
Provided by the Springer Nature SharedIt content-sharing initiative
- Publish with us
Policies and ethics
- Find a journal
- Track your research
Last updated 2nd August 2024: Online ordering is currently unavailable due to technical issues. As we resolve the issues resulting from this, we are also experiencing some delays to publication. We are working hard to restore services as soon as possible and apologise for the inconvenience. For further updates please visit our website https://www.cambridge.org/news-and-insights/technical-incident
We use cookies to distinguish you from other users and to provide you with a better experience on our websites. Close this message to accept cookies or find out how to manage your cookie settings .
Login Alert

- > Journals
- > Behavioral and Brain Sciences
- > Volume 30 Issue 5-6
- > Phenomenology without conscious access is a form of...
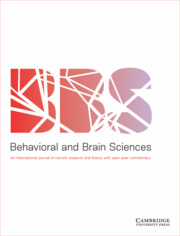
Article contents
Phenomenology without conscious access is a form of consciousness without top-down attention.
Published online by Cambridge University Press: 27 March 2008
We agree with Block's basic hypothesis postulating the existence of phenomenal consciousness without cognitive access. We explain such states in terms of consciousness without top-down, endogenous attention and speculate that their correlates may be a coalition of neurons that are consigned to the back of cortex, without access to working memory and planning in frontal cortex.
Access options
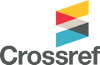
This article has been cited by the following publications. This list is generated based on data provided by Crossref .
- Google Scholar
View all Google Scholar citations for this article.
Save article to Kindle
To save this article to your Kindle, first ensure [email protected] is added to your Approved Personal Document E-mail List under your Personal Document Settings on the Manage Your Content and Devices page of your Amazon account. Then enter the ‘name’ part of your Kindle email address below. Find out more about saving to your Kindle .
Note you can select to save to either the @free.kindle.com or @kindle.com variations. ‘@free.kindle.com’ emails are free but can only be saved to your device when it is connected to wi-fi. ‘@kindle.com’ emails can be delivered even when you are not connected to wi-fi, but note that service fees apply.
Find out more about the Kindle Personal Document Service.
- Volume 30, Issue 5-6
- Christof Koch (a1) and Naotsugu Tsuchiya (a2)
- DOI: https://doi.org/10.1017/S0140525X07002907
Save article to Dropbox
To save this article to your Dropbox account, please select one or more formats and confirm that you agree to abide by our usage policies. If this is the first time you used this feature, you will be asked to authorise Cambridge Core to connect with your Dropbox account. Find out more about saving content to Dropbox .
Save article to Google Drive
To save this article to your Google Drive account, please select one or more formats and confirm that you agree to abide by our usage policies. If this is the first time you used this feature, you will be asked to authorise Cambridge Core to connect with your Google Drive account. Find out more about saving content to Google Drive .
Reply to: Submit a response
- No HTML tags allowed - Web page URLs will display as text only - Lines and paragraphs break automatically - Attachments, images or tables are not permitted

Your details
Your email address will be used in order to notify you when your comment has been reviewed by the moderator and in case the author(s) of the article or the moderator need to contact you directly.
You have entered the maximum number of contributors
Conflicting interests.
Please list any fees and grants from, employment by, consultancy for, shared ownership in or any close relationship with, at any time over the preceding 36 months, any organisation whose interests may be affected by the publication of the response. Please also list any non-financial associations or interests (personal, professional, political, institutional, religious or other) that a reasonable reader would want to know about in relation to the submitted work. This pertains to all the authors of the piece, their spouses or partners.
- DOI: 10.1038/nn0203-119
- Corpus ID: 13960489
A framework for consciousness
- F. Crick , C. Koch
- Published in Nature Neuroscience 1 February 2003
1,337 Citations
Open and closed loops: a computational approach to attention and consciousness, 2 1 where to look for the physical basis of phenomenal experience, consciousness: theories and models, current brain based models of consciousness, consciousness, brains and the replica problem, consciousness: converging insights from connectionist modeling and neuroscience.
- Highly Influenced
How can we construct a science of consciousness?
A cross-order integration hypothesis for the neural correlate of consciousness, biology of consciousness, mathematics and the brain: a category theoretical approach to go beyond the neural correlates of consciousness, 62 references, the unconscious homunculus, towards a cognitive neuroscience of consciousness: basic evidence and a workspace framework, consciousness and the computational mind, are we explaining consciousness yet, the quest for consciousness : a neurobiological approach, the zombie within, consciousness and neuroscience., synchrony unbound a critical evaluation of the temporal binding hypothesis, constraints on cortical and thalamic projections: the no-strong-loops hypothesis, the remembered present: a biological theory of consciousness, related papers.
Showing 1 through 3 of 0 Related Papers
Zoology and wildlife conservation
The zombie within
Article Abstract:
Direct conscious input is not required for a range of complex but routine tasks in many mammalian brain systems. This unconscious, or zombie, behaviour involves parts of the brain that can be characterized as online systems. These systems act rapidly, outpacing consciousness.
User Contributions:
Comment about this article or add new information about this topic:
Cortical areas in visual awareness
Two scientists have proposed that visual perception involving the V1 area of the cortex does not produce visual awareness as shown by people who respond to visual cues that they do not consciously see. However, this hypothesis is unproved and would be too complex to prove, though an experiment to disprove the hypothesis would require only that some V1 neurons fire in conjunction with higher cortical areas when visually stimulated.
Constraints on cortical and thalamic projections: The no-strong-loops hypothesis
The macaque cerebral cortex has several visual areas which have been arranged in a rough hierarchy by Felleman and Van Essen. It is now suggested that links between cortical areas do not form strong, directed loops. It is predicted that some types of link will not be found and that if strong, directed loops did exist, the cortex could undergo uncontrolled oscillations.
- Abstracts: Structure and ligand recognition of the phosphotyrosine binding domain of Shc
- Abstracts: Three key residues form a critical contact network in a protein folding transition state. Simultaneous determination of protein structure and dynamics
- Abstracts: Acoustic signalling of hunger and thermal state by nestling tree swallows. False alarms and begging in nestling birds
- Abstracts: Role of H5 domain in determining pore diameter and ion permeation through cyclic nucleotide-gated channels. Molecular mechanism of cAMP modulation of HCN pacemaker channels
Log in to add people & connections, or click here to create an account .
Already have an account? Log in here.
(Don't worry, your e-mail will not be distributed or made public. Its only purpose is for us to contact you about your account.)
- Recent Additions
- Sign In/Register

Francis Harry Compton Crick - Publications
, La Jolla, CA, United States |
90 high-probability publications. We are testing a new system for linking publications to authors. You can help! If you notice any inaccuracies, please sign in and mark papers as correct or incorrect matches. If you identify any major omissions or other inaccuracies in the publication list, please let us know .
Year | Citation | Score | |||
---|---|---|---|---|---|
2013 | , Wyman J. A footnote on allostery. . 425: 1500-8. PMID DOI: | 0.326 | |||
2008 | Eichmann K, Avery OT, , Von Behring E, , Bloor D, Bordet JJBV, Bona CA, Boyse EA, Burnet FM, Cantor H, Cazenave PA, Claman HN, Cohn M, Coutinho A, ... , et al. The network collective: Rise and fall of a scientific paradigm . 1-274. DOI: | 0.699 | |||
2007 | , . Molecular structure of nucleic acids: a structure for deoxyribose nucleic acid. . 462: 3-5. PMID DOI: | 0.682 | |||
2004 | , , , . Consciousness and neurosurgery. . 55: 273-281; discussion . PMID DOI: | 0.44 | |||
2003 | , . Molecular structure of nucleic acids. A structure for deoxyribose nucleic acid. 1953. . 55: 108-9. PMID | 0.657 | |||
2003 | , . Molecular structure of nucleic acids: a structure for deoxyribose nucleic acid. . 160: 623-4. PMID DOI: | 0.657 | |||
2003 | , . Molecular structure of nucleic acids. A structure for deoxyribose nucleic acid. 1953. . 138: 581-2. PMID DOI: | 0.682 | |||
2003 | , . A structure for deoxyribose nucleic acid. 1953. . 421: 397-8; discussion 39. PMID | 0.654 | |||
2003 | , . A framework for consciousness. . 6: 119-26. PMID DOI: | 0.458 | |||
2002 | . Max Ferdinand Perutz . 55: 62-63. DOI: | 0.298 | |||
2001 | , . The zombie within. . 411: 893. PMID DOI: | 0.406 | |||
1999 | . The impact of molecular biology on neuroscience. . 354: 2021-2025. PMID DOI: | 0.343 | |||
1998 | , . Consciousness and neuroscience. . 8: 97-107. PMID DOI: | 0.437 | |||
1998 | , . Constraints on cortical and thalamic projections: the no-strong-loops hypothesis. . 391: 245-50. PMID DOI: | 0.443 | |||
1996 | . Visual perception: rivalry and consciousness . 379: 485-486. PMID DOI: | 0.33 | |||
1995 | , . Are we aware of neural activity in primary visual cortex? . 375: 121-3. PMID DOI: | 0.44 | |||
1995 | , . Molecular structure of nucleic acids. A structure for deoxyribose nucleic acid. 1953. . 758: 13-4. PMID DOI: | 0.657 | |||
1995 | , Mitchison G. REM sleep and neural nets . 69: 147-155. PMID DOI: | 0.32 | |||
1995 | , . Cortical areas in visual awareness . 377: 294-295. DOI: | 0.428 | |||
1994 | Stewart AB, . The Astonishing Hypothesis: The Scientific Search for the Soul . 52: 533. DOI: | 0.316 | |||
1993 | , . Genetical implications of the structure of deoxyribonucleic acid. 1953. . 269: 1967-9. PMID DOI: | 0.657 | |||
1993 | , . Molecular structure of nucleic acids. A structure for deoxyribose nucleic acid. 1953. . 269: 1966-7. PMID DOI: | 0.657 | |||
1993 | , Jones E. Backwardness of human neuroanatomy . 361: 109-110. PMID DOI: | 0.305 | |||
1993 | . Looking backwards: a birthday card for the double helix. . 135: 15-18. PMID DOI: | 0.433 | |||
1993 | Orgel LE, . Anticipating an RNA world. Some past speculations on the origin of life: where are they today? . 7: 238-9. PMID DOI: | 0.331 | |||
1992 | , . Some reflections on visual awareness. . 55: 953-62. PMID DOI: | 0.428 | |||
1992 | , . The problem of consciousness. . 267: 152-159. PMID DOI: | 0.42 | |||
1991 | , . Understanding awareness at the neuronal level . 14: 683-685. DOI: | 0.437 | |||
1989 | . The recent excitement about neural networks . 337: 129-132. PMID DOI: | 0.336 | |||
1988 | . Neural networks and REM sleep. . 8: 531-535. PMID DOI: | 0.323 | |||
1984 | . Function of the thalamic reticular complex: the searchlight hypothesis. . 81: 4586-90. PMID DOI: | 0.326 | |||
1984 | . Memory and molecular turnover . 312: 101. PMID DOI: | 0.311 | |||
1984 | . Neurobiology: Memory and molecular turnover . 312: 101. DOI: | 0.202 | |||
1983 | , Mitchison G. The function of dream sleep . 304: 111-114. PMID DOI: | 0.306 | |||
1982 | . DNA today . 25: 512-517. PMID | 0.256 | |||
1982 | Mitchison G, . Long axons within the striate cortex: their distribution, orientation, and patterns of connection. . 79: 3661-5. PMID DOI: | 0.331 | |||
1980 | Orgel LE, , Sapienza C. Selfish DNA. . 288: 645-6. PMID | 0.255 | |||
1980 | Orgel LE, . Selfish DNA: the ultimate parasite. . 284: 604-7. PMID DOI: | 0.367 | |||
1980 | Bauer WR, , White JH. Supercoiled DNA. . 243: 100-13. PMID | 0.254 | |||
1979 | , , Bauer WR. Is DNA really a double helix? . 129: 449-57. PMID DOI: | 0.38 | |||
1979 | Prunell A, , Lutter L, , , . Periodicity of deoxyribonuclease I digestion of chromatin. . 204: 855-8. PMID DOI: | 0.675 | |||
1979 | . Crick looks back on DNA . 206: 667. PMID | 0.257 | |||
1979 | . Split genes and RNA splicing . 204: 264-271. PMID DOI: | 0.371 | |||
1979 | . Thinking about the brain. . 241: 219-32. PMID DOI: | 0.303 | |||
1978 | . Chromosome structure and function. Future prospects. . 83: 1-3. PMID DOI: | 0.374 | |||
1977 | Bak AL, Zeuthen J, . Higher-order structure of human mitotic chromosomes. . 74: 1595-9. PMID DOI: | 0.369 | |||
1976 | . Linking numbers and nucleosomes. . 73: 2639-43. PMID DOI: | 0.396 | |||
1976 | , , , . A speculation on the origin of protein synthesis. . 7: 389-97. PMID DOI: | 0.754 | |||
1975 | , . Kinky helix. . 255: 530-3. PMID | 0.478 | |||
1975 | , Lawrence PA. Compartments and polyclones in insect development. . 189: 340-7. PMID DOI: | 0.302 | |||
1974 | . The double helix: A personal view . 248: 766-769. PMID DOI: | 0.381 | |||
1974 | , . Molecular structure of nucleic acids: a structure for deoxyribose nucleic acid. J.D. Watson and F.H.C. Crick. Published in Nature, number 4356 April 25, 1953. . 248: 765. PMID DOI: | 0.629 | |||
1972 | Lawrence PA, , Munro M. A gradient of positional information in an insect, Rhodnius. . 11: 815-53. PMID | 0.175 | |||
1971 | Munro M, . The time needed to set up a gradient: detailed calculations. . 25: 439-53. PMID | 0.187 | |||
1971 | . Central dogma of mollecular biology | Tsentral'naia dogma molekuliarnoǐ biologii . 13: 906-910. PMID | 0.227 | |||
1971 | . General model for the chromosomes of higher organisms . 234: 25-27. PMID DOI: | 0.378 | |||
1971 | . The scale of pattern formation. . 25: 429-38. PMID | 0.184 | |||
1970 | . DNA: test of structure? . 167: 1694. PMID DOI: | 0.396 | |||
1970 | . Diffusion in embryogenesis . 225: 420-422. PMID DOI: | 0.304 | |||
1970 | . Molecular biology in the year 2000 . 228: 613-615. PMID DOI: | 0.33 | |||
1970 | . Central dogma of molecular biology . 227: 561-563. PMID DOI: | 0.397 | |||
1969 | . Molecular biology and medical research. . 36: 178-88. PMID | 0.199 | |||
1968 | . The origin of the genetic code. . 38: 367-79. PMID DOI: | 0.456 | |||
1968 | . On running a summer school . 220: 1275-1276. DOI: | 0.29 | |||
1967 | , . The absolute sign of certain phase-shift mutants in bacteriophage T4. . 26: 361-3. PMID DOI: | 0.588 | |||
1967 | , Barnett L, Katz ER, . UGA: a third nonsense triplet in the genetic code. . 213: 449-50. PMID DOI: | 0.68 | |||
1967 | . An error in model building. . 213: 798. PMID DOI: | 0.363 | |||
1967 | . The Croonian lecture, 1966. The genetic code. . 167: 331-47. PMID DOI: | 0.496 | |||
1966 | . Codon--anticodon pairing: the wobble hypothesis. . 19: 548-55. PMID DOI: | 0.395 | |||
1966 | . The genetic code. 3. . 215: 55-60 passim. PMID DOI: | 0.384 | |||
1966 | . The genetic code--yesterday, today, and tomorrow. . 31: 1-9. PMID DOI: | 0.49 | |||
1965 | . Recent research in molecular biology: introduction. . 21: 183-6. PMID DOI: | 0.334 | |||
1964 | , ORGEL LE. THE THEORY OF INTER-ALLELIC COMPLEMENTATION. . 8: 161-5. PMID DOI: | 0.298 | |||
1963 | . [ON THE GENETIC CODE. CONCLUSIONS ON THE GENERAL NATURE OF THE CODE RESULTING FROM BIOCHEMICAL EXPERIMENTS]. . 8: 529-35. PMID | 0.286 | |||
1963 | . On the genetic code. . 139: 461-4. PMID DOI: | 0.384 | |||
1962 | . The genetic code. . 207: 66-74. PMID DOI: | 0.504 | |||
1961 | , . The molecular structure of collagen. . 3: 483-506. PMID DOI: | 0.517 | |||
1961 | , BARNETT L, , WATTS-TOBIN RJ. General nature of the genetic code for proteins. . 192: 1227-32. PMID DOI: | 0.64 | |||
1961 | , , , . The molecular structure of polyadenylic acid. . 3: 71-86. PMID DOI: | 0.684 | |||
1959 | . Biochemical activities of nucleic acids. The present position of the coding problem. . 12: 35-9. PMID | 0.345 | |||
1958 | . On protein synthesis. . 12: 138-63. PMID | 0.22 | |||
1957 | , Griffith JS, Orgel LE. CODES WITHOUT COMMAS. . 43: 416-21. PMID DOI: | 0.354 | |||
1956 | , . Structure of small viruses. . 177: 473-5. PMID DOI: | 0.644 | |||
1955 | , . The structure of collagen. . 176: 915-6. PMID DOI: | 0.547 | |||
1955 | , . Structure of polyglycine II. . 176: 780-1. PMID DOI: | 0.573 | |||
1954 | . THE COMPLEMENTARY STRUCTURE OF DNA. . 40: 756-8. PMID DOI: | 0.412 | |||
1953 | , . The structure of DNA. . 18: 123-31. PMID DOI: | 0.64 | |||
1953 | , . Genetical implications of the structure of deoxyribonucleic acid. . 171: 964-7. PMID DOI: | 0.645 | |||
1953 | , . Molecular structure of nucleic acids; a structure for deoxyribose nucleic acid. . 171: 737-8. PMID DOI: | 0.684 | |||
1952 | . Is alpha-keratin a coiled coil? . 170: 882-3. PMID DOI: | 0.347 | |||
Thank you for visiting nature.com. You are using a browser version with limited support for CSS. To obtain the best experience, we recommend you use a more up to date browser (or turn off compatibility mode in Internet Explorer). In the meantime, to ensure continued support, we are displaying the site without styles and JavaScript.
- View all journals
- Explore content
- About the journal
- Publish with us
- Sign up for alerts
- Published: 05 October 2022
Meeting report for the 2022 UC Irvine Center for neural circuit mapping conference: linking brain function to cell types and circuits
- Steven F. Grieco 1 ,
- Todd C. Holmes ORCID: orcid.org/0000-0001-8152-8832 2 , 3 &
- Xiangmin Xu ORCID: orcid.org/0000-0002-5828-1533 1 , 3
Molecular Psychiatry volume 28 , pages 2–3 ( 2023 ) Cite this article
312 Accesses
1 Citations
Metrics details
- Molecular biology
- Neuroscience
Emerging evidence suggests that brain circuits can be therapeutically targeted to treat neurological, neuropsychiatric and neurodevelopmental disorders. To develop such therapeutic approaches in the clinic with precision, the targeted cell types and neural circuitry must be characterized with the brain function served. One successful recent approach is to identify cell type-specific circuits using genomic and neural tracing technologies along with experimental tests of their function. Despite remarkable progress on this front, there is still much work to be done. This motivated the program for the 2nd annual Center for Neural Circuit Mapping (CNCM) summer conference held in Irvine, California in August 2022. The meeting considered the strengths and weaknesses of the current state-of-the-art approaches and concepts for linking brain function to structure. The following topics were discussed by twenty-one invited world-class neuroscientists: (1) Cell-type specific analysis of gene regulatory networks in neural circuits, (2) Cortical cell types and circuit function, (3) Local and global neural circuits for navigation and learning, and (4) Linking cell types and circuits to disease. This stimulating CNCM conference series will continue as a productive venue for future neuroscience discussions concerning neural circuit organization and function.
A distinguished and multidisciplinary group of scientists and clinicians met in an air-conditioned and well-caffeinated venue at the National Academies of Sciences Beckman Center, first conceived by Dr. Arnold O. Beckman, the inventor of a pH meter precursor instrument and many other workhorse scientific instruments. Arnold Beckman envisioned a West Coast center comparable to the National Academies of Sciences Conference Center in the District of Columbia (D.C.), where experts would discuss the most pressing matters of science and solve big problems. The roughly one-hundred and twenty attendees participated towards the common goals of the conference including addressing the hurdles of (1) “explainability” for testing cause and effect across different levels of biological organization of the brain [ 2 , 3 ], and (2) how best to link brain function to cell types and circuits given the current state-of-the-art [ 4 , 5 , 6 ].
This is a preview of subscription content, access via your institution
Access options
Subscribe to this journal
Receive 12 print issues and online access
251,40 € per year
only 20,95 € per issue
Buy this article
- Purchase on Springer Link
- Instant access to full article PDF
Prices may be subject to local taxes which are calculated during checkout
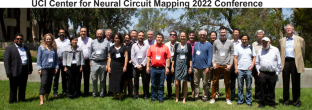
Whitlock DG. The Cajal Club: Its origin, originator and benefactor, Wendell J. S. Krieg. Brain Res Rev. 2007;55:450–62. https://doi.org/10.1016/j.brainresrev.2007.01.006 .
Article Google Scholar
Bassett DS, Gazzaniga MS. Understanding complexity in the human brain. Trends Cogn Sci. 2011;15:200–9. https://doi.org/10.1016/j.tics.2011.03.006 .
Bassett DS, Sporns O. Network neuroscience. Nat Neurosci. 2017;20:353–64. https://doi.org/10.1038/nn.4502 .
Article CAS Google Scholar
Xu X, Holmes TC, Luo M-H, Beier KT, Horwitz GD, Zhao F. et al. Viral vectors for neural circuit mapping and recent advances in trans-synaptic anterograde tracers. Neuron. 2020;107:1029–47. https://doi.org/10.1016/j.neuron.2020.07.010 .
Nectow AR, Nestler EJ. Viral tools for neuroscience. Nat Rev Neurosci. 2020;21:669–81. https://doi.org/10.1038/s41583-020-00382-z .
Genon S, Reid A, Langner R, Amunts K, Eickhoff SB. How to characterize the function of a brain region. Trends Cogn Sci. 2018;22:350–64. https://doi.org/10.1016/j.tics.2018.01.010 .
Wickersham IR, Lyon DC, Barnard RJ, Mori T, Finke S, Conzelmann KK. et al. Monosynaptic restriction of transsynaptic tracing from single, genetically targeted neurons. Neuron. 2007;53:639–47. https://doi.org/10.1016/j.neuron.2007.01.033 .
Gouwens NW, Sorensen SA, Baftizadeh F, Budzillo A, Lee BR, Jarsky T. et al. Integrated morphoelectric and transcriptomic classification of cortical GABAergic cells. Cell. 2020;183:935–953.e919. https://doi.org/10.1016/j.cell.2020.09.057 .
Kim EJ, Zhang Z, Huang L, Ito-Cole T, Jacobs MW, Juavinett AL. et al. Extraction of distinct neuronal cell types from within a genetically continuous population. Neuron. 2020;107:274–282.e276. https://doi.org/10.1016/j.neuron.2020.04.018 .
Kirchgessner MA, Franklin AD & Callaway EM. Distinct “driving” versus “modulatory” influences of different visual corticothalamic pathways. bioRxiv. 2021.2003.2030.437715. https://doi.org/10.1101/2021.03.30.437715 (2021).
Kirchgessner MA, Franklin AD & Callaway EM. Context-dependent and dynamic functional influence of corticothalamic pathways to first- and higher-order visual thalamus. bioRxiv. 738203. https://doi.org/10.1101/738203 (2020).
Yoshimura Y, Callaway EM. Fine-scale specificity of cortical networks depends on inhibitory cell type and connectivity. Nat Neurosci. 2005;8:1552–9. https://doi.org/10.1038/nn1565 .
Crick F, Koch C. Constraints on cortical and thalamic projections: the no-strong-loops hypothesis. Nature. 1998;391:245–50. https://doi.org/10.1038/34584 .
Luo L. Architectures of neuronal circuits. Science. 2021;373:eabg7285. https://doi.org/10.1126/science.abg7285 .
Download references
Acknowledgements
We acknowledge and thank the conference organizers, Edward Callaway, TCH, Kim Green, Douglas A. Nitz, Bing Ren, Bert Semler and XX and our Center Staff Michelle Wu and Ginny Wu.
Author information
Authors and affiliations.
Department of Anatomy and Neurobiology, School of Medicine, University of California, Irvine, CA, USA
Steven F. Grieco & Xiangmin Xu
Department of Physiology and Biophysics, School of Medicine, University of California, Irvine, CA, USA
- Todd C. Holmes
Center for Neural Circuit Mapping (CNCM), University of California, Irvine, CA, 92697, USA
Todd C. Holmes & Xiangmin Xu
You can also search for this author in PubMed Google Scholar
Contributions
SFG, TCH, and XX prepared the figure and wrote the report.
Corresponding author
Correspondence to Xiangmin Xu .
Ethics declarations
Competing interests.
This conference received partial corporate support from sponsors AAVnerGene and Vizgen.
Additional information
Publisher’s note Springer Nature remains neutral with regard to jurisdictional claims in published maps and institutional affiliations.
Rights and permissions
Reprints and permissions
About this article
Cite this article.
Grieco, S.F., Holmes, T.C. & Xu, X. Meeting report for the 2022 UC Irvine Center for neural circuit mapping conference: linking brain function to cell types and circuits. Mol Psychiatry 28 , 2–3 (2023). https://doi.org/10.1038/s41380-022-01810-5
Download citation
Published : 05 October 2022
Issue Date : January 2023
DOI : https://doi.org/10.1038/s41380-022-01810-5
Share this article
Anyone you share the following link with will be able to read this content:
Sorry, a shareable link is not currently available for this article.
Provided by the Springer Nature SharedIt content-sharing initiative
This article is cited by
Meeting report for the 2023 uc irvine center for neural circuit mapping conference: structure, function and development of neural circuits.
- Steven F. Grieco
- Xiangmin Xu
Molecular Psychiatry (2023)
Quick links
- Explore articles by subject
- Guide to authors
- Editorial policies


An official website of the United States government
The .gov means it’s official. Federal government websites often end in .gov or .mil. Before sharing sensitive information, make sure you’re on a federal government site.
The site is secure. The https:// ensures that you are connecting to the official website and that any information you provide is encrypted and transmitted securely.
- Publications
- Account settings
Preview improvements coming to the PMC website in October 2024. Learn More or Try it out now .
- Advanced Search
- Journal List
- v.27(20); 2007 May 16
A Fast, Reciprocal Pathway between the Lateral Geniculate Nucleus and Visual Cortex in the Macaque Monkey
Farran briggs.
Center for Neuroscience, University of California, Davis, Davis, California 95618
W. Martin Usrey
Neurons in the lateral geniculate nucleus (LGN) not only provide feedforward input to primary visual cortex (V1), but also receive robust feedback from the cortex. Accordingly, visual processing in the LGN is continuously influenced by previous patterns of activity. This study examines the temporal properties of feedforward and feedback pathways between the LGN and V1 in the macaque monkey to provide a lower bound on how quickly the cortex can influence the LGN. In so doing, we identified a subclass of corticogeniculate neurons that receives direct, suprathreshold input from the LGN that is similar in latency to that directed to other recipient neurons (4.2 ± 0.4 vs 4.0 ± 0.2 ms). These neurons also provide feedback to the LGN that is significantly shorter in latency than that supplied by corticogeniculate neurons lacking LGN input (5.1 ± 1.3 vs 11.1 ± 2.3 ms, respectively). Across our sample of corticogeniculate neurons, the shortest combined visual response latency and feedback latency was 37 ms (mean, 52.5 ± 3.8 ms), indicating that visual signals can rapidly travel from the periphery to the cortex and back to the LGN.
Introduction
A reciprocal arrangement of feedforward and feedback projections interconnects the thalamus and cerebral cortex. In the visual system, the feedforward pathway from the lateral geniculate nucleus (LGN) provides the primary visual cortex (V1) with visual information coming from the retina. Feedback projections from V1 then complete the loop, because axons from corticogeniculate neurons project directly back to the LGN. Such architecture allows each pathway to dynamically influence the other. To develop realistic models of the nature of this influence, it is important to know how fast activity can propagate in the feedforward and feedback directions, as well as the shortest circuit required for linking the feedforward and feedback pathways. This study provides the first account of how quickly signals can traverse the geniculo-corticogeniculate loop in the primate.
In the primate, magnocellular and parvocellular LGN neurons give rise to axons that terminate most densely in layer 4C of the V1 and less densely in layer 6 ( Hubel and Wiesel, 1972 ; Hendrickson et al., 1978 ; Blasdel and Lund, 1983 ). Corticogeniculate feedback arises exclusively from neurons with cell bodies in layer 6 ( Lund et al., 1975 ; Hendrickson et al., 1978 ; Fitzpatrick et al., 1994 ). Because many layer 6 neurons have apical dendrites that branch within layer 4C and basal dendrites that branch within layer 6, they have the opportunity to receive direct LGN input both within layer 4C and layer 6 ( Lund and Boothe, 1975 ; Wiser and Callaway, 1996 ). However, because corticogeniculate neurons comprise only a small fraction of layer 6 neurons in the primate (14%) ( Fitzpatrick et al., 1994 ), it is not clear that they actually receive direct LGN input or that LGN input, if present, can drive responses. In the cat, where LGN input to layer 6 may differ in density compared with the primate, physiological studies report that all layer 6 neurons, including corticogeniculate neurons, receive subthreshold geniculocortical input that rarely drives suprathreshold spikes ( Ferster and Lindstrom, 1983 ). In the macaque monkey, ∼50% of layer 6 neurons receive suprathreshold input from the LGN ( Bullier and Henry, 1980 ); whether or not corticogeniculate neurons are included among these neurons remains to be determined.
To examine the temporal properties of feedforward and feedback transmission between the LGN and V1 as well as the prevalence of LGN input onto corticogeniculate neurons, we recorded single-unit activity in V1 of anesthetized and alert macaque monkeys while evoking orthodromic and antidromic spikes with electrical stimulation in the LGN. Our results reveal a distinct subclass of corticogeniculate neurons that receive direct, fast suprathreshold geniculocortical input. These corticogeniculate neurons also give rise to feedback that is significantly faster than that supplied by the rest of the corticogeniculate population. These results demonstrate that visual signals can propagate rapidly from the periphery to the cortex and back to the LGN.
Materials and Methods
Eight adult male macaque monkeys ( Macacca mulatta ) were used in this study. Seven animals were used for anesthetized recordings and one for alert recordings. All procedures conformed to National Institutes of Health guidelines and were approved by the Animal Care and Use Committee at the University of California, Davis.
Surgical procedures.
Animals for anesthetized recordings were initially anesthetized with ketamine (10 mg/kg, i.m.) and maintained with sufentanil citrate (3–6 μg/kg/h, i.v.), isoflurane (0.5%), and nitrous oxide (1:2, in oxygen). Temperature, expired CO 2 , electrocardiogram (ECG), electroencephalogram (EEG), heart rate, and arterial oxygen saturation were monitored continuously. Proper anesthetic depth was assessed by monitoring the EEG for changes in slow-wave/spindle activity and the ECG and expired CO 2 . If changes in any of these measures indicated a decreased level of anesthesia, additional sufentanil was given and the rate of infusion increased. Craniotomies were made over the LGN and V1 and the dura was reflected. The eyes were fitted with contact lenses and focused on a tangent screen located 172 cm in front of the animal. Once all surgical procedures were complete, animals were paralyzed with vencuronium bromide (0.2 mg/kg/h, i.v.).
Under full surgical anesthesia, the animal used for alert recordings was equipped with a scleral eye coil and a cranial implant containing a head restraint post and two recording cylinders, one over the LGN and one over V1.
Neuronal recordings, electrical stimulation, and visual stimuli.
Single-unit responses from V1 of alert and anesthetized animals were recorded to a PC with a Power 1401 acquisition system and Spike2 software (Cambridge Electronic Design, Cambridge, UK). While recording from a V1 neuron, geniculocortical and corticogeniculate axons were stimulated electrically via one or two platinum/iridium electrodes implanted in the LGN. Stimulating electrodes(s) were positioned in retinotopic register with the cortical electrodes and the 500 μm exposed tips spanned multiple layers. Stimulating electrodes were connected to an AM Systems (Carlsborg, WA) isolated pulse stimulator that delivered a brief, biphasic shock (0.2 ms; ∼10 V) in one of two modes: a noncollision mode where shocks were delivered at regular intervals (every 5 s), or a collision mode where shocks were triggered to occur within 1 ms of a spontaneous spike from the cortical neuron.
Visual stimuli were generated with a VSG2/5 system (Cambridge Research Systems, Rochester, UK) and presented on a Sony (Tokyo, Japan) monitor with a refresh rate of 140 Hz and a mean luminance of 38 cd/m 2 . For recordings from the alert animal, stimuli were presented while the animal maintained fixation for fluid reward. Trials were aborted if eye position deviated by >0.35°. Drifting sinusoidal gratings of optimal orientation, spatial frequency, and 70% contrast were used to characterize neurons according to the ratio of the fundamental harmonic (f1) to mean response ( Skottun et al., 1991 ). For neurons with ratios <1.0 (and >1000 recorded spikes), visual response latency was determined from responses to the drifting sinusoidal stimulus.
In anesthetized animals, electrolytic lesions (4 μA; 4 s) were made to reconstruct the locations of recording sites. At the end of experiments, animals were killed with an overdose of sodium pentobarbital (80 mg/kg) and perfused with 4% paraformaldehyde. Tissue was sectioned on a freezing microtome and stained with thionin.
We recorded single-unit responses in V1 of anesthetized and alert macaque monkeys while electrically stimulating the LGN to determine how quickly information can travel in the reciprocal pathways that interconnect the two structures. In particular, we were interested in determining (1) the time course of spike propagation in the geniculocortical and corticogeniculate pathways, (2) whether geniculocortical input can directly drive suprathreshold responses from corticogeniculate neurons, and (3) the time course of visual responses among corticogeniculate neurons. Geniculocortical recipient (GR) neurons and corticogeniculate (CG) neurons were identified both by the location of neuronal recordings ( Fig. 1 B ) and by the responses evoked after brief electrical shocks in the LGN ( Figs. 1 A , ,2 2 ).

Location of stimulating electrodes and recording sites. A , Nissl-stained section of the LGN showing the track lesion from the stimulating electrode (arrow). B , Nissl-stained section of V1 showing a lesion (arrow) made at the recording site of a corticogeniculate neuron that did not receive LGN input. Dashed line represents the estimated layer 6/white matter border. D, Dorsal; L, lateral; M, medial; V, ventral.

Responses of three representative cortical neurons to electrical stimulation in the LGN. In each panel, solid blue and red lines represent average responses to noncollision and collision trials, respectively (average of 12 trials; aligned to stimulus artifact at time 0). Dashed lines represent SEs. Insets show overlays of 7–10 responses. A , Responses of a GR neuron. This neuron reliably fired spikes at a latency of 2.9 ms after LGN stimulation in noncollision and collision trials. B , Responses of a CG neuron. This neuron had a latency of 13.7 ms in noncollision trials, but failed to fire spikes in collision trials. C , Responses of a GRCG neuron. This neuron fired two spikes at 1.6 and 2.8 ms in noncollision trials, but only one spike at 2.6 ms in collision trials.
Once a cortical neuron was identified that reliably produced a spike at a fixed latency after an electrical shock, a collision test was performed to determine whether the neuron was a GR neuron and/or a CG neuron. Figure 2 shows the responses of three representative cortical neurons. Although all three neurons reliably followed the electrical shock in the noncollision trials (blue traces), the neurons displayed different response patterns in the collision trials (red traces). In a collision trial, a spontaneous spike from the recorded cortical neuron triggers the electrical shock. If the neuron is a GR neuron that receives feedforward input from the LGN, then the spontaneous spike will not affect the propagation of the orthodromic spike and the neuron will produce a spike at the fixed latency, as in Figure 2 A . However, if the neuron is a CG neuron that provides feedback to the LGN, then the spontaneous spike traveling toward the LGN will collide with the electrically evoked antidromic spike and the antidromic spike will not reach the cortex, as in Figure 2 B . Finally, if the neuron is both a GR and a CG neuron (a GRCG neuron), then the neuron will produce two spikes during a noncollision trial (one orthodromic, the other antidromic), but just one spike (orthodromic) during a collision trial, as in Figure 2 C .
In total, we recorded from 77 V1 neurons that reliably followed electrical stimulation in the LGN. Recordings were made from both alert and anesthetized animals to compare the activation latencies of neurons in the two preparations. Accordingly, 41 neurons were recorded from in the alert animal (25 GR, 11 CG, and 5 GRCG neurons) and 36 neurons were recorded from in anesthetized animals (17 GR, 15 CG, and 4 GRCG neurons). Importantly, there were no significant differences in the activation latencies between alert and anesthetized animals for GR, CG, and GRCG neurons ( p = 0.1–0.4, t tests).
Consistent with past results ( Bullier and Henry, 1980 ), spike propagation in the geniculocortical pathway was fast, as the mean orthodromic latency among GR neurons was 4.0 ± 0.2 ms ( Fig. 3 A , dashed line). Orthodromic latencies for GRCG neurons were similar with an average latency of 4.2 ± 0.4 ms ( p = 0.3, t test) ( Fig. 3 A ), suggesting that LGN input directed to layers 4 and 6 follow a similar time course.

Orthodromic and antidromic latencies of V1 neurons. A , Histogram showing the distribution of orthodromic latencies for 51 geniculocortical recipient neurons: 42 GR neurons (black bars) and 9 GRCG neurons (gray bars). Numbers above each bar indicate neurons recorded in the alert animal. The mean latency for all geniculocortical recipient neurons was 4.0 ± 0.2 ms (dashed line). B , Histogram showing the distribution of antidromic latencies for 35 corticogeniculate neurons: 26 CG neurons (black bars) and 9 GRCG neurons (gray bars). Numbers above each bar indicate neurons recorded in the alert animal. The mean latency for all corticogeniculate neurons was 9.5 ± 1.7 ms (dashed line). C , Histogram showing the distribution of orthodromic plus antidromic latencies for nine GRCG neurons. Gray and black bars represent neurons recorded in alert and anesthetized animals, respectively. The mean combined latency was 9.3 ± 1.6 ms (dashed line).
Antidromic latencies of CG neurons showed greater diversity ( Fig. 3 B ). Although the mean antidromic latency of CG neurons was significantly greater than the mean orthodromic latency of GR neurons (9.5 ± 1.7 vs 4.0 ± 0.2 ms, respectively; p < 0.002, t test) ( Fig. 3 A , B , dashed lines), most CG neurons responded with short latencies. Notably, 21 of 35 CG and GRCG neurons (60%) in our sample had antidromic latencies that were as fast as the orthodromic latencies measured from GR neurons. Interestingly, although the distribution of antidromic latencies of GRCG and CG neurons are partially overlapping, the antidromic latencies among GRCG neurons are significantly shorter than those for the remaining CG neurons (5.1 ± 1.3 vs 11.1 ± 2.3 ms, respectively; p = 0.01, t test) ( Fig. 3 B ). This suggests that GRCG neurons represent a distinct subclass of CG neurons, exhibiting fast orthodromic and antidromic latencies. Figure 3 C shows the distribution of orthodromic plus antidromic latencies for GRCG neurons. On average, the time required for spikes traveling in the reciprocal pathway from the LGN to GRCG neurons and back to the LGN was 9.3 ± 1.6 ms.
Given the diversity of conduction latencies among the feedback axons in our sample of CG neurons, we next asked whether there is a relationship between feedback latency and visual physiology. Using drifting grating stimuli, we found a range of f1:mean values with 13 of 17 neurons having values <1.0, indicating that complex cells represent the majority of neurons in our sample ( Fig. 4 A ). Hartigan's dip test revealed that the sample was not multimodal ( p = 0.98). Nevertheless, the antidromic latencies of neurons with f1:mean values >1.0 were significantly greater than those with values <1.0 (12.4 ± 2.9 vs 5.5 ± 0.9 ms; p = 0.03, t test). There was also a significant relationship between the antidromic latency and f1:mean response ( Fig. 4 A ) ( R 2 = 0.83; p ≪ 0.001).

Visual physiology of corticogeniculate neurons. A , Relationship between f1:mean response and antidromic latency for 17 corticogeniculate neurons (open symbols indicate GRCG neurons). The gray line shows linear fit of the data. B , C , Peristimulus time histograms showing visual responses of a CG and GRCG neuron, respectively. Arrows indicate onset of visual stimulation. Dashed lines represent time to reach 50% of maximum response (39 and 32 ms). D , Distribution of visual response plus antidromic latencies for 11 neurons (mean latency, 52.5 ± 3.8 ms; dashed line). The inset shows the relationship between visual response latency and antidromic latency. Gray and black symbols represent neurons recorded in alert and anesthetized animals, respectively. Open symbols indicate GRCG neurons.
To determine how quickly signals can propagate to V1 and back to the LGN after the onset of a visual stimulus, we measured the visual response latency of neurons with f1:mean values <1.0. This sample included both CG and GRCG neurons. The visually evoked responses of a representative CG and GRCG neuron are shown in Figure 4 , B and C . The firing rate of these neurons reached 50% of maximum response at 39 and 32 ms after stimulus onset (dashed lines). Across 11 GRCG and CG neurons, the mean latency to reach 50% of maximum response was 47.2 ± 3.5 ms ( Fig. 4 D , inset) (range, 32–63 ms). By combining the visual response latency of these corticogeniculate neurons with the latency they require to conduct spikes back to the LGN (mean 5.3 ± 1.0 ms), we determined that corticogeniculate neurons can deliver feedback signals to the LGN as early as 37 ms after visual stimulus presentation (mean, 52.5 ± 3.8 ms) ( Fig. 4 D ).
Corticogeniculate neurons are in a strategic position to influence the processing of visual information as they give rise to axons that terminate both in the LGN and the layers of V1 that receive LGN input. Here, we provide the first report of the timing and visual physiology of corticogeniculate feedback in the primate. Our results reveal a subclass of corticogeniculate neurons that receives direct, suprathreshold input from the LGN and gives rise to feedback that is significantly faster than other corticogeniculate neurons. We also demonstrate that corticogeniculate feedback signals are delivered back to the LGN within ∼50 ms (range, 37–72 ms) of the onset of a visual stimulus.
Functional diversity of corticogeniculate neurons and implications for fast feedback
Past studies in cats, ferrets, and rabbits have subdivided corticogeniculate neurons on the basis of antidromic activation latency ( Tsumoto and Suda, 1980 ; Swadlow and Weyand, 1987 ; Briggs and Usrey, 2005 ) and/or visual responses ( Tsumoto and Suda, 1980 ; Greive and Sillito, 1995 ; Hirsch et al., 1998 ). Our results from the monkey support the view that there are functionally distinct groups of corticogeniculate neurons. Consistent with results from cat and ferret ( Tsumoto and Suda, 1980 ; Briggs and Usrey, 2005 ), we find a broad distribution of antidromic activation latencies and a significant relationship between latency and cell classification: corticogeniculate neurons with fast conducting axons are complex cells whereas those with slower axons tend to be simple cells. In addition, we show that corticogeniculate neurons can be grouped according to whether or not they receive direct, suprathreshold input from the LGN.
Although corticogeniculate axons are the single greatest source of synaptic input to the LGN, it is generally agreed that these axons have a modulatory, rather than driving, influence on LGN neurons ( Guillery, 1969 ; Erisir et al., 1997 ; Crick and Koch, 1998 ; Sherman and Guillery, 1998 ). This modulatory influence need not be slow or follow a long time course, as results from the present study show that a subset of corticogeniculate neurons has axons with short conduction latencies. In addition, corticogeniculate synapses evoke postsynaptic currents that are mediated both by ionotropic and metabotropic glutamate receptors, suggesting that postsynaptic responses can be both fast and brief or longer lasting ( Scharfman et al., 1990 ; McCormick and von Krosigk, 1992 ; Godwin et al., 1996a , b ; von Krosigk et al., 1999 ).
Several functions for corticogeniculate feedback have been suggested over the years, including (1) sharpening the receptive fields of LGN neurons ( Murphy and Sillito, 1987 ; Sillito and Jones, 2002 ; Webb et al., 2002 ), (2) adjusting the gain or timing of LGN responses ( Molotchnikoff et al., 1984 ; Gulyas et al., 1990 ; Funke et al., 1996 ; Rao and Ballard, 1999 ; Przybyszewski et al., 2000 ), (3) shifting geniculate neurons between burst and tonic modes of firing ( Sherman, 1996 ), and (4) increasing the correlated activity of LGN neurons ( Sillito et al., 1994 ). Given the diversity of conduction latencies and response properties of corticogeniculate neurons, it seems likely that corticogeniculate neurons with different phenotypes contribute to different aspects of visual processing. Along these lines, because the subclass of corticogeniculate neurons that receive suprathreshold LGN input have short orthodromic and antidromic latencies, these neurons would seem particularly well suited for exerting an influence on the temporal properties of LGN responses.
Identifying corticogeniculate neurons and geniculocortical recipient neurons
We identified corticogeniculate neurons using electrical stimulation and a collision test. To reduce the possibility that we inadvertently excited cortical neurons with axons projecting to other subcortical targets, we placed stimulating electrodes in retinotopic register with cortical electrodes (to minimize stimulation parameters) and anatomically verified that cortical recordings were restricted to layer 6, the only layer to contain corticogeniculate neurons ( Lund et al., 1975 ; Hendrickson et al., 1978 ; Fitzpatrick et al., 1994 ).
We also used electrical stimulation to identify cortical neurons that receive input from the LGN. Although, in principle, cortical neurons could be driven by collaterals of antidromically activated corticogeniculate neurons, we believe the input recipient neurons in our sample were not collaterally driven. First, previous studies report that collateral activation of postsynaptic neurons requires higher-frequency stimulation than that used in this study (>7 Hz compared with <0.3 Hz) ( Ferster and Lindstrom, 1985 ). Second, unlike the reliable responses to stimulation that we measured, collateral activation does not reliably drive postsynaptic spikes ( Ferster and Lindstrom, 1985 ). Third, compared with the synaptic currents associated with LGN input to layer 4 neurons, synaptic currents from the collaterals of layer 6 neurons are relatively small and inconsistent with the responses we observed ( Stratford et al., 1996 ). Given these constraints, it seems unlikely that we have mislabeled the geniculocortical recipient neurons in our sample.
Concluding remarks
Few pathways in the brain are as prominent, yet poorly understood, as the corticothalamic feedback pathway (for review, see Alitto and Usrey, 2003 ; Briggs and Usrey, 2007 ). Here, we have shown that corticogeniculate neurons in the macaque monkey are a diverse group of neurons that display a wide range of axonal conduction latencies. Within this group, we have identified a special subclass of corticogeniculate neurons that receives direct, suprathreshold input from the LGN. Thus, with a single synapse, these neurons bring together the feedforward and feedback pathways. By taking into account the visual response latency and conduction latency of corticogeniculate neurons, we estimate that their axons can deliver feedback signals to the LGN within ∼50 ms of the presentation of a visual stimulus. In addition, the combined orthodromic and antidromic activation latency of these neurons is short, <10 ms. Because the time course of visual responses in both the LGN and V1 typically exceeds this value ( Maunsell and Gibson, 1992 ), the evolution of visual responses in both structures is likely influenced by fast conducting corticogeniculate neurons. These results underscore the view that the feedforward and feedback pathways should not be viewed in isolation, but rather as members of a dynamic reciprocal circuit interconnecting the thalamus and cerebral cortex.
This work was supported by National Institutes of Health Grants EY13588 and EY15580, the McKnight Foundation, and the Esther A. and Joseph Klingenstein Fund.
- Alitto HJ, Usrey WM. Corticothalamic feedback and sensory processing. Curr Opin Neurobiol. 2003; 13 :1–6. [ PubMed ] [ Google Scholar ]
- Blasdel GG, Lund JS. Termination of afferent axons in macaque striate cortex. J Neurosci. 1983; 3 :1389–1413. [ PMC free article ] [ PubMed ] [ Google Scholar ]
- Briggs F, Usrey WM. Temporal properties of feedforward and feedback pathways between the thalamus and visual cortex in the ferret. Thalamus Relat Syst. 2005; 3 :133–139. [ PMC free article ] [ PubMed ] [ Google Scholar ]
- Briggs F, Usrey WM. Encyclopedia of neuroscience. Ed 4. Elsevier; 2007. Corticothalamic circuits: structure and function. in press. [ Google Scholar ]
- Bullier J, Henry GH. Ordinal position and afferent input of neurons in monkey striate cortex. J Comp Neurol. 1980; 193 :913–935. [ PubMed ] [ Google Scholar ]
- Crick F, Koch C. Constraints on cortical and thalamic projections: the no-strong-loops hypothesis. Nature. 1998; 391 :245–250. [ PubMed ] [ Google Scholar ]
- Erisir A, Van Horn SC, Sherman SM. Relative numbers of cortical and brainstem inputs to the lateral geniculate nucleus. Proc Natl Acad Sci USA. 1997; 94 :1517–1520. [ PMC free article ] [ PubMed ] [ Google Scholar ]
- Ferster D, Lindstrom S. An intracellular analysis of geniculo-cortical connectivity in area 17 of the cat. J Physiol (Lond) 1983; 342 :181–215. [ PMC free article ] [ PubMed ] [ Google Scholar ]
- Ferster D, Lindstrom S. Augmenting responses evoked in area 17 of the cat by intracortical axon collaterals of cortico-geniculate cells. J Physiol (Lond) 1985; 367 :217–232. [ PMC free article ] [ PubMed ] [ Google Scholar ]
- Fitzpatrick D, Usrey WM, Schofield BR, Einstein G. The sublaminar organization of corticogeniculate neurons in layer 6 of macaque striate cortex. Vis Neurosci. 1994; 11 :307–315. [ PubMed ] [ Google Scholar ]
- Funke K, Nelle E, Li B, Worgotter F. Corticofugal feedback improves the timing of retino-geniculate signal transmission. NeuroReport. 1996; 7 :2130–2134. [ PubMed ] [ Google Scholar ]
- Godwin DW, Van Horn SC, Eriir A, Sesma M, Romano C, Sherman SM. Ultrastructural localization suggests that retinal and cortical inputs access different metabotropic glutamate receptors in the lateral geniculate nucleus. J Neurosci. 1996a; 16 :8181–8192. [ PMC free article ] [ PubMed ] [ Google Scholar ]
- Godwin DW, Vaughan JW, Sherman SM. Metabotropic glutamate receptors switch visual response mode of lateral geniculate nucleus cells from burst to tonic. J Neurophysiol. 1996b; 76 :1800–1816. [ PubMed ] [ Google Scholar ]
- Greive KL, Sillito AM. Differential properties of cells in the feline primary visual cortex providing the corticofugal feedback to the lateral geniculate nucleus and visual claustrum. J Neurosci. 1995; 15 :4868–4874. [ PMC free article ] [ PubMed ] [ Google Scholar ]
- Guillery RW. A quantitative study of synaptic interconnections in the dorsal lateral geniculate nucleus of the cat. Z Zellforsch. 1969; 96 :39–48. [ PubMed ] [ Google Scholar ]
- Gulyas B, Lagae L, Eysel U, Orban GA. Corticofugal feedback influences the responses of geniculate neurons to moving stimuli. Exp Brain Res. 1990; 79 :441–446. [ PubMed ] [ Google Scholar ]
- Hendrickson AE, Wilson JR, Ogren MP. The neuroanatomical organization of pathways between the dorsal lateral geniculate nucleus and visual cortex in the old world and new world primates. J Comp Neurol. 1978; 182 :123–136. [ PubMed ] [ Google Scholar ]
- Hirsch JA, Gallagher CA, Alonso JM, Martinez LM. Ascending projections of simple and complex cells in layer 6 of the cat striate cortex. J Neurosci. 1998; 18 :8086–8094. [ PMC free article ] [ PubMed ] [ Google Scholar ]
- Hubel DH, Wiesel TN. Laminar and columnar distribution of geniculo-cortical fibers in the macaque monkey. J Comp Neurol. 1972; 146 :421–450. [ PubMed ] [ Google Scholar ]
- Lund JS, Boothe R. Interlaminar connections and pyramidal neuron organization in the visual cortex, area 17, of the macaque monkey. J Comp Neurol. 1975; 159 :305–334. [ Google Scholar ]
- Lund JS, Lund RD, Hendrickson AE, Bunt AH, Fuchs AF. The origin of efferent pathways from the primary visual cortex, area 17, of the macaque monkey. J Comp Neurol. 1975; 164 :287–304. [ PubMed ] [ Google Scholar ]
- Maunsell JH, Gibson JR. Visual response latencies in striate cortex of the macaque monkey. J Neurophysiol. 1992; 68 :1332–1344. [ PubMed ] [ Google Scholar ]
- McCormick DA, von Krosigk M. Corticothalamic activation modulates thalamic firing through glutamate “metabotropic” receptors. Proc Natl Acad Sci USA. 1992; 89 :2774–2778. [ PMC free article ] [ PubMed ] [ Google Scholar ]
- Molotchnikoff S, Tremblay F, Lepore F. The role of the visual cortex in response properties of lateral geniculate cells in rats. Exp Brain Res. 1984; 53 :223–232. [ PubMed ] [ Google Scholar ]
- Murphy PC, Sillito AM. Corticofugal feedback influences the generation of length tuning in the visual pathway. Nature. 1987; 329 :727–729. [ PubMed ] [ Google Scholar ]
- Przybyszewski AW, Gaska JP, Foote W, Pollen DA. Striate cortex increases contrast gain of macaque LGN neurons. Vis Neurosci. 2000; 17 :485–494. [ PubMed ] [ Google Scholar ]
- Rao RP, Ballard DH. Predictive coding in the visual cortex: a functional interpretation of some extra-classical receptive-field effects. Nat Neurosci. 1999; 2 :79–87. [ PubMed ] [ Google Scholar ]
- Scharfman HE, Lu SM, Guido W, Adams PR, Sherman SM. N-methyl-D-aspartate receptors contribute to excitatory postsynaptic potentials of cat lateral geniculate neurons recorded in thalamic slices. Proc Natl Acad Sci USA. 1990; 87 :4548–4552. [ PMC free article ] [ PubMed ] [ Google Scholar ]
- Sherman SM. Dual response modes in lateral geniculate neurons: mechanisms and functions. Vis Neurosci. 1996; 13 :205–213. [ PubMed ] [ Google Scholar ]
- Sherman SM, Guillery RW. On the actions that one nerve cell can have on another: distinguishing “drivers” from “modulators.” Proc Natl Acad Sci USA. 1998; 95 :7121–7126. [ PMC free article ] [ PubMed ] [ Google Scholar ]
- Sillito AM, Jones HE. Corticothalamic interactions in the transfer of visual information. Philos Trans R Soc Lond B Biol Sci. 2002; 357 :1739–1752. [ PMC free article ] [ PubMed ] [ Google Scholar ]
- Sillito AM, Jones HE, Gerstein GL, West DC. Feature-linked synchronization of thalamic relay cell firing induced by feedback from the visual cortex. Nature. 1994; 369 :479–482. [ PubMed ] [ Google Scholar ]
- Skottun BC, De Valois RL, Grosof DH, Movshon JA, Albrecht DG, Bonds AB. Classifying simple and complex cells on the basis of response modulation. Vision Res. 1991; 31 :1079–1086. [ PubMed ] [ Google Scholar ]
- Stratford KJ, Tarczy-Hornoch K, Martin KA, Bannister NJ, Jack JJ. Excitatory synaptic inputs to spiny stellate cells in cat visual cortex. Nature. 1996; 382 :258–261. [ PubMed ] [ Google Scholar ]
- Swadlow HA, Weyand TG. Corticogeniculate neurons, corticotectal neurons, and suspected interneurons in visual cortex of awake rabbits: receptive-field properties, axonal properties, and effects of EEG arousal. J Neurophysiol. 1987; 57 :977–1001. [ PubMed ] [ Google Scholar ]
- Tsumoto T, Suda K. Three groups of cortico-geniculate neurons and their distribution in binocular and monocular segments of cat striate cortex. J Comp Neurol. 1980; 193 :223–236. [ PubMed ] [ Google Scholar ]
- von Krosigk M, Monckton JE, Reiner PB, McCormick DA. Dynamic properties of corticothalamic excitatory postsynaptic potentials and thalamic reticular inhibitory postsynaptic potentials in thalamocortical neurons of the guinea-pig dorsal lateral geniculate nucleus. Neuroscience. 1999; 91 :7–20. [ PubMed ] [ Google Scholar ]
- Webb BS, Tinsley CJ, Barraclough NE, Easton A, Parker A, Derrington AM. Feedback from V1 and inhibition from beyond the classical receptive field modulates the responses of neurons in the primate lateral geniculate nucleus. Vis Neurosci. 2002; 19 :583–592. [ PubMed ] [ Google Scholar ]
- Wiser AK, Callaway EM. Contributions of individual layer 6 pyramidal neurons to local circuitry in macaque primary visual cortex. J Neurosci. 1996; 16 :2724–2739. [ PMC free article ] [ PubMed ] [ Google Scholar ]

IMAGES
VIDEO
COMMENTS
c, Our no-strong-loops hypothesis rules out these types of strong connection between the thalamus and cortex. (In this pair of diagrams, the modulating pathways have been omitted to avoid confusion.)
Interestingly, they are consistent with the "no strong loop hypothesis" introduced by Crick and Koch regarding thalamic circuits that receive driving cortical inputs sending modulatory inputs to avoid strong drive-drive loops (Figure 1E) (Crick and Koch, 1998. Crick F.
Constraints on cortical and thalamic projections: the no-strong-loops hypothesis. Creators Crick, Francis Koch, Christof. Abstract. The many distinct cortical areas of the macaque monkey visual system can be arranged hierarchically, but not in a unique way. We suggest that the connections between these cortical areas never form strong, directed ...
thalamo-cortical loops cortical coordination space-based attention feature-based attention rhythmic sampling. Type ... the searchlight hypothesis. Proceedings of the National Academy of Sciences of the United States of America 81, 4586 (1984).Google Scholar. ... C. Constraints on cortical and thalamic projections: the no-strong-loops hypothesis.
The many distinct cortical areas of the macaque monkey visual system can be arranged hierarchically, but not in a unique way. We suggest that the connections between these cortical areas never form strong, directed loops. For connections between the visual cortex and particular thalamic nuclei, we predict that certain types of connections will ...
Given that HO thalamic projections to S1 and V1 appear modulatory, these reciprocal loops do not violate Francis Crick's no-strong-loops hypothesis, stipulating that closed driver-driver loops are unlikely to exist in the brain since they would cause runaway excitation . It remains unclear if such reciprocal loops in thalamocortical ...
One theoretical constraint on this level of organization, the "no strong loops hypothesis," considered only developmentally determined area to area connectivity patterns to implement its specific neuron to neuron network topological constraint (Crick and Koch, 1998).
Strong loops of driving connections probably do not occur under normal conditions 13. ... C. Constraints on cortical and thalamic projections: the no-strong-loops hypothesis. Nature 391, 245-250 ...
We suggest that the connections between these cortical areas never form strong, directed loops. For connections between the visual cortex and particular thalamic nuclei, we predict that certain types of connections will not be found. If strong, directed loops were to exist, we suggest that the cortex would go into uncontrolled oscillations.
One theoretical constraint on this level of organization, the \no strong loops hypothesis," considered only developmentally determined area to area connectivity patterns to imple-ment its speci c neuron to neuron network topological constraint [3]. While local synaptic
Crick, F. & Koch, C. Constraints on cortical and thalamic projections: the no-strong-loops hypothesis. Nature 391 , 245-250 (1998). Article CAS Google Scholar
F. Crick and C. Koch. Why is the a hierarchy of visual cortical and thalamic areas: The no-strong loops hypothesis. Nature, 391:245-250, 1998. Article Google Scholar A. Das and C. Gilbert. Topography of contextual modulations mediated by short-range interactions in primary visual cortex. Nature, 399:655-661, 1999.
1998 Constraints on cortical and thalamic projections: the no-strong-loops hypothesis. Nature. 391, 245-250. ... 2002 Completing the corticofugal loop: a visual role for the corticogeniculate type 1 metabotropic glutamate receptor. J. Neurosci. 22, 2956-2962.
Why is there a hierarchy of visual cortical and thalamic areas: The no-strong loops hypothesis. Nature. 1998; 391: 245-249. Crossref; PubMed; Scopus (268) Google Scholar] as well as so many cortico-cortical feedback connections, all of which are located in the deep layers, have no direct role to play in consciousness.
Crick, F. (1998 b) Why is there a hierarchy of visual cortical and thalamic areas: The no-strong loops hypothesis. Nature 391: 245 -49.CrossRef Google Scholar. Crick, F. (2005) What is the function of the claustrum? Philosophical Transactions of the Royal Society of London B 360: 1271 -319.
Abstract Our current understanding of thalamocortical (TC) circuits is largely based on studies investigating so-called 'specific' thalamic nuclei, which receive and transmit sensory-triggered inpu...
According to the no-strong-loops hypothesis, LP cells receiving this driving cortical input from L5 should not project back to L5 of the same cortical region. Several visual areas, including RL, AL, and AM, receive input from two separate patches in LP. It is possible that these are separate sets of cells that receive driving versus modulatory ...
Constraints on cortical and thalamic projections: the no-strong-loops hypothesis. F. Crick C. Koch. Biology. Nature. 1998; TLDR. It is suggested that the connections between these cortical areas of the macaque monkey visual system never form strong, directed loops, and it is predicted that certain types of connections will not be found. Expand ...
Crick F, Koch C. Constraints on cortical and thalamic projections: the no-strong-loops hypothesis. Nature. 1998 Jan 15; 391 (6664):245-250. [Google Scholar] Cusick CG, Scripter JL, Darensbourg JG, Weber JT. Chemoarchitectonic subdivisions of the visual pulvinar in monkeys and their connectional relations with the middle temporal and rostral ...
Constraints on cortical and thalamic projections: The no-strong-loops hypothesis. Article Abstract: The macaque cerebral cortex has several visual areas which have been arranged in a rough hierarchy by Felleman and Van Essen. It is now suggested that links between cortical areas do not form strong, directed loops. It is predicted that some ...
Constraints on cortical and thalamic projections: the no-strong-loops hypothesis. Nature. 391: 245-50. PMID 9440687 DOI: 10.1038/34584 : 0.443: 1996: Crick F. Visual perception: rivalry and consciousness Nature. 379: 485-486. PMID 8596623 DOI: 10.1038/379485A0 : 0.33: 1995: Crick F, Koch C. Are we aware of neural activity in primary visual cortex?
For example, a fundamental circuit logic rule proposed by Francis Crick and Christof Koch, is called the "no strong loops hypothesis" . This rule posits that the presence of strong recurrent ...
Importantly, there were no significant differences in the activation latencies between alert and anesthetized animals for GR, CG, and GRCG neurons (p = 0.1-0. ... Crick F, Koch C. Constraints on cortical and thalamic projections: the no-strong-loops hypothesis. Nature. 1998; 391:245-250. [Google Scholar] Erisir A, Van Horn SC, Sherman SM ...