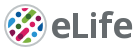
- Review Article
- Neuroscience

Open-source tools for behavioral video analysis: Setup, methods, and best practices
- Kevin Luxem
- Jennifer J Sun
- Sean P Bradley
- Keerthi Krishnan
- Jan Zimmermann

- Cellular Neuroscience, Leibniz Institute for Neurobiology, Germany ;
- Department of Computing and Mathematical Sciences, California Institute of Technology, United States ;
- Rodent Behavioral Core, National Institute of Mental Health, National Institutes of Health, United States ;
- Department of Biochemistry and Cellular & Molecular Biology, University of Tennessee, United States ;
- Department of Biological Sciences, Carnegie Mellon University, United States ;
- Department of Neuroscience, University of Minnesota, United States ;
- The Salk Institute of Biological Studies, United States ;
- Department of Neuroscience, American University, United States ;
- Open access
- Copyright information
Share this article
Cite this article.
- Talmo D Pereira
- Mark Laubach
- Copy to clipboard
- Download BibTeX
- Download .RIS
Quantitative tools for video analysis
A basic setup for video recordings in animal experiments, best practices for experimenters and developers, article and author information.
Recently developed methods for video analysis, especially models for pose estimation and behavior classification, are transforming behavioral quantification to be more precise, scalable, and reproducible in fields such as neuroscience and ethology. These tools overcome long-standing limitations of manual scoring of video frames and traditional ‘center of mass’ tracking algorithms to enable video analysis at scale. The expansion of open-source tools for video acquisition and analysis has led to new experimental approaches to understand behavior. Here, we review currently available open-source tools for video analysis and discuss how to set up these methods for labs new to video recording. We also discuss best practices for developing and using video analysis methods, including community-wide standards and critical needs for the open sharing of datasets and code, more widespread comparisons of video analysis methods, and better documentation for these methods especially for new users. We encourage broader adoption and continued development of these tools, which have tremendous potential for accelerating scientific progress in understanding the brain and behavior.
Traditional approaches to analyzing video data have involved researchers watching video playback and noting the times and locations of specific events of interest. These analyses are very time-consuming, require expert knowledge in the target species and experimental design, and are prone to user bias ( Anderson and Perona, 2014 ). Video recordings are often made for many different animals and behavioral test sessions, but only reviewed for a subset of experiments. Complete sets of videos are rarely made accessible in published studies and the analysis methods are often vaguely described. There are variations in scoring criteria across researchers and labs, even over time by a single researcher. Collectively, these issues present major challenges for research reproducibility and the difficulty and cost of manual video analysis has led to the dominance of easy-to-use measures (lever pressing, beam breaks) in the neuroscience literature, and this has limited our understanding of brain-behavior relationships ( Krakauer et al., 2017 ).
For example, ‘reward seeking’ has been a popular topic in recent years and is typically measured using beam breaks between response and reward ports located inside an operant arena (e.g., Cowen et al., 2012 ; Feierstein et al., 2006 ; Lardeux et al., 2009 ; van Duuren et al., 2009 ). By relying only on the discrete times when animals make a choice and receive a reward, it is not possible to describe how the animal moves during a choice or how it collects a reward. Animals may not move in the same way to a reward port when they expect a larger or smaller reward (e.g., Davidson et al., 1980 ). This could lead to, for example, a neural recording study labeling a cell as ‘reward encoding’ when it actually reflects differences in movement.
Commercial products (e.g., Ethovision by Noldus, Any-Maze by Stoelting) and open-source projects (e.g., JAABA: Kabra et al., 2013 ; SCORHE: Salem et al., 2015 ; OptiMouse: Ben-Shaul, 2017 ; ezTrack: Pennington et al., 2019 ) are available for semi-automated annotation and tracking of behaviors. These methods track animals based on differences between the animals and the background color or luminance. This can be challenging to do in naturalistic settings or for species or strains that do not have a uniform color (e.g., Long-Evans rats). These methods provide estimates of the overall position of an animal in its environment and can be used to measure the direction and velocity of its movements. These ‘center of mass’ tracking methods could be used to measure where an animal is and how fast it is moving. More sophisticated versions of these products may also detect the head and tail of common laboratory species such as rodents or zebrafish and draw inferences from the shape and location of the animal to classify a small subset of an animal’s behavioral repertoire. However, these simpler tracking methods cannot account for movements of discrete sets of body parts (e.g., head scanning in rodents, which is associated with a classic measure of reward-guided decisions called ‘vicarious trial-and-error’ behavior: see Redish, 2016 , for review).
More advanced analyses could be used to quantify movements across many pixels simultaneously in video recordings. For example, Stringer et al., 2019 , used dimensionality reduction methods to study the spontaneous coding of visual- and movement-related information in the mouse visual cortex in relation to facial movements. Musall et al., 2019 , used video recordings of motion data from several parts of the face of mice as they performed a decision-making task and related the measures from the video recordings to cortical imaging data. While these analyses would go beyond what is possible to achieve with a simple tracking method, the multivariate methods developed by Stringer and Musall are not themselves capable of categorizing movements, measuring transitions between different types of movements, or quantifying the dynamics of movement sequences. For these measures, a different approach is needed.
Methods for capturing the pose of an animal (the location and configuration of its body) have emerged in recent years (e.g., DeepLabCut: Mathis et al., 2018a ; SLEAP: Pereira et al., 2022 ). These methods can provide a description of an animal’s movement and posture during a behavioral task. They can be used to understand the dynamics of naturalistic movements and behaviors, as illustrated in Figure 1 . Pose estimation methods provide information on the position and orientation of multiple parts of an animal, with recent methods being able to measure pose information for groups of animals ( Chen et al., 2020 ; Lauer et al., 2021 ; Pereira et al., 2022 ; Walter and Couzin, 2021 ). Some recent methods now even allow for pose estimation to be run in real experimental time ( Kane et al., 2020 ; Lopes et al., 2015 ; Pereira et al., 2022 ; Schweihoff et al., 2021 ).
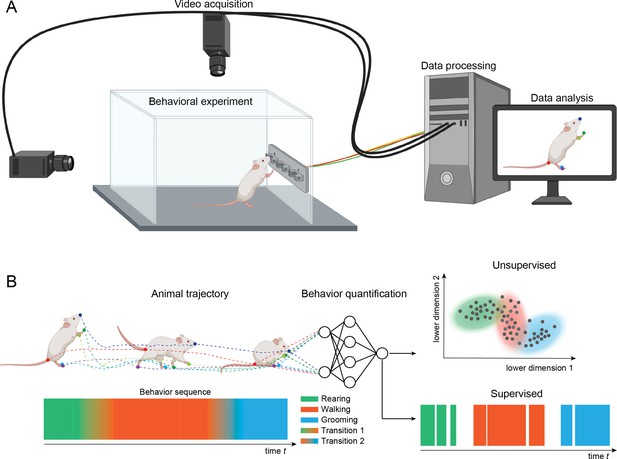
Setup for video recording.
( A ) Cameras are mounted above and to the side of a behavioral arena. The cameras record sequences of images of an animal performing a behavioral task. The recordings are stored on a computer and analyzed with methods for pose estimation and behavior classification. ( B ) The animal’s pose trajectory captures the relevant kinematics of the animal’s behavior and is used as input to behavior quantification algorithms. Quantification can be done using either unsupervised (learning to recognize behavioral states) or supervised (learning to classify behaviors based on human annotated labels). In this example, transitions among three example behaviors (rearing, walking, and grooming) are depicted on the lower left and classification of video frames into the three main behaviors are depicted on the lower right.
Methods for pose estimation emerged in computer vision research in the late 1970s ( Marr et al., 1978 ; Nevatia and Binford, 1973 ). The methods became widely available for the analysis of pose in human behavior following improvements in computer vision ( Behnke, 2003 ), deep learning ( Szegedy et al., 2013 ), and computing using graphical processing units (GPUs) ( Oh and Jung, 2004 ). However, these methods were often not robust or required a lot of training data, which were at the time not easily available for animal studies. As a result, a number of open-source tools emerged for pose estimation in animals (e.g., DeepLabCut: Mathis et al., 2018a , LEAP: Pereira et al., 2019 ; DeepPoseKit: Graving et al., 2019a ). These tools are especially notable in that they were developed to address specific scientific questions by researchers and are not available from commercial sources. They are an outstanding example of the ‘open-source creative process’ ( White et al., 2019 ).
One of these methods, DeepLabCut, has been shown to outperform the commercial software package EthoVision XT14 and a hardware-based measurement system from TSE Systems, based on IR beam breaks ( Sturman et al., 2020 ). When tested across a set of common behavioral assays used in neuroscience (open field test, elevated plus maze, forced swim test), data from the pose estimation method was evaluated using a neural network classifier and performed as well as classifications by human experts, required data from fewer animals to detect differences due to experimental treatments, and in some cases (head dips in an elevated plus maze) detected effects of treatment (a drug) that was not detected by EthoVision.
In the case of reward seeking behavior, human annotation of videos could resolve the animal’s position and when and for how long specific behaviors occurred. These measurements could be made by annotating frames in the video recordings, using tools such as the VIA annotator ( Dutta and Zisserman, 2019 ), and commercial (e.g., EthoVision) or open-source (e.g., ezTrack) methods for whole-animal tracking. These measurements would not be able to account for coordinated movements of multiple body parts or for the dynamics of transitions between different behaviors that together comprise reward seeking behavior. These measurements are easily made using methods for pose estimation. These methods learn to track multiple body parts (for a rodent, the tip of snout, the ears, the base of the tail) and the positions of these body parts can be compared for different kinds of trials (small or large reward) using standard statistical models or machine learning methods. These analyses, together, allow for movements to be categorized (e.g., direct and indirect approach toward a reward port) and for transitions between different types of movements to be quantified (e.g., from turning to walking). It would even be possible to detect unique states associated with deliberation (e.g., head scanning between available choice options). All these measures could then be compared as a function of an experimental manipulation (drug or lesion) or used to assist in the analysis of simultaneously collected electrophysiological or imaging data. None of these measures are possible using conventional methods for annotating video frames or tracking overall the overall position of the animal in a behavioral arena.
Pose estimation methods have been crucial for several recent publications on topics as diverse as tracking fluid consumption to understand the neural coding of reward prediction errors ( Ottenheimer et al., 2020 ), accounting for the effects of wind on the behavior of Drosophila ( Okubo et al., 2020 ), understanding the contributions of tactile afferents and nociceptors to the perception of touch in freely moving mice ( Schorscher-Petcu et al., 2021 ), understanding interactions between tactile processing by the rodent whisker system and its ability to guide locomotion ( Warren et al., 2021 ), and measuring the relationship between eye movements and neural activity in freely behaving rodents ( Keshavarzi et al., 2022 ). While a number of studies are emerging that take advantage of methods for pose estimation, there is still not enough widespread adoption of the methods across the research community, perhaps in part due to the technical nature of collecting high-quality video recordings as well as setting up and using methods for pose estimation. These methods depend on access to computing systems with GPUs and the ability to set up and use the required computer software, which is usually available as computer code written in Python or MATLAB. A researcher who wants to get started with these approaches will therefore face a number of questions about how to set up video methods in a laboratory setting. New users may also need to learn some of the jargon associated with video analysis methods, and some of these terms are defined in Table 1 . The primary goals of this document are twofold: to provide information for researchers interested in setting methods for video analysis in a research lab and to propose best practices for the use and development of video analysis methods .
Frequently used terms for video analysis.
pose | The configuration (position and/or orientation) of an animal, object, or body parts in an image or video recording |
keypoints/landmarks | Distinct identifiable morphological features (e.g., the tip of the snout or the base of the tail in a rodent) that can be localized in 2D or 3D from images, typically via pose estimation |
part grouping | A process for assigning keypoints to individual animals |
multi-object tracking | In multi-animal pose tracking, the task of determining which detected poses belong to which individual animal across time |
re-identification | A process for identifying all images containing the same individual animal based primarily on their distinct appearance |
kinematics | Information about the angles and velocities of a set of keypoints |
supervised learning | Machine learning methods that use experimenter-provided labels (e.g., ground truth poses, or ‘running’ vs ‘grooming’) to train a predictive model |
unsupervised learning | Machine learning methods that only use unlabeled data to find patterns based on its intrinsic structure (e.g., clustering behavioral motifs based on the statistics of their dynamics) |
transfer learning | Machine learning methods that use models trained on one dataset to analyze other datasets (e.g., models of grooming in mice applied to rats) |
self-supervised learning | Machine learning methods that use only unlabeled data for training by learning to solve artificially constructed tasks (e.g., comparing two variants of the same image with noise added against other images; predicting the future; or filling in blanks) |
embedding | A representation of high-dimensional data into lower dimensional representation |
lifting | A process through which 2D pose data are converted to 3D representations |
behavioral segmentation | A process for detecting occurrences of behaviors (i.e., starting and ending frames) from video or pose sequences |
In a typical setup for video recording, cameras are placed above, and in some cases to the side or below, the behavioral arena ( Figure 1 ). The cameras send data to a computer and can be integrated with inputs from behavioral devices using custom-written programs using popular libraries such as OpenCV ( Bradski, 2000 ), open-source data collection systems such as Bonsai ( Lopes et al., 2015 ), or software included with many common commercial video capture boards (loopbio Motif). Video files can then be analyzed using a variety of open-source tools.
A common approach is to use methods for pose estimation, which track the position and orientation of the animal. This is done by denoting a set of ‘keypoints’ or “landmarks” (body parts) in terms of pixel locations on frames in the video recordings. Packages for pose estimation provide graphical user interfaces for defining keypoints and the keypoints are then analyzed with video analysis methods. In the example shown in Figure 1 , keypoints are the colored dots on the tip of the snout, the ears, forelimbs and paw, midpoint of back, hindlimbs and paws, and base, middle, and end of tail. Once body parts have been defined, computer algorithms are used to track the skeleton formed by the points and to track the position and orientation of the skeleton over frames in the video file. Many open-source tools use machine learning methods for these intensive computational processes, which require GPUs to run in reasonable time. To run these analyses, many labs have either dedicated computers, institutional computing clusters, or cloud computing services such as Google Colab. The outputs of pose estimation can be analyzed to account for movement variability associated with different behaviors, to relate position and orientation to simultaneously collected brain activity (electrophysiology, optical imaging), or with algorithms that can describe and predict states and dynamical transitions of behaviors.
Data acquisition
The first step in setting up for video recording is to purchase a camera with an appropriate lens. Researchers should determine if they need precisely timed video frames, for example, for integration with electrical or optical recordings. Inexpensive USB webcams with frame rates of at least 30 fps are suitable for many neuroscience experiments. However, it is important to make sure that each camera is connected to a dedicated USB channel in the computer used for video recording. Webcam cameras can be a challenge to integrate with systems used for behavioral control and electrophysiology or imaging because they lack a means of precisely synchronizing video frames to other related data. As such, the timing of specific behaviors must be based on the animal’s location or an observable event in the video field (e.g., onset of an LED indicating reward availability).
For more precise recordings, specialized cameras used in computer vision applications are needed (e.g., FLIR, Basler). Power and combined data over Ethernet (GigE PoE) is commonly used as it combines long cable length headroom with joint DC power delivery. Alternatively, USB3 cameras can be used, but have a maximum data cable length of 5 m, although active extender cables are available. Most machine vision cameras (GigE PoE or USB3) have general-purpose input output capabilities that allow for time synchronization of multiple cameras with other laboratory equipment (e.g., electrical or optical recording system). A single camera running at high resolution or frame rate can quickly saturate a standard 1 Gbit Ethernet link. Therefore, it is important to consider the computer used to collect video data, ensuring that it has a fast processor with multiple cores and perhaps also a GPU, which can aid in handling video compression during data collection and can be used for off-line analysis using pose estimation methods.
After choosing a camera, researchers must determine how to save and archive data from their recordings. By default, recorded videos from cameras may be in several formats, such as MP4 (MPEG-4 AVC/H. 264 encoding), MOV (MPEG-4 encoding), and AVI (DivX codec, higher quality but larger file size). These formats are generally universal and can be read by a variety of tools. Generally, video data files tend to be large (1 hr of RGB video at 30 Hz with resolution 1000×1000 can be 2–20 GB depending on compression) so data storage solutions for large-scale experiments are crucial. File compression should be evaluated before a system is deployed, as the computer used for video recordings must have sufficient memory (RAM) to remain stable over long recording sessions. In addition to considerations of file formats and codecs, it is important to plan for data storage. Many labs maintain internal lab servers for their video data. Cloud storage is another option to enable sharing. For sharing data publicly, there are a variety of hosting services available, such as the Open Science Foundation, Figshare, and Dryad (see section on ‘Best practices for experimenters and developers’ below for further comments on data archives and sharing).
Once cameras and lenses are acquired and data formats and storage resolved, the next question is where to position the cameras relative to the experimental preparation. Occlusions due to obstacles, cables, or conspecifics will have effects on the usability of some video analysis methods. A bottom-up view (from below the animal) works best in an open-field, while a top-down approach can be useful for studies in operant chambers and home cages. Bottom-up views capture behavioral information from the position of the animal’s feet ( Hsu and Yttri, 2021a ; Luxem et al., 2022a ). When multiple cameras are used, to reduce the effect of occlusion for downstream video analysis, cameras should be positioned such that at least one camera can visualize each keypoint at all times.
It is also necessary to think about lighting for the experimental setup. If all or some of the study is to be performed while house lights are off, then infrared (IR) lighting and IR-compatible cameras may be needed. One should consider if diffuse lighting will work or if modifications to eliminate reflective surfaces (especially metals) are necessary. These can lead to artifacts in video recordings from devices like IR LEDs and other sources of illumination and complicate the training and interpretations of measures obtained with analyses such as pose estimation. For example, it is possible to reduce reflections from surfaces and objects that are in direct line with IR LEDs. For top-down recordings, cage floors can be made from colored materials to provide contrast such as Delrin or pre-anodized aluminum (an option for long-term use) and the metal pans typically used below operant chambers to collect animal waste can be painted with flat black paint. Addressing these issues before beginning an experiment can greatly improve the quality of video recordings.
Finally, for some applications, it is necessary to invest time in calibrating the video system. Calibration is often overlooked and not easily accessible in many current software packages. The intrinsic parameters of a camera include the focal length of the lens and if the lens has obvious distortions (i.e., fisheye lens). Extrinsic parameters also affect the quality of video recordings and are largely due to the camera’s position in the scene. It is fairly easy to calibrate a single camera using a checkerboard or ArUco board. To do so, one sweeps a precalibrated board manually around the field of view of a camera and uses the extracted images to estimate the camera’s intrinsic parameters (focal length and distortions). This approach can scale easily to cameras with overlapping fields of view but becomes difficult if larger camera networks do not share extrinsic parameters or need to be repeatedly recalibrated (e.g., if one of the cameras is moved between experiments). If the environment has enough structure in it, structure from motion can estimate the intrinsic and extrinsic parameters by treating the multiple cameras as an exhaustive sweep of the environment. This process can be fully scripted and automatically performed on a daily basis leading to substantially increased reliability and precision in multi-camera system performance. Several references on these topics include Bala et al., 2020 ; Rameau et al., 2022 ; Schönberger et al., 2016 ; Schonberger and Frahm, 2016 .
Hardware and software for data analysis
Once video recordings are acquired, the researcher may proceed to setting up their computing environment for pose estimation and tracking. Modern markerless motion capture software tools like DeepLabCut ( Mathis et al., 2018a ) and SLEAP ( Pereira et al., 2022 ) rely on deep learning to automate this process. The most compute-intensive step of these methods involves a ‘training’ stage in which a deep neural network is optimized to learn to predict poses from user-provided examples. Training is typically accelerated with a GPU, a hardware component traditionally used for computer graphics, but which has been co-opted for deep learning due to its massively parallel processing architecture. Having a GPU can speed up training by 10- to 100-fold, resulting in model training times in as little as minutes with lightweight network architectures ( Pereira et al., 2022 ). For most researchers, the most practical option is to purchase a consumer-grade workstation GPU which can be installed in conventional desktop computers to afford local access to this hardware from the pose tracking software. In this case, any recent NVIDIA GPU with greater than 6 GB of memory will suffice for practical use of pose estimation tools. This type of computer hardware has, in recent years, been significantly impacted by supply chain shortages, driving prices up to >$1000, which makes this a less accessible option for many labs just starting off in video analysis. For this situation, most tools provide the means for using Google Colab, which provides limited access to GPUs on the cloud. This is an excellent way to set up analysis workflows while getting familiar with deep learning-based video analysis but may not be practical for sustained usage (e.g., processing 100 s of videos). Another common scenario is that institutions with a high-performance computing center will typically have GPUs available as a local shared resource. Other than GPUs, most other computer requirements are modest (modern CPU, 8–16 GB of RAM, minimal disk space).
Researchers will need to set up their software environment to be able to install and use pose tracking tools. Most commonly available open-source methods for pose estimation were developed using the Python language. It is highly recommended to make use of ‘environment managers’ such as Anaconda (‘conda’) which enable the creation of isolated installations of Python for each video analysis method of interest. This allows for the methods to be installed with all its dependencies without affecting other Python libraries on the system. Alternatives include Docker, which allows for running an entire virtual machine in isolation. This is done to facilitate the installation of GPU-related dependencies, which may be technically challenging for novice users.
2D pose estimation and tracking
Pose tracking methods ( Figure 2 , part 1) enable researchers to extract positional information about the body parts of animals from video recordings. Tools for pose tracking (see Table 2 ) decompose the problem of pose tracking into sub-tasks outlined below. A note on nomenclature: pose estimation is the term typically reserved to mean single-animal keypoint localization within a single image; multi-animal pose estimation refers to ; multi-animal pose estimation refers to keypoint localization and part grouping of multiple animals within a single image; and multi-animal pose tracking refers to combined keypoint localization , part grouping, and identification across video frames.
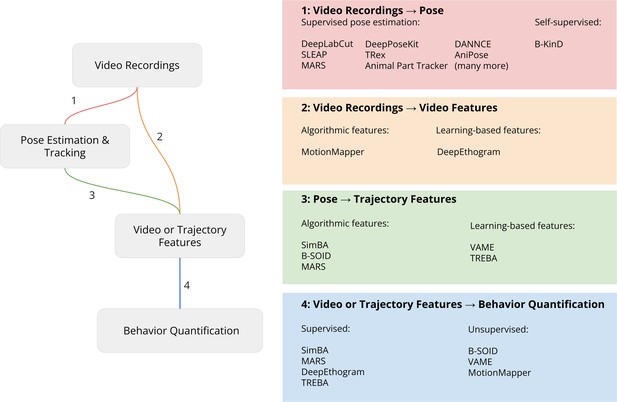
Pipeline for video analysis.
Video recordings are analyzed with either keypoints from 2D or 3D pose estimation or directly by computing video features. These videos or trajectory features are then used by downstream algorithms to relate the keypoints to behavioral constructs such as predicting human-defined behavior labels (supervised learning) or discovering behavior motifs (unsupervised learning). Each part of the analysis steps outlined in the figure is described in more detail below.
Methods for 2D pose estimation.
DeepLabCut ( ; ) uses a popular architecture for deep learning ( ), called ResNet. DeepLabCut models are pre-trained on a massive dataset for object recognition called ImageNet ( ). Through a process called transfer learning, the DeepLabCut model learns the position of keypoints using as few as 200 labeled frames. This makes the model very robust and flexible in terms of what body parts (or objects) users want to label as the model provides a strong backbone of image filters within their ResNet architecture. To detect the keypoint position, DeepLabCut replaces the classification layer of the with deconvolutional layers to produce spatial probability densities from which the model learns to assign high probabilities to regions with the user labeled keypoints. DeepLabCut can provide very accurate pose estimations but can require extensive time for training. | |
SLEAP ( ) is based on an earlier method called LEAP ( ), which performed pose estimation on single animals. SLEAP uses simpler CNN architectures with repeated convolutional and pooling layers. This makes the model more lightweight compared to DLC’s ResNet architecture and, hence, the model is faster to train with comparable accuracy. Similar to DeepLabCut, the model uses a stack of upsampling or deconvolutional layers to estimate confidence maps during training and inference. Unlike DLC, SLEAP does not solely rely on transfer learning from general-purpose network models (though this functionality is also provided for flexible experimentation). Instead, it uses customizable neural network architectures that can be tuned to the needs of the dataset. SLEAP can produce highly accurate pose estimates starting at about 100 labeled frames for training combined and is quick to train on a GPU (<1 hour). | |
DeepPoseKit ( ; ) uses a type of CNN architecture, called stacked DenseNet, an efficient variant of the stacked hourglass ( ), and uses multiple down- and upsampling steps with densely connected hourglass networks to produce confidence maps on the input image. The model uses only about 5% of the amount of parameters used by DeepLabCut, providing speed improvements over DeepLabCut and LEAP. | |
B-KinD ( ; ) discovers key points without human supervision. B-KinD has the potential to transform how pose estimation is done, as keypoint analysis is one of the most time-consuming aspects of doing pose estimation analysis. However, there are challenges for the approach when occlusions occur in the video recordings, e.g., recordings of animals tethered to brain recording systems. |
Keypoint localization involves recovering the spatial coordinates of each distinct keypoint. This is normally done by estimating body part confidence maps, that is, image-based representations that encode the probability of the body part being located at each pixel. Recovering the coordinates of each body part is reduced to the task of finding the pixel with highest probability. A key consideration of this task is that the larger the image, the larger the confidence maps. Computer memory requirements can potentially exceed the capacity of most consumer-grade GPUs. This can be compensated by reducing the resolution of the confidence maps, though this comes at the cost of potentially reduced accuracy. Subpixel refinement methods are typically employed to compensate for this, but ultimately confidence map resolution is one of the most impactful choices for achieving reliable keypoint localization.
For single-animal videos, there will be at most one instance of each keypoint type present in the image, so keypoint localization is the only step strictly required. For multi-animal videos, however, there may be multiple instances of each keypoint type, for example, multiple ‘heads’. Part grouping refers to the task of determining the set of keypoint detections that belong to the same animal within an image. This is often approached in either a bottom-up or top-down fashion. In bottom-up models, all parts are detected, the association between them estimated (e.g., by using part affinity fields: Cao et al., 2017 ), and then grouped. In top-down models, the animals are detected, cropped out of the image, and then keypoints are located in the same fashion as in the single-animal case. These approaches have specific trade-offs. Analyses of bottom-up recordings tend to be more memory-intensive but also more robust to transient occlusions and work well with animals with relatively large bodies (e.g., rodents). By contrast, top-down recordings tend to be analyzed in less time since only subsets of the image are processed. Top-down views work best with smaller body types that have fewer complex occlusions (e.g., flies). A notable consideration is that all single-animal pose estimation models can be used in the multi-animal setting if the animals can be detected and cropped as a preprocessing step ( Graving et al., 2019a ; Pereira et al., 2019 ). While both methods will work on most types of data, drastic improvements in performance and accuracy can be obtained by selecting the appropriate one – most pose estimation tools allow users to select between each approach type.
Once animals are detected and their keypoints located within a frame, the remaining task in multi-animal pose tracking is identification: repeatedly detecting the same animal across frame sequences. This can be approached as a multi-object tracking (MOT) problem, where animals are matched across frames based on a model or assumption about motion; or a re-identification (ReID) problem, where distinctive appearance features are used to unambiguously identify an animal. Both MOT and ReID (and hybrids) are available as standalone functionality in open-source tools, as well as part of multi-animal pose tracking packages. While MOT-based approaches can function on videos of animals with nearly indistinguishable appearances, they are prone to the error propagation issue inherent in methods with temporal dependencies: switching an animal’s identity even once will mean it is wrong for all subsequent frames. This presents a potentially intractable problem for long-term continuous recordings which may be impossible to manually proofread. ReID-like methods circumvent this problem by detecting distinguishing visual features, though this may not be compatible with existing datasets or all experimental paradigms.
The single most significant experimental consideration that will affect the identification problem is whether animals can be visually distinguished. A common experimental manipulation aimed at ameliorating this issue is to introduce visual markers to aid in unique identification of animals. This includes techniques such as grouping animals with different fur colors, painting them with non-toxic dyes ( Ohayon et al., 2013 ), or attaching barcode labels to a highly visible area of their body ( Crall et al., 2015 ). Though an essential part of the pose tracking workflow, identification remains a challenging problem in computer vision and its difficulty should not be underestimated when designing studies involving large numbers of interacting animals. We refer interested readers to previous reviews on multi-animal tracking ( Panadeiro et al., 2021 ; Pereira et al., 2020 ) for more comprehensive overviews of these topics.
Tools that are based on deep learning work by training deep neural networks (models) to reproduce human annotations of behavior. Methods that strictly depend on learning from human examples are referred to as fully supervised. In the case of animal pose tracking, these supervisory examples (labels) are provided in the form of images and the coordinates of the keypoints of each animal that can be found in them. Most pose tracking software tools fall within this designation and provide graphical interfaces to facilitate labeling. The usability of these interfaces is a crucial consideration as most of the time spent in setting up a pose tracking system will be devoted to manual labeling. The more examples and the greater their diversity, the better that pose tracking models will perform. Previous work has shown that hundreds to thousands of labeled examples may be required to achieve satisfactory results, with a single example taking as much as 2 min to manually label ( Mathis et al., 2018a ; Pereira et al., 2022 ; Pereira et al., 2019 ). To mitigate this, we strongly recommend adopting a human-in-the-loop labeling workflow. This is a practice in which the user trains a model with few labels, generates (potentially noisy) predictions, and imports those predictions into the labeling interface for manual refinement before retraining the model. This can drastically reduce the amount of time taken to generate thousands of labeled images necessary for reliable pose estimation models.
The rule of thumb is that ‘if you can see it, you can track it’, but this aphorism strongly depends on the examples provided to train the model. Important factors to consider in the labeling stage include labeling consistency and sample diversity. Labeling consistency involves minimizing the variability of keypoint placement within and across annotators which helps to ensure that models can learn generalizable rules for keypoint localization. This can be accomplished by formalizing a protocol for labeling, especially for ambiguous cases such as when an animal’s body part is occluded. For example, one convention may be to consistently place a ‘paw’ keypoint at the apex of the visible portion of the body rather than guessing where it may be located beneath an occluding object. Similarly, the principle of consistency should inform which body parts are selected as tracked keypoints. Body parts that are not easily located by the human eye will suffer from labeling inconsistency which may cause inferior overall performance as models struggle to find reliable solutions to detecting them. Sample diversity, on the other hand, refers to the notion that not all labeled examples have equal value when training neural networks. For example, labeling 1000 consecutive frames will ensure that the model is able to track data that looks similar to that segment of time, but will have limited capacity to generalize to data collected in a different session. As a best practice, labels should be sampled from the widest possible set of experimental conditions, time points, and imaging conditions that will be expected to be present in the final dataset.
Improving the capability of models to generalize to new data with fewer (or zero) labels is a currently active area of research. Techniques such as transfer learning and self-supervised learning aim to reduce the labeling burden by training models on related datasets or tasks. For example, B-KinD ( Sun et al., 2021a ) is able to discover semantically meaningful keypoints in behavioral videos using self-supervision without requiring human annotations. These approaches work by training models to solve similar problems and/or on similar data than those used for pose estimation, with the intuition that some of that knowledge can be reused and thereby will require fewer (or no) labeled examples before achieving the same performance as fully supervised equivalents. Future work in this domain is on track to produce reusable models for commonly encountered experimental species and conditions. We highly encourage practitioners to adopt open data and model sharing to facilitate these efforts where possible.
3D pose estimation
Several methods have emerged in recent years for 3D tracking based on pose data. For some applications, it is of interest to track animals in complete 3D space. This affords a more detailed representation of the kinematics by resolving ambiguities inherent in 2D projections – an especially desirable property when studying behaviors that involve significant out-of-plane movement, such as in large arenas or non-terrestrial behaviors.
It is important to note that 3D motion capture comes at a significant increase in technical complexity. As discussed above (see ‘Data acquisition’), camera synchronization and calibration are paramount for applications using 3D tracking as the result of this step will inform downstream algorithms as to the relative spatial configuration of the individual cameras. This step may be sensitive to small camera movements that occur during normal operation of behavioral monitoring systems, potentially requiring frequent recalibration. The number and positioning of cameras are also major determinants of 3D motion capture performance, both of which may depend on the specific behavior of interest, arena size and bandwidth, and computing capabilities on the acquisition computer. In some cases, it may be easiest to use mirrors instead of multiple cameras to allow for recording behavior from multiple perspectives.
Given a calibrated camera system, several approaches have emerged that can enable 3D pose estimation in animals. The simplest approaches rely on using 2D poses detected in each camera view, such as those produced by SLEAP or DeepLabCut as described above, and then triangulating them into 3D. 2D poses can be detected by training 2D pose models on each camera view independently, or by training a single model on all views, with varying results depending on how different the perspectives are. Once 2D poses can be obtained, methods such as Anipose ( Karashchuk et al., 2021 ), OpenMonkeyStudio ( Bala et al., 2020 ), and DeepFly3D ( Günel et al., 2019 ) are able to leverage camera calibration information to project poses into 3D for triangulation. This involves optimizing for the best 3D location of each keypoint that still maps back to the detected 2D location in each view. This can be further refined with temporal or spatial constraints, such as known limb lengths. Using this approach, more cameras will usually result in better triangulation, but will suffer (potentially catastrophically) when the initial 2D poses are incorrect. Since many viewpoints will have inherent ambiguities when not all body parts are visible, the 2D pose estimation error issue can be a major impediment to implementing 3D pose systems using the triangulation-based approach.
Alternative approaches attempt to circumvent triangulation entirely. LiftPose3D ( Gosztolai et al., 2021 ) describes a method for predicting 3D poses from single 2D poses, a process known as lifting . While this eliminates the need for multiple cameras, it requires a dataset of known 3D poses from which the 2D-3D correspondences can be obtained. This requirement depends on the multi-camera system being similar to the target 2D systems. DANNCE ( Dunn et al., 2021 ), on the other hand, achieves full 3D pose estimation by extending the standard 2D confidence map regression approach to 3D using volumetric convolutions. In their approach, images from each camera view are projected onto a common volume based on the calibration, before being fed into a 3D convolutional neural network that outputs a single volumetric part confidence map. This approach has the major advantage that it is not susceptible to 2D pose estimation errors since it solves for the 3D pose in a single step while also being able to reason about information present in distinct views. The trade-offs with this approach are that it requires significantly more computational power due to the 3D convolutions, as well as requiring 2D ground truth annotations on multiple views for a given frame.
Overall, a practitioner should be mindful of the caveats with implementing 3D pose estimation and is recommended to consider whether the advantages are truly necessary given the added complexity. We note that at the time of writing, none of the above methods can natively support the multi-animal case in 3D, other than by treating them as individual animals after preprocessing with a 2D multi-animal method for pose estimation. This limitation is due to issues with part grouping and identification as outlined above and would seem to be a future area of growth for animal pose estimation.
Behavior quantification
After using pose estimation to quantify the movements of animal body parts, there are a number of analyses that can be used to understand how movements differ by experimental conditions ( Figure 2 , parts 2–4). A simple option is to use statistical methods such as ANOVA to assess effects on discrete experimental variables such as the time spent in a given location or the velocity of movement between locations. These measures can also be performed with data from simpler tracking methods, such as the commercially available EthoVision, TopScan, and ANY-maze programs. The primary benefits of the open source pose estimation methods described in this paper over these commercially available programs are the richness of the data obtained from pose estimation (see Figure 1 ) and the flexibility and customization of behavioral features are tracked (see Figure 2 ).
If researchers want to go beyond kinematic readouts and investigate the behavior an animal is executing in more detail, then methods for segmenting behavior from the pose tracking data can be used. Behavioral segmentation methods are available to discern discrete episodes of individual events and/or map video or trajectory data to continuous lower-dimensional behavioral representations. Discrete episodes have a defined start and end in which the animal is performing a particular behavior, while continuous representations represent behavior more smoothly over time. For discrete episodes, depending on the experimental conditions, these episodes can last from milliseconds up to minutes or longer. Segmentation can be done per animal, for example, detecting locomotion, or globally per frame, which is especially of interest for social behavior applications. In a global setting researcher might be interested in finding behavioral episodes that are directed between animals such as attacking or mounting behaviors.
If one wants to understand sequences of behaviors, there are many methods available to embed pose data into lower-dimensional representations. Such structures can be discovered through unsupervised methods. Some methods provide generic embeddings and do not explicitly model the dynamics of the behaving animal. Two examples of this approach are B-SOiD ( Hsu and Yttri, 2021a ), which analyses pose data with unsupervised machine learning, and MotionMapper ( Berman et al., 2014 ), a method that does not use pose estimation methods. These models embed data points based on feature dynamics (e.g., distance, speed) into a lower-dimensional space. Within this space it is possible to apply clustering algorithms for the segmentation of behavioral episodes. Generally, dense regions in this space (regions with many data points grouped together) are considered to be conserved behaviors. Other methods are aimed at explicitly capturing structure from the dynamics ( Batty et al., 2019 ; Bregler, 1997 ; Costa et al., 2019 ; Luxem et al., 2022a ; Shi et al., 2021 ; Sun et al., 2021c ). These models learn a continuous embedding that can be used to identify lower-dimensional trajectory dynamics that can be correlated to neuronal activity and segmented in relation to significant behavioral events.
Behavioral segmentation and other methods for quantification require a similar computing environment to that used for pose estimation. The input to those methods is generally the output of a pose estimation method (i.e., keypoint coordinates) or time series from a dimensionality reduction method such as principal component analysis that accounts for the keypoints or the raw video. It is crucial that pose estimation is accurate as the segmentation capabilities of the subsequent methods is bounded by pose tracking quality. Highly noisy key points will drown out biological signals and make the segmentation results hard to interpret, especially for unsupervised methods. Furthermore, identity switches between virtual markers can be catastrophic for multi-animal tracking and segmentation. A summary of methods for behavioral segmentation is provided in Table 3 .
Methods for behavioral segmentation using pose data.
SimBA ( ; ) is a supervised learning pipeline for importing pose estimation data and a graphical interface for interacting with a popular machine learning algorithm called Random Forest ( ). SimBA was developed for studies in social behavior and aggression and has been shown to be able to discriminate between attack, pursuit, and threat behaviors in studies using rats and mice. | |
MARS ( ; ) is another supervised learning pipeline developed for studies of social interaction behaviors in rodents, such as attacking, mounting, and sniffing, and uses the XGBoost gradient boosting classifier ( ). | |
B-SOiD ( ; ) uses unsupervised methods to learn and discover the spatiotemporal features in pose data of ongoing behaviors, such as grooming and other naturalistic movements in rodents, flies, or humans. B-SOiD uses UMAP embedding ( ) to account for dynamic features within video frames that are grouped using an algorithm for cluster analysis, HDBSCAN ( ). Clustered spatiotemporal features are then used to train a classifier (Random Forest; ) to detect behavioral classes in data sets that were not used to train the model and with millisecond precision. | |
VAME ( ; ) uses self-supervised deep learning models to infer the full range of behavioral dynamics based on the animal movements from pose data. The variational autoencoder framework ( ) is used to learn a generative model. An encoder network learns a representation from the original data space into a latent space. A decoder network learns to decode samples from this space back into the original data space. The encoder and decoder are parameterized with recurrent neural networks. Once trained, the learned latent space is parameterized by a Hidden Markov Model to obtain behavioral motifs. | |
TREBA ( ; ) relates measures from pose estimation to other quantitative or qualitative data associated with each frame in a video recording. Similar to VAME, a neural network is trained to learn to predict movement trajectories in an unsupervised manner. TREBA can then incorporate behavioral attributes, such as movement speed, distance traveled, and heuristic labels for behavior (e.g., sniffing, mounting, attacking) into representations of the pose estimation data learned by its neural networks, thereby bringing aspects of supervised learning. This is achieved using a technique called task programming. |
Before selecting any approach to segment animal behavior, it is important to first define the desired outcome. If the goal is to identify episodes of well-defined behaviors like rearing or walking, then the most straightforward approach is to use a supervised method. Moreover, it is generally a good starting point to use a supervised learning approach and the outputs of these models can be layered on top of unsupervised models to give them immediate interpretability. One tradeoff, however, is the extensive training datasets that are often required to ensure good supervised segmentation. Such methods can be established quite easily using standard machine learning libraries available for the Python, R, and MATLAB, if one has already experience in building these methods. Alternatively, open-source packages such as SimBA ( Nilsson et al., 2020a ) or MARS ( Segalin et al., 2021a ) can be used, and is especially beneficial for those who are relatively new to the topic of machine learning. However, if the researcher wants to understand more about the spatiotemporal structure of the behaving animal, they either need to label many different behaviors within the video or turn to unsupervised methods. Unsupervised methods offer the advantage to identify clusters in the video or keypoint time series and quantify behavior in each frame. Recently, A-SOiD, an active-learning algorithm, iteratively combines these supervised and unsupervised approaches to reduce the amount of training data required and enable the discovery of additional behavior and structure ( Schweihoff et al., 2022 ).
Interpreting the lower-dimensional structures in a 2D/3D projection plot can be difficult and it is advised to visualize examples from this projection space. Generative methods like VAME offer the possibility to sample cluster categories from this embedding space to qualitatively check if similar patterns are learned. Another task unsupervised methods are capable of is fingerprinting. Here, the embedding space is used as a signature to discern general changes in phenotypes ( Wiltschko et al., 2020 ). An alternative to using an explicitly supervised or unsupervised approach is to combine these approaches (semi-supervised), as implemented in a package called TREBA ( Sun et al., 2021c ). TREBA uses generative modeling in addition to incorporating behavioral attributes, such as movement speed, distance traveled, or heuristic labels for behavior (e.g., sniffing, mounting, attacking) into learned behavioral representations. It has been used in a number of different experimental contexts, most notably for understanding social interactions between animals.
Finally, as behavior is highly hierarchically structured, multiple spatio-temporal scales of description may be desired, for example, to account for bouts of locomotion and transitions running to escaping behavior ( Berman, 2018 ). It is possible to create a network representation and identify ‘cliques’ or ‘communities’ on the resulting graph ( Luxem et al., 2022a ; Markowitz et al., 2018 ). These descriptions represent human identifiable behavioral categories within highly interconnected sub-second segments of behavior. These representations can provide insights into the connection between different behavioral states and the transitions between states and their biological meaning.
Having described how to set up and use video recording methods and analysis methods for pose estimation, we would like to close by discussing some best practices in the use and development of methods for video analysis, including recommendations for the open sharing of video data and analysis code.
Best practices for experimenters
For those using video analysis methods in a laboratory setting, there are several key issues that should be followed as best practices. It is most crucial to develop a means of storing files in a manner in which they can be accessed in the lab, through cloud computing resources, and in data archives. These issues are discussed above in the ‘Hardware and software for data analysis’ section of this paper. Documentation of hardware is also a key best practice. All methods sections of manuscripts that use methods for video analysis should include details on the camera and lens that were used, the locations of and distances from the cameras relative to the behavioral arena, the acquisition rate and image resolution, environmental lighting (e.g., IR grids placed above the behavioral arena), properties of the arena (size, material, color, etc.).
Beyond within-lab data management and reporting details on hardware used in research manuscripts, more widespread sharing of video data is very much needed and is a core aspect of best practices for experimenters. In accordance with the demands of funders such as the NIH for data sharing, the open sharing of raw and processed videos and pose tracking data is crucial for research reproducibility and also for training new users on video methods. Several groups have created repositories to address this need ( Computational Behavior , OpenBehavior ). With widespread use, these repositories will help new users learn the required methods for data analysis, enable new analyses of existing datasets that could lead to new findings without having to do new experiments, and would enable comparisons of existing and newly developed methods for pose estimation and behavioral quantification. The latter benefit of data sharing could lead to insight into a major open question about methods for animal pose estimation: how choices about the parameters of any method for pose estimation or subsequent analysis impact analysis time, accuracy, and generalizability. Without these resources, it has not been possible to make confident statements about how existing methods compare across a wide range of datasets involving multiple types of research animals and in different experimental contexts. Guidance for how to implement data sharing can be found in several existing efforts of the machine learning community ( Gebru et al., 2021 ; Hutchinson et al., 2021 ; Stoyanovich and Howe, 2019 ). A more widespread use of these frameworks for sharing data can improve the transparency and accessibility of research data for video analysis.
Best practices for developers
We recommend three topics receive more attention by developers of methods for video analysis. First, there is a need for a common file format for storing results from pose estimation. Second, there is a need for methods to compare pose estimation packages and assess the impact of the parameters of each package on performance in terms of accuracy and user time. Third, there is a need for better code documentation and analysis reproducibility. Each of these issues is discussed below. In addition to these topics, we would like to encourage developers to design interfaces to make their tools more accessible to novice users. This will allow the tools to become more widely used and studied, and will further not limit use of the tools to researchers with advanced technical skills such as programming.
First, it is important to point out that there is no common and efficient data format available for tools that enable pose estimation in animal research. Such a format would allow users to compare methods without having to recode their video data. The FAIR data principles ( Wilkinson et al., 2016 ) are particularly apt for developing a common data format for video due to the large heterogeneity of data sources, intermediate analysis outputs, and end goals of the study. These principles call for data to be Findable (available in searchable repositories and with persistent and citable identifiers [DOIs]), Accessible (easily retrieved using the Internet), Interoperable (having a common set of terms to describe video data across datasets), and Reusable (containing information about the experimental conditions and outputs of any analysis or model to allow another group to readily make use of the data). A common file format for saving raw and processed video recordings and data from pose estimation models is needed to address these issues.
Second, there has also been a general lack of direct comparisons of different methods and parameter exploration within a given method on a standard set of videos. The choice of deep learning method and specific hyperparameters can affect the structural biases embedded in video data, thereby affecting the effectiveness of a given method ( Sculley et al., 2015 ). Yet, it seems that many users stick to default parameters available in popular packages. For example, in pose estimation, certain properties of neural network architectures such as its maximum receptive field size can dramatically impact the performance across species owing to the variability in morphological features ( Pereira et al., 2022 ). In addition to the intrinsic properties of particular species (e.g., Hayden et al., 2022 ), the analysis type will also dictate the importance of particular parameters on the task performance. For example, algorithms that achieve temporal smoothness in pose tracking are crucial for studies of fine motor control ( Wu et al., 2020 ), but perhaps not as essential as preventing identity swaps for studies of social behavior ( Pereira et al., 2022 ; Segalin et al., 2021a ). Another important issue is that most methods do not report well-calibrated measures of the confidence of model fits or predictions. This is important as it has become clear that machine learning tools tend to be overconfident in their predictions ( Abdar et al., 2021 ). Establishing standardized, interoperable data formats and datasets that include estimates of the fitted models and their predictions will enable comprehensive comparisons of existing and new methods for pose estimation and behavioral quantification.
For evaluating specific methods on lab-specific data, appropriate metrics and baseline methods for the research questions should be chosen. There may be cases where comparable baseline methods may not exist. For example, if a lab develops a new method for quantifying behavior for a specific organism or task on a lab-specific dataset, and there are no existing studies for that task. However, if related methods exist, it would be beneficial to compare performance of the new method against existing methods to study the advantages and disadvantages of the method. For more general claims (e.g., state-of-the-art pose estimator across organisms), evaluations on existing datasets and comparisons with baselines is important (see Table 4 ), to demonstrate the generality of the method and improvements over existing methods. A consensus on a standard set of data in the community for evaluation and an expansion to include more widely used behavioral tasks and assays would facilitate general model development and comparison. We show existing datasets in the community for method development in Table 4 and encourage the community to continue to open-source data and expand this list of available datasets to accelerate model development.
Datasets for model development.
Dataset | Task | Setting | Organism |
---|---|---|---|
2D/3D Pose Estimation | Videos from 4 camera views with poses from motion capture | Human (single-agent) | |
2D Pose Estimation | Images from uncontrolled settings with annotated poses | Human (multi-agent) | |
2D Pose Estimation & Tracking | Videos from crowded scenes with annotated poses | Human (multi-agent) | |
2D Pose Estimation | Images of diverse animal species with annotated poses | Diverse species (single & multi-agent) | |
2D Pose Estimation | Videos from 2 camera views with annotated poses | Mouse (multi-agent) | |
2D/3D Pose Estimation & Tracking | Videos from 2 camera views with annotated poses | Zebrafish (multi-agent) | |
2D/3D Pose Estimation | Images with annotated poses from a 62 camera setup | Monkey (single-agent) | |
2D/3D Pose Estimation & Tracking | Videos from 12 camera views with poses from motion capture | Rat (multi-agent) | |
2D/3D Pose Estimation & Tracking | Videos from moving phone camera in challenging outdoor settings | Human (multi-agent) | |
2D/3D Pose Estimation | Videos from 14 camera views with poses from motion capture | Human (single-agent) | |
2D/3D Pose Estimation | Videos from 12 camera views with poses from motion capture | Rat (single-agent) | |
Video-level Action Classification | Videos from uncontrolled settings that cover 700 human actions | Human (single & agent, may interact with other organisms/objects) | |
Video-level Action Classification (also has 3D poses) | Videos from 80 views and depth with 60 human actions | Human (single & multi-agent) | |
Frame-level Action Classification | Videos from uncontrolled settings with 65 action classes | Human (single & multi-agent) | |
Frame-level Behavior Classification | Videos from 2 views, with 13 annotated social behaviors | Mouse (multi-agent) | |
Frame-level Behavior Classification (also has 2D poses) | Videos & trajectory, with 10 annotated social behaviors | Fly (multi-agent) | |
Frame-level Behavior Classification (also has 2D poses) | Videos & trajectory, with 10 annotated social behaviors | Mouse (multi-agent) | |
Frame-level Behavior Classification (also has 2D poses) | Top-down views, 7 annotated keypoints, hundreds of videos | Mouse (multi-agent) |
Third, reproducibility of results is crucial for acceptance of new methods for video analysis within the research community and for research transparency. Guidance for documenting the details of models and algorithms can be obtained from the Machine Learning Reproducibility Checklist . It is applicable to any computational model in general. Importantly, the checklist calls for including the range of hyperparameters considered for experiments, mean and variance of results from multiple runs, and an explanation of how samples were allocated for train/validation/test. Further guidance for sharing code is available in this GitHub resource: Publishing Research Code . It provides tips on open-sourcing research code, including specifications of code dependencies, training and evaluation code, and including pre-trained models as part of any code repository. Beyond these resources, we note that there is also a broader definition of reproducibility in that experiments should be robustly reproducible : experimental results should ideally not vary significantly under minor perturbations. For example, even if there are minor variations to lighting or arena size from the original experiments, the video analysis results should not change significantly. A framework to ensure robust reproducibility is currently an open question, but the existing frameworks should facilitate producing the same results under the same experimental conditions. Model interpretability is another important consideration depending on the purpose of the video analysis experiment. Many machine learning models are ‘black box’ models, and not easily interpretable; as such, post hoc explanations may not always be reliable ( Rudin, 2019 ). One way to generate human-interpretable models is through program synthesis ( Balog et al., 2017 ) and neurosymbolic learning ( Sun et al., 2022 ; Zhan et al., 2021 ). These methods learn compositions of symbolic primitives, which are closer in form to human-constructed models than neural networks. Interpretable models can facilitate reproducibility and trustworthiness in model predictions for scientific applications. Efforts at deploying these approaches for methods for video analysis and behavioral quantification are very much needed.
We hope that our review of the current state of open-source tools for behavioral video analysis will be helpful to the community. We described how to set up video methods in a lab, provided an overview on currently available methods, and provided guidance for best practices in using and developing the methods. As newer tools emerge and more research groups become proficient at using available methods, there is a clear potential for the tools to help with advancing our understanding of the neural basis of behavior.
- Pourpanah F
- Rezazadegan D
- Ghavamzadeh M
- Makarenkov V
- Nahavandi S
- Google Scholar
- Anderson DJ
- Eisenreich BR
- Zimmermann J
- Brockschmidt M
- Markowitz J
- Churchland A
- Cunningham JP
- Linderman S
- Ben-Shaul Y
- Shaevitz JW
- Eva Zhang Y
- Padilla-Coreano N
- Stephens GJ
- Mountcastle AM
- Davidson TL
- Marshall JD
- Severson KS
- Aldarondo DE
- Hildebrand DGC
- Freiwald WA
- Ölveczky BP
- Zisserman A
- Feierstein CE
- Sosulski DL
- Morgenstern J
- Vecchione B
- Gosztolai A
- Lobato-Ríos V
- Pietro Abrate M
- Costelloe BR
- Campagnolo J
- Hutchinson B
- Kjartansson O
- Rivera-Alba M
- Saunders JL
- Karashchuk P
- Dickinson ES
- Walling-Bell S
- Keshavarzi S
- Campagner D
- Krakauer JW
- Ghazanfar AA
- Gomez-Marin A
- Paleressompoulle D
- Soberanes D
- Calcaterra L
- Markowitz JE
- Robertson K
- Peterson RE
- Linderman SW
- Sabatini BL
- Nishihara HK
- Mamidanna P
- Juavinett AL
- Churchland AK
- Norville ZC
- Bentzley BS
- McLaughlin RJ
- Roian Egnor SE
- D’Alessandro I
- Ottenheimer DJ
- Panadeiro V
- Rodriguez A
- Wlodkowic D
- Andersson M
- Pennington ZT
- Page-Harley L
- Pereira T.D
- Ravindranath S
- Papadoyannis ES
- McKenzie-Smith GC
- Russakovsky O
- Bernstein M
- Krynitsky J
- Garmendia-Cedillos M
- Abuhatzira L
- Gottesman MM
- Mitchell JB
- Schonberger JL
- Schönberger JL
- Pollefeys M
- Schorscher-Petcu A
- Schweihoff JF
- Chaudhary V
- Zelikowsky M
- Stoyanovich J
- Pachitariu M
- Steinmetz N
- Carandini M
- von Ziegler L
- Privitera M
- Slominski D
- Goldshmid RH
- Weissbourd B
- Tjandrasuwita M
- Solar-Lezama A
- Chaudhuri S
- Costilla-Reyes O
- van Duuren E
- van der Plasse G
- Feenstra MGP
- Pennartz CMA
- Amarante LM
- Wilkinson MD
- Dumontier M
- Aalbersberg IJJ
- da Silva Santos LB
- Gonzalez-Beltran A
- ’t Hoen PAC
- Rocca-Serra P
- van Schaik R
- van der Lei J
- van Mulligen E
- Waagmeester A
- Wittenburg P
- Wolstencroft K
- Wiltschko AB
- Tsukahara T
- Buchanan EK
- Whiteway MR
- Schartner M
- Rodriguez E
- Laboratory TIB
- Cunningham J
Author details
Contribution, contributed equally with, competing interests.

For correspondence
National science foundation (1948181), national institutes of health (da046375), natural sciences and engineering research council of canada (pgsd3-532647-2019), national institutes of health (mh002952), national institutes of health (mh124042), national institutes of health (mh128177), national science foundation (2024581).
The funders had no role in study design, data collection and interpretation, or the decision to submit the work for publication.
Acknowledgements
This paper emerged from a working group on methods for video analysis organized by the OpenBehavior project in the summer and fall of 2021. Ann Kennedy, Greg Corder, and Sam Golden were major contributors to the working group and their ideas impacted this manuscript. We would like to thank Ann Kennedy, Samantha White, and Jensen Palmer for helpful comments on the manuscript. NSERC Award #PGSD3-532647-2019 to JJS; NIH MH002952 for SPB; NIH MH124042 for KK; NIH MH128177 and NSF 2024581 to JZ; NSF 1948181 and NIH DA046375 to ML.
© 2023, Luxem, Sun et al.
This article is distributed under the terms of the Creative Commons Attribution License , which permits unrestricted use and redistribution provided that the original author and source are credited.
- 11,244 views
- 981 downloads
- 30 citations
Views, downloads and citations are aggregated across all versions of this paper published by eLife.
Download links
Downloads (link to download the article as pdf).
- Article PDF
Open citations (links to open the citations from this article in various online reference manager services)
Cite this article (links to download the citations from this article in formats compatible with various reference manager tools), categories and tags.
- pose estimation
- open source
- reproducibility
Further reading
Serial dependence: connecting past and present.
A neural signature of serial dependence has been found, which mirrors the attractive bias of visual information seen in behavioral experiments.
- Developmental Biology
Semaphorin7A patterns neural circuitry in the lateral line of the zebrafish
In a developing nervous system, axonal arbors often undergo complex rearrangements before neural circuits attain their final innervation topology. In the lateral line sensory system of the zebrafish, developing sensory axons reorganize their terminal arborization patterns to establish precise neural microcircuits around the mechanosensory hair cells. However, a quantitative understanding of the changes in the sensory arbor morphology and the regulators behind the microcircuit assembly remain enigmatic. Here, we report that Semaphorin7A (Sema7A) acts as an important mediator of these processes. Utilizing a semi-automated three-dimensional neurite tracing methodology and computational techniques, we have identified and quantitatively analyzed distinct topological features that shape the network in wild-type and Sema7A loss-of-function mutants. In contrast to those of wild-type animals, the sensory axons in Sema7A mutants display aberrant arborizations with disorganized network topology and diminished contacts to hair cells. Moreover, ectopic expression of a secreted form of Sema7A by non-hair cells induces chemotropic guidance of sensory axons. Our findings propose that Sema7A likely functions both as a juxtracrine and as a secreted cue to pattern neural circuitry during sensory organ development.
Periaqueductal gray activates antipredatory neural responses in the amygdala of foraging rats
Pavlovian fear conditioning research suggests that the interaction between the dorsal periaqueductal gray (dPAG) and basolateral amygdala (BLA) acts as a prediction error mechanism in the formation of associative fear memories. However, their roles in responding to naturalistic predatory threats, characterized by less explicit cues and the absence of reiterative trial-and-error learning events, remain unexplored. In this study, we conducted single-unit recordings in rats during an ‘approach food-avoid predator’ task, focusing on the responsiveness of dPAG and BLA neurons to a rapidly approaching robot predator. Optogenetic stimulation of the dPAG triggered fleeing behaviors and increased BLA activity in naive rats. Notably, BLA neurons activated by dPAG stimulation displayed immediate responses to the robot, demonstrating heightened synchronous activity compared to BLA neurons that did not respond to dPAG stimulation. Additionally, the use of anterograde and retrograde tracer injections into the dPAG and BLA, respectively, coupled with c-Fos activation in response to predatory threats, indicates that the midline thalamus may play an intermediary role in innate antipredatory-defensive functioning.
Be the first to read new articles from eLife
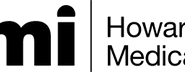
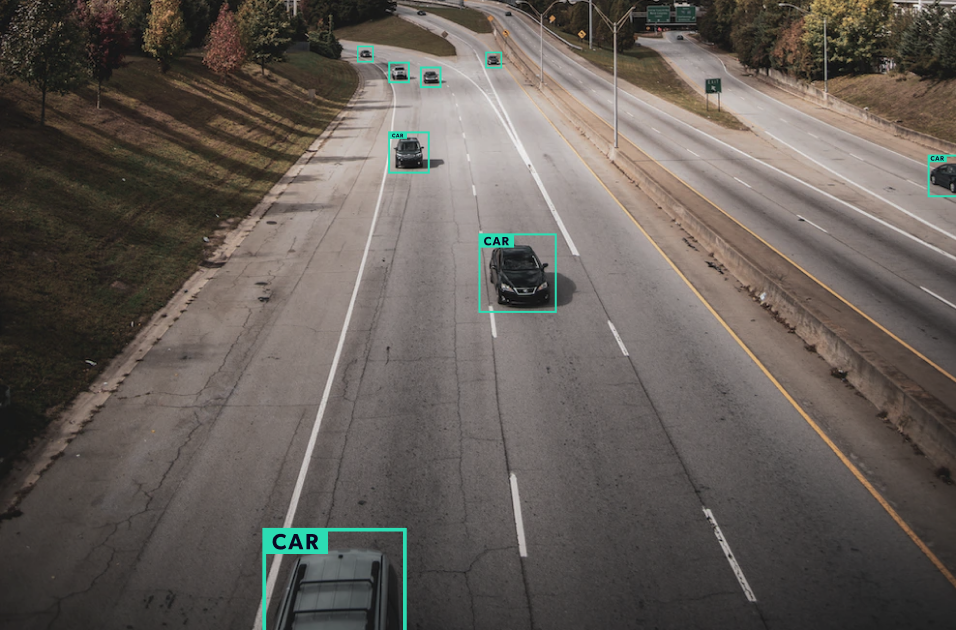
Introduction
In the past few years, video analytics, also known as video content analysis or intelligent video analytics , has attracted increasing interest from both industry and the academic world. Thanks to the popularization of deep learning , video analytics has introduced the automation of tasks that were once the exclusive purview of humans.
Recent improvements in video analytics have been a game-changer , ranging from applications that monitor traffic jams and alert in real-time, to others that analyze customers’ flow in retail to maximize sales, along with other more well-known scenarios such as facial recognition or smart parking.
This kind of technology looks great, but how does it work and how can it benefit your business ?
In this guide, you'll discover the basic concept of video analytics , how it's used in the real world to automate processes and gain valuable insights, and what you should consider when implementing intelligent video analytics solutions in your organization.
What is intelligent video analytics?
The main goal of video analytics is to automatically recognize temporal and spatial events in videos . A person who moves suspiciously, traffic signs that are not obeyed, the sudden appearance of flames and smoke; these are just a few examples of what a video analytics solution can detect.
Real-time video analytics and video mining
Usually, these systems perform real-time monitoring in which objects, object attributes, movement patterns, or behavior related to the monitored environment are detected. However, video analytics can also be used to analyze historical data to mine insights . This forensic analysis task can detect trends and patterns that answer business questions such as:
- When is customer presence at its peak in my store and what is their age distribution?
- How many times is a red light run, and what are the specific license plates of the vehicles doing it?
Some known applications
Some applications in the field of video analytics are widely known to the general public. One such example is video surveillance , a task that has existed for approximately 50 years. In principle, the idea is simple: install cameras strategically to allow human operators to control what happens in a room, area, or public space.
In practice, however, it is a task that is far from simple. An operator is usually responsible for more than one camera and, as several studies have shown, upping the number of cameras to be monitored adversely affects the operator’s performance. In other words, even if a large amount of hardware is available and generating signals, a bottleneck is formed when it is time to process those signals due to human limitations .
Video analysis software can contribute in a major way by providing a means of accurately dealing with volumes of information.
Video analytics with deep learning
Machine learning and, in particular, the spectacular development of deep learning approaches, has revolutionized video analytics .
The use of Deep Neural Networks (DNNs) has made it possible to train video analysis systems that mimic human behavior, resulting in a paradigm shift. It started with systems based on classic computer vision techniques (e.g. triggering an alert if the camera image gets too dark or changes drastically) and moved to systems capable of identifying specific objects in an image and tracking their path.
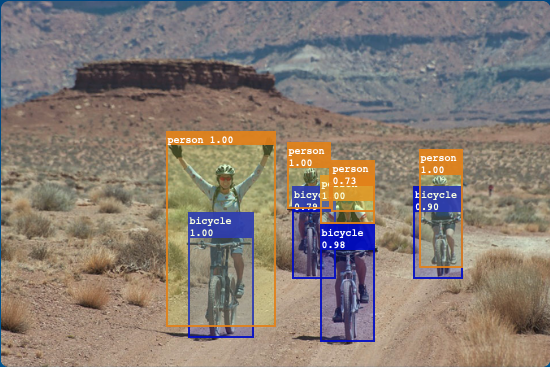
For example, Optical Character Recognition (OCR) has been used for decades to extract text from images. In principle, it could suffice to apply OCR algorithms directly to an image of a license plate to discern its number. In the previous paradigm, this might work if the camera was positioned in such a way that, at the time of executing the OCR, we were certain that we were filming a license plate.
A real-world application of this would be the recognition of license plates at parking facilities, where the camera is located near the gates and could film the license plate when the car stops. However, running OCR constantly on images from a traffic camera is not reliable: if the OCR returns a result, how can we be sure that it really corresponds to a license plate?
In the new paradigm, models based on deep learning are able to identify the exact area of an image in which license plates appear. With this information, OCR is applied only to the exact region in question, leading to reliable results .
Let's talk about building new solutions
Industry applications.
Historically, healthcare institutions have invested large amounts of money in video surveillance solutions to ensure the safety of their patients, staff, and visitors, at levels that are often regulated by strict legislation. Theft, infant abduction, and drug diversion are some of the most common problems addressed by surveillance systems.
In addition to facilitating surveillance tasks, video analytics allows us to go further, by exploiting the data collected in order to achieve business goals . For example, a video analytics solution could detect when a patient has not been checked on according to their needs and alert the staff. Analysis of patient and visitor traffic can be extremely valuable in determining ways to shorten wait times , while ensuring clear access to the emergency area .
At-home monitoring of older adults or people with health issues is another example of an application that provides great value. For instance, falls are a major cause of injury and death in older people. Although personal medical devices can detect falls, they must be worn and are frequently disregarded by the consumer. A video analytics solution can process the signals of home cameras to detect in real time if a person has fallen. With proper setup, such a system could also determine if a person took a given medication when they were supposed to, for instance.
Mental healthcare is another area in which video analytics can make significant contributions. Systems that analyze facial expressions, body posture, and gaze can be developed to assist clinicians in the evaluation of patients. Such a system is able to detect emotions from body language and micro-expressions, offering clinicians objective information that can confirm their hypotheses or give them new clues.
Real-world example
The University at Buffalo developed a smartphone application designed to help detect autism spectrum disorder (ASD) in children. Using only the smartphone camera, the app tracks facial expression and gaze attention of a child looking at pictures of social scenes (showing multiple people). The app monitors the eye movements and can accurately detect children with ASD since their eye movements are different from those of a person without autism.
Smart cities / Transportation
Video analytics has proven to be a tremendous help in the area of transport, aiding in the development of smart cities.
An increase in traffic, especially in urban areas, can result in an increase in accidents and traffic jams if adequate traffic management measures are not taken. Intelligent video analysis solutions can play a key role in this scenario.
Traffic analysis can be used to dynamically adjust traffic light control systems and to monitor traffic jams . It can also be useful in detecting dangerous situations in real time, such as a vehicle stopped in an unauthorized space on the highway, someone driving in the wrong direction, a vehicle moving erratically, or vehicles that have been in an accident. In the case of an accident, these systems are helpful in collecting evidence in case of litigation .
At Tryolabs we developed a video analytics platform to detect pedestrian’s misbehaviors on street videos and to be able to process high volumes of data. This project gave relevant statistics to the client to be able to take actions in areas where misbehaviors were abundant generating traffic issues.
Vehicle counting , or differentiating between cars, trucks, buses, taxis, and so on, generates high-value statistics used to obtain insights about traffic. Installing speed cameras allows for precise control of drivers en masse. Automatic license plate recognition identifies cars that commit an infraction or, thanks to real-time searching, spots a vehicle that has been stolen or used in a crime.
Instead of using sensors in each parking space, a smart parking system based on video analytics helps drivers find a vacant spot by analyzing images from security cameras.
These are just some examples of the contributions that video analysis technology can make to build safer cities that are more pleasant to live in.
A great example of video analytics used to solve real-world problems is the one of the city of New York. In order to better understand major traffic events, the New York City Department of Transportation used video analytics and machine learning to detect traffic jams, weather patterns, parking violations and more. The cameras capture the activities, process them and send real-time alerts to city officials.
The use of machine learning, and video analytics in particular, in the retail sector has been one of the most important technological trends in recent years.
Brick and mortar retailers can use video analytics to understand who their customers are and how they behave.
State-of-the-art algorithms are able to recognize faces and determine people’s key characteristics such as gender and age. These algorithms can also track customers' journeys through stores and analyze navigational routes to detect walking patterns . Adding in the detection of direction of gaze , retailers can identify how long a customer looks at a certain product and finally answer a crucial question: where is the best place to put items in order to maximize sales and improve customer experience?
A lot of actionable information can be gathered with a video analytics solution, such as: number of customers, customer's characteristics, duration of visit, and walking patterns. All of this data can be analyzed while taking into account its temporal nature, in order to optimize the organization of the store according to the day of the week, the seasons of the year, or holidays . In this way, a retailer can get an extremely accurate sense of who their customers are, when they visit their store, and how they behave once inside.
A typical solution of counting entrances and exits of customers in a store can give useful information to calculate high impact metrics such as conversion rates. This approach can be leveraged by previously installed security cameras making it fast and cost effective to deploy.
Video analytics is also great for developing anti-theft mechanisms . For instance, face recognition algorithms can be trained to spot known shoplifters or spot in real-time a person hiding an item in their backpack.
What is more, information extracted from video analytics can serve as input data for training machine learning models , which aim to solve larger challenges. As an example, walking patterns and the number of people in the store, can be useful information to add to machine learning powered solutions for demand forecasting, price optimization and inventory forecasting.
Amazon Go is how Amazon entered the grocery industry. It attempts to simplify the customers' shopping experience by avoiding checkouts and letting the customers just walk out of the grocery store, automatically charging them according to what they grabbed. It has been around for several years now, and it is still a disruptive solution. Amazon Go leverages an accurate video analysis software based on several cameras to track the customers' behavior in the store . This software, combined with several sensors placed around the store, lets Amazon Go make confident decisions when it comes to charging users for their purchases.
Video surveillance is an old task of the security domain. However, from the time that systems were monitored exclusively by humans to current solutions based on video analytics, much water has passed under the bridge.
Facial and license plate recognition (LPR) techniques can be used to identify people and vehicles in real-time and make appropriate decisions. For instance, it’s possible to search for a suspect both in real-time and in stored video footage, or to recognize authorized personnel and grant access to a secured facility.
Crowd management is another key function of security systems. Cutting edge video analysis tools can make a big difference in places such as shopping malls, hospitals, stadiums, and airports. These tools can provide an estimated crowd count in real time and trigger alerts when a threshold is reached or surpassed. They can also analyze crowd flow to detect movement in unwanted or prohibited directions .
In the video above, a surveillance system was trained to recognize people in real-time. This lays the groundwork for obtaining other results. The most immediate: a count of the number of people passing by daily. More advanced goals, based on historical data, might be to determine the "normal" flow of people according to the day of the week and time and generate alerts in case of unusual traffic. If the monitored area is pedestrian-only, the system could be trained to detect unauthorized objects such as motorcycles or cars and, again, trigger some kind of alert.
This is one of the great advantages of these approaches: video content analysis systems can be trained to detect specific events , sometimes with a high degree of sophistication. One such example is to detect fires as soon as possible. Or, in the case of airports, to raise an alert when someone enters a forbidden area or walks against the direction intended for passengers . Another great use case is the real-time detection of unattended baggage in a public space.
As for classic tasks such as intruder detection, they can be performed robustly, thanks to algorithms that can filter out motion caused by wind, rain, snow, or animals.
The functionality offered by intelligent video analysis grows day by day in the security domain, and this is a trend that will continue in the future.
The Danish football club Brondby was the first soccer club to officially introduce facial recognition technology in 2019 to improve safety on matchdays at its stadium. The system identifies banned people from attending games and enables staff to prevent them from entering the stadium.
It has been a long since data arrived in sports. From soccer coaches to personal trainers, from professional athletes to beginners, everyone is leveraging data to achieve better results.
Soccer matches statistics, such as ball possession or counting the number of passes , have become a default tool for coaches to understand their team's performance. Studies have been made analyzing the importance of ball possession in UEFA Champions League matches, concluding that teams with more ball possession won 49.2%, drew 22.0%, and lost 28.7% of the matches overall, exceeding the winning rates of their rivals. If you are interested in this topic, at Tryolabs we have a tutorial on how to automatically measure soccer ball possession with AI and video analytics .
Understanding an athlete's pose when practicing sports is essential for improving the technique. Video analytics solutions can give this information to athletes or coaches to make it easier to achieve their goals. Also, pose information can be used to prevent injuries by understanding if there are any risky moves.
Video analytics solutions can also be leveraged to understand how the opponents play. Learning their game can help to build effective counters to their strategies. Solutions may range from automatically selecting relevant plays in a match to giving useful statistics to understand the opponent's weaknesses.
In the UK, soccer teams are competing with each other not only in the Premier League but also in the race to have the best possible data . From hiring rocket scientists to chess champions and even using missile tech, more than scouts, teams have started looking for engineers, mathematicians, physicists, and experts in statistics or algorithms.
Some teams, such as Arsenal, have their own in-house data company, while many others rely on third-party companies to give them all the necessary data. This data is used for every single decision: to hire players and coaches, to know what are the best positions in the field for every player, and to track youngsters’ performance in their loans, to name a few.
How does video analytics work?
Let's take a look at a general scheme of how a video analytics solution works. Depending on the particular use case, the architecture of a solution may vary, but the scheme remains the same.
Video content analysis can be done in two different ways: in real time , by configuring the system to trigger alerts for specific events and incidents that unfold in the moment, or in post processing , by performing advanced searches to facilitate forensic analysis tasks.
Feeding the system
The data being analyzed can come from various streaming video sources. The most common are CCTV cameras , traffic cameras and online video feeds . However, any video source that uses the appropriate protocol (e.g. RTSP: real-time streaming protocol or HTTP) can generally be integrated into the solution.
A key goal is coverage : we need to have a clear view of the entire area, and from various angles, where the events being monitored might occur. Remember, more data is better, given that it can be processed.
Central processing vs edge processing
Video analysis software can be run centrally on servers that are generally located in the monitoring station, which is known as central processing . Or, it can be embedded in the cameras themselves, a strategy known as edge processing .
The choice of cameras should be carefully considered when designing a solution. A lot of legacy software was developed with central processing capabilities only. In recent years, though, it is not uncommon to come across hybrid solutions. In fact, a good practice is to concentrate, whenever possible, real-time processing on cameras and forensic analysis functionalities on the central server.
With a hybrid approach , the processing performed by the cameras reduces the data being processed by the central servers, which otherwise could require extensive processing capabilities and bandwidth as the number of cameras increases. In addition, it is possible to configure the software to only send data about suspicious events to the server over the network, reducing network traffic and the need for storage.
Meanwhile, centralizing the data for forensic analysis allows for multiple search and analysis tools to be used, from general algorithms to ad-hoc implementations, all utilizing different sets of parameters that help to balance the noise and silence in the results obtained. Essentially, you can enter in your own algorithms to get the desired results, which is a particularly flexible and attractive scheme.
Defining scenarios and training models
Once the physical architecture is planned for and installed, it is necessary to define the scenarios on which you want to focus and then train the models that are going to detect the target events.
Vehicle crashes? Crowd flow? Facial recognition at a retail store to recognize known shoplifters? Each scenario leads to a series of basic tasks that the system must know how to perform.
An example: detect vehicles, eventually recognize their type (e.g. motorcycle, car, truck), track their trajectory frame by frame, and then study the evolution of those paths to detect a possible crash.
The most frequent, basic tasks in video analytics are:
- Image classification: select the category of an image from among a set of predetermined categories (e.g. car, person, horse, scissors, statue).
- Localization : locate an object in an image (generally involves drawing a bounding box around the object).
- Object detection : locate and categorize an object in an image.
- Object identification : given a target object, identify all of its instances in an image (e.g. find all soccer players in the image).
- Object tracking : track an object that moves over time in a video.
To know more about the basic tasks performed and the types of algorithms that are used to develop video analysis software, we recommend you read this introductory guide to computer vision . More specifically, if you want to dive deeper into object detection and tracking tasks, you can refer to our step-by-step tutorial. .
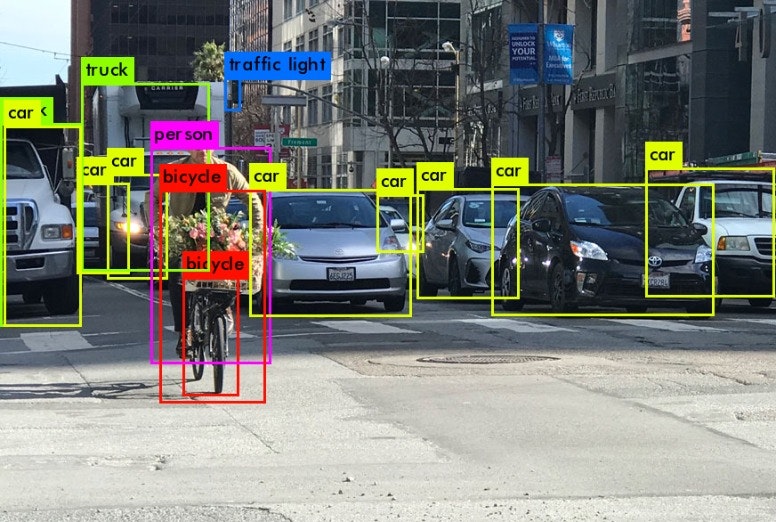
Training models from scratch requires considerable effort. Luckily, there are a fair amount of resources available that make this a less burdensome task.
Image datasets such as ImageNet or Microsoft Common Objects in Context (COCO) are key resources that simplify the training of new models.
There are several pre-trained models available for tasks such as image classification, object detection, and facial recognition, which, thanks to transfer learning techniques , allow for the adaptation (fine tuning) of a model for a given use case. This is much less expensive than a complete training.
Finally, open source projects have been increasingly published in recent years by the community to facilitate the building of custom video analysis systems. Relying on computer vision libraries, such as the ones presented in the following section, greatly helps build solutions faster and with more accuracy.
Human review
In virtually all cases, a human is needed to monitor the alerts generated by a video analysis system and decide what should be done, if anything. In this sense, these systems act as valuable support for operators, helping them to detect events that might otherwise be overlooked or take a long time to detect manually.
Open source projects
There's no well-established library for video analytics at the moment. The ones that exist are usually some implementation of a research paper, so they tend to be hard to use in a practical context. In other cases, the libraries are meant to be easy to use but perform poorly.
The best option is to hunt for object-tracking or pose-tracking libraries and create something custom .
At Tryolabs, we use image-level algorithms like object detection and pose estimation to perform video analytics, then add our own tracking algorithm layer over them and proceed from there.
The Open Source Computer Vision Library (OpenCV) is the most well-known computer vision library. It contains a comprehensive set of machine learning algorithms to perform common tasks such as image classification, face recognition, and object detection and tracking. It is widely used by companies and research groups, as it can be used via its native C++ interface, or though Java and Python wrappers.
Since it is a general computer vision library, it is possible to implement a video analysis system with OpenCV. However, as it is not a specialized video analytics library, it may be more interesting to turn to other available libraries (depending on the use case). In general, OpenCV is a great tool for approaching classical computer vision tasks and also for pre processing and post processing tasks.
As mentioned before, at Tryolabs we use object detection and pose estimation algorithms and add tracking on top them to create video analytics solutions. To achieve this we’ve built Norfair , a customizable lightweight Python library for real-time multi-object tracking. Using Norfair you can add tracking capabilities to any detector with just a few lines of code.
Norfair is highly customizable letting users define their own distance functions, it is modular since it can be easily inserted into complex video processing pipelines and it is fast as the only thing bounding inference speed is the detection network.
Norfair not only lets you track simple bounding boxes but is also compatible with keypoints and even 3D objects. You can also accurately track objects even if the camera is moving by estimating camera motion, potentially accounting for pan, tilt, rotation, movement in any direction, and zoom. Re-identification (ReID) is also supported, allowing the inclusion of appearance embeddings to achieve a more robust tracking system.
Back in 2016, Joseph Redmon et al. published the first single-stage object detector, You Only Look Once: Unified, Real-Time Object Detection , at the CVPR conference. YOLO was designed with both speed and accuracy in mind, which is why it is one of the most popular object detection models for production environments. YOLO is not only a model but a family of object detection models. Over the years, several modifications have been made to the original architecture to achieve even better results. YOLOv4, YOLOv5, YOLOv7, and YOLOX are some of the most popular variations, and this evolution is not to be stopped soon.
The authors of YOLOv7 (2022) open-sourced the implementation using PyTorch . This code allows for quickly developing video analytics solutions by making pre trained object detection models available for users. Another great advantage of YOLOv7's implementation is that it can be extended for pose estimation and instance segmentation tasks.
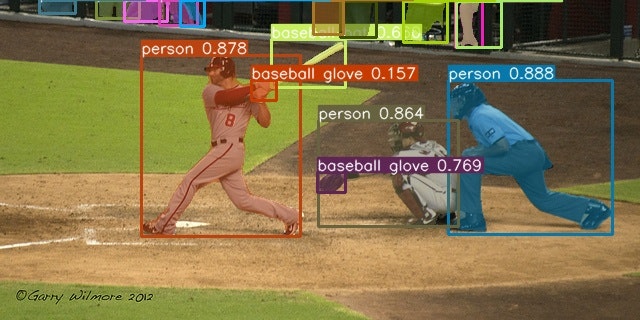
Video analytics solutions
There is a plethora of off-the-shelf solutions in video analytics, from classic security systems to more complex scenarios such as smart home or healthcare applications.
If your use case is satisfied by one of these standard solutions, they may be an option for you. Be aware that, in general, some kind of adaptation or parameterization of the software has to be done and these solutions only allow customization to a certain degree.
However, most companies aim to gain specific insights to reach individual goals with a video analytics solution, which requires more optimized software . In this case, the ideal solution is to turn to a company specializing in video analytics services, such as we do here at Tryolabs . A custom solution is likely to be more accurate and can address unusual or extremely particular use cases.
Video analytics solutions are invaluable in helping us in our daily tasks. There are a vast number of sectors that can benefit from this technology, especially as the complexity of potential applications has been growing in recent years.
From smart cities, to security controls in hospitals and airports, to people tracking for retail and shopping centers, the field of video analytics enables processes that are simultaneously more effective and less tedious for humans, and less expensive for companies.
We hope you enjoyed this post, and that you gained a better understanding of what video analytics is all about, how it works, and how you can leverage it in your organization in order to automate processes and gain valuable insights to make better decisions.
We have been developing Machine Learning solutions since 2010. Partnering up with companies in different industries let us better understand their challenges and how they can use data to drive business results. Please don’t hesitate to drop us a line if you have any questions or comments about it.
Thinking about new solutions?
Video Analytics
Demand Forecasting
Price Optimization
Product Matching
Predictive Maintenance
AI On The Edge
Transformers
Data Engineering
Collections
Open Source
Partnerships
Terms and Conditions
© 2024 . All rights reserved.
- Methodology
- Open access
- Published: 28 July 2018
Research as storytelling: the use of video for mixed methods research
- Erica B. Walker ORCID: orcid.org/0000-0001-9258-3036 1 &
- D. Matthew Boyer 2
Video Journal of Education and Pedagogy volume 3 , Article number: 8 ( 2018 ) Cite this article
18k Accesses
5 Citations
4 Altmetric
Metrics details
Mixed methods research commonly uses video as a tool for collecting data and capturing reflections from participants, but it is less common to use video as a means for disseminating results. However, video can be a powerful way to share research findings with a broad audience especially when combining the traditions of ethnography, documentary filmmaking, and storytelling.
Our literature review focused on aspects relating to video within mixed methods research that applied to the perspective presented within this paper: the history, affordances and constraints of using video in research, the application of video within mixed methods design, and the traditions of research as storytelling. We constructed a Mind Map of the current literature to reveal convergent and divergent themes and found that current research focuses on four main properties in regards to video: video as a tool for storytelling/research, properties of the camera/video itself, how video impacts the person/researcher, and methods by which the researcher/viewer consumes video. Through this process, we found that little has been written about how video could be used as a vehicle to present findings of a study.
From this contextual framework and through examples from our own research, we present current and potential roles of video storytelling in mixed methods research. With digital technologies, video can be used within the context of research not only as data and a tool for analysis, but also to present findings and results in an engaging way.
Conclusions
In conclusion, previous research has focused on using video as a tool for data collection and analysis, but there are emerging opportunities for video to play an increased role in mixed methods research as a tool for the presentation of findings. By leveraging storytelling techniques used in documentary film, while staying true to the analytical methods of the research design, researchers can use video to effectively communicate implications of their work to an audience beyond academics and use video storytelling to disseminate findings to the public.
Using motion pictures to support ethnographic research began in the late nineteenth century when both fields were early in their development (Henley, 2010 ; “Using Film in Ethnographic Field Research, - The University of Manchester,” n.d ). While technologies have changed dramatically since the 1890s, researchers are still employing visual media to support social science research. Photographic imagery and video footage can be integral aspects of data collection, analysis, and reporting research studies. As digital cameras have improved in quality, size, and affordability, digital video has become an increasingly useful tool for researchers to gather data, aid in analysis, and present results.
Storytelling, however, has been around much longer than either video or ethnographic research. Using narrative devices to convey a message visually was a staple in the theater of early civilizations and remains an effective tool for engaging an audience today. Within the medium of video, storytelling techniques are an essential part of a documentary filmmaker’s craft. Storytelling can also be a means for researchers to document and present their findings. In addition, multimedia outputs allow for interactions beyond traditional, static text (R. Goldman, 2007 ; Tobin & Hsueh, 2007 ). Digital video as a vehicle to share research findings builds on the affordances of film, ethnography, and storytelling to create new avenues for communicating research (Heath, Hindmarsh, & Luff, 2010 ).
In this study, we look at the current literature regarding the use of video in research and explore how digital video affordances can be applied in the collection and analysis of quantitative and qualitative human subject data. We also investigate how video storytelling can be used for presenting research results. This creates a frame for how data collection and analysis can be crafted to maximize the potential use of video data to create an audiovisual narrative as part of the final deliverables from a study. As researchers we ask the question: have we leveraged the use of video to communicate our work to its fullest potential? By understanding the role of video storytelling, we consider additional ways that video can be used to not only collect and analyze data, but also to present research findings to a broader audience through engaging video storytelling. The intent of this study is to develop a frame that improves our understanding of the theoretical foundations and practical applications of using video in data collection, analysis, and the presentation of research findings.

Literature review
The review of relevant literature includes important aspects for situating this exploration of video research methods: the history, affordances and constraints of using video in research, the use of video in mixed methods design, and the traditions of research as storytelling. Although this overview provides an extensive foundation for understanding video research methods, this is not intended to serve as a meta-analysis of all publications related to video and research methods. Examples of prior work provide a conceptual and operational context for the role of video in mixed methods research and present theoretical and practical insights for engaging in similar studies. Within this context, we examine ethical and logistical/procedural concerns that arise in the design and application of video research methods, as well as the affordances and constraints of integrating video. In the following sections, the frame provided by the literature is used to view practical examples of research using video.
The history of using video in research is founded first in photography and next in film followed more recently, by digital video. All three tools provide the ability to create instant artifacts of a moment or period of time. These artifacts become data that can be analyzed at a later date, perhaps in a different place and by a different audience, giving researchers the chance to intricately and repeatedly examine the archive of information contained within. These records “enable access to the fine details of conduct and interaction that are unavailable to more traditional social science methods” (Heath et al., 2010 , p. 2).
In social science research, video has been used for a range of purposes and accompanies research observation in many situations. For example, in classroom research, video is used to record a teacher in practice and then used as a guide and prompt to interview the teacher as they reflect upon their practice (e.g. Tobin & Hsueh, 2007 ). Video captures events from a situated perspective, providing a record that “resists, at least in the first instance, reduction to categories or codes, and thus preserves the original record for repeated scrutiny” (Heath et al., 2010 , p. 6). In analysis, these audio-visual recordings allow the social science researcher the chance to reflect on their subjectivities throughout analysis and use the video as a microscope that “allow(s) actions to be observed in a detail not even accessible to the actors themselves” (Knoblauch & Tuma, 2011 , p. 417).
Examining the affordances and constraints of video in research provides a researcher the opportunity to examine the value of including video within a study . An affordance of video, when used in research, is that it allows the researcher to see an event through the camera lens either actively or passively and later share what they have seen, or more specifically, the way they saw it (Chalfen, 2011 ). Cameras can be used to capture an event in three different modes: Responsive, Interactive, and Constructive. Responsive mode is reactive. In this mode, the researcher captures and shows the viewer what is going on in front of the lens but does not directly interfere with the participants or events. Interactive mode puts the filmmaker into the storyline as a participant and allows the viewer to observe the interactions between the researcher and participant. One example of video captured in Interactive mode is an interview. In Constructive mode, the researcher reprocesses the recorded events to create an explicitly interpretive final product through the process of editing the video (MacDougall, 2011 ). All of these modes, in some way, frame or constrain what is captured and consequently shared with the audience.
Due to the complexity of the classroom-research setting, everything that happens during a study cannot be captured using video, observation, or any other medium. Video footage, like observation, is necessarily selective and has been stripped of the full context of the events, but it does provide a more stable tool for reflection than the ever-changing memories of the researcher and participants (Roth, 2007 ). Decisions regarding inclusion and exclusion are made by the researcher throughout the entire research process from the initial framing of the footage to the final edit of the video. Members of the research team should acknowledge how personal bias impacts these decisions and make their choices clear in the research protocol to ensure inclusivity (Miller & Zhou, 2007 ).
One affordance of video research is that analysis of footage can actually disrupt the initial assumptions of a study. Analysis of video can be standardized or even mechanized by seeking out predetermined codes, but it can also disclose the subjective by revealing the meaning behind actions and not just the actions themselves (S. Goldman & McDermott, 2007 ; Knoblauch & Tuma, 2011 ). However, when using subjective analysis the researcher needs to keep in mind that the footage only reveals parts of an event. Ideally, a research team has a member who acts as both a researcher and a filmmaker. That team member can provide an important link between the full context of the event and the narrower viewpoint revealed through the captured footage during the analysis phase.
Although many participants are initially camera-shy, they often find enjoyment from participating in a study that includes video (Tobin & Hsueh, 2007 ). Video research provides an opportunity for participants to observe themselves and even share their experience with others through viewing and sharing the videos. With increased accessibility of video content online and the ease of sharing videos digitally, it is vital from an ethical and moral perspective that participants understand the study release forms and how their image and words might continue to be used and disseminated for years after the study is completed.
Including video in a research study creates both affordances and constraints regarding the dissemination of results. Finding a journal for a video-based study can be difficult. Traditional journals rely heavily on static text and graphics, but newly-created media journals include rich and engaging data such as video and interactive, web-based visualizations (Heath et al., 2010 ). In addition, videos can provide opportunities for research results to reach a broader audience outside of the traditional research audience through online channels such as YouTube and Vimeo.
Use of mixed methods with video data collection and analysis can complement the design-based, iterative nature of research that includes human participants. Design-based video research allows for both qualitative and quantitative collection and analysis of data throughout the project, as various events are encapsulated for specific examination as well as analyzed comparatively for changes over time. Design research, in general, provides the structure for implementing work in practice and iterative refinement of design towards achieving research goals (Collins, Joseph, & Bielaczyc, 2004 ). Using an integrated mixed method design that cycles through qualitative and quantitative analyses as the project progresses gives researchers the opportunity to observe trends and patterns in qualitative data and quantitative frequencies as each round of analysis informs additional insights (Gliner et al., 2009 ). This integrated use also provides a structure for evaluating project fidelity in an ongoing basis through a range of data points and findings from analyses that are consistent across the project. The ability to revise procedures for data collection, systematic analysis, and presenting work does not change the data being collected, but gives researchers the opportunity to optimize procedural aspects throughout the process.
Research as storytelling refers to the narrative traditions that underpin the use of video methods to analyze in a chronological context and present findings in a story-like timeline. These traditions are evident in ethnographic research methods that journal lived experiences through a period of time and in portraiture methods that use both aesthetic and scientific language to construct a portrait (Barone & Eisner, 2012 ; Heider, 2009 ; Lawrence-Lightfoot, 2005 ; Lenette, Cox & Brough, 2013 ).
In existing research, there is also attention given to the use of film and video documentaries as sources of data (e.g. Chattoo & Das, 2014 ; Warmington, van Gorp & Grosvenor, 2011 ), however, our discussion here focuses on using media to capture information and communicate resulting narratives for research purposes. In our work, we promote a perspective on emergent storytelling that develops from data collection and analysis, allowing the research to drive the narrative, and situating it in the context from where data was collected. We rely on theories and practices of research and storytelling that leverage the affordances of participant observation and interview for the construction of narratives (Bailey & Tilley, 2002 ; de Carteret, 2008 ; de Jager, Fogarty & Tewson, 2017 ; Gallagher, 2011 ; Hancox, 2017 ; LeBaron, Jarzabkowski, Pratt & Fetzer, 2017 ; Lewis, 2011 ; Meadows, 2003 ).
The type of storytelling used with research is distinctly different from methods used with documentaries, primarily with the distinction that, while documentary filmmakers can edit their film to a predetermined narrative, research storytelling requires that the data be analyzed and reported within a different set of ethical standards (Dahlstrom, 2014 ; Koehler, 2012 ; Nichols, 2010 ). Although documentary and research storytelling use a similar audiovisual medium, creating a story for research purposes is ethically-bounded by expectations in social science communities for being trustworthy in reporting and analyzing data, especially related to human subjects. Given that researchers using video may not know what footage will be useful for future storytelling, they may need to design their data collection methods to allow for an abundance of video data, which can impact analysis timelines as well. We believe it important to note these differences in the construction of related types of stories to make overt the essential need for research to consider not only analysis but also creation of the reporting narrative when designing and implementing data collection methods.
This study uses existing literature as a frame for understanding and implementing video research methods, then employs this frame as perspective on our own work, illuminating issues related to the use of video in research. In particular, we focus on using video research storytelling techniques to design, implement, and communicate the findings of a research study, providing examples from Dr. Erica Walker’s professional experience as a documentary filmmaker as well as evidence from current and former academic studies. The intent is to improve understanding of the theoretical foundations and practical applications for video research methods and better define how those apply to the construction of story-based video output of research findings.
The study began with a systematic analysis of theories and practices, using interpretive analytic methods, with thematic coding of evidence for conceptual and operational aspects of designing and implementing video research methods. From this information, a frame was constructed that includes foundational aspects of using digital video in research as well as the practical aspects of using video to create narratives with the intent of presenting research findings. We used this frame to interpret aspects of our own video research, identifying evidence that exemplifies aspects of the frame we used.
A primary goal for the analysis of existing literature was to focus on evidentiary data that could provide examples that illuminate the concepts that underpin the understanding of how, when, and why video research methods are useful for a range of publishing and dissemination of transferable knowledge from research. This emphasis on communicating results in both theoretical and practical ways highlighted areas within the analysis for potential contextual similarities between our work and other projects. A central reason for interpreting findings and connecting them with evidence was the need to provide examples that could serve as potentially transferable findings for others using video with their research. Given the need for a fertile environment (Zhao & Frank, 2003 ) and attention to contextual differences to avoid lethal mutations (Brown & Campione, 1996 ), understand that these examples may not work for every situation, but the intent is to provide clear evidence of how video research methods can leverage storytelling to report research findings in a way that is consumable by a broader audience.
In the following section, we present findings from the review of research and practice, along with evidence from our work with video research, connecting the conceptual and operational frame to examples and teasing out aspects from existing literature.
Results and findings
When looking at the current literature regarding the use of video in research, we developed a Mind Map to categorize convergent and divergent themes in the current literature, see Fig. 1 . Although this is far from a complete meta-analysis on video research (notably absent is a comprehensive discussion of ethical concerns regarding video research), the Mind Map focuses on four main properties in regards to video: video as a tool for storytelling/research, properties of the camera/video itself, how video impacts the person/researcher, and methods by which the researcher/viewer consumes video.
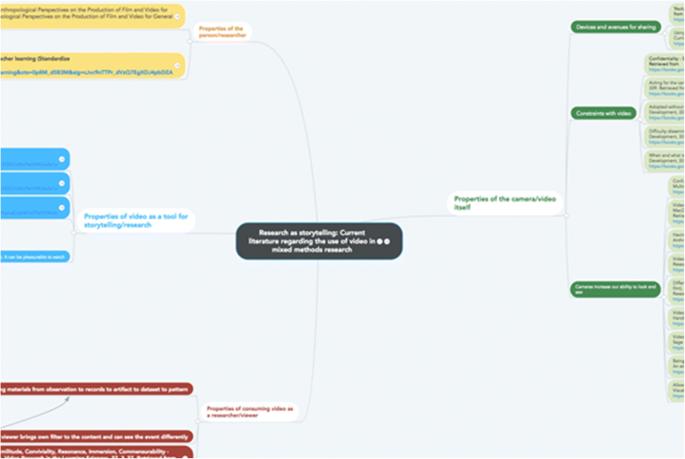
Mind Map of current literature regarding the use of video in mixed methods research. Link to the fully interactive Mind Map- http://clemsongc.com/ebwalker/mindmap/
Video, when used as a tool for research, can document and share ethnographic, epistemic, and storytelling data to participants and to the research team (R. Goldman, 2007 ; Heath et al., 2010 ; Miller & Zhou, 2007 ; Tobin & Hsueh, 2007 ). Much of the research in this area focuses on the properties (both positive and negative) inherent in the camera itself such as how video footage can increase the ability to see and experience the world, but can also act as a selective lens that separates an event from its natural context (S. Goldman & McDermott, 2007 ; Jewitt, n.d .; Knoblauch & Tuma, 2011 ; MacDougall, 2011 ; Miller & Zhou, 2007 ; Roth, 2007 ; Sossi, 2013 ).
Some research speaks to the role of the video-researcher within the context of the study, likening a video researcher to a participant-observer in ethnographic research (Derry, 2007 ; Roth, 2007 ; Sossi, 2013 ). The final category of research within the Mind Map focuses on the process of converting the video from an observation to records to artifact to dataset to pattern (Barron, 2007 ; R. Goldman, 2007 ; Knoblauch & Tuma, 2011 ; Newbury, 2011 ). Through this process of conversion, the video footage itself becomes an integral part of both the data and findings.
The focus throughout current literature was on video as data and the role it plays in collection and analysis during a study, but little has been written about how video could be used as a vehicle to present findings of a study. Current literature also did not address whether video-data could be used as a tool to communicate the findings of the research to a broader audience.
In a recent two-year study, the research team led by Dr. Erica Walker collected several types of video footage with the embedded intent to use video as both data and for telling the story of the study and findings once concluded (Walker, 2016 ). The study focused on a multidisciplinary team that converted a higher education Engineering course from lecture-based to game-based learning using the Cognitive Apprenticeship educational framework. The research questions examined the impact that the intervention had on student learning of domain content and twenty-first Century Skills. Utilizing video as both a data source and a delivery method was built into the methodology from the beginning. Therefore, interviews were conducted with the researchers and instructors before, during, and after the study to document consistency and changes in thoughts and observations as the study progressed. At the conclusion of the study, student participants reflected on their experience directly through individual video interviews. In addition, every class was documented using two static cameras, placed at different angles and framing, and a mobile camera unit to capture closeup shots of student-instructor, student-student, and student-content interactions. This resulted in more than six-hundred minutes of interview footage and over five-thousand minutes of classroom footage collected for the study.
Video data can be analyzed through quantitative methods (frequencies and word maps) as well as qualitative methods (emergent coding and commonalities versus outliers). Ideally, both methods are used in tandem so that preliminary results can continue to inform the overall analysis as it progresses. In order to capitalize on both methods, each interview was transcribed. The researchers leveraged digital and analog methods of coding such as digital word-search alongside hand coding the printed transcripts. Transcriptions contained timecode notations throughout, so coded segments could quickly be located in the footage and added to a timeline creating preliminary edits.
There are many software workflows that allow researchers to code, notate timecode for analysis, and pre-edit footage. In the study, Opportunities for Innovation: Game-based Learning in an Engineering Senior Design Course, NVivo qualitative analysis software was used together with paper-based analog coding. In a current study, also based on a higher education curriculum intervention, we are digitally coding and pre-trimming the footage in Adobe Prelude in addition to analog coding on the printed transcripts. Both workflows offer advantages. NVivo has built-in tools to create frequency maps and export graphs and charts relevant to qualitative analysis whereas Adobe Prelude adds coding notes directly into the footage metadata and connects directly with Adobe Premiere video editing software, which streamlines the editing process.
From our experience with both workflows, Prelude works better for a research team that has multiple team members with more video experience because it aligns with video industry workflows, implements tools that filmmakers already use, and Adobe Team Projects allows for co-editing and coding from multiple off-site locations. On the other hand, NVivo works better for research teams where members have more separate roles. NVivo is a common qualitative-analysis software so team members more familiar with traditional qualitative research can focus on coding and those more familiar with video editing can edit based on those codes allowing each team member to work within more familiar software workflows.
In both of these studies, assessments regarding storytelling occurred in conjunction with data processing and analysis. As findings were revealed, appropriate clips were grouped into timelines and edited to produce a library of short, topic-driven videos posted online , see Fig. 2 . A collection of story-based, topic-driven videos can provide other practitioners and researchers a first-hand account of how a study was designed and conducted, what worked well, recommendations of what to do differently, participant perspectives, study findings, and suggestions for further research. In fact, the videos cover many of the same topics traditionally found in publications, but in a collection of short videos accessible to a broad audience online.
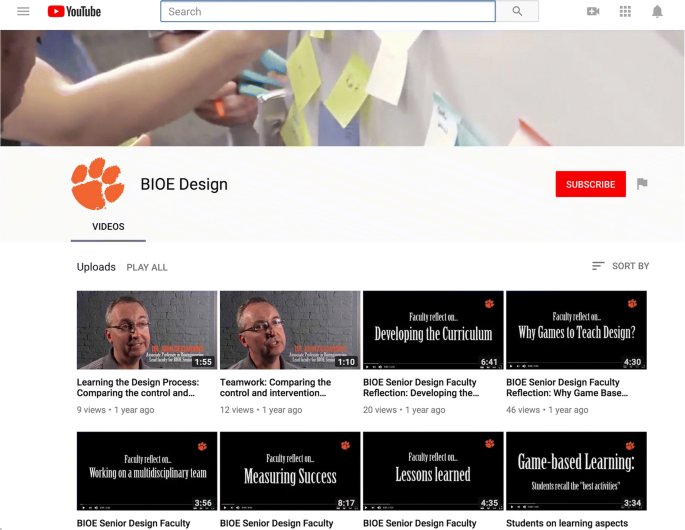
The YouTube channel created for Opportunities for Innovation: Game-based Learning in an Engineering Senior Design Course containing twenty-four short topical videos. Direct link- https://goo.gl/p8CBGG
By sharing the results of the study publicly online, conversations between practitioners and researchers can develop on a public stage. Research videos are easy to share across social media channels which can broaden the academic audience and potentially open doors for future research collaborations. As more journals move to accept multi-media studies, publicly posted videos provide additional ways to expose both academics and the general public to important study results and create easy access to related resources.
Video research as storytelling: The intersection and divergence of documentary filmmaking and video research
“Film and writing are such different modes of communication, filmmaking is not just a way of communicating the same kinds of knowledge that can be conveyed by an anthropological text. It is a way of creating different knowledge” (MacDougall, 2011 ).
When presenting research, choosing either mode of communication comes with affordances and constraints for the researcher, the participants, and the potential audience.
Many elements of documentary filmmaking, but not all, are relevant and appropriate when applied to gathering data and presenting results in video research. Documentary filmmakers have a specific angle on a story that they want to share with a broad audience. In many cases, they hope to incite action in viewers as a response to the story that unfolds on screen. In order to further their message, documentarians carefully consider the camera shots and interview clips that will convey the story clearly in a similar way to filmmakers in narrative genres. Decisions regarding what to capture and how to use the footage happen throughout the entire filmmaking process: prior to shooting footage (pre-production), while capturing footage (production), and during the editing phase (post-production).
Video researchers can employ many of the same technical skills from documentary filmmaking including interview techniques such as pre-written questions; camera skills such as framing, exposure, and lighting; and editing techniques that help draw a viewer through the storyline (Erickson, 2007 ; Tobin & Hsueh, 2007 ). In both documentary filmmaking and in video research, informed decisions are made about what footage to capture and how to employ editing techniques to produce a compelling final video.
Where video research diverges from documentary filmmaking is in how the researcher thinks about, captures, and processes the footage. Video researchers collect video as data in a more exploratory way whereas documentary filmmakers often look to capture preconceived video that will enable them to tell a specific story. For a documentary filmmaker, certain shots and interview responses are immediately discarded as they do not fit the intended narrative. For video researchers, all the video that is captured throughout a study is data and potentially part of the final research narrative. It is during the editing process (post-production) where the distinction between data and narrative becomes clear.
During post-production, video researchers are looking for clips that clearly reflect the emergent storylines seen in the collective data pool rather than the footage necessary to tell a predetermined story. Emergent storylines can be identified in several ways. Researchers look for divergent statements (where an interview subject makes unique observation different from other interviewees), convergent statements (where many different interviewees respond similarly), and unexpected statements (where something different from what was expected is revealed) (Knoblauch & Tuma, 2011 ).
When used thoughtfully, video research provides many sources of rich data. Examples include reflections of the experience, in the direct words of participants, that contain insights provided by body language and tone, an immersive glimpse into the research world as it unfolds, and the potential to capture footage throughout the entire research process rather than just during prescribed times. Video research becomes especially powerful when combined with qualitative and quantitative data from other sources because it can help reveal the context surrounding insights discovered during analysis.
We are not suggesting that video researchers should become documentary filmmakers, but researchers can learn from the stylistic approaches employed in documentary filmmaking. Video researchers implementing these tools can leverage the strengths of short-format video as a storytelling device to share findings with a more diverse audience, increase audience understanding and consumption of findings, and encourage a broader conversation around the research findings.
Implications for future work
As the development of digital media technologies continues to progress, we can expect new functionalities far exceeding current tools. These advancements will continue to expand opportunities for creating and sharing stories through video. By considering the role of video from the first stages of designing a study, researchers can employ methods that capitalize on these emerging technologies. Although they are still rapidly advancing, researchers can look for ways that augmented reality and virtual reality could change data analysis and reporting of research findings. Another emergent area is the use of machine learning and artificial intelligence to rapidly process video footage based on automated thematic coding. Continued advancements in this area could enable researchers to quickly quantify data points in large quantities of footage.
In addition to exploring new functionalities, researchers can still use current tools more effectively for capturing data, supporting analysis, and reporting findings. Mobile devices provide ready access to collect periodic video reflections from study participants and even create research vlogs (video blogs) to document and share ongoing studies as they progress. In addition, participant-created videos are rich artifacts for evaluating technical and conceptual knowledge as well as affective responses. Most importantly, as a community, researchers, designers, and documentarians can continue to take strengths from each field to further the reach of important research findings into the public sphere.
In conclusion, current research is focused on using video as a tool for data collection and analysis, but there are new, emerging opportunities for video to play an increased and diversified role in mixed methods research, especially as a tool for the presentation and consumption of findings. By leveraging the storytelling techniques used in documentary filmmaking, while staying true to the analytical methods of research design, researchers can use video to effectively communicate implications of their work to an audience beyond academia and leverage video storytelling to disseminate findings to the public.
Bailey, P. H., & Tilley, S. (2002). Storytelling and the interpretation of meaning in qualitative research. J Adv Nurs, 38(6), 574–583. http://doi.org/10.1046/j.1365-2648.2000.02224.x
Barone, T., & Eisner, E. W. (2012). Arts based research (pp. 1–183). https://doi.org/10.4135/9781452230627
Barron B (2007) Video as a tool to advance understanding of learning and development in peer, family, and other informal learning contexts. Video Research in the Learning Sciences:159–187
Brown AL, Campione JC (1996) Psychological theory and the design of innovative learning environments: on procedures, principles and systems. In: Schauble L, Glaser R (eds) Innovations in learning: new environments for education. Lawrence Erlbaum Associates, Hillsdale, NJ, pp 234–265
Google Scholar
Chalfen, R. (2011). Looking Two Ways: Mapping the Social Scientific Study of Visual Culture. In E. Margolis & L. Pauwels (Eds.), The Sage handbook of visual research methods . books.google.com
Chattoo, C. B., & Das, A. (2014). Assessing the Social Impact of Issues-Focused Documentaries: Research Methods and Future Considerations Center for Media & Social Impact, 24. Retrieved from https://www.namac.org/wpcontent/uploads/2015/01/assessing_impact_social_issue_documentaries_cmsi.pdf
Collins, A., Joseph, D., & Bielaczyc, K. (2004). Design research: theoretical and methodological issues. Journal of the Learning Sciences, 13(1), 15–42. https://doi.org/ https://doi.org/10.1207/s15327809jls1301_2
Dahlstrom, M. F. (2014). Using narratives and storytelling to communicate science with nonexpert audiences. Proc Natl Acad Sci, 111(Supplement_4), 13614–13620. http://doi.org/10.1073/pnas.1320645111
de Carteret, P. (2008). Storytelling as research praxis, and conversations that enabled it to emerge. Int J Qual Stud Educ, 21(3), 235–249. http://doi.org/10.1080/09518390801998296
de Jager A, Fogarty A, Tewson A (2017) Digital storytelling in research: a systematic review. Qual Rep 22(10):2548–2582
Derry SJ (2007) Video research in classroom and teacher learning (Standardize that!). Video Research in the Learning Sciences:305–320
Erickson F (2007) Ways of seeing video: toward a phenomenology of viewing minimally edited footage. Video Research in the Learning Sciences:145–155
Gallagher, K. M. (2011). In search of a theoretical basis for storytelling in education research: story as method. International Journal of Research and Method in Education, 34(1), 49–61. http://doi.org/10.1080/1743727X.2011.552308
Gliner, J. A., Morgan, G. A., & Leech, N. L. (2009). Research Methods in Applied Settings: An Integrated Approach to Design and Analysis, Second Edition . Taylor & Francis
Goldman R (2007) Video representations and the perspectivity framework: epistemology, ethnography, evaluation, and ethics. Video Research in the Learning Sciences 37:3–37
Goldman S, McDermott R (2007) Staying the course with video analysis Video Research in the Learning Sciences:101–113
Hancox, D. (2017). From subject to collaborator: transmedia storytelling and social research. Convergence, 23(1), 49–60. http://doi.org/10.1177/1354856516675252
Heath, C., Hindmarsh, J., & Luff, P.(2010). Video in Qualitative Research. SAGE Publications. Retrieved from https://market.android.com/details?id=book-MtmViguNi4UC
Heider KG (2009) Ethnographic film: revised edition. University of Texas Press
Henley P (2010) The Adventure of the Real: Jean Rouch and the Craft of Ethnographic Cinema. University of Chicago Press
Jewitt, C. (n.d). An introduction to using video for research - NCRM EPrints Repository. National Centre for Research Methods. Institute for Education, London. Retrieved from http://eprints.ncrm.ac.uk/2259/4/NCRM_workingpaper_0312.pdf
Knoblauch H, Tuma R (2011) Videography: An interpretative approach to video-recorded micro-social interaction. The SAGE Handbook of Visual Research Methods :414–430
Koehler D (2012) Documentary and ethnography: exploring ethical fieldwork models. Elon Journal Undergraduate Research in Communications 3(1):53–59 Retrieved from https://www.elon.edu/docs/e-web/academics/communications/research/vol3no1/EJSpring12_Full.pdf#page=53i
Lawrence-Lightfoot, S. (2005). Reflections on portraiture: a dialogue between art and science. Qualitative Inquiry: QI, 11(1), 3–15. https://doi.org/10.1177/1077800404270955
LeBaron, C., Jarzabkowski, P., Pratt, M. G., & Fetzer, G. (2017). An introduction to video methods in organizational research. Organ Res Methods, 21(2), 109442811774564. http://doi.org/10.1177/1094428117745649
Lenette, C., Cox, L., & Brough, M. (2013). Digital storytelling as a social work tool: learning from ethnographic research with women from refugee backgrounds. Br J Soc Work, 45(3), 988–1005. https://doi.org/10.1093/bjsw/bct184
Lewis, P. J. (2011). Storytelling as research/research as storytelling. Qual Inq, 17(6), 505–510. http://doi.org/10.1177/1077800411409883
(2011) Anthropological filmmaking: An empirical art. In: The sage handbook of visual research methods. MacDougall, D, pp 99–113
Meadows D (2003) Digital storytelling: research-based practice in new media. Visual Com(2):189–193
Miller K, Zhou X (2007) Learning from classroom video: what makes it compelling and what makes it hard. Video Research in the Learning Sciences:321–334
Newbury, D. (2011). Making arguments with images: Visual scholarship and academic publishing. In Eric Margolis & (Ed.), The SAGE Handbook of Visual Research Methods . na
Nichols B (2010) Why are ethical issues central to documentary filmmaking? Introduction to Documentary , Second Edition . In: 42–66
Roth W-M (2007) Epistemic mediation: video data as filters for the objectification of teaching by teachers. In: Goldman R, Pea R, Barron B, Derry SJ (eds) Video research in the learning sciences. Lawrence Erlbaum Ass Mahwah, NJ, pp 367–382
Sossi, D. (2013). Digital Icarus? Academic Knowledge Construction and Multimodal Curriculum Development, 339
Tobin J, Hsueh Y (2007) The poetics and pleasures of video ethnography of education. Video Research in the Learning Sciences:77–92
Using Film in Ethnographic Field Research - Methods@Manchester - The University of Manchester. (n.d.). Retrieved March 12, 2018, from https://www.methods.manchester.ac.uk/themes/ethnographic-methods/ethnographic-field-research/
Walker, E. B. (2016). Opportunities for Innovation: Game-based Learning in an Engineering Senior Design Course (PhD). Clemson University. Retrieved from http://tigerprints.clemson.edu/all_dissertations/1805/
Warmington, P., van Gorp, A., & Grosvenor, I. (2011). Education in motion: uses of documentary film in educational research. Paedagog Hist, 47(4), 457–472. https://doi.org/10.1080/00309230.2011.588239
Zhao, Y., & Frank, K. A. (2003). Factors affecting technology uses in schools: an ecological perspective. Am Educ Res J , 40(4), 807–840. https://doi.org/10.3102/00028312040004807
Download references
There was no external or internal funding for this study.
Availability of data and materials
Data is available in the Mind Map online which visually combines and interprets the full reference section available at the end of the full document.
Author information
Authors and affiliations.
Department of Graphic Communication, Clemson University, 207 Godfrey Hall, Clemson, SC, 29634, USA
Erica B. Walker
College of Education, Clemson University, 207 Tillman Hall, Clemson, SC, 29634, USA
D. Matthew Boyer
You can also search for this author in PubMed Google Scholar
Contributions
Article was co-written by both authors and based on previous work by Dr. Walker where Dr. Boyer served in the role of dissertation Committee Chair. Both authors read and approved the final manuscript.
Corresponding author
Correspondence to Erica B. Walker .
Ethics declarations
Competing interests.
Neither of the authors have any competing interest regarding this study or publication.
Publisher’s Note
Springer Nature remains neutral with regard to jurisdictional claims in published maps and institutional affiliations.
Rights and permissions
Open Access This article is distributed under the terms of the Creative Commons Attribution 4.0 International License ( http://creativecommons.org/licenses/by/4.0/ ), which permits unrestricted use, distribution, and reproduction in any medium, provided you give appropriate credit to the original author(s) and the source, provide a link to the Creative Commons license, and indicate if changes were made.
Reprints and permissions
About this article
Cite this article.
Walker, E.B., Boyer, D.M. Research as storytelling: the use of video for mixed methods research. Video J. of Educ. and Pedagogy 3 , 8 (2018). https://doi.org/10.1186/s40990-018-0020-4
Download citation
Received : 10 May 2018
Accepted : 04 July 2018
Published : 28 July 2018
DOI : https://doi.org/10.1186/s40990-018-0020-4
Share this article
Anyone you share the following link with will be able to read this content:
Sorry, a shareable link is not currently available for this article.
Provided by the Springer Nature SharedIt content-sharing initiative
- Mixed methods
- Storytelling
- Video research
Root out friction in every digital experience, super-charge conversion rates, and optimize digital self-service
Uncover insights from any interaction, deliver AI-powered agent coaching, and reduce cost to serve
Increase revenue and loyalty with real-time insights and recommendations delivered to teams on the ground
Know how your people feel and empower managers to improve employee engagement, productivity, and retention
Take action in the moments that matter most along the employee journey and drive bottom line growth
Whatever they’re are saying, wherever they’re saying it, know exactly what’s going on with your people
Get faster, richer insights with qual and quant tools that make powerful market research available to everyone
Run concept tests, pricing studies, prototyping + more with fast, powerful studies designed by UX research experts
Track your brand performance 24/7 and act quickly to respond to opportunities and challenges in your market
Explore the platform powering Experience Management
- Free Account
- Product Demos
- For Digital
- For Customer Care
- For Human Resources
- For Researchers
- Financial Services
- All Industries
Popular Use Cases
- Customer Experience
- Employee Experience
- Net Promoter Score
- Voice of Customer
- Customer Success Hub
- Product Documentation
- Training & Certification
- XM Institute
- Popular Resources
- Customer Stories
- Artificial Intelligence
Market Research
- Partnerships
- Marketplace
The annual gathering of the experience leaders at the world’s iconic brands building breakthrough business results, live in Salt Lake City.
- English/AU & NZ
- Español/Europa
- Español/América Latina
- Português Brasileiro
- REQUEST DEMO
- Experience Management
- Video in Qualitative Research
Try Qualtrics for free
Why use video in qualitative research.
10 min read If you or your team are running qualitative research, you may wonder if it’s useful to use video as a data source. We give you insights on the use of video in qualitative research and how best to use this data format.
What is video in qualitative research?
One of the major challenges and opportunities for organizations today is gaining a deeper and more authentic understanding of their customers.
From concerns and feedback to approval and advocacy, uncovering customer sentiment , needs and expectations is what will empower organizations to take the next step in designing, developing and improving experiences.
Most of this starts with qualitative research — e.g. focus groups, interviews, ethnographic studies — but the fundamental problem is that traditional approaches are sometimes restrictive, costly and difficult to scale.
Free eBook: The qualitative research design handbook
The challenges of traditional and disparate qualitative research
Slow: Long time to value while teams or agencies scope, recruit, design , build, execute, analyze & report out on studies. It also takes a long time to sift through qualitative data (e.g. video) and turn it into insights at scale.
Expensive: Qualitative methods of research can be costly, both when outsourcing to agencies or managing the process in-house. Organizations also have to factor in participant management costs (travel, hotels, venues, etc.).
Siloed: Data and research tools are outdated, siloed and scattered across teams and vendors, resulting in valuable data loss; no centralized approach to executing studies and incorporating customer/market insights across teams to support multiple initiatives. It also makes it hard to perform meta-analysis (e.g. perform analysis across multiple studies & methods) .
Hard to scale: Requires a lot of manual analysis; lack of in-house research & insights expertise due to cost of hiring/maintaining internal teams; heavy reliance on expertise of consultants to deliver quality insights; data from studies only used for initial research question, when it could be used by other teams.
Of course, while some organizations are using qualitative research, most rely heavily on in-person methods, manual work and a high degree of expertise to run these studies, meaning it’s near impossible to execute on data without these three things.
Alternative options that many organizations rely on:
- Agencies: Services-dependent with either an in-person or digital collection process, usually performed in a moderation style. Are slow, expensive, and result in loss of IP. Recruitment for interviews and discussion/diary studies can take a couple weeks or more. Reporting takes another couple weeks.
- In-person: Results in loss of efficiency & IP; data can’t be leveraged in concert with quantitative data easily; data can’t be reused. Very labor intensive to review and analyze all content.
- Point solutions: Technology providers for collecting synchronous or asynchronous video feedback. Can be either moderated or unprompted. Disconnected from other research and teams that could benefit from data and insights.
- Traditional qualitative studies: Organizations typically depend on traditional studies like, focus groups, personal interviews, phone calls, etc.
The reality is that the world has changed: the pandemic, coupled with the emergence of widespread remote and hybrid working, has forced researchers to move their efforts online.
Consequently, many organizations have turned to video feedback, a cost-effective type of qualitative research that can support existing methods. And it’s paying massive dividends.
History of video in qualitative research
Video in qualitative research is nothing new, and organizations have been using this response-gathering method for years to collect more authentic data from customers at scale.
It’s long been viewed as a medium through which an audiovisual record of everyday events, such as people shopping or students learning, is preserved and later made available for closer scrutiny, replay and analysis. In simple terms, video offers a window through which researchers can view more authentic and specific situations, interactions and feedback to uncover deeper and more meaningful insights.
But with the rapid shift to digital, video has quickly become a popular type of supplementary qualitative research — and is often used to support existing qualitative efforts. Plus, the growing ubiquity of video recording technologies (Zoom, Skype, Teams), has made it even easier for researchers to acquire necessary data in streamlined and more effective ways.
The reason it’s so effective is because it’s as simple as using a mobile phone to record audio and video with just a press of a button. Plus, the affordability of high-quality technical equipment, e.g. wearable microphones, and superior camera quality compared to other forms of digital recording has made video a strong supplementary component of qualitative research.
As a result, video provides respondents with the freedom and flexibility to respond at their leisure — removing any issue of time — and with smartphone technologies that are increasingly of high grade, helping to mitigate costs while increasing scale.
And the best part? Organizations can get more insightful and actionable insights from data around-the-clock, and that data can be utilized for as long as necessary.
Types of video qualitative research methods
In 2016, university researcher, Rebecca Whiting, in ‘ Who’s Behind the Lens?: A Reflexive Analysis of Roles in Participatory Video Research ’, described the four types of video research methods that are now available:
- “Participatory video research, which uses participant-generated videos, such as video diaries,
- Videography, which entails filming people in the field as a way to document their activities,
- Video content analysis, which involves analysis of material not recorded by the researcher, or
- Video elicitation, which uses footage (either created for this purpose by the researcher, or extant video) to prompt discussion.”
What’s increasingly clear is that as technology advances and cloud-based platforms provide video streaming and capture services that are increasingly accessible, researchers now have an always-on, scalable and highly effective way to capture feedback from diverse audiences.
With video feedback, researchers can get to the “why” far faster than ever before. The scope of video in qualitative research has never been wider. Let’s take a closer look at what it can do for organizations.
Scope of video in qualitative research
There are several benefits to using video feedback in qualitative research studies, namely:
- More verifiability and less researcher bias – If a video record exists of a conversation, it’s possible to review.
- More authentic responses – in an open-ended survey, some participants may feel able to describe their views better in film, than by translating it down into text. This often results in them providing more “content” (or insight) than they would have done via a traditional survey or interview.
- Perception of being faster – The perceived immediacy and speed of giving feedback by video may appeal to people with less time, who otherwise may not have responded to the survey.
- Language support – The option for people to answer in their own language can help participants open up about topics in a way that writing down their thoughts may not allow for.
- Provides visual cues – Videos can provide context and additional information if the participant provides visual cues like body language or showing something physical to the camera.
- Video has some benefits over audio recordings and field notes – A working paper from the National Center of Research Methods found three benefits: “1) its character as a real-time sequential record; 2) a fine-grained multimodal record; and 3) its durability, malleable, and share-ability.” [1]
- Empowers every team to carry out and act on insight — With video responses available for all teams to view and utilize, it becomes significantly easier for teams to act on insights and make critical changes to their experience initiatives.
How can Qualtrics help with video in qualitative research?
Qualtrics Video Feedback solution is a for-purpose benefit to the Qualtrics products that help businesses manage their video feedback and insights, right alongside traditional survey data types.
It brings insights to life by making it easy for respondents to deliver their thoughts and feelings through a medium they’re familiar and comfortable with, resulting in 6x more content than traditional open feedback.
As well as this, Qualtrics Video Feedback features built-in AI-powered analytics that enables researchers to analyze and pull sentence-level topics and sentiment from video responses to see exactly how respondents feel at scale.
Finally, customizable video editing allows researchers to compile and showcase the best clips to tell the story of the data, helping to deliver a more authentic narrative that lands with teams and key stakeholders.
Qualtrics Video Feedback provides:
- End-to-end research: Conduct all types of video feedback research with point-and-click question types, data collection, analytics and reporting — all in the same platform. This means it’s easy to compare and combine qualitative and quantitative research , as well as make insights available to all relevant stakeholders.
- Scalability and security: Easily empower teams outside the research department to conduct their own market research with intuitive tools, guided solutions, and center of excellence capabilities to overcome skill gaps and governance concerns.
- Better, faster insights: As respondents can deliver more authentic responses at their leisure (or at length), you can gather quality insights in hours and days, not weeks or months, and at an unmatched scale.
Related resources
Market intelligence 10 min read, marketing insights 11 min read, ethnographic research 11 min read, qualitative vs quantitative research 13 min read, qualitative research questions 11 min read, qualitative research design 12 min read, primary vs secondary research 14 min read, request demo.
Ready to learn more about Qualtrics?
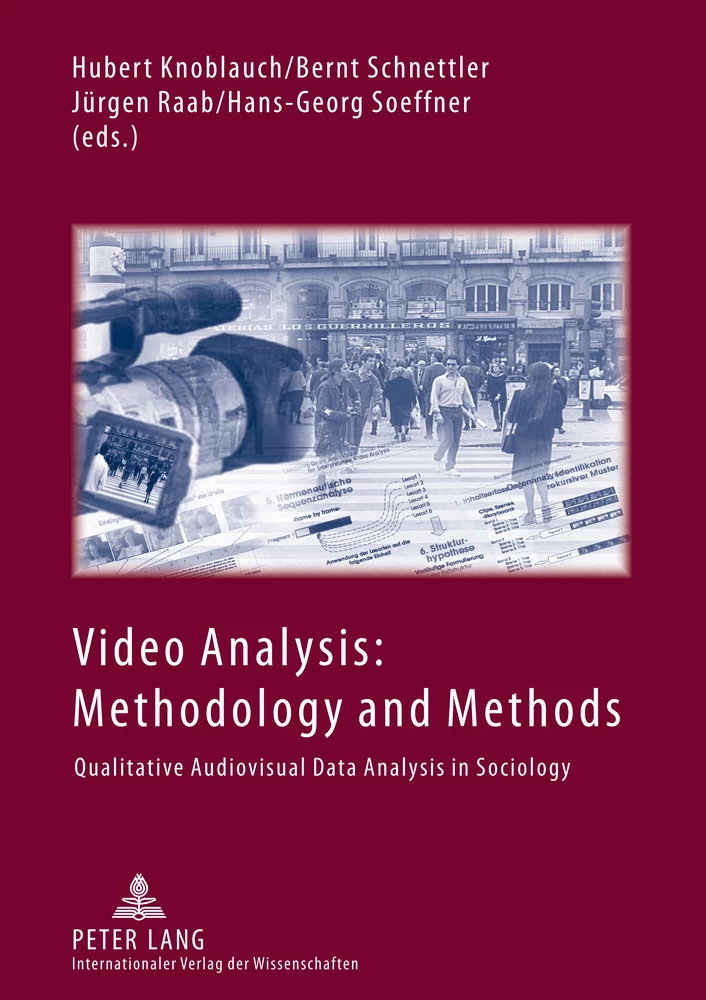
Preview Citation
Export citation.
Reference Manager
Video Analysis: Methodology and Methods
Qualitative Audiovisual Data Analysis in Sociology
- eBook for 51.15 € Download immediately. Incl. VAT Format: PDF and ePUB – for all devices
- Softcover for 55.25 € Shipping in approx. 10 working days national, international shipping possible
Biographical notes
Hubert Knoblauch (Volume editor) Bernt Schnettler (Volume editor) Jürgen Raab (Volume editor) Hans-Georg Soeffner (Volume editor)
Hubert Knoblauch is Professor of Sociology at the Technical University Berlin. Bernt Schnettler is Professor of Sociology at the University of Bayreuth. Both are conducting research on interaction, communicative genres and social forms and have build up laboratories of video analysis. Jürgen Raab is Professor of Sociology at the University of Magdeburg. Hans-Georg Soeffner is Professor emeritus at the University of Konstanz and former President of the German Sociological Association. They have developed and applied an original approach to visual data called Sociological Hermeneutics.
Key Subject Areas
- English Studies
- German Studies
- History & Political Science
- Law, Economics & Management
- Linguistics
- Media & Communication
- Romance Studies
- Science, Society and Culture
- Slavic Studies
- Theology & Philosophy
- Peter Lang Classics
Video Analysis
Video analysis is a field within computer vision that involves the automatic interpretation of digital video using computer algorithms. Although humans are readily able to interpret digital video, developing algorithms for the computer to perform the same task has been highly evasive and is now an active research field. Applications include tracking people who are walking; interpreting actions of moving objects and people; and using the technology to replace the array of screens used in monitoring high risk environments, such as airport security. Fundamental problems in video analysis include denoising, searching for events in video, object extraction (e.g., extract all the people in the scene), scale indifference (e.g. recognizing small trees or large trees, or trees close or far to the camera), reconstructing 3d scene information from video, removing vibration or jitter in the video, and spatially and temporally aligning video captured from multiple cameras. The VIP lab has active research in the field of video analysis.
Related people
Alexander Wong , David A. Clausi , Paul Fieguth
Shahid Haider , Shelley Wang , Devinder Kumar , Ruben Yousuf , M. Javad Shafiee , Hicham Sekkati
Abhishek Kumar , Akshaya Mishra , Amir H. Shabani , Fred Tung , Kurtis McBride , Natalie El-Nabbout , Neil Cavan , Parthipan Siva , Qiyao Yu , Ying Liu , Simon Booth , Christian Scharfenberger , Zohreh Azimifar
Related demos
Action Recognition in Video
Related publications
Journal articles.

Wong, A. , M. J. Shafiee , and Z. Azimifar , " Statistical Conditional Sampling for Variable-Resolution Video Compression ", Public Library of Science ONE , 2012. Details
Conference papers
Shabani, A. H. , D. A. Clausi , and J. S. Zelek, " Improved Spatio-temporal Salient Feature Detection for Action Recognition ", British Machine Vision Conference , University of Dundee, Dundee, UK, August, 2011. Details
Liu, Y. , P. Fieguth , and A. Wong , " A structure-guided conditional sampling model for video resolution enhancement ", IEEE International Conference on Image Processing (IEEE ICIP) , 2011. Details
Chan, C., and A. Wong , " Shot Boundary Detection using Genetic Algorithm Optimization ", IEEE International Symposium on Multimedia , 2011. Details
Ghaeminia, M. H., A. H. Shabani , and S. B. Shokouhi, " Two-level Parallel Histogram of Appearance for Better Human Tracking in Video ", IEEE Machine Vision and Image Processing Conference, Oct., 2010. Details
Shabani, A. H. , J. S. Zelek, and D. A. Clausi , " Human action recognition using salient opponent-based motion features ", 7th Canadian Conference on Computer and Robotic Vision , Ottawa, Ontario, Canada, pp. 362 - 369, March, 2010. Details
Shabani, A. H ., M. H. Ghaeminia, and S. B. Shokouhi, " Human tracking using spatialized multi-level histogramming and mean shift ", IEEE Canadian Conference on Computer and Robot Vision, May, 2010. Details
Shabani, A. H. , J. S. Zelek, and D. A. Clausi , " Robust Local Video Event Detection for Action Recognition ", Advances in Neural Information Processing Systems (NIPS), Machine Learning for Assistive Technology Workshop, Whistler, Canada , December, 2010. Details
Ghaeminia, M. H., A. H. Shabani , and S. B. Shokouhi, " Adaptive motion model for human tracking using Particle Filter ", International Conference on Pattern Recognition, Aug., 2010. Details
Shabani, A. H. , D. A. Clausi , and J. S. Zelek, " Towards a robust spatio-temporal interest point detection for human action recognition ", IEEE Canadian Conference on Computer and Robot Vision, Kelowna, BC, Canada , Kelowna, British Columbia, Canada, pp. 237-243, February, 2009. Details
Kumar, A , Panic Detection in Human Crowds using Sparse Coding , , Waterloo, University of Waterloo, August, 2012. Details

- Contact Waterloo
- Maps & Directions
- Accessibility
The University of Waterloo acknowledges that much of our work takes place on the traditional territory of the Neutral, Anishinaabeg, and Haudenosaunee peoples. Our main campus is situated on the Haldimand Tract, the land granted to the Six Nations that includes six miles on each side of the Grand River. Our active work toward reconciliation takes place across our campuses through research, learning, teaching, and community building, and is co-ordinated within the Office of Indigenous Relations .
- Media Center
- Not yet translated
Content Analysis
What is content analysis.
Content analysis is a research technique used to systematically analyze the content of communication. It involves identifying patterns, themes, or biases within qualitative data such as text, images, audio, or video and works to interpret the meaning of the content and its context.
The Basic Idea
Theory, meet practice.
TDL is an applied research consultancy. In our work, we leverage the insights of diverse fields—from psychology and economics to machine learning and behavioral data science—to sculpt targeted solutions to nuanced problems.
Analyzing research data is hard work, no matter what type of data we’re using. Let’s imagine a group of researchers analyzing people’s choices around plant-based food. You might envision experimental groups and a control group being assigned to different conditions: reading an article about factory farming, reading about the effects of meat production on greenhouse gases, or no article for the control group. Then, they take everyone to lunch and see what they order: the meat or plant-based option? The data from this study would be quantitative ; Researchers would use statistical analysis to determine the percentage of people in each group who chose meat versus vegetarian options, and to identify any influencing factors in their results. While this might be what we typically picture when we think of data analysis, that's not what every type of study uses.
Content analysis focuses on qualitative data . In this case, rather than analyzing a binary lunch choice, researchers may bring participants in for a focus group. They would ask open-ended questions about how participants make food decisions, how they feel about what they read, and what other factors may be at play for them. Maybe there’s a third group of researchers, interested in understanding why people switch to vegan diets in the first place. They would turn to the internet (where there are endless youtube videos, reddit threads, and facebook posts from people explaining exactly what they eat and why). For both of these research groups, the data they are working with isn’t numerical. Instead, they’re analyzing an abundance of content .
To make sense of focus group transcripts, social media posts, or any other large qualitative data, researchers use content analysis, which helps us uncover patterns in the data. There are a number of ways we can analyze this content, and even though the data is qualitative, we can quantify some of the results : how often is a certain word used? Are certain phrases more likely to be said together? Are certain groups more likely to reference the same thing?
Alternatively, we can perform a more qualitative analysis , coding the words from the data, grouping the codes into themes, and reflecting on patterns within the themes to understand the meaning or bigger picture behind the data. Regardless of which method we choose, when working with qualitative data, we’ll be doing some sort of content analysis.
- Coding: The process of categorizing and labeling pieces of data to identify themes, patterns, and meanings. By identifying recurring topics that emerge from the data, codes can then be grouped into themes, which are key to understanding the deeper meaning of the content.
- Manifest Content: This refers to the explicit, surface-level elements of the content that are directly observable and measurable. Manifest content is straightforward and involves counting and categorizing visible elements, such as words, phrases, or images. For example, looking at social media posts, one could count the number of posts with #vegan, or analyze the number of keywords like ‘animal,’ ‘climate change,’ and ‘veganism’ that come up in an interview. 1
- Latent Content: Latent content refers to the underlying, implicit meanings and themes that are not immediately apparent on the surface. This type of content requires interpretation and understanding of the context to uncover the deeper significance of the communication. In the same analysis, this might involve interpreting the tone or sentiment behind the keywords (e.g., whether the thought is positive, negative, or neutral). This can mean unpacking whether words are used sarcastically or supportively. 1
- Intercoder Reliability: This measures the degree of agreement among different coders analyzing the same content. High intercoder reliability would indicate consistent coding across researchers, and is a way of examining potential bias in the analysis process.
- Sampling: In some content analysis, when sourcing data from an incredibly large resource (for example, reddit), not every post can be analyzed. Sampling is the process of selecting a representative subset of the content which ensures that the analysis is manageable and that the findings are generalizable.
- Content Validity: In content analysis, content validity refers to how well the categories, themes, or codes used in the analysis represent all aspects of the phenomena being studied. Achieving high content validity means ensuring that the analysis comprehensively covers the content relevant to the research question and accurately reflects the subject matter.
Content analysis has seriously evolved, transitioning from simple quantitative methods to incorporating more complex qualitative techniques. The beginnings of content analysis date back to the 18th century with the study of newspapers and pamphlets to understand public opinion and propaganda, with early researchers using simple counting techniques to analyze word frequency. The first known systematic content analysis was performed by Carl Robert Vilhelm Bjerre in the 19th century, focusing on hymn texts. 2
During World War II, this method gained prominence when researchers, including Harold Lasswell, analyzed propaganda to understand its effects on public opinion. Lasswell's work laid the groundwork for content analysis as a systematic research method, emphasizing the importance of studying communication to understand its influence on audiences. In the post-war period, content analysis expanded into journalism, political science, psychology, and marketing. In 1952, Bernard Berelson published Content Analysis in Communication Research , which became a seminal work, formalizing the methodology and its applications. 2
Berelson suggested that there are five main purposes of content analysis 2 :
1. To describe substance characteristics of message content;
2. To describe form characteristics of message content;
3. To make inferences to producers of content;
4. To make inferences to audiences of content;
5. To predict the effects of content on audiences.
Because early content analysis focused heavily on quantitative measures, such as counting word frequencies, themes, and concepts, there was still a world of qualitative research that had been left largely unacknowledged (or at least, not given any scientific credit) until the 1970s. At this point, qualitative content analysis emerged, emphasizing the interpretation of context and underlying meanings in communication. Klaus Krippendorff's work, particularly his book Content Analysis: An Introduction to Its Methodology , introduced more sophisticated and interpretive techniques, blending quantitative rigor with qualitative depth. 2
The advent of computers and digital tools in the late 20th century revolutionized content analysis, enabling researchers to handle larger datasets and perform more complex analyses, and software such as NVivo, Atlas.ti, and MAXQDA facilitated both quantitative and qualitative content analysis, allowing for more efficient coding and categorization of textual data.
Nowadays, content analysis has increasingly focused on digital content, leveraging the wide world of the internet and its limitless and rich content. Researchers can now use automated text analysis, machine learning, and natural language processing to analyze vast amounts of data more quickly.
What does the process of content analysis look like in a study? The first step in a research project is to define the research question , meaning identifying the objective that the content analysis aims to address. Next, researchers must choose the content to be analyzed. This could be text, images, audio, or video from various sources such as newspapers, social media, interviews, or advertisements. Then it’s time to develop a coding scheme , creating a set of codes and categories that will be used to analyze the content. This involves defining what each code represents and how it should be applied to the data and then systematically applying the codes in multiple rounds.
Researchers will then analyze the data , which could involve both quantitative counting of codes and/or qualitative interpretation of their meaning. Either way, they’ll examine the coded data to identify patterns, themes, and relationships. This synthesizing of the data is an important step to allow for interpretation of the results , which may involve relating the findings to existing theories, identifying implications for practice, or suggesting areas for further research. Lastly, the process of the content analysis and its findings will be written up, reflected on for limitations, and researchers will identify key areas that can be expanded on in future research endeavors.
Controversies
Content analysis, like any analysis method, is not without room for error. Researchers must navigate various potential biases that can impact the validity and reliability of their findings. Major errors can occur right from the beginning, namely when sampling. The selection of content to analyze can introduce bias if it isn’t representative of the entire population or domain of interest. Sampling decisions can significantly affect the outcomes and generalizability of the analysis. 3
As is true in the rest of the research world, researchers using content analysis are sometimes criticized for choosing content that aligns with their interests or hypotheses. Because they can’t interview every person on the planet or study every Facebook post on the internet, researchers may consciously or unconsciously be selecting content that aligns with what they’re hoping to find. This can be partially mitigated through random sampling or stratified sampling techniques to select content that’s more representative.
Once data has been gathered, the coding process brings more opportunity for subjectivity and bias. The process of coding and categorizing data can be influenced by the researcher’s personal beliefs, values, and potential research interests, changing how data is coded and interpreted . In the same vein, interpretive validity can be called into question, with the potential for cultural bias or contextual misunderstandings. Researchers may misinterpret the meaning of content because they don’t share the same background/culture of the creator, or they may be missing important context for when, where, and with whom the content was created. All of this can lead to inaccurate coding and faulty conclusions.
We’ve talked about how content analysis can be both quantitative or qualitative. While quantitative methods can provide valuable data, an overemphasis on counting and frequency can overlook the deeper meanings and complexities of the content. Focusing on surface-level data without exploring underlying themes or contexts can be superficial and lose the richness of qualitative insights. The mixed methods style (combining quantitative and qualitative approaches) or thematic type of content analysis allow researchers to dive further into the true experiences of people and the way they communicate.
Increasingly, AI can be leveraged at the retrieving and coding stages to promote efficiency and efficacy. While some are open to this new age, other researchers reject the concept of fully-automated content analysis. Even in quantitative content analysis, many argue that human ability to understand nuances, metaphors, and sarcasm is crucial for accurate interpretation—skills that many automated processes might miss. 3 However, as AI develops and its capability of comprehending more subtle language use improves, there is a lot of potential for AI in content analysis.
In the end, qualitative data can provide many insights that quantitative data can’t, and in addition to the limited generalizability and subjective and potentially biased nature of sampling, coding, and analyzing, there’s still the issue of the immense amount of time and resources required, as well as the ethical implications. In research that involves interacting with people directly, ensuring confidentiality and protecting the privacy of participants can be challenging , and there are many barriers to ensuring informed consent, especially in sensitive research areas. If researchers use data from social media, especially without users' knowledge, it's crucial to consider whether participants would consent to their information being used. When informed consent can't be obtained, how do we determine the appropriate use of their data? How can we ensure their privacy and protection? Content analysis must balance the wealth of available information with respect for individuals' privacy.
Related Content
Machine Learning
Machine Learning is increasingly being used in content analysis, allowing us to analyze huge amounts of data more quickly. This technique is a subset of artificial intelligence that uses statistical techniques to enable machines to learn from data and improve over time, loosely based on human learning.
Contextual Inquiry
Contextual inquiry is a research method used in user experience (UX) design to understand how people use a product or service in their real-world environment and context. Learn how contextual inquiry relates to, and differs from, content analysis.
Grounded Theory
Grounded theory is a qualitative research methodology designed to construct theories that are grounded in systematically gathered and analyzed data and, unlike other research methods that start with a hypothesis, grounded theory starts with data collection first and then uses that data to develop a theory. Learn how grounded theory and content analysis are related.
References
- Delve. (n.d.). Manifest content analysis vs. latent content analysis . https://delvetool.com/blog/manifest-content-analysis-latent-content-analysis
- Schreier, M. (2012). Qualitative content analysis in practice (pp. 10-23). SAGE Publications.
- Macnamara, J. (2018). Content analysis . University of Technology Sydney. Media and Communication Research Methods. https://www.researchgate.net/profile/Jim-Macnamara-2/publication/327910121_Content_Analysis/links/5db12fac92851c577eba6c90/Content-Analysis.pdf
About the Author

Annika Steele
Annika completed her Masters at the London School of Economics in an interdisciplinary program combining behavioral science, behavioral economics, social psychology, and sustainability. Professionally, she’s applied data-driven insights in project management, consulting, data analytics, and policy proposal. Passionate about the power of psychology to influence an array of social systems, her research has looked at reproductive health, animal welfare, and perfectionism in female distance runners.

Survey Design

Fogg Behavior Model

Consumer Behavior

Digital Ethnography
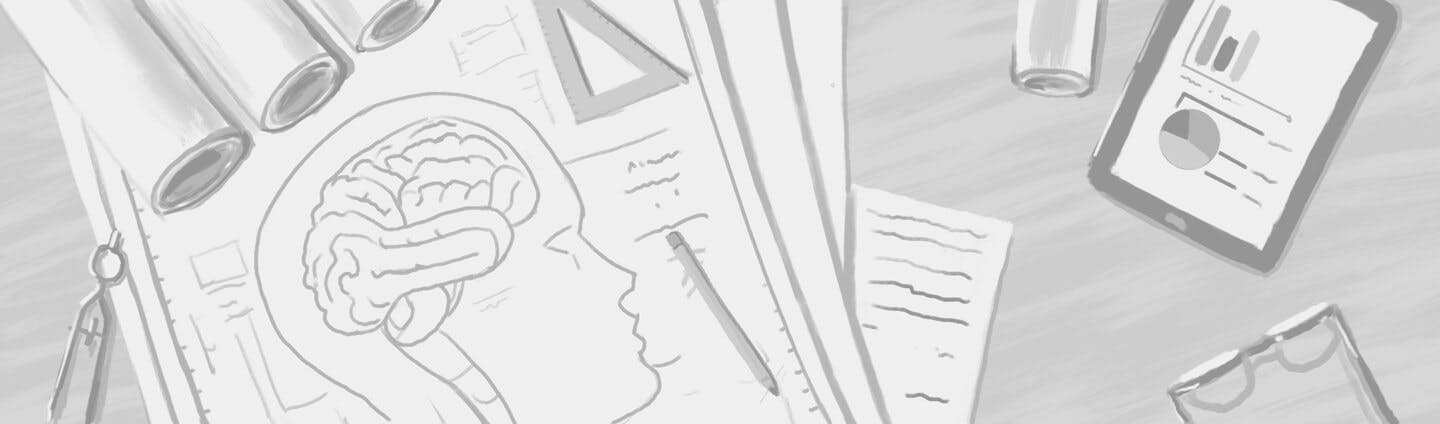
Eager to learn about how behavioral science can help your organization?
Get new behavioral science insights in your inbox every month..

21st Annual Bank Research Conference Videos
- Center for Financial Research
- Researchers
- Research Fellows
- Senior Advisor
- Special Advisor
- 2023 Fellows
- 2022 Fellows
- Visiting Scholar
- Working Papers
- 23rd Bank Research Conference
Conference Videos
- Speaker Information
- Poster Videos
- Poster Session Videos
- Previous Conference Programs
- Other Conferences
- 2021-2022 FDIC Academic Challenge
- 2020-2021 FDIC Academic Challenge
- Prior Years
- Research Assistants
- Internships
- Visiting Scholars
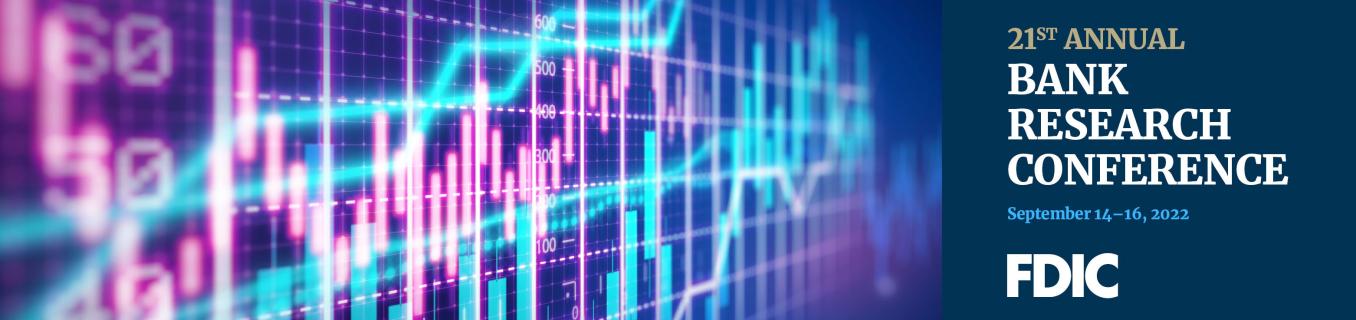
September 14, 2022
Fast Track Sessions
September 15, 2022
September 16, 2022
Last Updated: December 20, 2022

IMAGES
COMMENTS
The Visual-Verbal Video Analysis (VVVA) method provides a complete set of guidelines for researchers pursuing visual analysis in the social sciences, humanities, and medicine. ... The write-up, the final stage in qualitative video research, aims to convey findings in an understandable and impactful way for readers (Sandelowski, 1998).
Analyzing Video Data: Qualitative. Data Analysis Methods Innovation. Sep 26, 2023. By Janet Salmons, Ph.D., Research Community Manager for Sage Research Methods Community. Anyone who owns a smartphone has a video camera in their pocket. We can easily share digital pictures or media with friends and family, or the world of social media.
The video data session is discussed as a central part of research in the methodological literature (c.f. subsection 2), but without including empirical descriptions of the practice to develop new methodologies. Our empirical and self-reflexive video analysis of video analysis helps to uncover various practices of doing video analysis.
Video Data Analysis: How to Use 21st Century Video in the Social Sciences by Anne Nassauer and Nicolas M. Legewie (2022) Video data is transforming the possibilities of social science research. Whether through mobile phone footage, body-worn cameras or public video surveillance, we have access to an ever-expanding pool of data on real-life situations and interactions.
The second refers to data collection. We stress that, in addition to sequential analysis, the ethnographic dimension of video analysis should be taken into account methodologically. Video analysis requires, thirdly, a systematic account of the subjectivity, both of the actors analysed as well as of the analysts.
video analysis that draws on videos which have. been recorded - and produced in a more or less. professional way - by actors other than. researchers, for example private tapes made. available ...
This entry addresses the use of video data for the analysis of interaction in social situations. Videography means the combination of an ethnography focused on natural social situations, the collection of video data in these situations, and their analysis. In order to define videography, different forms of video data need to be distinguished first.
A Reflexive Analysis of Roles in Participatory Video Research," Whiting et al. (2016) used participatory video research methods. This team of researchers examined the "three-way relationship between researcher, participant, and videocam" in order to understand the roles of researchers and participants, and the materiality of methods using ...
The book discusses a range of video-based projects including studies of control centers, operating theatres, medical consultations, auction houses, and museums and galleries. Video in Qualitative Research is a valuable guide for students and researchers across the social sciences thinking of using video as part of their research.
A more widespread use of these frameworks for sharing data can improve the transparency and accessibility of research data for video analysis. Best practices for developers. We recommend three topics receive more attention by developers of methods for video analysis. First, there is a need for a common file format for storing results from pose ...
Qualitative video data analysis is an interpretive endeavor encompassing many modalities of engagement that aims to reach an integrated understanding of social situations that occur in, and ...
This article introduces an "Inquiry Graphics" (IG) approach for multimodal, Peircean semiotic video analysis and coding. It builds on Charles Sanders Peirce's core triadic interpretation of sign meaning-making. Multimodal methods offer analytical frameworks, templates and software to analyse video data. However, multimodal video analysis has been scarcely linked to semiotics in/of ...
Introduction. In the past few years, video analytics, also known as video content analysis or intelligent video analytics, has attracted increasing interest from both industry and the academic world. Thanks to the popularization of deep learning, video analytics has introduced the automation of tasks that were once the exclusive purview of humans.. Recent improvements in video analytics have ...
This paper reports on the opportunities and challenges of undertaking video analysis by reporting on the qualitative video analysis of a subset of 30 purposively selected videos from #notanurse ...
Nicolas M. Legewie is a postdoctoral researcher at the German Institute of Economic Research (DIW Berlin). He teaches and writes about social inequality and mobility, migration, social networks, as well as research design, digital social science research, research ethics, and video data analysis.
When looking at the current literature regarding the use of video in research, we developed a Mind Map to categorize convergent and divergent themes in the current literature, see Fig. 1.Although this is far from a complete meta-analysis on video research (notably absent is a comprehensive discussion of ethical concerns regarding video research), the Mind Map focuses on four main properties in ...
Video has some benefits over audio recordings and field notes - A working paper from the National Center of Research Methods found three benefits: "1) its character as a real-time sequential record; 2) a fine-grained multimodal record; and 3) its durability, malleable, and share-ability.". [1]
Video content analysis or video content analytics (VCA), also known as video analysis or video analytics (VA), is the capability of automatically analyzing video to detect and determine temporal and spatial events.. This technical capability is used in a wide range of domains including entertainment, [1] video retrieval and video browsing, [2] health-care, retail, automotive, transport, home ...
This meta-analysis reports on the overall effectiveness of video analysis when used with special educators, as well as on moderator analyses related to participant and instructional characteristics. Tau-U, a nonparametric effect size commonly used in single-case research, was used to aggregate the results from 191 AB phase contrasts across 12 ...
Used as a «microscope of interaction», this «video revolution» is expected to exert profound impact on research practice. But despite its popularity as an instrument, the methodological discussion of video is still underdeveloped. This book gathers a selection of outstanding European researchers in the field of qualitative interpretive ...
Video analysis is a field within computer vision that involves the automatic interpretation of digital video using computer algorithms. Although humans are readily able to interpret digital video, developing algorithms for the computer to perform the same task has been highly evasive and is now an active research field. Applications include tracking people who are walking;
video analysis that draws on videos which have. been recorded - and produced in a more or less. professional way - by actors other than. researchers, for example private tapes made. available ...
Content analysis is a research technique used to systematically analyze the content of communication. It involves identifying patterns, themes, or biases within qualitative data such as text, images, audio, or video and works to interpret the meaning of the content and its context.
developments, we briefly review the evolution of research, theory and practice related to. instructional decision making 1, assess the potential of new-generation video technology for. improving ...
The FDIC is proud to be a pre-eminent source of U.S. banking industry research, including quarterly banking profiles, working papers, and state banking performance data. Browse our extensive research tools and reports. More FDIC Analysis. Center for Financial Research Consumer Research FDIC National Survey of Unbanked and Underbanked Households