

Application Opening and Closing Dates for Autumn 202 5
Applications to the MA/PhD and PhD open on 1 September 2024. The closing date is December 2, 2024. A NOTE ON THIS DUE DATE : This application due date is real and is a completion due date. Applications and all required documents received after the due date will not be considered. Applications and all required documents must be uploaded to our online application system. We do not accept any mailed materials.
- Minimum 3.0 or B grade point average over the two most recent years of study (90 quarter or 60 semester credits) guarantees consideration. However, average GPAs for the students we have admitted have been higher. See recent applicant statistics below on this page.
- Ph.D. applicants must show proof of completion of a master’s degree prior to starting the program, though it is common for those applying to the Ph.D. program to be working on their thesis in the year during which they apply.
- M.A./Ph.D. applicants must show proof of completion of a U.S. equivalent bachelor’s degree prior to starting the program, though it is common for applicants to be in their senior year of college when they apply to the M.A./Ph.D. program.
- Full-time status is required for all funded students, but domestic U.S. residents who do not receive assistantships can enroll half-time (please note this is exceedingly rare).
- Applicants who are not native speakers of English must provide evidence of English language proficiency (see Policy 3.2: Graduate School English Language Proficiency Requirements ) and evidence of spoken English proficiency (see Policy 5.2: Conditions of Appointment for TAs who are not Native Speakers of English ).
- Applicants who hold a bachelor’s degree from a regionally accredited institution in the United States meet both requirements (Note: A master’s or doctoral degree does not satisfy this requirement). Applicants who do not hold a bachelor’s degree from a U.S. institution must have a minimum total score of 92 and speaking score of 26 if using the TOEFL, and a minimum total score of 7.0 and speaking score of 7.0 if using the IELTS. If proof is not provided that these minimums are met as of December 1, the applicant will not be considered.
Submit the following materials online:
- Graduate School online application . The Graduate School requires all applicants to use the web application (if you are truly unable to use the web application, e-mail [email protected] ).
- Application fee . The application fee must be received and recorded by Graduate Admissions prior to the application deadline. You must pay the fee online using a MasterCard or Visa card (both credit and debit cards are accepted) or a TeleCheck (an online “check” taken from your existing checking account). If eligible for and requesting an application fee waiver, please note you must submit your application a minimum of 7 days prior to the application deadline, and earlier is recommended. Details on application fee waivers are available here .
- Unofficial transcripts from each institution from which you received a degree of Bachelor or higher within the online application. Official transcripts are not required for the admissions application and should not be mailed. An unofficial transcript could be a scan of an opened official transcript, or a printout of your academic history from your university’s web portal. It must include all classes taken, grades received, and degrees granted.
- Important : It can take up to two weeks after your testing date or report request for UW to receive your scores, so the GRE should be taken/scores requested no later than November 15 to ensure your scores arrive by the December 1 application completion deadline.
- Important : It can take up to two weeks after your testing date or report request for UW to receive your scores, so the TOEFL or IELTS should be taken/scores requested no later than November 15 to ensure your scores arrive by the December 1 application completion deadline.
- Three recommendations . Letters of recommendation from professors are strongly preferred, but if you have been out of school for many years or have another reason for using non-academic references, other professional recommendations are acceptable. These recommendations are completed online and should not be mailed. The online recommendation includes a short questionnaire, ratings assessment, as well as the opportunity to paste or upload a letter. Please designate your recommenders within the online application early to allow enough time for them to submit their online recommendations no later than December 1.
- Describe explicitly why you have chosen to apply to earn an M.A./Ph.D. or Ph.D. in communication, and why specifically at the University of Washington. If there are additional UW departments in which you would like to take courses, feel free to mention them.
- Describe the main ideas and/or veins of theory that interest you as well as the kind of communication phenomena you wish to study.
- State your academic goals so that the admissions committee may determine whether your goals could be met in our department.
- Explain how the expertise of the 2-3 particular Graduate Faculty whom you selected in the Overview Questions section could support scholarly interests you would like to pursue.
- Write this letter honestly and to the best of your ability, because the admissions committee will read it for its substance and as an indicator of your readiness for and fit with this program.
- For Ph.D. applicants : Please provide a sole-authored academic writing sample , roughly 10-30 pages long, that demonstrates your research competence and your ability to produce original scholarship. This might be a thesis chapter, a journal publication, a seminar paper, or something similar. If necessary, edit your paper for length before submitting it with your application. The admissions committee will read your paper to evaluate your writing competence, the clarity of your argument, and the depth of your analysis and insight.
- For M.A./Ph.D. applicants : Please provide a sole-authored academic writing sample , roughly 10-30 pages. This is typically a paper you wrote for a college or university class, but it can also be a thesis chapter, a journal publication, or a seminar paper. The admissions committee will read your paper to evaluate your writing competence, the clarity of your argument, and the depth of your analysis and insight.
- Resume or curriculum vitae . If you don’t have a vitae, don’t worry. A resume will do. If you do have a vitae, use it instead.
- Overview questions. You will be asked about your career goals and research experiences, given the opportunity to explain any transcript anomalies, and asked to specify 2-3 Graduate Faculty by whom you would like to be mentored.
- In one or two pages, please describe how your experiences and/or academic interests could contribute to a diverse community of communication scholars. For example, you might describe interactions you have had with people of cultural or ethnic backgrounds different from your own, or you might write about intellectual exchanges you have had with persons holding different viewpoints. Your essay might also discuss the unique qualities of your own intellectual or cultural background and how these are likely to enhance diversity in any community.
Are you an international applicant? An international applicant is anyone who is not a United States citizen or a Permanent Resident (green card holder). Please go to the following at the Graduate School website for further information for international applicants: Graduate School international applicant information . Undocumented applicants should follow the instructions here for completing the online application.
- Applicants who hold a bachelor’s degree from a regionally accredited institution in the United States meet both requirements (Note: A master’s or doctoral degree does not satisfy this requirement). Applicants who do not hold a bachelor’s degree from a US institution must have a minimum total score of 92 and speaking score of 26 if using the TOEFL, and a minimum total score of 7.0 and speaking score of 7.0 if using the IELTS. If proof is not provided that these minimums are met as of December 1, the applicant will not be considered.
- Newly-admitted international students will be required to demonstrate adequate financial support without resorting to unauthorized employment. More information will be sent to applicants who receive an offer of admission. Financial documentation is not necessary at this stage of the application process.
- Full-time status is mandatory for all international students.
102 Communications Box 353740 Seattle, WA 98195 Phone: (206) 543-2660 Fax: (206) 616-3762
Graduate Program (206) 543-6745 Undergraduate Program (206) 543-8860
Make a Gift Privacy Terms of Use -->
Copyright 2020 Department of Communication
University of Washington
PhD Completion Process
Congratulations!
As a PhD student, you have spent spend significant time at the University of Rochester taking courses, completing research in your field, completing milestones and are now ready to complete your degree.
The final requirement in earning a PhD degree is the completion and defense of the doctoral dissertation. Understanding the steps and associated deadlines in the dissertation submission and degree conferral process is necessary to establish a successful plan. For complete descriptions of the process, please review the Regulations and University Policies Concerning Graduate Students .
The page below is organized by before, during and post-oral defense. Please read the page in it’s entirety and email questions you have to [email protected]
Doctoral Qualifying Exam / Admission to Candidacy
All PhD programs must administer a qualifying examination as part of the PhD program requirements. The qualifying examination may be either written or oral or both, at the discretion of the department/program, and must be passed at least six months before the final examination may be taken.
The associate dean of a school certifies that a student has passed the qualifying examinations and is recommended for candidacy.
For more details, please review page 12 of the Regulations and University Policies Concerning Graduate Students .
Planning for the Final Oral Exam / Dissertation Defense
At least six months before you plan to defend your dissertation, you should contact the graduate coordinator of your program for details regarding the submitting the defense. During the months leading up to the anticipated defense, your graduate coordinator will walk you through the process and explain any department specific nuances.
Pay careful attention to the five PhD degree cycle deadlines. In each degree cycle, there is a deadline for the last day to complete your degree requirements. There are no exceptions to the deadlines. If a deadline is missed, your name cannot be approved by the Council on Graduate Education and presented to the Board of Trustees until the following degree date.
The oral exam/defense/dissertation is approved at multiple levels of the University. Starting with the program, oral exam/defense/dissertation committee, school graduate deans and the University Dean of Graduate Education. You will receive emails a few days before your defense confirming that the approvals for the defense have all been received.
PhD academic calendar
PhD students can reference their specific academic calendar in the drop-downs below.
To enable review by the Graduate Education Offices in the Schools and University Graduate Education, online dissertation committee and program director approvals must be completed the following number of working days before the defense:
- SMD and SON: At least 10 working days before the defense
- AS&E, Warner and Simon: At least 15 working days before the defense
- ESM: At least 20 working days before the defense
A minimum of five working days must elapse between the approval of the candidate’s School Dean to advance the record to University Graduate Education and the day of the defense. This time has been included in the schools’ lead times listed above.
Defenses may be held during regular business hours on any University working day with the exceptions listed below. See calendar below for additional non-working days.
Monday, May 27: The University is closed in observance of Memorial Day. This day cannot be counted as a working day.
Wednesday, June 19: The University is closed in observance of Juneteenth. This day cannot be counted as a working day.
Thursday, July 4: The University is closed in observance of Independence Day. This day cannot be counted as a working day.
Friday, August 23, 4 p.m.: Last day to submit final corrected dissertation to the ProQuest website to fulfill degree requirements for an August 2024 degree conferral.
Monday, August 26: Fall semester begins.
Saturday, August 31: August 2024 PhD date.
Monday, August 26 at 4 p.m.: Last day for students who have completed defenses to submit final corrected dissertation for October 2024 degree to the ProQuest website without having to register for Fall 2024. AFTER THIS DATE, SUBMISSION OF DOCUMENTS FOR DEGREE COMPLETION REQUIRES REGISTRATION FOR THE FALL SEMESTER.
Monday, September 2: The University is closed in observance of Labor Day. This day cannot be counted as a working day
Thursday, September 26, 4 p.m.: Last day to submit final corrected dissertation to the ProQuest website to fulfill degree requirements for an October 2024 degree. NOTE: Students must be registered for the Fall 2024 Semester if submitting documents after August 26.
Friday, October 4: October 2024 PhD conferral date.
Monday, September 2: The University is closed in observance of Labor Day. This day cannot be counted as a working day.
Wednesday, November 27 through Friday, November 29: The University is closed in observance of Thanksgiving. These three days cannot be counted as working days.
Monday, December 16 at 4 p.m.: Last day to submit final corrected dissertation to the ProQuest website to fulfill degree requirements for a December degree. NOTE: Students must be registered for the Fall 2024 Semester if submitting final documents after August 28.
Friday, December 13: Last day for dissertation defense registration approval by University Dean of Graduate Education for defenses scheduled January 2, 2025.
Thursday, December 19 through Friday, December 31: Due to recess and holidays, no dissertation registrations will be completed in the University Graduate Education office. No PhD defenses may be held during this time period.
December 31: December 2024 PhD conferral date.
Friday, February 28 at 4 p.m.: Last day to submit final corrected dissertation to the ProQuest website to fulfill degree requirements for a March degree.
March 7, 2025: March 2025 PhD conferral date.
Wednesday, April 30 at 4 p.m.: Last day to submit final corrected dissertation to the ProQuest website to fulfill degree requirements for a May 2025 degree.
Friday, May 16: University Doctoral Commencement
Additional Information about Planning for your Defense
Writing your dissertation.
The dissertation process webpage offers several writing resources to help you get started, meet your goals, and complete your thesis/dissertation on time.
You also will want to take full advantage of internal reviews of the dissertation before uploading the thesis for defense registration, in order to minimize the number of errors in the registration version.
Final Oral Examination Committee
Page 11 of the Regulations and University Policies Concerning Graduate Students (“Redbook”) goes into detail about the make-up of the committee.
PhD Committee Matrix
Approval Process for Non-Standard Committee Membership
Approval must be obtained in writing, based on a petition that includes a rationale for the request and a CV of the proposed nonstandard member.
Contact your Graduate Coordinator to start the petition process.
Page 12 of the Regulations and University Policies Concerning Graduate Students (“Redbook”) goes into detail.
Scheduling your Defense
Contact the graduate coordinator of your program for details regarding the scheduling the defense.
Click here to Download a checklist of information needed to schedule defense
Rubric for Oral Defense
Click here to download the Oral Defense Rubric.
After the final oral exam / defense
Submitting your final dissertation.
Approximately, 24 hours after oral exam, an email is sent that details out the next steps. Including uploading the final abstract and dissertation to ProQuest®, submitting a UR Research authorization form, and completing two required surveys.
UR Research Form
The libraries at University of Rochester, electronically store and publish the dissertations based on a students embargo restrictions.
You can access our database of dissertations on the UR Research page hosted by the library.
Survey Completion
There are two required survey’s for completion of the PhD Process. The University of Rochester PhD Survey and the national Survey of Earned Doctorates. The links to completing these surveys will be included in the completion memo sent post-defense.
We use a service called ProQuest to administer the electronic final thesis/dissertation (ETD) submission. ProQuest provides services that enable strategic acquisition, management and discovery of information collections. Once you have made any necessary revisions and the thesis/dissertation is final, you are ready to begin the submission process.
PhD Completion Confirmation
Once you have completed the steps above, you will receive an email the confirms that all of the requirements have been completed and will include a PhD Completion memo.
We offer both an electronic diploma and a paper copy diploma to students after their graduation date. Information on ordering replacement diplomas is located on the registrar’s webpage .
Commencement
The University holds one doctoral commencement ceremony each year in May. Eligibility to walk in the May ceremony includes students that graduated between August of the previous year through August of the current year. As an example, the May 2025 ceremony is for students who graduated August 2024, October 2024, December 2024, February 2025, May 2025 and August 2025. Doctoral students are only eligible to walk once.
You will receive information about the ceremony that your are eligible to walk in during the Spring semester.
About Stanford GSB
- The Leadership
- Dean’s Updates
- School News & History
- Commencement
- Business, Government & Society
- Centers & Institutes
- Center for Entrepreneurial Studies
- Center for Social Innovation
- Stanford Seed
About the Experience
- Learning at Stanford GSB
- Experiential Learning
- Guest Speakers
- Entrepreneurship
- Social Innovation
- Communication
- Life at Stanford GSB
- Collaborative Environment
- Activities & Organizations
- Student Services
- Housing Options
- International Students
Full-Time Degree Programs
- Why Stanford MBA
- Academic Experience
- Financial Aid
- Why Stanford MSx
- Research Fellows Program
- See All Programs
Non-Degree & Certificate Programs
- Executive Education
- Stanford Executive Program
- Programs for Organizations
- The Difference
- Online Programs
- Stanford LEAD
- Seed Transformation Program
- Aspire Program
- Seed Spark Program
- Faculty Profiles
- Academic Areas
- Awards & Honors
- Conferences
Faculty Research
- Publications
- Working Papers
- Case Studies
Research Hub
- Research Labs & Initiatives
- Business Library
- Data, Analytics & Research Computing
- Behavioral Lab
Research Labs
- Cities, Housing & Society Lab
- Golub Capital Social Impact Lab
Research Initiatives
- Corporate Governance Research Initiative
- Corporations and Society Initiative
- Policy and Innovation Initiative
- Rapid Decarbonization Initiative
- Stanford Latino Entrepreneurship Initiative
- Value Chain Innovation Initiative
- Venture Capital Initiative
- Career & Success
- Climate & Sustainability
- Corporate Governance
- Culture & Society
- Finance & Investing
- Government & Politics
- Leadership & Management
- Markets and Trade
- Operations & Logistics
- Opportunity & Access
- Technology & AI
- Opinion & Analysis
- Email Newsletter
Welcome, Alumni
- Communities
- Digital Communities & Tools
- Regional Chapters
- Women’s Programs
- Identity Chapters
- Find Your Reunion
- Career Resources
- Job Search Resources
- Career & Life Transitions
- Programs & Webinars
- Career Video Library
- Alumni Education
- Research Resources
- Volunteering
- Alumni News
- Class Notes
- Alumni Voices
- Contact Alumni Relations
- Upcoming Events
Admission Events & Information Sessions
- MBA Program
- MSx Program
- PhD Program
- Alumni Events
- All Other Events
- Operations, Information & Technology
- Organizational Behavior
- Political Economy
- Classical Liberalism
- The Eddie Lunch
- Accounting Summer Camp
- California Econometrics Conference
- California Quantitative Marketing PhD Conference
- California School Conference
- China India Insights Conference
- Homo economicus, Evolving
- Political Economics (2023–24)
- Scaling Geologic Storage of CO2 (2023–24)
- A Resilient Pacific: Building Connections, Envisioning Solutions
- Adaptation and Innovation
- Changing Climate
- Civil Society
- Climate Impact Summit
- Climate Science
- Corporate Carbon Disclosures
- Earth’s Seafloor
- Environmental Justice
- Operations and Information Technology
- Organizations
- Sustainability Reporting and Control
- Taking the Pulse of the Planet
- Urban Infrastructure
- Watershed Restoration
- Junior Faculty Workshop on Financial Regulation and Banking
- Ken Singleton Celebration
- Marketing Camp
- Quantitative Marketing PhD Alumni Conference
- Presentations
- Theory and Inference in Accounting Research
- Stanford Closer Look Series
- Quick Guides
- Core Concepts
- Journal Articles
- Glossary of Terms
- Faculty & Staff
- Researchers & Students
- Research Approach
- Charitable Giving
- Financial Health
- Government Services
- Workers & Careers
- Short Course
- Adaptive & Iterative Experimentation
- Incentive Design
- Social Sciences & Behavioral Nudges
- Bandit Experiment Application
- Conferences & Events
- Get Involved
- Reading Materials
- Teaching & Curriculum
- Energy Entrepreneurship
- Faculty & Affiliates
- SOLE Report
- Responsible Supply Chains
- Current Study Usage
- Pre-Registration Information
- Participate in a Study
Matrix Completion Methods for Causal Panel Data Models
In this paper we develop new methods for estimating causal effects in settings with panel data, where a subset of units are exposed to a treatment during a subset of periods, and the goal is estimating counterfactual (untreated) outcomes for the treated unit/period combinations. We develop a class of estimators that uses the observed elements of the matrix of control outcomes corresponding to untreated unit/periods to predict the “missing” elements of the matrix, corresponding to treated units/periods. The approach estimates a matrix that well-approximates the original (incomplete) matrix, but has lower complexity according to a matrix norm, where we consider the family of Schatten norms based on the singular values of the matrix. The proposed methods have attractive computational properties. From a technical perspective, we generalize results from the matrix completion literature by allowing the patterns of missing data to have a time series dependency structure. We also present new insights concerning the connections between the interactive fixed effects models and the literatures on program evaluation under unconfoundedness as well as on synthetic control methods. If there are few time periods and many units, our method approximates a regression approach where counterfactual outcomes are estimated through a regression of current outcomes on lagged outcomes for the same unit. In contrast, if there are few units and many periods, our proposed method approximates a synthetic control estimator where counterfactual outcomes are estimated through a regression of the lagged outcomes for the treated unit on lagged outcomes for the control units. The advantage of our proposed method is that it moves seamlessly between these two different approaches, utilizing both cross-sectional and within-unit patterns in the data.
- See the Current DEI Report
- Supporting Data
- Research & Insights
- Share Your Thoughts
- Search Fund Primer
- Affiliated Faculty
- Faculty Advisors
- Louis W. Foster Resource Center
- Defining Social Innovation
- Impact Compass
- Global Health Innovation Insights
- Faculty Affiliates
- Student Awards & Certificates
- Changemakers
- Dean Jonathan Levin
- Dean Garth Saloner
- Dean Robert Joss
- Dean Michael Spence
- Dean Robert Jaedicke
- Dean Rene McPherson
- Dean Arjay Miller
- Dean Ernest Arbuckle
- Dean Jacob Hugh Jackson
- Dean Willard Hotchkiss
- Faculty in Memoriam
- Stanford GSB Firsts
- Annual Alumni Dinner
- Class of 2024 Candidates
- Certificate & Award Recipients
- Dean’s Remarks
- Keynote Address
- Teaching Approach
- Analysis and Measurement of Impact
- The Corporate Entrepreneur: Startup in a Grown-Up Enterprise
- Data-Driven Impact
- Designing Experiments for Impact
- Digital Marketing
- The Founder’s Right Hand
- Marketing for Measurable Change
- Product Management
- Public Policy Lab: Financial Challenges Facing US Cities
- Public Policy Lab: Homelessness in California
- Lab Features
- Curricular Integration
- View From The Top
- Formation of New Ventures
- Managing Growing Enterprises
- Startup Garage
- Explore Beyond the Classroom
- Stanford Venture Studio
- Summer Program
- Workshops & Events
- The Five Lenses of Entrepreneurship
- Leadership Labs
- Executive Challenge
- Arbuckle Leadership Fellows Program
- Selection Process
- Training Schedule
- Time Commitment
- Learning Expectations
- Post-Training Opportunities
- Who Should Apply
- Introductory T-Groups
- Leadership for Society Program
- Certificate
- 2024 Awardees
- 2023 Awardees
- 2022 Awardees
- 2021 Awardees
- 2020 Awardees
- 2019 Awardees
- 2018 Awardees
- Social Management Immersion Fund
- Stanford Impact Founder Fellowships
- Stanford Impact Leader Prizes
- Social Entrepreneurship
- Stanford GSB Impact Fund
- Economic Development
- Energy & Environment
- Stanford GSB Residences
- Environmental Leadership
- Stanford GSB Artwork
- A Closer Look
- California & the Bay Area
- Voices of Stanford GSB
- Business & Beneficial Technology
- Business & Sustainability
- Business & Free Markets
- Business, Government, and Society Forum
- Second Year
- Global Experiences
- JD/MBA Joint Degree
- MA Education/MBA Joint Degree
- MD/MBA Dual Degree
- MPP/MBA Joint Degree
- MS Computer Science/MBA Joint Degree
- MS Electrical Engineering/MBA Joint Degree
- MS Environment and Resources (E-IPER)/MBA Joint Degree
- Academic Calendar
- Clubs & Activities
- LGBTQ+ Students
- Military Veterans
- Minorities & People of Color
- Partners & Families
- Students with Disabilities
- Student Support
- Residential Life
- Student Voices
- MBA Alumni Voices
- A Week in the Life
- Career Support
- Employment Outcomes
- Cost of Attendance
- Knight-Hennessy Scholars Program
- Yellow Ribbon Program
- BOLD Fellows Fund
- Application Process
- Loan Forgiveness
- Contact the Financial Aid Office
- Evaluation Criteria
- GMAT & GRE
- English Language Proficiency
- Personal Information, Activities & Awards
- Professional Experience
- Letters of Recommendation
- Optional Short Answer Questions
- Application Fee
- Reapplication
- Deferred Enrollment
- Joint & Dual Degrees
- Entering Class Profile
- Event Schedule
- Ambassadors
- New & Noteworthy
- Ask a Question
- See Why Stanford MSx
- Is MSx Right for You?
- MSx Stories
- Leadership Development
- How You Will Learn
- Admission Events
- Personal Information
- GMAT, GRE & EA
- English Proficiency Tests
- Career Change
- Career Advancement
- Career Support and Resources
- Daycare, Schools & Camps
- U.S. Citizens and Permanent Residents
- Requirements
- Requirements: Behavioral
- Requirements: Quantitative
- Requirements: Macro
- Requirements: Micro
- Annual Evaluations
- Field Examination
- Research Activities
- Research Papers
- Dissertation
- Oral Examination
- Current Students
- Education & CV
- International Applicants
- Statement of Purpose
- Reapplicants
- Application Fee Waiver
- Deadline & Decisions
- Job Market Candidates
- Academic Placements
- Stay in Touch
- Faculty Mentors
- Current Fellows
- Standard Track
- Fellowship & Benefits
- Group Enrollment
- Program Formats
- Developing a Program
- Diversity & Inclusion
- Strategic Transformation
- Program Experience
- Contact Client Services
- Campus Experience
- Live Online Experience
- Silicon Valley & Bay Area
- Digital Credentials
- Faculty Spotlights
- Participant Spotlights
- Eligibility
- International Participants
- Stanford Ignite
- Frequently Asked Questions
- Founding Donors
- Location Information
- Participant Profile
- Network Membership
- Program Impact
- Collaborators
- Entrepreneur Profiles
- Company Spotlights
- Seed Transformation Network
- Responsibilities
- Current Coaches
- How to Apply
- Meet the Consultants
- Meet the Interns
- Intern Profiles
- Collaborate
- Research Library
- News & Insights
- Program Contacts
- Databases & Datasets
- Research Guides
- Consultations
- Research Workshops
- Career Research
- Research Data Services
- Course Reserves
- Course Research Guides
- Material Loan Periods
- Fines & Other Charges
- Document Delivery
- Interlibrary Loan
- Equipment Checkout
- Print & Scan
- MBA & MSx Students
- PhD Students
- Other Stanford Students
- Faculty Assistants
- Research Assistants
- Stanford GSB Alumni
- Telling Our Story
- Staff Directory
- Site Registration
- Alumni Directory
- Alumni Email
- Privacy Settings & My Profile
- Event Registration Help
- Success Stories
- The Story of Circles
- Support Women’s Circles
- Stanford Women on Boards Initiative
- Alumnae Spotlights
- Insights & Research
- Industry & Professional
- Entrepreneurial Commitment Group
- Recent Alumni
- Half-Century Club
- Fall Reunions
- Spring Reunions
- MBA 25th Reunion
- Half-Century Club Reunion
- Faculty Lectures
- Ernest C. Arbuckle Award
- Alison Elliott Exceptional Achievement Award
- ENCORE Award
- Excellence in Leadership Award
- John W. Gardner Volunteer Leadership Award
- Robert K. Jaedicke Faculty Award
- Jack McDonald Military Service Appreciation Award
- Jerry I. Porras Latino Leadership Award
- Tapestry Award
- Student & Alumni Events
- Executive Recruiters
- Interviewing
- Land the Perfect Job with LinkedIn
- Negotiating
- Elevator Pitch
- Email Best Practices
- Resumes & Cover Letters
- Self-Assessment
- Whitney Birdwell Ball
- Margaret Brooks
- Bryn Panee Burkhart
- Margaret Chan
- Ricki Frankel
- Peter Gandolfo
- Cindy W. Greig
- Natalie Guillen
- Carly Janson
- Sloan Klein
- Sherri Appel Lassila
- Stuart Meyer
- Tanisha Parrish
- Virginia Roberson
- Philippe Taieb
- Michael Takagawa
- Terra Winston
- Johanna Wise
- Debbie Wolter
- Rebecca Zucker
- Complimentary Coaching
- Changing Careers
- Work-Life Integration
- Career Breaks
- Flexible Work
- Encore Careers
- Join a Board
- D&B Hoovers
- Data Axle (ReferenceUSA)
- EBSCO Business Source
- Global Newsstream
- Market Share Reporter
- ProQuest One Business
- RKMA Market Research Handbook Series
- Student Clubs
- Entrepreneurial Students
- Stanford GSB Trust
- Alumni Community
- How to Volunteer
- Springboard Sessions
- Consulting Projects
- 2020 – 2029
- 2010 – 2019
- 2000 – 2009
- 1990 – 1999
- 1980 – 1989
- 1970 – 1979
- 1960 – 1969
- 1950 – 1959
- 1940 – 1949
- Service Areas
- ACT History
- ACT Awards Celebration
- ACT Governance Structure
- Building Leadership for ACT
- Individual Leadership Positions
- Leadership Role Overview
- Purpose of the ACT Management Board
- Contact ACT
- Business & Nonprofit Communities
- Reunion Volunteers
- Ways to Give
- Fiscal Year Report
- Business School Fund Leadership Council
- Planned Giving Options
- Planned Giving Benefits
- Planned Gifts and Reunions
- Legacy Partners
- Giving News & Stories
- Giving Deadlines
- Development Staff
- Submit Class Notes
- Class Secretaries
- Board of Directors
- Health Care
- Sustainability
- Class Takeaways
- All Else Equal: Making Better Decisions
- If/Then: Business, Leadership, Society
- Grit & Growth
- Think Fast, Talk Smart
- Spring 2022
- Spring 2021
- Autumn 2020
- Summer 2020
- Winter 2020
- In the Media
- For Journalists
- DCI Fellows
- Other Auditors
- Academic Calendar & Deadlines
- Course Materials
- Entrepreneurial Resources
- Campus Drive Grove
- Campus Drive Lawn
- CEMEX Auditorium
- King Community Court
- Seawell Family Boardroom
- Stanford GSB Bowl
- Stanford Investors Common
- Town Square
- Vidalakis Courtyard
- Vidalakis Dining Hall
- Catering Services
- Policies & Guidelines
- Reservations
- Contact Faculty Recruiting
- Lecturer Positions
- Postdoctoral Positions
- Accommodations
- CMC-Managed Interviews
- Recruiter-Managed Interviews
- Virtual Interviews
- Campus & Virtual
- Search for Candidates
- Think Globally
- Recruiting Calendar
- Recruiting Policies
- Full-Time Employment
- Summer Employment
- Entrepreneurial Summer Program
- Global Management Immersion Experience
- Social-Purpose Summer Internships
- Process Overview
- Project Types
- Client Eligibility Criteria
- Client Screening
- ACT Leadership
- Social Innovation & Nonprofit Management Resources
- Develop Your Organization’s Talent
- Centers & Initiatives
- Student Fellowships
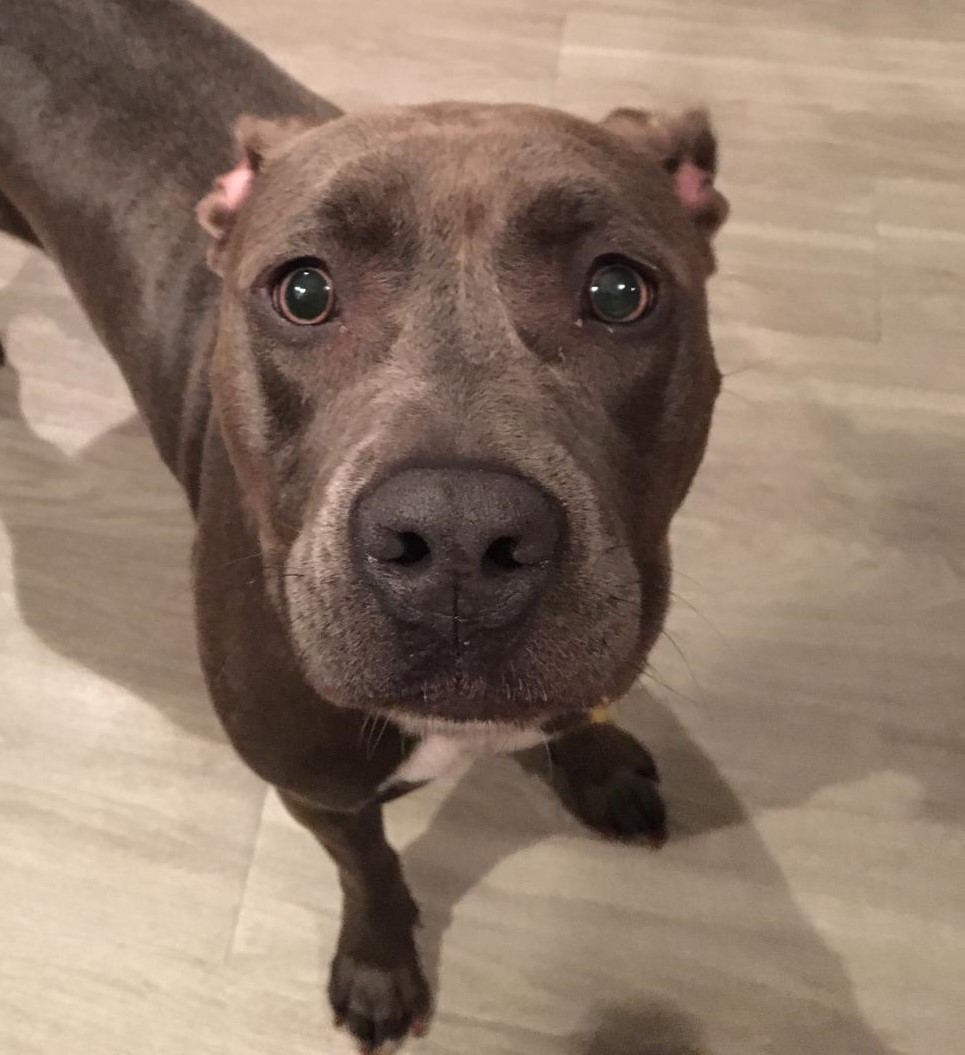
Myles Akin, PhD
I am an applied mathematician working as a ML/AI consultant for Avanade. I recieved a PhD in Mathematics from Drexel University where I studied Computational Neuroscience.
- Atlanta, GA
- Custom Social Profile Link
Matrix Completion
16 minute read
Matrix Approximation by Singular Value Decomposition
Electronic retailers have inundated consumers with a huge number of products to choose from. Therefore, matching consumers with the most appropriate product is important for consumer satisfaction and helping to maintain loyalty. To address the task of recommending the best product, many strategies have been developed. A review of most of these methods can be found in the wonderful resource Recommendation Systems Handbook edited by Ricci, et al (refered to here a RSH). For this post, I will be focusing on a particular methods, and a few associated algorithms, know as matrix completion.
Matrix completion is a collaborative filtering technique for finding the best products to recommend. To start, collaborative filtering is a strategy of recommendation based on similarity of past consumption patterns by users. For example, suppose user A and user B have rated movies in a similar manner in the past. We might then expect for a movie A has seen but B has not, and A has rated highly, B would also enjoy this movie. By this method, we can find products for users based on similar users. Another strategy I will not discuss more here is content filtering. Content filtering observes the similarity of product features in order to recommend new products. For instance, sticking with the movie theme, if a user enjoyed the movie Aliens, we might recommend Predator as they are action packed, sci-fi movies. For more on content filtering see RSH.
One of the most successful techniques for collaborative filtering has been matrix completion, also sometimes called matrix factorization (for further techniques, again see RSH). This methods generally relies on one of two types of data, explicit feedback of implicit feedback. Explicit feedback, such as the old NetFlix star rating system, provides information explicitly given about products by users/consumers. Implicit feedback is not directly provided by users or consumers, such as purchase history. For the purpose of this post, I will assume explicit feedback.
We start by constructing a ratings table where the rows are the users (consumers), the columns are the products and entries are the explicit ratings given by the user for a product. As matrix completion was made popular by the NetFlix competition, I will use movies as the product to recommend and a rating from 1 to five.
Aliens | Predator | Pretty Woman | Sleepless in Seattle | Notting Hill | Terminator | |
---|---|---|---|---|---|---|
1 | 2 | 4 | 5 | 4 | 1 | |
1 | 1 | 5 | 4 | 5 | 2 | |
5 | 4 | 2 | 2 | 1 | 5 | |
4 | 5 | 1 | 1 | 2 | 5 |
This table is complete and as we can see, has some clear patterns. Two users, Mark and Simone, clearly prefer sci-fi movies to romantic movies, while Charles and Laura are the opposite. Of course, this is fairly unrealistic, but is used to make a point. The main assumption of matrix completion is that what influences users to rate products high or low is based on only a few item features known as latent features . These latent features, which we generally cannot interpret, can be found by a low rank approximation of the rating matrix. The rank of the approximation matrix determines the number of latent features. The rating matrix is simply the entries of the ratings table:
The rank of a rectangular matrix can be found by the number of non-zero singular values in singular value decomposition . Singular value decomposition decomposes a \(m\times n\) matrix \(M\) as follows:
Where, \(U\) and \(V\) are unitary matrices with sizes \(m\times m\) and \(n\times n\) respectively. The columns of these matrices are known as left and right singular vectors. The matrix \(S\) is a \(m\times n\) diagonal matrix with the singular values on the diagonal. For our example, we can factorize using the following python code:
This gives the following result (rounded to two decimal places)
\(U = \begin{bmatrix} -0.47 & 0.49 & -0.47 & -0.56\\ -0.49 & 0.54 & 0.47 & 0.49\\ -0.53 & -0.48 & -0.53 & 0.48\\ -0.51 & -0.50 & 0.52 & -0.47 \end{bmatrix},\) \(S = \begin{bmatrix} 14.73& 0 & 0 & 0 & 0 & 0\\ 0 & 7.77 & 0 & 0 & 0 & 0\\ 0 & 0 & 1.58 & 0 & 0 & 0\\ 0 & 0 & 0 & 1.49 & 0 & 0 \end{bmatrix}\) \(V^T = \begin{bmatrix} -0.38 & -0.41 & -0.40 & -0.40 & -0.40 & -0.45\\ -0.42 & -0.36 & 0.42 & 0.41 & 0.41 & -0.42\\ -0.34 & 0.02 & -0.03 & -0.63 & 0.63 & 0.29\\ 0.31 & -0.70 & 0.46 & -0.24 & -0.18 & 0.33\\ 0.61 & -0.30 & -0.45 & 0.03 & 0.49 & -0.30\\ -0.29 & -0.35 & -0.49 & 0.46 & 0.02 & 0.58 \end{bmatrix}\)
We see that two of the singular values are much larger than the other two, indicating the importance of these dimensions in the reconstruction. We therefore might be interested in how close our an approximation of the matrix \(M\) would be if we used only those two singular values and their corresponding singular vectors. This is called truncated singular value decomposition. The following code gives an approximation with the first two singular values.
As we can see, this is a fairly good approximation. Using mean square error given by
we get an error of 0.197. Note, \( | |_F \) is the Frobenius norm. Not bad. In fact, with respect to the Frobenius norm, this is the best approximation we can get with a rank 2 matrix. This follows from the Eckart-Young theorem:
Theorem : (Eckart and Young) Let \(A = USV^T\) with \(rank(r)\). Then for some \(k\) such that \(0< k \leq r\) and \(A_k = U_kS_kV_k^T\) is the \(k\) truncated SVD,
That is, the \(k\) truncated SVD of some matrix \(A\) is the best \(k\) rank approximation of \(A\).
So far, we have considered matrices in which all entries are known. However, this is generally not the case for recommendations systems. Users almost never review all possible product. Therefore, we need to be able to approximate the matrix knowing only a some of the values. This is where SVD comes in handy for matrix completion, by assuming user ratings are based on a lower dimensional set of features. Let’s look at a version of the movie ratings matrix with a few entries removed (unobserved).
In our python code, we will use zeros in place of unobserved entries.
One thing to be concerned about is how many observations do we need in order to be able to approximate it with a lower rank matrix? A straightforward requirement is that we need an observation for each row and column, that is for each user and movie in our example. There are stronger requirments, but that is beyond the scope of this post, see the paper Exact Matrix Completion via Convex Optimization by Candes and Recht. Suffice it say that our matrix meets the necessary requirements.
There are a many algorithms for matrix completion based on singular value decomposition, here I will first talk about one that makes explicit use of SVD, the singular value thresholding method, then I will discuss the more popular stochastic gradient descent (SGD) technique. I will also relate the SGD and AM techniques back to SVD.
Matrix Completion with Singular Value Thresholding
The Singular Value Thresholding (SVT) I will discuss here was introduced in A Singular Value Thresholding Algorithm for Matrix Completion by Cai, Candes and Shen. Suppose we are given a matrix \(M\) consisting a set of observations \(\Omega = (i,j)\), that is \(M_{ij}\) is observed when \((i,j)\in \Omega\). We define the orthogonal projector \(P_\Omega\) on a matrix \(X\) to be
This will allow us to find the error between the observed entries of \(M\) and our low-rank approximation matrix. Our goal now is to find a low-rank approximation, \(X\), of the matrix \(M\) given only a few observations of it’s entries. In other word, we want to minimize the rank of our matrix with the constraint that the observed values set \(\Omega\) are equivalent in both \(X\) and \(M\).
Where \(|X|_* = \sum_i \sigma_i(X)\) is the called the nuclear norm and \(\sigma_i(X)\) are the singular values of \(X\). Techinically this is a convex relaxtion to the non-convex rank minimization problem, for more on that see the references paapers. Minimizing the nuclear norm will send some of the less important singular values to zero. This requires advanced semidefinite programming optimization methods that have problems with larger matrices due to solving huge systems of linear equations. However, we can simplify this by approximately solving the nuclear norm minimization problem using singular value thresholding.
Let’s restate our optimization problem as follows using the Frobenius norm to ensure the equivalence of the observes set \(\Omega\) in \(X\) and \(M\)
Let’s also define the soft-thresholding operator as
It was proved in the paper A Singular Value Thresholding Algorithm for Matrix Completion by Cai, Candes and Shen that the matrix \(D_\tau(X)\) is a solution to the restated minimization problem. The following algorithm finds the optimal matrix iteratively
- Initialize \(X^{(k)}\) for \(k=0\) as \(X^{(0)} = M\) and set a threshold \(\tau\)
- Apply the soft threshold \(Y^{(k)} = D_\tau(X^{(k)})\)
- Set \(X^{(k+1)} = X^{(k)}+\delta P_\Omega(Y^{(k)}-M)\), \(\delta\) is the learning rate
Note: the learning rate \(\delta\) is usually a function of \(k\), decreasing as the number of steps in creases. Here I will leave \(\delta\) static for simplicity.
The following python code implements this algorithm and applies it to the sample movie ratings matrix.
The resulting optimized matrix and the original complete matrix are
\(X = \begin{bmatrix} 1.02 & \color{red}{0.44} & 3.97 & 4.86 & \color{red}{3.19} & 0.99\\ \color{red}{0.64} & 1.02 & 4.91 & \color{red}{3.87} & 4.88 & 1.99\\ 4.86 & 3.98 & 1.97 & 1.99 & 1.01 & \color{red}{3.62}\\ 4.01 & 4.90 & \color{red}{2.24} & 1.00 & 1.99 & 4.89 \end{bmatrix}\) ,\(M = \begin{bmatrix} 1 & \color{red}{2} & 4 & 5 & \color{red}{4} & 1\\ \color{red}{1} & 1 & 5 & \color{red}{4} & 5 & 2\\ 5 & 4 & 2 & 2 & 1 & \color{red}{5}\\ 4 & 5 & 1 & \color{red}{1} & 2 & 5 \end{bmatrix}\)
the missing entries, \(\Omega^c\) are colored red. As we can see, the algorithm does a decent job of completing the matrix. Overall, the MSE \(X\) is 0.2817; not too bad. Fine tuning the hyperparameters \(\delta\) and \(\tau\) through cross validation, as well as letting both be functions of \(k\) should result in a better approximation.
Now based, on these results we can build a decision rule to recommend new movies, say if the filled in value is greater than 3. In this case, we would want to recommend Notting Hill to Charles, but not Predator. Thus this simple algorithm allows us to personalize recommendations for each user.
While this method is simple, it has some drawbacks. At each iteration, we must calculate the SVD of the \(X^{(k)}\) matrix. For large matrices, this can be prohibitively time consuming. We may thus search for a different method to find a low rank approximation.
Stochastic Gradient Descent
Since we want to move away from calculating SVDs, we may need to reformulate the optimization problem. The most popular method for low rank approximation reformulates the approximate factorization of the matrix \(M \approx WH^T\) where \(M\) is still and \(m\times n\) matrix and now \(W\) and \(H\) are \(m\times r\) and \(n\times r\) matrices respectively. \(r\) is now the target rank of the approximation. So unlike the soft-thresholding method, in this case we must specify a priori the target rank for our approximation.
Using this new factorization, we can restate our optimization problem as
Here we use \(L^2\) regularization constraints on the matrices \(W\) and \(H\) to prevent overfitting. You may be asking some questions now; Where does this factorization come from? Why do we use this factorization when we know truncated SVD is the best solution?
To answer the first, consider the following truncated SVD for some matrix \(A\)
As we can see, the rank \(r\) solution \(WH^T\) can arise from truncated SVD. In fact, this is the optimal solution. What is interesting, is that right multiplication of \(W\) and \(H\) by an orthogonal \(r\times r\) matrix leaves the product \(WH^T\) unchanged! We will see the importance of this later.
As to the second question, while this is a non-convex optimization problem, we can still use stochastic gradient descent (SGD) to find an at least locally optimal solution. As you may know, SGD is incredibly simple to implement and is quick to run even on large matrices. The SGD algorithm for this particular problem is implemented as:
- Initialize \(W\) and \(H\) to random values, typically uniformly over \([0,1]\)
- For each observation \((i,j) \in \Omega\), calculate \(e_{ij} = M_{ij} - W_iH^T_j\), where \(W_i\) and \(H_j\) are the ith and jth columns respectively
- \(W_i \leftarrow W_i + \alpha(e_{ij}H_i-\lambda W_j)\)
- \(H_i \leftarrow H_j + \alpha(e_{ij}W_j-\lambda H_i)\)
The following python script implements simple SGD for our movie ratings matrix. I use rank \(r=2\) for this example.
The results are \(X = \begin{bmatrix} 1.03 & \color{red}{0.16} & 3.98 & 4.98 & \color{red}{4.00} & 0.98\\ \color{red}{1.99} & 0.98 & 4.99 & \color{red}{6.09} & 4.94 & 2.02\\ 4.44 & 4.43 & 1.99 & 1.61 & 1.60 & \color{red}{4.88}\\ 4.51 & 4.55 & \color{red}{1.82} & 1.37 & 1.42 & 4.96 \end{bmatrix}\) ,\(M = \begin{bmatrix} 1 & \color{red}{2} & 4 & 5 & \color{red}{4} & 1\\ \color{red}{1} & 1 & 5 & \color{red}{4} & 5 & 2\\ 5 & 4 & 2 & 2 & 1 & \color{red}{5}\\ 4 & 5 & 1 & \color{red}{1} & 2 & 5 \end{bmatrix}\)
The overall MSE is 0.4759. This is pretty good. We do see that for one rating we have gone above the highest possible value of 5, but overall, it does a decent job of capturing the tastes of our users. What is particularly interesting about this factorization method is observing the matrices \(W\) and \(H\)
Observing these matrices, due to the simplicity of our original matrix, we can find an interpretation of our factorization. Each row of the \(W\) matrix corresponds to a user, and looking at the number, we can say that the first column is the users prefernce for Sci-Fi/Action and the second column is preference for Romantic Comedies!! We have interpretable latent variables! We can say the same for the \(H\) matrix, the composition of each movie as a Sci-Fi or Romantic Comedy. While not a perfect interpretation, it is useful. Unfortunately, with larger, not so obviously constructed matrices, interpretation of the latent variables is significantly more challenging, if possible at all. But this gives an idea of why latent varibles are important and the underlying idea of why a ratings matrix may be factorizable into lower rank matrices.
As mentioned, this method is non-convex. However, by alternately fixing one of the matrices \(W\) or \(H\), and performing optimization on the other, the problem becomes convex. This is the Alternating Minimization algorithm for SGD. It is a simple code modification of the above to achieve this, but not included here. There are also extensions of SGD to include bias and temporal dynamics. For more on these extensions, see Matrix Factorization Techniques for Recommender Systems by Koren, Bell and Volinsky.
Our last task now is to relate the matrices \(W\) and \(H\) back to SVD. As I mentioned, the optimal solution to the optimization problem is given by \(\hat{W} = U_rS_r^{1/2}\) and \(\hat{H} = V_rS_r^{1/2}\) (hats added to distinguish from the SGD matrices). When we calculate these from the original, filled matrix we get
These don’t look anything like the \(W\) and \(H\) we found from the SGD, what’s the deal? This goes back to the fact that the product \(WH^T\) is unaffected by right multiplication of \(\hat{W}\) and \(\hat{H}\) by a \(r\times r\) matrix. We can find this matric, call it \(R\), by the following
Note that since these are not square matrices, we have to use the pseudoinverse \(^+\)of \(\hat{W}\). Solving this, we get
While not perfect, we see that \(\hat{W}R\) is very close to the \(W\) found by SGD! You can verify for yourself that \(\hat{H}R\) is very close to \(H\). Most likely the difference is due to algorithmic approximation or rounding errors. You can also verify that \(R\) is orthogonal, that is \(RR=I\) where \(I\) is the identity matrix the same size as \(R\). What this should also tell you is that performing SVD on the matrix \(WH^T\) will give you an approximation to \(U_rS_rV_r^T\)! Hence, why the SGD method is actually called the SVD method in a lot of literature.
And there you have it, some matrix completion techniques, where they come from and how they are related. I have seen some posts that claim SVD and the \(WH^T\) factorizations are completely separate and unrelated. As you can see here, that’s simply not true and they are based on the same idea of low rank approximation, for which truncated SVD is the best solution. The \(WH^T\) factorization is just a different way of obtaining the truncated SVD, without actually performing truncated SVD. Hope you enjoyed!
You may also enjoy
Binomial asset pricing model.
4 minute read
This post is a short discussion of the Binomial Asset Pricing Model for call options.
A New Beginning - Options
7 minute read
A new beginning for my blog. I am no longer going to post about DS related subjects, I get enough of that at my job. I will now use this as a place to discus...

Fundamentals - Simple Linear Regression
This post is a short discussion of Simple Linear Regression and the Ordinary Least Square solution to estimating parameters.
Fundamentals - Decision Trees
A discussion of the decision tree model for classification and regression
PhD Candidate at EECS, UC Berkeley
- Google Scholar
Solving Matrix Completion as Noisy Matrix Sensing
Cyrus Samii
Notes on matrix completion methods
(Note: some typos in the notes corrected now.)
Below, I have posted some notes on matrix completion, inspired by this great Twitter thread by Scott Cunningham:
I've been working on a matrix completion project for a while; ever since I saw Athey, Bayati, Doudchenko, Imbens, and Khosravi 2017 paper (now updated at NBER 2018).I thought I'd share what I've learned, which is still very primitive. https://t.co/Z8eZPXEefM — scott cunningham (@causalinf) November 26, 2018
Have a look at Scott’s thread first. Also, have a look at the material that he posted. Then, the following may be helpful for further deciphering that methods (in formats friendly for online and offline reading):
Update: I had a very useful twitter discussion with @analisereal on the identification conditions behind matrix completion for estimating the ATT. Here is the thread and then I am updating the notes to incorporate these points:
What are the identification conditions for these methods to work? — Análise Real (@analisereal) November 28, 2018

Federico Nutarelli, Ph.D.
Unveiling Matrix Completion
Recently Prof. Scott Cunningham initiated a series on Matrix Completion (MC) for causal inference starting from the influential paper of Athey et al. (2021).
As someone who has been actively working in the field and has authored three papers involving Matrix Completion (MC), I'm excited to share a quick introduction to MC, its importance in causal inference, and where beginners can find helpful online resources.
Matrix Completion is a powerful tool in machine learning. It's like a smart way of filling in missing pieces in a vast dataset. Imagine you have a huge jigsaw puzzle with some parts missing. MC helps you figure out what those missing parts might be based on the existing pieces.
This technique is especially useful in causal inference, which is about understanding cause-and-effect relationships in data. MC helps in identifying these relationships even when some data points are missing, which is often the case in real-world scenarios.
For those just starting out, there are plenty of online resources to dive deeper into Matrix Completion. Websites like arXiv for research papers, online courses from platforms like Coursera or edX, and even specific tutorials on YouTube offer a wealth of information. These resources are great for building a foundational understanding and keeping up with the latest developments in the field.
Let's dive into it!
What is Matrix Completion?
MC is an exciting area in machine learning that has recently found widespread use in various fields such as recommending products or movies (like what you see on Netflix), understanding images in computer vision, and processing human language.
The core idea of MC is to figure out the missing pieces in a puzzle of data.
Imagine you have a big spreadsheet filled with lots of numbers, but some of them are missing. What MC does is like magic - it predicts these missing numbers based on the numbers that are already there. It's a bit like guessing the missing pieces in a partially completed crossword puzzle by looking at the words that are already filled in.
How does it do this? Well, MC methods use a special approach. They balance two things: fitting the data they can see and using something called 'regularization.' Regularization is like a guide that helps the system not to jump to wild conclusions based on limited data. It's like telling someone to make sensible guesses in our crossword puzzle without straying too far from the words that are already there. Regularization is useful to avoid the so called "overfitting". Overfitting is like memorizing the answers to a test without understanding the subject. Imagine you have a history test, and instead of learning about the events, causes, and effects, you just memorize the exact questions and answers from a practice test. On the actual test day, if the questions are the same, you'll do great. But if the questions are different, you'll struggle because you didn't really learn the subject.
In machine learning, overfitting happens when a computer model is trained too much on a specific set of data. It gets really good at answering questions for that data, just like memorizing the practice test. But when it sees new, different data, it doesn't perform well because it didn't learn the underlying patterns, just the specific details of the training data. So, overfitting is like being great at a practice test but failing in a real test because the questions aren't exactly the same.

Specifically, these methods often use what's called the 'nuclear norm' of the matrix, which is a technical way of measuring the matrix. You can think of the nuclear norm as a tool that helps the system understand how complex or simple the missing data might be. It's like having a rule in our crossword puzzle that says, "your guess should be as simple as possible but still make sense with the given words."
This video of Stanford University provides with a more deepened introduction to the topic:
Now the nuclear norm, more formally forces the matrix to be as much low-rank as possible (i.e. with columns or rows linearly dependent to each other) while preserving a good fit. Why? For two reasons mainly (i) Because without regularization we would incur in overfitting (since the only term left is the data-fitting term as we will see below); (ii) because in many application it is useful to have a low-rank approximation. This is the case, for instance, in innovative process. Many authors in the literature consider innovation as a linear combination of pre-existing technologies or knowledge domains which is able to generate novel solutions, thereby fostering the emergence of new domains or technologies.
Under which conditions does MC work generally?
Generally, MC works best when data is missing at random . The reason is simple: fairness in the missing information. Think of it like having a puzzle with some pieces missing. If the missing pieces are randomly spread out, you can still guess what the picture is about by looking at the remaining pieces. But if all the missing pieces are from one specific part of the puzzle, like the sky or a person's face, it's much harder to guess what that part should look like.
In MC, if data is missing randomly, the patterns or relationships in the data that are visible (the parts of the puzzle you can see) are likely to be similar to the patterns in the missing parts. This makes it easier for the model to accurately predict the missing values. However, if data is missing in a specific pattern (like all information from one category is missing), the model might not have enough information to understand the full picture, leading to inaccurate predictions.
So, MC is most effective when the gaps in the data don't follow any specific pattern, allowing the model to make better guesses about what's missing.
However, novel versions of MC have been implemented including also FE, encompassing longitudinal data and not-at-random. missing values. Below I provide various sources to find them
For the braves...
MC methods use a special formula that includes two main parts: a data-fitting term and a regularization term. To give you an idea, let's look at an example from Mazumder and colleagues in 2010. In their approach, the data-fitting term is expressed as (A-Z)^2. This part of the formula measures how well their model's predictions match the actual data. Think of it as checking how close their puzzle-solving guesses are to the real missing pieces.
The second part is the regularization term, represented as ||Z||. This term uses what's called the 'nuclear norm' (that's what the ||.|| symbolizes). It helps the model avoid jumping to wild conclusions based on limited or overly complex information. You can think of it as a guiding rule that keeps the model's guesses realistic and straightforward.
To summarize, the formulas used in MC methods balance fitting the available data as accurately as possible while keeping their predictions sensible and grounded

Here A is the partially observed matrix which is reconstructed by Z which has the same dimension. The Omega term denotes observations belonging to the training set and lambda is a regularization parameter.
Notice finally, that we do not have to confound the formula of MC with the algorithm used to implement it called SVT.
Where to find online sources to implement MC:
For R lovers, the recently implemented FECT package gives the possibility of adopting MC while controlling for covariates and Fixed Effects;
For MATLAB lovers, stay tuned, me and my collegues are implementing an easy tool for performing MC in all its shapes;
For Pythonista, check out this simple code and this one;
Of course refer to the github of Athey directly here .
Athey, S., Bayati, M., Doudchenko, N., Imbens, G. and Khosravi, K., 2021. Matrix completion methods for causal panel data models. Journal of the American Statistical Association , 116 (536), pp.1716-1730.
Mazumder, R., Hastie, T. and Tibshirani, R., 2010. Spectral regularization algorithms for learning large incomplete matrices. The Journal of Machine Learning Research , 11 , pp.2287-2322.
Yoo, Y., Boland Jr, R.J., Lyytinen, K. and Majchrzak, A., 2012. Organizing for innovation in the digitized world. Organization science , 23 (5), pp.1398-1408.
Varian, H.R., 2010. Computer mediated transactions. American Economic Review , 100 (2), pp.1-10.
Recent Posts
What processes govern the emerging of innovation ideas?
I*nnovating and then? The uncertain world of "innovation diffusion" (an introduction)
IV: the forgotten realm
The emergence of Machine Learning has led to a significant influx of computer science professionals into the field of econometrics....
- Request Information
- Current Students
- Faculty and Staff
- Scholarships and Awards
- Student News
- Dean’s List
- Doctor of Philosophy (PhD)
- Master of Science (MS)
- Master of Applied Statistics (MAS)
- Co-Major PhD
- Graduate Admissions FAQ
- Administrative Faculty
- Graduate Students
- Affiliate Faculty
- Emeritus/a Faculty
- Mission Statement
- DEI Information
PhD Seminar: Zerui Zhang, "Topics in Genomic Selection and Matrix Completion"
- Add to Google Calendar
- Outlook/iCalendar
Presenter: Zerui Zhang, PhD Candidate in Bioinformatics and Statistics
Title: Topics in Genomic Selection and Matrix Completion
Abstract: Genomic selection in plant breeding is a common technique to explore molecular genetic markers and accumulate favorable ones to assist in trait selection. We aim to incorporate simulation-based operations research into decision making under uncertainties including recombination and environmental effects. In the first section of the presentation, we introduce a modular simulation platform to extend attention from additive effects into non-additive effects to handle hybrid breeding. We propose the idea of using the opaque simulator to mimic nature more realistically and highlight the differences and benefits brought by resource allocation.
In the second section, we present a latent feature model to complete a partially observed matrix. Inspired by neighbor-based collaborative filtering and model-based singular value decomposition, we define radial neighbors and distance measure approximation between latent feature vectors, so that we could pool information into kernel regression for the prediction. We provide theoretical results to show the consistency of all estimators, and both simulation and empirical studies show that the new method gives better prediction accuracy and recovery capability.
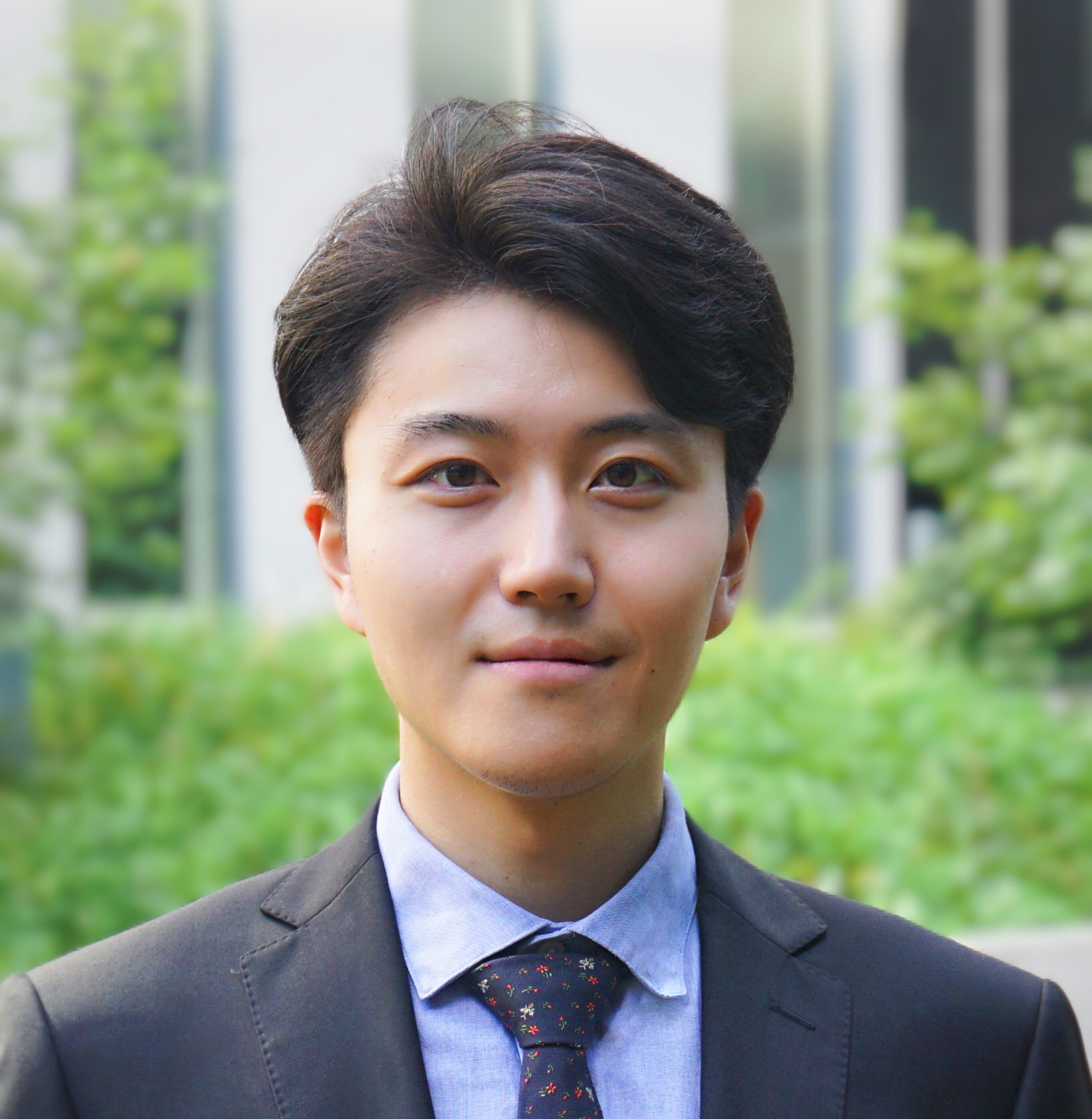
Department of Information Systems W. P. Carey School of Business Arizona State University Tempe, AZ 85287 kanxu1 [AT] asu [DOT] edu
Google Scholar | Linkedin | Twitter
I am currently an Assistant Professor of Information Systems at Arizona State University, W. P. Carey School of Business . Previously, I completed my PhD degree from University of Pennsylvania, Department of Economics under the supervision of Hamsa Bastani . I received a B.S. in Mathematics and a B.A. in Economics from Tsinghua University, and a M.S. in Statistics from University of Chicago.
My research focuses on developing novel machine learning methods for data-driven decision making practices, with applications to healthcare, textual analytics, digital platform, and pricing. In particular, I have designed tools for sequential decision making (e.g., bandits, reinforcement learning), collaborative decision making (e.g., multitask learning, transfer learning), and decision making with unstructured (e.g. natural language processing (NLP)) or matrix form (e.g., matrix completion).
I am actively looking for motivated graduate and undergraduate students (and colleagues who are interested in collaborating). The topics include all areas related to Information Systems, Operations Management, and Marketing, of both applied and theoretical machine learning. Please feel free to send me an email if you would like to chat about potential opportunities!
- I will be presenting "Group-Sparse Matrix Factorization for Transfer Learning of Word Embeddings" at Michigan Ross Workshop on Unstructured Data and Language Models , Jun 28-29, 2024. - I will be presenting "Online Learning in Matching Market through Matrix Completion" at 2023 AIML Conference , Dec 1-2, 2023.

A PhD timeline for finishing quickly [Free Gantt Download]
Navigating the labyrinthine journey of a PhD program is no small feat.
From the day you step into your graduate program as a bright-eyed doctoral student, you’re immediately thrust into a complex weave of coursework, research, and milestones.
By the second year, you’ve transitioned from coursework to research, laying the groundwork for your dissertation—a pivotal component in your scholarly endeavour.
Come the third year, you face the critical oral examination, a hurdle that could make or break years of hard work.
But how does one streamline this multifaceted journey? The answer lies in a well-planned PhD timeline.
This blog serves as an invaluable guide for any PhD student looking to complete their doctoral studies efficiently, walking you through each milestone from coursework to graduation.
How to Begin with the PhD Timeline Planning?
Planning your PhD timeline is an essential first step in your PhD program.
Success in any PhD program depends, to a large extent, on effective time management and keeping track of progress through a thoughtfully crafted PhD timeline.
Start with outlining all your major requirements:
- coursework,
- dissertation,
- and the expected time needed for each task.
I also highly recommend factoring in failure time – give yourself a little bit of wiggle room for when things, invariably – go wrong.
It’s crucial to remain realistic about the time you can commit daily or weekly while keeping long-term goals in mind.
Regular check-ins on your PhD timeline and supervisor will help you stay on track and allow you to adapt if necessary.
Adjustments may be needed as you progress through your PhD program, but having a timeline as a guide can make the journey less daunting and more achievable.
Elements to include in a 3-year PhD timeline
The initial stage in this timeline typically involves coursework, often lasting one year, where the student engrosses themselves in advanced study in their chosen field.
Once the coursework is done (USA PhDs), they focus on proposing, conducting, and presenting their initial research.
By the end of the second year, most students should have a clear direction for their dissertation, a core component of the PhD process.
In this third and final year of the PhD timeline, the student focuses primarily on completing their dissertation, which involves collecting data, analyzing results, and organizing their research into a substantial, original, and cohesive document that contributes to contemporary knowledge in their field.
Regular reviews and modifications of the PhD timeline may also be necessary to accommodate various unpredictable circumstances, thus making this timeline both a guide and a flexible workplan.
It is a significant tool in successfully navigating the maze of becoming a PhD holder.
Create Your PhD Timeline for a 3 year completion
Creating a timeline for a 3-year PhD program requires careful planning, as you’ll have multiple milestones and tasks to complete.
This timeline may vary depending on your specific field, institution, or country, but here is a general outline you can use as a starting point:
Year & Quarter | Activity/Milestone | Description | Outcome/Output |
---|---|---|---|
Admission & Onboarding | Formalities for joining the program, including orientation. | Official start of the program. | |
Initial Literature Review | Familiarize yourself with the existing research in your field. | Foundation for your research. | |
Coursework | Complete required or optional courses. | Credits/Education | |
Research Proposal Outline | Develop a draft outline for your PhD proposal. | Outline for proposal | |
Coursework & Seminars | Continue with coursework and attend relevant seminars. | Credits/Education | |
Meet with Advisor | Discuss research interests and outline. | Feedback for refinement | |
Complete Research Proposal | Finalize your research proposal with your advisor’s input. | Approved Proposal | |
Ethics Approval (if needed) | Submit proposal for ethics approval if required. | Ethics Clearance |
Year & Quarter | Activity/Milestone | Description | Outcome/Output |
---|---|---|---|
Data Collection | Start gathering data according to your proposal. | Initial Data | |
Intermediate Review | Review progress with advisor. | Feedback for refinement | |
Data Analysis | Start analyzing the collected data. | Preliminary Findings | |
Draft Chapters | Start writing initial chapters of your thesis. | Draft Chapters | |
Further Analysis | Conduct additional analysis if necessary. | Refined Findings | |
Publish/Conference | Consider publishing initial findings or presenting at a conference. | Paper/Presentation | |
Complete Data Collection | Finish gathering all necessary data. | Finalized Data | |
Update Thesis Draft | Update your thesis draft with the complete analysis. | Updated Draft |
Year & Quarter | Activity/Milestone | Description | Outcome/Output |
---|---|---|---|
Thesis Writing | Focus primarily on writing your thesis. | Near-final draft | |
Peer Review | Have peers or mentors review the thesis draft. | Feedback for refinement | |
Thesis Submission | Finalize and submit your thesis for review. | Submitted Thesis | |
Defense Preparation | Prepare for your thesis defense. | Defense Presentation | |
Thesis Defense | Defend your thesis in front of a committee. | Committee’s Decision | |
Revisions (if needed) | Make any revisions recommended by the committee. | Final Thesis | |
Final Submission | Submit the finalized thesis. | PhD Thesis | |
Graduation | Complete any remaining formalities. | PhD Awarded |
Free Gantt chart excel template
Here is a free template you can modify for your own research:
Example Gantt chart for a USA PhD
Here are some common steps involved in completing a PhD, which I’ll use to create the Gantt chart:

- Orientation and Coursework (Semester 1) : Familiarization with the university, department, and coursework.
- Coursework (Semester 2) : Continued coursework and possible teaching/research assistantships.
- Select Advisor and Research Topic : Usually done towards the end of the first year or the beginning of the second year.
- Preliminary Research : Initial research and literature review.
- Complete Coursework (Semester 3) : Wrap up any remaining required courses.
- Research Proposal : Develop a full research proposal including methodology.
- Qualifying Exams : Exams to transition from a Ph.D. student to a Ph.D. candidate.
- Begin Research : Start of actual research based on the approved proposal.
- Conduct Research : Data collection, experiments, and analysis.
- Intermediate Review : A review to assess the progress of the research.
- Write Papers : Start writing papers and possibly publishing in journals.
- Finalize Research : Final experiments and data analysis.
- Write Dissertation : Writing the actual Ph.D. dissertation.
- Dissertation Defense : Defending the dissertation before the committee.
- Graduation : Completing all requirements and graduating.
Example Gantt chart for a UK, European and Australian PhD
For Ph.D. programs outside the United States, especially in Europe and some other parts of the world, students often go straight into research without the need for coursework. Here are some common steps for such programs:

- Orientation : Familiarization with the university and department.
- Select Advisor and Research Topic : Usually done at the beginning of the program.
Wrapping up
The journey to earning a PhD is complex and demanding, filled with academic milestones from coursework to research to dissertation writing.
The key to a smooth and efficient doctoral journey lies in well-planned time management—a structured PhD timeline.
This blog serves as an invaluable guide, offering detailed tips for planning out each academic year in both U.S. and international PhD programs. It emphasizes the importance of starting with an outline of major requirements and factoring in “failure time” for unforeseen challenges.
For those looking to navigate their PhD journey in three years or beyond, having a flexible but comprehensive timeline can be the compass that guides them successfully through the academic labyrinth.
Whether you’re just starting out or already deep into your research, the principles and strategies outlined here can help streamline your path to that coveted doctoral hood.

Dr Andrew Stapleton has a Masters and PhD in Chemistry from the UK and Australia. He has many years of research experience and has worked as a Postdoctoral Fellow and Associate at a number of Universities. Although having secured funding for his own research, he left academia to help others with his YouTube channel all about the inner workings of academia and how to make it work for you.
Thank you for visiting Academia Insider.
We are here to help you navigate Academia as painlessly as possible. We are supported by our readers and by visiting you are helping us earn a small amount through ads and affiliate revenue - Thank you!

2024 © Academia Insider

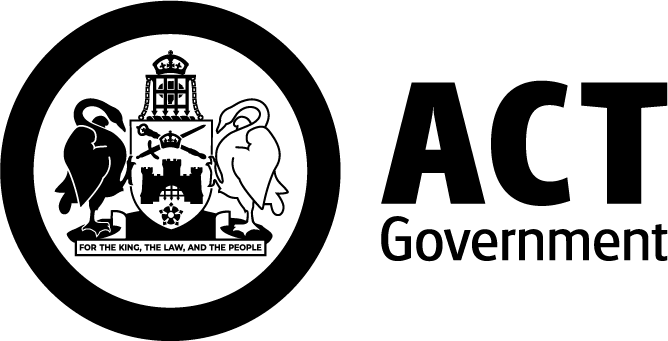
- Skilled migrants
Doctorate streamlined nomination
The ACT offers streamlined nomination for holders of a Doctorate completed at an ACT university. You will benefit from a simplified application process with minimal supporting documentation, priority processing and fee free.
Your nominated occupation does not have to be on the ACT Critical Skills List. You may nominate any occupation on the relevant Department of Home Affairs List of Eligible Skilled Occupations as long as you have the mandatory skill assessment.
Step 1: Check your eligibility
Carefully check the details on the Department of Home Affairs website for your chosen visa type to make sure that you:
- choose the right visa for your situation.
- meet all of Department of Home Affairs' eligibility criteria for that visa.
Canberra resident
You are eligible to apply for ACT doctorate streamlined nomination if you have:
- Lived in Canberra for at least 12 months at time of invitation.
- Completed a professional or research doctoral degree at an ACT university.
Interstate resident
If you are living in another state or territory, you are eligible to apply for ACT doctorate streamlined nomination if you were awarded a professional or research doctoral degree from an ACT university within the last two years.
Overseas applicant
If you are living overseas, you are eligible to apply for ACT doctorate streamlined nomination if you were awarded a professional or research doctoral degree from an ACT university within the last two years.
Step 2: Expressing an interest in ACT nomination
You may express your interest in ACT doctorate streamlined nomination by completing the online Canberra Matrix. You are not required to claim Matrix points.
Completing the Matrix
- Enter your personal information.
- Select either ACT 491 nomination or ACT 190 nomination.
- Select Completed a doctorate at an ACT university.
- Select ‘Canberra resident’ or ‘Interstate / Overseas applicant’.
Step 3: Applying for ACT nomination
Once the Canberra Matrix is submitted, you will receive an invitation email with a link to the online application within seven working days.
The application for ACT nomination must be lodged within 14 days of date of invitation. If the application is not lodged within 14 days, the invitation will automatically expire.
Step 4: Document checklist
You must attach the following information to the online application:
- A suitable skill assessment in your nominated occupation and meet the Department of Home Affairs points test (currently 65 points).
- Select the ACT as your preferred location to live in Australia. If the ACT is not selected, you will not meet the genuine commitment criteria for ACT nomination.
- Meet the Department of Home Affairs criteria at the date of ACT nomination decision. The ACT is unable to nominate an applicant who does not meet the age criteria, has an expired skill assessment or English test result.
- Skilled Occupation: Current skill assessment in the nominated occupation.
- Doctorate course completion letter or Doctorate award from an ACT university (if Canberra resident).
- Doctorate award from an ACT university (if interstate or overseas).
No fee: The application for ACT doctorate streamlined nomination is free.
Average processing time: We will normally process your application for doctorate streamlined nomination within one week.
Browse Theses
January 2004
- Massachusetts Institute of Technology
- Supervisor:
- 201 Vassar Street, W59-200 Cambridge, MA
- United States

Matrices that can be factored into a product of two simpler matrices can serve as a useful and often natural model in the analysis of tabulated or high-dimensional data. Models based on matrix factorization (Factor Analysis, PCA) have been extensively used in statistical analysis and machine learning for over a century, with many new formulations and models suggested in recent years (Latent Semantic Indexing, Aspect Models, Probabilistic PCA, Exponential PCA, Non-Negative Matrix Factorization and others). In this thesis we address several issues related to learning with matrix factorizations: we study the asymptotic behavior and generalization ability of existing methods, suggest new optimization methods, and present a novel maximum-margin high-dimensional matrix factorization formulation. (Copies available exclusively from MIT Libraries, Rm. 14-0551, Cambridge, MA 02139-4307. Ph. 617-253-5668; Fax 617-253-1690.)
- Hazan E, Kalai A, Kanade V, Mohri C and Sun Y Partial matrix completion Proceedings of the 37th International Conference on Neural Information Processing Systems, (30134-30145)
- Kadri H, Ayache S, Huusari R, Rakotomamonjy A and Ralaivola L Partial trace regression and low-rank Kraus decomposition Proceedings of the 37th International Conference on Machine Learning, (5031-5041)
- Cai C, Poor H and Chen Y Uncertainty quantification for nonconvex tensor completion Proceedings of the 37th International Conference on Machine Learning, (1271-1282)
- Yu Y, Peng J and Yue S (2019). A new nonconvex approach to low-rank matrix completion with application to image inpainting, Multidimensional Systems and Signal Processing , 30 :1 , (145-174), Online publication date: 1-Jan-2019 .
- Min J, Jin K, Unser M and Ye J (2018). Grid-Free Localization Algorithm Using Low-Rank Hankel Matrix for Super-Resolution Microscopy, IEEE Transactions on Image Processing , 27 :10 , (4771-4786), Online publication date: 1-Oct-2018 .
- Huang S and Wolkowicz H (2018). Low-rank matrix completion using nuclear norm minimization and facial reduction, Journal of Global Optimization , 72 :1 , (5-26), Online publication date: 1-Sep-2018 .
- Hutchinson B, Ostendorf M and Fazel M (2015). A sparse plus low-rank exponential language model for limited resource scenarios, IEEE/ACM Transactions on Audio, Speech and Language Processing , 23 :3 , (494-504), Online publication date: 1-Mar-2015 .

- Huang B, Ma S and Goldfarb D (2013). Accelerated Linearized Bregman Method, Journal of Scientific Computing , 54 :2-3 , (428-453), Online publication date: 1-Feb-2013 .
- Grosse R, Salakhutdinov R, Freeman W and Tenenbaum J Exploiting compositionality to explore a large space of model structures Proceedings of the Twenty-Eighth Conference on Uncertainty in Artificial Intelligence, (306-315)
- Narita A, Hayashi K, Tomioka R and Kashima H Tensor factorization using auxiliary information Proceedings of the 2011 European conference on Machine learning and knowledge discovery in databases - Volume Part II, (501-516)
- Narita A, Hayashi K, Tomioka R and Kashima H Tensor factorization using auxiliary information Proceedings of the 2011th European Conference on Machine Learning and Knowledge Discovery in Databases - Volume Part II, (501-516)
- Raymond R and Kashima H Fast and scalable algorithms for semi-supervised link prediction on static and dynamic graphs Proceedings of the 2010 European conference on Machine learning and knowledge discovery in databases: Part III, (131-147)
- Raymond R and Kashima H Fast and scalable algorithms for semi-supervised link prediction on static and dynamic graphs Proceedings of the 2010th European Conference on Machine Learning and Knowledge Discovery in Databases - Volume Part III, (131-147)
- Seldin Y and Tishby N (2010). PAC-Bayesian Analysis of Co-clustering and Beyond, The Journal of Machine Learning Research , 11 , (3595-3646), Online publication date: 1-Mar-2010 .
- Srebro N and Shraibman A Rank, trace-norm and max-norm Proceedings of the 18th annual conference on Learning Theory, (545-560)
- Tian D, Mansour H and Vetro A Depth-weighted group-wise principal component analysis for video foreground/background separation 2015 IEEE International Conference on Image Processing (ICIP), (3230-3234)
Save to Binder
- Publication Years 2001 - 2024
- Publication counts 102
- Citation count 3,194
- Available for Download 39
- Downloads (cumulative) 13,892
- Downloads (12 months) 1,886
- Downloads (6 weeks) 354
- Average Downloads per Article 356
- Average Citation per Article 31
- Publication Years 1993 - 2024
- Publication counts 127
- Citation count 2,705
- Available for Download 35
- Downloads (cumulative) 8,006
- Downloads (12 months) 1,441
- Downloads (6 weeks) 246
- Average Downloads per Article 229
- Average Citation per Article 21
Recommendations
Localization of matrix factorizations.
Matrices with off-diagonal decay appear in a variety of fields in mathematics and in numerous applications, such as signal processing, statistics, communications engineering, condensed matter physics, and quantum chemistry. Numerical algorithms dealing ...
Convex and Semi-Nonnegative Matrix Factorizations
We present several new variations on the theme of nonnegative matrix factorization (NMF). Considering factorizations of the form X=FG^T, we focus on algorithms in which G is restricted to containing nonnegative entries, but allowing the data matrix X to ...
Matrix factorizations, triadic matrices, and modified cholesky factorizations for optimization
Export citations.
- Please download or close your previous search result export first before starting a new bulk export. Preview is not available. By clicking download, a status dialog will open to start the export process. The process may take a few minutes but once it finishes a file will be downloadable from your browser. You may continue to browse the DL while the export process is in progress. Download
- Download citation
- Copy citation
We are preparing your search results for download ...
We will inform you here when the file is ready.
Your file of search results citations is now ready.
Your search export query has expired. Please try again.

IMAGES
VIDEO
COMMENTS
Applications to the MA/PhD and PhD open on 1 September 2024. The closing date is December 2, 2024. A NOTE ON THIS DUE DATE: This application due date is real and is a completion due date. ... Ph.D. applicants must show proof of completion of a master's degree prior to starting the program, though it is common for those applying to the Ph.D ...
PhD Completion Process. PhD Committee Matrix. All Members of the committee must have an earned doctorate. For Qualifying exam (QUAL): 3 members, with at least two eligible to be internal members for the relevant Ph.D. program. For Oral Defense (DEFENSE): At least 4 members: 1 Chair- must be tenure-eligible faculty without primary affiliation ...
The final requirement in earning a PhD degree is the completion and defense of the doctoral dissertation. Understanding the steps and associated deadlines in the dissertation submission and degree conferral process is necessary to establish a successful plan. For complete descriptions of the process, please review the Regulations and University ...
From a technical perspective, we generalize results from the matrix completion literature by allowing the patterns of missing data to have a time series dependency structure. We also present new insights concerning the connections between the interactive fixed effects models and the literatures on program evaluation under unconfoundedness as ...
Iterative Matrix Completion and Topic Modeling Using Matrix and Tensor Factorizations. Lara Kassab Advisor: Prof. Henry Adams. Committee: Prof. Bailey Fosdick, Prof. Michael Kirby, Prof. Chris Peterson. Colorado State University, Department of Mathematics PhD Defense, October 19, 2021. Overview. Overview. We propose three techniques that take ...
Matrix completion is a collaborative filtering technique for finding the best products to recommend. To start, collaborative filteringis a strategy of recommendation based on similarity of past consumption patterns by users. For example, suppose user A and user B have rated movies in a similar manner in the past.
Ziye Ma. PhD Candidate at EECS, UC Berkeley. Follow. AOE; Email; LinkedIn; Github; Google Scholar; Solving Matrix Completion as Noisy Matrix Sensing
I've been working on a matrix completion project for a while; ever since I saw Athey, Bayati, Doudchenko, Imbens, and Khosravi 2017 paper (now updated at NBER 2018).I thought I'd share what I've learned, which is still very primitive. ... Summer funding for NYU Politics PhD students [2024 Spring] POLS GA 1251 Quant II [x2022 Fall] POLS GA 3200 ...
This leads to a matrix that well-approximates the original (incomplete) matrix, but has lower complexity according to the nuclear norm for matrices. We generalize results from the matrix completion literature by allowing the patterns of missing data to have a time series dependency structure that is common in social science applications.
Exit surveys submitted to the Completion Project should be completed anonymously by students who are either completing the Ph.D. or withdrawing from their doctoral degree program prior to completion. The survey templates provided by the Completion Project consists of two parts, a generic demographic information sheet and the survey itself.
Recently Prof. Scott Cunningham initiated a series on Matrix Completion (MC) for causal inference starting from the influential paper of Athey et al. (2021). As someone who has been actively working in the field and has authored three papers involving Matrix Completion (MC), I'm excited to share a quick introduction to MC, its importance in causal inference, and where beginners can find ...
Presenter: Zerui Zhang, PhD Candidate in Bioinformatics and Statistics Title: Topics in Genomic Selection and Matrix Completion Abstract: Genomic selection in plant breeding is a common technique to explore molecular genetic markers and accumulate favorable ones to assist in trait selection.We aim to incorporate simulation-based operations research into decision making under uncertainties ...
1959 PhD with Friedrich L. Bauer Professor positions at several universities in the United States, Canada and Mexico. Picture by Claude Brezinski 1974. ... Our matrix completion problem is a xed point problem. 20/34 Alternative Implementation of "-Algorithm Don't store the whole "-scheme, keep only last row and diagonal step i 0 e i = x i e
Previously, I completed my PhD degree from University of Pennsylvania, ... (NLP)) or matrix form (e.g., matrix completion). I am actively looking for motivated graduate and undergraduate students (and colleagues who are interested in collaborating). The topics include all areas related to Information Systems, Operations Management, and ...
Create Your PhD Timeline for a 3 year completion. Creating a timeline for a 3-year PhD program requires careful planning, as you'll have multiple milestones and tasks to complete. This timeline may vary depending on your specific field, institution, or country, but here is a general outline you can use as a starting point: ...
Matrix Rank Minimization with Applications Maryam Fazel Information Systems Lab Electrical Engineering Department Stanford University April 17, 2001 PhD Orals Exam. Outline 1. Problem statement and motivation 2. Existing approaches 3. New approaches and results: 3.1. Trace and log-det heuristics for PSD matrices
Suppose the by matrix (with <) we are trying to recover has rank.There is an information theoretic lower bound on how many entries must be observed before can be uniquely reconstructed. The set of by matrices with rank less than or equal to is an algebraic variety in with dimension (+).Using this result, one can show that at least entries must be observed for matrix completion in to have a ...
dition matrix methods for topic modeling. This dissertation will investigate three popular tech-niques used in the data sciences: (i) structured matrix completion, (ii) supervision-aware di-mensionality reduction, and (iii) dynamic topic modeling. In Chapter 2, we describe our work [88] on iterative methods for structured matrix comple-tion ...
The Ph.D. Completion Project is a seven-year, grant-funded project that addresses the issues surrounding Ph.D. completion and attrition. The Council of Graduate Schools (CGS), with generous support from Pfizer Inc and the Ford Foundation, has provided funding in two phases to 29 major U.S. and Canadian research universities to create intervention strategies and pilot projects, and to evaluate ...
Doctorate streamlined nomination. The ACT offers streamlined nomination for holders of a Doctorate completed at an ACT university. You will benefit from a simplified application process with minimal supporting documentation, priority processing and fee free. Your nominated occupation does not have to be on the ACT Critical Skills List.
Learning with matrix factorizations. Matrices that can be factored into a product of two simpler matrices can serve as a useful and often natural model in the analysis of tabulated or high-dimensional data. Models based on matrix factorization (Factor Analysis, PCA) have been extensively used in statistical analysis and machine learning for ...
This article is the first in a series of articles on low-rank matrix completion of longitudinal data. The article is loosely based on the paper Langberg, et al. "Matrix factorization for the…
matrix of preferences or observations with its transpose. Oftentimes, such real-world matrices are missing many entries and a fundamental data-analysis task, known by the term PD-matrix completion, is the inference of these missing entries. In this paper, we introduce the active version of PD-matrix completion, in which we assume