How to Write Limitations of the Study (with examples)
This blog emphasizes the importance of recognizing and effectively writing about limitations in research. It discusses the types of limitations, their significance, and provides guidelines for writing about them, highlighting their role in advancing scholarly research.
Updated on August 24, 2023

No matter how well thought out, every research endeavor encounters challenges. There is simply no way to predict all possible variances throughout the process.
These uncharted boundaries and abrupt constraints are known as limitations in research . Identifying and acknowledging limitations is crucial for conducting rigorous studies. Limitations provide context and shed light on gaps in the prevailing inquiry and literature.
This article explores the importance of recognizing limitations and discusses how to write them effectively. By interpreting limitations in research and considering prevalent examples, we aim to reframe the perception from shameful mistakes to respectable revelations.

What are limitations in research?
In the clearest terms, research limitations are the practical or theoretical shortcomings of a study that are often outside of the researcher’s control . While these weaknesses limit the generalizability of a study’s conclusions, they also present a foundation for future research.
Sometimes limitations arise from tangible circumstances like time and funding constraints, or equipment and participant availability. Other times the rationale is more obscure and buried within the research design. Common types of limitations and their ramifications include:
- Theoretical: limits the scope, depth, or applicability of a study.
- Methodological: limits the quality, quantity, or diversity of the data.
- Empirical: limits the representativeness, validity, or reliability of the data.
- Analytical: limits the accuracy, completeness, or significance of the findings.
- Ethical: limits the access, consent, or confidentiality of the data.
Regardless of how, when, or why they arise, limitations are a natural part of the research process and should never be ignored . Like all other aspects, they are vital in their own purpose.
Why is identifying limitations important?
Whether to seek acceptance or avoid struggle, humans often instinctively hide flaws and mistakes. Merging this thought process into research by attempting to hide limitations, however, is a bad idea. It has the potential to negate the validity of outcomes and damage the reputation of scholars.
By identifying and addressing limitations throughout a project, researchers strengthen their arguments and curtail the chance of peer censure based on overlooked mistakes. Pointing out these flaws shows an understanding of variable limits and a scrupulous research process.
Showing awareness of and taking responsibility for a project’s boundaries and challenges validates the integrity and transparency of a researcher. It further demonstrates the researchers understand the applicable literature and have thoroughly evaluated their chosen research methods.
Presenting limitations also benefits the readers by providing context for research findings. It guides them to interpret the project’s conclusions only within the scope of very specific conditions. By allowing for an appropriate generalization of the findings that is accurately confined by research boundaries and is not too broad, limitations boost a study’s credibility .
Limitations are true assets to the research process. They highlight opportunities for future research. When researchers identify the limitations of their particular approach to a study question, they enable precise transferability and improve chances for reproducibility.
Simply stating a project’s limitations is not adequate for spurring further research, though. To spark the interest of other researchers, these acknowledgements must come with thorough explanations regarding how the limitations affected the current study and how they can potentially be overcome with amended methods.
How to write limitations
Typically, the information about a study’s limitations is situated either at the beginning of the discussion section to provide context for readers or at the conclusion of the discussion section to acknowledge the need for further research. However, it varies depending upon the target journal or publication guidelines.
Don’t hide your limitations
It is also important to not bury a limitation in the body of the paper unless it has a unique connection to a topic in that section. If so, it needs to be reiterated with the other limitations or at the conclusion of the discussion section. Wherever it is included in the manuscript, ensure that the limitations section is prominently positioned and clearly introduced.
While maintaining transparency by disclosing limitations means taking a comprehensive approach, it is not necessary to discuss everything that could have potentially gone wrong during the research study. If there is no commitment to investigation in the introduction, it is unnecessary to consider the issue a limitation to the research. Wholly consider the term ‘limitations’ and ask, “Did it significantly change or limit the possible outcomes?” Then, qualify the occurrence as either a limitation to include in the current manuscript or as an idea to note for other projects.
Writing limitations
Once the limitations are concretely identified and it is decided where they will be included in the paper, researchers are ready for the writing task. Including only what is pertinent, keeping explanations detailed but concise, and employing the following guidelines is key for crafting valuable limitations:
1) Identify and describe the limitations : Clearly introduce the limitation by classifying its form and specifying its origin. For example:
- An unintentional bias encountered during data collection
- An intentional use of unplanned post-hoc data analysis
2) Explain the implications : Describe how the limitation potentially influences the study’s findings and how the validity and generalizability are subsequently impacted. Provide examples and evidence to support claims of the limitations’ effects without making excuses or exaggerating their impact. Overall, be transparent and objective in presenting the limitations, without undermining the significance of the research.
3) Provide alternative approaches for future studies : Offer specific suggestions for potential improvements or avenues for further investigation. Demonstrate a proactive approach by encouraging future research that addresses the identified gaps and, therefore, expands the knowledge base.
Whether presenting limitations as an individual section within the manuscript or as a subtopic in the discussion area, authors should use clear headings and straightforward language to facilitate readability. There is no need to complicate limitations with jargon, computations, or complex datasets.
Examples of common limitations
Limitations are generally grouped into two categories , methodology and research process .
Methodology limitations
Methodology may include limitations due to:
- Sample size
- Lack of available or reliable data
- Lack of prior research studies on the topic
- Measure used to collect the data
- Self-reported data

The researcher is addressing how the large sample size requires a reassessment of the measures used to collect and analyze the data.
Research process limitations
Limitations during the research process may arise from:
- Access to information
- Longitudinal effects
- Cultural and other biases
- Language fluency
- Time constraints

The author is pointing out that the model’s estimates are based on potentially biased observational studies.
Final thoughts
Successfully proving theories and touting great achievements are only two very narrow goals of scholarly research. The true passion and greatest efforts of researchers comes more in the form of confronting assumptions and exploring the obscure.
In many ways, recognizing and sharing the limitations of a research study both allows for and encourages this type of discovery that continuously pushes research forward. By using limitations to provide a transparent account of the project's boundaries and to contextualize the findings, researchers pave the way for even more robust and impactful research in the future.
Charla Viera, MS
See our "Privacy Policy"
Ensure your structure and ideas are consistent and clearly communicated
Pair your Premium Editing with our add-on service Presubmission Review for an overall assessment of your manuscript.

21 Research Limitations Examples
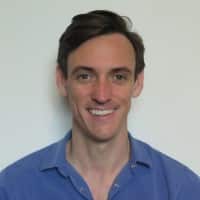
Chris Drew (PhD)
Dr. Chris Drew is the founder of the Helpful Professor. He holds a PhD in education and has published over 20 articles in scholarly journals. He is the former editor of the Journal of Learning Development in Higher Education. [Image Descriptor: Photo of Chris]
Learn about our Editorial Process
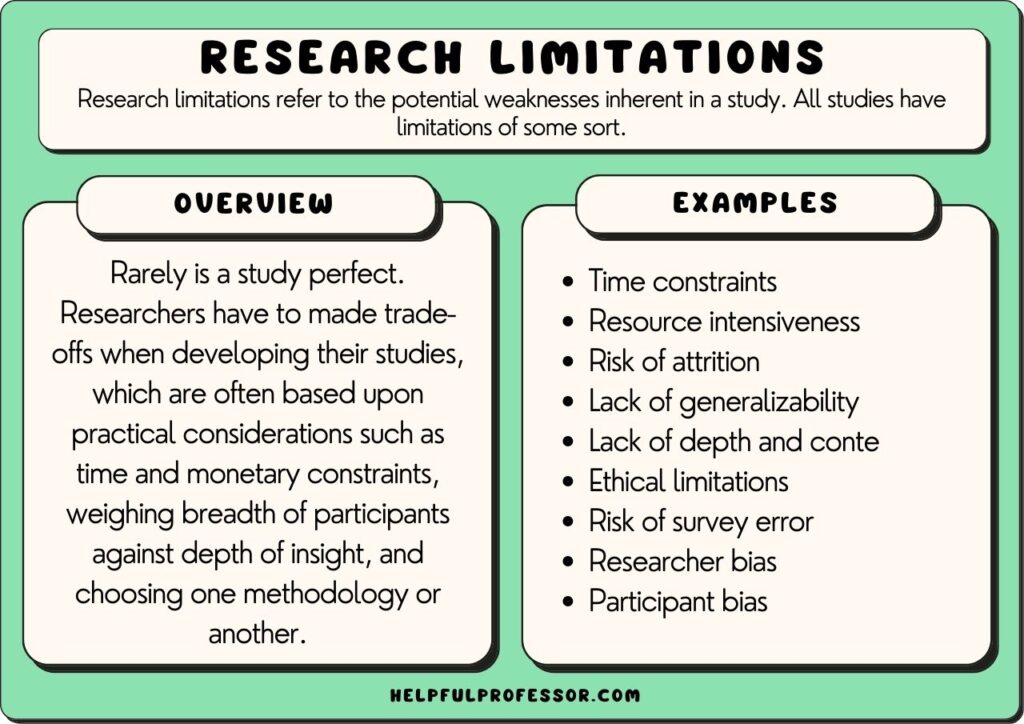
Research limitations refer to the potential weaknesses inherent in a study. All studies have limitations of some sort, meaning declaring limitations doesn’t necessarily need to be a bad thing, so long as your declaration of limitations is well thought-out and explained.
Rarely is a study perfect. Researchers have to make trade-offs when developing their studies, which are often based upon practical considerations such as time and monetary constraints, weighing the breadth of participants against the depth of insight, and choosing one methodology or another.
In research, studies can have limitations such as limited scope, researcher subjectivity, and lack of available research tools.
Acknowledging the limitations of your study should be seen as a strength. It demonstrates your willingness for transparency, humility, and submission to the scientific method and can bolster the integrity of the study. It can also inform future research direction.
Typically, scholars will explore the limitations of their study in either their methodology section, their conclusion section, or both.
Research Limitations Examples
Qualitative and quantitative research offer different perspectives and methods in exploring phenomena, each with its own strengths and limitations. So, I’ve split the limitations examples sections into qualitative and quantitative below.
Qualitative Research Limitations
Qualitative research seeks to understand phenomena in-depth and in context. It focuses on the ‘why’ and ‘how’ questions.
It’s often used to explore new or complex issues, and it provides rich, detailed insights into participants’ experiences, behaviors, and attitudes. However, these strengths also create certain limitations, as explained below.
1. Subjectivity
Qualitative research often requires the researcher to interpret subjective data. One researcher may examine a text and identify different themes or concepts as more dominant than others.
Close qualitative readings of texts are necessarily subjective – and while this may be a limitation, qualitative researchers argue this is the best way to deeply understand everything in context.
Suggested Solution and Response: To minimize subjectivity bias, you could consider cross-checking your own readings of themes and data against other scholars’ readings and interpretations. This may involve giving the raw data to a supervisor or colleague and asking them to code the data separately, then coming together to compare and contrast results.
2. Researcher Bias
The concept of researcher bias is related to, but slightly different from, subjectivity.
Researcher bias refers to the perspectives and opinions you bring with you when doing your research.
For example, a researcher who is explicitly of a certain philosophical or political persuasion may bring that persuasion to bear when interpreting data.
In many scholarly traditions, we will attempt to minimize researcher bias through the utilization of clear procedures that are set out in advance or through the use of statistical analysis tools.
However, in other traditions, such as in postmodern feminist research , declaration of bias is expected, and acknowledgment of bias is seen as a positive because, in those traditions, it is believed that bias cannot be eliminated from research, so instead, it is a matter of integrity to present it upfront.
Suggested Solution and Response: Acknowledge the potential for researcher bias and, depending on your theoretical framework , accept this, or identify procedures you have taken to seek a closer approximation to objectivity in your coding and analysis.
3. Generalizability
If you’re struggling to find a limitation to discuss in your own qualitative research study, then this one is for you: all qualitative research, of all persuasions and perspectives, cannot be generalized.
This is a core feature that sets qualitative data and quantitative data apart.
The point of qualitative data is to select case studies and similarly small corpora and dig deep through in-depth analysis and thick description of data.
Often, this will also mean that you have a non-randomized sample size.
While this is a positive – you’re going to get some really deep, contextualized, interesting insights – it also means that the findings may not be generalizable to a larger population that may not be representative of the small group of people in your study.
Suggested Solution and Response: Suggest future studies that take a quantitative approach to the question.
4. The Hawthorne Effect
The Hawthorne effect refers to the phenomenon where research participants change their ‘observed behavior’ when they’re aware that they are being observed.
This effect was first identified by Elton Mayo who conducted studies of the effects of various factors ton workers’ productivity. He noticed that no matter what he did – turning up the lights, turning down the lights, etc. – there was an increase in worker outputs compared to prior to the study taking place.
Mayo realized that the mere act of observing the workers made them work harder – his observation was what was changing behavior.
So, if you’re looking for a potential limitation to name for your observational research study , highlight the possible impact of the Hawthorne effect (and how you could reduce your footprint or visibility in order to decrease its likelihood).
Suggested Solution and Response: Highlight ways you have attempted to reduce your footprint while in the field, and guarantee anonymity to your research participants.
5. Replicability
Quantitative research has a great benefit in that the studies are replicable – a researcher can get a similar sample size, duplicate the variables, and re-test a study. But you can’t do that in qualitative research.
Qualitative research relies heavily on context – a specific case study or specific variables that make a certain instance worthy of analysis. As a result, it’s often difficult to re-enter the same setting with the same variables and repeat the study.
Furthermore, the individual researcher’s interpretation is more influential in qualitative research, meaning even if a new researcher enters an environment and makes observations, their observations may be different because subjectivity comes into play much more. This doesn’t make the research bad necessarily (great insights can be made in qualitative research), but it certainly does demonstrate a weakness of qualitative research.
6. Limited Scope
“Limited scope” is perhaps one of the most common limitations listed by researchers – and while this is often a catch-all way of saying, “well, I’m not studying that in this study”, it’s also a valid point.
No study can explore everything related to a topic. At some point, we have to make decisions about what’s included in the study and what is excluded from the study.
So, you could say that a limitation of your study is that it doesn’t look at an extra variable or concept that’s certainly worthy of study but will have to be explored in your next project because this project has a clearly and narrowly defined goal.
Suggested Solution and Response: Be clear about what’s in and out of the study when writing your research question.
7. Time Constraints
This is also a catch-all claim you can make about your research project: that you would have included more people in the study, looked at more variables, and so on. But you’ve got to submit this thing by the end of next semester! You’ve got time constraints.
And time constraints are a recognized reality in all research.
But this means you’ll need to explain how time has limited your decisions. As with “limited scope”, this may mean that you had to study a smaller group of subjects, limit the amount of time you spent in the field, and so forth.
Suggested Solution and Response: Suggest future studies that will build on your current work, possibly as a PhD project.
8. Resource Intensiveness
Qualitative research can be expensive due to the cost of transcription, the involvement of trained researchers, and potential travel for interviews or observations.
So, resource intensiveness is similar to the time constraints concept. If you don’t have the funds, you have to make decisions about which tools to use, which statistical software to employ, and how many research assistants you can dedicate to the study.
Suggested Solution and Response: Suggest future studies that will gain more funding on the back of this ‘ exploratory study ‘.
9. Coding Difficulties
Data analysis in qualitative research often involves coding, which can be subjective and complex, especially when dealing with ambiguous or contradicting data.
After naming this as a limitation in your research, it’s important to explain how you’ve attempted to address this. Some ways to ‘limit the limitation’ include:
- Triangulation: Have 2 other researchers code the data as well and cross-check your results with theirs to identify outliers that may need to be re-examined, debated with the other researchers, or removed altogether.
- Procedure: Use a clear coding procedure to demonstrate reliability in your coding process. I personally use the thematic network analysis method outlined in this academic article by Attride-Stirling (2001).
Suggested Solution and Response: Triangulate your coding findings with colleagues, and follow a thematic network analysis procedure.
10. Risk of Non-Responsiveness
There is always a risk in research that research participants will be unwilling or uncomfortable sharing their genuine thoughts and feelings in the study.
This is particularly true when you’re conducting research on sensitive topics, politicized topics, or topics where the participant is expressing vulnerability .
This is similar to the Hawthorne effect (aka participant bias), where participants change their behaviors in your presence; but it goes a step further, where participants actively hide their true thoughts and feelings from you.
Suggested Solution and Response: One way to manage this is to try to include a wider group of people with the expectation that there will be non-responsiveness from some participants.
11. Risk of Attrition
Attrition refers to the process of losing research participants throughout the study.
This occurs most commonly in longitudinal studies , where a researcher must return to conduct their analysis over spaced periods of time, often over a period of years.
Things happen to people over time – they move overseas, their life experiences change, they get sick, change their minds, and even die. The more time that passes, the greater the risk of attrition.
Suggested Solution and Response: One way to manage this is to try to include a wider group of people with the expectation that there will be attrition over time.
12. Difficulty in Maintaining Confidentiality and Anonymity
Given the detailed nature of qualitative data , ensuring participant anonymity can be challenging.
If you have a sensitive topic in a specific case study, even anonymizing research participants sometimes isn’t enough. People might be able to induce who you’re talking about.
Sometimes, this will mean you have to exclude some interesting data that you collected from your final report. Confidentiality and anonymity come before your findings in research ethics – and this is a necessary limiting factor.
Suggested Solution and Response: Highlight the efforts you have taken to anonymize data, and accept that confidentiality and accountability place extremely important constraints on academic research.
13. Difficulty in Finding Research Participants
A study that looks at a very specific phenomenon or even a specific set of cases within a phenomenon means that the pool of potential research participants can be very low.
Compile on top of this the fact that many people you approach may choose not to participate, and you could end up with a very small corpus of subjects to explore. This may limit your ability to make complete findings, even in a quantitative sense.
You may need to therefore limit your research question and objectives to something more realistic.
Suggested Solution and Response: Highlight that this is going to limit the study’s generalizability significantly.
14. Ethical Limitations
Ethical limitations refer to the things you cannot do based on ethical concerns identified either by yourself or your institution’s ethics review board.
This might include threats to the physical or psychological well-being of your research subjects, the potential of releasing data that could harm a person’s reputation, and so on.
Furthermore, even if your study follows all expected standards of ethics, you still, as an ethical researcher, need to allow a research participant to pull out at any point in time, after which you cannot use their data, which demonstrates an overlap between ethical constraints and participant attrition.
Suggested Solution and Response: Highlight that these ethical limitations are inevitable but important to sustain the integrity of the research.
For more on Qualitative Research, Explore my Qualitative Research Guide
Quantitative Research Limitations
Quantitative research focuses on quantifiable data and statistical, mathematical, or computational techniques. It’s often used to test hypotheses, assess relationships and causality, and generalize findings across larger populations.
Quantitative research is widely respected for its ability to provide reliable, measurable, and generalizable data (if done well!). Its structured methodology has strengths over qualitative research, such as the fact it allows for replication of the study, which underpins the validity of the research.
However, this approach is not without it limitations, explained below.
1. Over-Simplification
Quantitative research is powerful because it allows you to measure and analyze data in a systematic and standardized way. However, one of its limitations is that it can sometimes simplify complex phenomena or situations.
In other words, it might miss the subtleties or nuances of the research subject.
For example, if you’re studying why people choose a particular diet, a quantitative study might identify factors like age, income, or health status. But it might miss other aspects, such as cultural influences or personal beliefs, that can also significantly impact dietary choices.
When writing about this limitation, you can say that your quantitative approach, while providing precise measurements and comparisons, may not capture the full complexity of your subjects of study.
Suggested Solution and Response: Suggest a follow-up case study using the same research participants in order to gain additional context and depth.
2. Lack of Context
Another potential issue with quantitative research is that it often focuses on numbers and statistics at the expense of context or qualitative information.
Let’s say you’re studying the effect of classroom size on student performance. You might find that students in smaller classes generally perform better. However, this doesn’t take into account other variables, like teaching style , student motivation, or family support.
When describing this limitation, you might say, “Although our research provides important insights into the relationship between class size and student performance, it does not incorporate the impact of other potentially influential variables. Future research could benefit from a mixed-methods approach that combines quantitative analysis with qualitative insights.”
3. Applicability to Real-World Settings
Oftentimes, experimental research takes place in controlled environments to limit the influence of outside factors.
This control is great for isolation and understanding the specific phenomenon but can limit the applicability or “external validity” of the research to real-world settings.
For example, if you conduct a lab experiment to see how sleep deprivation impacts cognitive performance, the sterile, controlled lab environment might not reflect real-world conditions where people are dealing with multiple stressors.
Therefore, when explaining the limitations of your quantitative study in your methodology section, you could state:
“While our findings provide valuable information about [topic], the controlled conditions of the experiment may not accurately represent real-world scenarios where extraneous variables will exist. As such, the direct applicability of our results to broader contexts may be limited.”
Suggested Solution and Response: Suggest future studies that will engage in real-world observational research, such as ethnographic research.
4. Limited Flexibility
Once a quantitative study is underway, it can be challenging to make changes to it. This is because, unlike in grounded research, you’re putting in place your study in advance, and you can’t make changes part-way through.
Your study design, data collection methods, and analysis techniques need to be decided upon before you start collecting data.
For example, if you are conducting a survey on the impact of social media on teenage mental health, and halfway through, you realize that you should have included a question about their screen time, it’s generally too late to add it.
When discussing this limitation, you could write something like, “The structured nature of our quantitative approach allows for consistent data collection and analysis but also limits our flexibility to adapt and modify the research process in response to emerging insights and ideas.”
Suggested Solution and Response: Suggest future studies that will use mixed-methods or qualitative research methods to gain additional depth of insight.
5. Risk of Survey Error
Surveys are a common tool in quantitative research, but they carry risks of error.
There can be measurement errors (if a question is misunderstood), coverage errors (if some groups aren’t adequately represented), non-response errors (if certain people don’t respond), and sampling errors (if your sample isn’t representative of the population).
For instance, if you’re surveying college students about their study habits , but only daytime students respond because you conduct the survey during the day, your results will be skewed.
In discussing this limitation, you might say, “Despite our best efforts to develop a comprehensive survey, there remains a risk of survey error, including measurement, coverage, non-response, and sampling errors. These could potentially impact the reliability and generalizability of our findings.”
Suggested Solution and Response: Suggest future studies that will use other survey tools to compare and contrast results.
6. Limited Ability to Probe Answers
With quantitative research, you typically can’t ask follow-up questions or delve deeper into participants’ responses like you could in a qualitative interview.
For instance, imagine you are surveying 500 students about study habits in a questionnaire. A respondent might indicate that they study for two hours each night. You might want to follow up by asking them to elaborate on what those study sessions involve or how effective they feel their habits are.
However, quantitative research generally disallows this in the way a qualitative semi-structured interview could.
When discussing this limitation, you might write, “Given the structured nature of our survey, our ability to probe deeper into individual responses is limited. This means we may not fully understand the context or reasoning behind the responses, potentially limiting the depth of our findings.”
Suggested Solution and Response: Suggest future studies that engage in mixed-method or qualitative methodologies to address the issue from another angle.
7. Reliance on Instruments for Data Collection
In quantitative research, the collection of data heavily relies on instruments like questionnaires, surveys, or machines.
The limitation here is that the data you get is only as good as the instrument you’re using. If the instrument isn’t designed or calibrated well, your data can be flawed.
For instance, if you’re using a questionnaire to study customer satisfaction and the questions are vague, confusing, or biased, the responses may not accurately reflect the customers’ true feelings.
When discussing this limitation, you could say, “Our study depends on the use of questionnaires for data collection. Although we have put significant effort into designing and testing the instrument, it’s possible that inaccuracies or misunderstandings could potentially affect the validity of the data collected.”
Suggested Solution and Response: Suggest future studies that will use different instruments but examine the same variables to triangulate results.
8. Time and Resource Constraints (Specific to Quantitative Research)
Quantitative research can be time-consuming and resource-intensive, especially when dealing with large samples.
It often involves systematic sampling, rigorous design, and sometimes complex statistical analysis.
If resources and time are limited, it can restrict the scale of your research, the techniques you can employ, or the extent of your data analysis.
For example, you may want to conduct a nationwide survey on public opinion about a certain policy. However, due to limited resources, you might only be able to survey people in one city.
When writing about this limitation, you could say, “Given the scope of our research and the resources available, we are limited to conducting our survey within one city, which may not fully represent the nationwide public opinion. Hence, the generalizability of the results may be limited.”
Suggested Solution and Response: Suggest future studies that will have more funding or longer timeframes.
How to Discuss Your Research Limitations
1. in your research proposal and methodology section.
In the research proposal, which will become the methodology section of your dissertation, I would recommend taking the four following steps, in order:
- Be Explicit about your Scope – If you limit the scope of your study in your research question, aims, and objectives, then you can set yourself up well later in the methodology to say that certain questions are “outside the scope of the study.” For example, you may identify the fact that the study doesn’t address a certain variable, but you can follow up by stating that the research question is specifically focused on the variable that you are examining, so this limitation would need to be looked at in future studies.
- Acknowledge the Limitation – Acknowledging the limitations of your study demonstrates reflexivity and humility and can make your research more reliable and valid. It also pre-empts questions the people grading your paper may have, so instead of them down-grading you for your limitations; they will congratulate you on explaining the limitations and how you have addressed them!
- Explain your Decisions – You may have chosen your approach (despite its limitations) for a very specific reason. This might be because your approach remains, on balance, the best one to answer your research question. Or, it might be because of time and monetary constraints that are outside of your control.
- Highlight the Strengths of your Approach – Conclude your limitations section by strongly demonstrating that, despite limitations, you’ve worked hard to minimize the effects of the limitations and that you have chosen your specific approach and methodology because it’s also got some terrific strengths. Name the strengths.
Overall, you’ll want to acknowledge your own limitations but also explain that the limitations don’t detract from the value of your study as it stands.
2. In the Conclusion Section or Chapter
In the conclusion of your study, it is generally expected that you return to a discussion of the study’s limitations. Here, I recommend the following steps:
- Acknowledge issues faced – After completing your study, you will be increasingly aware of issues you may have faced that, if you re-did the study, you may have addressed earlier in order to avoid those issues. Acknowledge these issues as limitations, and frame them as recommendations for subsequent studies.
- Suggest further research – Scholarly research aims to fill gaps in the current literature and knowledge. Having established your expertise through your study, suggest lines of inquiry for future researchers. You could state that your study had certain limitations, and “future studies” can address those limitations.
- Suggest a mixed methods approach – Qualitative and quantitative research each have pros and cons. So, note those ‘cons’ of your approach, then say the next study should approach the topic using the opposite methodology or could approach it using a mixed-methods approach that could achieve the benefits of quantitative studies with the nuanced insights of associated qualitative insights as part of an in-study case-study.
Overall, be clear about both your limitations and how those limitations can inform future studies.
In sum, each type of research method has its own strengths and limitations. Qualitative research excels in exploring depth, context, and complexity, while quantitative research excels in examining breadth, generalizability, and quantifiable measures. Despite their individual limitations, each method contributes unique and valuable insights, and researchers often use them together to provide a more comprehensive understanding of the phenomenon being studied.
Attride-Stirling, J. (2001). Thematic networks: an analytic tool for qualitative research. Qualitative research , 1 (3), 385-405. ( Source )
Atkinson, P., Delamont, S., Cernat, A., Sakshaug, J., & Williams, R. A. (2021). SAGE research methods foundations . London: Sage Publications.
Clark, T., Foster, L., Bryman, A., & Sloan, L. (2021). Bryman’s social research methods . Oxford: Oxford University Press.
Köhler, T., Smith, A., & Bhakoo, V. (2022). Templates in qualitative research methods: Origins, limitations, and new directions. Organizational Research Methods , 25 (2), 183-210. ( Source )
Lenger, A. (2019). The rejection of qualitative research methods in economics. Journal of Economic Issues , 53 (4), 946-965. ( Source )
Taherdoost, H. (2022). What are different research approaches? Comprehensive review of qualitative, quantitative, and mixed method research, their applications, types, and limitations. Journal of Management Science & Engineering Research , 5 (1), 53-63. ( Source )
Walliman, N. (2021). Research methods: The basics . New York: Routledge.
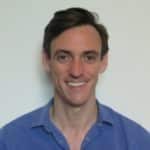
- Chris Drew (PhD) https://helpfulprofessor.com/author/chris-drew-phd-2/ 25 Number Games for Kids (Free and Easy)
- Chris Drew (PhD) https://helpfulprofessor.com/author/chris-drew-phd-2/ 25 Word Games for Kids (Free and Easy)
- Chris Drew (PhD) https://helpfulprofessor.com/author/chris-drew-phd-2/ 25 Outdoor Games for Kids
- Chris Drew (PhD) https://helpfulprofessor.com/author/chris-drew-phd-2/ 50 Incentives to Give to Students
Leave a Comment Cancel Reply
Your email address will not be published. Required fields are marked *
- Affiliate Program

- UNITED STATES
- 台灣 (TAIWAN)
- TÜRKIYE (TURKEY)
- Academic Editing Services
- - Research Paper
- - Journal Manuscript
- - Dissertation
- - College & University Assignments
- Admissions Editing Services
- - Application Essay
- - Personal Statement
- - Recommendation Letter
- - Cover Letter
- - CV/Resume
- Business Editing Services
- - Business Documents
- - Report & Brochure
- - Website & Blog
- Writer Editing Services
- - Script & Screenplay
- Our Editors
- Client Reviews
- Editing & Proofreading Prices
- Wordvice Points
- Partner Discount
- Plagiarism Checker
APA Citation Generator
MLA Citation Generator
Chicago Citation Generator
Vancouver Citation Generator
- - APA Style
- - MLA Style
- - Chicago Style
- - Vancouver Style
- Writing & Editing Guide
- Academic Resources
- Admissions Resources
Limitations of the Study – How to Write & Examples
What are the limitations of a study?
The limitations of a study are the elements of methodology or study design that impact the interpretation of your research results. The limitations essentially detail any flaws or shortcomings in your study. Study limitations can exist due to constraints on research design, methodology, materials, etc., and these factors may impact the findings of your study. However, researchers are often reluctant to discuss the limitations of their study in their papers, feeling that bringing up limitations may undermine its research value in the eyes of readers and reviewers.
In spite of the impact it might have (and perhaps because of it) you should clearly acknowledge any limitations in your research paper in order to show readers—whether journal editors, other researchers, or the general public—that you are aware of these limitations and to explain how they affect the conclusions that can be drawn from the research.
In this article, we provide some guidelines for writing about research limitations, show examples of some frequently seen study limitations, and recommend techniques for presenting this information. And after you have finished drafting and have received manuscript editing for your work, you still might want to follow this up with academic editing before submitting your work to your target journal.
Why do I need to include limitations of research in my paper?
Although limitations address the potential weaknesses of a study, writing about them toward the end of your paper actually strengthens your study by identifying any problems before other researchers or reviewers find them.
Furthermore, pointing out study limitations shows that you’ve considered the impact of research weakness thoroughly and have an in-depth understanding of your research topic. Since all studies face limitations, being honest and detailing these limitations will impress researchers and reviewers more than ignoring them.
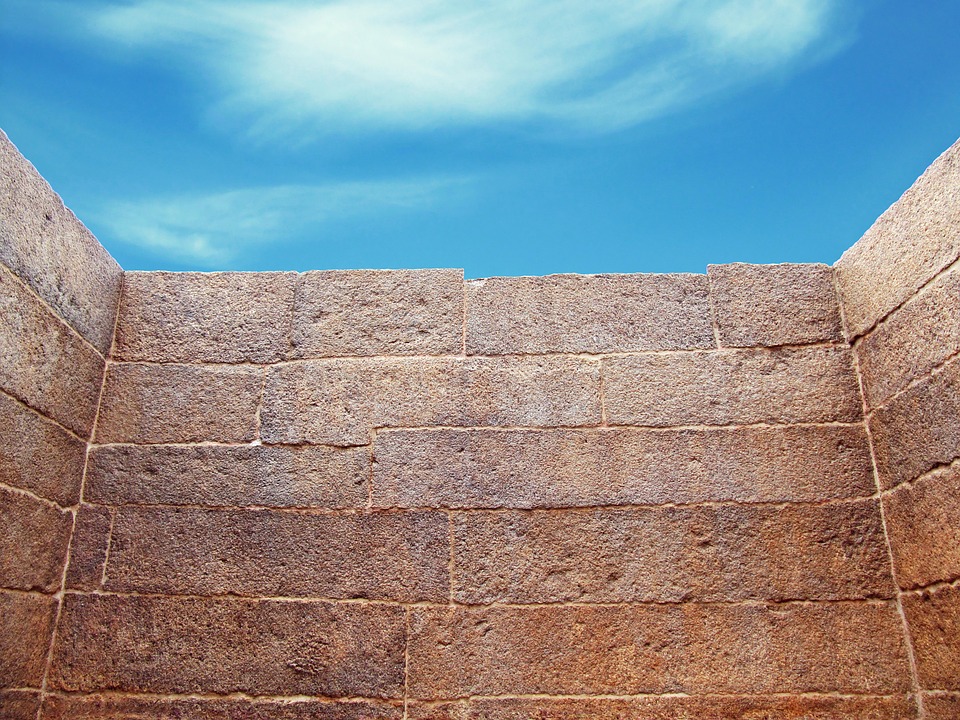
Where should I put the limitations of the study in my paper?
Some limitations might be evident to researchers before the start of the study, while others might become clear while you are conducting the research. Whether these limitations are anticipated or not, and whether they are due to research design or to methodology, they should be clearly identified and discussed in the discussion section —the final section of your paper. Most journals now require you to include a discussion of potential limitations of your work, and many journals now ask you to place this “limitations section” at the very end of your article.
Some journals ask you to also discuss the strengths of your work in this section, and some allow you to freely choose where to include that information in your discussion section—make sure to always check the author instructions of your target journal before you finalize a manuscript and submit it for peer review .
Limitations of the Study Examples
There are several reasons why limitations of research might exist. The two main categories of limitations are those that result from the methodology and those that result from issues with the researcher(s).
Common Methodological Limitations of Studies
Limitations of research due to methodological problems can be addressed by clearly and directly identifying the potential problem and suggesting ways in which this could have been addressed—and SHOULD be addressed in future studies. The following are some major potential methodological issues that can impact the conclusions researchers can draw from the research.
Issues with research samples and selection
Sampling errors occur when a probability sampling method is used to select a sample, but that sample does not reflect the general population or appropriate population concerned. This results in limitations of your study known as “sample bias” or “selection bias.”
For example, if you conducted a survey to obtain your research results, your samples (participants) were asked to respond to the survey questions. However, you might have had limited ability to gain access to the appropriate type or geographic scope of participants. In this case, the people who responded to your survey questions may not truly be a random sample.
Insufficient sample size for statistical measurements
When conducting a study, it is important to have a sufficient sample size in order to draw valid conclusions. The larger the sample, the more precise your results will be. If your sample size is too small, it will be difficult to identify significant relationships in the data.
Normally, statistical tests require a larger sample size to ensure that the sample is considered representative of a population and that the statistical result can be generalized to a larger population. It is a good idea to understand how to choose an appropriate sample size before you conduct your research by using scientific calculation tools—in fact, many journals now require such estimation to be included in every manuscript that is sent out for review.
Lack of previous research studies on the topic
Citing and referencing prior research studies constitutes the basis of the literature review for your thesis or study, and these prior studies provide the theoretical foundations for the research question you are investigating. However, depending on the scope of your research topic, prior research studies that are relevant to your thesis might be limited.
When there is very little or no prior research on a specific topic, you may need to develop an entirely new research typology. In this case, discovering a limitation can be considered an important opportunity to identify literature gaps and to present the need for further development in the area of study.
Methods/instruments/techniques used to collect the data
After you complete your analysis of the research findings (in the discussion section), you might realize that the manner in which you have collected the data or the ways in which you have measured variables has limited your ability to conduct a thorough analysis of the results.
For example, you might realize that you should have addressed your survey questions from another viable perspective, or that you were not able to include an important question in the survey. In these cases, you should acknowledge the deficiency or deficiencies by stating a need for future researchers to revise their specific methods for collecting data that includes these missing elements.
Common Limitations of the Researcher(s)
Study limitations that arise from situations relating to the researcher or researchers (whether the direct fault of the individuals or not) should also be addressed and dealt with, and remedies to decrease these limitations—both hypothetically in your study, and practically in future studies—should be proposed.
Limited access to data
If your research involved surveying certain people or organizations, you might have faced the problem of having limited access to these respondents. Due to this limited access, you might need to redesign or restructure your research in a different way. In this case, explain the reasons for limited access and be sure that your finding is still reliable and valid despite this limitation.
Time constraints
Just as students have deadlines to turn in their class papers, academic researchers might also have to meet deadlines for submitting a manuscript to a journal or face other time constraints related to their research (e.g., participants are only available during a certain period; funding runs out; collaborators move to a new institution). The time available to study a research problem and to measure change over time might be constrained by such practical issues. If time constraints negatively impacted your study in any way, acknowledge this impact by mentioning a need for a future study (e.g., a longitudinal study) to answer this research problem.
Conflicts arising from cultural bias and other personal issues
Researchers might hold biased views due to their cultural backgrounds or perspectives of certain phenomena, and this can affect a study’s legitimacy. Also, it is possible that researchers will have biases toward data and results that only support their hypotheses or arguments. In order to avoid these problems, the author(s) of a study should examine whether the way the research problem was stated and the data-gathering process was carried out appropriately.
Steps for Organizing Your Study Limitations Section
When you discuss the limitations of your study, don’t simply list and describe your limitations—explain how these limitations have influenced your research findings. There might be multiple limitations in your study, but you only need to point out and explain those that directly relate to and impact how you address your research questions.
We suggest that you divide your limitations section into three steps: (1) identify the study limitations; (2) explain how they impact your study in detail; and (3) propose a direction for future studies and present alternatives. By following this sequence when discussing your study’s limitations, you will be able to clearly demonstrate your study’s weakness without undermining the quality and integrity of your research.
Step 1. Identify the limitation(s) of the study
- This part should comprise around 10%-20% of your discussion of study limitations.
The first step is to identify the particular limitation(s) that affected your study. There are many possible limitations of research that can affect your study, but you don’t need to write a long review of all possible study limitations. A 200-500 word critique is an appropriate length for a research limitations section. In the beginning of this section, identify what limitations your study has faced and how important these limitations are.
You only need to identify limitations that had the greatest potential impact on: (1) the quality of your findings, and (2) your ability to answer your research question.

Step 2. Explain these study limitations in detail
- This part should comprise around 60-70% of your discussion of limitations.
After identifying your research limitations, it’s time to explain the nature of the limitations and how they potentially impacted your study. For example, when you conduct quantitative research, a lack of probability sampling is an important issue that you should mention. On the other hand, when you conduct qualitative research, the inability to generalize the research findings could be an issue that deserves mention.
Explain the role these limitations played on the results and implications of the research and justify the choice you made in using this “limiting” methodology or other action in your research. Also, make sure that these limitations didn’t undermine the quality of your dissertation .
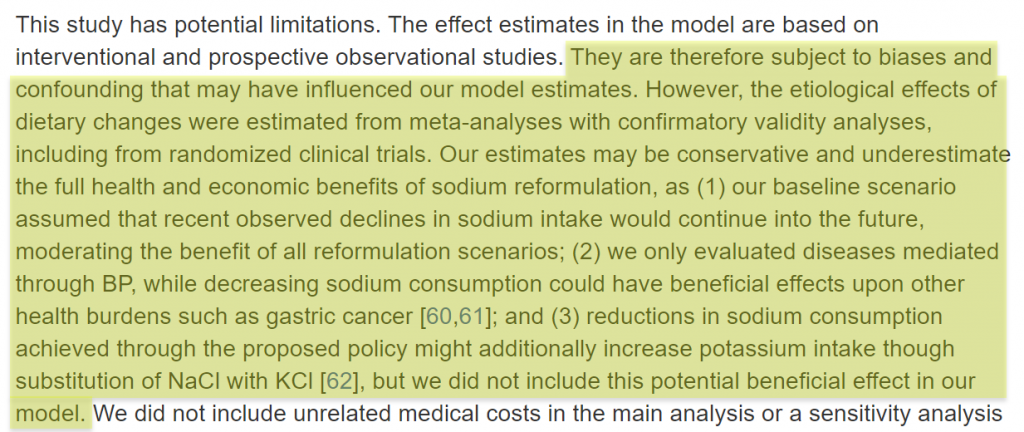
Step 3. Propose a direction for future studies and present alternatives (optional)
- This part should comprise around 10-20% of your discussion of limitations.
After acknowledging the limitations of the research, you need to discuss some possible ways to overcome these limitations in future studies. One way to do this is to present alternative methodologies and ways to avoid issues with, or “fill in the gaps of” the limitations of this study you have presented. Discuss both the pros and cons of these alternatives and clearly explain why researchers should choose these approaches.
Make sure you are current on approaches used by prior studies and the impacts they have had on their findings. Cite review articles or scientific bodies that have recommended these approaches and why. This might be evidence in support of the approach you chose, or it might be the reason you consider your choices to be included as limitations. This process can act as a justification for your approach and a defense of your decision to take it while acknowledging the feasibility of other approaches.
P hrases and Tips for Introducing Your Study Limitations in the Discussion Section
The following phrases are frequently used to introduce the limitations of the study:
- “There may be some possible limitations in this study.”
- “The findings of this study have to be seen in light of some limitations.”
- “The first is the…The second limitation concerns the…”
- “The empirical results reported herein should be considered in the light of some limitations.”
- “This research, however, is subject to several limitations.”
- “The primary limitation to the generalization of these results is…”
- “Nonetheless, these results must be interpreted with caution and a number of limitations should be borne in mind.”
- “As with the majority of studies, the design of the current study is subject to limitations.”
- “There are two major limitations in this study that could be addressed in future research. First, the study focused on …. Second ….”
For more articles on research writing and the journal submissions and publication process, visit Wordvice’s Academic Resources page.
And be sure to receive professional English editing and proofreading services , including paper editing services , for your journal manuscript before submitting it to journal editors.
Wordvice Resources
Proofreading & Editing Guide
Writing the Results Section for a Research Paper
How to Write a Literature Review
Research Writing Tips: How to Draft a Powerful Discussion Section
How to Captivate Journal Readers with a Strong Introduction
Tips That Will Make Your Abstract a Success!
APA In-Text Citation Guide for Research Writing
Additional Resources
- Diving Deeper into Limitations and Delimitations (PhD student)
- Organizing Your Social Sciences Research Paper: Limitations of the Study (USC Library)
- Research Limitations (Research Methodology)
- How to Present Limitations and Alternatives (UMASS)
Article References
Pearson-Stuttard, J., Kypridemos, C., Collins, B., Mozaffarian, D., Huang, Y., Bandosz, P.,…Micha, R. (2018). Estimating the health and economic effects of the proposed US Food and Drug Administration voluntary sodium reformulation: Microsimulation cost-effectiveness analysis. PLOS. https://journals.plos.org/plosmedicine/article?id=10.1371/journal.pmed.1002551
Xu, W.L, Pedersen, N.L., Keller, L., Kalpouzos, G., Wang, H.X., Graff, C,. Fratiglioni, L. (2015). HHEX_23 AA Genotype Exacerbates Effect of Diabetes on Dementia and Alzheimer Disease: A Population-Based Longitudinal Study. PLOS. Retrieved from https://journals.plos.org/plosmedicine/article?id=10.1371/journal.pmed.1001853
How to present limitations in research
Last updated
30 January 2024
Reviewed by
Short on time? Get an AI generated summary of this article instead
Limitations don’t invalidate or diminish your results, but it’s best to acknowledge them. This will enable you to address any questions your study failed to answer because of them.
In this guide, learn how to recognize, present, and overcome limitations in research.
- What is a research limitation?
Research limitations are weaknesses in your research design or execution that may have impacted outcomes and conclusions. Uncovering limitations doesn’t necessarily indicate poor research design—it just means you encountered challenges you couldn’t have anticipated that limited your research efforts.
Does basic research have limitations?
Basic research aims to provide more information about your research topic . It requires the same standard research methodology and data collection efforts as any other research type, and it can also have limitations.
- Common research limitations
Researchers encounter common limitations when embarking on a study. Limitations can occur in relation to the methods you apply or the research process you design. They could also be connected to you as the researcher.
Methodology limitations
Not having access to data or reliable information can impact the methods used to facilitate your research. A lack of data or reliability may limit the parameters of your study area and the extent of your exploration.
Your sample size may also be affected because you won’t have any direction on how big or small it should be and who or what you should include. Having too few participants won’t adequately represent the population or groups of people needed to draw meaningful conclusions.
Research process limitations
The study’s design can impose constraints on the process. For example, as you’re conducting the research, issues may arise that don’t conform to the data collection methodology you developed. You may not realize until well into the process that you should have incorporated more specific questions or comprehensive experiments to generate the data you need to have confidence in your results.
Constraints on resources can also have an impact. Being limited on participants or participation incentives may limit your sample sizes. Insufficient tools, equipment, and materials to conduct a thorough study may also be a factor.
Common researcher limitations
Here are some of the common researcher limitations you may encounter:
Time: some research areas require multi-year longitudinal approaches, but you might not be able to dedicate that much time. Imagine you want to measure how much memory a person loses as they age. This may involve conducting multiple tests on a sample of participants over 20–30 years, which may be impossible.
Bias: researchers can consciously or unconsciously apply bias to their research. Biases can contribute to relying on research sources and methodologies that will only support your beliefs about the research you’re embarking on. You might also omit relevant issues or participants from the scope of your study because of your biases.
Limited access to data : you may need to pay to access specific databases or journals that would be helpful to your research process. You might also need to gain information from certain people or organizations but have limited access to them. These cases require readjusting your process and explaining why your findings are still reliable.
- Why is it important to identify limitations?
Identifying limitations adds credibility to research and provides a deeper understanding of how you arrived at your conclusions.
Constraints may have prevented you from collecting specific data or information you hoped would prove or disprove your hypothesis or provide a more comprehensive understanding of your research topic.
However, identifying the limitations contributing to your conclusions can inspire further research efforts that help gather more substantial information and data.
- Where to put limitations in a research paper
A research paper is broken up into different sections that appear in the following order:
Introduction
Methodology
The discussion portion of your paper explores your findings and puts them in the context of the overall research. Either place research limitations at the beginning of the discussion section before the analysis of your findings or at the end of the section to indicate that further research needs to be pursued.
What not to include in the limitations section
Evidence that doesn’t support your hypothesis is not a limitation, so you shouldn’t include it in the limitation section. Don’t just list limitations and their degree of severity without further explanation.
- How to present limitations
You’ll want to present the limitations of your study in a way that doesn’t diminish the validity of your research and leave the reader wondering if your results and conclusions have been compromised.
Include only the limitations that directly relate to and impact how you addressed your research questions. Following a specific format enables the reader to develop an understanding of the weaknesses within the context of your findings without doubting the quality and integrity of your research.
Identify the limitations specific to your study
You don’t have to identify every possible limitation that might have occurred during your research process. Only identify those that may have influenced the quality of your findings and your ability to answer your research question.
Explain study limitations in detail
This explanation should be the most significant portion of your limitation section.
Link each limitation with an interpretation and appraisal of their impact on the study. You’ll have to evaluate and explain whether the error, method, or validity issues influenced the study’s outcome and how.
Propose a direction for future studies and present alternatives
In this section, suggest how researchers can avoid the pitfalls you experienced during your research process.
If an issue with methodology was a limitation, propose alternate methods that may help with a smoother and more conclusive research project . Discuss the pros and cons of your alternate recommendation.
Describe steps taken to minimize each limitation
You probably took steps to try to address or mitigate limitations when you noticed them throughout the course of your research project. Describe these steps in the limitation section.
- Limitation example
“Approaches like stem cell transplantation and vaccination in AD [Alzheimer’s disease] work on a cellular or molecular level in the laboratory. However, translation into clinical settings will remain a challenge for the next decade.”
The authors are saying that even though these methods showed promise in helping people with memory loss when conducted in the lab (in other words, using animal studies), more studies are needed. These may be controlled clinical trials, for example.
However, the short life span of stem cells outside the lab and the vaccination’s severe inflammatory side effects are limitations. Researchers won’t be able to conduct clinical trials until these issues are overcome.
- How to overcome limitations in research
You’ve already started on the road to overcoming limitations in research by acknowledging that they exist. However, you need to ensure readers don’t mistake weaknesses for errors within your research design.
To do this, you’ll need to justify and explain your rationale for the methods, research design, and analysis tools you chose and how you noticed they may have presented limitations.
Your readers need to know that even when limitations presented themselves, you followed best practices and the ethical standards of your field. You didn’t violate any rules and regulations during your research process.
You’ll also want to reinforce the validity of your conclusions and results with multiple sources, methods, and perspectives. This prevents readers from assuming your findings were derived from a single or biased source.
- Learning and improving starts with limitations in research
Dealing with limitations with transparency and integrity helps identify areas for future improvements and developments. It’s a learning process, providing valuable insights into how you can improve methodologies, expand sample sizes, or explore alternate approaches to further support the validity of your findings.
Should you be using a customer insights hub?
Do you want to discover previous research faster?
Do you share your research findings with others?
Do you analyze research data?
Start for free today, add your research, and get to key insights faster
Editor’s picks
Last updated: 18 April 2023
Last updated: 27 February 2023
Last updated: 6 February 2023
Last updated: 5 February 2023
Last updated: 16 April 2023
Last updated: 9 March 2023
Last updated: 30 April 2024
Last updated: 12 December 2023
Last updated: 11 March 2024
Last updated: 4 July 2024
Last updated: 6 March 2024
Last updated: 5 March 2024
Last updated: 13 May 2024
Latest articles
Related topics, .css-je19u9{-webkit-align-items:flex-end;-webkit-box-align:flex-end;-ms-flex-align:flex-end;align-items:flex-end;display:-webkit-box;display:-webkit-flex;display:-ms-flexbox;display:flex;-webkit-flex-direction:row;-ms-flex-direction:row;flex-direction:row;-webkit-box-flex-wrap:wrap;-webkit-flex-wrap:wrap;-ms-flex-wrap:wrap;flex-wrap:wrap;-webkit-box-pack:center;-ms-flex-pack:center;-webkit-justify-content:center;justify-content:center;row-gap:0;text-align:center;max-width:671px;}@media (max-width: 1079px){.css-je19u9{max-width:400px;}.css-je19u9>span{white-space:pre;}}@media (max-width: 799px){.css-je19u9{max-width:400px;}.css-je19u9>span{white-space:pre;}} decide what to .css-1kiodld{max-height:56px;display:-webkit-box;display:-webkit-flex;display:-ms-flexbox;display:flex;-webkit-align-items:center;-webkit-box-align:center;-ms-flex-align:center;align-items:center;}@media (max-width: 1079px){.css-1kiodld{display:none;}} build next, decide what to build next, log in or sign up.
Get started for free
What are the limitations in research and how to write them?
Learn about the potential limitations in research and how to appropriately address them in order to deliver honest and ethical research.
It is fairly uncommon for researchers to stumble into the term research limitations when working on their research paper. Limitations in research can arise owing to constraints on design, methods, materials, and so on, and these aspects, unfortunately, may have an influence on your subject’s findings.
In this Mind The Graph’s article, we’ll discuss some recommendations for writing limitations in research , provide examples of various common types of limitations, and suggest how to properly present this information.
What are the limitations in research?
The limitations in research are the constraints in design, methods or even researchers’ limitations that affect and influence the interpretation of your research’s ultimate findings. These are limitations on the generalization and usability of findings that emerge from the design of the research and/or the method employed to ensure validity both internally and externally.
Researchers are usually cautious to acknowledge the limitations of their research in their publications for fear of undermining the research’s scientific validity. No research is faultless or covers every possible angle. As a result, addressing the constraints of your research exhibits honesty and integrity .
Why should include limitations of research in my paper?
Though limitations tackle potential flaws in research, commenting on them at the conclusion of your paper, by demonstrating that you are aware of these limitations and explaining how they impact the conclusions that may be taken from the research, improves your research by disclosing any issues before other researchers or reviewers do .
Additionally, emphasizing research constraints implies that you have thoroughly investigated the ramifications of research shortcomings and have a thorough understanding of your research problem.
Limits exist in any research; being honest about them and explaining them would impress researchers and reviewers more than disregarding them.
Remember that acknowledging a research’s shortcomings offers a chance to provide ideas for future research, but be careful to describe how your study may help to concentrate on these outstanding problems.
Possible limitations examples
Here are some limitations connected to methodology and the research procedure that you may need to explain and discuss in connection to your findings.
Methodological limitations
Sample size.
The number of units of analysis used in your study is determined by the sort of research issue being investigated. It is important to note that if your sample is too small, finding significant connections in the data will be challenging, as statistical tests typically require a larger sample size to ensure a fair representation and this can be limiting.
Lack of available or reliable data
A lack of data or trustworthy data will almost certainly necessitate limiting the scope of your research or the size of your sample, or it can be a substantial impediment to identifying a pattern and a relevant connection.
Lack of prior research on the subject
Citing previous research papers forms the basis of your literature review and aids in comprehending the research subject you are researching. Yet there may be little if any, past research on your issue.
The measure used to collect data
After finishing your analysis of the findings, you realize that the method you used to collect data limited your capacity to undertake a comprehensive evaluation of the findings. Recognize the flaw by mentioning that future researchers should change the specific approach for data collection.
Issues with research samples and selection
Sampling inaccuracies arise when a probability sampling method is employed to choose a sample, but that sample does not accurately represent the overall population or the relevant group. As a result, your study suffers from “sampling bias” or “selection bias.”
Limitations of the research
When your research requires polling certain persons or a specific group, you may have encountered the issue of limited access to these interviewees. Because of the limited access, you may need to reorganize or rearrange your research. In this scenario, explain why access is restricted and ensure that your findings are still trustworthy and valid despite the constraint.
Time constraints
Practical difficulties may limit the amount of time available to explore a research issue and monitor changes as they occur. If time restrictions have any detrimental influence on your research, recognize this impact by expressing the necessity for a future investigation.
Due to their cultural origins or opinions on observed events, researchers may carry biased opinions, which can influence the credibility of a research. Furthermore, researchers may exhibit biases toward data and conclusions that only support their hypotheses or arguments.
The structure of the limitations section
The limitations of your research are usually stated at the beginning of the discussion section of your paper so that the reader is aware of and comprehends the limitations prior to actually reading the rest of your findings, or they are stated at the end of the discussion section as an acknowledgment of the need for further research.
The ideal way is to divide your limitations section into three steps:
1. Identify the research constraints;
2. Describe in great detail how they affect your research;
3. Mention the opportunity for future investigations and give possibilities.
By following this method while addressing the constraints of your research, you will be able to effectively highlight your research’s shortcomings without jeopardizing the quality and integrity of your research.
Present your research or paper in an innovative way
If you want your readers to be engaged and participate in your research, try Mind The Graph tool to add visual assets to your content. Infographics may improve comprehension and are easy to read, just as the Mind The Graph tool is simple to use and offers a variety of templates from which you can select the one that best suits your information.
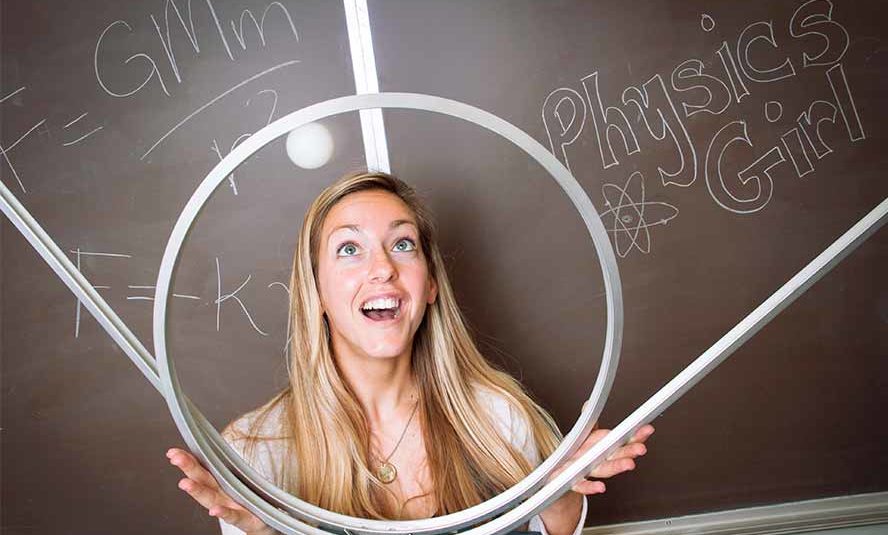
Subscribe to our newsletter
Exclusive high quality content about effective visual communication in science.
Sign Up for Free
Try the best infographic maker and promote your research with scientifically-accurate beautiful figures
no credit card required
About Jessica Abbadia
Jessica Abbadia is a lawyer that has been working in Digital Marketing since 2020, improving organic performance for apps and websites in various regions through ASO and SEO. Currently developing scientific and intellectual knowledge for the community's benefit. Jessica is an animal rights activist who enjoys reading and drinking strong coffee.
Content tags


Diving Deeper into Limitations and Delimitations

If you are working on a thesis, dissertation, or other formal research project, chances are your advisor or committee will ask you to address the delimitations of your study. When faced with this request, many students respond with a puzzled look and then go on to address what are actually the study’s limitations.
In a previous article , we covered what goes into the limitations, delimitations, and assumptions sections of your thesis or dissertation. Here, we will dive a bit deeper into the differences between limitations and delimitations and provide some helpful tips for addressing them in your research project—whether you are working on a quantitative or qualitative study.
Acknowledging Weaknesses vs. Defining Boundaries
These concepts are easy to get confused because both limitations and delimitations restrict (or limit) the questions you’ll be able to answer with your study, most notably in terms of generalizability.
However, the biggest difference between limitations and delimitations is the degree of control you have over them—that is, how much they are based in conscious, intentional choices you made in designing your study.
Limitations occur in all types of research and are, for the most part, outside the researcher’s control (given practical constraints, such as time, funding, and access to populations of interest). They are threats to the study’s internal or external validity.
Limitations may include things such as participant drop-out, a sample that isn’t entirely representative of the desired population, violations to the assumptions of parametric analysis (e.g., normality, homogeneity of variance), the limits of self-report, or the absence of reliability and validity data for some of your survey measures.
Limitations can get in the way of your being able to answer certain questions or draw certain types of inferences from your findings. Therefore, it’s important to acknowledge them upfront and make note of how they restrict the conclusions you’ll be able to draw from your study. Frequently, limitations can get in the way of our ability to generalize our findings to the larger populations or to draw causal conclusions, so be sure to consider these issues when you’re thinking about the potential limitations of your study.
Delimitations are also factors that can restrict the questions you can answer or the inferences you can draw from your findings. However, they are based on intentional choices you make a priori (i.e., as you’re designing the study) about where you’re going to draw the boundaries of your project. In other words, they define the project’s scope.
Like limitations, delimitations are a part of every research project, and this is not a bad thing. In fact, it’s very important! You can’t study everything at once. If you try to do so, your project is bound to get huge and unwieldy, and it will become a lot more difficult to interpret your results or come to meaningful conclusions with so many moving parts. You have to draw the line somewhere, and the delimitations are where you choose to draw these lines.
One of the clearest examples of a delimitation that applies to almost every research project is participant exclusion criteria. In conducting either a quantitative or a qualitative study, you will have to define your population of interest. Defining this population of interest means that you will need to articulate the boundaries of that population (i.e., who is not included). Those boundaries are delimitations.
For example, if you’re interested in understanding the experiences of elementary school teachers who have been implementing a new curriculum into their classrooms, you probably won’t be interviewing or sending a survey to any of the following people: non-teachers, high-school teachers, college professors, principals, parents of elementary school children, or the children themselves. Furthermore, you probably won’t be talking to elementary school teachers who have not yet had the experience of implementing the curriculum in question. You would probably only choose to gather data from elementary school teachers who have had this experience because that is who you’re interested in for the purposes of your study. Perhaps you’ll narrow your focus even more to elementary school teachers in a particular school district who have been teaching for a particular length of time. The possibilities can go on. These are choices you will need to make, both for practical reasons (i.e., the population you have access to) and for the questions you are trying to answer.
Of course, for this particular example, this does not mean that it wouldn’t be interesting to also know what principals think about the new curriculum. Or parents. Or elementary school children. It just means that, for the purposes of your project and your research questions, you’re interested in the experience of the teachers, so you’re excluding anyone who does not meet those criteria. Having delimitations to your population of interest also means that you won’t be able to answer any questions about the experiences of those other populations; this is ok because those populations are outside of the scope of your project . As interesting as their experiences might be, you can save these questions for another study. That is the part of the beauty of research: there will always be more studies to do, more questions to ask. You don’t have to (and can’t) do it all in one project.
Continuing with the previous example, for instance, let’s suppose that the problem you are most interested in addressing is the fact that we know relatively little about elementary school teachers’ experiences of implementing a new curriculum. Perhaps you believe that knowing more about teachers’ experiences could inform their training or help administrators know more about how to support their teachers. If the identified problem is our lack of knowledge about teachers’ experiences, and your research questions focus on better understanding these experiences, that means that you are choosing not to focus on other problems or questions, even those that may seem closely related. For instance, you are not asking how effective the new curriculum is in improving student test scores or graduation rates. You might think that would be a very interesting question, but it will have to wait for another study. In narrowing the focus of your research questions, you limit your ability to answer other questions, and again, that’s ok. These other questions may be interesting and important, but, again, they are beyond the scope of your project .
Common Examples of Limitations
While each study will have its own unique set of limitations, some limitations are more common in quantitative research, and others are more common in qualitative research.
In quantitative research, common limitations include the following:
– Participant dropout
– Small sample size, low power
– Non-representative sample
– Violations of statistical assumptions
– Non-experimental design, lack of manipulation of variables, lack of controls
– Potential confounding variables
– Measures with low (or unknown) reliability or validity
– Limits of an instrument to measure the construct of interest
– Data collection methods (e.g., self-report)
– Anything else that might limit the study’s internal or external validity
In qualitative research, common limitations include the following:
– Lack of generalizability of findings (not the goal of qualitative research, but still worth mentioning as a limitation)
– Inability to draw causal conclusions (again, not the goal of qualitative research, but still worth mentioning)
– Researcher bias/subjectivity (especially if there is only one coder)
– Limitations in participants’ ability/willingness to share or describe their experiences
– Any factors that might limit the rigor of data collection or analysis procedures
Common Examples of Delimitations
As noted above, the two most common sources of delimitations in both quantitative and qualitative research include the following:
– Inclusion/exclusion criteria (or how you define your population of interest)
– Research questions or problems you’ve chosen to examine
Several other common sources of delimitations include the following:
– Theoretical framework or perspective adopted
– Methodological framework or paradigm chosen (e.g., quantitative, qualitative, or mixed-methods)
– In quantitative research, the variables you’ve chosen to measure or manipulate (as opposed to others)
Whether you’re conducting a quantitative or qualitative study, you will (hopefully!) have chosen your research design because it is well suited to the questions you’re hoping to answer. Because these questions define the boundaries or scope of your project and thus point to its delimitations, your research design itself will also be related to these delimitations.
Questions to Ask Yourself
As you are considering the limitations and delimitations of your project, it can be helpful to ask yourself a few different questions.
Questions to help point out your study’s limitations :
1. If I had an unlimited budget, unlimited amounts of time, access to all possible populations, and the ability to manipulate as many variables as I wanted, how would I design my study differently to be better able to answer the questions I want to answer? (The ways in which your study falls short of this will point to its limitations.)
2. Are there design issues that get in the way of my being able to draw causal conclusions?
3. Are there sampling issues that get in the way of my being able to generalize my findings?
4. Are there issues related to the measures I’m using or the methods I’m using to collect data? Do I have concerns about participants telling the truth or being able to provide accurate responses to my questions?
5. Are there any other factors that might limit my study’s internal or external validity?
Questions that help point out your study’s delimitations :
1. What are my exclusion criteria? Who did I not include in my study, and why did I make this choice?
2. What questions did I choose not to address in my study? (Of course, the possibilities are endless here, but consider related questions that you chose not to address.)
3. In what ways did I narrow the scope of my study in order to hone in on a particular issue or question?
4. What other methodologies did I not use that might have allowed me to answer slightly different questions about the same topic?
How to Write About Limitations and Delimitations
Remember, having limitations and delimitations is not a bad thing. They’re present in even the most rigorous research. The important thing is to be aware of them and to acknowledge how they may impact your findings or the conclusions you can draw.
In fact, writing about them and acknowledging them gives you an opportunity to demonstrate that you can think critically about these aspects of your study and how they impact your findings, even if they were out of your control.
Keep in mind that your study’s limitations will likely point to important directions for future research. Therefore, when you’re getting ready to write about your recommendations for future research in your discussion, remember to refer back to your limitations section!
As you write about your delimitations in particular, remember that they are not weaknesses, and you don’t have to apologize for them. Good, strong research projects have clear boundaries. Also, keep in mind that you are the researcher and you can choose whatever delimitations you want for your study. You’re in control of the delimitations. You just have to be prepared—both in your discussion section and in your dissertation defense itself—to justify the choices you make and acknowledge how these choices impact your findings.
Browse More on PhDStudent

Everything You Need to Know About References and Citations: Part 1
When you conduct your research, it is important to record the details of all the information you find to provide accurate references, …

How to Write a Proposal: For a Master’s Thesis or Dissertation
Note: Many thanks to fellow PhDStudent blogger Ryan Krone for his contributions and insight to this post. Your thesis/dissertation proposal provides an …

How to Find Free Money for Graduate School Part 2
Getting into graduate school is already a challenge on its own, and funding the program once admitted is even harder. Graduate studies …
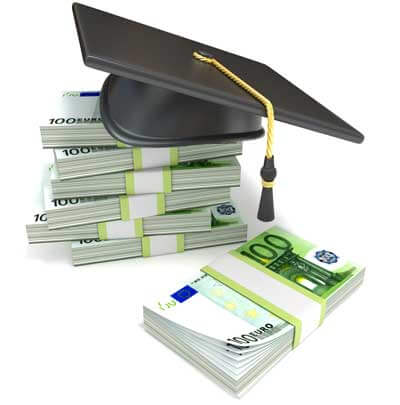
How to Find Free Money for Graduate School
You’ve finally earned your Bachelor’s degree and have made it into graduate school. Whether you already have massive student loans from undergrad …
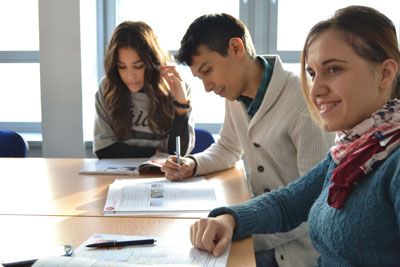
Part 3 of How to Pick Your Defense Committee
What strategies can a doctoral student employ to maneuver the trials and tribulations of a dissertation committee? In Part 2, we …
Best Dissertation Proofreading and Editing Tips to Make Your Work Spotless
You’ve heard this statement many times before: “the dissertation is the most important project you’ve ever worked on.” That may sound like …

Part 2 of How to Pick Your Defense Committee
Choosing a committee can be a daunting task for a doctoral student. We’ve already covered two strategies that can help you through this …

Part 1 of How to Pick Your Defense Committee
So you’re ready to pick your committee members; there are a few things to keep in mind first—after all, it is a …

Intro To Series on How to Pick Your Defense Committee
Choosing the right defense committee can potentially be the difference between a smooth transition of receiving your doctoral degree or dodging bullets …

On Babies and Dissertations: Part 3
I recently had the experience of expecting my first baby a month before I graduated. Throughout the process, I accidentally learned several …

On Babies and Dissertations: Part 2

On Babies and Dissertations: Part 1
I recently had the experience of expecting my first baby a month before I graduated and accidentally learned tips on graduating on time with a …
Click here to cancel reply.
You must be logged in to post a comment.
Copyright © 2024 PhDStudent.com. All rights reserved. Designed by Divergent Web Solutions, LLC .

Research Limitations & Delimitations
What they are and how they’re different (with examples)
By: Derek Jansen (MBA) | Expert Reviewed By: David Phair (PhD) | September 2022
If you’re new to the world of research, you’ve probably heard the terms “ research limitations ” and “ research delimitations ” being thrown around, often quite loosely. In this post, we’ll unpack what both of these mean, how they’re similar and how they’re different – so that you can write up these sections the right way.
Overview: Limitations vs Delimitations
- Are they the same?
- What are research limitations
- What are research delimitations
- Limitations vs delimitations
First things first…
Let’s start with the most important takeaway point of this post – research limitations and research delimitations are not the same – but they are related to each other (we’ll unpack that a little later). So, if you hear someone using these two words interchangeably, be sure to share this post with them!
Research Limitations
Research limitations are, at the simplest level, the weaknesses of the study , based on factors that are often outside of your control as the researcher. These factors could include things like time , access to funding, equipment , data or participants . For example, if you weren’t able to access a random sample of participants for your study and had to adopt a convenience sampling strategy instead, that would impact the generalizability of your findings and therefore reflect a limitation of your study.
Research limitations can also emerge from the research design itself . For example, if you were undertaking a correlational study, you wouldn’t be able to infer causality (since correlation doesn’t mean certain causation). Similarly, if you utilised online surveys to collect data from your participants, you naturally wouldn’t be able to get the same degree of rich data that you would from in-person interviews .
Simply put, research limitations reflect the shortcomings of a study , based on practical (or theoretical) constraints that the researcher faced. These shortcomings limit what you can conclude from a study, but at the same time, present a foundation for future research . Importantly, all research has limitations , so there’s no need to hide anything here – as long as you discuss how the limitations might affect your findings, it’s all good.
Research Delimitations
Alright, now that we’ve unpacked the limitations, let’s move on to the delimitations .
Research delimitations are similar to limitations in that they also “ limit ” the study, but their focus is entirely different. Specifically, the delimitations of a study refer to the scope of the research aims and research questions . In other words, delimitations reflect the choices you, as the researcher, intentionally make in terms of what you will and won’t try to achieve with your study. In other words, what your research aims and research questions will and won’t include.
As we’ve spoken about many times before, it’s important to have a tight, narrow focus for your research, so that you can dive deeply into your topic, apply your energy to one specific area and develop meaningful insights. If you have an overly broad scope or unfocused topic, your research will often pull in multiple, even opposing directions, and you’ll just land up with a muddy mess of findings .
So, the delimitations section is where you’ll clearly state what your research aims and research questions will focus on – and just as importantly, what they will exclude . For example, you might investigate a widespread phenomenon, but choose to focus your study on a specific age group, ethnicity or gender. Similarly, your study may focus exclusively on one country, city or even organization. As long as the scope is well justified (in other words, it represents a novel, valuable research topic), this is perfectly acceptable – in fact, it’s essential. Remember, focus is your friend.
Need a helping hand?
Conclusion: Limitations vs Delimitations
Ok, so let’s recap.
Research limitations and research delimitations are related in that they both refer to “limits” within a study. But, they are distinctly different. Limitations reflect the shortcomings of your study, based on practical or theoretical constraints that you faced.
Contrasted to that, delimitations reflect the choices that you made in terms of the focus and scope of your research aims and research questions. If you want to learn more about research aims and questions, you can check out this video post , where we unpack those concepts in detail.
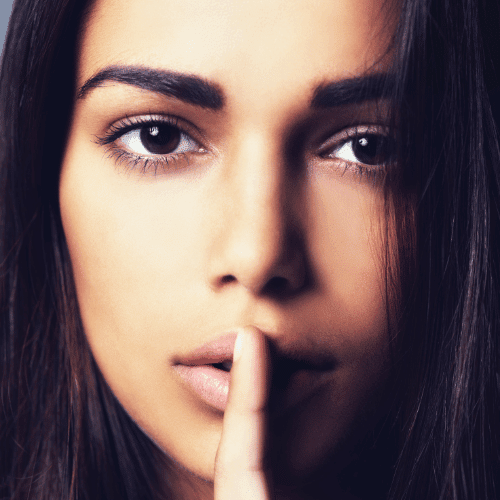
Psst... there’s more!
This post was based on one of our popular Research Bootcamps . If you're working on a research project, you'll definitely want to check this out ...
18 Comments
Good clarification of ideas on how a researcher ought to do during Process of choice
Thank you so much for this very simple but explicit explanation on limitation and delimitation. It has so helped me to develop my masters proposal. hope to recieve more from your site as time progresses
Thank you for this explanation – very clear.
Thanks for the explanation, really got it well.
This website is really helpful for my masters proposal
Thank you very much for helping to explain these two terms
I spent almost the whole day trying to figure out the differences
when I came across your notes everything became very clear
thanks for the clearly outlined explanation on the two terms, limitation and delimitation.
Very helpful Many thanks 🙏
Excellent it resolved my conflict .
I would like you to assist me please. If in my Research, I interviewed some participants and I submitted Questionnaires to other participants to answered to the questions, in the same organization, Is this a Qualitative methodology , a Quantitative Methodology or is it a Mixture Methodology I have used in my research? Please help me
How do I cite this article in APA format
Really so great ,finally have understood it’s difference now
Getting more clear regarding Limitations and Delimitation and concepts
I really appreciate your apt and precise explanation of the two concepts namely ; Limitations and Delimitations.
This is a good sources of research information for learners.
thank you for this, very helpful to researchers
Very good explained
Great and clear explanation, after a long confusion period on the two words, i can now explain to someone with ease.
Submit a Comment Cancel reply
Your email address will not be published. Required fields are marked *
Save my name, email, and website in this browser for the next time I comment.
- Print Friendly
Training videos | Faqs

Limitations in Research – A Simplified Guide with Examples
Limitations are flaws and shortcomings of your study. It is very important that you discuss the limitations of your study in the discussion section of your research paper. In this blog, we provide tips for presenting study limitations in your paper along with some real-world examples.
1. Should I Report the Limitations of My Work?
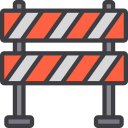
Most studies will have some form of limitation. So be honest and don’t hide your limitations. You have to tell your readers how your limitations might influence the outcomes and conclusions of your research. In reality, your readers and reviewers will be impressed with your paper if you are upfront about your limitations.
2. Examples
Let’s look at some examples. We have selected a variety of examples from different research topics.
2.1. Limitations Example 1
Following example is from a Medical research paper.
✔ The authors talk about the limitations and emphasis the importance of reconfirming the findings in a much larger study Study design and small sample size are important limitations. This could have led to an overestimation of the effect. Future research should reconfirm these findings by conducting larger-scale studies. _ Limitation s _ How it might affect the results? _ How to fix the limitation?
The authors are saying that the main limitations of the study are the small sample size and weak study design. Then they explain how this might have affected their results. They are saying that it is possible that they are overestimating the actual effect they are measuring. Then finally they are telling the readers that more studies with larger sample sizes should be conducted to reconfirm the findings.
As you can see, the authors are clearly explaining three things here: (1) What is the limitation? (2) How it might affect the study outcomes? and (3) What should be done to address the limitation?
2.2. Limitations Example 2
Following example is from an Engineering research paper.
✔ The authors are acknowledging the limitations and warning readers against generalizing the research findings However, some study limitations should be acknowledged. The experiments do not fully consider the problems that can appear in real situations. Hence, caution should be taken with generalizing the findings and applying them to real-life situations. _ Acknowledging limitations _ Explaining the limitation _ How it might affect the results?
The authors acknowledge that their study has some limitations. Then they explain what the limitations are. They are saying that their experiments do not consider all problems that might occur in real-life situations. Then they explain how this might affect their research outcomes. They are saying that readers should be careful when generalizing the results to practical real-world situations, because there is a possibility that the methods might fail.
2.3. Limitations Example 3
It is important to remember not to end your paper with limitations. Finish your paper on a positive note by telling your readers about the benefits of your research and possible future directions. In the following example, right after listing the limitations, the authors proceed to talk about the positive aspects of the work.
✔ The authors finish their paper on a positive note by talking about the benefits of their work and possible future work With this limited study, it is not known whether this finding can be applied to all clinical scenarios. Notwithstanding these limitations, this study has proven that Ultrasound can potentially serve as a more efficient alternative to X-rays in diagnosis. Future directions include studying the effects of different ultrasound pulsing schemes on pain relief. Another interesting direction would be to consider applications in nonhuman primates. _ Limitations _ Benefits of the work _ Possible future directions
The authors are saying that their experiments were somewhat limited and are not sure if their findings apply to the wider clinical practice. Then the authors highlight the benefits of their research. The authors say that their study has proven that ultrasound can be used instead of X-rays for diagnosis of certain types of diseases. Then they are explaining how future research can extend this work further. The authors are suggesting that it will be interesting to explore if ultrasound can be used for the treatment of chronic pain. And they are also suggesting that future studies can explore treating certain types of animal diseases with ultrasound. This is a very good example of how to finish the discussion section of your paper on a positive note.
Limitations are a vital component of the discussion section of your research paper. Remember, every study has limitations. There is no such thing as a perfect study. One of the major mistakes beginner writers make is hiding the limitations in the paper. Don’t do this, reviewers will reject your paper. Explain clearly how your limitations might have impacted your results, and provide ideas to mitigate them in the future. For further reading, please refer to our blogs on handling negative results and advanced tactics to address study limitations.
If you have any questions, please drop a comment below, and we will answer as soon as possible. We also recommend you to refer to our other blogs on academic writing tools , academic writing resources , academic writing phrases and research paper examples which are relevant to the topic discussed in this blog.
Similar Posts
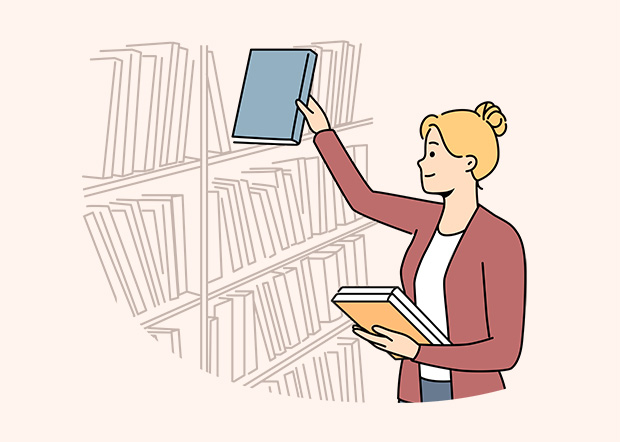
Literature Review Examples and Writing Tips
In this blog, we will go through many literature review examples and understand different ways to present past literature in your paper.
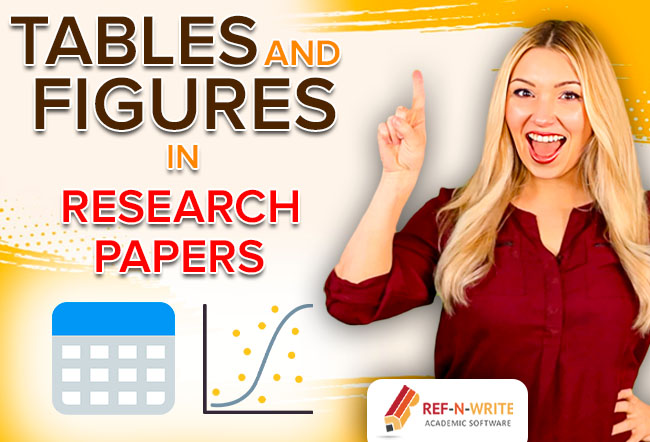
Figures and Tables in Research Papers – Tips and Examples
In this blog, we will look at best practices for presenting tables and figures in your research paper.
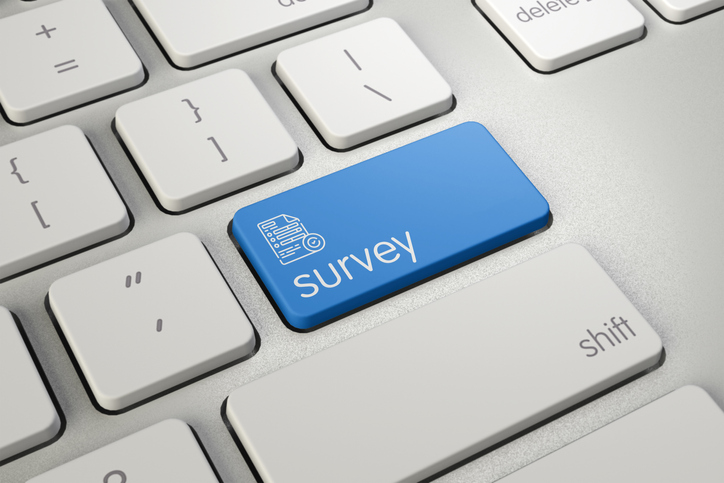
Writing a Questionnaire Survey Research Paper – Example & Format
In this blog, we will explain how to write a survey questionnaire paper and discuss all the important points to consider while writing the research paper.
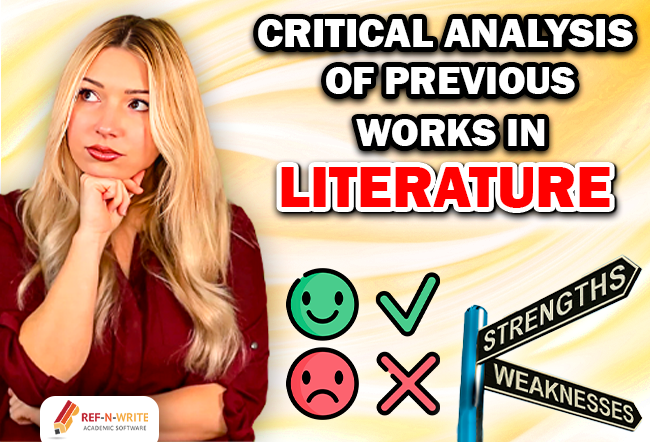
Critical Literature Review : How to Critique a Research Article?
In this blog, we will look at how to use constructive language when critiquing other’s work in your research paper.

How to Reuse a Method from a Previous Paper?
In this blog, we will look at how to replicate someone’s methodology in your paper.
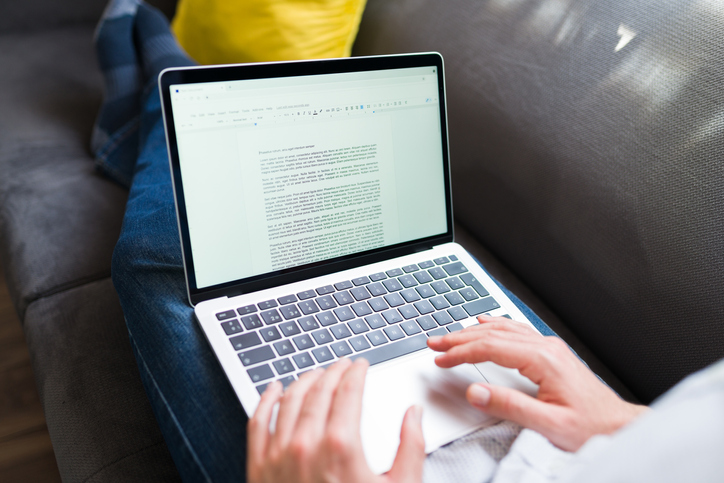
Research Paper Structure – Main Sections and Parts of a Research Paper
In this blog, we explain various topics and sub-topics to be included under each section of a research paper via word cloud diagrams.
Leave a Reply Cancel reply
Your email address will not be published. Required fields are marked *
Save my name, email, and website in this browser for the next time I comment.
- 10 Share Facebook
- 1 Share Twitter
- 0 Share LinkedIn
- 0 Share Email
Educational resources and simple solutions for your research journey
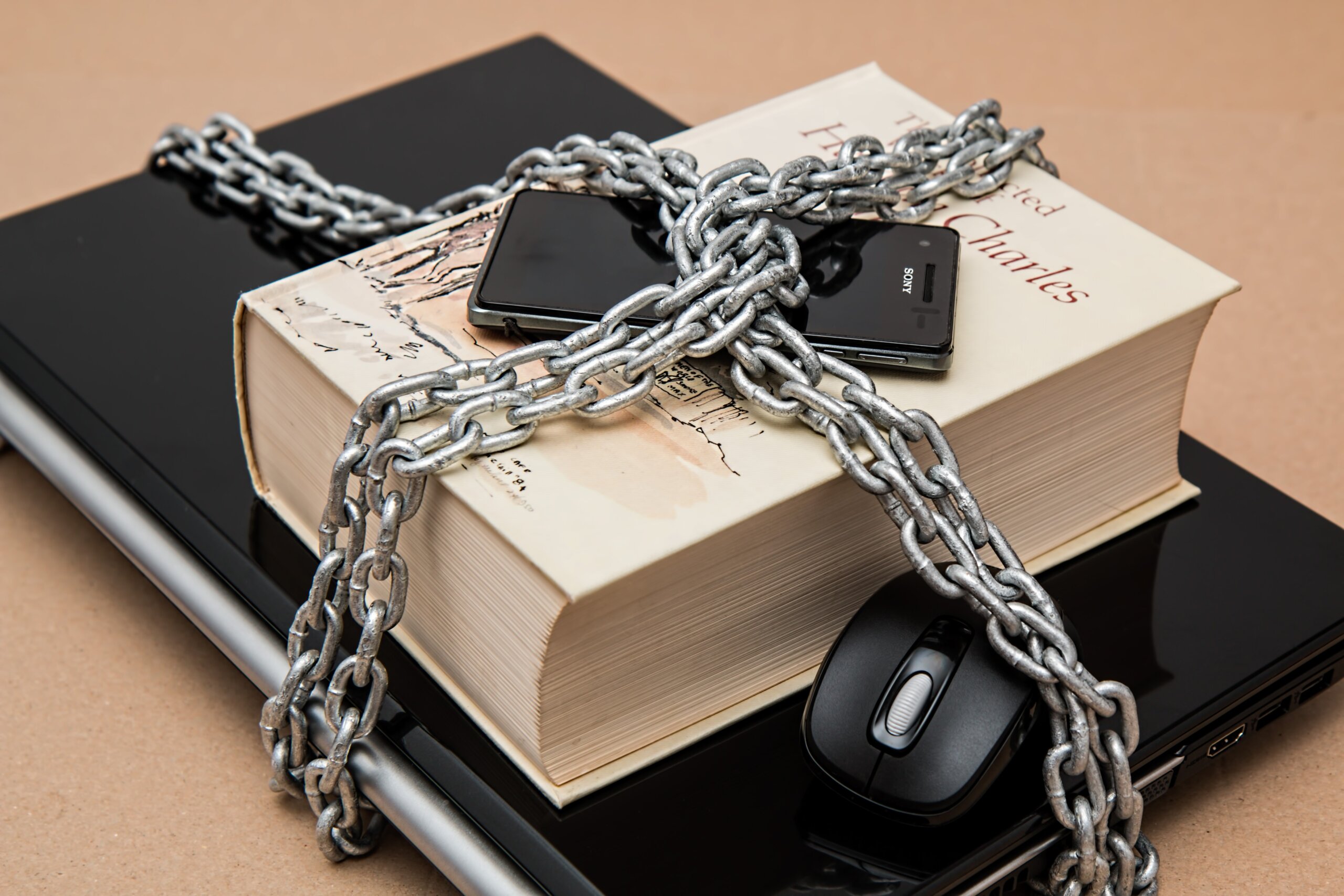
How to Present the Limitations of a Study in Research?
The limitations of the study convey to the reader how and under which conditions your study results will be evaluated. Scientific research involves investigating research topics, both known and unknown, which inherently includes an element of risk. The risk could arise due to human errors, barriers to data gathering, limited availability of resources, and researcher bias. Researchers are encouraged to discuss the limitations of their research to enhance the process of research, as well as to allow readers to gain an understanding of the study’s framework and value.
Limitations of the research are the constraints placed on the ability to generalize from the results and to further describe applications to practice. It is related to the utility value of the findings based on how you initially chose to design the study, the method used to establish internal and external validity, or the result of unanticipated challenges that emerged during the study. Knowing about these limitations and their impact can explain how the limitations of your study can affect the conclusions and thoughts drawn from your research. 1
Table of Contents
What are the limitations of a study
Researchers are probably cautious to acknowledge what the limitations of the research can be for fear of undermining the validity of the research findings. No research can be faultless or cover all possible conditions. These limitations of your research appear probably due to constraints on methodology or research design and influence the interpretation of your research’s ultimate findings. 2 These are limitations on the generalization and usability of findings that emerge from the design of the research and/or the method employed to ensure validity internally and externally. But such limitations of the study can impact the whole study or research paper. However, most researchers prefer not to discuss the different types of limitations in research for fear of decreasing the value of their paper amongst the reviewers or readers.
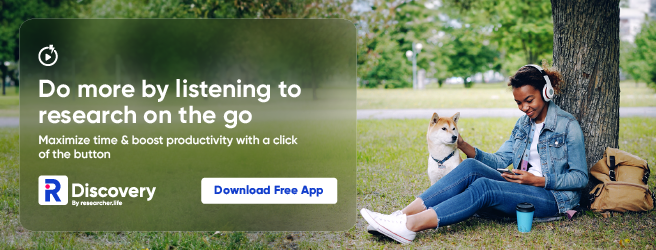
Importance of limitations of a study
Writing the limitations of the research papers is often assumed to require lots of effort. However, identifying the limitations of the study can help structure the research better. Therefore, do not underestimate the importance of research study limitations. 3
- Opportunity to make suggestions for further research. Suggestions for future research and avenues for further exploration can be developed based on the limitations of the study.
- Opportunity to demonstrate critical thinking. A key objective of the research process is to discover new knowledge while questioning existing assumptions and exploring what is new in the particular field. Describing the limitation of the research shows that you have critically thought about the research problem, reviewed relevant literature, and correctly assessed the methods chosen for studying the problem.
- Demonstrate Subjective learning process. Writing limitations of the research helps to critically evaluate the impact of the said limitations, assess the strength of the research, and consider alternative explanations or interpretations. Subjective evaluation contributes to a more complex and comprehensive knowledge of the issue under study.
Why should I include limitations of research in my paper
All studies have limitations to some extent. Including limitations of the study in your paper demonstrates the researchers’ comprehensive and holistic understanding of the research process and topic. The major advantages are the following:
- Understand the study conditions and challenges encountered . It establishes a complete and potentially logical depiction of the research. The boundaries of the study can be established, and realistic expectations for the findings can be set. They can also help to clarify what the study is not intended to address.
- Improve the quality and validity of the research findings. Mentioning limitations of the research creates opportunities for the original author and other researchers to undertake future studies to improve the research outcomes.
- Transparency and accountability. Including limitations of the research helps maintain mutual integrity and promote further progress in similar studies.
- Identify potential bias sources. Identifying the limitations of the study can help researchers identify potential sources of bias in their research design, data collection, or analysis. This can help to improve the validity and reliability of the findings.
Where do I need to add the limitations of the study in my paper
The limitations of your research can be stated at the beginning of the discussion section, which allows the reader to comprehend the limitations of the study prior to reading the rest of your findings or at the end of the discussion section as an acknowledgment of the need for further research.
Types of limitations in research
There are different types of limitations in research that researchers may encounter. These are listed below:
- Research Design Limitations : Restrictions on your research or available procedures may affect the research outputs. If the research goals and objectives are too broad, explain how they should be narrowed down to enhance the focus of your study. If there was a selection bias in your sample, explain how this may affect the generalizability of your findings. This can help readers understand the limitations of the study in terms of their impact on the overall validity of your research.
- Impact Limitations : Your study might be limited by a strong regional-, national-, or species-based impact or population- or experimental-specific impact. These inherent limitations on impact affect the extendibility and generalizability of the findings.
- Data or statistical limitations : Data or statistical limitations in research are extremely common in experimental (such as medicine, physics, and chemistry) or field-based (such as ecology and qualitative clinical research) studies. Sometimes, it is either extremely difficult to acquire sufficient data or gain access to the data. These limitations of the research might also be the result of your study’s design and might result in an incomplete conclusion to your research.

Limitations of study examples
All possible limitations of the study cannot be included in the discussion section of the research paper or dissertation. It will vary greatly depending on the type and nature of the study. These include types of research limitations that are related to methodology and the research process and that of the researcher as well that you need to describe and discuss how they possibly impacted your results.
Common methodological limitations of the study
Limitations of research due to methodological problems are addressed by identifying the potential problem and suggesting ways in which this should have been addressed. Some potential methodological limitations of the study are as follows. 1
- Sample size: The sample size 4 is dictated by the type of research problem investigated. If the sample size is too small, finding a significant relationship from the data will be difficult, as statistical tests require a large sample size to ensure a representative population distribution and generalize the study findings.
- Lack of available/reliable data: A lack of available/reliable data will limit the scope of your analysis and the size of your sample or present obstacles in finding a trend or meaningful relationship. So, when writing about the limitations of the study, give convincing reasons why you feel data is absent or untrustworthy and highlight the necessity for a future study focused on developing a new data-gathering strategy.
- Lack of prior research studies: Citing prior research studies is required to help understand the research problem being investigated. If there is little or no prior research, an exploratory rather than an explanatory research design will be required. Also, discovering the limitations of the study presents an opportunity to identify gaps in the literature and describe the need for additional study.
- Measure used to collect the data: Sometimes, the data gathered will be insufficient to conduct a thorough analysis of the results. A limitation of the study example, for instance, is identifying in retrospect that a specific question could have helped address a particular issue that emerged during data analysis. You can acknowledge the limitation of the research by stating the need to revise the specific method for gathering data in the future.
- Self-reported data: Self-reported data cannot be independently verified and can contain several potential bias sources, such as selective memory, attribution, and exaggeration. These biases become apparent if they are incongruent with data from other sources.
General limitations of researchers
Limitations related to the researcher can also influence the study outcomes. These should be addressed, and related remedies should be proposed.
- Limited access to data : If your study requires access to people, organizations, data, or documents whose access is denied or limited, the reasons need to be described. An additional explanation stating why this limitation of research did not prevent you from following through on your study is also needed.
- Time constraints : Researchers might also face challenges in meeting research deadlines due to a lack of timely participant availability or funds, among others. The impacts of time constraints must be acknowledged by mentioning the need for a future study addressing this research problem.
- Conflicts due to biased views and personal issues : Differences in culture or personal views can contribute to researcher bias, as they focus only on the results and data that support their main arguments. To avoid this, pay attention to the problem statement and data gathering.
Steps for structuring the limitations section
Limitations are an inherent part of any research study. Issues may vary, ranging from sampling and literature review to methodology and bias. However, there is a structure for identifying these elements, discussing them, and offering insight or alternatives on how the limitations of the study can be mitigated. This enhances the process of the research and helps readers gain a comprehensive understanding of a study’s conditions.
- Identify the research constraints : Identify those limitations having the greatest impact on the quality of the research findings and your ability to effectively answer your research questions and/or hypotheses. These include sample size, selection bias, measurement error, or other issues affecting the validity and reliability of your research.
- Describe their impact on your research : Reflect on the nature of the identified limitations and justify the choices made during the research to identify the impact of the study’s limitations on the research outcomes. Explanations can be offered if needed, but without being defensive or exaggerating them. Provide context for the limitations of your research to understand them in a broader context. Any specific limitations due to real-world considerations need to be pointed out critically rather than justifying them as done by some other author group or groups.
- Mention the opportunity for future investigations : Suggest ways to overcome the limitations of the present study through future research. This can help readers understand how the research fits into the broader context and offer a roadmap for future studies.
Frequently Asked Questions
- Should I mention all the limitations of my study in the research report?
Restrict limitations to what is pertinent to the research question under investigation. The specific limitations you include will depend on the nature of the study, the research question investigated, and the data collected.
- Can the limitations of a study affect its credibility?
Stating the limitations of the research is considered favorable by editors and peer reviewers. Connecting your study’s limitations with future possible research can help increase the focus of unanswered questions in this area. In addition, admitting limitations openly and validating that they do not affect the main findings of the study increases the credibility of your study. However, if you determine that your study is seriously flawed, explain ways to successfully overcome such flaws in a future study. For example, if your study fails to acquire critical data, consider reframing the research question as an exploratory study to lay the groundwork for more complete research in the future.
- How can I mitigate the limitations of my study?
Strategies to minimize limitations of the research should focus on convincing reviewers and readers that the limitations do not affect the conclusions of the study by showing that the methods are appropriate and that the logic is sound. Here are some steps to follow to achieve this:
- Use data that are valid.
- Use methods that are appropriate and sound logic to draw inferences.
- Use adequate statistical methods for drawing inferences from the data that studies with similar limitations have been published before.
Admit limitations openly and, at the same time, show how they do not affect the main conclusions of the study.
- Can the limitations of a study impact its publication chances?
Limitations in your research can arise owing to restrictions in methodology or research design. Although this could impact your chances of publishing your research paper, it is critical to explain your study’s limitations to your intended audience. For example, it can explain how your study constraints may impact the results and views generated from your investigation. It also shows that you have researched the flaws of your study and have a thorough understanding of the subject.
- How can limitations in research be used for future studies?
The limitations of a study give you an opportunity to offer suggestions for further research. Your study’s limitations, including problems experienced during the study and the additional study perspectives developed, are a great opportunity to take on a new challenge and help advance knowledge in a particular field.
References:
- Brutus, S., Aguinis, H., & Wassmer, U. (2013). Self-reported limitations and future directions in scholarly reports: Analysis and recommendations. Journal of Management , 39 (1), 48-75.
- Ioannidis, J. P. (2007). Limitations are not properly acknowledged in the scientific literature. Journal of Clinical Epidemiology , 60 (4), 324-329.
- Price, J. H., & Murnan, J. (2004). Research limitations and the necessity of reporting them. American Journal of Health Education , 35 (2), 66.
- Boddy, C. R. (2016). Sample size for qualitative research. Qualitative Market Research: An International Journal , 19 (4), 426-432.
R Discovery is a literature search and research reading platform that accelerates your research discovery journey by keeping you updated on the latest, most relevant scholarly content. With 250M+ research articles sourced from trusted aggregators like CrossRef, Unpaywall, PubMed, PubMed Central, Open Alex and top publishing houses like Springer Nature, JAMA, IOP, Taylor & Francis, NEJM, BMJ, Karger, SAGE, Emerald Publishing and more, R Discovery puts a world of research at your fingertips.
Try R Discovery Prime FREE for 1 week or upgrade at just US$72 a year to access premium features that let you listen to research on the go, read in your language, collaborate with peers, auto sync with reference managers, and much more. Choose a simpler, smarter way to find and read research – Download the app and start your free 7-day trial today !
Related Posts

What is IMRaD Format in Research?

What is a Review Article? How to Write it?
- Link to facebook
- Link to linkedin
- Link to twitter
- Link to youtube
- Writing Tips
How to Identify Limitations in Research
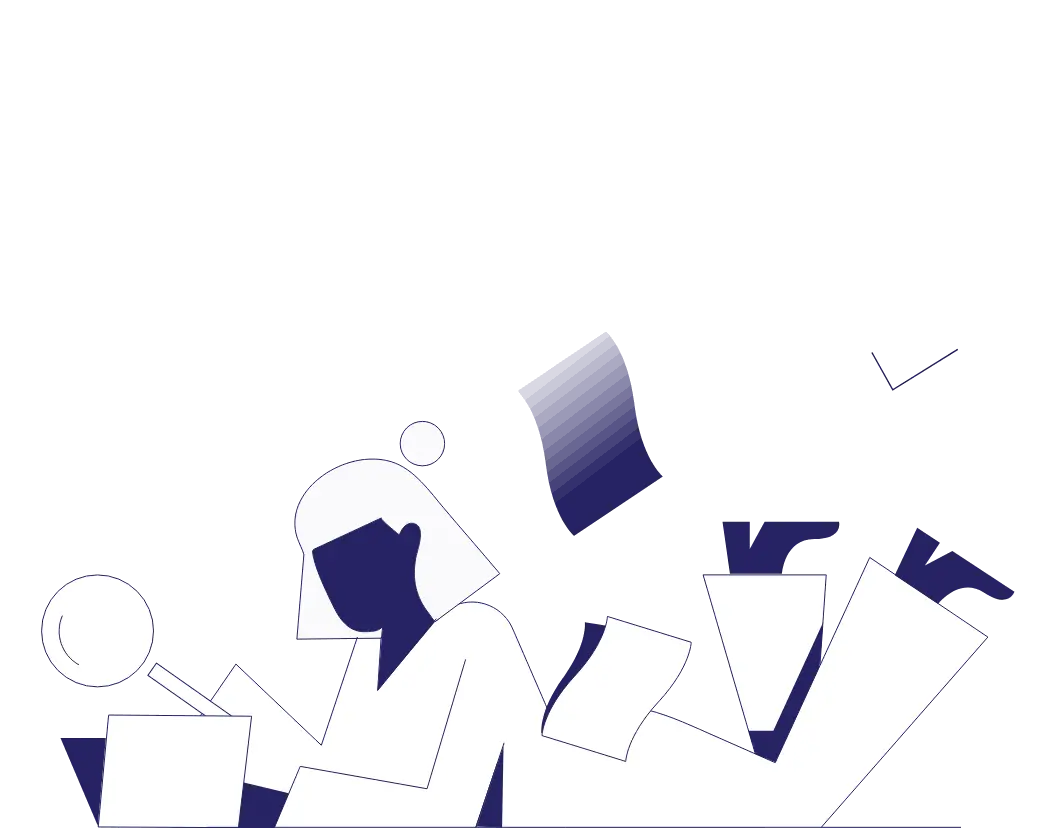
4-minute read
- 7th March 2022
Whether you’re a veteran researcher with years of experience under your belt or a novice to the field that’s feeling overwhelmed with where to start, you must understand that every study has its limitations. These are restrictions that arise from the study’s design, or the methodology implemented during the testing phase. Unfortunately, research limitations will always exist due to the subjective nature of testing a hypothesis. We’ve compiled some helpful information below on how to identify and accept research limitations and use them to your advantage. Essentially, we’ll show you how to make lemonade (a brilliant piece of academic work ) from the lemons you receive (the constraints your study reveals).
Research Limitations
So, let’s dive straight in, shall we? It’s always beneficial (and good practice) to disclose your research limitations . A common thought is that divulging these shortcomings will undermine the credibility and quality of your research. However, this is certainly not the case— stating the facts upfront not only reinforces your reputation as a researcher but also lets the assessor or reader know that you’re confident and transparent about the results and relevance of your study, despite these constraints.
Additionally, it creates a gap for more research opportunities, where you can analyze these limitations and determine how to incorporate or address them in a new batch of tests or create a new hypothesis altogether. Another bonus is that it helps readers to understand the optimum conditions for how to apply the results of your testing. This is a win-win, making for a far more persuasive research paper .
Now that you know why you should clarify your research limitations, let’s focus on which ones take precedence and should be disclosed. Any given research project can be vulnerable to various hindrances, so how do you identify them and single out the most significant ones to discuss? Well, that depends entirely on the nature of your study. You’ll need to comb through your research approach, methodology, testing processes, and expected results to identify the type of limitations your study may be exposed to. It’s worth noting that this understanding can only offer a broad idea of the possible restrictions you’ll face and may potentially change throughout the study.
We’ve compiled a list of the most common types of research limitations that you may encounter so you can adequately prepare for them and remain vigilant during each stage of your study.
Sample Size:
It’s critical that you choose a sample size that accurately represents the population you wish to test your theory on. If a sample is too small, the results cannot reliably be generalized across a large population.
Methodology:
The method you choose before you commence testing might seem effective in theory, but too many stumbling blocks during the testing phase can influence the accuracy and reliability of the results.
Find this useful?
Subscribe to our newsletter and get writing tips from our editors straight to your inbox.
Collection of Data:
The methods you utilize to obtain your research—surveys, emails, in-person interviews, phone calls—will directly influence the type of results your study yields.
Age of Data:
The nature of the information—and how far back it goes—affects the type of assumptions you can make. Extrapolating older data for a current hypothesis can significantly change the outcome of your testing.
Time Constraints:
Working within the deadline of when you need to submit your findings will determine the extent of your research and testing and, therefore, can heavily impact your results. Limited time frames for testing might mean not achieving the scope of results you were originally looking for.
Limited Budget:
Your study may require equipment and other resources that can become extremely costly. Budget constraints may mean you cannot acquire advanced software, programs, or travel to multiple destinations to interview participants. All of these factors can substantially influence your results.
So, now that you know how to determine your research limitations and the types you might experience, where should you document it? It’s commonly disclosed at the beginning of your discussion section , so the reader understands the shortcomings of your study before digging into the juicy bit—your findings. Alternatively, you can detail the constraints faced at the end of the discussion section to emphasize the requirements for the completion of further studies.
We hope this post will prepare you for some of the pitfalls you may encounter when conducting and documenting your research. Once you have a first draft ready, consider submitting a free sample to us for proofreading to ensure that your writing is concise and error-free and your results—despite their limitations— shine through.
Share this article:
Post A New Comment
Got content that needs a quick turnaround? Let us polish your work. Explore our editorial business services.
5-minute read
Free Email Newsletter Template (2024)
Promoting a brand means sharing valuable insights to connect more deeply with your audience, and...
6-minute read
How to Write a Nonprofit Grant Proposal
If you’re seeking funding to support your charitable endeavors as a nonprofit organization, you’ll need...
9-minute read
How to Use Infographics to Boost Your Presentation
Is your content getting noticed? Capturing and maintaining an audience’s attention is a challenge when...
8-minute read
Why Interactive PDFs Are Better for Engagement
Are you looking to enhance engagement and captivate your audience through your professional documents? Interactive...
7-minute read
Seven Key Strategies for Voice Search Optimization
Voice search optimization is rapidly shaping the digital landscape, requiring content professionals to adapt their...
Five Creative Ways to Showcase Your Digital Portfolio
Are you a creative freelancer looking to make a lasting impression on potential clients or...
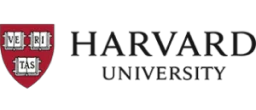
Make sure your writing is the best it can be with our expert English proofreading and editing.
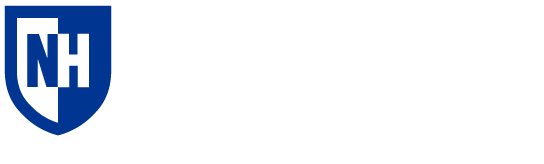
CPS Online Graduate Studies Research Paper (UNH Manchester Library): Limitations of the Study
- Overview of the Research Process for Capstone Projects
- Types of Research Design
- Selecting a Research Problem
- The Title of Your Research Paper
- Before You Begin Writing
- 7 Parts of the Research Paper
- Background Information
- Quanitative and Qualitative Methods
- Qualitative Methods
- Quanitative Methods
- Resources to Help You With the Literature Review
- Non-Textual Elements
Limitations of the Study
- Format of Capstone Research Projects at GSC
- Editing and Proofreading Your Paper
- Acknowledgements
- UNH Scholar's Repository
The limitations of the study are those characteristics of design or methodology that impacted or influenced the interpretation of the findings from your research. They are the constraints on generalizability, applications to practice, and/or utility of findings that are the result of the ways in which you initially chose to design the study and/or the method used to establish internal and external validity.
Price, James H. and Judy Murnan. “Research Limitations and the Necessity of Reporting Them.” American Journal of Health Education 35 (2004): 66-67.
Always acknowledge a study's limitations. It is far better that you identify and acknowledge your study’s limitations than to have them pointed out by your professor and be graded down because you appear to have ignored them.
Keep in mind that acknowledgement of a study's limitations is an opportunity to make suggestions for further research. If you do connect your study's limitations to suggestions for further research, be sure to explain the ways in which these unanswered questions may become more focused because of your study.
Acknowledgement of a study's limitations also provides you with an opportunity to demonstrate that you have thought critically about the research problem, understood the relevant literature published about it, and correctly assessed the methods chosen for studying the problem. A key objective of the research process is not only discovering new knowledge but to also confront assumptions and explore what we don't know.
Claiming limitations is a subjective process because you must evaluate the impact of those limitations . Don't just list key weaknesses and the magnitude of a study's limitations. To do so diminishes the validity of your research because it leaves the reader wondering whether, or in what ways, limitation(s) in your study may have impacted the results and conclusions. Limitations require a critical, overall appraisal and interpretation of their impact. You should answer the question: do these problems with errors, methods, validity, etc. eventually matter and, if so, to what extent?
Price, James H. and Judy Murnan. “Research Limitations and the Necessity of Reporting Them.” American Journal of Health Education 35 (2004): 66-67; Structure: How to Structure the Research Limitations Section of Your Dissertation . Dissertations and Theses: An Online Textbook. Laerd.com.
Descriptions of Possible Limitations
All studies have limitations . However, it is important that you restrict your discussion to limitations related to the research problem under investigation. For example, if a meta-analysis of existing literature is not a stated purpose of your research, it should not be discussed as a limitation. Do not apologize for not addressing issues that you did not promise to investigate in the introduction of your paper.
Here are examples of limitations related to methodology and the research process you may need to describe and to discuss how they possibly impacted your results. Descriptions of limitations should be stated in the past tense because they were discovered after you completed your research.
Possible Methodological Limitations
- Sample size -- the number of the units of analysis you use in your study is dictated by the type of research problem you are investigating. Note that, if your sample size is too small, it will be difficult to find significant relationships from the data, as statistical tests normally require a larger sample size to ensure a representative distribution of the population and to be considered representative of groups of people to whom results will be generalized or transferred. Note that sample size is less relevant in qualitative research.
- Lack of available and/or reliable data -- a lack of data or of reliable data will likely require you to limit the scope of your analysis, the size of your sample, or it can be a significant obstacle in finding a trend and a meaningful relationship. You need to not only describe these limitations but to offer reasons why you believe data is missing or is unreliable. However, don’t just throw up your hands in frustration; use this as an opportunity to describe the need for future research.
- Lack of prior research studies on the topic -- citing prior research studies forms the basis of your literature review and helps lay a foundation for understanding the research problem you are investigating. Depending on the currency or scope of your research topic, there may be little, if any, prior research on your topic. Before assuming this to be true, though, consult with a librarian. In cases when a librarian has confirmed that there is no prior research, you may be required to develop an entirely new research typology [for example, using an exploratory rather than an explanatory research design]. Note again that discovering a limitation can serve as an important opportunity to identify new gaps in the literature and to describe the need for further research.
- Measure used to collect the data -- sometimes it is the case that, after completing your interpretation of the findings, you discover that the way in which you gathered data inhibited your ability to conduct a thorough analysis of the results. For example, you regret not including a specific question in a survey that, in retrospect, could have helped address a particular issue that emerged later in the study. Acknowledge the deficiency by stating a need for future researchers to revise the specific method for gathering data.
- Self-reported data -- whether you are relying on pre-existing data or you are conducting a qualitative research study and gathering the data yourself, self-reported data is limited by the fact that it rarely can be independently verified. In other words, you have to take what people say, whether in interviews, focus groups, or on questionnaires, at face value. However, self-reported data can contain several potential sources of bias that you should be alert to and note as limitations. These biases become apparent if they are incongruent with data from other sources. These are: (1) selective memory [remembering or not remembering experiences or events that occurred at some point in the past]; (2) telescoping [recalling events that occurred at one time as if they occurred at another time]; (3) attribution [the act of attributing positive events and outcomes to one's own agency but attributing negative events and outcomes to external forces]; and, (4) exaggeration [the act of representing outcomes or embellishing events as more significant than is actually suggested from other data].
Possible Limitations of the Researcher
- Access -- if your study depends on having access to people, organizations, or documents and, for whatever reason, access is denied or limited in some way, the reasons for this need to be described.
- Longitudinal effects -- unlike your professor, who can literally devote years [even a lifetime] to studying a single topic, the time available to investigate a research problem and to measure change or stability over time is pretty much constrained by the due date of your assignment. Be sure to choose a research problem that does not require an excessive amount of time to complete the literature review, apply the methodology, and gather and interpret the results. If you're unsure whether you can complete your research within the confines of the assignment's due date, talk to your professor.
- Cultural and other type of bias -- we all have biases, whether we are conscience of them or not. Bias is when a person, place, or thing is viewed or shown in a consistently inaccurate way. Bias is usually negative, though one can have a positive bias as well, especially if that bias reflects your reliance on research that only support for your hypothesis. When proof-reading your paper, be especially critical in reviewing how you have stated a problem, selected the data to be studied, what may have been omitted, the manner in which you have ordered events, people, or places, how you have chosen to represent a person, place, or thing, to name a phenomenon, or to use possible words with a positive or negative connotation.
NOTE: If you detect bias in prior research, it must be acknowledged and you should explain what measures were taken to avoid perpetuating that bias.
- Fluency in a language -- if your research focuses on measuring the perceived value of after-school tutoring among Mexican-American ESL [English as a Second Language] students, for example, and you are not fluent in Spanish, you are limited in being able to read and interpret Spanish language research studies on the topic. This deficiency should be acknowledged.
Aguinis, Hermam and Jeffrey R. Edwards. “Methodological Wishes for the Next Decade and How to Make Wishes Come True.” Journal of Management Studies 51 (January 2014): 143-174; Brutus, Stéphane et al. "Self-Reported Limitations and Future Directions in Scholarly Reports: Analysis and Recommendations." Journal of Management 39 (January 2013): 48-75; Senunyeme, Emmanuel K. Business Research Methods . Powerpoint Presentation. Regent University of Science and Technology; ter Riet, Gerben et al. “All That Glitters Isn't Gold: A Survey on Acknowledgment of Limitations in Biomedical Studies.” PLOS One 8 (November 2013): 1-6.
Structure and Writing Style
Information about the limitations of your study are generally placed either at the beginning of the discussion section of your paper so the reader knows and understands the limitations before reading the rest of your analysis of the findings, or, the limitations are outlined at the conclusion of the discussion section as an acknowledgement of the need for further study. Statements about a study's limitations should not be buried in the body [middle] of the discussion section unless a limitation is specific to something covered in that part of the paper. If this is the case, though, the limitation should be reiterated at the conclusion of the section. If you determine that your study is seriously flawed due to important limitations, such as, an inability to acquire critical data, consider reframing it as an exploratory study intended to lay the groundwork for a more complete research study in the future. Be sure, though, to specifically explain the ways that these flaws can be successfully overcome in a new study. But, do not use this as an excuse for not developing a thorough research paper! Review the tab in this guide for developing a research topic. If serious limitations exist, it generally indicates a likelihood that your research problem is too narrowly defined or that the issue or event under study is too recent and, thus, very little research has been written about it. If serious limitations do emerge, consult with your professor about possible ways to overcome them or how to revise your study. When discussing the limitations of your research, be sure to: Describe each limitation in detailed but concise terms; Explain why each limitation exists; Provide the reasons why each limitation could not be overcome using the method(s) chosen to acquire or gather the data [cite to other studies that had similar problems when possible]; Assess the impact of each limitation in relation to the overall findings and conclusions of your study; and, If appropriate, describe how these limitations could point to the need for further research. Remember that the method you chose may be the source of a significant limitation that has emerged during your interpretation of the results [for example, you didn't interview a group of people that you later wish you had]. If this is the case, don't panic. Acknowledge it, and explain how applying a different or more robust methodology might address the research problem more effectively in a future study. A underlying goal of scholarly research is not only to show what works, but to demonstrate what doesn't work or what needs further clarification. Aguinis, Hermam and Jeffrey R. Edwards. “Methodological Wishes for the Next Decade and How to Make Wishes Come True.” Journal of Management Studies 51 (January 2014): 143-174; Brutus, Stéphane et al. "Self-Reported Limitations and Future Directions in Scholarly Reports: Analysis and Recommendations." Journal of Management 39 (January 2013): 48-75; Ioannidis, John P.A. "Limitations are not Properly Acknowledged in the Scientific Literature." Journal of Clinical Epidemiology 60 (2007): 324-329; Pasek, Josh. Writing the Empirical Social Science Research Paper: A Guide for the Perplexed. January 24, 2012. Academia.edu; Structure: How to Structure the Research Limitations Section of Your Dissertation. Dissertations and Theses: An Online Textbook. Laerd.com; What Is an Academic Paper? Institute for Writing Rhetoric. Dartmouth College; Writing the Experimental Report: Methods, Results, and Discussion. The Writing Lab and The OWL. Purdue University.
Information about the limitations of your study are generally placed either at the beginning of the discussion section of your paper so the reader knows and understands the limitations before reading the rest of your analysis of the findings, or, the limitations are outlined at the conclusion of the discussion section as an acknowledgement of the need for further study. Statements about a study's limitations should not be buried in the body [middle] of the discussion section unless a limitation is specific to something covered in that part of the paper. If this is the case, though, the limitation should be reiterated at the conclusion of the section.
If you determine that your study is seriously flawed due to important limitations , such as, an inability to acquire critical data, consider reframing it as an exploratory study intended to lay the groundwork for a more complete research study in the future. Be sure, though, to specifically explain the ways that these flaws can be successfully overcome in a new study.
But, do not use this as an excuse for not developing a thorough research paper! Review the tab in this guide for developing a research topic . If serious limitations exist, it generally indicates a likelihood that your research problem is too narrowly defined or that the issue or event under study is too recent and, thus, very little research has been written about it. If serious limitations do emerge, consult with your professor about possible ways to overcome them or how to revise your study.
When discussing the limitations of your research, be sure to:
- Describe each limitation in detailed but concise terms;
- Explain why each limitation exists;
- Provide the reasons why each limitation could not be overcome using the method(s) chosen to acquire or gather the data [cite to other studies that had similar problems when possible];
- Assess the impact of each limitation in relation to the overall findings and conclusions of your study; and,
- If appropriate, describe how these limitations could point to the need for further research.
Remember that the method you chose may be the source of a significant limitation that has emerged during your interpretation of the results [for example, you didn't interview a group of people that you later wish you had]. If this is the case, don't panic. Acknowledge it, and explain how applying a different or more robust methodology might address the research problem more effectively in a future study. A underlying goal of scholarly research is not only to show what works, but to demonstrate what doesn't work or what needs further clarification.
Aguinis, Hermam and Jeffrey R. Edwards. “Methodological Wishes for the Next Decade and How to Make Wishes Come True.” Journal of Management Studies 51 (January 2014): 143-174; Brutus, Stéphane et al. "Self-Reported Limitations and Future Directions in Scholarly Reports: Analysis and Recommendations." Journal of Management 39 (January 2013): 48-75; Ioannidis, John P.A. "Limitations are not Properly Acknowledged in the Scientific Literature." Journal of Clinical Epidemiology 60 (2007): 324-329; Pasek, Josh. Writing the Empirical Social Science Research Paper: A Guide for the Perplexed . January 24, 2012. Academia.edu; Structure: How to Structure the Research Limitations Section of Your Dissertation . Dissertations and Theses: An Online Textbook. Laerd.com; What Is an Academic Paper? Institute for Writing Rhetoric. Dartmouth College; Writing the Experimental Report: Methods, Results, and Discussion . The Writing Lab and The OWL. Purdue University.
- << Previous: The Discussion
- Next: Conclusion >>
- Last Updated: Nov 6, 2023 1:43 PM
- URL: https://libraryguides.unh.edu/cpsonlinegradpaper
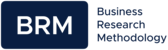
Research Limitations
It is for sure that your research will have some limitations and it is normal. However, it is critically important for you to be striving to minimize the range of scope of limitations throughout the research process. Also, you need to provide the acknowledgement of your research limitations in conclusions chapter honestly.
It is always better to identify and acknowledge shortcomings of your work, rather than to leave them pointed out to your by your dissertation assessor. While discussing your research limitations, don’t just provide the list and description of shortcomings of your work. It is also important for you to explain how these limitations have impacted your research findings.
Your research may have multiple limitations, but you need to discuss only those limitations that directly relate to your research problems. For example, if conducting a meta-analysis of the secondary data has not been stated as your research objective, no need to mention it as your research limitation.
Research limitations in a typical dissertation may relate to the following points:
1. Formulation of research aims and objectives . You might have formulated research aims and objectives too broadly. You can specify in which ways the formulation of research aims and objectives could be narrowed so that the level of focus of the study could be increased.
2. Implementation of data collection method . Because you do not have an extensive experience in primary data collection (otherwise you would not be reading this book), there is a great chance that the nature of implementation of data collection method is flawed.
3. Sample size. Sample size depends on the nature of the research problem. If sample size is too small, statistical tests would not be able to identify significant relationships within data set. You can state that basing your study in larger sample size could have generated more accurate results. The importance of sample size is greater in quantitative studies compared to qualitative studies.
4. Lack of previous studies in the research area . Literature review is an important part of any research, because it helps to identify the scope of works that have been done so far in research area. Literature review findings are used as the foundation for the researcher to be built upon to achieve her research objectives.
However, there may be little, if any, prior research on your topic if you have focused on the most contemporary and evolving research problem or too narrow research problem. For example, if you have chosen to explore the role of Bitcoins as the future currency, you may not be able to find tons of scholarly paper addressing the research problem, because Bitcoins are only a recent phenomenon.
5. Scope of discussions . You can include this point as a limitation of your research regardless of the choice of the research area. Because (most likely) you don’t have many years of experience of conducing researches and producing academic papers of such a large size individually, the scope and depth of discussions in your paper is compromised in many levels compared to the works of experienced scholars.
You can discuss certain points from your research limitations as the suggestion for further research at conclusions chapter of your dissertation.
My e-book, The Ultimate Guide to Writing a Dissertation in Business Studies: a step by step assistance offers practical assistance to complete a dissertation with minimum or no stress. The e-book covers all stages of writing a dissertation starting from the selection to the research area to submitting the completed version of the work within the deadline. John Dudovskiy
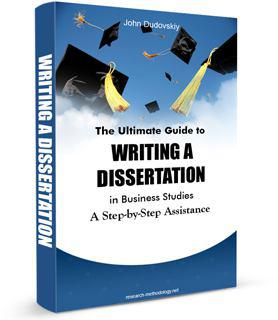

Organizing Academic Research Papers: Limitations of the Study
- Purpose of Guide
- Design Flaws to Avoid
- Glossary of Research Terms
- Narrowing a Topic Idea
- Broadening a Topic Idea
- Extending the Timeliness of a Topic Idea
- Academic Writing Style
- Choosing a Title
- Making an Outline
- Paragraph Development
- Executive Summary
- Background Information
- The Research Problem/Question
- Theoretical Framework
- Citation Tracking
- Content Alert Services
- Evaluating Sources
- Primary Sources
- Secondary Sources
- Tertiary Sources
- What Is Scholarly vs. Popular?
- Qualitative Methods
- Quantitative Methods
- Using Non-Textual Elements
- Limitations of the Study
- Common Grammar Mistakes
- Avoiding Plagiarism
- Footnotes or Endnotes?
- Further Readings
- Annotated Bibliography
- Dealing with Nervousness
- Using Visual Aids
- Grading Someone Else's Paper
- How to Manage Group Projects
- Multiple Book Review Essay
- Reviewing Collected Essays
- About Informed Consent
- Writing Field Notes
- Writing a Policy Memo
- Writing a Research Proposal
- Acknowledgements
The limitations of the study are those characteristics of design or methodology that impacted or influenced the application or interpretation of the results of your study. They are the constraints on generalizability and utility of findings that are the result of the ways in which you chose to design the study and/or the method used to establish internal and external validity.
Importance of...
Always acknowledge a study's limitations. It is far better for you to identify and acknowledge your study’s limitations than to have them pointed out by your professor and be graded down because you appear to have ignored them.
Keep in mind that acknowledgement of a study's limitations is an opportunity to make suggestions for further research. If you do connect your study's limitations to suggestions for further research, be sure to explain the ways in which these unanswered questions may become more focused because of your study.
Acknowledgement of a study's limitations also provides you with an opportunity to demonstrate to your professor that you have thought critically about the research problem, understood the relevant literature published about it, and correctly assessed the methods chosen for studying the problem. A key objective of the research process is not only discovering new knowledge but also to confront assumptions and explore what we don't know.
Claiming limitiations is a subjective process because you must evaluate the impact of those limitations . Don't just list key weaknesses and the magnitude of a study's limitations. To do so diminishes the validity of your research because it leaves the reader wondering whether, or in what ways, limitation(s) in your study may have impacted the findings and conclusions. Limitations require a critical, overall appraisal and interpretation of their impact. You should answer the question: do these problems with errors, methods, validity, etc. eventually matter and, if so, to what extent?
Structure: How to Structure the Research Limitations Section of Your Dissertation . Dissertations and Theses: An Online Textbook. Laerd.com.
Descriptions of Possible Limitations
All studies have limitations . However, it is important that you restrict your discussion to limitations related to the research problem under investigation. For example, if a meta-analysis of existing literature is not a stated purpose of your research, it should not be discussed as a limitation. Do not apologize for not addressing issues that you did not promise to investigate in your paper.
Here are examples of limitations you may need to describe and to discuss how they possibly impacted your findings. Descriptions of limitations should be stated in the past tense.
Possible Methodological Limitations
- Sample size -- the number of the units of analysis you use in your study is dictated by the type of research problem you are investigating. Note that, if your sample size is too small, it will be difficult to find significant relationships from the data, as statistical tests normally require a larger sample size to ensure a representative distribution of the population and to be considered representative of groups of people to whom results will be generalized or transferred.
- Lack of available and/or reliable data -- a lack of data or of reliable data will likely require you to limit the scope of your analysis, the size of your sample, or it can be a significant obstacle in finding a trend and a meaningful relationship. You need to not only describe these limitations but to offer reasons why you believe data is missing or is unreliable. However, don’t just throw up your hands in frustration; use this as an opportunity to describe the need for future research.
- Lack of prior research studies on the topic -- citing prior research studies forms the basis of your literature review and helps lay a foundation for understanding the research problem you are investigating. Depending on the currency or scope of your research topic, there may be little, if any, prior research on your topic. Before assuming this to be true, consult with a librarian! In cases when a librarian has confirmed that there is a lack of prior research, you may be required to develop an entirely new research typology [for example, using an exploratory rather than an explanatory research design]. Note that this limitation can serve as an important opportunity to describe the need for further research.
- Measure used to collect the data -- sometimes it is the case that, after completing your interpretation of the findings, you discover that the way in which you gathered data inhibited your ability to conduct a thorough analysis of the results. For example, you regret not including a specific question in a survey that, in retrospect, could have helped address a particular issue that emerged later in the study. Acknowledge the deficiency by stating a need in future research to revise the specific method for gathering data.
- Self-reported data -- whether you are relying on pre-existing self-reported data or you are conducting a qualitative research study and gathering the data yourself, self-reported data is limited by the fact that it rarely can be independently verified. In other words, you have to take what people say, whether in interviews, focus groups, or on questionnaires, at face value. However, self-reported data contain several potential sources of bias that should be noted as limitations: (1) selective memory (remembering or not remembering experiences or events that occurred at some point in the past); (2) telescoping [recalling events that occurred at one time as if they occurred at another time]; (3) attribution [the act of attributing positive events and outcomes to one's own agency but attributing negative events and outcomes to external forces]; and, (4) exaggeration [the act of representing outcomes or embellishing events as more significant than is actually suggested from other data].
Possible Limitations of the Researcher
- Access -- if your study depends on having access to people, organizations, or documents and, for whatever reason, access is denied or otherwise limited, the reasons for this need to be described.
- Longitudinal effects -- unlike your professor, who can literally devote years [even a lifetime] to studying a single research problem, the time available to investigate a research problem and to measure change or stability within a sample is constrained by the due date of your assignment. Be sure to choose a topic that does not require an excessive amount of time to complete the literature review, apply the methodology, and gather and interpret the results. If you're unsure, talk to your professor.
- Cultural and other type of bias -- we all have biases, whether we are conscience of them or not. Bias is when a person, place, or thing is viewed or shown in a consistently inaccurate way. It is usually negative, though one can have a positive bias as well. When proof-reading your paper, be especially critical in reviewing how you have stated a problem, selected the data to be studied, what may have been omitted, the manner in which you have ordered events, people, or places and how you have chosen to represent a person, place, or thing, to name a phenomenon, or to use possible words with a positive or negative connotation. Note that if you detect bias in prior research, it must be acknowledged and you should explain what measures were taken to avoid perpetuating bias.
- Fluency in a language -- if your research focuses on measuring the perceived value of after-school tutoring among Mexican-American ESL [English as a Second Language] students, for example, and you are not fluent in Spanish, you are limited in being able to read and interpret Spanish language research studies on the topic. This deficiency should be acknowledged.
Brutus, Stéphane et al. Self-Reported Limitations and Future Directions in Scholarly Reports: Analysis and Recommendations. Journal of Management 39 (January 2013): 48-75; Senunyeme, Emmanuel K. Business Research Methods . Powerpoint Presentation. Regent University of Science and Technology.
Structure and Writing Style
Information about the limitations of your study are generally placed either at the beginning of the discussion section of your paper so the reader knows and understands the limitations before reading the rest of your analysis of the findings, or, the limitations are outlined at the conclusion of the discussion section as an acknowledgement of the need for further study. Statements about a study's limitations should not be buried in the body [middle] of the discussion section unless a limitation is specific to something covered in that part of the paper. If this is the case, though, the limitation should be reiterated at the conclusion of the section.
If you determine that your study is seriously flawed due to important limitations , such as, an inability to acquire critical data, consider reframing it as a pilot study intended to lay the groundwork for a more complete research study in the future. Be sure, though, to specifically explain the ways that these flaws can be successfully overcome in later studies.
But, do not use this as an excuse for not developing a thorough research paper! Review the tab in this guide for developing a research topic . If serious limitations exist, it generally indicates a likelihood that your research problem is too narrowly defined or that the issue or event under study is too recent and, thus, very little research has been written about it. If serious limitations do emerge, consult with your professor about possible ways to overcome them or how to reframe your study.
When discussing the limitations of your research, be sure to:
- Describe each limitation in detailed but concise terms;
- Explain why each limitation exists;
- Provide the reasons why each limitation could not be overcome using the method(s) chosen to gather the data [cite to other studies that had similar problems when possible];
- Assess the impact of each limitation in relation to the overall findings and conclusions of your study; and,
- If appropriate, describe how these limitations could point to the need for further research.
Remember that the method you chose may be the source of a significant limitation that has emerged during your interpretation of the results [for example, you didn't ask a particular question in a survey that you later wish you had]. If this is the case, don't panic. Acknowledge it, and explain how applying a different or more robust methodology might address the research problem more effectively in any future study. A underlying goal of scholarly research is not only to prove what works, but to demonstrate what doesn't work or what needs further clarification.
Brutus, Stéphane et al. Self-Reported Limitations and Future Directions in Scholarly Reports: Analysis and Recommendations. Journal of Management 39 (January 2013): 48-75; Ioannidis, John P.A. Limitations are not Properly Acknowledged in the Scientific Literature. Journal of Clinical Epidemiology 60 (2007): 324-329; Pasek, Josh. Writing the Empirical Social Science Research Paper: A Guide for the Perplexed . January 24, 2012. Academia.edu; Structure: How to Structure the Research Limitations Section of Your Dissertation . Dissertations and Theses: An Online Textbook. Laerd.com; What Is an Academic Paper? Institute for Writing Rhetoric. Dartmouth College; Writing the Experimental Report: Methods, Results, and Discussion. The Writing Lab and The OWL. Purdue University.
Writing Tip
Don't Inflate the Importance of Your Findings! After all the hard work and long hours devoted to writing your research paper, it is easy to get carried away with attributing unwarranted importance to what you’ve done. We all want our academic work to be viewed as excellent and worthy of a good grade, but it is important that you understand and openly acknowledge the limitiations of your study. Inflating of the importance of your study's findings in an attempt hide its flaws is a big turn off to your readers. A measure of humility goes a long way!
Another Writing Tip
Negative Results are Not a Limitation!
Negative evidence refers to findings that unexpectedly challenge rather than support your hypothesis. If you didn't get the results you anticipated, it may mean your hypothesis was incorrect and needs to be reformulated, or, perhaps you have stumbled onto something unexpected that warrants further study. Moreover, the absence of an effect may be very telling in many situations, particularly in experimental research designs. In any case, your results may be of importance to others even though they did not support your hypothesis. Do not fall into the trap of thinking that results contrary to what you expected is a limitation to your study. If you carried out the research well, they are simply your results and only require additional interpretation.
Yet Another Writing Tip
A Note about Sample Size Limitations in Qualitative Research
Sample sizes are typically smaller in qualitative research because, as the study goes on, acquiring more data does not necessarily lead to more information. This is because one occurrence of a piece of data, or a code, is all that is necessary to ensure that it becomes part of the analysis framework. However, it remains true that sample sizes that are too small cannot adequately support claims of having achieved valid conclusions and sample sizes that are too large do not permit the deep, naturalistic, and inductive analysis that defines qualitative inquiry. Determining adequate sample size in qualitative research is ultimately a matter of judgment and experience in evaluating the quality of the information collected against the uses to which it will be applied and the particular research method and purposeful sampling strategy employed. If the sample size is found to be a limitation, it may reflect your judgement about the methodological technique chosen [e.g., single life history study versus focus group interviews] rather than the number of respondents used.
Huberman, A. Michael and Matthew B. Miles. Data Management and Analysis Methods. In Handbook of Qualitative Research. Norman K. Denzin and Yvonna S. Lincoln, eds. (Thousand Oaks, CA: Sage, 1994), pp. 428-444.
- << Previous: 8. The Discussion
- Next: 9. The Conclusion >>
- Last Updated: Jul 18, 2023 11:58 AM
- URL: https://library.sacredheart.edu/c.php?g=29803
- QuickSearch
- Library Catalog
- Databases A-Z
- Publication Finder
- Course Reserves
- Citation Linker
- Digital Commons
- Our Website
Research Support
- Ask a Librarian
- Appointments
- Interlibrary Loan (ILL)
- Research Guides
- Databases by Subject
- Citation Help
Using the Library
- Reserve a Group Study Room
- Renew Books
- Honors Study Rooms
- Off-Campus Access
- Library Policies
- Library Technology
User Information
- Grad Students
- Online Students
- COVID-19 Updates
- Staff Directory
- News & Announcements
- Library Newsletter
My Accounts
- Interlibrary Loan
- Staff Site Login

FIND US ON
- Privacy Policy

Home » Delimitations in Research – Types, Examples and Writing Guide
Delimitations in Research – Types, Examples and Writing Guide
Table of Contents

Delimitations
Definition:
Delimitations refer to the specific boundaries or limitations that are set in a research study in order to narrow its scope and focus. Delimitations may be related to a variety of factors, including the population being studied, the geographical location, the time period, the research design , and the methods or tools being used to collect data .
The Importance of Delimitations in Research Studies
Here are some reasons why delimitations are important in research studies:
- Provide focus : Delimitations help researchers focus on a specific area of interest and avoid getting sidetracked by tangential topics. By setting clear boundaries, researchers can concentrate their efforts on the most relevant and significant aspects of the research question.
- Increase validity : Delimitations ensure that the research is more valid by defining the boundaries of the study. When researchers establish clear criteria for inclusion and exclusion, they can better control for extraneous variables that might otherwise confound the results.
- Improve generalizability : Delimitations help researchers determine the extent to which their findings can be generalized to other populations or contexts. By specifying the sample size, geographic region, time frame, or other relevant factors, researchers can provide more accurate estimates of the generalizability of their results.
- Enhance feasibility : Delimitations help researchers identify the resources and time required to complete the study. By setting realistic parameters, researchers can ensure that the study is feasible and can be completed within the available time and resources.
- Clarify scope: Delimitations help readers understand the scope of the research project. By explicitly stating what is included and excluded, researchers can avoid confusion and ensure that readers understand the boundaries of the study.
Types of Delimitations in Research
Here are some types of delimitations in research and their significance:
Time Delimitations
This type of delimitation refers to the time frame in which the research will be conducted. Time delimitations are important because they help to narrow down the scope of the study and ensure that the research is feasible within the given time constraints.
Geographical Delimitations
Geographical delimitations refer to the geographic boundaries within which the research will be conducted. These delimitations are significant because they help to ensure that the research is relevant to the intended population or location.
Population Delimitations
Population delimitations refer to the specific group of people that the research will focus on. These delimitations are important because they help to ensure that the research is targeted to a specific group, which can improve the accuracy of the results.
Data Delimitations
Data delimitations refer to the specific types of data that will be used in the research. These delimitations are important because they help to ensure that the data is relevant to the research question and that the research is conducted using reliable and valid data sources.
Scope Delimitations
Scope delimitations refer to the specific aspects or dimensions of the research that will be examined. These delimitations are important because they help to ensure that the research is focused and that the findings are relevant to the research question.
How to Write Delimitations
In order to write delimitations in research, you can follow these steps:
- Identify the scope of your study : Determine the extent of your research by defining its boundaries. This will help you to identify the areas that are within the scope of your research and those that are outside of it.
- Determine the time frame : Decide on the time period that your research will cover. This could be a specific period, such as a year, or it could be a general time frame, such as the last decade.
- I dentify the population : Determine the group of people or objects that your study will focus on. This could be a specific age group, gender, profession, or geographic location.
- Establish the sample size : Determine the number of participants that your study will involve. This will help you to establish the number of people you need to recruit for your study.
- Determine the variables: Identify the variables that will be measured in your study. This could include demographic information, attitudes, behaviors, or other factors.
- Explain the limitations : Clearly state the limitations of your study. This could include limitations related to time, resources, sample size, or other factors that may impact the validity of your research.
- Justify the limitations : Explain why these limitations are necessary for your research. This will help readers understand why certain factors were excluded from the study.
When to Write Delimitations in Research
Here are some situations when you may need to write delimitations in research:
- When defining the scope of the study: Delimitations help to define the boundaries of your research by specifying what is and what is not included in your study. For instance, you may delimit your study by focusing on a specific population, geographic region, time period, or research methodology.
- When addressing limitations: Delimitations can also be used to address the limitations of your research. For example, if your data is limited to a certain timeframe or geographic area, you can include this information in your delimitations to help readers understand the limitations of your findings.
- When justifying the relevance of the study : Delimitations can also help you to justify the relevance of your research. For instance, if you are conducting a study on a specific population or region, you can explain why this group or area is important and how your research will contribute to the understanding of this topic.
- When clarifying the research question or hypothesis : Delimitations can also be used to clarify your research question or hypothesis. By specifying the boundaries of your study, you can ensure that your research question or hypothesis is focused and specific.
- When establishing the context of the study : Finally, delimitations can help you to establish the context of your research. By providing information about the scope and limitations of your study, you can help readers to understand the context in which your research was conducted and the implications of your findings.
Examples of Delimitations in Research
Examples of Delimitations in Research are as follows:
Research Title : “Impact of Artificial Intelligence on Cybersecurity Threat Detection”
Delimitations :
- The study will focus solely on the use of artificial intelligence in detecting and mitigating cybersecurity threats.
- The study will only consider the impact of AI on threat detection and not on other aspects of cybersecurity such as prevention, response, or recovery.
- The research will be limited to a specific type of cybersecurity threats, such as malware or phishing attacks, rather than all types of cyber threats.
- The study will only consider the use of AI in a specific industry, such as finance or healthcare, rather than examining its impact across all industries.
- The research will only consider AI-based threat detection tools that are currently available and widely used, rather than including experimental or theoretical AI models.
Research Title: “The Effects of Social Media on Academic Performance: A Case Study of College Students”
Delimitations:
- The study will focus only on college students enrolled in a particular university.
- The study will only consider social media platforms such as Facebook, Twitter, and Instagram.
- The study will only analyze the academic performance of students based on their GPA and course grades.
- The study will not consider the impact of other factors such as student demographics, socioeconomic status, or other factors that may affect academic performance.
- The study will only use self-reported data from students, rather than objective measures of their social media usage or academic performance.
Purpose of Delimitations
Some Purposes of Delimitations are as follows:
- Focusing the research : By defining the scope of the study, delimitations help researchers to narrow down their research questions and focus on specific aspects of the topic. This allows for a more targeted and meaningful study.
- Clarifying the research scope : Delimitations help to clarify the boundaries of the research, which helps readers to understand what is and is not included in the study.
- Avoiding scope creep : Delimitations help researchers to stay focused on their research objectives and avoid being sidetracked by tangential issues or data.
- Enhancing the validity of the study : By setting clear boundaries, delimitations help to ensure that the study is valid and reliable.
- Improving the feasibility of the study : Delimitations help researchers to ensure that their study is feasible and can be conducted within the time and resources available.
Applications of Delimitations
Here are some common applications of delimitations:
- Geographic delimitations : Researchers may limit their study to a specific geographic area, such as a particular city, state, or country. This helps to narrow the focus of the study and makes it more manageable.
- Time delimitations : Researchers may limit their study to a specific time period, such as a decade, a year, or a specific date range. This can be useful for studying trends over time or for comparing data from different time periods.
- Population delimitations : Researchers may limit their study to a specific population, such as a particular age group, gender, or ethnic group. This can help to ensure that the study is relevant to the population being studied.
- Data delimitations : Researchers may limit their study to specific types of data, such as survey responses, interviews, or archival records. This can help to ensure that the study is based on reliable and relevant data.
- Conceptual delimitations : Researchers may limit their study to specific concepts or variables, such as only studying the effects of a particular treatment on a specific outcome. This can help to ensure that the study is focused and clear.
Advantages of Delimitations
Some Advantages of Delimitations are as follows:
- Helps to focus the study: Delimitations help to narrow down the scope of the research and identify specific areas that need to be investigated. This helps to focus the study and ensures that the research is not too broad or too narrow.
- Defines the study population: Delimitations can help to define the population that will be studied. This can include age range, gender, geographical location, or any other factors that are relevant to the research. This helps to ensure that the study is more specific and targeted.
- Provides clarity: Delimitations help to provide clarity about the research study. By identifying the boundaries and limitations of the research, it helps to avoid confusion and ensures that the research is more understandable.
- Improves validity: Delimitations can help to improve the validity of the research by ensuring that the study is more focused and specific. This can help to ensure that the research is more accurate and reliable.
- Reduces bias: Delimitations can help to reduce bias by limiting the scope of the research. This can help to ensure that the research is more objective and unbiased.
About the author
Muhammad Hassan
Researcher, Academic Writer, Web developer
You may also like

Appendix in Research Paper – Examples and...

Research Paper Format – Types, Examples and...

Institutional Review Board – Application Sample...

Research Findings – Types Examples and Writing...

Research Paper Outline – Types, Example, Template

Dissertation vs Thesis – Key Differences
Information
- Author Services
Initiatives
You are accessing a machine-readable page. In order to be human-readable, please install an RSS reader.
All articles published by MDPI are made immediately available worldwide under an open access license. No special permission is required to reuse all or part of the article published by MDPI, including figures and tables. For articles published under an open access Creative Common CC BY license, any part of the article may be reused without permission provided that the original article is clearly cited. For more information, please refer to https://www.mdpi.com/openaccess .
Feature papers represent the most advanced research with significant potential for high impact in the field. A Feature Paper should be a substantial original Article that involves several techniques or approaches, provides an outlook for future research directions and describes possible research applications.
Feature papers are submitted upon individual invitation or recommendation by the scientific editors and must receive positive feedback from the reviewers.
Editor’s Choice articles are based on recommendations by the scientific editors of MDPI journals from around the world. Editors select a small number of articles recently published in the journal that they believe will be particularly interesting to readers, or important in the respective research area. The aim is to provide a snapshot of some of the most exciting work published in the various research areas of the journal.
Original Submission Date Received: .
- Active Journals
- Find a Journal
- Proceedings Series
- For Authors
- For Reviewers
- For Editors
- For Librarians
- For Publishers
- For Societies
- For Conference Organizers
- Open Access Policy
- Institutional Open Access Program
- Special Issues Guidelines
- Editorial Process
- Research and Publication Ethics
- Article Processing Charges
- Testimonials
- Preprints.org
- SciProfiles
- Encyclopedia
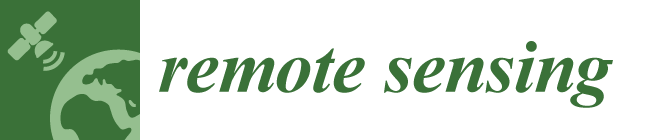
Article Menu
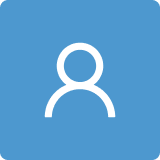
- Subscribe SciFeed
- Recommended Articles
- Google Scholar
- on Google Scholar
- Table of Contents
Find support for a specific problem in the support section of our website.
Please let us know what you think of our products and services.
Visit our dedicated information section to learn more about MDPI.
JSmol Viewer
Assessment of semi-automated techniques for crop mapping in chile based on global land cover satellite data.
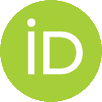
1. Introduction
2. study area, 3. data and methods, 3.1.1. in situ data, 3.1.2. satellite-based data, esa world cover, dynamic world, 3.2. methods: agricultural area calculation, 3.2.1. reprojection and filtering, 3.2.2. training sample selection and classification, 3.2.3. accuracy assessment, 3.3. work strategy, 5. discussion, 6. conclusions, author contributions, data availability statement, acknowledgments, conflicts of interest.
Class | Trees | Cropland | Urban | Grassland | Shrub | Bare |
---|---|---|---|---|---|---|
ED threshold | 0.06 | 0.07 | 0.10 | 0.10 | 0.05 | 0.10 |
Year | 2007 | 2021 | |||||||||
---|---|---|---|---|---|---|---|---|---|---|---|
Commune (Area [km ]) | AS | MODIS | MODIS v2 | AS | MODIS | MODIS v2 | ESA | ESA v2 | DW | DW v2 | CONAF |
Chillán (473.69) | 29.72 | 4.94 | 192.23 | 105.89 | 26.44 | 57.05 | 130.99 | 93.93 | 177.09 | 72.02 | 270.84 |
Bulnes (425.09) | 36.84 | 7.19 | 227.15 | 149.18 | 22.47 | 70.83 | 154.39 | 123.84 | 200.41 | 104.18 | 281.18 |
Chillán Viejo (262.80) | 12.92 | 2.20 | 65.97 | 32.75 | 6.20 | 12.77 | 54.99 | 30.12 | 76.83 | 24.53 | 86.73 |
El Carmen (667.39) | 87.83 | 133.72 | 173.23 | 214.63 | 207.54 | 178.05 | 247.75 | 220.97 | 280.18 | 192.04 | 334.21 |
Pemuco (561.52) | 68.24 | 72.24 | 103.69 | 94.88 | 75.73 | 93.97 | 129.20 | 117.90 | 188.22 | 109.29 | 181.57 |
Pinto (1099.70) | 28.09 | 5.78 | 129.67 | 59.93 | 33.14 | 86.08 | 94.54 | 145.47 | 109.26 | 95.27 | 139.49 |
Quillón (405.44) | 3.41 | 0 | 110.17 | 15.53 | 0 | 24.40 | 16.56 | 59.91 | 46.35 | 35.35 | 121.00 |
San Ignacio (363.48) | 88.87 | 11.78 | 174.09 | 130.19 | 35.05 | 92.16 | 183.49 | 163.16 | 213.91 | 141.07 | 261.56 |
Yungay (824.40) | 78.10 | 125.06 | 146.59 | 130.69 | 132.65 | 136.98 | 170.52 | 165.63 | 203.80 | 156.02 | 231.53 |
Quirihue (591.07) | 8.95 | 0 | 87.03 | 7.09 | 0.60 | 42.90 | 20.07 | 58.55 | 73.41 | 59.38 | 89.89 |
Cobquecura (570.05) | 15.12 | 0 | 38.65 | 7.09 | 0.60 | 6.12 | 18.05 | 39.35 | 41.37 | 21.73 | 64.01 |
Coelemu (341.66) | 4.50 | 0 | 47.84 | 9.76 | 0.20 | 29.37 | 11.40 | 29.37 | 33.04 | 22.19 | 57.83 |
Ninhue (402.63) | 11.07 | 2.28 | 101.16 | 10.93 | 4.90 | 73.74 | 36.75 | 79.64 | 70.41 | 58.92 | 104.94 |
Portezuelo (290.03) | 5.01 | 0 | 67.08 | 11.25 | 0 | 31.10 | 20.88 | 42.16 | 59.30 | 36.50 | 92.72 |
Ránquil (247.57) | 1.06 | 0 | 51.28 | 9.78 | 0 | 10.53 | 8.19 | 23.95 | 29.14 | 20.40 | 57.46 |
Treguaco (315.22) | 7.42 | 0 | 46.68 | 5.28 | 0 | 21.77 | 10.63 | 31.23 | 37.69 | 25.83 | 81.32 |
San Carlos (873.53) | 108.99 | 16.72 | 417.43 | 196.58 | 69.92 | 134.31 | 306.15 | 228.83 | 416.46 | 191.82 | 599.61 |
Coihueco (1773.29) | 90.16 | 24.13 | 304.12 | 170.01 | 54.52 | 206.33 | 206.73 | 318.07 | 286.23 | 181.38 | 341.98 |
Ñiquen (492.22) | 65.70 | 100.25 | 205.12 | 98.05 | 146.00 | 73.02 | 186.06 | 113.72 | 236.89 | 99.77 | 327.87 |
San Fabián (1540.85) | 4.53 | 0 | 74.16 | 6.14 | 1.20 | 25.38 | 17.79 | 67.13 | 25.14 | 24.75 | 39.16 |
San Nicolás (567.59) | 24.22 | 4.54 | 189.59 | 77.49 | 18.72 | 32.28 | 121.94 | 59.76 | 185.13 | 55.15 | 237.98 |
Click here to enlarge figure
- Zambrano, F.; Vrieling, A.; Nelson, A.; Meroni, M.; Tadesse, T. Prediction of drought-induced reduction of agricultural productivity in Chile from MODIS, rainfall estimates, and climate oscillation indices. Remote Sens. Environ. 2018 , 219 , 15–30. [ Google Scholar ] [ CrossRef ]
- Zúñiga, F.; Jaime, M.; Salazar, C. Crop farming adaptation to droughts in small-scale dryland agriculture in Chile. Water Resour. Econ. 2021 , 34 , 100176. [ Google Scholar ] [ CrossRef ]
- Instituto Nacional de Estadísticas. Available online: https://www.ine.gob.cl/ (accessed on 6 May 2024).
- Nguyen, L.H.; Joshi, D.R.; Clay, D.E.; Henebry, G.M. Characterizing land cover/land use from multiple years of Landsat and MODIS time series: A novel approach using land surface phenology modeling and random forest classifier. Remote Sens. Environ. 2020 , 238 , 111017. [ Google Scholar ] [ CrossRef ]
- Regasa, M.S.; Nones, M.; Adeba, D. A review on land use and land cover change in Ethiopian basins. Land 2021 , 10 , 585. [ Google Scholar ] [ CrossRef ]
- Ustaoglu, E.; Aydınoglu, A.C. Regional variations of land-use development and land-use/cover change dynamics: A case study of Turkey. Remote Sens. 2019 , 11 , 885. [ Google Scholar ] [ CrossRef ]
- Brown, C.F.; Brumby, S.P.; Guzder-Williams, B.; Birch, T.; Hyde, S.B.; Mazzariello, J.; Czerwinski, W.; Pasquarella, V.; Haertel, R.; Ilyushchenko, S.; et al. Dynamic World, Near real-time global 10 m land use land cover mapping. Sci. Data 2022 , 9 , 251. [ Google Scholar ] [ CrossRef ]
- Dong, R.; Li, C.; Fu, H.; Wang, J.; Li, W.; Yao, Y.; Gan, L.; Yu, L.; Gong, P. Improving 3-m resolution land cover mapping through efficient learning from an imperfect 10-m resolution map. Remote Sens. 2020 , 12 , 1418. [ Google Scholar ] [ CrossRef ]
- Petrişor, A.I.; Sirodoev, I.; Ianoş, I. Trends in the national and regional transitional dynamics of land cover and use changes in Romania. Remote Sens. 2020 , 12 , 230. [ Google Scholar ] [ CrossRef ]
- Friedl, M.A.; Woodcock, C.E.; Olofsson, P.; Zhu, Z.; Loveland, T.; Stanimirova, R.; Arevalo, P.; Bullock, E.; Hu, K.-T.; Zhang, Y.; et al. Medium spatial resolution mapping of global land cover and land cover change across multiple decades from Landsat. Front. Remote Sens. 2020 , 3 , 894571. [ Google Scholar ] [ CrossRef ]
- Plummer, S.; Lecomte, P.; Doherty, M. The ESA Climate Change Initiative (CCI): A European contribution to the generation of the Global Climate Observing System. Remote Sens. Environ. 2017 , 203 , 2–8. [ Google Scholar ] [ CrossRef ]
- Sulla-Menashe, D.; Gray, J.M.; Abercrombie, S.P.; Friedl, M.A. Hierarchical mapping of annual global land cover 2001 to present: The MODIS Collection 6 Land Cover product. Remote Sens. Environ. 2019 , 222 , 183–194. [ Google Scholar ] [ CrossRef ]
- Zhao, T.; Zhang, X.; Gao, Y.; Mi, J.; Liu, W.; Wang, J.; Jiang, M.; Liu, L. Assessing the accuracy and consistency of six fine-resolution global land cover products using a novel stratified random sampling validation dataset. Remote Sens. 2023 , 15 , 2285. [ Google Scholar ] [ CrossRef ]
- Graesser, J.; Ramankutty, N. Detection of cropland field parcels from Landsat imagery. Remote Sens. Environ. 2017 , 201 , 165–180. [ Google Scholar ] [ CrossRef ]
- Phan, D.C.; Trung, T.H.; Truong, V.T.; Sasagawa, T.; Vu, T.P.T.; Bui, D.T.; Hayashi, M.; Tadono, T.; Nasahara, K.N. First comprehensive quantification of annual land use/cover from 1990 to 2020 across mainland Vietnam. Sci. Rep. 2021 , 11 , 9979. [ Google Scholar ] [ CrossRef ]
- Gorelick, N.; Hancher, M.; Dixon, M.; Ilyushchenko, S.; Thau, D.; Moore, R. Google Earth Engine: Análisis geoespacial a escala planetaria para todos. Teledetección Del Medio Ambiente 2017 , 202 , 18–27. [ Google Scholar ]
- Volke, M.; Abarca-Del-Rio, R.; Warner, T.A. Cost-effective disaster-induced land cover analysis: A semi-automatic methodology Using machine learning and satellite imagery. Int. J. Remote Sens. 2024 , 45 , 279–305. [ Google Scholar ] [ CrossRef ]
- Venter, Z.S.; Barton, D.N.; Chakraborty, T.; Simensen, T.; Singh, G. Global 10 m land use land cover datasets: A comparison of dynamic world, world cover and esri land cover. Remote Sens. 2022 , 14 , 4101. [ Google Scholar ] [ CrossRef ]
- Bagan, H.; Millington, A.; Takeuchi, W.; Yamagata, Y. Spatiotemporal analysis of deforestation in the Chapare region of Bolivia using LANDSAT images. Land Degrad. Dev. 2020 , 31 , 3024–3039. [ Google Scholar ] [ CrossRef ]
- Zhang, H.K.; Roy, D.P. Using the 500 m MODIS land cover product to derive a consistent continental scale 30 m Landsat land cover classification. Remote Sens. Environ. 2017 , 197 , 15–34. [ Google Scholar ] [ CrossRef ]
- Huang, H.; Wang, J.; Liu, C.; Liang, L.; Li, C.; Gong, P. The migration of training samples towards dynamic global land cover mapping. ISPRS J. Photogramm. Remote Sens. 2020 , 161 , 27–36. [ Google Scholar ] [ CrossRef ]
- Teluguntla, P.; Thenkabail, P.; Oliphant, A.; Xiong, J.; Gumma, M.K.; Congalton, R.G.; Yadav, K.; Huete, A. A 30-m landsat-derived cropland extent product of Australia and China using random forest machine learning algorithm on Google Earth Engine cloud computing platform. ISPRS J. Photogramm. Remote Sens. 2018 , 144 , 325–340. [ Google Scholar ] [ CrossRef ]
- Phalke, A.R.; Özdoğan, M.; Thenkabail, P.S.; Erickson, T.; Gorelick, N.; Yadav, K.; Congalton, R.G. Mapping croplands of Europe, Middle East, Russia, and Central Asia using Landsat, Random Forest, and Google Earth Engine. ISPRS J. Photogramm. Remote Sens. 2020 , 167 , 104–122. [ Google Scholar ] [ CrossRef ]
- Radoux, J.; Lamarche, C.; Van Bogaert, E.; Bontemps, S.; Brockmann, C.; Defourny, P. Automated Training Sample Extraction for Global Land Cover Mapping. Remote Sens. 2014 , 6 , 3965–3987. [ Google Scholar ] [ CrossRef ]
- Roy, D.P.; Kumar, S.S. Multi-year MODIS active fire type classification over the Brazilian Tropical Moist Forest Biome. Int. J. Digit. Earth 2017 , 10 , 54–84. [ Google Scholar ] [ CrossRef ]
- Zhang, X.; Liu, L.; Chen, X.; Gao, Y.; Xie, S.; Mi, J. GLC_FCS30: Global land-cover product with fine classification system at 30 m using time-series Landsat imagery. Earth Syst. Sci. Data 2021 , 13 , 2753–2776. [ Google Scholar ] [ CrossRef ]
- Zanaga, D.; Van De Kerchove, R.; Daems, D.; De Keersmaecker, W.; Brockmann, C.; Kirches, G.; Wevers, J.; Cartus, O.; Santoro, M.; Fritz, S.; et al. ESA WorldCover 10 m 2021 v200. 2022. Available online: https://zenodo.org/records/7254221 (accessed on 15 May 2024).
- USGS: Landsat Missions. Available online: https://www.usgs.gov/landsat-missions (accessed on 9 May 2024).
- Copernicus Data Space Ecosystem (CDSE): Sentinel-2. Available online: https://dataspace.copernicus.eu/explore-data/data-collections/sentinel-data/sentinel-2 (accessed on 9 May 2024).
- Earth Engine Data Catalog (EEDC). Available online: https://developers.google.com/earth-engine/datasets (accessed on 9 May 2024).
- Valderrama, N.; Azocar, G.; Juarez, F. Agricultura y productividad: Tendencias y determinantes en una región de Chile central (Determining Factors and Trends of Agricultural Productivity in a Region of Central Chile). RAN-Rev. Acad. Neg. 2019 , 5 . [ Google Scholar ]
- ODEPA: Región de Ñuble Información Regional 2019. Available online: https://www.odepa.gob.cl/wp-content/uploads/2018/11/%C3%91uble.pdf (accessed on 24 May 2024).
- National Statistics Institute (INE). Available online: https://www.ine.gob.cl/censoagropecuario (accessed on 9 May 2024).
- Tucker, C.J. Red and photographic infrared linear combinations for monitoring vegetation. Remote Sens. Environ. 1979 , 8 , 127–150. [ Google Scholar ] [ CrossRef ]
- Xu, H. Modification of normalised difference water index (NDWI) to enhance open water features in remotely sensed imagery. Int. J. Remote Sens. 2006 , 27 , 3025–3033. [ Google Scholar ] [ CrossRef ]
- Huete, A.R.; Didan, K.; van Leeuwen, W.J.D.; Vermote, E.F. Global-scale analysis of vegetation indices for moderate resolution monitoring of terrestrial vegetation. Remote Sens. Earth Sci. Ocean Sea Ice Appl. 1999 , 3868 , 141–151. [ Google Scholar ]
- Miller, J.D.; Thode, A.E. Quantifying burn severity in a heterogeneous landscape with a relative version of the delta Normalized Burn Ratio (dNBR). Remote Sens. Environ. 2007 , 109 , 66–80. [ Google Scholar ] [ CrossRef ]
- Wang, Q.; Blackburn, G.A.; Onojeghuo, A.O.; Dash, J.; Zhou, L.; Zhang, Y.; Atkinson, P.M. Fusion of Landsat 8 OLI and Sentinel-2 MSI Data. IEEE Trans. Geosci. Remote Sens. 2017 , 55 , 3885–3899. [ Google Scholar ] [ CrossRef ]
- Zhu, Z.; Wang, S.; Woodcock, C.E. Improvement and expansion of the Fmask algorithm: Cloud, cloud shadow, and snow detection for Landsats 4–7, 8, and Sentinel 2 images. Remote Sens. Environ. 2015 , 159 , 269–277. [ Google Scholar ] [ CrossRef ]
- Yang, J.; Yu, L.; Mallinis, G.; Orusa, T.; Cammareri, D.; Mondino, E.B. A Possible Land Cover EAGLE Approach to Overcome Remote Sensing Limitations in the Alps Based on Sentinel-1 and Sentinel-2: The Case of Aosta Valley (NW Italy). Remote Sens. 2023 , 15 , 178. [ Google Scholar ]
- Wang, X.; Zhang, Y.; Zhang, K.; Wang, X.; Zhang, Y.; Zhang, K. Automatic 10 m Forest Cover Mapping in 2020 at China’s Han River Basin by Fusing ESA Sentinel-1/Sentinel-2 Land Cover and Sentinel-2 near Real-Time Forest Cover Possibility. Forests 2023 , 14 , 1133. [ Google Scholar ] [ CrossRef ]
- Venkatappa, M.; Sasaki, N.; Shrestha, R.P.; Tripathi, N.K.; Ma, H.O. Determination of Vegetation Thresholds for Assessing Land Use and Land Use Changes in Cambodia using the Google Earth Engine Cloud-Computing Platform. Remote Sens. 2019 , 11 , 1514. [ Google Scholar ] [ CrossRef ]
- Ghorbanian, A.; Kakooei, M.; Amani, M.; Mahdavi, S.; Mohammadzadeh, A.; Hasanlou, M. Improved land cover map of Iran using Sentinel imagery within Google Earth Engine and a novel automatic workflow for land cover classification using migrated training samples. ISPRS J. Photogramm. Remote Sens. 2020 , 167 , 276–288. [ Google Scholar ] [ CrossRef ]
- Olofsson, P.; Foody, G.M.; Herold, M.; Stehman, S.V.; Woodcock, C.E.; Wulder, M.A. Good practices for estimating area and assessing accuracy of land change. Remote Sens. Environ. 2014 , 148 , 42–57. [ Google Scholar ] [ CrossRef ]
- Breiman, L. Random forests. Mach. Learn. 2001 , 45 , 5–32. [ Google Scholar ] [ CrossRef ]
- He, Y.; Lee, E.; Warner, T.A. A time series of annual land use and land cover maps of China from 1982 to 2013 generated using AVHRR GIMMS NDVI3g data. Remote Sens. Environ. 2017 , 199 , 201–217. [ Google Scholar ] [ CrossRef ]
- Naidoo, L.; Cho, M.A.; Mathieu, R.; Asner, G. Classification of savanna tree species, in the Greater Kruger National Park region, by integrating hyperspectral and LiDAR data in a Random Forest data mining environment. ISPRS J. Photogramm. Remote Sens. 2012 , 69 , 167–179. [ Google Scholar ] [ CrossRef ]
- Rodriguez-Galiano, V.F.; Ghimire, B.; Rogan, J.; Chica-Olmo, M.; Rigol-Sanchez, J.P. An assessment of the effectiveness of a random forest classifier for land-cover classification. ISPRS J. Photogramm. Remote Sens. 2012 , 67 , 93–104. [ Google Scholar ] [ CrossRef ]
- Belgiu, M.; Drăguţ, L. Random forest in remote sensing: A review of applications and future directions. ISPRS J. Photogramm. Remote Sens. 2016 , 114 , 24–31. [ Google Scholar ] [ CrossRef ]
- Pelletier, C.; Valero, S.; Inglada, J.; Champion, N.; Dedieu, G. Assessing the robustness of Random Forests to map land cover with high resolution satellite image time series over large areas. Remote Sens. Environ. 2016 , 187 , 156–168. [ Google Scholar ] [ CrossRef ]
- Shiferaw, H.; Bewket, W.; Eckert, S. Performances of machine learning algorithms for mapping fractional cover of an invasive plant species in a dryland ecosystem. Ecol. Evol. 2019 , 9 , 2562–2574. [ Google Scholar ] [ CrossRef ]
- Prudente, V.H.R.; Martins, V.S.; Vieira, D.C.; e Silva, N.R.D.F.; Adami, M.; Sanches, I.D.A. Limitations of cloud cover for optical remote sensing of agricultural areas across South America. Remote Sens. Appl. Soc. Environ. 2020 , 20 , 100414. [ Google Scholar ] [ CrossRef ]
- Heck, E.; de Beurs, K.M.; Owsley, B.C.; Henebry, G.M. Evaluation of the MODIS collections 5 and 6 for change analysis of vegetation and land surface temperature dynamics in North and South America. ISPRS J. Photogramm. Remote Sens. 2019 , 156 , 121–134. [ Google Scholar ] [ CrossRef ]
- IDE Minagri: Catastro de Uso de Suelo y Vegetación (CONAF). Available online: https://ide.minagri.gob.cl/geoweb/2019/11/22/planificacion-catastral/ (accessed on 9 May 2024).
- Inglada, J.; Vincent, A.; Arias, M.; Tardy, B.; Morin, D.; Rodes, I. Operational High Resolution Land Cover Map Production at the Country Scale Using Satellite Image Time Series. Remote Sens. 2017 , 9 , 95. [ Google Scholar ] [ CrossRef ]
- Wessels, K.J.; van den Bergh, F.; Roy, D.P.; Salmon, B.P.; Steenkamp, K.C.; MacAlister, B.; Swanepoel, D.; Jewitt, D. Rapid Land Cover Map Updates Using Change Detection and Robust Random Forest Classifiers. Remote Sens. 2016 , 8 , 888. [ Google Scholar ] [ CrossRef ]
Categories | 2020–2021 | |
---|---|---|
Original classes | Total Cereals Tubers Industrial crops Vegetables fruit trees Vines Flowers Seedbeds Plant nurseries Fodder Improved-grasslands * | |
Grouped classes | Irrigation, dry, total | |
Covered area | Ñuble region | Chillán, Bulnes, Chillán Viejo, El Carmen, Pemuco, Pinto, Quillón, San Ignacio, Yungay, Quirihue, Cobquecura, Coelemu. Ninhue, Portezuelo, Ránquil, Treguaco, San Carlos, Coihueco, Ñiquén, San Fabián y San Nicolás. |
Database | Available Period | Used Period | Spatial Resol. [m] | Classes or Variables | Selected Classes for Agriculture |
---|---|---|---|---|---|
ESA WorldCover 10 m v200 [ ] | 2021 | 10 | Tree cover, shrubland, grassland, cropland, built-up, bare/sparse vegetation, snow and ice, permanent water bodies, herbaceous wetland, mangroves, moss and lichen | Cropland: Land covered with annual crops that are sown/planted and harvestable at least once within the 12 months after the sowing/planting date. The annual cropland produces an herbaceous cover and is sometimes combined with trees or woody vegetation. Note that perennial woody crops are classified as the appropriate tree cover or shrub land cover type. Greenhouses are considered built-up. | |
Dynamic World V1 [ ] | 27 June 2015–present (revisit time: 2–5 days depending on latitude) | 8/2020–3/2021 | 10 | Water, trees, grass, flooded vegetation, crops, shrub and scrub, built, bare, snow and ice | Crops: Human-planted/plotted cereals, grasses and crops. |
MCD12Q1.061 MODIS Land Cover Type Yearly Global 500 m [ , ] | 2001–2022 (yearly) | 2021 | 500 | Several types of forest: evergreen needleleaf, evergreen broadleaf, deciduous needleleaf, deciduous broadleaf, and mixed, closed shrublands, open shrublands, woody savannas, savannas, grasslands, permanent wetlands, croplands, urban and built-up lands, cropland/natural vegetation mosaics, permanent snow and ice, barren and water bodies. | Cropland: Areas where at least 60% is cultivated cropland. Cropland/natural vegetation mosaics: Mosaics of small-scale cultivation (40–60%) with natural trees, shrubs or herbaceous vegetation. |
Landsat 8 Surface Reflectance | February 2013–present (16-day revisit time) | August 2020 –March 2021 | 30 | NDVI (normalized difference vegetation index) [ ], NDWI (normalized difference water index) [ ], EVI (enhanced vegetation index) [ ] and NBR (normalized burn rate) [ ] | - |
Sentinel-2 Surface Reflectance | March 2017–present (10-day revisit time) | 10 |
Commune | MODIS | MODIS v2 | ESA | ESA v2 | DW | DW v2 |
---|---|---|---|---|---|---|
Chillán | −75 | −46 | 24 | −11 | 67 | −32 |
Bulnes | −85 | −53 | 3 | −17 | 34 | −30 |
Chillán Viejo | −81 | −61 | 68 | −8 | 135 | −25 |
El Carmen | −3 | −17 | 15 | 3 | 31 | −11 |
Pemuco | −20 | −1 | 36 | 24 | 98 | 15 |
Pinto | −45 | 43 | 58 | 143 | 82 | 59 |
Quillón | −100 | 57 | 7 | 286 | 198 | 128 |
San Ignacio | −73 | −29 | 41 | 25 | 64 | 8 |
Yungay | 2 | 5 | 30 | 27 | 56 | 19 |
Quirihue | −92 | 505 | 83 | 726 | 935 | 738 |
Cobquecura | −92 | −14 | 155 | 455 | 483 | 206 |
Coelemu | −98 | 201 | 17 | 201 | 239 | 127 |
Ninhue | −55 | 575 | 236 | 629 | 544 | 439 |
Portezuelo | −100 | 176 | 86 | 275 | 427 | 224 |
Ránquil | −100 | 8 | −16 | 145 | 198 | 109 |
Treguaco | −100 | 312 | 101 | 491 | 614 | 389 |
San Carlos | −64 | −32 | 56 | 16 | 112 | −2 |
Coihueco | −68 | 21 | 22 | 87 | 68 | 7 |
Ñiquen | 49 | −26 | 90 | 16 | 142 | 2 |
San Fabián | −80 | 313 | 190 | 993 | 309 | 303 |
San Nicolás | −76 | −58 | 57 | −23 | 139 | −29 |
MODIS | Tr | Cr | Ur | Gr | Sh | Ba | UA | ||
Tr | 238 | 4 | 8 | 7 | 1 | 42 | 0.77 | ||
Cr | 21 | 1156 | 266 | 5 | 0 | 52 | 0.92 | ||
Ur | 0 | 53 | 231 | 2 | 0 | 14 | 0.40 | ||
Gr | 5 | 10 | 16 | 168 | 45 | 56 | 0.62 | ||
Sh | 0 | 0 | 0 | 33 | 265 | 0 | 0.84 | ||
Ba | 47 | 36 | 51 | 56 | 4 | 106 | 0.39 | ||
PA | 0.79 | 0.77 | 0.77 | 0.56 | 0.89 | 0.35 | OA | 0.72 | |
ESA | Tr | Cr | Ur | Gr | Sh | Ba | UA | ||
Tr | 289 | 1 | 8 | 1 | 0 | 0 | 0.95 | ||
Cr | 14 | 1161 | 268 | 56 | 1 | 0 | 0.99 | ||
Ur | 0 | 4 | 251 | 17 | 28 | 0 | 0.44 | ||
Gr | 1 | 4 | 21 | 268 | 6 | 0 | 0.77 | ||
Sh | 0 | 0 | 19 | 2 | 272 | 7 | 0.84 | ||
Ba | 0 | 0 | 0 | 2 | 17 | 279 | 0.98 | ||
PA | 0.97 | 0.77 | 0.84 | 0.89 | 0.91 | 0.94 | OA | 0.85 | |
DW | Tr | Cr | Ur | Gr | Sh | Ba | UA | ||
Tr | 281 | 2 | 0 | 1 | 15 | 0 | 0.88 | ||
Cr | 16 | 1390 | 36 | 4 | 54 | 0 | 0.96 | ||
Ur | 0 | 2 | 291 | 5 | 2 | 0 | 0.82 | ||
Gr | 4 | 42 | 19 | 195 | 39 | 0 | 0.76 | ||
Sh | 17 | 5 | 8 | 2 | 266 | 0 | 0.71 | ||
Ba | 0 | 0 | 2 | 50 | 0 | 244 | 1.00 | ||
PA | 0.94 | 0.93 | 0.97 | 0.65 | 0.89 | 0.82 | OA | 0.89 |
The statements, opinions and data contained in all publications are solely those of the individual author(s) and contributor(s) and not of MDPI and/or the editor(s). MDPI and/or the editor(s) disclaim responsibility for any injury to people or property resulting from any ideas, methods, instructions or products referred to in the content. |
Share and Cite
Volke, M.; Pedreros-Guarda, M.; Escalona, K.; Acuña, E.; Orrego, R. Assessment of Semi-Automated Techniques for Crop Mapping in Chile Based on Global Land Cover Satellite Data. Remote Sens. 2024 , 16 , 2964. https://doi.org/10.3390/rs16162964
Volke M, Pedreros-Guarda M, Escalona K, Acuña E, Orrego R. Assessment of Semi-Automated Techniques for Crop Mapping in Chile Based on Global Land Cover Satellite Data. Remote Sensing . 2024; 16(16):2964. https://doi.org/10.3390/rs16162964
Volke, Matías, María Pedreros-Guarda, Karen Escalona, Eduardo Acuña, and Raúl Orrego. 2024. "Assessment of Semi-Automated Techniques for Crop Mapping in Chile Based on Global Land Cover Satellite Data" Remote Sensing 16, no. 16: 2964. https://doi.org/10.3390/rs16162964
Article Metrics
Article access statistics, further information, mdpi initiatives, follow mdpi.
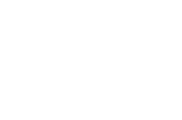
Subscribe to receive issue release notifications and newsletters from MDPI journals
- Open access
- Published: 12 August 2024
Enhancing mutation detection in multiple myeloma with an error-corrected ultra-sensitive NGS assay without plasma cell enrichment
- Jin Ju Kim 1 ,
- Soo-Jeong Kim 2 ,
- Seoyoung Lim 3 ,
- Seung-Tae Lee 4 , 5 ,
- Jong Rak Choi 4 , 5 ,
- Saeam Shin 4 &
- Doh Yu Hwang 6
Cancer Cell International volume 24 , Article number: 282 ( 2024 ) Cite this article
Metrics details
Risk stratification in multiple myeloma (MM) patients is crucial, and molecular genetic studies play a significant role in achieving this objective. Enrichment of plasma cells for next-generation sequencing (NGS) analysis has been employed to enhance detection sensitivity. However, these methods often come with limitations, such as high costs and low throughput. In this study, we explore the use of an error-corrected ultrasensitive NGS assay called positional indexing sequencing (PiSeq-MM). This assay can detect somatic mutations in MM patients without relying on plasma cell enrichment.
Diagnostic bone marrow aspirates (BMAs) and blood samples from 14 MM patients were used for exploratory and validation sets.
PiSeq-MM successfully detected somatic mutations in all BMAs, outperforming conventional NGS using plasma cells. It also identified 38 low-frequency mutations that were missed by conventional NGS, enhancing detection sensitivity below the 5% analytical threshold. When tested in an actual clinical environment, plasma cell enrichment failed in most BMAs (14/16), but the PiSeq-MM enabled mutation detection in all BMAs. There was concordance between PiSeq-MM using BMAs and ctDNA analysis in paired blood samples.
This research provides valuable insights into the genetic landscape of MM and highlights the advantages of error-corrected NGS for detecting low-frequency mutations. Although the current standard method for mutation analysis is plasma cell-enriched BMAs, total BMA or ctDNA testing with error correction is a viable alternative when plasma cell enrichment is not feasible.
Introduction
Multiple myeloma (MM), is a plasma cell neoplasm that predominantly affects elderly individuals and accounts for 10% of hematologic neoplasms [ 1 ]. The initial choice of therapy for patients with plasma cell neoplasms is based on clinical criteria; however, identifying cytogenetic abnormalities in plasma cells is valuable for risk stratification [ 2 ]. Several somatic driver mutations, such as KRAS , NRAS , and TP53 , are related to MM [ 3 , 4 ]. Therefore, many molecular genetic studies, including chromosomal analysis, interphase fluorescence in situ hybridization (FISH) and next-generation sequencing (NGS), are performed on MM patients.
Bone marrow aspirates (BMAs) from MM patients are mixture of normal hematopoietic cells and malignant plasma cells. The composition fraction of plasma cells varies from 10% to > 80%, so it is crucial to detect the genetic abnormality of malignant plasma cells, which can be diluted by normal cells. To increase the analytical sensitivity of FISH or NGS to detect molecular abnormalities, plasma cell enrichment techniques such as fluorescence immunophenotyping and interphase cytogenetics as a tool for the investigation of neoplasms (FICTION), fluorescence-activated cell sorter (FACS), or magnetic-activated cell sorting (MACS) are used in laboratories [ 5 , 6 , 7 ]. However, the plasma cell enrichment process has some disadvantages, such as the associated cost (for equipment, reagents, and labor), time (particularly for the cell sorting step), technician training, and the need for large amounts of fresh samples [ 7 ]. Due to these drawbacks, the application of enrichment techniques in routine clinical practice is limited.
There are frequent errors in NGS during processing due to DNA damage and sequencing steps. These errors create barriers to sensitive mutation detection. Therefore, several error correction strategies have recently emerged in the clinical NGS field to detect low-allele frequency mutations for circulating tumor DNA (ctDNA) or measurable residual disease (MRD) analysis [ 8 , 9 ]. Error correction strategies, such as molecular barcoding or in silico error suppression, can increase the detection capability of NGS to below 1% of variant allele frequencies (VAF) [ 10 , 11 , 12 , 13 ]. We developed a positional indexing sequencing (PiSeq) analysis method that tags the beginning and end parts of DNA molecules. By recognizing sequencing reads with the same start and end points as a group, the method is able to distinguish and correct errors in sequencing [ 14 , 15 ].
This study evaluated whether mutation detection sensitivity could be increased in MM patients using our error-corrected ultrasensitive NGS assay (PiSeq-MM) without plasma cell enrichment. We hypothesized that an error-corrected algorithm would enable us to detect somatic mutations in BMA without the need for plasma cell enrichment, similar to blood sample analysis. Using total cells for NGS can streamline the clinical workflow by eliminating the need for cell enrichment, and thereby reduce time and effort. Additionally, we conducted NGS on matched blood samples to investigate whether ctDNA analysis can infer somatic mutations in malignant plasma cells. An overview of this study is depicted in Fig. 1 .
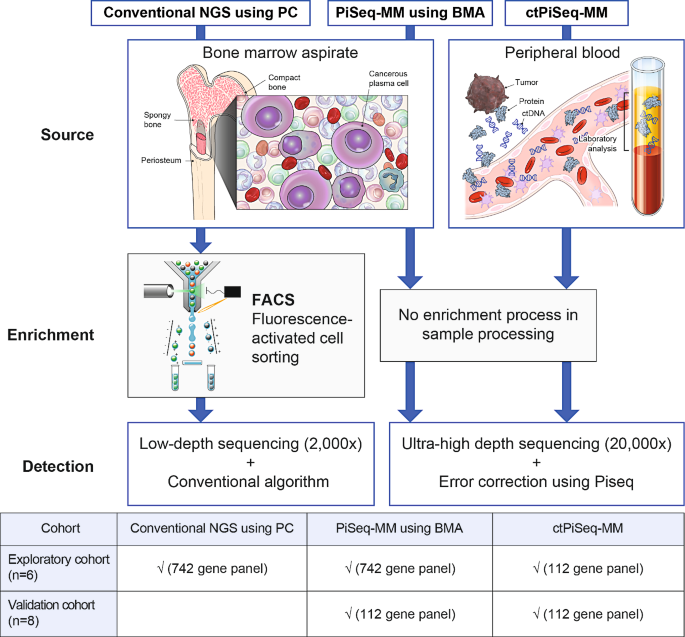
Overview of the study design for validation of an ultrasensitive NGS assay An illustration of the study design
Materials and methods
Study samples.
Diagnostic BMAs and matching blood samples from 14 patients who visited the Yongin Severance Hospital between March 2020 and March 2023 were included. Six patients were included in the exploratory cohort and eight in the validation cohort. Patients in the exploratory cohort had NGS results from fresh BMAs with a plasma cell enrichment step performed using our institute diagnostic panels (conventional NGS using PC). The panel included 742 target genes with therapeutic, prognostic, and diagnostic properties in a variety of cancers, including lymphomas and myeloma (Supplementary Table S1 ). The plasma cell enrichment process was successful in five out of six patients’ samples and the samples are proceeded to NGS analysis. In the sample in which the enrichment step failed (P3), the total DNA from the BMA was used for NGS analysis. General NGS strategies were applied without error-corrected bioinformatics algorithms with a mean sequencing depth of 522×. In this case, an analytical sensitivity of 5% was assumed.
The patients were pathologically diagnosed with MM according to the 2014 International Myeloma Working Group (IMWG) criteria [ 16 ]. The following clinical data were collected from the electronic medical records: age, sex, test results (including cytogenetics), bone marrow study, and clonality test results. The baseline clinical features of the 14 MM patients whose clinical samples were used in this validation are summarized in Table 1 .
Sample preparation
Fresh BMAs from exploratory cohorts collected in ethylene diamine tetra-acetic acid (EDTA) tubes were used for conventional NGS using PC. Plasma cell enrichment was performed as follows: buffy coats were isolated from BMAs and diluted with erythrocyte lysis buffer. The mix was incubated at room temperature for 20 min, and then centrifuged at 2100 rpm for 5 min. The cell pellet was resuspended in phosphate-buffered saline. After three washing cycles, the concentration was adjusted to 1 × 10⁶ − 4 × 10⁷ cells/mL. Antibody staining was performed using anti-CD38-FITC and anti-CD138-PE (Beckman Coulter, CA, USA). Then, plasma cell sorting was conducted on a BD FACS Melody™ (BD Biosciences, San Jose, CA, USA) or S3e™ Cell Sorter (Bio-Rad Laboratories, Hercules, CA, USA).
For ctDNA analysis, blood samples were obtained from patients at the time of diagnosis. Twenty milliliters of whole blood in a DxTube (Dxome, Seoul, Republic of Korea) was used. The samples were processed within 4 h at a constant temperature of 4 °C. Plasma was isolated by double centrifugation (1900 × g for 15 min). Peripheral blood mononuclear cells (PBMCs) were transferred to fresh tubes in 1 ml aliquots. Supernatants were also separately aliquoted in fresh tubes. Frozen aliquots of plasma were stored at -80 °C until ctDNA extraction. The ctDNA was extracted from 4 mL of plasma using magnetic circulating DNA Maxi Reagent (Dxome) according to the manufacturer’s instructions.
EDTA-BMAs from all 14 patients were also double centrifuged. Buffy coats were collected and then frozen in aliquots at -80 °C. Genomic DNA (gDNA) from PBMCs and BMA buffy coats was extracted using the QIAsymphony DNA Mini Kit (Qiagen, Hilden, Germany) according to the manufacturer’s guidelines. Library preparation was performed using 2.5–30 ng of ctDNA and 110–200 ng of sheared gDNA using the DxSeq Library prep reagent (Dxome). For each sample, PBMCs were sequenced as germline-matched controls using identical panel and library kits targeting an average depth of > 2,500×. The pooled libraries were paired-end sequenced (2 × 150 bp) on the NovaSeq 6000 System (Illumina, San Diego, CA, USA). Bioinformatics pipelines used for the analysis of NGS data consist of multiple steps, such as demultiplexing, read alignment, deduplication, base calibration and variant calling. An additional variant calling step with our error-correction pipeline, the PiSeq algorithm (Dxome), was used to differentiate low-frequency mutations from amplification artifacts and sequencing errors by calculating the genomic positions of mapped reads [ 14 ]. Variants were annotated using DxSeq software (Dxome) with public database information. Identified variants were visually confirmed with Integrative Genome Viewer (Broad Institute, Cambridge, MA, USA). Genic copy number variants (CNVs) and CNVs at the whole genome level were analyzed using DxSeq software (Dxome, Sungnam, South Korea). Germline variants were removed using parallel NGS data from PBMC-derived DNA.
Statistical analysis
Statistical analyses were performed using MedCalc version 18.2.1 (MedCalc Software; Mariakerke, Belgium). For continuous data, the Shapiro–Wilk test was used to detect departures from normality. Variables were compared using the Mann–Whitney U test. The Passing–Bablock regression was used to compare the VAF between samples. The Spearman rank correlation coefficient (r) was calculated. Statistical significance was defined as p < 0.05.
Ultra-high depth ngs sequencing with the piseq algorithm: enhancing mutation detection in MM without plasma cell enrichment
Our primary objective was to determine whether ultrahigh-depth NGS sequencing with the Piseq algorithm could effectively detect meaningful variants in MM without the need for plasma cell enrichment (PiSeq-MM). To achieve this, we conducted NGS using BMA samples without performing the enrichment step. Our study cohort consisted of six MM patients who had previously undergone conventional NGS using PC.
For the analysis, we used the same targeted NGS panel of 742 genes. The median sequencing depth of PiSeq-MM using the six BMAs was 14,427×. In the comparison, mutations were detected in five out of six conventional NGS using PCs (83.3%), while all six PiSeq-MM using BMAs had detectable mutations (Supplemental Table S2 ).
The total number of somatic mutations detected in conventional NGS using PCs ranged from 0 to 7 mutations per patient, amounting to 23 mutations in total. In contrast, PiSeq-MM using BMAs identified 47 somatic mutations, with a range of 2 to 16 mutations per patient. Notably, 39.1% ( n = 9/23) of the mutations detected in PiSeq-MM using BMAs also showed a median VAF of 46.8% in the plasma cell-enriched samples (Fig. 2 A and B). Mutations that were not discovered in PiSeq-MM using BMAs had a low representation in conventional NGS using PCs, with a median VAF of 28.8% (Fig. 2 B). None of the 14 non-overlapping mutations are known to impact the clinical diagnostic outcome.
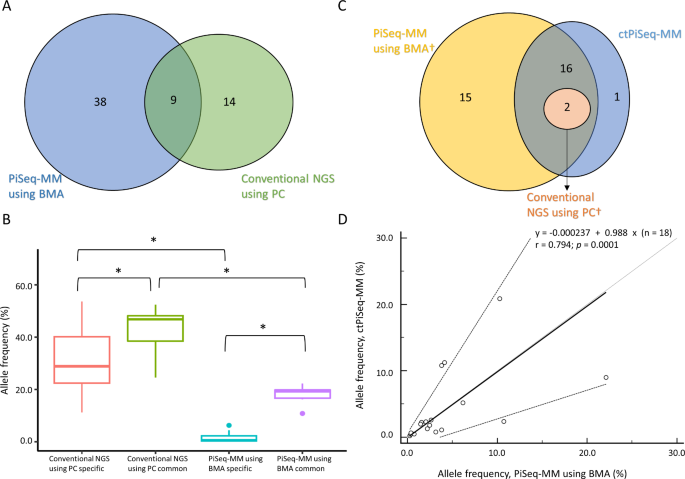
Concordance analysis of SNV/indel mutations detected in samples from the exploratory cohort. (A) Venn diagram showing the number of mutations detected in PiSeq-MM using BMA and conventional NGS using PC. (B) VAF distribution of mutations identified by conventional NGS using PC specific, shared by both conventional NGS using PC and PiSeq-MM using BMA, and by PiSeq-MM using BMA specific. (C) Venn diagram of mutations shared by PiSeq-MM using BMA, conventional NGS using PC and ctPiSeq-MM. Only targeted genes shared between each NGS panels were considered. (D) Correlation of VAFs between the two NGS panels. * p < 0.05 †Targeted genes shared by both NGS panels, 104 out of 742 genes are considered
PiSeq-MM using BMAs uncovered an additional 38 somatic mutations that were not detectable in conventional NGS using PCs. Among these, there were 7 variants affecting KRAS [ 17 , 18 ] and 6 variants affecting NRAS [ 18 ], both of which are known driver mutations in MM (Supplemental Table S2 ). The VAFs of these 38 somatic mutations ranged from 0.1 to 10.3% with a median VAF of 0.5% (Fig. 2 B), which is near or below the analytical sensitivity of general NGS, 5%.
Importantly, there was no correlation between the VAF of conventional NGS using PCs and PiSeq-MM using BMA ( r = 0.367, p = 0.3317). This finding indicates that the mutation detection with PiSeq-MM using BMA is not solely dependent on the VAF observed in plasma cell-enriched samples.
Exploring the potential of ctDNA analysis in MM: concordance and mutational landscape compared to conventional NGS
To investigate the potential of ctDNA in MM, we developed a targeted NGS panel comprising a smaller number of genes (112 genes) than were included in the comprehensive panel (742 genes) (Supplementary Table S1 ). The selected 112 genes were chosen based on the following criteria: (a) commonly found in myeloma patients tested with our institute’s conventional NGS panel (742 genes), (b) involved in important signaling pathways in multiple myeloma e.g. the MAPK, MYC, DNA repair and NFKB pathways, and (c) treatment targets or candidates for drug resistance in multiple myeloma (e.g. IKZF3 , BCL2 , PTEN and NFKB2 ) [ 19 , 20 , 21 , 22 ]. Additionally, to ensure that the ctDNA NGS panel can be used for patients with both myeloma and lymphoma, genes found in non-Hodgkin lymphoma (e.g., CD7 ) were also included. This selection of these genes was meticulously curated based on an extensive review of relevant literature, databases, and guidelines by a team of expert medical oncologists.
We conducted ctDNA analysis using the targeted NGS panel comprising 112 genes and employed the Piseq algorithm (ctPiSeq-MM) on matched blood samples from six patients in an exploratory cohort. The median sequencing depth of ctPiSeq-MM was 68,048×. A total of 19 somatic mutations were detected across the six ctPiSeq-MM samples, ranging from 0 to 6 mutations per patient.
Considering only the mutations present in the genes shared between the two NGS panels (Supplementary Table S1 ), we found that all mutations identified in conventional NGS using PC, specifically NRAS Q61R and NRAS G13D, were consistently detected in both Piseq-MM using BMAs and ctPiSeq-MM analyses. Almost all mutations (94.7%, 18/19) were detected in ctPiSeq-MM, except one mutation ( KRAS G12S) with a very low VAF of 0.24% (Fig. 2 C, Supplementary Table S2 ). Furthermore, there was a substantial correlation between the VAF of Piseq-MM using BMAs and ctPiSeq-MM ( r = 0.794, p = 0.0001; Fig. 2 D), indicating a strong concordance between the mutation profiles obtained from both methods.
Based on our initial observations in a small exploratory test cohort, we proceeded to validate our findings in a separate cohort of eight MM patients to assess the concordance of detected mutations between gDNA from BMA and ctDNA using the 112 gene NGS panel. For this validation cohort, the median sequencing depths of PiSeq-MM using BMAs and ctPiseq-MM were 60,444× and 78,862×, respectively.
We identified a total of 78 somatic mutations from PiSeq-MM using BMAs and 45 somatic mutations from ctPiseq-MM (Supplementary Table S3 ). The median VAF of somatic mutations detected in PiSeq-MM using BMAs was 0.15% (range: 0.04 − 9.58%), while the median VAF of mutations in ctPiSeq-MM was 0.96% (range: 0.09 − 21.19%).
Among the mutations detected, 25 mutations were shared in both PiSeq-MM using BMAs and ctPiSeq-MM of matched patients. There was a moderate degree of correlation between the VAF of PiSeq-MM using BMAs and ctPiSeq-MMs ( r = 0.665, p = 0.0003; Fig. 3 A). These results suggest a reasonable concordance in mutation detection between the two sample types, further supporting the potential utility of ctDNA analysis in MM.

(A) Correlation of SNV/indel allele frequency between PiSeq-MM using BMA and ctPiSeq-MM with a 112 gene targeted panel in the validation cohort. Mutation spectrum of (B) ctPiSeq-MM and (C) Piseq-MM using BMAs in the validation cohort
In all eight patients, at least one mutation was detected in both the BMA and blood samples. On average, PiSeq-MM using BMAs identified 11 mutations (range: 2–16), whereas ctPiSeq-MMs detected 6 mutations (range: 1–11). Interestingly, the most frequently mutated gene in the blood samples was KMT2C (Fig. 3 B ) . In contrast, the most frequently mutated gene detected in PiSeq-MM using BMAs was DNMT3A , followed by KMT2C , TP53 , MGA , ATM , and KRAS (Fig. 3 C). These findings provide valuable insights into the mutational landscape of MM, and highlight differences in mutation frequencies between the two sample types.
In one patient (S4), a CNV was detected, specifically a partial KIT gene deletion involving deletion of exons 8–21. Notably, this CNV was detected only in PiSeq-MM using BMAs and not in ctPiSeq-MMs.
Chromosomal abnormalities and detection challenges in MM patients: insights from multiple NGS protocols
In the exploratory set, a majority of patients (83.3%, 5/6) exhibited chromosomal structural abnormalities as determined by cytogenetic analysis. Specifically, two patients (P1 and P6) showed hyperdiploidy, while three patients (P2, P3, and P5) had complex karyotypes. Notably, cases displaying hyperdiploidy in karyotyping also had identifiable chromosomal abnormalities in conventional NGS using PC, PiSeq-MM using BMA, and ctPiseq-MM. However, it is essential to acknowledge that the chromosomal abnormality results using NGS may not be entirely consistent with karyotyping (Fig. 4 ).
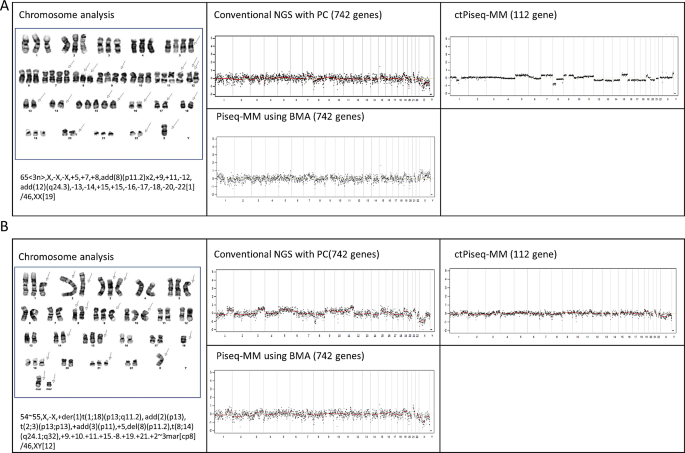
Chromosome analysis results by test method in patients with hyperdiploidy: (A) P1 and (B) P6
In contrast, patients with complex karyotypes or normal karyotypes sometimes exhibited abnormalities in conventional NGS using PC or Piseq-MM with BMA. However, interestingly, ctPiseq-MM did not show abnormalities in cases with normal karyotypes. This finding reveals the limitations of whole gene CNV analysis when using blood samples (Supplementary Fig. 1 ). Additionally, even when employing the Piseq algorithm in the samples from the validation cohort, it was challenging to detect chromosome abnormalities using whole gene CNV analysis (Supplementary Fig. 2 ).
Implementation of the Piseq Algorithm for NGS analysis in MM patients
Based on the insights gained from this study, we adopted the Piseq algorithm for BMAs from MM patients undergoing NGS, commencing in March 2022. NGS testing was initiated using plasma cell-enriched BMAs. In cases where enrichment was unsuccessful, the analysis was conducted on total BMAs. Between March 2022 and July 2023, 16 BMAs were subjected to NGS (Supplementary Table S4 ). The median arrival time at the laboratory was 41.0 h (range: 39.0–112.3 h), with plasma cell enrichment failing in the majority of cases (14/16, 87.5%).
Despite relying on total BMAs for most cases, mutations were identified in all samples, with potential driver mutations detected in 14 of 16 samples. The median VAF spanned from 0.1 to 46.6%, while the plasma cell burden in BMAs ranged from 10.8 to 99.6%. Notably, the median VAF of mutations and the plasma cell burden in BMAs showed no correlation ( p = 0.793).
Genetic variants have been linked to drug resistance and prognosis in MM patients [ 23 , 24 , 25 ], and NGS tests, cytogenetic studies, and FISH analyses are crucial for comprehensive genetic analysis. Additionally, detecting residual cancer or low-fraction mutations requires very sensitive methods. Enriching plasma cells from bone marrow aspirations is a commonly employed technique to enhance detection sensitivity in FISH analysis and NGS [ 26 ]. However, this enrichment step adds labor and technical costs and necessitates timely sample delivery to prevent CD138 shedding [ 27 ], which can lead to false negative results. False negatives may also occur in cases where plasma cell neoplasms lack CD138 expression, although this is rare [ 26 ]. Other challenges of plasma enrichment in clinical laboratories include the need for relatively large sample amounts, and occasional failure. These limitations show the need for an alternate strategy for profiling unenriched cells (Table 2 ).
Error-corrected bioinformatics can help to overcome the limitations of the plasma cell enrichment step while still generating compatible mutation analysis data for plasma cell- unenriched total BMA samples. When utilizing samples collected within a 24-hour timeframe, the enrichment process using the MAC method has been reported to exhibit a failure rate ranging between 10 and 22% depending on the plasma cell burden in the bone marrow [ 28 ]. Moreover, enrichment failure was observed in 16.7% (1/6) of an exploratory set using fresh BMA samples in this study. However, in clinical samples from actual patients, the failure rate of the enrichment step exceeded expectations, likely due to the extended duration between bone marrow sample aspiration and the enrichment process.
Despite the predominant use of total BMAs, mutations were identified across all samples, with potential driver mutations identified in most cases. In clinical testing with patient specimens, enrichment often encounters failures, potentially exacerbated by prolonged transit time of specimens when samples are referred to other medical facilities. The VAF of mutations was notably low, considering the plasma cell fractions of BMAs. Some mutations were detected only in conventional NGS using PCs, and these mutations tended to have lower VAFs than those detected by PiSeq-MM using BMAs. This discrepancy could arise from reduced plasma cell fractions that include other hematopoietic cells or hemodilution when using total BMA.
Although the average VAF of these mutations was lower than that of mutations detected simultaneously in the total BMA, some mutations had VAFs exceeding 45% (e.g., IGLL5 M42T (P2) and, ZFHX4 P3154A (P6)). This difference is thought to be due to variations in clone composition caused by the degree of hemodilution in the BMAs used for the tests. Conventional NGS using PCs was conducted using fresh, first or second pulled BMAs obtained during the aspiration procedure. In cases of PiseqMM using BMAs, frozen aliquots likely included subsequently aspirated samples. As a result, differences in clone burden and composition might exist between the samples [ 29 ]. However, in our study, these 14 mutations were not clinically significantly different in terms of diagnostic outcomes. In contrast, the nine mutations detected by both methods had a higher average VAFs compared to those found only by conventional NGS using PCs, including clinically significant NRAS gene mutations. Therefore, mutations detected in both tests are likely crucial for the disease, regardless of VAF, and may represent founder mutations that occurred early in clonal evolution and are shared among various clones. In addition, working with total BMA instead of sorted PCs may have disadvantages related to clonality/subclonality determination, our method effectively detected variants with VAF as low as 5%, ensuring variant identification across all samples and detect key mutations in disease development.
However, due to the relatively low VAF and difficulties in CNV analysis at the whole genome level, plasma cell-enriched BMA samples remain optimal for genetic analysis. Therefore, the most accurate option for NGS analysis remains plasma cell-enriched BMA with error-corrected bioinformatics. Nevertheless, if plasma cell enrichment is not feasible due to limited sample size or technical issues, applying error-corrected bioinformatics alone can still detect some informative mutations for risk stratification.
Although BMA is the preferred sample for sequencing, its ability to detect MM clones may be hindered by an inhomogeneous infiltration pattern [ 30 ]. In recent years, ctDNA analysis has emerged as an alternative method for tissue genomic DNA analysis and monitoring residual cancers noninvasively in many solid cancers [ 8 , 14 ]. Our study demonstrated that ctDNA testing with error-corrected bioinformatics not only yielded similar results to bone marrow samples but also detected mutations with low variant frequency.
This study was conducted with a very small number of patient samples. Therefore, it is challenging to interpret the results as representative of the mutation prevalence in the MM patient population. However, the mutations with a high prevalence in larger existing MM cohorts with NGS data such as those in the KRAS , NRAS , and TP53 genes were identified in the total BMA (eight validation cohort and fourteen clinical patients whose plasma cell enrichment failed) and blood samples (eight validation cohort) of patients using Piseq algorithm (Supplementary Table S5 ). This suggests general concordance with the findings of existing studies [ 3 , 31 , 32 ]. However, DNMT3A and KMT2C mutations were observed at higher frequency in total BMA and blood samples compared to other studies. Kogure et al. [ 33 ]. reported the same pattern in ctDNA analysis in relapsed/refractory MM patients, in whom the majority of mutations in clonal hematopoietic (CH)-related genes, such as DNMT3A and TET2 were detected only by ctDNA, in line with our results. CH gene mutations might have originated from nonmalignant hematopoietic cells not only in blood, but also in BMA, resulting in greater detection of CH mutations with very low frequency when using our PiseqMM. However, this should be further researched with a larger cohort to determine whether the detection frequency is indeed higher when applying this method to the MM patient group.
By jointly analyzing and interpreting genetic results from both BMA and ctDNA analyses in MM patients, not only disease monitoring but also information on the mutation spectrum of myeloma burden from sites other than the biopsy can be obtained. This approach proves valuable even in challenging scenarios, such as plasmacytoma or a dry-tapped marrow [ 34 , 35 ]. Moreover, recent study suggesting risk stratification model using ctDNA mutations in relapsed/refractory patients highlights the possible clinical application of ctDNA in near future [ 33 ].
As this was a pilot study for method validation, further research involving a larger number of patients is required in the future. More extensive investigations are also necessary to confirm the association between the obtained results and prognosis. Prospective studies on MM patients, including those with plasmacytoma, will be essential to establish the ctDNA test method’s utility as a comprehensive genetic analysis tool.
This research provides valuable insights into the genetic landscape of MM and highlights the advantages of error-corrected NGS for detecting low-frequency mutations. The results suggest that PiSeq-MM can effectively detect somatic mutations in MM patients without the need for plasma cell enrichment. ctDNA analysis showed potential utility in identifying somatic mutations in malignant plasma cells. Although the current standard method for mutation analysis is still the use of plasma cell-enriched BMAs, total BMA or ctDNA testing with error correction is a viable alternative when plasma cell enrichment is not feasible.
Data availability
The data that support the findings of this study are available from the corresponding author, [SS], upon reasonable request.
Abbreviations
Multiple Myeloma
Next-Generation Sequencing
Fluorescence In Situ Hybridization
Fluorescence Immunophenotyping and Interphase Cytogenetics as a Tool for the Investigation of Neoplasms
Fluorescence-Activated Cell Sorter
Magnetic-Activated Cell Sorting
Circulating Tumor DNA
Measurable Residual Disease
Variant Allele Frequencies
Positional Indexing Sequencing
Bone Marrow Aspirate
Peripheral Blood Mononuclear Cells
Genomic DNA
Copy Number Variants
International Myeloma Working Group
Rajkumar SV. Multiple myeloma: 2022 update on diagnosis, risk stratification, and management. Am J Hematol. 2022;97(8):1086–107.
Article CAS PubMed PubMed Central Google Scholar
Kyle R, Rajkumar SV. Criteria for diagnosis, staging, risk stratification and response assessment of multiple myeloma. Leukemia. 2009;23(1):3–9.
Article CAS PubMed Google Scholar
Lohr JG, Stojanov P, Carter SL, Cruz-Gordillo P, Lawrence MS, Auclair D, Sougnez C, Knoechel B, Gould J, Saksena G, et al. Widespread genetic heterogeneity in multiple myeloma: implications for targeted therapy. Cancer Cell. 2014;25(1):91–101.
Chapman MA, Lawrence MS, Keats JJ, Cibulskis K, Sougnez C, Schinzel AC, Harview CL, Brunet JP, Ahmann GJ, Adli M, et al. Initial genome sequencing and analysis of multiple myeloma. Nature. 2011;471(7339):467–72.
Stevens-Kroef M, Weghuis DO, Croockewit S, Derksen L, Hooijer J, Elidrissi-Zaynoun N, Siepman A, Simons A, Kessel AG. High detection rate of clinically relevant genomic abnormalities in plasma cells enriched from patients with multiple myeloma. Genes Chromosomes Cancer. 2012;51(11):997–1006.
Lu G, Muddasani R, Orlowski RZ, Abruzzo LV, Qazilbash MH, You MJ, Wang Y, Zhao M, Chen S, Glitza IC, et al. Plasma cell enrichment enhances detection of high-risk cytogenomic abnormalities by fluorescence in situ hybridization and improves risk stratification of patients with plasma cell neoplasms. Arch Pathol Lab Med. 2013;137(5):625–31.
Article PubMed PubMed Central Google Scholar
Hartmann L, Biggerstaff JS, Chapman DB, Scott JM, Johnson KR, Ghirardelli KM, Fritschle WK, Martinez DL, Bennington RK, de Baca ME, et al. Detection of genomic abnormalities in multiple myeloma: the application of FISH analysis in combination with various plasma cell enrichment techniques. Am J Clin Pathol. 2011;136(5):712–20.
Article PubMed Google Scholar
Ghannam J, Dillon LW, Hourigan CS. Next-generation sequencing for measurable residual disease detection in acute myeloid leukaemia. Br J Haematol. 2020;188(1):77–85.
Heuser M, Freeman SD, Ossenkoppele GJ, Buccisano F, Hourigan CS, Ngai LL, Tettero JM, Bachas C, Baer C, Béné MC, et al. 2021 Update on MRD in acute myeloid leukemia: a consensus document from the European LeukemiaNet MRD Working Party. Blood. 2021;138(26):2753–67.
Wang TT, Abelson S, Zou J, Li T, Zhao Z, Dick JE, Shlush LI, Pugh TJ, Bratman SV. High efficiency error suppression for accurate detection of low-frequency variants. Nucleic Acids Res. 2019;47(15):e87.
Schmitt MW, Kennedy SR, Salk JJ, Fox EJ, Hiatt JB, Loeb LA. Detection of ultra-rare mutations by next-generation sequencing. Proc Natl Acad Sci U S A. 2012;109(36):14508–13.
Newman AM, Lovejoy AF, Klass DM, Kurtz DM, Chabon JJ, Scherer F, Stehr H, Liu CL, Bratman SV, Say C, et al. Integrated digital error suppression for improved detection of circulating tumor DNA. Nat Biotechnol. 2016;34(5):547–55.
Pécuchet N, Rozenholc Y, Zonta E, Pietrasz D, Didelot A, Combe P, Gibault L, Bachet JB, Taly V, Fabre E, et al. Analysis of base-position error rate of Next-Generation sequencing to detect tumor mutations in circulating DNA. Clin Chem. 2016;62(11):1492–503.
Lee KS, Seo J, Lee CK, Shin S, Choi Z, Min S, Yang JH, Kwon WS, Yun W, Park MR, et al. Analytical and clinical validation of cell-free circulating tumor DNA assay for the estimation of Tumor Mutational Burden. Clin Chem. 2022;68(12):1519–28.
Kim JJ, Jang JE, Lee HA, Park MR, Kook HW, Lee ST, Choi JR, Min YH, Shin S, Cheong JW. Development of a next-generation sequencing-based Gene Panel Test to detect measurable residual disease in Acute myeloid leukemia. Ann Lab Med. 2023;43(4):328–36.
Rajkumar SV, Dimopoulos MA, Palumbo A, Blade J, Merlini G, Mateos MV, Kumar S, Hillengass J, Kastritis E, Richardson P, et al. International Myeloma Working Group updated criteria for the diagnosis of multiple myeloma. Lancet Oncol. 2014;15(12):e538–548.
Weissbach S, Heredia-Guerrero SC, Barnsteiner S, Grosshans L, Bodem J, Starz H, Langer C, Appenzeller S, Knop S, Steinbrunn T et al. Exon-4 mutations in KRAS affect MEK/ERK and PI3K/AKT signaling in human multiple myeloma cell lines. Cancers 2020, 12(2).
Walker BA, Mavrommatis K, Wardell CP, Ashby TC, Bauer M, Davies FE, Rosenthal A, Wang H, Qu P, Hoering A, et al. Identification of novel mutational drivers reveals oncogene dependencies in multiple myeloma. Blood. 2018;132(6):587–97.
Punnoose EA, Leverson JD, Peale F, Boghaert ER, Belmont LD, Tan N, Young A, Mitten M, Ingalla E, Darbonne WC, et al. Expression Profile of BCL-2, BCL-XL, and MCL-1 predicts pharmacological response to the BCL-2 selective antagonist Venetoclax in multiple myeloma models. Mol Cancer Ther. 2016;15(5):1132–44.
Lu G, Middleton RE, Sun H, Naniong M, Ott CJ, Mitsiades CS, Wong K-K, Bradner JE, Kaelin WG. The Myeloma Drug Lenalidomide promotes the Cereblon-Dependent Destruction of Ikaros Proteins. Science. 2014;343(6168):305–9.
Keats JJ, Fonseca R, Chesi M, Schop R, Baker A, Chng W-J, Van Wier S, Tiedemann R, Shi C-X, Sebag M, et al. Promiscuous mutations activate the noncanonical NF-κB pathway in multiple myeloma. Cancer Cell. 2007;12(2):131–44.
Annunziata CM, Davis RE, Demchenko Y, Bellamy W, Gabrea A, Zhan F, Lenz G, Hanamura I, Wright G, Xiao W, et al. Frequent Engagement of the classical and alternative NF-κB pathways by Diverse Genetic abnormalities in multiple myeloma. Cancer Cell. 2007;12(2):115–30.
Shirazi F, Jones RJ, Singh RK, Zou J, Kuiatse I, Berkova Z, Wang H, Lee HC, Hong S, Dick L, et al. Activating KRAS, NRAS, and BRAF mutants enhance proteasome capacity and reduce endoplasmic reticulum stress in multiple myeloma. Proc Natl Acad Sci U S A. 2020;117(33):20004–14.
Flietner E, Wen Z, Rajagopalan A, Jung O, Watkins L, Wiesner J, You X, Zhou Y, Sun Y, Kingstad-Bakke B, et al. Ponatinib sensitizes myeloma cells to MEK inhibition in the high-risk VQ model. Sci Rep. 2022;12(1):10616.
Hu Y, Chen W, Wang J. Progress in the identification of gene mutations involved in multiple myeloma. Onco Targets Ther. 2019;12:4075–80.
Pugh TJ, Fink JM, Lu X, Mathew S, Murata-Collins J, Willem P, Fang M. Assessing genome-wide copy number aberrations and copy-neutral loss-of-heterozygosity as best practice: an evidence-based review from the Cancer Genomics Consortium working group for plasma cell disorders. Cancer Genet. 2018;228–229:184–96.
Frigyesi I, Adolfsson J, Ali M, Kronborg Christophersen M, Johnsson E, Turesson I, Gullberg U, Hansson M, Nilsson B. Robust isolation of malignant plasma cells in multiple myeloma. Blood. 2014;123(9):1336–40.
Panakkal V, Rana S, Rathore S, Anshu A, Balakrishnan A, Singh C, Jandial A, Sachdeva MUS, Varma N, Lad D, et al. The success rate of interphase fluorescence in situ hybridization in plasma cell disorders can be improved using unconventional sources of plasma cells. Int J Lab Hematol. 2022;44(1):157–62.
Óskarsson JÞ, Rögnvaldsson S, Thorsteinsdottir S, Aspelund T, Gunnarsson SB, Hákonardóttir GK, Sigurðardóttir GÁ, Þórðardóttir ÁR, Gíslason GK, Ólafsson A, et al. Determining hemodilution in diagnostic bone marrow aspirated samples in plasma cell disorders by next-generation flow cytometry: proposal for a bone marrow quality index. Blood Cancer J. 2023;13(1):177.
Zamagni E, Tacchetti P, Barbato S, Cavo M. Role of imaging in the evaluation of minimal residual disease in multiple myeloma patients. J Clin Med 2020, 9(11).
Bolli N, Biancon G, Moarii M, Gimondi S, Li Y, de Philippis C, Maura F, Sathiaseelan V, Tai Y-T, Mudie L, et al. Analysis of the genomic landscape of multiple myeloma highlights novel prognostic markers and disease subgroups. Leukemia. 2018;32(12):2604–16.
Forbes SA, Beare D, Boutselakis H, Bamford S, Bindal N, Tate J, Cole CG, Ward S, Dawson E, Ponting L, et al. COSMIC: somatic cancer genetics at high-resolution. Nucleic Acids Res. 2016;45(D1):D777–83.
Kogure Y, Handa H, Ito Y, Ri M, Horigome Y, Iino M, Harazaki Y, Kobayashi T, Abe M, Ishida T, et al. ctDNA improves prognostic prediction for patients with relapsed/refractory MM receiving ixazomib, lenalidomide, and dexamethasone. Blood. 2024;143(23):2401–13.
Long X, Xu Q, Lou Y, Li C, Gu J, Cai H, Wang D, Xu J, Li T, Zhou X, et al. The utility of non-invasive liquid biopsy for mutational analysis and minimal residual disease assessment in extramedullary multiple myeloma. Br J Haematol. 2020;189(2):e45–8.
Heestermans R, Schots R, De Becker A, Van Riet I. Liquid biopsies as non-invasive tools for mutation profiling in multiple myeloma: application potential, challenges, and opportunities. Int J Mol Sci 2024, 25(10).
Download references
Acknowledgements
The authors thank Medical Illustration & Design, part of the Medical Research Support Services of Yonsei University College of Medicine, for all artistic support related to this work.
This research was supported by faculty research grant of Yonsei University College of Medicine for 2022 (6-2022-0085) and a grant from the National Research Foundation of Korea (NRF-2022R1I1A1A01068590).
Author information
Authors and affiliations.
Department of Laboratory Medicine, Yonsei University College of Medicine, Yongin Severance Hospital, Yongin, Korea
Department of Internal Medicine, Division of Hemato-Oncology, Yonsei University College of Medicine, Yongin Severance Hospital, Yongin, Korea
Soo-Jeong Kim
Graduate School of Medical Science, Brain Korea PLUS Project, Yonsei University College of Medicine, Seoul, Republic of Korea
Seoyoung Lim
Department of Laboratory Medicine, Yonsei University College of Medicine, Severance Hospital, 50-1 Yonsei-ro, Seodaemun-gu, Seoul, 03722, Korea
Seung-Tae Lee, Jong Rak Choi & Saeam Shin
Dxome, Seoul, Republic of Korea
Seung-Tae Lee & Jong Rak Choi
Department of Internal Medicine, Division of Hematology, Yonsei University College of Medicine, Yongin Severance Hospital, 50-1 Yonsei-ro, Seodaemun-gu, Seoul, 03722, Republic of Korea
Doh Yu Hwang
You can also search for this author in PubMed Google Scholar
Contributions
DYH and SS conceived and designed the analysis; JJK and SJK managed specimen collection and collected the data; SL, STL and JRC contributed data and analysis tools, JJK performed the analysis; JJK wrote the manuscript, SS reviewed the manuscript.
Corresponding authors
Correspondence to Saeam Shin or Doh Yu Hwang .
Ethics declarations
Ethics approval and consent to participate.
The Institutional Review Board at Yongin Severance hospital approved this study (IRB number 9-2023-0154), and all methods were carried out in accordance with the approved guidelines. All patients were registered to the prospective cohort study after written informed consents.
Consent for publication
Patient participation in the study was voluntary and they all signed consent forms, including consent to publish.
Competing interests
Authors ST Lee and JR Choi were employed by company Dxome. The remaining authors declare that the research was conducted in the absence of any commercial or financial relationships that could be construed as a potential conflict of interest.
Additional information
Publisher’s note.
Springer Nature remains neutral with regard to jurisdictional claims in published maps and institutional affiliations.
Electronic supplementary material
Below is the link to the electronic supplementary material.
Supplementary Material 1
Rights and permissions.
Open Access This article is licensed under a Creative Commons Attribution 4.0 International License, which permits use, sharing, adaptation, distribution and reproduction in any medium or format, as long as you give appropriate credit to the original author(s) and the source, provide a link to the Creative Commons licence, and indicate if changes were made. The images or other third party material in this article are included in the article’s Creative Commons licence, unless indicated otherwise in a credit line to the material. If material is not included in the article’s Creative Commons licence and your intended use is not permitted by statutory regulation or exceeds the permitted use, you will need to obtain permission directly from the copyright holder. To view a copy of this licence, visit http://creativecommons.org/licenses/by/4.0/ . The Creative Commons Public Domain Dedication waiver ( http://creativecommons.org/publicdomain/zero/1.0/ ) applies to the data made available in this article, unless otherwise stated in a credit line to the data.
Reprints and permissions
About this article
Cite this article.
Kim, J.J., Kim, SJ., Lim, S. et al. Enhancing mutation detection in multiple myeloma with an error-corrected ultra-sensitive NGS assay without plasma cell enrichment. Cancer Cell Int 24 , 282 (2024). https://doi.org/10.1186/s12935-024-03470-7
Download citation
Received : 20 September 2023
Accepted : 31 July 2024
Published : 12 August 2024
DOI : https://doi.org/10.1186/s12935-024-03470-7
Share this article
Anyone you share the following link with will be able to read this content:
Sorry, a shareable link is not currently available for this article.
Provided by the Springer Nature SharedIt content-sharing initiative
- Multiple myeloma
- Error-corrected next-generation sequencing
- Circulating tumor DNA
- Plasma cell enrichment
Cancer Cell International
ISSN: 1475-2867
- General enquiries: [email protected]
Thank you for visiting nature.com. You are using a browser version with limited support for CSS. To obtain the best experience, we recommend you use a more up to date browser (or turn off compatibility mode in Internet Explorer). In the meantime, to ensure continued support, we are displaying the site without styles and JavaScript.
- View all journals
- Explore content
- About the journal
- Publish with us
- Sign up for alerts
- Open access
- Published: 06 August 2024
AI and ethics: Investigating the first policy responses of higher education institutions to the challenge of generative AI
- Attila Dabis ORCID: orcid.org/0000-0003-4924-7664 1 &
- Csaba Csáki ORCID: orcid.org/0000-0002-8245-1002 1
Humanities and Social Sciences Communications volume 11 , Article number: 1006 ( 2024 ) Cite this article
544 Accesses
1 Altmetric
Metrics details
- Science, technology and society
This article addresses the ethical challenges posed by generative artificial intelligence (AI) tools in higher education and explores the first responses of universities to these challenges globally. Drawing on five key international documents from the UN, EU, and OECD, the study used content analysis to identify key ethical dimensions related to the use of generative AI in academia, such as accountability, human oversight, transparency, or inclusiveness. Empirical evidence was compiled from 30 leading universities ranked among the top 500 in the Shanghai Ranking list from May to July 2023, covering those institutions that already had publicly available responses to these dimensions in the form of policy documents or guidelines. The paper identifies the central ethical imperative that student assignments must reflect individual knowledge acquired during their education, with human individuals retaining moral and legal responsibility for AI-related wrongdoings. This top-down requirement aligns with a bottom-up approach, allowing instructors flexibility in determining how they utilize generative AI especially large language models in their own courses. Regarding human oversight, the typical response identified by the study involves a blend of preventive measures (e.g., course assessment modifications) and soft, dialogue-based sanctioning procedures. The challenge of transparency induced the good practice of clear communication of AI use in course syllabi in the first university responses examined by this study.
Similar content being viewed by others
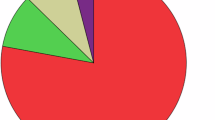
Exploring the impact of artificial intelligence on higher education: The dynamics of ethical, social, and educational implications
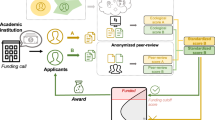
Intersectionality of social and philosophical frameworks with technology: could ethical AI restore equality of opportunities in academia?
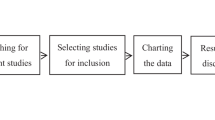
Research on flipped classrooms in foreign language teaching in Chinese higher education
Introduction.
The competition in generative artificial intelligence (AI) ignited by the arrival of ChatGPT, the conversational platform based on a large language model (LLM) in late November 2022 (OpenAI, 2022 ) had a shocking effect even on those who are not involved in the industry (Rudolph et al. 2023 ). Within four months, on 22 March 2023, an open letter was signed by several hundred IT professionals, corporate stakeholders, and academics calling on all AI labs to immediately pause the training of AI systems more powerful than GPT-4 (i.e., those that may trick a human being into believing it is conversing with a peer rather than a machine) for at least six months (Future of Life Institute, 2023 ).
Despite these concerns, competition in generative AI and LLMs does not seem to lose momentum, forcing various social systems to overcome the existential distress they might feel about the changes and the uncertainty of what the future may bring (Roose, 2023 ). Organisations and individuals from different sectors of the economy and various industries are looking for adaptive strategies to accommodate the emerging new normal. This includes lawmakers, international organisations, employers, and employees, as well as academic and higher education institutions (Ray, 2023 ; Wach et al. 2023 ). This fierce competition generates gaps in real-time in everyday and academic life, the latter of which is also trying to make sense of the rapid technological advancement and its effects on university-level education (Perkins, 2023 ). Naturally, these gaps can only be filled, and relevant questions answered much slower by academia, making AI-related research topics timely.
This article aims to reduce the magnitude of these gaps and is intended to help leaders, administrators, teachers, and students better understand the ramifications of AI tools on higher education institutions. It will do so by providing a non-exhaustive snapshot of how various universities around the world responded to generative AI-induced ethical challenges in their everyday academic lives within six-eights months after the arrival of ChatGPT. Thus, the research had asked what expectations and guidelines the first policies introduced into existing academic structures to ensure the informed, transparent, responsible and ethical use of the new tools of generative AI (henceforth GAI) by students and teachers. Through reviewing and evaluating first responses and related difficulties the paper helps institutional decision-makers to create better policies to address AI issues specific to academia. The research reported here thus addressed actual answers to the question of what happened at the institutional (policy) level as opposed to what should happen with the use of AI in classrooms. Based on such a descriptive overview, one may contemplate normative recommendations and their realistic implementability.
Given the global nature of the study’s subject matter, the paper presents examples from various continents. Even though it was not yet a widespread practice to adopt separate, AI-related guidelines, the research focused on universities that had already done so quite early. Furthermore, as best practices most often accrue from the highest-ranking universities, the analysis only considered higher education institutions that were represented among the top 500 universities in the Shanghai Ranking list (containing 3041 Universities at the time), a commonly used source to rank academic excellence. Footnote 1 The main sources of this content analysis are internal documents (such as Codes of Ethics, Academic Regulations, Codes of Practice and Procedure, Guidelines for Students and Teachers or similar policy documents) from those institutions whose response to the GAI challenge was publicly accessible.
The investigation is organised around AI-related ethical dilemmas as concluded from relevant international documents, such as the instruments published by the UN, the EU, and the OECD (often considered soft law material). Through these sources, the study inductively identifies the primary aspects that these AI guidelines mention and can be connected to higher education. Thus it only contains concise references to the main ethical implications of the manifold pedagogical practices in which AI tools can be utilised in the classroom. The paper starts with a review of the challenges posed by AI technology to higher education with special focus on ethical dilemmas. Section 3 covers the research objective and the methodology followed. Section 4 presents the analysis of the selected international documents and establishes a list of key ethical principles relevant in HE contexts and in parallel presents the analysis of the examples distilled from the institutional policy documents and guidelines along that dimension. The paper closes with drawing key conclusions as well as listing limitations and ideas for future research.
Generative AI and higher education: Developments in the literature
General ai-related challenges in the classroom from a historical perspective.
Jacque Ellul fatalistically wrote already in 1954 that the “infusion of some more or less vague sentiment of human welfare” cannot fundamentally alter technology’s “rigorous autonomy”, bringing him to the conclusion that “technology never observes the distinction between moral and immoral use” (Ellul, 1964 , p. 97). Footnote 2 Jumping ahead nearly six decades, the above quote comes to the fore, among others, when evaluating the moral and ethical aspects of the services offered by specific software programs, like ChatGPT. While they might be trained to give ethical answers, these moral barriers can be circumvented by prompt injection (Blalock, 2022 ), or manipulated with tricks (Alberti, 2022 ), so generative AI platforms can hardly be held accountable for the inaccuracy of their responses Footnote 3 or how the physical user who inserted a prompt will make use of the output. Indeed, the AI chatbot is now considered to be a potentially disruptive technology in higher education practices (Farazouli et al. 2024 ).
Educators and educational institution leaders have from the beginning sought solutions on how “to use a variety of the strategies and technologies of the day to help their institutions adapt to dramatically changing social needs” (Miller, 2023 , p. 3). Education in the past had always had high hopes for applying the latest technological advances (Reiser, 2001 ; Howard and Mozejko, 2015 ), including the promise of providing personalised learning or using the latest tools to create and manage courses (Crompton and Burke, 2023 ).
The most basic (and original) educational settings include three components: the blackboard with chalk, the instructor, and textbooks as elementary “educational technologies” at any level (Reiser, 2001 ). Beyond these, one may talk about “educational media” which, once digital technology had entered the picture, have progressed from Computer Based Learning to Learning Management Systems to the use of the Internet, and lately to online shared learning environments with various stages in between including intelligent tutoring system, Dialogue-based Tutoring System, and Exploratory Learning Environment and Artificial Intelligence (Paek and Kim, 2021 ). And now the latest craze is about the generative form of AI often called conversational chatbot (Rudolph et al. 2023 ).
The above-mentioned promises appear to be no different in the case of using generative AI tools in education (Baskara, 2023a ; Mhlanga, 2023 ; Yan et al. 2023 ). The general claim is that GAI chatbots have transformative potential in HE (Mollick and Mollick, 2022 ; Ilieva et al. 2023 ). It is further alleged, that feedback mechanisms supposedly provided by GAI can be used to provide personalised guidance to students (Baskara, 2023b ). Some argue, that “AI education should be expanded and improved, especially by presenting realistic use cases and the real limitations of the technology, so that students are able to use AI confidently and responsibly in their professional future” (Almaraz-López et al. 2023 , p. 1). It is still debated whether the hype is justified, yet the question still remains, how to address the issues arising in the wake of the educational application of GAI tools (Ivanov, 2023 ; Memarian and Doleck, 2023 ).
Generative AI tools, such as their most-known representative, ChatGPT impact several areas of learning and teaching. From the point of view of students, chatbots may help with so-called Self-Regulated or Self-Determined Learning (Nicol and Macfarlane‐Dick, 2006 ; Baskara, 2023b ), where students either dialogue with chatbots or AI help with reviewing student work, even correcting it and giving feedback (Uchiyama et al. 2023 ). There are innovative ideas on how to use AI to support peer feedback (Bauer et al. 2023 ). Some consider that GAI can provide adaptive and personalised environments (Qadir, 2023 ) and may offer personalised tutoring (see, for example, Limo et al. ( 2023 ) on ChatGPT as a virtual tutor for personalized learning experiences). Furthermore, Yan et al. ( 2023 ) lists nine different categories of educational tasks that prior studies have attempted to automate using LLMs: Profiling and labelling (various educational or related content), Detection, Assessment and grading, Teaching support (in various educational and communication activities), Prediction, Knowledge representation, Feedback, Content generation (outline, questions, cases, etc.), Recommendation.
From the lecturers’ point of view, one of the most argued impacts is that assessment practices need to be revisited (Chaudhry et al. 2023 ; Gamage et al. 2023 ; Lim et al. 2023 ). For example, ChatGPT-written responses to exam questions may not be distinguished from student-written answers (Rudolph et al. 2023 ; Farazouli et al. 2024 ). Furthermore, essay-type works are facing special challenges (Sweeney, 2023 ). On the other hand, AI may be utilised to automate a range of educational tasks, such as test question generation, including open-ended questions, test correction, or even essay grading, feedback provision, analysing student feedback surveys, and so on (Mollick and Mollick, 2022 ; Rasul et al. 2023 ; Gimpel et al. 2023 ).
There is no convincing evidence, however, that either lecturers or dedicated tools are able to distinguish AI-written and student-written text with high enough accuracy that can be used to prove unethical behaviour in all cases (Akram, 2023 ). This led to concerns regarding the practicality and ethicality of such innovations (Yan et al. 2023 ). Indeed, the appearance of ChatGPT in higher education has reignited the (inconclusive) debate on the potential and risks associated with AI technologies (Ray, 2023 ; Rudolph et al. 2023 ).
When new technologies appear in or are considered for higher education, debates about their claimed advantages and potential drawbacks heat up as they are expected to disrupt traditional practices and require teachers to adapt to their potential benefits and drawbacks (as collected by Farrokhnia et al. 2023 ). One key area of such debates is the ethical issues raised by the growing accessibility of generative AI and discursive chatbots.
Key ethical challenges posed by AI in higher education
Yan et al. ( 2023 ), while investigating the practicality of AI in education in general, also consider ethicality in the context of educational technology and point out that related debates over the last decade (pre-ChatGPT, so to say), mostly focused on algorithmic ethics, i.e. concerns related to data mining and using AI in learning analytics. At the same time, the use of AI by teachers or, especially, by students has received less attention (or only under the scope or traditional human ethics). However, with the arrival of generative AI chatbots (such as ChatGPT), the number of publications about their use in higher education grew rapidly (Rasul et al. 2023 ; Yan et al. 2023 ).
The study by Chan ( 2023 ) offers a (general) policy framework for higher education institutions, although it focuses on one location and is based on the perceptions of students and teachers. While there are studies that collect factors to be considered for the ethical use of AI in HE, they appear to be restricted to ChatGPT (see, for example, Mhlanga ( 2023 )). Mhlanga ( 2023 ) presents six factors: respect for privacy, fairness, and non-discrimination, transparency in the use of ChatGPT, responsible use of AI (including clarifying its limitations), ChatGPT is not a substitute for human teachers, and accuracy of information. The framework by Chan ( 2023 ) is aimed at creating policies to teach students about GAI and considers three dimensions: pedagogical, governance, and operational. Within those dimensions, ten key areas identified covering ethical concerns such as academic integrity versus academic misconduct and related ethical dilemmas (e.g. cheating or plagiarism), data privacy, transparency, accountability and security, equity in access to AI technologies, critical AI literacy, over-reliance on AI technologies (not directly ethical), responsible use of AI (in general), competencies impeded by AI (such as leadership and teamwork). Baskara ( 2023b ), while also looking at ChatGPT only, considers the following likely danger areas: privacy, algorithmic bias issues, data security, and the potential negative impact of ChatGPT on learners’ autonomy and agency, The paper also questions the possible negative impact of GAI on social interaction and collaboration among learners. Although Yan et al. ( 2023 ) considers education in general (not HE in particular) during its review of 118 papers published since 2017 on the topic of AI ethics in education, its list of areas to look at is still relevant: transparency (of the models used), privacy (related to data collection and use by AI tools), equality (such as availability of AI tools in different languages), and beneficence (e.g. avoiding bias and avoiding biased and toxic knowledge from training data). While systematically reviewing recent publications about AI’s “morality footprint” in higher education, Memarian and Doleck ( 2023 ) consider the Fairness, Accountability, Transparency, and Ethics (FATE) approach as their framework of analyses. They note that “Ethics” appears to be the most used term as it serves as a general descriptor, while the other terms are typically only used in their descriptive sense, and their operationalisation is often lacking in related literature.
Regarding education-related data analytics, Khosravi et al. ( 2022 ) argue that educational technology that involves AI should consider accountability, explainability, fairness, interpretability and safety as key ethical concerns. Ferguson et al. ( 2016 ) also looked at learning analytics solutions using AI and warned of potential issues related to privacy, beneficence, and equality. M.A. Chaudhry et al. ( 2022 ) emphasise that enhancing the comprehension of stakeholders of a new educational AI system is the most important task, which requires making all information and decision processes available to those affected, therefore the key concern is related to transparency according to their arguments.
As such debates continue, it is difficult to identify an established definition of ethical AI in HE. It is clear, however, that the focus should not be on detecting academic misconduct (Rudolph et al. 2023 ). Instead, practical recommendations are required. This is especially true as even the latest studies focus mostly on issues related to assessment practices (Chan, 2023 ; Farazouli et al. 2024 ) and often limit their scope to ChatGPT (Cotton et al. 2024 ) (this specific tool still dominates discourses of LLMs despite the availability of many other solutions since its arrival). At the same time, the list of issues addressed appears to be arbitrary, and most publications do not look at actual practices on a global scale. Indeed, reviews of actual current practices of higher education institutions are rare, and this aspect is not yet the focus of recent HE AI ethics research reports.
As follows from the growing literature and the debate shaping up about the implications of using GAI tools in HE, there was a clear need for a systematic review of how first responses in actual academic policies and guidelines in practice have represented and addressed known ethical principles.
Research objective and methodology
In order to contribute to the debate on the impact of GAI on HE, this study aimed to review how leading institutions had reacted to the arrival of generative AI (such as ChatGPT) and what policies or institutional guidelines they have put in place shortly after. The research intended to understand whether key ethical principles were reflected in the first policy responses of HE institutions and, if yes, how they were handled.
As potential principles can diverge and could be numerous, as well as early guidelines may cover wide areas, the investigation is intended to be based on a few broad categories instead of trying to manage a large set of ideals and goals. To achieve this objective, the research was executed in three steps:
It was started with identifying and collecting general ethical ideals, which were then translated and structured for the context of higher education. A thorough content analysis was performed with the intention to put emphasis on positive values instead of simply focusing on issues or risks and their mitigation.
Given those positive ideals, this research collected actual examples of university policies and guidelines already available: this step was executed from May to July 2023 to find early responses addressing such norms and principles developed by leading HE institutions.
The documents identified were then analysed to understand how such norms and principles had been addressed by leading HE institutions.
As a result, this research managed to highlight and contrast differing practical views, and the findings raise awareness about the difficulties of creating relevant institutional policies. The research considered the ethics of using GAI and not expectations towards their development. The next two sections provide details of the two steps.
Establishing ethical principles for higher education
While the review of relevant ethical and HE literature (as presented above) was not fully conclusive, it highlighted the importance and need for some ideals specific to HE. Therefore, as a first step, this study sought to find highly respected sources of such ethical dimensions by executing a directed content analysis of relevant international regulatory and policy recommendations.
In order to establish what key values and ideas drive the formation of future AI regulations in general, Corrêa et al. ( 2023 ) investigated 200 publications discussing governance policies and ethical guidelines for using AI as proposed by various organisations (including national governments and institutions, civil society and academic organisations, private companies, as well as international bodies). The authors were also interested in whether there are common patterns or missing ideals and norms in this extensive set of proposals and recommendations. As the research was looking for key principles and normative attributes that could form a common ground for the comparison of HE policies, this vast set of documents was used to identify internationally recognised bodies that have potential real influence in this arena and decided to consider the guidelines and recommendations they have put forward for the ethical governance of AI. Therefore, for the purpose of this study, the following sources were selected (some organisations, such as the EU were represented by several bodies):
European Commission ( 2021 ): Regulation of the European Parliament and of the Council Laying Down Harmonised Rules on Artificial Intelligence (Artificial Intelligence Act) and Amending Certain Union Legislative Acts (2021/0106 (COD)) . Footnote 4
European Parliament Committee on Culture and Education ( 2021 ): Report on artificial intelligence in education, culture and the audiovisual sector (2020/2017(INI)) . Footnote 5
High-Level Expert Group on Artificial Intelligence (EUHLEX) ( 2019 ): Ethics Guidelines for Trustworthy AI . Footnote 6
UNESCO ( 2022 ): Recommendation on the Ethics of Artificial Intelligence (SHS/BIO/PI/2021/1) . Footnote 7
OECD ( 2019 ): Recommendation of the Council on Artificial Intelligence (OECD/LEGAL/0449) . Footnote 8
The ethical dilemmas established by these international documents (most of which is considered soft law material) were then used to inductively identify the primary aspects around which the investigation of educational AI principles may be organised.
Among the above documents, the EUHLEX material is the salient one as it contains a Glossary that defines and explains, among others, the two primary concepts that will be used in this paper: “artificial intelligence” and “ethics”. As this paper is, to a large extent, based on the deducted categorisation embedded in these international documents, it will follow suit in using the above terms as EUHLEX did, supporting it with the definitions contained in the other four referenced international documents. Consequently, artificial intelligence (AI) systems are referred to in this paper as software and hardware systems designed by humans that “act in the physical or digital dimension by perceiving their environment through data acquisition, interpreting the collected structured or unstructured data, reasoning on the knowledge, or processing the information, derived from this data and deciding the best action(s) to take to achieve the given goal” (EUHLEX, 2019 ). With regards to ethics, the EUHLEX group defines this term, in general as an academic discipline which is a subfield of philosophy, dealing with questions like “What is a good action?”, “What is the value of a human life?”, “What is justice?”, or “What is the good life?”. It also mentions that academia distinguishes four major fields: (i) Meta-ethics, (ii) normative ethics, (iii) descriptive ethics, and (iv) applied ethics ” (EUHLEX, 2019 , p. 37). Within these, AI ethics belongs to the latter group of applied ethics that focuses on the practical issues raised by the design, development, implementation, and use of AI systems. By extension, the application of AI systems in higher education also falls under the domain of applied ethics.
The selection of sample universities
The collection of cases started with the AI guidelines compiled by the authors as members of the AI Committee at their university from May to July 2023. The AI Committee consisted of 12 members and investigated over 150 cases to gauge international best practices of GAI use in higher education when formulating a policy recommendation for their own university leadership. Given the global nature of the subject matter, examples from various continents were collected. From this initial pool authors narrowed the scope to the Top 500 higher education institutions of the Shanghai Ranking list for this study, as best practices most often accrue from the highest-ranking universities. Finally, only those institutions were included which, at the time of data collection, have indeed had publicly available policy documents or guidelines with clearly identifiable ethical considerations (such as relevant internal documents, Codes of Ethics, Academic Regulations, Codes of Practice and Procedure, or Guidelines for Students and Teachers). By the end of this selection process, 30 samples proved to be substantiated enough to be included in this study (presented in Table 1 ).
All documents were contextually analysed and annotated by both authors individually looking for references or mentions of ideas, actions or recommendations related to the ethical principles identified during the first step of the research. These comments were then compared and commonalities analysed regarding the nature and goal of the ethical recommendation.
Principles and practices of responsible use of AI in higher education
Ai-related ethical codes forming the base of this investigation.
A common feature of the selected AI ethics documents issued by international organisations is that they enumerate a set of ethical principles based on fundamental human values. The referenced international documents have different geographical- and policy scopes, yet they overlap in their categorisation of the ethical dimensions relevant to this research, even though they might use discrepant language to describe the same phenomenon (a factor we took into account when establishing key categories). For example, what EUHLEX dubs as “Human agency and oversight” is addressed by UNESCO under the section called “Human oversight and determination”, yet they essentially cover the same issues and recommended requirements. Among the many principles enshrined in these documents, the research focuses on those that can be directly linked to the everyday education practices of universities in relation to AI tools, omitting those that, within this context, are less situation-dependent and should normally form the overarching basis of the functioning of universities at all times, such as: respecting human rights and fundamental freedoms, refraining from all forms of discrimination, the right to privacy and data protection, or being aware of environmental concerns and responsibilities regarding sustainable development. As pointed out by Nikolinakos ( 2023 ), such principles and values provide essential guidance not only for development but also during the deployment and use of AI systems. Synthesising the common ethical codes in these instruments has led to the following cluster of ethical principles that are directly linked to AI-related higher education practices:
Accountability and responsibility;
Human agency and oversight;
Transparency and explainability
Inclusiveness and diversity.
The following subsections will give a comprehensive definition of these ethical areas and relate them to higher education expectations. Each subsection will first explain the corresponding ethical cluster, then present the specific university examples, concluding with a summary of the identified best practice under that particular cluster.
Accountability and responsibility
Definition in ethical codes and relevance.
The most fundamental requirements, appearing in almost all relevant documents, bring forward the necessity that mechanisms should be implemented to ensure responsibility and accountability for AI systems and their outcomes. These cover expectations both before and after their deployment, including development and use. They entail the basic requirements of auditability (i.e. the enablement of the assessment of algorithms), clear roles in the management of data and design processes (as a means for contributing to the trustworthiness of AI technology), the minimalisation and reporting of negative impacts (focusing on the possibility of identifying, assessing, documenting and reporting on the potential negative impacts of AI systems), as well as the ability of redress (understood as the capability to utilise mechanisms that offer legal and practical remedy when unjust adverse impact occurs) (EUHLEX, 2019 , pp. 19–20).
Additionally, Points 35–36 of the UNESCO recommendations remind us that it is imperative to “attribute ethical and legal responsibility for any stage of the life cycle of AI systems, as well as in cases of remedy related to AI systems, to physical persons or to existing legal entities. AI system can never replace ultimate human responsibility and accountability” (UNESCO, 2022 , p. 22).
The fulfilment of this fundamental principle is also expected from academic authors, as per the announcements of some of the largest publishing houses in the world. Accordingly, AI is not an author or co-author, Footnote 9 and AI-assisted technologies should not be cited as authors either, Footnote 10 given that AI-generated content cannot be considered capable of initiating an original piece of research without direction from human authors. The ethical guidelines of Wiley ( 2023 ) stated that ”[AI tools] also cannot be accountable for a published work or for research design, which is a generally held requirement of authorship, nor do they have legal standing or the ability to hold or assign copyright.” Footnote 11 This research angle carries over to teaching as well since students are also expected to produce outputs that are the results of their own work. Furthermore, they also often do their own research (such as literature search and review) in support of their projects, homework, thesis, and other forms of performance evaluation.
Accountability and responsibility in university first responses
The rapidly changing nature of the subject matter poses a significant challenge for scholars to assess the state of play of human responsibility. This is well exemplified by the reversal of hearts by some Australian universities (see Rudolph et al. ( 2023 ) quoting newspaper articles) who first disallowed the use of AI by students while doing assignments, just to reverse that decision a few months later and replace it by a requirement of disclosing the use of AI in homeworks. Similarly, Indian governments have been oscillating between a non-regulatory approach to foster an “innovation-friendly environment” for their universities in the summer of 2023 (Liu, 2023 ), only to roll back on this pledge a few months later (Dhaor, 2023 ).
Beyond this regulatory entropy, a fundamental principle enshrined in university codes of ethics across the globe is that students need to meet existing rules of scientific referencing and authorship. Footnote 12 In other words, they should refrain from any form of plagiarism in all their written work (including essays, theses, term papers, or in-class presentations). Submitting any work and assessments created by someone or something else (including AI-generated content) as if it was their own usually amounts to either a violation of scientific referencing, plagiarism or is considered to be a form of cheating (or a combination of these), depending on the terminology used by the respective higher education institution.
As a course description of Johns Hopkins puts it, “academic honesty is required in all work you submit to be graded …., you must solve all homework and programming assignments without the help of outside sources (e.g., GAI tools)” (Johns Hopkins University, 2023 ).
The Tokyo Institute of Technology applies a more flexible approach, as they “trust the independence of the students and expect the best use” of AI systems from them based on good sense and ethical standards. They add, however, that submitting reports that rely almost entirely on the output of GenAI is “highly improper, and its continued use is equivalent to one’s enslavement to the technology” (Tokyo Institute of Technology, 2023 ).
In the case of York University, the Senate’s Academic Standards, Curriculum, and Pedagogy Committee clarified in February 2023 that students are not authorised to use “text-, image-, code-, or video-generating AI tools when completing their academic work unless explicitly permitted by a specific instructor in a particular course” (York University Senate, 2023 ).
In the same time frame (6 February 2023), the University of Oxford stated in a guidance material for staff members that “the unauthorised use of AI tools in exams and other assessed work is a serious disciplinary offence” not permitted for students (University of Oxford, 2023b ).
Main message and best practice: honesty and mutual trust
In essence, students are not allowed to present AI-generated content as their own, Footnote 13 and they should have full responsibility and accountability for their own papers. Footnote 14 This is in line with the most ubiquitous principle enshrined in almost all university guidelines, irrespective of AI, that students are expected to complete their tasks based on their own knowledge and skills obtained throughout their education.
Given that the main challenge here is unauthorised use and overreliance on GAI platforms, the best practice answer is for students to adhere to academic honesty and integrity, scientific referencing standards, existing anti-plagiarism rules, and complete university assignments without fully relying on GAI tools, using, first and foremost, their own skills. The only exception is when instructed otherwise by their professors. By extension, preventing overuse and unauthorised use of AI assists students in avoiding undermining their own academic capacity-building efforts.
Human agency and oversight
AI systems have the potential to manipulate and influence human behaviour in ways that are not easily detectable. AI systems must, therefore, follow human-centric design principles and leave meaningful opportunities for human choice and intervention. Such systems should not be able to unjustifiably subordinate, coerce, deceive, manipulate, condition or herd humans (EUHLEX, 2019 , p. 16).
Human oversight thus refers to the capability for human intervention in every decision cycle of the AI system and the ability of users to make informed, autonomous decisions regarding AI systems. This encompasses the ability to choose not to use an AI system in a particular situation or to halt AI-related operations via a “stop” button or a comparable procedure in case the user detects anomalies, dysfunctions and unexpected performance from AI tools (European Commission, 2021 , Art. 14).
The sheer capability of active oversight and intervention vis-á-vis GAI systems is strongly linked to ethical responsibility and legal accountability. As Liao puts it, “the sufficient condition for human beings being rightsholders is that they have a physical basis for moral agency.” (Liao, 2020 , pp. 496–497). Wagner complemented this with the essential point that entity status for non-human actors would help to shield other parties from liability, i.e., primarily manufacturers and users (Wagner, 2018 ). This, in turn, would result in risk externalisation, which serves to minimise or relativise a person’s moral accountability and legal liability associated with wrongful or unethical acts.
Users, in our case, are primarily students who, at times, might be tempted to make use of AI tools in an unethical way, hoping to fulfil their university tasks faster and more efficiently than they could without these.
Human agency and oversight in university first responses
The crucial aspect of this ethical issue is the presence of a “stop” button or a similar regulatory procedure to streamline the operation of GAI tools. Existing university guidelines in this question point clearly in the direction of soft sanctions, if any, given the fact that there is a lack of evidence that AI detection platforms are effective and reliable tools to tell apart human work from AI-generated ones. Additionally, these tools raise some significant implications for privacy and data security issues, which is why university guidelines are particularly cautious when referring to these. Accordingly, the National Taiwan University, the University of Toronto, the University of Waterloo, the University of Miami, the National Autonomous University of Mexico, and Yale, among others, do not recommend the use of AI detection platforms in university assessments. The University of Zürich further added the moral perspective in a guidance note from 13 July 2023, that “forbidding the use of undetectable tools on unsupervised assignments or demanding some sort of honour code likely ends up punishing the honest students” (University of Zürich, 2023 ). Apart from unreliability, the University of Cape Town also drew attention in its guide for staff that AI detection tools may “disproportionately flag text written by non-first language speakers as AI-generated” (University of Cape Town, 2023 , p. 8).
Macquarie University took a slightly more ambiguous stance when they informed their staff that, while it is not “proof” for anything, an AI writing detection feature was launched within Turnitin as of 5 April 2023 (Hillier, 2023 ), claiming that the software has a 97% detection rate with a 1% false positive rate in the tests that they had conducted (Turnitin, 2023 ). Apart from these, Boston University is among the few examples that recommend employing AI detection tools, but only in a restricted manner to ”evaluate the degree to which AI tools have likely been employed” and not as a source for any punitive measures against students (University of Boston, 2023 ). Remarkably, they complement the above with suggestions for a merit-based scoring system, whereby instructors shall treat work by students who declare no use of AI tools as the baseline for grading. A lower baseline is suggested for students who declare the use of AI tools (depending on how extensive the usage was), and for the bottom of this spectrum, the university suggests imposing a significant penalty for low-energy or unreflective reuse of material generated by AI tools and assigning zero points for merely reproducing the output from AI platforms.
A discrepant approach was adopted at the University of Toronto. Here, if an instructor indicates that the use of AI tools is not permitted on an assessment, and a student is later found to have used such a tool nevertheless, then the instructor should consider meeting with the student as the first step of a dialogue-based process under the Code of Behaviour on Academic Matters (the same Code, which categorises the use of ChatGPT and other such tools as “unauthorised aid” or as “any other form of cheating” in case, an instructor specified that no outside assistance was permitted on an assignment) (University of Toronto, 2019 ).
More specifically, Imperial College London’s Guidance on the Use of Generative AI tools envisages the possibility of inviting a random selection of students to a so-called “authenticity interview” on their submitted assignments (Imperial College London, 2023b ). This entails requiring students to attend an oral examination of their submitted work to ensure its authenticity, which includes questions about the subject or how they approached their assignment.
As a rare exception, the University of Helsinki represents one of the more rigorous examples. The “Guidelines for the Use of AI in Teaching at the University of Helsinki” does not lay down any specific procedures for AI-related ethical offences. On the contrary, as para. 7 stipulates the unauthorised use of GAI in any course examination “constitutes cheating and will be treated in the same way as other cases of cheating” (University of Helsinki, 2023 ). Footnote 15
Those teachers who are reluctant to make AI tools a big part of their courses should rather aim to develop course assessment methods that can plausibly prevent the use of AI tools instead of attempting to filter these afterwards. Footnote 16 For example, the Humboldt-Universität zu Berlin instructs that, if possible, oral or practical examinations or written examinations performed on-site are recommended as alternatives to “classical” written home assignments (Humboldt-Universität zu Berlin, 2023a ).
Monash University also mentions some examples in this regard (Monash University, 2023a ), such as: asking students to create oral presentations, videos, and multimedia resources; asking them to incorporate more personal reflections tied to the concepts studied; implementing programmatic assessment that focuses on assessing broader attributes of students, using multiple methods rather than focusing on assessing individual kinds of knowledge or skills using a single assessment method (e.g., writing an essay).
Similarly, the University of Toronto suggest instructors to: ask students to respond to a specific reading that is very new and thus has a limited online footprint; assign group work to be completed in class, with each member contributing; or ask students to create a first draft of an assignment by hand, which could be complemented by a call to explain or justify certain elements of their work (University of Toronto, 2023 ).
Main message and best practice: Avoiding overreaction
In summary, the best practice that can be identified under this ethical dilemma is to secure human oversight through a blend of preventive measures (e.g. a shift in assessment methods) and soft sanctions. Given that AI detectors are unreliable and can cause a series of data privacy issues, the sanctioning of unauthorised AI use should happen on a “soft basis”, as part of a dialogue with the student concerned. Additionally, universities need to be aware and pay due attention to potentially unwanted rebound effects of bona fide measures, such as the merit-based scoring system of the University of Boston. In that case, using different scoring baselines based on the self-declared use of AI could, in practice, generate incentives for not declaring any use of AI at all, thereby producing counter-effective results.
While explainability refers to providing intelligible insight into the functioning of AI tools with a special focus on the interplay between the user’s input and the received output, transparency alludes to the requirement of providing unambiguous communication in the framework of system use.
As the European Commission’s Regulation proposal ( 2021 ) puts it under subchapter 5.2.4., transparency obligations should apply for systems that „(i) interact with humans, (ii) are used to detect emotions or determine association with (social) categories based on biometric data, or (iii) generate or manipulate content (‘deep fakes’). When persons interact with an AI system or their emotions or characteristics are recognised through automated means, people must be informed of that circumstance. If an AI system is used to generate or manipulate image, audio or video content that appreciably resembles authentic content, there should be an obligation to disclose that the content is generated through automated means, subject to exceptions for legitimate purposes (law enforcement, freedom of expression). This allows persons to make informed choices or step back from a given situation.”
People (in our case, university students and teachers) should, therefore, be fully informed when a decision is influenced by or relies on AI algorithms. In such instances, individuals should be able to ask for further explanation from the decision-maker using AI (e.g., a university body). Furthermore, individuals should be afforded the choice to present their case to a dedicated representative of the organisation in question who should have the power to reviset the decision and make corrections if necessary (UNESCO, 2022 , p. 22). Therefore, in the context of courses and other related education events, teachers should be clear about their utilisation of AI during the preparation of the material. Furthermore, instructors must unambiguously clarify ethical AI use in the classroom. Clear communication is essential about whether students have permission to utilise AI tools during assignments and how to report actual use.
As both UN and EU sources point out, raising awareness about and promoting basic AI literacy should be fostered as a means to empower people and reduce the digital divides and digital access inequalities resulting from the broad adoption of AI systems (EUHLEX, 2019 , p. 23; UNESCO, 2022 , p. 34).
Transparency and explainability in university first responses
The implementation of this principle seems to revolve around the challenge of decentralisation of university work, including the respect for teachers’ autonomy.
Teachers’ autonomy entails that teachers can decide if and to what extent they will allow their students to use AI platforms as part of their respective courses. This, however, comes with the essential corollary, that they must clearly communicate their decision to both students and university management in the course syllabus. To support transparency in this respect, many universities decided to establish 3-level- or 4-level admissibility frameworks (and even those who did not establish such multi-level systems, e.g., the University of Toronto, urge instructors to explicitly indicate in the course syllabus the expected use of AI) (University of Toronto, 2023 ).
The University of Auckland is among the universities that apply a fully laissez passer laissez-faire approach in this respect, meaning that there is a lack of centralised guidance or recommendations on this subject. They rather confer all practical decision-making of GAI use on course directors, adding that it is ultimately the student’s responsibility to correctly acknowledge the use of Gen-AI software (University of Auckland, 2023 ). Similarly, the University of Helsinki gives as much manoeuvring space to their staff as to allow them to change the course of action during the semester. As para 1 of their earlier quoted Guidelines stipulates, teachers are responsible for deciding how GAI can be used on a given course and are free to fully prohibit their use if they think it impedes the achievement of the learning objectives.
Colorado State University, for example, provides its teachers with 3 types of syllabus statement options (Colorado State University, 2023 ): (a) the prohibitive statement: whereby any work created, or inspired by AI agents is considered plagiarism and will not be tolerated; (b) the use-with-permission statement: whereby generative AI can be used but only as an exception and in line with the teachers further instruction, and (c) the abdication statement: where the teacher acknowledges that the course grade will also be a reflection of the students ability to harness AI technologies as part of their preparation for their future in a workforce that will increasingly require AI-literacy.
Macquarie University applies a similar system and provides it’s professors with an Assessment Checklist in which AI use can be either “Not permitted” or “Some use permitted” (meaning that the scope of use is limited while the majority of the work should be written or made by the student.), or “Full use permitted (with attribution)”, alluding to the adaptive use of AI tools, where the generated content is edited, mixed, adapted and integrated into the student’s final submission – with attribution of the source (Macquarie University, 2023 ).
The same approach is used at Monash University where generative AI tools can be: (a) used for all assessments in a specific unit; (b) cannot be used for any assessments; (c) some AI tools may be used selectively (Monash University, 2023b ).
The University of Cape Town (UCT) applies a 3-tier system not just in terms of the overall approach to the use or banning of GAI, but also with regard to specific assessment approaches recommended to teachers. As far as the former is concerned, they differentiate between the strategies of: (a) Avoiding (reverting to in-person assessment, where the use of AI isn’t possible); (b) Outrunning (devising an assessment that AI cannot produce); and (c) Embracing (discussing the appropriate use of AI with students and its ethical use to create the circumstances for authentic assessment outputs). The assessment possibilities, in turn, are categorised into easy, medium, and hard levels. Easy tasks include, e.g., generic short written assignments. Medium level might include examples such as personalised or context-based assessments (e.g. asking students to write to a particular audience whose knowledge and values must be considered or asking questions that would require them to give a response that draws from concepts that were learnt in class, in a lab, field trip…etc). In contrast, hard assessments include projects involving real-world applications, synchronous oral assessments, or panel assessments (University of Cape Town, 2023 ).
4-tier-systems are analogues. The only difference is that they break down the “middle ground”. Accordingly, the Chinese University of Hong Kong clarifies that Approach 1 (by default) means the prohibition of all use of AI tools; Approach 2 entails using AI tools only with prior permission; Approach 3 means using AI tools only with explicit acknowledgement; and Approach 4 is reserved for courses in which the use of AI tools is freely permitted with no acknowledgement needed (Chinese University of Hong Kong, 2023 ).
Similarly, the University of Delaware provides course syllabus statement examples for teachers including: (1) Prohibiting all use of AI tools; (2) Allowing their use only with prior permission; (3) Allow their use only with explicit acknowledgement; (4) Freely allow their use (University of Delaware, 2023 ).
The Technical University of Berlin also proposes a 4-tier system but uses a very different logic based on the practical knowledge one can obtain by using GAI. Accordingly, they divide AI tools as used to: (a) acquire professional competence; (b) learn to write scientifically; (c) be able to assess AI tools and compare them with scientific methods; d) professional use of AI tools in scientific work. Their corresponding guideline even quotes Art. 5 of the German Constitution referencing the freedom of teaching ( Freiheit der Lehre ), entailing that teachers should have the ability to decide for themselves which teaching aids they allow or prohibit. Footnote 17
This detailed approach, however, is rather the exception. According to the compilation on 6 May 2023 by Solis ( 2023 ), among the 100 largest German universities, 2% applied a general prohibition on the use of ChatGPT, 23% granted partial permission, 12% generally permitted its use, while 63% of the universities had none or only vague guidelines in this respect.
Main message and best practice: raising awareness
Overall, the best practice answer to the dilemma of transparency is the internal decentralisation of university work and the application of a “bottom-up” approach that respects the autonomy of university professors. Notwithstanding the potential existence of regulatory frameworks that set out binding rules for all citizens of an HE institution, this means providing university instructors with proper manoeuvring space to decide on their own how they would like to make AI use permissible in their courses, insofar as they communicate their decision openly.
Inclusiveness and diversity
Para. 34 of the Report by the European Parliament Committee on Culture and Education ( 2021 ) highlights that inclusive education can only be reached with the proactive presence of teachers and stresses that “AI technologies cannot be used to the detriment or at the expense of in-person education, as teachers must not be replaced by any AI or AI-related technologies”. Additionally, para. 20 of the same document highlights the need to create diverse teams of developers and engineers to work alongside the main actors in the educational, cultural, and audiovisual sectors in order to prevent gender or social bias from being inadvertently included in AI algorithms, systems, and applications.
This approach also underlines the need to consider the variety of different theories through which AI has been developed as a precursor to ensuring the application of the principle of diversity (UNESCO, 2022 , pp. 33–35), and it also recognises that a nuanced answer to AI-related challenges is only possible if affected stakeholders have an equal say in regulatory and design processes. An idea closely linked to the principle of fairness and the pledge to leave no one behind who might be affected by the outcome of using AI systems (EUHLEX, 2019 , pp. 18–19).
Therefore, in the context of higher education, the principle of inclusiveness aims to ensure that an institution provides the same opportunities to access the benefits of AI technologies for all its students, irrespective of their background, while also considering the particular needs of various vulnerable groups potentially marginalised based on age, gender, culture, religion, language, or disabilities. Footnote 18 Inclusiveness also alludes to stakeholder participation in internal university dialogues on the use and impact of AI systems (including students, teachers, administration and leadership) as well as in the constant evaluation of how these systems evolve. On a broader scale, it implies communication with policymakers on how higher education should accommodate itself to this rapidly changing environment (EUHLEX, 2019 , p. 23; UNESCO, 2022 , p. 35).
Inclusiveness and diversity in university first responses
Universities appear to be aware of the potential disadvantages for students who are either unfamiliar with GAI or who choose not to use it or use it in an unethical manner. As a result, many universities thought that the best way to foster inclusive GAI use was to offer specific examples of how teachers could constructively incorporate these tools into their courses.
The University of Waterloo, for example, recommends various methods that instructors can apply on sight, with the same set of tools for all students during their courses, which in itself mitigates the effects of any discrepancies in varying student backgrounds (University of Waterloo, 2023 ): (a) Give students a prompt during class, and the resulting text and ask them to critique and improve it using track changes; (b) Create two distinct texts and have students explain the flaws of each or combine them in some way using track changes; (c) Test code and documentation accuracy with a peer; or (d) Use ChatGPT to provide a preliminary summary of an issue as a jumping-off point for further research and discussion.
The University of Pittsburgh ( 2023 ) and Monash added similar recommendations to their AI guidelines (Monash University, 2023c ).
The University of Cambridge mentions under its AI-deas initiative a series of projects aimed to develop new AI methods to understand and address sensory, neural or linguistic challenges such as hearing loss, brain injury or language barriers to support people who find communicating a daily challenge in order to improve equity and inclusion. As they put it, “with AI we can assess and diagnose common language and communication conditions at scale, and develop technologies such as intelligent hearing aids, real-time machine translation, or other language aids to support affected individuals at home, work or school.” (University of Cambridge, 2023 ).
The homepage of the Technical University of Berlin (Technische Universität Berlin) displays ample and diverse materials, including videos Footnote 19 and other documents, as a source of inspiration for teachers on how to provide an equitable share of AI knowledge for their students (Glathe et al. 2023 ). More progressively, the university’s Institute of Psychology offers a learning modul called “Inclusive Digitalisation”, available for students enrolled in various degree programmes to understand inclusion and exclusion mechanisms in digitalisation. This modul touches upon topics such as barrier-free software design, mechanisms and reasons for digitalised discrimination or biases in corporate practices (their homepage specifically alludes to the fact that input and output devices, such as VR glasses, have exclusively undergone testing with male test subjects and that the development of digital products and services is predominantly carried out by men. The practical ramifications of such a bias result in input and output devices that are less appropriate for women and children) (Technische Universität Berlin, 2023 ).
Columbia recommends the practice of “scaffolding”, which is the process of breaking down a larger assignment into subtasks (Columbia University, 2023 ). In their understanding, this method facilitates regular check-ins and enables students to receive timely feedback throughout the learning process. Simultaneously, the implementation of scaffolding helps instructors become more familiar with students and their work as the semester progresses, allowing them to take additional steps in the case of students who might need more attention due to their vulnerable backgrounds or disabilities to complete the same tasks.
The Humboldt-Universität zu Berlin, in its Recommendations, clearly links the permission of GAI use with the requirement of equal accessibility. They remind that if examiners require students to use AI for an examination, “students must be provided with access to these technologies free of charge and in compliance with data protection regulations” (Humboldt-Universität zu Berlin, 2023b ).
Concurringly, the University of Cape Town also links inclusivity to accessibility. As they put it, “there is a risk that those with poorer access to connectivity, devices, data and literacies will get unequal access to the opportunities being provided by AI”, leading to the conclusion that the planning of the admissible use of GAI on campus should be cognizant of access inequalities (University of Cape Town, 2023 ). They also draw their staff’s attention to a UNESCO guide material containing useful methods to incorporate ChatGPT into the course, including methods such as the “Socratic opponent” (AI acts as an opponent to develop an argument), the “study buddy” (AI helps the student reflect on learning material) or the “dynamic assessor” (AI provides educators with a profile of each student’s current knowledge based on their interactions with ChatGPT) (UNESCO International Institute for Higher Education in Latin America and the Caribbean, 2023 ).
Finally, the National Autonomous University of Mexico’s Recommendations suggest using GAI tools, among others, for the purposes of community development. They suggest that such community-building activities, whether online or in live groups, kill two birds with one stone. On the one hand, they assist individuals in keeping their knowledge up to date with a topic that is constantly evolving, while it offers people from various backgrounds the opportunity to become part of communities in the process where they can share their experiences and build new relations (National Autonomous University of Mexico, 2023 ).
Main message and best practice: Proactive central support and the pledge to leave no one behind
To conclude, AI-related inclusivity for students is best fostered if the university does not leave its professors solely to their own resources to come up with diverging initiatives. The best practice example for this dilemma thus lies in a proactive approach that results in the elaboration of concrete teaching materials (e.g., subscriptions to AI tools to ensure equal accessibility for all students, templates, video tutorials, open-access answers to FAQs…etc.), specific ideas, recommendations and to support specialised programmes and collaborations with an inclusion-generating edge. With centrally offered resources and tools institutions seem to be able to ensure accessability irrespective of students’ background and financial abilities.
Discussion of the First Responses
While artificial intelligence and even its generative form has been around for a while, the arrival of application-ready LLMs – most notably ChatGPT has changed the game when it comes to grammatically correct large-scale and content-specific text generation. This has invoked an immediate reaction from the higher education community as the question arose as to how it may affect various forms of student performance evaluation (such as essay and thesis writing) (Chaudhry et al. 2023 ; Yu, 2023 ; Farazouli et al. 2024 ).
Often the very first reaction (a few months after the announcement of the availability of ChatGPT) was a ban on these tools and a potential return to hand-written evaluation and oral exams. In the institutions investigated under this research, notable examples may be most Australian universities (such as Monash) or even Oxford. On the other hand, even leading institutions have immediately embraced this new tool as a great potential helper of lecturers – the top name here being Harvard. Very early responses thus ranged widely – and have changed fast over the first six-eight months “post-ChatGPT”.
Over time responses from the institutions investigated started to put out clear guidelines and even created dedicated policies or modified existing ones to ensure a framework of acceptable use. The inspiration leading these early regulatory efforts was influenced by the international ethics documents reviewed in this paper. Institutions were aware of and relied on those guidelines. The main goal of this research was to shed light on the questions of how much and in what ways they took them on board regarding first responses. Most first reactions were based on “traditional” AI ethics and understanding of AI before LLMs and the generative revolution. First responses by institutions were not based on scientific literature or arguments from journal publications. Instead, as our results demonstrated it was based on publicly available ethical norms and guidelines published by well-known international organizations and professional bodies.
Conclusions, limitations and future research
Ethical dilemmas discussed in this paper were based on the conceptualisation embedded in relevant documents of various international fora. Each ethical dimension, while multifaceted in itself, forms a complex set of challenges that are inextricably intertwined with one another. Browsing university materials, the overall impression is that Universities primarily aim to explore and harness the potential benefits of generative AI but not with an uncritical mindset. They are focusing on the opportunities while simultaneously trying to address the emerging challenges in the field.
Accordingly, the main ethical imperative is that students must complete university assignments based on the knowledge and skills they acquired during their university education unless their instructors determine otherwise. Moral and legal responsibility in this regard always rests with human individuals. AI agents possess neither the legal standing nor the physical basis for moral agency, which makes them incapable of assuming such responsibilities. This “top-down” requirement is most often complemented by the “bottom-up” approach of providing instructors with proper maneuvering space to decide how they would like to make AI use permissible in their courses.
Good practice in human oversight could thus be achieved through a combination of preventive measures and soft, dialogue-based procedures. This latter category includes the simple act of teachers providing clear, written communications in their syllabi and engaging in a dialogue with their students to provide unambiguous and transparent instructions on the use of generative AI tools within their courses. Additionally, to prevent the unauthorised use of AI tools, changing course assessment methods by default is more effective than engaging in post-assessment review due to the unreliability of AI detection tools.
Among the many ethical dilemmas that generative AI tools pose to social systems, this paper focused on those pertaining to the pedagogical aspects of higher education. Due to this limitation, related fields, such as university research, were excluded from the scope of the analysis. However, research-related activities are certainly ripe for scientific scrutiny along the lines indicated in this study. Furthermore, only a limited set of institutions could be investigated, those who were the ”first respondents” to the set of issues covered by this study. Hereby, this paper hopes to inspire further research on the impact of AI tools on higher education. Such research could cover more institutions, but it would also be interesting to revisit the same institutions again to see how their stance and approach might have changed over time considering how fast this technology evolves and how much we learn about its capabilities and shortcomings.
Data availability
Data sharing is not applicable to this article as no datasets were generated or analysed during the current study. All documents referenced in this study are publicly available on the corresponding websites provided in the Bibliography or in the footnotes. No code has been developed as part of this research.
For the methodology behind the Shanghai Rankings see: https://www.shanghairanking.com/methodology/arwu/2022 . Accessed: 14 November 2023.
While the original French version was published in 1954, the first English translation is dated 1964.
As the evaluation by Bang et al. ( 2023 ) found, ChatGPT is only 63.41% accurate on average in ten different reasoning categories under logical reasoning, non-textual reasoning, and common-sense reasoning, making it an unreliable reasoner.
Source: https://digital-strategy.ec.europa.eu/en/library/proposal-regulation-laying-down-harmonised-rules-artificial-intelligence . Accessed: 14 November 2023.
Source https://www.europarl.europa.eu/doceo/document/A-9-2021-0127_EN.html . Accessed: 14 November 2023.
Source: https://digital-strategy.ec.europa.eu/en/library/ethics-guidelines-trustworthy-ai . Accessed: 14 November 2023.
Source: https://unesdoc.unesco.org/ark:/48223/pf0000381137 . Accessed: 14 November 2023.
Source: https://legalinstruments.oecd.org/en/instruments/OECD-LEGAL-0449#mainText . Accessed: 14 November 2023.
The editors-in-chief of Nature and Science stated that ChatGPT does not meet the standard for authorship: „ An attribution of authorship carries with it accountability for the work, which cannot be effectively applied to LLMs…. We would not allow AI to be listed as an author on a paper we published, and use of AI-generated text without proper citation could be considered plagiarism,” (Stokel-Walker, 2023 ). See also (Nature, 2023 ).
While there was an initial mistake that credited ChatGPT as an author of an academic paper, Elsevier issued a Corrigendum on the subject in February 2023 (O’Connor, 2023 ). Elsevier then clarified in its “Use of AI and AI-assisted technologies in writing for Elsevier” announcement, issued in March 2023, that “Authors should not list AI and AI-assisted technologies as an author or co-author, nor cite AI as an author”. See https://www.elsevier.com/about/policies-and-standards/the-use-of-generative-ai-and-ai-assisted-technologies-in-writing-for-elsevier . Accessed 23 Nov 2023.
The ethical guidelines of Wiley was updated on 28 February 2023 to clarify the publishing house’s stance on AI-generated content.
See e.g.: Section 2.4 of Princeton University’s Academic Regulations (Princeton University, 2023 ); the Code of Practice and Procedure regarding Misconduct in Research of the University of Oxford (University of Oxford, 2023a ); Section 2.1.1 of the Senate Guidelines on Academic Honesty of York University, enumerating cases of cheating (York University, 2011 ); Imperial College London’s Academic Misconduct Policy and Procedures document (Imperial College London, 2023a ); the Guidelines for seminar and term papers of the University of Vienna (Universität Wien, 2016 ); Para 4. § (1) - (4) of the Anti-plagiarism Regulation of the Corvinus University of Budapest (Corvinus University of Budapest, 2018 ), to name a few.
15 Art. 2 (c)(v) of the early Terms of Use of OpenAI Products (including ChatGPT) dated 14 March 2023 clarified the restrictions of the use of their products. Accordingly, users may not represent the output from their services as human-generated when it was not ( https://openai.com/policies/mar-2023-terms/ . Accessed 14 Nov 2023). Higher education institutions tend to follow suit with this policy. For example, the List of Student Responsibilities enumerated under the “Policies and Regulations” of the Harvard Summer School from 2023 reminds students that their “academic integrity policy forbids students to represent work as their own that they did not write, code, or create” (Harvard University, 2023 ).
A similar view was communicated by Taylor & Francis in a press release issued on 17 February 2023, in which they clarified that: “Authors are accountable for the originality, validity and integrity of the content of their submissions. In choosing to use AI tools, authors are expected to do so responsibly and in accordance with our editorial policies on authorship and principles of publishing ethics” (Taylor and Francis, 2023 ).
This is one of the rare examples where the guideline was adopted by the university’s senior management, in this case, the Academic Affairs Council.
It should be noted that abundant sources recommend harnessing AI tools’ opportunities to improve education instead of attempting to ban them. Heaven, among others, advocated on the pages of the MIT Technology Review the use of advanced chatbots such as ChatGPT as these could be used as “powerful classroom aids that make lessons more interactive, teach students media literacy, generate personalised lesson plans, save teachers time on admin” (Heaven, 2023 ).
This university based its policies on the recommendations of the German Association for University Didactics (Deutsche Gesellschaft für Hochschuldidaktik). Consequently, they draw their students’ attention to the corresponding material, see: (Glathe et al. 2023 ).
For a detailed review of such groups affected by AI see the Artificial Intelligence and Democratic Values Index by the Center for AI and Digital Policy at https://www.caidp.org/reports/aidv-2023/ . Accessed 20 Nov 2023.
See for example: https://www.youtube.com/watch?v=J9W2Pd9GnpQ . Accessed: 14 November 2023.
Akram A (2023) An empirical study of AI generated text detection tools. ArXiv Prepr ArXiv231001423. https://doi.org/10.48550/arXiv.2310.01423
Alberti S (2022) Silas Alberti on X: ChatGPT is trained to not be evil. X Formerly Twitter, 1 December 2022. https://t.co/ZMFdqPs17i . Accessed 23 Nov 2023
Almaraz-López C, Almaraz-Menéndez F, López-Esteban C (2023) Comparative study of the attitudes and perceptions of university students in business administration and management and in education toward Artificial Intelligence. Educ. Sci. 13(6):609. https://doi.org/10.3390/educsci13060609
Article Google Scholar
Bang Y, Cahyawijaya S, Lee N et al. (2023) A multitask, multilingual, multimodal evaluation of ChatGPT on reasoning, hallucination, and interactivity. arXiv. https://doi.org/10.48550/arXiv.2302.04023
Baskara FXR (2023a) ChatGPT as a virtual learning environment: multidisciplinary simulations. In: Proceeding of the 3rd International Conference on Innovations in Social Sciences Education and Engineering, Paper 017. https://conference.loupiasconference.orag/index.php/icoissee3/index
Baskara FXR (2023b) The promises and pitfalls of using ChatGPT for self-determined learning in higher education: An argumentative review. Pros. Semin. Nas. Fakultas Tarb. dan. Ilmu Kegur. IAIM Sinjai 2:95–101. https://doi.org/10.47435/sentikjar.v2i0.1825
Bauer E, Greisel M, Kuznetsov I et al. (2023) Using natural language processing to support peer‐feedback in the age of artificial intelligence: A cross‐disciplinary framework and a research agenda. Br. J. Educ. Technol. 54(5):1222–1245. https://doi.org/10.1111/bjet.13336
Blalock D (2022) Here are all the ways to get around ChatGPT’s safeguards: [1/n]. X Formerly Twitter, 13 December 2022. https://twitter.com/davisblalock/status/1602600453555961856 . Accessed 23 Nov 2023
Chan CKY (2023) A comprehensive AI policy education framework for university teaching and learning. Int J. Educ. Technol. High. Educ. 20(1):1–25. https://doi.org/10.1186/s41239-023-00408-3
Chaudhry IS, Sarwary SAM, El Refae GA, Chabchoub H (2023) Time to revisit existing student’s performance evaluation approach in higher education sector in a new era of ChatGPT—A case study. Cogent Educ. 10(1):2210461. https://doi.org/10.1080/2331186x.2023.2210461
Chaudhry MA, Cukurova M, Luckin R (2022) A transparency index framework for AI in education. In: International Conference on Artificial Intelligence in Education. Springer, Cham, Switzerland, pp 195–198. https://doi.org/10.35542/osf.io/bstcf
Chinese University of Hong Kong (2023) Use of Artificial Intelligence tools in teaching, learning and assessments - A guide for students. https://www.aqs.cuhk.edu.hk/documents/A-guide-for-students_use-of-AI-tools.pdf . Accessed 23 Nov 2023
Colorado State University (2023) What should a syllabus statement on AI look like? https://tilt.colostate.edu/what-should-a-syllabus-statement-on-ai-look-like/ . Accessed 23 Nov 2023
Columbia University (2023) Considerations for AI tools in the classroom. https://ctl.columbia.edu/resources-and-technology/resources/ai-tools/ . Accessed 23 Nov 2023
Corrêa NK, Galvão C, Santos JW et al. (2023) Worldwide AI ethics: A review of 200 guidelines and recommendations for AI governance. Patterns 4(10):100857. https://doi.org/10.1016/j.patter.2023.100857
Article PubMed PubMed Central Google Scholar
Corvinus University of Budapest (2018) Anti-Plagiarism rules. https://www.uni-corvinus.hu/contents/uploads/2020/11/I.20_Plagiumszabalyzat_2018_junius_19_EN.6b1.pdf . Accessed 23 Nov 2023
Cotton DR, Cotton PA, Shipway JR (2024) Chatting and cheating: Ensuring academic integrity in the era of ChatGPT. Innov. Educ. Teach. Int 61(2):228–239. https://doi.org/10.1080/14703297.2023.2190148
Crompton H, Burke D (2023) Artificial intelligence in higher education: the state of the field. Int J. Educ. Technol. High. Educ. 20(1):1–22. https://doi.org/10.1186/s41239-023-00392-8
Dhaor A (2023) India will regulate AI, ensure data privacy, says Rajeev Chandrasekhar. Hindustan Times, 12 October 2023. https://www.hindustantimes.com/cities/noida-news/india-will-regulate-ai-ensure-data-privacy-says-rajeev-chandrasekhar-101697131022456.html . Accessed 23 Nov 2023
Ellul J (1964) The technological society. Vintage Books
EUHLEX (2019) Ethics guidelines for trustworthy AI | Shaping Europe’s digital future. https://digital-strategy.ec.europa.eu/en/library/ethics-guidelines-trustworthy-ai . Accessed 23 Nov 2023
European Commission (2021) Proposal for a Regulation laying down harmonised rules on artificial intelligence | Shaping Europe’s digital future. https://digital-strategy.ec.europa.eu/en/library/proposal-regulation-laying-down-harmonised-rules-artificial-intelligence . Accessed 23 Nov 2023
European Parliament - Committee on Culture and Education (2021) Report on artificial intelligence in education, culture and the audiovisual sector | A9-0127/2021. https://www.europarl.europa.eu/doceo/document/A-9-2021-0127_EN.html . Accessed 23 Nov 2023
Farazouli A, Cerratto-Pargman T, Bolander-Laksov K, McGrath C (2024) Hello GPT! Goodbye home examination? An exploratory study of AI chatbots impact on university teachers’ assessment practices. Assess. Eval. High. Educ. 49(3):363–375. https://doi.org/10.1080/02602938.2023.2241676
Farrokhnia M, Banihashem SK, Noroozi O, Wals A (2023) A SWOT analysis of ChatGPT: Implications for educational practice and research. Innov. Educ. Teach. Int 61(3):460–474. https://doi.org/10.1080/14703297.2023.2195846
Ferguson R, Hoel T, Scheffel M, Drachsler H (2016) Guest editorial: Ethics and privacy in learning analytics. J. Learn Anal. 3(1):5–15. https://doi.org/10.18608/jla.2016.31.2
Future of Life Institute (2023) Pause giant AI experiments: An open letter. https://futureoflife.org/open-letter/pause-giant-ai-experiments/ . Accessed 15 Nov 2023
Gamage KA, Dehideniya SC, Xu Z, Tang X (2023) ChatGPT and higher education assessments: more opportunities than concerns? J Appl Learn Teach 6(2). https://doi.org/10.37074/jalt.2023.6.2.32
Gimpel H, Hall K, Decker S, et al. (2023) Unlocking the power of generative AI models and systems such as GPT-4 and ChatGPT for higher education: A guide for students and lecturers. Hohenheim Discussion Papers in Business, Economics and Social Sciences 2023, 02:2146. http://opus.uni-hohenheim.de/frontdoor.php?source_opus=2146&la=en
Glathe A, Mörth M, Riedel A (2023) Vorschläge für Eigenständigkeitserklärungen bei möglicher Nutzung von KI-Tools. European University Viadrina. https://opus4.kobv.de/opus4-euv/files/1326/Forschendes-Lernen-mit-KI_SKILL.pdf . Accessed 23 Nov 2023
Harvard University (2023) Student Responsibilities. Harvard Summer School 2023. https://summer.harvard.edu/academic-opportunities-support/policies-and-regulations/student-responsibilities/ . Accessed 23 Nov 2023
Heaven WD (2023) ChatGPT is going to change education, not destroy it. MIT Technology Review. https://www.technologyreview.com/2023/04/06/1071059/chatgpt-change-not-destroy-education-openai/ . Accessed 14 Nov 2023
Hillier M (2023) Turnitin Artificial Intelligence writing detection. https://teche.mq.edu.au/2023/03/turnitin-artificial-intelligence-writing-detection/ . Accessed 23 Nov 2023
Howard SK, Mozejko A (2015) Considering the history of digital technologies in education. In: Henderson M, Romeo G (eds) Teaching and digital technologies: Big issues and critical questions. Cambridge University Press, Port Melbourne, Australia, pp 157–168. https://doi.org/10.1017/cbo9781316091968.017
Humboldt-Universität zu Berlin (2023a) ChatGPT & Co: Empfehlungen für das Umgehen mit Künstlicher Intelligenz in Prüfungen. https://www.hu-berlin.de/de/pr/nachrichten/september-2023/nr-2397-1 . Accessed 23 Nov 2023
Humboldt-Universität zu Berlin (2023b) Empfehlungen zur Nutzung von Künstlicher Intelligenz in Studienleistungen und Prüfungen an der Humboldt-Universität zu Berlin. https://www.hu-berlin.de/de/pr/nachrichten/september-2023/hu_empfehlungen_ki-in-pruefungen_20230905.pdf . Accessed 23 Nov 2023
Ilieva G, Yankova T, Klisarova-Belcheva S et al. (2023) Effects of generative chatbots in higher education. Information 14(9):492. https://doi.org/10.3390/info14090492
Imperial College London (2023a) Academic misconduct policy and procedure. https://www.imperial.ac.uk/media/imperial-college/administration-and-support-services/registry/academic-governance/public/academic-policy/academic-integrity/Academic-Misconduct-Policy-and-Procedure-v1.3-15.03.23.pdf . Accessed 14 Nov 2023
Imperial College London (2023b) College guidance on the use of generative AI tools. https://www.imperial.ac.uk/about/leadership-and-strategy/provost/vice-provost-education/generative-ai-tools-guidance/ . Accessed 23 Nov 2023
Ivanov S (2023) The dark side of artificial intelligence in higher education. Serv. Ind. J. 43(15–16):1055–1082. https://doi.org/10.1080/02642069.2023.2258799
Johns Hopkins University (2023) CSCI 601.771: Self-supervised Models. https://self-supervised.cs.jhu.edu/sp2023/ . Accessed 23 Nov 2023
Khosravi H, Shum SB, Chen G et al. (2022) Explainable artificial intelligence in education. Comput Educ. Artif. Intell. 3:100074. https://doi.org/10.1016/j.caeai.2022.100074
Liao SM (2020) The moral status and rights of Artificial Intelligence. In: Liao SM (ed) Ethics of Artificial Intelligence. Oxford University Press, pp 480–503. https://doi.org/10.1093/oso/9780190905033.003.0018
Lim T, Gottipati S, Cheong M (2023) Artificial Intelligence in today’s education landscape: Understanding and managing ethical issues for educational assessment. Research Square Preprint. https://doi.org/10.21203/rs.3.rs-2696273/v1
Limo FAF, Tiza DRH, Roque MM et al. (2023) Personalized tutoring: ChatGPT as a virtual tutor for personalized learning experiences. Soc. Space 23(1):293–312. https://socialspacejournal.eu/article-page/?id=176
Google Scholar
Liu S (2023) India’s AI Regulation Dilemma. The Diplomat, 27 October 2023. https://thediplomat.com/2023/10/indias-ai-regulation-dilemma/ . Accessed 23 Nov 2023
Macquarie University (2023) Academic integrity vs the other AI (Generative Artificial Intelligence). https://teche.mq.edu.au/2023/03/academic-integrity-vs-the-other-ai-generative-artificial-intelligence/ . Accessed 14 Nov 2023
Memarian B, Doleck T (2023) Fairness, Accountability, Transparency, and Ethics (FATE) in Artificial Intelligence (AI), and higher education: A systematic review. Comput Educ Artif Intell 100152. https://doi.org/10.1016/j.caeai.2023.100152
Mhlanga D (2023) Open AI in Education, the Responsible and Ethical Use of ChatGPT Towards Lifelong Learning. SSRN Electron J 4354422. https://doi.org/10.2139/ssrn.4354422
Miller GE (2023) eLearning and the Transformation of Higher Education. In: Miller GE, Ives K (eds) Leading the eLearning Transformation of Higher Education. Routledge, pp 3–23. https://doi.org/10.4324/9781003445623-3
Mollick ER, Mollick L (2022) New modes of learning enabled by AI chatbots: Three methods and assignments. SSRN Electron J 4300783. https://doi.org/10.2139/ssrn.4300783
Monash University (2023a) Generative AI and assessment: Designing assessment for achievement and demonstration of learning outcomes. https://www.monash.edu/learning-teaching/teachhq/Teaching-practices/artificial-intelligence/generative-ai-and-assessment . Accessed 23 Nov 2023
Monash University (2023b) Policy and practice guidance around acceptable and responsible use of AI technologies. https://www.monash.edu/learning-teaching/teachhq/Teaching-practices/artificial-intelligence/policy-and-practice-guidance-around-acceptable-and-responsible-use-of-ai-technologies . Accessed 23 Nov 2023
Monash University (2023c) Choosing assessment tasks. https://www.monash.edu/learning-teaching/teachhq/Assessment/choosing-assessment-tasks . Accessed 23 Nov 2023
National Autonomous University of Mexico (2023) Recomendaciones para el uso de Inteligencia Artificial Generativa en la docencia. https://cuaed.unam.mx/descargas/recomendaciones-uso-iagen-docencia-unam-2023.pdf . Accessed 14 Oct 2023
Nature (2023) Tools such as ChatGPT threaten transparent science; here are our ground rules for their use. Nature 613:612. https://doi.org/10.1038/d41586-023-00191-1 . Editorial
Article CAS Google Scholar
Nicol DJ, Macfarlane‐Dick D (2006) Formative assessment and self‐regulated learning: A model and seven principles of good feedback practice. Stud. High. Educ. 31(2):199–218. https://doi.org/10.1080/03075070600572090
Nikolinakos NT (2023) Ethical Principles for Trustworthy AI. In: Nikolinakos NT (ed) EU Policy and Legal Framework for Artificial Intelligence, Robotics and Related Technologies -The AI Act. Springer International Publishing, Cham, Switzerland, pp 101–166. https://doi.org/10.1007/978-3-031-27953-9
O’Connor S (2023) Corrigendum to “Open artificial intelligence platforms in nursing education: Tools for academic progress or abuse?” [Nurse Educ. Pract. 66 (2023) 103537]. Nurse Educ. Pr. 67:103572. https://doi.org/10.1016/j.nepr.2023.103572
OECD (2019) Recommendation of the Council on Artificial Intelligence. https://legalinstruments.oecd.org/en/instruments/OECD-LEGAL-0449#mainText . Accessed 23 Nov 2023
OpenAI (2022) Introducing ChatGPT. https://openai.com/blog/chatgpt . Accessed 14 Nov 2022
Paek S, Kim N (2021) Analysis of worldwide research trends on the impact of artificial intelligence in education. Sustainability 13(14):7941. https://doi.org/10.3390/su13147941
Perkins M (2023) Academic Integrity considerations of AI Large Language Models in the post-pandemic era: ChatGPT and beyond. J. Univ. Teach. Learn Pr. 20(2):07. https://doi.org/10.53761/1.20.02.07
Princeton University (2023) Academic Regulations: Rights, rules, responsibilities. https://rrr.princeton.edu/2023/students-and-university/24-academic-regulations . Accessed 23 Nov 2023
Qadir J (2023) Engineering education in the era of ChatGPT: Promise and pitfalls of generative AI for education. In: 2023 IEEE Global Engineering Education Conference (EDUCON). IEEE, pp 1–9. https://doi.org/10.1109/educon54358.2023.10125121
Rasul T, Nair S, Kalendra D et al. (2023) The role of ChatGPT in higher education: Benefits, challenges, and future research directions. J. Appl Learn Teach. 6(1):41–56. https://doi.org/10.37074/jalt.2023.6.1.29
Ray PP (2023) ChatGPT: A comprehensive review on background, applications, key challenges, bias, ethics, limitations and future scope. Internet Things Cyber-Phys. Syst. 3:121–154. https://doi.org/10.1016/j.iotcps.2023.04.003
Reiser RA (2001) A history of instructional design and technology: Part I: A history of instructional media. Educ. Technol. Res Dev. 49(1):53–64. https://doi.org/10.1007/BF02504506
Roose K (2023) GPT-4 is exciting and scary. New York Times, 15 March 2023. https://www.nytimes.com/2023/03/15/technology/gpt-4-artificial-intelligence-openai.html . Accessed 23 Nov 2023
Rudolph J, Tan S, Tan S (2023) War of the chatbots: Bard, Bing Chat, ChatGPT, Ernie and beyond. The new AI gold rush and its impact on higher education. J. Appl Learn Teach. 6(1):364–389. https://doi.org/10.37074/jalt.2023.6.1.23
Solis T (2023) Die ChatGPT-Richtlinien der 100 größten deutschen Universitäten. Scribbr, 6 May 2023. https://www.scribbr.de/ki-tools-nutzen/chatgpt-universitaere-richtlinien/ . Accessed 23 Nov 2023
Stokel-Walker C (2023) ChatGPT listed as author on research papers: Many scientists disapprove. Nature 613:620–621. https://doi.org/10.1038/d41586-023-00107-z
Article ADS CAS PubMed Google Scholar
Sweeney S (2023) Who wrote this? Essay mills and assessment – Considerations regarding contract cheating and AI in higher education. Int J. Manag Educ. 21(2):100818. https://doi.org/10.1016/j.ijme.2023.100818
Taylor and Francis (2023) Taylor & Francis clarifies the responsible use of AI tools in academic content creation. Taylor Francis Newsroom, 17 February 2023. https://newsroom.taylorandfrancisgroup.com/taylor-francis-clarifies-the-responsible-use-of-ai-tools-in-academic-content-creation/ . Accessed 23 Nov 2023
Technische Universität Berlin (2023) Inklusive Digitalisierung Modul. https://moseskonto.tu-berlin.de/moses/modultransfersystem/bolognamodule/beschreibung/anzeigen.html?nummer=51021&version=2&sprache=1 . Accessed 05 Aug 2024
Tokyo Institute of Technology (2023) Policy on Use of Generative Artificial Intelligence in Learning. https://www.titech.ac.jp/english/student/students/news/2023/066592.html . Accessed 23 Nov 2023
Turnitin (2023) Turnitin announces AI writing detector and AI writing resource center for educators. https://www.turnitin.com/press/turnitin-announces-ai-writing-detector-and-ai-writing-resource-center-for-educators . Accessed 14 Nov 2023
Uchiyama S, Umemura K, Morita Y (2023) Large Language Model-based system to provide immediate feedback to students in flipped classroom preparation learning. ArXiv Prepr ArXiv230711388. https://doi.org/10.48550/arXiv.2307.11388
UNESCO (2022) Recommendation on the ethics of Artificial Intelligence. https://unesdoc.unesco.org/ark:/48223/pf0000381137 . Accessed 23 Nov 2023
UNESCO International Institute for Higher Education in Latin America and the Caribbean (2023) ChatGPT and Artificial Intelligence in higher education. https://www.iesalc.unesco.org/wp-content/uploads/2023/04/ChatGPT-and-Artificial-Intelligence-in-higher-education-Quick-Start-guide_EN_FINAL.pdf . Accessed 14 Nov 2023
Universität Wien (2016) Guidelines for seminar and term papers. https://bda.univie.ac.at/fileadmin/user_upload/p_bda/Teaching/PaperGuidlines.pdf . Accessed 23 Nov 2023
University of Auckland (2023) Advice for students on using Generative Artificial Intelligence in coursework. https://www.auckland.ac.nz/en/students/forms-policies-and-guidelines/student-policies-and-guidelines/academic-integrity-copyright/advice-for-student-on-using-generative-ai.html . Accessed 24 Nov 2023
University of Boston (2023) Using Generative AI in coursework. https://www.bu.edu/cds-faculty/culture-community/gaia-policy/ . Accessed 23 Nov 2023
University of Cambridge (2023) Artificial Intelligence and teaching, learning and assessment. https://www.cambridgeinternational.org/support-and-training-for-schools/artificial-intelligence/ . Accessed 23 Nov 2023
University of Cape Town (2023) Staff Guide - Assessment and academic integrity in the age of AI. https://docs.google.com/document/u/0/d/1o5ZIOBjPsP6Nh2VIlM56_kcuqB-Y7xTf/edit?pli=1&usp=embed_facebook . Accessed 14 Nov 2023
University of Delaware (2023) Considerations for using and addressing advanced automated tools in coursework and assignments. https://ctal.udel.edu/advanced-automated-tools/ . Accessed 14 Nov 2023
University of Helsinki (2023) Using AI to support learning | Instructions for students. https://studies.helsinki.fi/instructions/article/using-ai-support-learning . Accessed 24 Nov 2023
University of Oxford (2023a) Code of practice and procedure on academic integrity in research. https://hr.admin.ox.ac.uk/academic-integrity-in-research . Accessed 23 Nov 2023
University of Oxford (2023b) Unauthorised use of AI in exams and assessment. https://academic.admin.ox.ac.uk/article/unauthorised-use-of-ai-in-exams-and-assessment . Accessed 23 Nov 2023
University of Pittsburgh (2023) Generative AI Resources for Faculty. https://teaching.pitt.edu/generative-ai-resources-for-faculty/ . Accessed 23 Nov 2023
University of Toronto (2019) Code of behaviour on academic matters. https://governingcouncil.utoronto.ca/secretariat/policies/code-behaviour-academic-matters-july-1-2019 . Accessed 23 Nov 2023
University of Toronto (2023) ChatGPT and Generative AI in the classroom. https://www.viceprovostundergrad.utoronto.ca/strategic-priorities/digital-learning/special-initiative-artificial-intelligence/ . Accessed 20 Nov 2023
University of Waterloo (2023) Artificial Intelligence at UW. https://uwaterloo.ca/associate-vice-president-academic/artificial-intelligence-uw . Accessed 23 Nov 2023
University of Zürich (2023) ChatGPT. https://ethz.ch/en/the-eth-zurich/education/educational-development/ai-in-education/chatgpt.html . Accessed 23 Nov 2023
Wach K, Duong CD, Ejdys J et al. (2023) The dark side of generative artificial intelligence: A critical analysis of controversies and risks of ChatGPT. Entrep. Bus. Econ. Rev. 11(2):7–24. https://doi.org/10.15678/eber.2023.110201
Wagner G (2018) Robot liability. SSRN Electron J 3198764. https://doi.org/10.2139/ssrn.3198764
Wiley (2023) Best practice guidelines on research integrity and publishing ethics. https://authorservices.wiley.com/ethics-guidelines/index.html . Accessed 20 Nov 2023
Yan L, Sha L, Zhao L et al. (2023) Practical and ethical challenges of large language models in education: A systematic scoping review. Br. J. Educ. Technol. 55(1):90–112. https://doi.org/10.1111/bjet.13370
York University (2011) Senate Policy on Academic Honesty. https://www.yorku.ca/secretariat/policies/policies/academic-honesty-senate-policy-on/ . Accessed 23 Nov 2023
York University Senate (2023) Academic Integrity and Generative Artificial Intelligence Technology. https://www.yorku.ca/unit/vpacad/academic-integrity/wp-content/uploads/sites/576/2023/03/Senate-ASCStatement_Academic-Integrity-and-AI-Technology.pdf . Accessed 23 Nov 2023
Yu H (2023) Reflection on whether Chat GPT should be banned by academia from the perspective of education and teaching. Front Psychol. 14:1181712. https://doi.org/10.3389/fpsyg.2023.1181712
Download references
The authors have received no funding, grants, or other support for the research reported here. Open access funding provided by Corvinus University of Budapest.
Author information
Authors and affiliations.
Covinus University of Budapest, Budapest, Hungary
Attila Dabis & Csaba Csáki
You can also search for this author in PubMed Google Scholar
Contributions
AD had established the initial idea and contributed to the collection of ethical standards as well as to the collection of university policy documents. Also contributed to writing the initial draft and the final version. CsCs had reviewed and clarified the initial concept and then developed the first structure including methodological considerations. Also contributed to the collection of university policy documents as well as to writing the second draft and the final version.
Corresponding author
Correspondence to Attila Dabis .
Ethics declarations
Competing interests.
The authors declare no competing interests.
Ethical approval
This research did not involve any human participants or animals and required no ethical approval.
Informed consent
This article does not contain any studies with human participants performed by any of the authors. No consent was required as no private data was collected or utilized.
Additional information
Publisher’s note Springer Nature remains neutral with regard to jurisdictional claims in published maps and institutional affiliations.
Rights and permissions
Open Access This article is licensed under a Creative Commons Attribution 4.0 International License, which permits use, sharing, adaptation, distribution and reproduction in any medium or format, as long as you give appropriate credit to the original author(s) and the source, provide a link to the Creative Commons licence, and indicate if changes were made. The images or other third party material in this article are included in the article’s Creative Commons licence, unless indicated otherwise in a credit line to the material. If material is not included in the article’s Creative Commons licence and your intended use is not permitted by statutory regulation or exceeds the permitted use, you will need to obtain permission directly from the copyright holder. To view a copy of this licence, visit http://creativecommons.org/licenses/by/4.0/ .
Reprints and permissions
About this article
Cite this article.
Dabis, A., Csáki, C. AI and ethics: Investigating the first policy responses of higher education institutions to the challenge of generative AI. Humanit Soc Sci Commun 11 , 1006 (2024). https://doi.org/10.1057/s41599-024-03526-z
Download citation
Received : 21 February 2024
Accepted : 29 July 2024
Published : 06 August 2024
DOI : https://doi.org/10.1057/s41599-024-03526-z
Share this article
Anyone you share the following link with will be able to read this content:
Sorry, a shareable link is not currently available for this article.
Provided by the Springer Nature SharedIt content-sharing initiative
Quick links
- Explore articles by subject
- Guide to authors
- Editorial policies


IMAGES
COMMENTS
Common types of limitations and their ramifications include: Theoretical: limits the scope, depth, or applicability of a study. Methodological: limits the quality, quantity, or diversity of the data. Empirical: limits the representativeness, validity, or reliability of the data. Analytical: limits the accuracy, completeness, or significance of ...
In research, studies can have limitations such as limited scope, researcher subjectivity, and lack of available research tools. Acknowledging the limitations of your study should be seen as a strength. It demonstrates your willingness for transparency, humility, and submission to the scientific method and can bolster the integrity of the study.
Limitation #3: Sample Size & Composition. As we've discussed before, the size and representativeness of your sample are crucial, especially in quantitative research where the robustness of your conclusions often depends on these factors.All too often though, students run into issues achieving a sufficient sample size and composition. To ensure adequacy in terms of your sample size, it's ...
Identify the limitations: Start by identifying the potential limitations of your research. These may include sample size, selection bias, measurement error, or other issues that could affect the validity and reliability of your findings. Be honest and objective: When describing the limitations of your research, be honest and objective.
Step 1. Identify the limitation (s) of the study. This part should comprise around 10%-20% of your discussion of study limitations. The first step is to identify the particular limitation (s) that affected your study. There are many possible limitations of research that can affect your study, but you don't need to write a long review of all ...
Sample Size Limitations in Qualitative Research. Sample sizes are typically smaller in qualitative research because, as the study goes on, acquiring more data does not necessarily lead to more information. This is because one occurrence of a piece of data, or a code, is all that is necessary to ensure that it becomes part of the analysis framework.
sentence tha. signals what you're about to discu. s. For example:"Our study had some limitations."Then, provide a concise sentence or two identifying each limitation and explaining how the limitation may have affected the quality. of the study. s findings and/or their applicability. For example:"First, owing to the rarity of the ...
Methodology limitations. Not having access to data or reliable information can impact the methods used to facilitate your research. A lack of data or reliability may limit the parameters of your study area and the extent of your exploration. Your sample size may also be affected because you won't have any direction on how big or small it ...
3. Identify your limitations of research and explain their importance. 4. Provide the necessary depth, explain their nature, and justify your study choices. 5. Write how you are suggesting that it is possible to overcome them in the future. Limitations can help structure the research study better.
Limitations of a dissertation are potential weaknesses in your study that are mostly out of your control, given limited funding, choice of research design, statistical model constraints, or other factors. In addition, a limitation is a restriction on your study that cannot be reasonably dismissed and can affect your design and results.
The ideal way is to divide your limitations section into three steps: 1. Identify the research constraints; 2. Describe in great detail how they affect your research; 3. Mention the opportunity for future investigations and give possibilities. By following this method while addressing the constraints of your research, you will be able to ...
While each study will have its own unique set of limitations, some limitations are more common in quantitative research, and others are more common in qualitative research. In quantitative research, common limitations include the following: - Participant dropout. - Small sample size, low power. - Non-representative sample.
re impressive to reviewers than ignoring them.A fourfold approach can be used when presenting limitations as outlined in the Figure 13.1: (1) describe the potential limitation, (2) describe the potential impact of the limitation on your study findings, (3) discuss alternatives and why they were not selected, and (4) describe the methods that ...
Research Limitations. Research limitations are, at the simplest level, the weaknesses of the study, based on factors that are often outside of your control as the researcher. These factors could include things like time, access to funding, equipment, data or participants.For example, if you weren't able to access a random sample of participants for your study and had to adopt a convenience ...
We have selected a variety of examples from different research topics. 2.1. Limitations Example 1. Following example is from a Medical research paper. The authors talk about the limitations and emphasis the importance of reconfirming the findings in a much larger study. Study design and small sample size are important limitations.
Learn about the 5 most common research limitations, how to avoid them and how to mitigate the impacts of each limitation. Seasoned Grad Coachs, David Phair a...
Writing the limitations of the research papers is often assumed to require lots of effort. However, identifying the limitations of the study can help structure the research better. Therefore, do not underestimate the importance of research study limitations. 3. Opportunity to make suggestions for further research.
Once you have a first draft ready, consider submitting a free sample to us for proofreading to ensure that your writing is concise and error-free and your results—despite their limitations— shine through. Whether you're a veteran researcher or a novice to the field, every study has its limitations. Here's how to identify them.
Possible Methodological Limitations. Sample size-- the number of the units of analysis you use in your study is dictated by the type of research problem you are investigating. Note that, if your sample size is too small, it will be difficult to find significant relationships from the data, as statistical tests normally require a larger sample ...
Learn about research limitations and research delimitations - what they are, how they're similar and how they're different. Emma unpacks each concept using p...
Research limitations in a typical dissertation may relate to the following points: 1. Formulation of research aims and objectives. You might have formulated research aims and objectives too broadly. You can specify in which ways the formulation of research aims and objectives could be narrowed so that the level of focus of the study could be ...
A Note about Sample Size Limitations in Qualitative Research. Sample sizes are typically smaller in qualitative research because, as the study goes on, acquiring more data does not necessarily lead to more information. This is because one occurrence of a piece of data, or a code, is all that is necessary to ensure that it becomes part of the ...
Delimitations refer to the specific boundaries or limitations that are set in a research study in order to narrow its scope and focus. Delimitations may be related to a variety of factors, including the population being studied, the geographical location, the time period, the research design, and the methods or tools being used to collect data.
While there are limitations in certain regions, the use of global land cover collections and filtering methods as training samples can be valuable in areas where high-resolution data are lacking. Future research should focus on validating and adapting these approaches to ensure their effectiveness in sustainable agriculture and ecosystem ...
Risk stratification in multiple myeloma (MM) patients is crucial, and molecular genetic studies play a significant role in achieving this objective. Enrichment of plasma cells for next-generation sequencing (NGS) analysis has been employed to enhance detection sensitivity. However, these methods often come with limitations, such as high costs and low throughput.
Research indicates that the target population is skewed towards a younger demographic (GlobalWebIndex, 2017). Reflective of this, 58.2% of the sample is in the 18 to 34 age bracket. The final sample was representative of the U.S. population that uses ride-share services in terms of age and gender (GlobalWebIndex, 2017).
The COVID-19 pandemic resulted in substantial changes to family life. This study examined associations between pandemic conditions and mothers' and fathers' food, physical activity, and media parenting practices and whether these associations were moderated by parenting styles and family functioning. Two independent samples of Canadian parents (nonpandemic n = 270; pandemic n = 357) self ...
Conclusions, limitations and future research Ethical dilemmas discussed in this paper were based on the conceptualisation embedded in relevant documents of various international fora.